Early Diagnosis of Iron Deficiency in Commercial Tomato Crop Using Electrical Signals
- Institute for Plant Production Systems, Agroscope, Conthey, Switzerland
Adequate plant nutrition is essential for commercial crop production. There are 18 nutrients that are essential for proper crop development. Each is equally important to the plant, although they are required in vastly different amounts. The absence of any one of these nutrients has the potential to decrease crop yields and quality by negatively affecting associated growth factors. Hence, early diagnosis of nutrient imbalances or deficiencies is of crucial importance for farmers. In this work, we provide compelling evidence that electrical potential variation in a commercial tomato crop contains information, which can be modeled to detect iron (Fe) deficiency before visual symptoms appear. The proposed supervised machine learning model showed accurate prediction on test data of above 75%. A model built to classify normal conditions (full nutrients) vs. strong Fe deficiency conditions (visible symptoms), enables early detection of slight Fe deprivation i.e., 6 days prior to the appearance of the earliest visual symptoms. Continuous real-time monitoring of crop electrical signals and deployment of predictive algorithms could constitute a great practical tool to help and assist farmers in iron deficiency detection.
Introduction
Abiotic and biotic stresses provoke unfavorable environmental fluctuations, which require plants to mount defenses reprogram and adjust metabolism, growth, and development to adapt and survive. In particular, crop species are continuously challenged by inadequate supply of nutrients. Plants require various ions as essential nutrients, which are taken up from the soil and distributed throughout the whole plant (White and Brown, 2010; Maathuis and Diatloff, 2013). Each of these nutrients, once they are transferred to their destination within plant tissues via corresponding transporters/ion channels, plays diverse and critical roles in maintaining plant growth and development (Hänsch and Mendel, 2009). For agricultural crops, iron (Fe) deficiency is a major nutritional disorder that reduces vegetative growth and causes marked yield and quality losses (Abadía et al., 2011). Even though Fe is the fourth most abundant element in the Earth's crust (Morgan and Anders, 1980), its chemical properties limit its availability for plant uptake due to soil conditions that result in poor solubility (Abadía et al., 2011; Zhang et al., 2019). Fe is involved in a variety of metabolic processes, including mitochondrial respiration, fatty acid and protein synthesis, as well as plant photosynthesis (Kobayashi et al., 2019). It is of crucial importance for CO2 fixation and therefore for biomass production (Zhang et al., 2019). With an essential role for chlorophyll development and function, Fe-deficient plants exhibit reduced photosynthetic activity and tend to have yellow coloration and interveinal chlorosis, which affects young leaves. These visual symptoms appear several days or even weeks after Fe deprivation commences, depending on crop species (Chakraborty et al., 2015).
A well-developed and healthy crop is of paramount importance to farmers. Plant fitness has a profound impact on crop production, yield and quality. A variety of factors influence crop production, a correct nutrient supply can be highlighted among other. While most of the crop are subjected to Fe deficiency, when chlorosis occurs, it can cause up to 30% of yield losses (Khan and Nguyen, 2020). Optimal crop management relies on regular monitoring to scout and detect problems before major crop stress occurs. We have previously shown that an electrophysiological sensor allows continuous and stable long–term monitoring of plant electrical signals for several weeks without affecting plant functions, which can be performed in a commercial greenhouse for crop production without a Faraday cage (Tran et al., 2019). We hypothesized that monitoring slow and long-term changes in electrical potential (EP) with the help of machine learning algorithms can be used as an agronomic tool to detect physiological plant state modifications. It follows that this approach could potentially support real-time crop supervision.
Tomato (Solanum lycopersicum) ranks third by weight in global production of fruits and vegetables in the world (FAOSTAT, 2019). It is cultivated in all five continent and is of great economic importance. It has therefore been used as model in our study. Here, we present results of machine learning modeling using electrical signals to diagnose Fe deficiency before visual symptoms appear in hydroponic tomato crops in soilless culture grown in commercial greenhouse.
Materials and Methods
Plant Material
The experiment was conducted during the growing season 2019 in greenhouse at Agroscope research station (Conthey, Switzerland). The compartment measured 370 m2 floor area and was equipped with technology comparable to commercial greenhouses.
Tomato plants (Solanum lycopersicum), variety Admiro (De Ruiter), grafted on Beaufort (De Ruiter) were used in the present study. Plants were grown in rockwool cubes, transplanted at four-leaf stage end of January 2019 on organic slabs composed of compost of bark (35%), a peat substitute (30%), Coco peat (20%) and topsoil 15% (Substrate 127, Ricoter, CH), located on hanging and elevated gutters. Plants were cultivated with two-truss with a planting density of 3.8 truss per m2.
Fifteen tomato plants were used from April to May 2019 (4–5 month old, four meters high). Irrigation water and nutrients were supplied with drippers operated by a valve. Iron was specifically removed from nutrients premixed at the beginning of deprivation experiment.
Electrophysiology
Electrical signals produced by plants have been recorded using PhytlSigns devices from Vivent SA (Crans-près-Celigny, Switzerland) as previously described (Tran et al., 2019). For each plant, we obtained a 3 weeks' time-series of data representing 315 daily cycles; the PhytlSigns device recorded the difference in electric potential between the main stem and a leaf petiole of the plant. The signal is sampled at 500 Hz with a notch filters at 50 and 100 Hz are applied. To reduce the sample rate of the dataset, raw electrical signal data are decimated at 2, 10, and 500 to obtain 250, 50, and 1 Hz, respectively. The signal has been recorded in mV as a function of time. Digitized signal data were extracted and customized using Matlab software (Matlab, R2020a).
Data Pre-processing and Classification
For the modeling of the classifiers, several pre-processing steps were applied as previously described (Tran et al., 2019) with reduced time windows size namely 30 s, 1, 5, and 15 min. We developed gradient boosted tree (GBT) supervised machine learning algorithms to model a plant state (healthy or stressed) classifier and evaluate its performance. The training dataset was split randomly into a learning set (80% of data) and a validation set (20%). Among the 15 plants, 12 plants were used to train the classifier with 3 days in full nutrient conditions (comfort) and 3 days in iron deprivation condition with visible chlorosis (stressed) leading to 1,728 hours of recorded data. The test dataset were used to evaluate the model performance. It is composed of three other independent plants.
The 338,688 normalized and labeled samples compose the training dataset used for the supervised classification. The test dataset is composed of 84,672 samples. The classes' balance of both datasets is half iron-stressed and half normal comfort conditions.
Results and Discussion
Puzzled With Nutrient Deficiency: Case of Iron
Upon Fe deprivation, agricultural crops develop typical symptoms such as interveinal chlorosis on leaves. These symptoms are usually visible several days after removal of the nutrient. In growing condition similar to those used by commercial growers, hydroponic tomato crops in soilless culture grown under Fe deprivation exhibit these visible symptoms first at the top of the stem after 8 days (D +8) with pale green or slight yellowing leaves. At this stage, this can be due to sulfur, zinc, manganese or iron deficiencies. After 10 days (D +10), interveinal chlorosis started to appear at the top of the plant removing the sulfur possibility. The chlorosis become more pronounced after 14 days (D +12) with shortened stems refining the diagnosis to iron or manganese (Figure 1A). From these observations, one can note that specific nutrient deficiencies are hard to diagnose at the earliest stage since deprivation of different nutrients causes common symptoms (Graham, 2008; Jones, 2020). Moreover, interactions between nutrients can influence uptake. Too much of one nutrient can restrict the crop's ability to take in another. For instance, excess nitrogen, phosphorus or manganese can lead to Fe deficiency (Panda et al., 2012). Development of an early diagnosis tool could help growers to rapidly correct the imbalance.
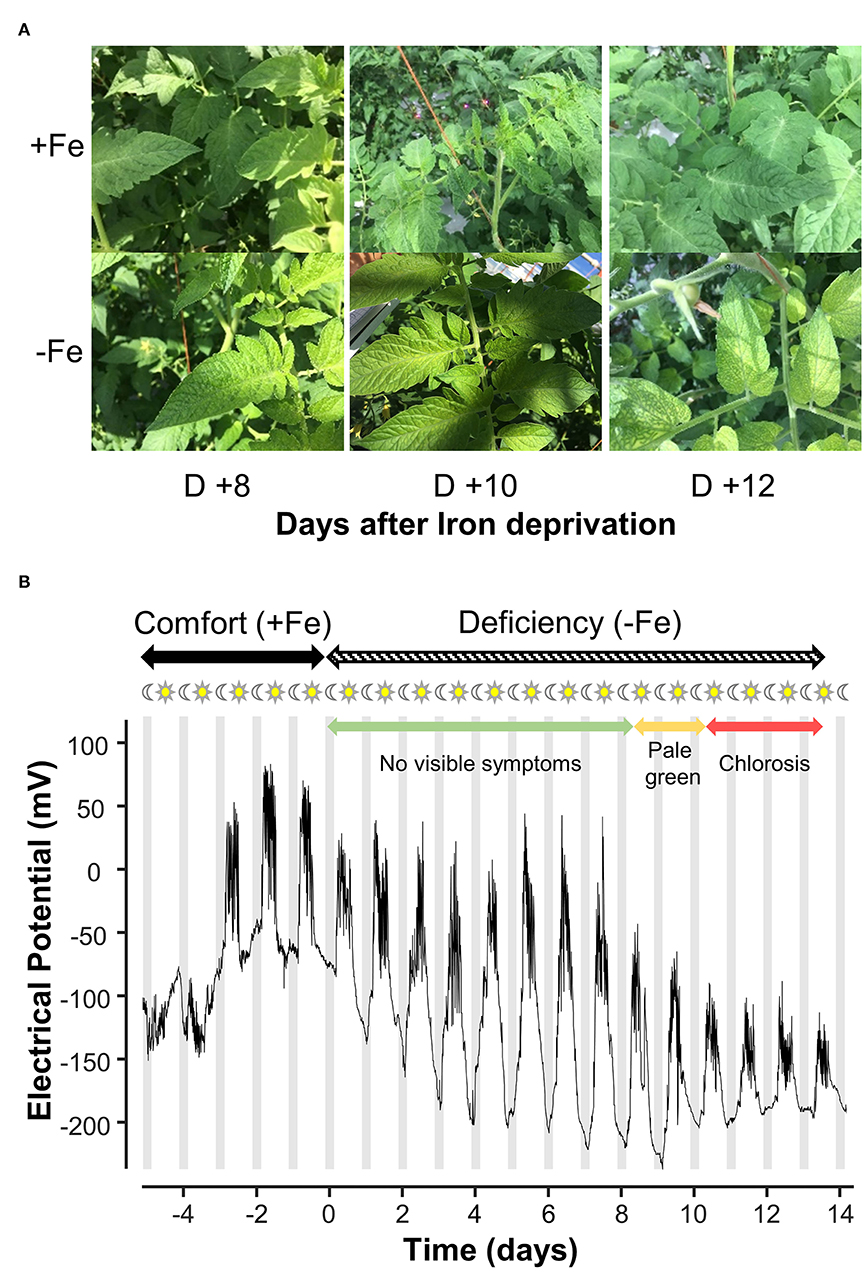
Figure 1. (A) Hydroponic tomato plants in soilless culture grown in commercial greenhouse were submitted to different fertigation regimens: optimal nutrient condition (+Fe) or without iron during 14 days (–Fe). First symptoms of iron deficiency appeared 8 days (D +8) after deprivation with pale green leaves on top. Plants exhibit typical interveinal chlorosis after 10 days (D +10) becoming more pronounced after 12 days (D +12). (B) Representative long-term recordings of electric potential (EP) used as training dataset for modeling. Plants were submitted to optimal nutrient condition (comfort, +Fe) followed by iron deprivation (deficiency, –Fe) in fertigation. Colored arrows represent different stage of symptoms on young leaves from non-visible (green) to pale green (yellow) then chlorosis (red).
Modeling Electrical Signal for Early Detection
Long-term EP monitoring were carried out on hydroponic tomato crops in soilless culture in response to Fe deprivation. Upon optimal growing and full nutrient conditions, EP showed typical cyclic variations (Tran et al., 2019) with a higher EP amplitude during day time compared to night time (Figure 1B). During Fe deprivation, EP variations showed a modification of the baseline and the amplitude. The slight and long-term modifications of electrical signal patterns probably reflect the adaptation of plant metabolism in order to cope the lack of Fe.
We then investigate whether recorded EPs provide information for early diagnosis of Fe deficiency through the use of machine learning-based algorithms. For predicting Fe deficiency, gradient boosted trees (GBT) model showed an accuracy of 70.85% with a recall and precision value of 83.1 and 79.2%, respectively (Table 1). In order to improve processing computer speeds, we assess the effect of sample rate on model performance. Reducing the sample rate from 500 Hz to 250, 50, and 1 Hz lead to an accuracy of 76.0, 79.0, and 79.3%, respectively (Table 1). The more the sample rate is reduced, the more the model performance is increased. In the same manner, the model performance with the test dataset (e.g., not used for the training) improved with a decrease of the sample rate. Overall, model on data processed at 1 Hz showed the best performance with an accuracy reaching 76.0% with test dataset. The effect of sampling rate has been shown in different modeling approaches leading to better performance of classification (Branco et al., 2018; Dirand et al., 2019). This down sampling approach would allow reduced computing power and enable implementation in actual commercial greenhouse set-ups for fertigation management.

Table 1. Accuracy, Precision, Recall and Validation values for all prediction models at different sample rate to determine iron deficiency.
We undertook a prediction with unseen data (test dataset) starting from the beginning until the end of iron deprivation. The model predicted a control state over 70% during full nutrient irrigation condition and during the first days (D +1) of Fe deprivation (Figure 2). On the contrary, model predicted a strongly stressed state, when the first visible chlorosis appears at D +10 of Fe deprivation, which is reflected by a control state predicted below 30%. These results demonstrate that both full nutrient and Fe deprivation condition can be efficiently predicted using the developed algorithm. It is noteworthy that the prediction rate dropped from D +2 until D +9 with a control state value around 50% showing that model prediction is oscillating between a control and stressed state. This oscillating behavior probably reflect the transition state between normal to visible symptoms state, representing the nutrient imbalance caused by iron. Moreover, in our conditions, long-term iron deprivation in hydroponic soilless tomato induced a yield loss of around 23 ± 8.8% (data not shown). Further investigations are required to assess how early detection of iron deficiency will translate into higher fruit yield and quality of fruit. Altogether, these results support the idea that electrical signals provide information for early diagnosis of the lack of Fe in fertigation.
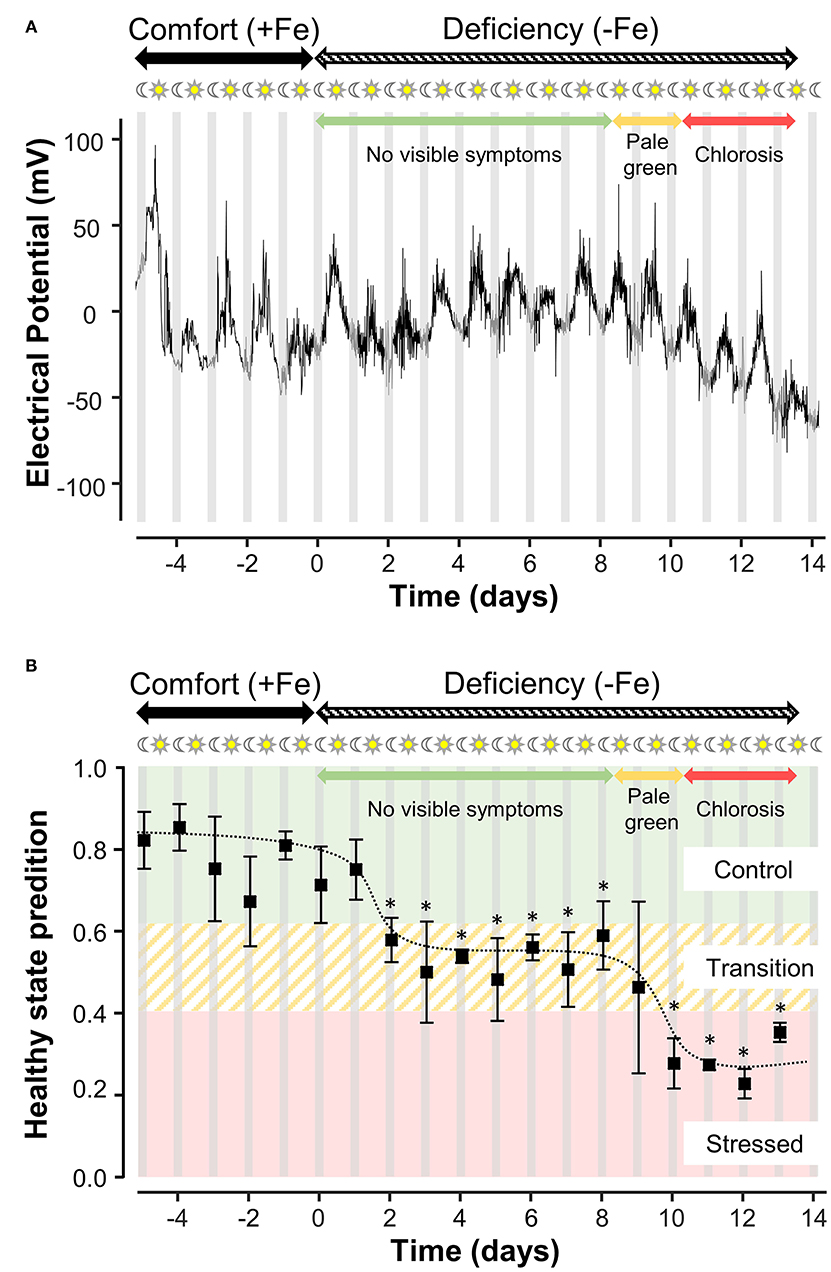
Figure 2. (A) Representative long-term recording of electric potential (EP) used as the test dataset to evaluate model performance. Tomato plants were submitted to optimal nutrient condition (Comfort, +Fe) followed by iron deprivation (Deficiency, –Fe) in fertigation. Colored arrows represent different stages of visible symptoms on young leaves from non-visible (green) to pale green (yellow) then chlorosis (red). (B) Model prediction of healthy plant state on hydroponic tomato plants during different fertigation conditions i.e., with (+Fe) or without (–Fe) iron. Different physiological states were defined depending on prediction rate: above 0.6, considered as control or healthy (green), between 0.4 and 0.6, considered as transition state from healthy to stressed (orange) and below 0.4 as stressed (red). Results represents mean ± s.e.m (n = 3). Asterisk (*) indicates significantly different from comfort condition (Mann–Whitney Rank Sum Test, p < 0.05).
Conclusion
In the present study, we provide compelling evidence that assessment of plant EP in commercial tomato crop contains information to predict Fe deprivation in fertigation. Electrical signal patterns can be modeled to detect iron deficiency prior to visual symptoms appearing in tomato plants. The proposed classification model showed classification accuracy of 79.3% with an efficient prediction rate on unseen data reaching 76.0%. With a model built to classify normal (e.g., full nutrient) vs. strong Fe deficiency condition (e.g., visible chlorosis), the approach allows early detection of slight Fe deprivation. Indeed, the Fe deficiency was detected 2 days (D +2) after deprivation whereas the very first symptoms (pale green young leaf) appeared 6 days later (D+8).
Signal transduction for various nutrients mediate through the xylem and phloem. When investigating plant nutrition, a sensor monitoring electrical variations in conductive tissue, could provide clues in understanding the underlying principles of nutrient stress-induced warning mechanisms. Further investigations are required to define the specificity amongst other nutrient deficit responses. This study represents a potential application of EP monitoring combined with machine learning and opens avenue of research that can be extended to others essentials nutrient for crop. Algorithms should be developed further to be deployed and then tested allowing real-time diagnosis and act as an early detection system for identifying any nutrient deficiency. In turn, this enables implementation in actual commercial greenhouse set-ups. This would potentially be a useful tool to enable efficient crop production and provide assistance for fertilizer management to farmers in the future.
Data Availability Statement
The raw data supporting the conclusions of this article will be made available by the authors, without undue reservation.
Author Contributions
DT performed experiments, analyzed data, and wrote the manuscript. DT and CC designed the study. Both authors discussed the results and commented on the manuscript.
Funding
This work was funded by Innosuisse, the Swiss Innovation Agency, grant #27661.1 PFLS-LS PISA.
Conflict of Interest
The authors declare that the research was conducted in the absence of any commercial or financial relationships that could be construed as a potential conflict of interest.
Acknowledgments
We would like to thank Carrol Plummer and Nigel Wallbridge (Vivent SA, CH) for carefully reading the manuscript and for insightful discussions.
References
Abadía, J., Vázquez, S., Rellán-Álvarez, R., El-Jendoubi, H., Abadía, A., Álvarez-Fernández, A., et al. (2011). Towards a knowledge-based correction of iron chlorosis. Plant Physiol. Biochem. 49, 471–482. doi: 10.1016/j.plaphy.2011.01.026
Branco, M. P., Freudenburg, Z. V., Aarnoutse, E. J., Vansteensel, M. J., and Ramsey, N. F. (2018). Optimization of sampling rate and smoothing improves classification of high frequency power in electrocorticographic brain signals. Biomed. Phys. Eng. Express. 4:045012. doi: 10.1088/2057-1976/aac3ac
Chakraborty, B., Chakraborty, K., and Bhaduri, D. (2015). “An insight of iron chlorosis in horticultural crops: physiological and molecular basis, and possible management strategies,” in Advances in Plant Physiology, ed A. Hemantaranjan (Jodhpur: Scientific Publishers), 239–270.
Dirand, A.-S., Frouin, F., and Buvat, I. (2019). A downsampling strategy to assess the predictive value of radiomic features. Sci. Rep. 9:17869. doi: 10.1038/s41598-019-54190-2
Graham, R. D. (2008). “Micronutrient deficiencies in crops and their global significance,” in Micronutrient Deficiencies in Global Crop Production (Dordrecht: Springer), 41–61. doi: 10.1007/978-1-4020-6860-7_2
Hänsch, R., and Mendel, R. R. (2009). Physiological functions of mineral micronutrients (Cu, Zn, Mn, Fe, Ni, Mo, B, Cl). Curr. Opin. Plant Biol. 12, 259–266. doi: 10.1016/j.pbi.2009.05.006
Jones, J. D. (2020). Iron availability and management considerations: a 4R approach. Crop. Soils 53, 32–37. doi: 10.1002/crso.20019
Khan, M. A., and Nguyen, N. T. (2020). “Chapter eight - Genome editing of staple crop plants to combat iron deficiency,” in Transgenic Technology Based Value Addition in Plant Biotechnology, eds U. Kiran, M. Z. Abdin, and Kamaluddin (London: Academic Press), 187–206. doi: 10.1016/B978-0-12-818632-9.00008-3
Kobayashi, T., Nozoye, T., and Nishizawa, N. K. (2019). Iron transport and its regulation in plants. Free Radic. Biol. Med. 133, 11–20. doi: 10.1016/j.freeradbiomed.2018.10.439
Maathuis, F. J. M., and Diatloff, E. (2013). Roles and functions of plant mineral nutrients. Methods Mol. Biol. 953, 1–21. doi: 10.1007/978-1-62703-152-3_1
Morgan, J. W., and Anders, E. (1980). Chemical composition of Earth, Venus, and Mercury. Proc. Natl. Acad. Sci. 77, 6973–6977. doi: 10.1073/pnas.77.12.6973
Panda, B. B., Sharma, S., Mohapatra, P. K., and Das, A. (2012). Application of excess nitrogen, phosphorus, and potassium fertilizers leads to lowering of grain iron content in high-yielding tropical rice. Commun. Soil Sci. Plant Anal. 43, 2590–2602. doi: 10.1080/00103624.2012.716122
Tran, D., Dutoit, F., Najdenovska, E., Wallbridge, N., Plummer, C., Mazza, M., et al. (2019). Electrophysiological assessment of plant status outside a Faraday cage using supervised machine learning. Sci. Rep. 9:17073. doi: 10.1038/s41598-019-53675-4
White, P. J., and Brown, P. H. (2010). Plant nutrition for sustainable development and global health. Ann. Bot. 105, 1073–1080. doi: 10.1093/aob/mcq085
Keywords: electrical signal, greenhouse, iron deficiency, machine learning, classification
Citation: Tran D and Camps C (2021) Early Diagnosis of Iron Deficiency in Commercial Tomato Crop Using Electrical Signals. Front. Sustain. Food Syst. 5:631529. doi: 10.3389/fsufs.2021.631529
Received: 20 November 2020; Accepted: 03 February 2021;
Published: 25 February 2021.
Edited by:
Jitendra Paul Khurana, University of Delhi, IndiaReviewed by:
Tahira Fatima, Purdue University, United StatesAgnieszka Barbara Najda, University of Life Sciences of Lublin, Poland
Copyright © 2021 Tran and Camps. This is an open-access article distributed under the terms of the Creative Commons Attribution License (CC BY). The use, distribution or reproduction in other forums is permitted, provided the original author(s) and the copyright owner(s) are credited and that the original publication in this journal is cited, in accordance with accepted academic practice. No use, distribution or reproduction is permitted which does not comply with these terms.
*Correspondence: Daniel Tran, qnoctnandaniel.tran@agroscope.admin.ch