- 1Department of Applied Economics and Marketing, School of Agriculture, Policy and Development, University of Reading, Reading, United Kingdom
- 2Department of Sustainable Land Management, School of Agriculture, Policy and Development, University of Reading, Reading, United Kingdom
Integration of crop and livestock production systems (ICLS) represents a method for enhancing the sustainability of agricultural systems. Introducing more diversified farm production plans increases profitability and resilience by minimising the negative environmental impacts of agricultural production. Examining farm businesses located in Less Favoured Areas (LFAs) of England, we investigate how conversion into more integrated systems impacts on profitability. Thus, providing knowledge that can enable structural changes on the farm level towards enhancing financial performance and the sustainable intensification of the production system. Through Linear Programming (LP), four distinct optimisation scenarios are estimated, demonstrating the different dynamics between more specialised and more integrated-diversified (intensified) production systems. Data regarding physical and financial performances of 139 farm businesses were derived from the Farm Business Survey (FBS) for the accounting year of 2013–2014. Our findings suggest that there is a lot of potential for increasing profitability of hill farms through optimisation of ICLS. Policy interventions may accommodate productivity challenges within the LFAs via the construction of networks of transferrable knowledge to enable farmers gain knowledge on benefits emerging from ICLS. Hence, promote strategies and risk mitigation practises that could allow hill farmers to develop a sustainably intensified production system that is maximising the production capacity of the available natural resources.
Introduction
The European Union (EU) has designated areas in which agricultural production is more challenging due to challenging biophysical conditions, the Less Favoured Areas (LFAs). To mitigate production risks, ensure continuous use of agricultural land, maintenance of the countryside and to enhance the future sustainability of farming systems, the EU has historically provided both financial and structural support to hill farmers (European Commission, 2005). Agricultural production in these landscapes is restricted by poor climate, lower soil productivity, steep slopes and harsh conditions (European Commission, 2006; Harvey and Scott, 2015). These natural handicaps associated with the spatial characteristics of the production system can decrease productivity within the LFAs and consequently, result in decrease or cessation of farming activities hence in land abandonment and a series of environmental and social risks related to loss of biodiversity, depopulation or loss of agricultural land (European Commission, 2005, 2010). Therefore, an emerging need for the design of strategies and policies to enhance productivity while simultaneously reducing the environmental pressures generated by the production system is realised. A sustainable development paradigm which will also account for the balance between sustainability and enhanced productivity will enable the Sustainable Intensification (SI) (Gadanakis et al., 2015) of hill farming systems.
Grazing livestock production is the predominant agricultural activity within the LFAs of England (DEFRA, 2010). On average, crop production accounts for ~5% of the total output of farm businesses in the LFAs (Harvey and Scott, 2015). Specialisation of livestock production has emerged in areas dominated by natural handicaps with smaller farm holdings, where animal production has long been established as the predominant type of agricultural activity (Peyraud et al., 2014; Martin et al., 2016). However, specialisation of production on hill farms has led to environmental degradation and reduction of biodiversity (Tichit et al., 2011; Bonaudo et al., 2014; Peyraud et al., 2014).
Integration of crop and livestock production systems (ICLS) has gained momentum as a strategic management approach in the sustainable planning of agricultural systems (Hendrickson et al., 2008; Sanderson et al., 2013; Duru and Therond, 2015). From the economic perspective, diversification of agricultural production enables benefits from economies of scope (Sanderson et al., 2013) which result in lower costs of production in integrated production systems when compared to specialised ones (Panzar and Willig, 1981). ICLS allow the farm businesses to be less dependent on external inputs by utilising home grown crops as feeding stuffs as well as, by using animal manure as natural fertilisation for crop production (Soussana and Lemaire, 2014). Furthermore, utilisation of crops as forage at the farm level has been described as a process that enhances productivity within integrated agricultural systems (Bell et al., 2014). ICLS is therefore promoting a production system based in the circular economy concept as the system is closing the loop between materials and substances (Van Zanten et al., 2019).
From the environmental perspective, integration of agricultural production systems provides the option of reusing resources at the farm level (Schiere et al., 2002) which leads to nutrient cycle improvement (Reganold et al., 2010). In addition, ICLS provide environmental benefits through management of intractable weeds and diseases, enhanced water consumption efficiency (production of dual-purpose crops, that are being foraged during vegetative phase while harvesting for grain, are sown earlier and have longer vegetative phase and thus, higher water use efficiency due to deeper rooting) and improvement of soil quality (Bell et al., 2014).
While ICLS create synergies that increase productivity and resource use efficiency (Herrero et al., 2010), a series of parameters discourage farmers from implementing it. Within the economic and political context, historically, mass-production objectives promoted the development of economies of scale which resulted in specialisation of production and larger farm sizes (Ryschawy et al., 2013). Additionally, various farm structures require the relevant knowledge of the dynamics between crop and livestock production systems which emerges as another barrier as many farmers may lack the technical knowledge (Martin et al., 2016). Furthermore, the implementation of ICLS depends on the suitability of the spatial features of the landscape and climate which implies that in areas with more harsh conditions it may be more difficult to adopt such organisation plans. More specifically, in areas with more favourable conditions, production of cash crops is preferred because of the high-yield potentials (Gil et al., 2015), while in areas with more unfavourable environmental and physical conditions the cultivation of land is not feasible due to restrictions on mechanisation (e.g., steep slopes). The latter provides an interpretation of why ICLS is to be implemented in intermediate areas, in which crop production is feasible but on the other hand does not attain high yield productivity (Bonaudo et al., 2014). Furthermore, relevant studies have suggested that the adoption of ICLS depends on labour availability (as greater workload may be required) (Lemaire et al., 2014; Poffenbarger et al., 2017), local supply chain infrastructure (storage plants, production-input suppliers) and financial capital to invest in the new production systems and technology (Garrett et al., 2017).
Linear Programming (LP) modelling can be employed to examine how various levels of integration between crop and livestock production affect farm level sustainability (Sneessens et al., 2016). Furthermore, LP can optimise agricultural land use allocation by proposing minimisation of costs as well as maximisation of spatial compactness at the field scale (Aerts et al., 2003; Memmah et al., 2015). Additionally, approaches have attempted to optimise the integration between the various enterprises within the farm business, aiming towards profit maximisation (Glen, 1986; Annetts and Audsley, 2002; Veysset et al., 2005; Gameiro et al., 2016).
Here we quantified the potential transition of the English LFA livestock systems through integrated crop and livestock production strategies and optimal spatial allocation of 11 major crops and two livestock species to estimate a higher level of attainable farm-level profitability. We analysed differences in profitability (here Annual Gross Margin1 and Net Farm Income, AGM and NFI respectively) emerging from the optimised crop-livestock integration and utilisation of available resources. In addition, structural changes in farm businesses from optimised farm organisation were examined, along with resulting land use changes.
To explore the different strategies promoting the future model of the SI of LFA farms, a LP model was constructed integrating the parameters of feed requirements, housing capacity, stocking densities, labour and land use. The LP model distinguishes several agricultural production land adjustments scenarios that resemble a gradual set of production systems ranging from systems that utilise poor permanent pasture only, to farms that cultivate arable land and integrate crop and livestock production. This allowed the investigation of various levels of integration between crop and livestock production systems on economic as well as environmental performance.
Methods
Dataset and Variables
To investigate the optimisation of the profitability of upland farms by integrating crop and livestock enterprises, we use data derived from the Farm Business Survey (FBS). The FBS is a comprehensive dataset that provides information on financial and physical performances of a sample of farm businesses across England2 In the present analysis data for the 2013–2014 accounting year were employed using records of 139 farm businesses. Additionally, respective data from the previous four accounting years (2009–2013) were employed to calculate the historic maximum numbers of beef cattle per farm business. In addition, financial and physical data for the crop and livestock production were obtained from the Farm Management Pocketbook by Nix and Redman (2016) and also the Farm Management Handbook provided by Scotland's Farm Advisory Service (2016). While this set of data reflects a particular temporal point, we make the conservative assumption that the economic balances between crop and livestock enterprises at the farm level would remain similar as the factors determining them are strongly related to the fixed biophysical constraints inherent to the study area.
The objective function of this method estimates the Agricultural Gross Margin (AGM) which corresponds to market returns (enterprise outputs) less variable costs. Variable costs for the livestock enterprises include costs for concentrated and homegrown feeding stuffs, veterinary and medicines and other livestock costs (livestock haulage, packaging material, working dog expenses, bio-security costs etc.). Crop enterprise variable costs include costs for seed, fertilisers, crop protection (pesticides) and other requirements (soil analysis, soil sterilisation, marketing and haulage, packaging materials etc.). Livestock components in the modelling are expressed on a “per livestock unit” basis for the beef cattle and sheep production while a “per hectare” basis is used for the crop components. Furthermore, the costs of forage land are included in the objective function as the FBS dataset does not consider them in the calculation of livestock variable costs. In this method, forage land is disaggregated into the classes of temporary grassland which consists of grass/clover mixtures <5 years old, permanent grassland that comprises permanent pastures over 5 years old and rough grazing. Descriptive statistics of the key input and output variables in the model are presented in Table 1. Further description of the model inputs is available in the model activities section.
Linear Programming
This study constructs a deterministic, single period LP model that maximises the AGM of farm businesses in the LFAs of England (Benoit and Laignel, 2010; Hosu and Mushunje, 2013; Olaizola et al., 2015). Environmental backgrounds are incorporated in the approach by constraining the optimisation according to environmental conservation objectives (appropriate stocking densities) and physical (spatial) barriers (land availability). The solution of the model provides the corresponding optimal allocation of the various production enterprises including land use, labour and fertiliser requirements along with stocking rates at the farm level. To better examine the effects of integrating crop and livestock production, we introduce four distinct agricultural production system land adjustments via a linear programming optimisation process—for an ease of expression these will now be referred for the remaining of the discussion as scenarios (Table 2). These scenarios correspond to type and quality of farmed land ranging from systems that utilise poorer permanent pasture only3, to systems that utilise higher quality of pasture (temporary grassland) in combination to arable land. Comparisons between scenarios enable the investigation of the most feasible type of production system from the economic as well as the environmental perspective. In this section, the general form of the LP model along with the objective function is discussed and then the model activities along with the constraints are described.
Model Structure
The structure of the model is based on the general form of Linear Programming models proposed by Hazell and Norton (1989):
Where Z is the AGM at farm level, c is the vector of gross margins or costs per unit of activity, x the vector of activities, A is the matrix of technical coefficients and b is the vector of resource availability and technical constraints. The maximisation of (1) is subject to a range of constraining factors (2) that regard livestock housing capacity, stocking densities, livestock feeding requirements, labour and land use. These factors form eight constraints that are applied in the optimisation method (detailed description of constrains in the “Modelling set up” below):
The production activities of the farm business are organised in 15 categories describing production of beef cattle, sheep, temporary and permanent grass, wheat (spring and winter), barley (spring and winter), triticale, oats (spring and winter), beans, peas and oilseed rape (spring and winter).
Modelling Set Up
Livestock Housing Capacity
Housing is a significant parameter for the production of grazing livestock and has been considered as a constraining factor in relevant studies (Jansen and Wilton, 1984; Veysset et al., 2005; Acs et al., 2010). However, for the areas under consideration, housing is rarely required for sheep enterprises thus, it is only applied to the beef cattle enterprises. Specifically, this parameter indicates the number of beef cattle that can be maintained on each farm. However, the FBS dataset does not provide information on carrying capacity of buildings as such. Therefore, the maximum historic record (across the years of 2009–2014) was used as an indicator for the carrying capacity of the farm. This assumes that building capacity remains the same and is available for use.
Feeding Stuffs Requirement
Farm businesses can utilise their land for cultivation of temporary or permanent grassland as well as rough grazing to meet the feeding requirements of their stock. This type of intake can fulfil part of the dietary requirements of livestock. Additionally, home grown forage cereals (such as wheat or barley) can be produced and used on farm as feeding stuffs and/or compound feeds can be purchased. However, in the present method, crop production and concentrates purchased are not set to contribute to the feeding requirements of livestock. This was decided as the nutritional requirements of the flocks and cattle under consideration remain unknown due to lack of information on age and liveweight of the sheep and beef cattle. The use of feeding requirements as a constraint in this study relates to land used for rough grazing, permanent pasture and the cultivation of temporary grassland. FBS data was used to calculate the current number of hectares per animal on each farm. Through this calculation we estimate the requirement per head in hectares which varies between farms due to quality of pasture. Additionally, a ratio between temporary and permanent grassland was estimated. This ratio captured the balance between poorer (permanent) and higher (temporary) quality of grassland. The vast majority of the farms under consideration (66%) had a ratio of temporary and permanent grass land of 0 to 0.25. Specifically, this parameter forced the model to utilise some of the arable land for cultivation of temporary grass and forage crops. The latter was vital for the accuracy of the estimations as stocking capacity depend greatly on the quality of pasture. These are employed as fixed parameters per farm business in the LP modelling so that adequate pasture or temporary grassland is provided to the livestock.
Stocking Rate
This rate indicates the number of livestock units per hectare (LU/ha) that a farm is capable of supporting, with respect to environmental conservation as well as to economic interests. No recommendations exist for a maximum stocking density in English livestock farms, and thus, this was calculated from the FBS dataset. Due to heterogeneity of pasture quality in the study area, the forage land used for this calculation had to be adjusted on the basis of effective stocking density. This is provided only for the rough grazing areas while the permanent and temporary grassland remain unadjusted. The maximum effective stocking rate in the study area is 2.54 LU/ha (~1 cow per acre4) which is incorporated in the model as the upper bound. According to Scotland's Farm Advisory Service (2016), stocking rates range from 0.5 LU/ha which indicate very extensive production systems to 2.5 LU/ha which correspond to very intensive systems.
Land Use
The present method allows the optimal spatial reallocation of crop and livestock enterprises within the farm. Typically, in the upland farms there are some lower altitude improved areas (arable land) within their UAA in which crops can be grown (forage crops, other crops and temporary grass) and also there are areas situated at higher altitude facing harsh conditions providing only poor permanent pasture (permanent grass and rough grazing) (Acs et al., 2010) in which the steep slopes do not allow the use of machinery and equipment (Figure 1). Thus, in this method, main and fodder crop enterprises and temporary grassland are allowed to be reallocated only within the existing arable land while land currently used for permanent grassland and rough grazing cannot be converted into a different land use.
Labour Requirements
Labour is incorporated in the model as a constraining factor, as the supply of external labour inputs is limited in such remote agricultural areas (Darnhofer et al., 2010). Each enterprise requires a certain amount of labour in and this is derived in the form of Standard Labour Requirement (SLR) from the Farm Business Survey (FBS) dataset. SLR calculates the annual number of hours that each farm enterprise requires per unit of key input (hectares for crop and LU for livestock enterprises). In our model, the sum of the labour requirements of the optimised allocation of the ICLS cannot exceed the total SLR that the farm business has during the current accounting year. This limits the optimisation process to a degree, but the approach provides a useful proxy indicator of the availability of labour as no relevant data exists for the study area.
Robustness Testing of the Model
The models used in the present methodology integrate all types of livestock and crop production that exist within LFA farms and may therefore represent several specific types of farmland organisation ranging from specialised to diversified. Within the model, farm businesses can adjust their production organisation plan and level of integration between crop and livestock systems based on the suitability of available land for cultivation. In order to examine whether the optimisation scenarios provide a realistic optimal solution, the results of each scenario-model were compared to the actual performance of farms with equivalent production organisation (i.e., comparison of the averages of farm businesses between the current FBS data and the LP estimations). The major components of this process comprise the AGM derived from (i) livestock production and (ii) crop production. Table 3 presents a summary of these parameters for each type of farm organisation both for the current as well as the optimised estimations. Although LP assumes that farmers' objectives relate purely to profit maximisation (Acs et al., 2010), the models obtain rational results from production planning optimisation for all models.

Table 3. Comparison of current and optimised financial performance for each optimisation scenario. Figures show the percentage of total farm AGM derived from livestock and crop enterprises, plus the contribution of sheep and beef enterprise AGMs to the total livestock AGM.
Results
Financial Results
From the economic point of view, changes occur through increased AGM per hectare particularly in the PPA (Permanent pasture and rough grazing integrated with arable land) (μ = 762, s.d. = 205) and AMP (Mainly arable land with some permanent pasture and rough grazing) (μ = 757, s.d. = 217) scenario, which produces the highest average AGM per hectare. Additionally, information on the distribution of the results in quartiles, in relation to the median of the sample are presented in Figure 2. The optimised NFI obtains the highest average value under the PPA scenario (μ = 185, s.d. = 286) whereas the AMP has the lowest NFI (μ = 1, s.d. = 212). This implies high fixed costs for the AMP scenario that result in lower NFI. On the other hand, the AMP scenario produces the largest increase of NFI in absolute numbers (initial μ = −191, s.d. = 242) after PPA, indicating that farm businesses in these scenarios have the highest potentials for economic improvement. The latter applies especially to the AMP scenario which becomes marginally profitable, even though it shows the lowest current NFI (further information in Figure 3). Structural changes are estimated through the integration scenarios, as crop production is preferred over livestock production from the economic perspective. Across all scenarios (except for the PPO—Permanent grassland and rough grazing only) the percentage of AGM generated from livestock enterprises is decreased (Figure 4). The latter indicates that more arable land is allocated to crop production rather than temporary grassland. Furthermore, the optimal allocation of livestock within the farm business results in reduced numbers of beef cattle and increased numbers of sheep (Table 4). In terms of the total livestock units per farm, the PPO and PPM (Mainly permanent pasture and rough grazing with some arable land) and PPA scenarios yield increased livestock units, while in the AMP the livestock units per farm decline and livestock production is almost eliminated.
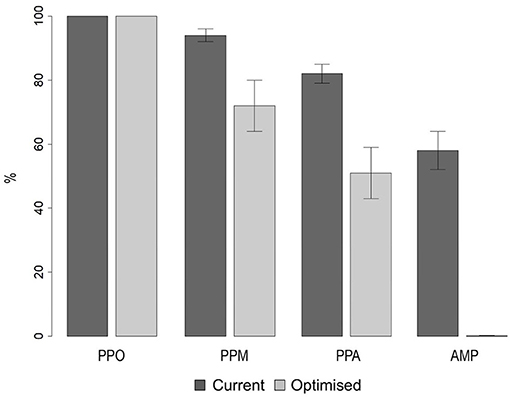
Figure 4. Mean percentage of Annual Gross Margin (±SE) from livestock production in the optimisation scenarios.
Spatial Considerations and Environmental Externalities
Land use change implications under the PPO scenario (where land is suitable only for livestock production) demonstrate a decrease for the permanent grass area (approximately 28%) (Table 5). In the PPM scenario all arable land is being utilised while 2/3 of this area is allocated to crop production. Through the PPA scenario, less permanent and temporary grass area is being used for livestock production which is triggered by a significant decrease in livestock numbers. In general, within arable land, production of main crops (66.2 ha) is preferred over fodder crops and temporary grass (15.8 ha). The highest decrease of forage land is estimated under the AMP scenario (approximately 89% for permanent and 95% for temporary grass). This decrease in forage land is justified for the AMP scenario since the land in consideration is mainly arable and hence, it is more likely that the model will allocate land to arable production since the AGM will be higher than the livestock enterprise.
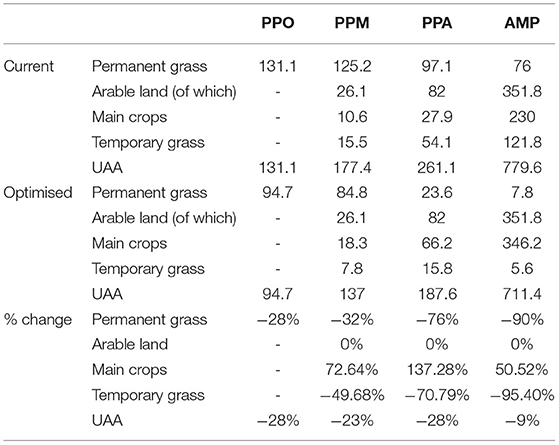
Table 5. Land use change for the different optimisation scenarios (average hectares per farm and percentage change).
It is estimated that across all optimisation scenarios some permanent and temporary grass land will come out of production. This mainly results from reductions in beef cattle numbers within all models and declining total livestock units in PPA and particularly for the AMP scenario (Table 4). Although some surplus of land emerges, the optimised solutions do not propose increase of livestock since the associated costs for production are greater than the market returns. The latter is significantly relevant for the AMP scenario where the model considers mainly arable land with some permanent pasture and rough grazing. Thus, releasing agricultural land (here permanent and temporary grass land) emerges as the most profitable option for the hill farm businesses. In general, the stocking densities for all scenarios remain relatively low and close to the current rates (ranging from 0.55 to 1.13 LU/ha). Stocking rates gradually increase from systems with higher percentages of poor permanent pasture to systems with more arable land (Table 6).
Environmental implications from such land use changes are presently examined through N fertiliser balances. Specifically, we estimate declining numbers of N application for all scenarios of integration (in tonnes per hectare). The highest reduction of N fertiliser application occurs in the AMP scenario (μ = 21, s.d. = 32). This mainly results from reductions of temporary grass land which requires significant amounts of N fertiliser (0.25 t/ha) to meet nutrient requirements. Furthermore, a significant increase is estimated for the PPA scenario (μ = 26, s.d. = 25) (Figure 5 demonstrates information on the distribution of these findings).
Sensitivity Analysis
Key findings of this method were further investigated through a sensitivity analysis to examine the validity of our estimations, as well as to explore how sensitive the estimation of AGM is to changes in model parameters (endowments) (Kaiser and Messer, 2011). The implications on AGM were examined with reference to livestock numbers, stocking densities and land use change. Regarding the livestock housing capacity, it is estimated that in the case that further housing becomes available, space for each additional beef cattle would increase AGM on average by £305. Furthermore, in the cases that permanent pasture land constrained the optimisation scenarios, it is estimated that for each additional hectare, an average increase of AGM by £352 would result. The gross margin of models for farms with a higher percentage of arable land are more sensitive to changes in permanent grass areas. Specifically, an increase of 1 hectare in permanent grass results in an increase of £293, £466 and £813 for the PPO, PPM and PPA scenarios respectively. This implies that on farms with more arable land, the pasture is of higher quality thus, resulting in higher gross margins per hectare. Concerning arable land, relaxing the availability by an additional hectare results in an increase of £520 in AGM on average. It is estimated that AGM is most sensitive to changes in arable land availability, as an increase of £637 is estimated for each additional hectare.
Discussion—Implications
Results indicate that there is a potential for increasing the AGM in LFA farm businesses through integration of crop and livestock production systems, however the four optimisation scenarios are suggesting different pathways. The profitability of hill farming systems is greatly affected by the quality of forage land. In particular, higher quality pasture land (here arable land used as temporary grassland) allows higher effective stocking densities due to higher forage yield, which in turn triggers higher AGM per hectare. This finding is in line with Willems et al. (2013), who found that good quality forage is a positive determinant of production performance in alpine sheep farming systems.
Moreover, our economic and spatial estimations point out that different levels of crop-livestock integration are related to financial performance on a per hectare basis. Studies have examined the direct effects of crop-livestock integration, highlighting that farm income increases when the level of crop production system increases within the farm business (Sneessens et al., 2016). On the other hand, Ripoll-Bosch et al. (2012), suggest that the economic performance of sheep farms does not depend on the diversity of production itself, rather it is the lower dependency on external inputs and thus improved feed self-sufficiency that drives performance. All scenarios examined by the optimisation model estimate the production of crops utilising as much of the land suitable for cultivation as is available hence, home produced feeding stuffs and feed self-sufficiency are promoted, contributing to improvements in financial performances (Bonaudo et al., 2014; Peyraud et al., 2014) via the pathway of circular economy (Van Zanten et al., 2019). Thus, it concludes towards a two-fold strategy where (a). land use intensity increases in the parts of land where optimally reallocated agricultural systems can return profits to the farmer and (b). land is being released when costs over exceed market returns and thus production is not economically sustainable. The optimisation results indicate a mix of inputs and the spatial allocation of natural resources such as land which improve farm level production efficiency. The suggested solutions by the LP in the four scenarios examined could serve as a framework to review by farm managers to review their current production structure and update their long-term goals towards a more sustainable and resilient farm business model.
Concerning environmental performance, declining requirements for N fertiliser use are estimated for all scenarios (median values). The latter is due to reductions in grassland (temporary and permanent) which requires significant amounts of N fertiliser. However, across the models, integration with higher levels of crop production indicate higher levels of N fertiliser application. Nonetheless, this is compensated by an average release of grassland of 22%. This is in accordance with the findings of Perrot et al. (2012) and Sneessens et al. (2016), who report that mixed livestock-crop production systems have worse environmental performances in comparison to specialised livestock production systems.
The effects of structural changes derived from optimal land use allocation suggest reductions in permanent pasture areas while land that is suitable for cultivation is utilised completely for crop production (that is suggested within the limits of land availability at a farm level and farm suitability). Among arable land, cereals (wheat, barley and oats) and peas production dominate while temporary grass land is also included to cover the feeding requirements of livestock within the farm business. This is a plausible result as crop enterprises obtain relatively higher AGM than livestock (Sneessens et al., 2016) thus, they are preferred over grass financially. In an analysis of strategies for integrated crop-sheep production systems Olaizola et al. (2015) show that land use integration provides resilience in prices fluctuation and greater financial stability for the farm business. Furthermore, studies have found that an additional positive effect emerges from land use diversification with grazing livestock, that is to support agri-tourism activities (Martin et al., 2016; Moraine et al., 2017).
In the optimisation scenarios, the total livestock units are reduced. Specifically, the optimisation solution increases sheep numbers and reduces beef cattle stocks as the former systems are more preferable from the financial perspective. On average, through all the scenarios, sheep numbers increase by 92% while beef cattle decrease by 92%. This result is in accordance to Acs et al. (2010) who note a structural change in optimised livestock production systems in which livestock numbers generally decrease while beef cattle production declines the most and sheep production is preferred especially on the areas with semi-natural flora or rock outcrops (e.g., upland moorland). One of the factors that constrained the number of livestock (for beef cattle enterprises) is the livestock housing capacity of farms. Specifically, 50% of the farm businesses that maintained beef cattle production after the optimisation method were constrained by this particular parameter. In other words, land abandonment is partially triggered due to fixed housing capacity.
As with all modelling studies, our findings are subject to some uncertainties and limitations. Limitations in the current approach emerged due to aggregated data in the FBS dataset for beef cattle and sheep enterprises. In particular, the FBS dataset disaggregates the livestock enterprises on the basis of different age groups such as store or fat cattle. However, through this classification, the live weight of the animals remains unknown. Thus, calculation of accurate feeding rations was not achievable. As a result, the study considered the feeding requirements of livestock covering only forage rather than including bought concentrates and homegrown silage cereals. Application of the latter would increase the accuracy of the estimations while assessing the cost effectiveness of crop production that contributes to the feed requirements of livestock (Dillon, 2007; Kilcline et al., 2014). In addition, this methodology studied the maximisation of one parameter only (AGM) without accounting for the multiple objectives that farmers might have relating to maintenance of environmental quality and cultural values of hill farming (DEFRA, 2010). Furthermore, systems transitions often require farmers adaptiveness to new knowledge and technologies while also include a certain degree of risk associated with returns on investment at harvest (Hochman et al., 2013) which can be a very important factor for profit maximisation (Monjardino et al., 2015).
Conclusions
This paper presents a method to investigate optimised integration of crop and livestock production systems (ICLS) for farm businesses in the LFAs of England examining a range of scenarios and integration options. The methodology incorporates the parameters of housing capacity, stocking densities, feed requirements, labour and land use. Through this analysis the farm organisation was reformed to maximise AGM under optimised utilisation of available resources and the spatial features of hill farms. The most significant impacts from structural changes were reflected in livestock numbers, livestock type, land use and fertiliser application.
Findings suggested that there is a potential for improving the input use efficiency therefore, the profitability of farming systems in the study area. Farm businesses with higher percentages of arable land within their farmed area have much higher gross margins than their counterparts with no arable land, as crop production is more profitable than livestock (Sneessens et al., 2016). This triggered increased levels of crop production (when arable land was available) which in terms of land use decreased areas of temporary grass and increased areas for cash crops such as cereals and beans. The latter highlights how the modelling identifies transition pathways towards the intensification of integrated crop and livestock production systems. Concerning livestock production, in all optimisation scenarios sheep enterprises were preferred over beef cattle from the perspective of profitability while livestock units in total remained the same under the PPO and PPM and decreased under PPA and AMP scenario.
A future step may expand this study towards enhancing the spatial analysis of these findings with the use of Geographic Information Systems (GIS). More specifically, this process may investigate the occurrence of spatial patterns or clusters of farm businesses with higher or lower potentials for profit maximisation. Furthermore, this examination may also seek spatial aggregations of the constraining factors (endowments). This will enable the identification of broad geographic blocks in which agricultural performance is more sensitive to existence of particular factors.
The focus of future policies for the development of the LFAs could be on promoting the integration of crop and livestock production systems, providing a range of structural alternatives for farmers to adopt towards future proofing their farm businesses and sustainably intensifying their production capacity. This suggestion however, highlights the importance of knowledge and information and thus, requires assisting farmers in getting access to such information. A potential policy tool to address this could be the establishment of knowledge transfer networks and social networks of farmers that will enable their collaboration with research institutes, scientists and advisory services (Asai et al., 2018). This will inform farmers about the positive effects of ICLS for their production systems and increase their willingness to change and adopt (Chalak et al., 2017). Furthermore training and technical assistance will be provided on agricultural production methods and farming practises that hill farmers may not have experience with (e.g., livestock farmers expanding to crop production) (Gil et al., 2016). The latter may also relate to lack of harvest equipment and high initial transaction costs (Asai et al., 2018) that can be accommodated by the implementation of machinery-sharing arrangements and joint ownership of equipment within established networks or farmer associations (Larsén, 2010). In that way, the principal factors affecting the adoption of integrated production systems will be narrowed down, thus providing solutions to encourage integration.
To ensure the future sustainability of hill farms it is necessary to both increase profitability but also to release natural resources that have not previously been used efficiently by the production systems. The modelling exercise developed herein suggested the release of grassland in favour of profitability of the production systems. This in average allows the restructure of land use for the provision of other land-based services and products. Thus, farming systems would have the opportunity to increase the provision of ecosystem services (carbon storage, biodiversity, habitat creation, water management, pollination, soil health etc.) on the spared land and support policy instruments for the provision of public goods from the agricultural sector.
Towards the direction of designing and evaluating scenarios that analyse the trade-offs between various integration options, simulation models can provide essential insights and point out acceptable interactions between ecosystem services and socioeconomic benefits (Martin et al., 2016; Ryschawy et al., 2017). Finally, in doing so the decision makers will be able to accommodate restrictions on productivity, emerging from natural constraints or availability of resources and thus mitigate the risks resulting from the challenges that agricultural production systems face in the LFAs.
Data Processing and Visualisation
Data preparation, analysis and estimations were carried out in R (R Core Team, 2014) using the package “dplyr” (Wickham et al., 2020), where visualisations and plots were created using the packages “ggplot2” (Wickham, 2016). Geographical visualisations of results and inputs were produced with ESRI ArcGIS 10.6.1 [Environmental Systems Research Institute (ESRI), 2018].
Data Availability Statement
The datasets presented in this article are not readily available because data is private and confidential. Access to data can be requested from the Farm Business Survey (UK). Requests to access the datasets should be directed to http://www.farmbusinesssurvey.co.uk.
Author Contributions
YV, YG, and SM contributed to conception and design of the study and wrote the sections of the manuscript. YV organised the datasets, performed the analysis of the model, and wrote the first draft of the manuscript. All authors contributed to manuscript revision, read, and approved the submitted version.
Funding
This study is part of a PhD project that has been funded partially from the University of Reading and partially from the Edith Mary Gayton bequest managed by the Farm Management Unit of the School of Agriculture, Policy and Development.
Conflict of Interest
The authors declare that the research was conducted in the absence of any commercial or financial relationships that could be construed as a potential conflict of interest.
Publisher's Note
All claims expressed in this article are solely those of the authors and do not necessarily represent those of their affiliated organizations, or those of the publisher, the editors and the reviewers. Any product that may be evaluated in this article, or claim that may be made by its manufacturer, is not guaranteed or endorsed by the publisher.
Footnotes
1. ^Gross Margin: Output from the enterprise less the Variable Costs, including the allocated variable costs of grass and other forage; Net Farm Income: It is equal to Gross Margin less Fixed costs that consist of Unpaid Labour, Rental Value, Imputed Rent on Tenants Improvements and adds to Ownership Charges, Net Interest Charges, and the Imputed rental value of the farmhouse.
2. ^Further information regarding the FBS dataset, methods of data collection or getting access can be found in the following link: https://www.gov.uk/government/collections/farm-business-survey.
3. ^No discrete classes of quality of permanent pasture exist within the method. Permanent pasture is generally located in the areas that face the most challenging conditions and have lower productivity. On the other hand, temporary grassland (based within arable land) corresponds to fairer conditions (topography and climate) and thus is more productive. This is why we classify permanent pasture as of poorer quality.
4. ^Here we use the per acre stocking density as this is the combination of unit and magnitude of the stocking density that has traditionally been considered across the study area (as derived by expert advice from an FBS lead).
References
Acs, S., Hanley, N., Dallimer, M., Gaston, K. J., Robertson, P., Wilson, P., et al. (2010). The effect of decoupling on marginal agricultural systems: implications for farm incomes, land use and upland ecology. Land Use Policy 27, 550–563. doi: 10.1016/j.landusepol.2009.07.009
Aerts, J. C. J. H., Eisinger, E., Heuvelink, G. B. M., and Stewart, T. J. (2003). Using linear integer programming for multi-site land-use allocation. Geogr. Anal. 35, 148–169. doi: 10.1111/j.1538-4632.2003.tb01106.x
Annetts, J. E., and Audsley, E. (2002). Multiple objective linear programming for environmental farm planning. J. Oper. Res. Soc. 53, 933–943. doi: 10.1057/palgrave.jors.2601404
Asai, M., Moraine, M., Ryschawy, J., de Wit, J., Hoshide, A. K., and Martin, G. (2018). Critical factors for crop-livestock integration beyond the farm level: a cross-analysis of worldwide case studies. Land Use Policy 73, 184–194. doi: 10.1016/j.landusepol.2017.12.010
Bell, L. W., Moore, A. D., and Kirkegaard, J. A. (2014). Evolution in crop–livestock integration systems that improve farm productivity and environmental performance in Australia. Eur. J. Agron. 57, 10–20. doi: 10.1016/j.eja.2013.04.007
Benoit, M., and Laignel, G. (2010). Energy consumption in mixed crop-sheep farming systems: what factors of variation and how to decrease? Animal 4, 1597–1605. doi: 10.1017/S1751731110000480
Bonaudo, T., Bendahan, A. B., Sabatier, R., Ryschawy, J., Bellon, S., Leger, F., et al. (2014). Agroecological principles for the redesign of integrated crop–livestock systems. Eur. J. Agron. 57, 43–51. doi: 10.1016/j.eja.2013.09.010
Chalak, A., Irani, A., Chaaban, J., Bashour, I., Seyfert, K., Smoot, K., et al. (2017). Farmers' Willingness to adopt conservation agriculture: new evidence from Lebanon. Environ. Manage. 60, 693–704. doi: 10.1007/s00267-017-0904-6
Darnhofer, I., Bellon, S., Dedieu, B., and Milestad, R. (2010). Adaptiveness to enhance the sustainability of farming systems. A review. Agron. Sustain. Dev. 30, 545–555. doi: 10.1051/agro/2009053
DEFRA (2010). Farming in the English Uplands (No. 20), Agricultural Change and Environment Observatory. London: Parliament UK Publications.
Dillon, P. (2007). Achieving high dry-matter intake from pasture with grazing dairy cows. Frontis 18, 1–26. doi: 10.1007/978-1-4020-5452-5_1
Duru, M., and Therond, O. (2015). Livestock system sustainability and resilience in intensive production zones: which form of ecological modernization? Reg. Environ. Change 15, 1651–1665. doi: 10.1007/s10113-014-0722-9
European Commission (2005). Rural Development policy 2007-2013 : Aid to farmers in Less Favoured Areas (LFA). Available online at: https://ec.europa.eu/info/food-farming-fisheries (accessed November 14, 2016).
European Commission (2006). LFA Aid to farmers in Less Favoured Areas. Available online at: http://ec.europa.eu/agriculture/rurdev/lfa/index_en.htm (accessed November 14, 2016).
European Commission (2010). The CAP Towards 2020: Meeting the Food, Natural Resources and Territorial Challenges of the Future.
Gadanakis, Y., Bennett, R., Park, J., and Areal, F. J. (2015). Evaluating the sustainable intensification of arable farms. J. Environ. Manag. 150, 288–298. doi: 10.1016/j.jenvman.2014.10.005
Gameiro, A. H., Rocco, C. D., and Caixeta Filho, J. V. (2016). Linear programming in the economic estimate of livestock-crop integration: application to a Brazilian dairy farm. Rev. Bras. Zootec. 45, 181–189. doi: 10.1590/S1806-92902016000400006
Garrett, R. D., Niles, M., Gil, J., Dy, P., Reis, J., and Valentim, J. (2017). Policies for reintegrating crop and livestock systems: a comparative analysis. Sustainability 9:473. doi: 10.3390/su9030473
Gil, J., Siebold, M., and Berger, T. (2015). Adoption and development of integrated crop–livestock–forestry systems in Mato Grosso, Brazil. Agric. Ecosyst. Environ. 199, 394–406. doi: 10.1016/j.agee.2014.10.008
Gil, J. D. B., Garrett, R., and Berger, T. (2016). Determinants of crop-livestock integration in Brazil: evidence from the household and regional levels. Land Use Policy 59, 557–568. doi: 10.1016/j.landusepol.2016.09.022
Glen, J. J. (1986). A linear programming model for an integrated crop and intensive beef production enterprise. J. Oper. Res. Soc. 37:487. doi: 10.2307/2582671
Harvey, D., and Scott, C. (2015). Farm Business Study 2013/2014: Hill farming in England. Newcastle: Rural Business Research.
Hazell, P. B. R., and Norton, R. D. (1989). Mathematical programming for economic analysis in agriculture. Biom. J. 31, 930–930. doi: 10.1002/bimj.4710310805
Hendrickson, J. R., Hanson, J. D., Tanaka, D. L., and Sassenrath, G. (2008). Principles of integrated agricultural systems: introduction to processes and definition. Renew. Agric. Food Syst. 23, 265–271. doi: 10.1017/S1742170507001718
Herrero, M., Thornton, P. K., Notenbaert, A. M., Wood, S., Msangi, S., Freeman, H. A., et al. (2010). Smart investments in sustainable food production: revisiting mixed crop-livestock systems. Science 327, 822–825. doi: 10.1126/science.1183725
Hochman, Z., Carberry, P. S., Robertson, M. J., Gaydon, D. S., Bell, L. W., and McIntosh, P. C. (2013). Prospects for ecological intensification of Australian agriculture. Eur. J. Agron. 44, 109–123. doi: 10.1016/j.eja.2011.11.003
Hosu, S., and Mushunje, A. (2013). Optimizing resource use and economics of crop-livestock integration among small farmers in semiarid regions of South Africa. Agroecol. Sustain. Food Syst. 37, 985–1000. doi: 10.1080/21683565.2013.802755
Jansen, G. B., and Wilton, J. W. (1984). Linear programming in selection of livestock. J. Dairy Sci. 67, 897–901. doi: 10.3168/jds.S0022-0302(84)81385-5
Kaiser, H. M., and Messer, K. D. (2011). Mathematical Programming for Agricultural, Environmental, and Resource Economics. Hoboken, NJ: Wiley.
Kilcline, K., O'Donoghue, C., Hennessy, T., and Hynes, S. (2014). Economic factors affecting concentrate usage on Irish sheep farms. Int. J. Agric. Manag. 3, 243–252. doi: 10.22004/ag.econ.236917
Larsén, K. (2010). Effects of machinery-sharing arrangements on farm efficiency: evidence from Sweden. Agric. Econ. 41, 497–506. doi: 10.1111/j.1574-0862.2010.00461.x
Lemaire, G., Franzluebbers, A., Carvalho, P. C. de F., and Dedieu, B. (2014). Integrated crop–livestock systems: strategies to achieve synergy between agricultural production and environmental quality. Agric. Ecosyst. Environ. 190, 4–8. doi: 10.1016/j.agee.2013.08.009
Martin, G., Moraine, M., Ryschawy, J., Magne, M.-A., Asai, M., Sarthou, J. P., et al. (2016). Crop–livestock integration beyond the farm level: a review. Agron. Sustain. Dev. 36:53. doi: 10.1007/s13593-016-0390-x
Memmah, M. M., Lescourret, F., Yao, X., and Lavigne, C. (2015). Metaheuristics for agricultural land use optimization. A review. Agron. Sustain. Dev. 35, 975–998. doi: 10.1007/s13593-015-0303-4
Monjardino, M., McBeath, T., Ouzman, J., Llewellyn, R., and Jones, B. (2015). Farmer risk-aversion limits closure of yield and profit gaps: A study of nitrogen management in the southern Australian wheatbelt. Agric. Syst. 137, 108–118. doi: 10.1016/j.agsy.2015.04.006
Moraine, M., Duru, M., and Therond, O. (2017). A social-ecological framework for analyzing and designing integrated crop–livestock systems from farm to territory levels. Renew. Agric. Food Syst. 32, 43–56. doi: 10.1017/S1742170515000526
Nix, J., and Redman, G. (2016). Farm Management Pocketbook, 47th Edn. Leicestershire: Agro Business Consultants Ltd.
Olaizola, A. M., Ameen, F., and Manrique, E. (2015). Potential strategies of adaptation of mixed sheep-crop systems to changes in the economic environment in a Mediterranean mountain area. Livest. Sci. 176, 166–180. doi: 10.1016/j.livsci.2015.03.012
Perrot, C., Caillaud, D., and Chambaut, H. (2012). Économies d'échelle et économies de gamme en production laitière. Analyse technico-économique et environnementale des exploitations de polyculture-élevage françaises. Rencontres Autour Rech. Sur Rumin. 33–36. Available online at: https://agriculture.gouv.fr/economies-dechelle-et-economies-de-gamme-en-production-laitiere-analyse-technico-economique-et
Peyraud, J.-L., Taboada, M., and Delaby, L. (2014). Integrated crop and livestock systems in Western Europe and South America: a review. Eur. J. Agron. 57, 31–42. doi: 10.1016/j.eja.2014.02.005
Poffenbarger, H., Artz, G., Dahlke, G., Edwards, W., Hanna, M., Russell, J., et al. (2017). An economic analysis of integrated crop-livestock systems in Iowa, U.S.A. Agric. Syst. 157, 51–69. doi: 10.1016/j.agsy.2017.07.001
R Core Team. (2014). R: A Language and Environment for Statistical Computing. Vienna: R Foundation for Statistical Computing. Available online at: https://www.R-project.org/
Reganold, J. P., Andrews, P. K., Reeve, J. R., Carpenter-Boggs, L., Schadt, C. W., Alldredge, J. R., et al. (2010). Fruit and soil quality of organic and conventional strawberry agroecosystems. PLoS ONE 5:e12346. doi: 10.1371/journal.pone.0012346
Ripoll-Bosch, R., Díez-Unquera, B., Ruiz, R., Villalba, D., Molina, E., Joy, M., et al. (2012). An integrated sustainability assessment of mediterranean sheep farms with different degrees of intensification. Agric. Syst. 105, 46–56. doi: 10.1016/j.agsy.2011.10.003
Ryschawy, J., Choisis, N., Choisis, J. P., and Gibon, A. (2013). Paths to last in mixed crop–livestock farming: lessons from an assessment of farm trajectories of change. Animal 7, 673–681. doi: 10.1017/S1751731112002091
Ryschawy, J., Martin, G., Moraine, M., Duru, M., and Therond, O. (2017). Designing crop–livestock integration at different levels: toward new agroecological models? Nutr. Cycl. Agroecosystems 108, 5–20. doi: 10.1007/s10705-016-9815-9
Sanderson, M. A., Archer, D., Hendrickson, J., Kronberg, S., Liebig, M., Nichols, K., et al. (2013). Diversification and ecosystem services for conservation agriculture: outcomes from pastures and integrated crop–livestock systems. Renew. Agric. Food Syst. 28, 129–144. doi: 10.1017/S1742170512000312
Schiere, J. B., Ibrahim, M. N. M., and Van Keulen, H. (2002). The role of livestock for sustainability in mixed farming: criteria and scenario studies under varying resource allocation. Agric. Ecosyst. Environ. 90, 139–153. doi: 10.1016/S0167-8809(01)00176-1
Scotland's Farm Advisory Service (2016). Farm Management Handbook, 37th Edn. Edinburgh: Farm Advisory Service, SAC Consulting.
Sneessens, I., Veysset, P., Benoit, M., Lamadon, A., and Brunschwig, G. (2016). Direct and indirect impacts of crop-livestock organization on mixed crop-livestock systems sustainability: a model-based study. Animal 10, 1911–1922. doi: 10.1017/S1751731116000720
Soussana, J. F., and Lemaire, G. (2014). Coupling carbon and nitrogen cycles for environmentally sustainable intensification of grasslands and crop-livestock systems. Agric. Ecosyst. Environ. 190, 9–17. doi: 10.1016/j.agee.2013.10.012
Tichit, M., Puillet, L., Sabatier, R., and Teillard, F. (2011). Multicriteria performance and sustainability in livestock farming systems: functional diversity matters. Livest. Sci. 139, 161–171. doi: 10.1016/j.livsci.2011.03.006
Van Zanten, H. H. E., Van Ittersum, M. K., and De Boer, I. J. M. (2019). The role of farm animals in a circular food system. Glob. Food Sec. 21, 18–22. doi: 10.1016/j.gfs.2019.06.003
Veysset, P., Bebin, D., and Lherm, M. (2005). Adaptation to Agenda 2000 (CAP reform) and optimisation of the farming system of French suckler cattle farms in the Charolais area: a model-based study. Agric. Syst. 83, 179–202. doi: 10.1016/j.agsy.2004.03.006
Wickham, H. (2016). ggplot2: Elegant Graphics for Data Analysis. New York, NY: Springer-Verl. doi: 10.1007/978-3-319-24277-4
Wickham, H., François, R., Henry, L., Müller, K., and RStudio (2020). dplyr: A Grammar of Data Manipulation.
Keywords: hill farming systems, livestock, optimisation, spatial reallocation of resources, integrated crop livestock system
Citation: Vittis Y, Gadanakis Y and Mortimer S (2021) Optimising the Spatial and Production Input Features to Improve Efficiency of Hill Farm Production Systems. Front. Sustain. Food Syst. 5:730614. doi: 10.3389/fsufs.2021.730614
Received: 25 June 2021; Accepted: 22 September 2021;
Published: 25 October 2021.
Edited by:
Valerien Pede, International Rice Research Institute (IRRI), PhilippinesReviewed by:
Laura Riesgo, Pablo de Olavide University, SpainPaul Wilson, University of Nottingham, United Kingdom
Copyright © 2021 Vittis, Gadanakis and Mortimer. This is an open-access article distributed under the terms of the Creative Commons Attribution License (CC BY). The use, distribution or reproduction in other forums is permitted, provided the original author(s) and the copyright owner(s) are credited and that the original publication in this journal is cited, in accordance with accepted academic practice. No use, distribution or reproduction is permitted which does not comply with these terms.
*Correspondence: Yiorgos Gadanakis, Zy5nYWRhbmFraXNAcmVhZGluZy5hYy51aw==