- Faculty of Agriculture, University of Miyazaki, Miyazaki, Japan
To enhance productivity and welfare of individual animals maintained as a group, management based on individual behavioral tendencies is essential, which requires individual monitoring of animal behavior. Several behavior monitoring systems are currently available to livestock producers. The data obtained from these systems are analyzed to detect significantly high or low frequencies or intensities of behavior associated with estrus, calving and poor health conditions based on thresholds or past trends of the monitored individual. However, because behavior under grazing is more complex and changeable than under confinement, behavioral symptoms are more difficult to detect, and on-farm monitoring of individual animal behavior has been less validated and utilized in grazing systems. Nevertheless, individual monitoring of all animals in a herd is more feasible and cost-effective in small-scale intensive grazing systems because these systems pursue high productivity at the individual level with smaller herd size than large-scale extensive systems. Individually tailored management to enhance productivity and welfare will be possible by focusing on inter-individual differences in behavior within a herd. Behavior of an individual can be analyzed and understood in more detail by comparing it with those of the herd mates. Higher or lower levels of specific activities than the other animals can be associated with health disorders, temporal changes in physiological states, or productivity- or welfare-related traits. More sensitive monitoring and detection of behavioral responses of individuals to changes in nutritional, physical and social environments will lead to more efficient and welfare-conscious management that better meets the needs of individuals.
Importance of Individual Monitoring of Animal Behavior
Behavior of animals maintained as a group such as cattle has often been represented as an average of the herd, and the average has been used to aid management as an indicator of external and/or internal environments of animals. In grazing systems, for example, decreased daily grazing time under extended stocking in a paddock, as a possible indicator of depleting forage availability, can be used to assess the need for supplementary feeding or switching to a new paddock. Prolonged resting time during the summer days, as an indicator of heat stress, can be a consideration for modification of the access time to pasture. However, individual animals exhibit different behavioral tendencies due to differences in traits such as age, sex, body size, physiological and emotional states, social dominance, past experiences and personality. Hence, management based on the average behavior does not equally benefit all animals in the herd and may even negatively affect animals biased from the average (Richter and Hintze, 2019). To enhance productivity and welfare of individual animals, individually tailored management based on individual behavioral tendencies is essential, which in turn requires individual monitoring of animal behavior. Technological advances and cost reductions in sensor use are making it feasible to constantly and precisely monitor behavior of individual animals in a herd with minimal interference. In this mini-review, we aimed at (1) briefly reviewing current situation of commercially available monitoring systems of animal behavior and (2) assessing prospects and challenges for individual monitoring of behavior to enhance productivity and welfare of animals, with particular reference to small-scale intensive cattle grazing systems. Here, “small-scale intensive grazing systems” refer to the systems in which fenced sward paddocks are rotationally or seasonally grazed by some hundreds of animals or less according to forage availability, while pursuing high productivity at the individual level, as distinct from pastoralism systems on rangelands covering the landscape.
Current Situation of Commercially Available Monitoring Systems of Animal Behavior
Several monitoring systems of individual animal behavior are currently available to livestock producers. These systems generally include wearable devices to mount on animals, terminals to remotely collect data from the devices and applications to see and manage behavioral data on smart devices. In cattle production systems, devices including three-axis accelerometers (with additional sensors) to mount on the neck [e.g., Farmnote® (Farmnote Holdings Inc., 2020) and U-motion® (Desamis Co. Ltd., 2020)], an ear [CowManager® (CowManager, 2020)] or a hind leg [CowAleart® (IceRobotics Ltd., 2020)] of an animal are currently most popular because data on static and dynamic acceleration can respectively provide information on angles and movements to classify the posture and behavior of the animal (Andriamandroso et al., 2017). Information on daily activity patterns (time spent feeding, ruminating, resting, drinking, etc.) and activity intensities or step counts can be obtained from these systems. The time-series behavioral data are analyzed to detect significantly high or low frequencies or intensities of behavioral variables associated with estrus (Valenza et al., 2012), calving (Saint-Dizier and Chastant-Maillard, 2015) and poor health conditions such as lameness (Thorup et al., 2015) and dysstasia (difficulty in standing) based on thresholds or past trends of the monitored individual. The behavioral information is useful to avoid missing the timing of artificial insemination, to decrease accidents at calving (e.g., fetal death due to dystocia) and to find and treat health disorders in early stages, and thus can reduce economic loss and enhance management efficiency. Despite the recent spread into various systems (cow-calf or fattening of beef, or dairy), on-farm monitoring of individual cattle behavior has been primarily validated and utilized in confinement systems such as free-stall barns, with much fewer uses in grazing systems (Jaeger et al., 2019).
Prospects and Challenges for Grazing Management Based on Individual Animal Behavior
Behavior under grazing is more complex and changeable than that under confinement because animals adapt their behavior to their ever-changing environments such as sward and weather conditions. Therefore, behavioral symptoms are more difficult to detect under grazing (Kamphuis et al., 2012). Nevertheless, individual monitoring of all animals in a herd is more feasible and cost-effective in small-scale intensive grazing systems because these systems pursue high productivity at the individual level with smaller herd size than large-scale extensive systems. Data transfer from animal-mounted devices to a terminal is also easier in small-scale systems because animals visit a fixed place such as a barn every day. Information on continuous individual animal behavior around the clock can be much more detailed than a single manual inspection per day, and to some extent, but not completely, it can replace manual labor to monitor animals, thus saving time and money (Herlin et al., 2021). In addition, although daily visual observations or milk yield and quality information can help managers to notice clear abnormalities or clinical signs such as lameness or mastitis, behavioral monitoring has a potential to capture minor changes in individuals and early signs of abnormality. The current financial constraints on the introduction of the systems will be acceleratingly reduced through a cycle of further increasing production and decreasing prices along with the widespread use. In the near future, the monitoring systems of behavior are expected to be adopted widely in small-scale intensive grazing systems, and producers will be able to know daily behavior of all of their animals. What findings and benefits for enhancement of productivity and welfare of animals can be gained from individual monitoring of behavior in small-scale intensive grazing systems?
Even though behavior under grazing is complex and changeable, individually tailored management to enhance productivity and welfare will be possible by focusing on inter-individual differences in behavior within a herd. Behavior of an individual within a herd can be analyzed and understood in more detail by comparing the time-series data of the individual with those of the herd mates. Through the improved detection algorithms, a behavioral change in an individual can be judged as a change deviated from the herd mates (Figure 1A) or synchronized with them (Figure 1B). Even if an individual maintains behavioral stability, the animal can be detected as an outlier when a difference from the herd mates is generated (Figure 1C) or maintained (Figure 1D). The comparison can also detect an animal that behaves differently from the herd mates but not as distinctly as an outlier (e.g. Figures 1E–H). Higher or lower levels of specific activities in an animal than the others can be associated with health disorders, temporal changes in physiological states or higher or lower behavioral responsiveness to the environment (behavioral plasticity; Biro and Adriaenssens, 2013). Longer time spent grazing by an animal than the herd mates may be suspected as a temporal negative energy balance (Matthews et al., 2012). Although such cases are often seen in cows in early lactation stages, such animals may lose their body weights and conditions, which results in reproductive inefficiency and temporary deprivation of freedom from hunger, one of the Five Freedoms (Farm Animal Welfare Council, 1993). Temporary isolation of such animals from the herd and intensive treatment may mitigate the negative energy balance. Individually tailored supplemental feeding according to each animal behavior is also effective if feeders that can identify individuals are available. However, efficient management methods for individual animals kept mainly on pasture need to be developed in the future. Health alarms based on the outlier detection of a few behavioral indicators may lead to a high number of false positive alerts, as with the detection models based on thresholds (Brassel et al., 2019). Data collected from various automatic recording technologies need to be processed and integrated into a single outcome of animal production or welfare (which is easy to understand by the consumer) (Stygar et al., 2021). Further research is required to develop methods to effectively combine multiple behavioral indicators with each other and with physiological indicators obtained from milking robots and other sources and to integrate different analytical algorithms (threshold-based detection and comparison within a herd).
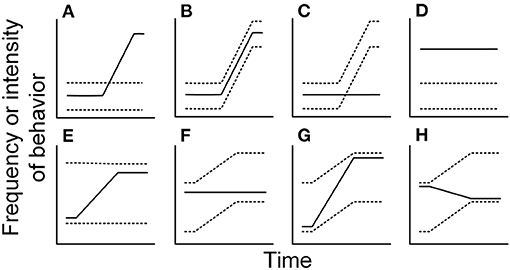
Figure 1. Schematic diagrams of comparison of time-series behavioral data between an animal (solid lines) and the herd mates (dotted lines). By comparing individual behavior with those of the herd mates, a behavioral change in an individual can be judged as a change deviated from the herd mates (A) or synchronized with them (B). Even if an individual maintains behavioral stability, the animal can be detected as an outlier when a difference from the herd mates is generated (C) or maintained (D). The comparison can also detect an animal that behaves differently from the herd mates but not as distinctly as an outlier (E–H).
Consistently higher or lower tendency of behavioral variable values over a long period (e.g., years) can be related with less changeable individual traits such as personality (Figure 1D). Owing to increasing interests in animal personality and similar concepts such as temperament, behavioral syndrome and coping style, the relationships of behavioral consistency with fitness (Smith and Blumstein, 2008) and energy metabolism (Biro and Stamps, 2010), which support growth, survival and breeding success of individuals, have been investigated and discussed for various species of animals. Cattle personality has been found to be associated with productivity-related traits [e.g., weight gain (Petherick et al., 2009), feed conversion efficiency (Gregorini et al., 2015), days from calving to body weight nadir, calf weaning weight (Wesley et al., 2012), carcass quality (Hall et al., 2011), and milk and fat yield (Jaeger et al., 2019)] and welfare-related traits [e.g., body condition score as a measure of hunger (Matthews et al., 2012) and somatic cell count as a measure of udder health (Jaeger et al., 2019)]. Since several personality traits are heritable (García et al., 2020), application of the associations to breeding programs of a herd by selecting animals that have more productive and adaptive personality traits may enhance productivity and welfare of animals. However, this idea is still controversial because the risks along with reduced variation of behavioral traits are still unknown (Richter and Hintze, 2019). Because past experience affects individual behavioral traits, giving opportunities to gain desirable behavioral traits during development can enhance lifetime productivity (Mulliniks et al., 2016) and welfare (Richter and Hintze, 2019) of animals. Further research is warranted for the relationships of personality with productivity- and welfare-related traits of individual animals.
Incorporation of GPS into the monitoring systems can provide valuable information on movement and spatial distribution of individual animals under grazing (Bailey et al., 2018). Traveling distance can be used as a proxy of energy expenditure (Brosh et al., 2006). Spatial distribution can be related with exploration–avoidance dimensions of livestock temperaments (Wesley et al., 2012). Inter-individual positional relations such as distance from herd mates and spatial position relative to herd movement that can be calculated from GPS data can provide information on sociability, leadership and dominance of individuals (Šárová et al., 2010).
More sensitive monitoring and detection of behavioral responses of individuals to changes in nutritional, physical and social environments will lead to more efficient and welfare-conscious management that better meets the needs of individuals in small-scale intensive grazing systems.
Author Contributions
HA conceived the idea and prepared the review. MH contributed to the content, wrote parts, and edited the manuscript. Both authors contributed to the article and approved the submitted version.
Conflict of Interest
The authors declare that this work was conducted in the absence of any commercial or financial relationships that could be construed as a potential conflict of interest.
Publisher's Note
All claims expressed in this article are solely those of the authors and do not necessarily represent those of their affiliated organizations, or those of the publisher, the editors and the reviewers. Any product that may be evaluated in this article, or claim that may be made by its manufacturer, is not guaranteed or endorsed by the publisher.
References
Andriamandroso, A. L. H., Lebeau, F., Beckers, Y., Froidmont, E., Dufrasne, I., Heinesch, B., et al. (2017). Development of an open-source algorithm based on inertial measurement units (IMU) of a smartphone to detect cattle grass intake and ruminating behaviors. Comput. Electron. Agric. 139, 126–137. doi: 10.1016/j.compag.2017.05.020
Bailey, D. W., Trotter, M. G., Knight, C. W., and Thomas, M. G. (2018). Use of GPS tracking collars and accelerometers for rangeland livestock production research. Transl. Anim. Sci. 2, 81–88. doi: 10.1093/tas/txx006
Biro, P. A., and Adriaenssens, B. (2013). Predictability as a personality trait: consistent differences in intraindividual behavioral variation. Am. Nat. 182, 621–629. doi: 10.1086/673213
Biro, P. A., and Stamps, J. A. (2010). Do consistent individual differences in metabolic rate promote consistent individual differences in behavior? Trends Ecol. Evol. 25, 653–659. doi: 10.1016/j.tree.2010.08.003
Brassel, J., Rohrssen, F., Failing, K., and Wehrend, A. (2019). Automated detection of health disorders in lactating dairy cattle on pasture: a preliminary study. Polish J. Vet. Sci. 22, 761–767. doi: 10.24425/pjvs.2019.131406
Brosh, A., Henkin, Z., Ungar, E. D., Dolev, A., Orlov, A., Yehuda, Y., et al. (2006). Energy cost of cows' grazing activity: use of the heart rate method and the Global Positioning System for direct field estimation. J. Anim. Sci. 84, 1951–1967. doi: 10.2527/jas.2005-315
CowManager, B. V. (2020). CowManager®. Available online at: https://www.cowmanager.com/en-us/ (accessed March 31, 2020).
Desamis Co. Ltd. (2020). U-motion®. Available online at: http://desamis.co.jp/en/ (accessed March 31, 2020).
Farm Animal Welfare Council (1993). Second Report on Priorities for Animal Welfare Research and Development. London: Department for Environment, Food and Rural Affairs Publications.
Farmnote Holdings Inc. (2020). Farmnote®. Available online at: https://farmnote.jp/en/ (accessed March 31, 2020).
García, C. A. M., Maxwell, T. M., Hickford, J., and Gregorini, P. (2020). On the search for grazing personalities: from individual to collective behaviors. Front. Vet. Sci. 7:74. doi: 10.3389/fvets.2020.00074
Gregorini, P., Waghorn, G. C., Kuhn-Sherlock, B., Romera, A. J., and Macdonald, K. A. (2015). Grazing pattern of dairy cows that were selected for divergent residual feed intake as calves. J. Dairy Sci. 98, 6486–6491. doi: 10.3168/jds.2015-9614
Hall, N. L., Buchanan, D. S., Anderson, V. L., Ilse, B. R., Carlin, K. R., and Berg, E. P. (2011). Working chute behavior of feedlot cattle can be an indication of cattle temperament and beef carcass composition and quality. Meat Sci. 89, 52–57. doi: 10.1016/j.meatsci.2011.03.020
Herlin, A., Brunberg, E., Hultgren, J., Högberg, N., Rydberg, A., and Skarin, A. (2021). Animal welfare implications of digital tools for monitoring and management of cattle and sheep on pasture. Animals 11, 829. doi: 10.3390/ani11030829
IceRobotics Ltd. (2020). CowAleart®. Available online at: https://www.icerobotics.com/ (accessed March 31, 2020).
Jaeger, M., Brügemann, K., Brandt, H., and König, S. (2019). Associations between precision sensor data with productivity, health and welfare indicator traits in native black and white dual-purpose cattle under grazing conditions. Appl. Anim. Behav. Sci. 212, 9–18. doi: 10.1016/j.applanim.2019.01.008
Kamphuis, C., DelaRue, B., Burke, C. R., and Jago, J. (2012). Field evaluation of 2 collar-mounted activity meters for detecting cows in estrus on a large pasture-grazed dairy farm. J. Dairy Sci. 95, 3045–3056. doi: 10.3168/jds.2011-4934
Matthews, L. R., Cameron, C., Sheahan, A. J., Kolver, E. S., and Roche, J. R. (2012). Associations among dairy cow body condition and welfare-associated behavioral traits. J. Dairy Sci. 95, 2595–2601. doi: 10.3168/jds.2011-4889
Mulliniks, J. T., Cope, E. R., McFarlane, Z. D., Hobbs, J. D., and Waterman, R. C. (2016). Drivers of grazing livestock efficiency: how physiology, metabolism, experience and adaptability influence productivity. J. Anim. Sci. 94, 111–119. doi: 10.2527/jas.2015-0711
Petherick, J. C., Doogan, V. J., Venus, B. K., Holroyd, R. G., and Olsson, P. (2009). Quality of handling and holding yard environment, and beef cattle temperament: 2. Consequences for stress and productivity. Appl. Anim. Behav. Sci. 120, 28–38. doi: 10.1016/j.applanim.2009.05.009
Richter, S. H., and Hintze, S. (2019). From the individual to the population–and back again? Emphasising the role of the individual in animal welfare science. Appl. Anim. Behav. Sci. 212, 1–8. doi: 10.1016/j.applanim.2018.12.012
Saint-Dizier, M., and Chastant-Maillard, S. (2015). Methods and on-farm devices to predict calving time in cattle. Vet. J. 205, 349–356. doi: 10.1016/j.tvjl.2015.05.006
Šárová, R., Špinka, M., Panamá, J. L. A., and Šimeček, P. (2010). Graded leadership by dominant animals in a herd of female beef cattle on pasture. Anim. Behav. 79, 1037–1045. doi: 10.1016/j.anbehav.2010.01.019
Smith, B. R., and Blumstein, D. T. (2008). Fitness consequences of personality: a meta-analysis. Behav. Ecol. 19, 448–455. doi: 10.1093/beheco/arm144
Stygar, A. H., Gómez, Y., Berteselli, G. V., Dalla Costa, E., Canali, E., Niemi, J. K., et al. (2021). A systematic review on commercially available and validated sensor technologies for welfare assessment of dairy cattle. Front. Vet. Sci. 8:177. doi: 10.3389/fvets.2021.634338
Thorup, V. M., Munksgaard, L., Robert, P. E., Erhard, H. W., Thomsen, P. T., and Friggens, N. C. (2015). Lameness detection via leg-mounted accelerometers on dairy cows on four commercial farms. Animal 9, 1704–1712. doi: 10.1017/S1751731115000890
Valenza, A., Giordano, J. O., Lopes Jr, G., Vincenti, L., Amundson, M. C., and Fricke, P. M. (2012). Assessment of an accelerometer system for detection of estrus and treatment with gonadotropin-releasing hormone at the time of insemination in lactating dairy cows. J. Dairy Sci. 95, 7115–7127. doi: 10.3168/jds.2012-5639
Keywords: accelerometer, behavioral consistency, cattle, GPS, individually tailored management
Citation: Anzai H and Hirata M (2021) Individual Monitoring of Behavior to Enhance Productivity and Welfare of Animals in Small-Scale Intensive Cattle Grazing Systems. Front. Sustain. Food Syst. 5:694413. doi: 10.3389/fsufs.2021.694413
Received: 13 April 2021; Accepted: 16 August 2021;
Published: 14 September 2021.
Edited by:
Pablo Gregorini, Lincoln University, New ZealandReviewed by:
Xavier Manteca, Universitat Autònoma de Barcelona, SpainGiovanni Molle, Agris Sardinia, Italy
Copyright © 2021 Anzai and Hirata. This is an open-access article distributed under the terms of the Creative Commons Attribution License (CC BY). The use, distribution or reproduction in other forums is permitted, provided the original author(s) and the copyright owner(s) are credited and that the original publication in this journal is cited, in accordance with accepted academic practice. No use, distribution or reproduction is permitted which does not comply with these terms.
*Correspondence: Hiroki Anzai, YW56YWkuaGlyb2tpLnE2QGNjLm1peWF6YWtpLXUuYWMuanA=