- 1International Institute of Tropical Agriculture, NRM, Arusha, Tanzania
- 2Wageningen University and Research, Farm Systems Ecology Group, Wageningen, Netherlands
- 3The World Vegetable Center (AVRDC), Arusha, Tanzania
Land suitability assessment matches crop requirements with available resources to promote sustainable production. Scaling out of sustainable agricultural intensification practices to suitable biophysical and socio-economic conditions reduces the risk of failure and increases their adoption rate. This study applies a geospatial framework to identify potentially suitable sites for extrapolating two improved vegetable cultivars grown under integrated management practices (IMP's) in Babati District of Tanzania. On-farm trial data on the yield and income of two cultivars were used as a reference. Extrapolation was based on the gridded biophysical and socio-economic layers that limit the production of the two improved vegetable cultivars'. The extrapolation suitability index (ESI) showed the areas where cultivation of the two improved vegetable cultivars under IMP's can be scaled-out with a potentially low risk of failure. We generate maps of the most important limiting factor for each cultivar in every pixel to guide the spatial targeting of appropriate remedial measures. Application of these maps will promote evidence-based scaling out of improved vegetable technologies by the extension and development agencies.
1. Introduction
Vegetables are an essential dietary component as most are rich in micro-nutrients such as iron, Zinc, Magnesium, vitamins A and C (Ojiewo et al., 2010). These micro-nutrients play a vital role in human growth, development, and health. 90% of the population with Ca and Zn deficiency is in Africa and Asia (Kumssa et al., 2015). Efforts to increase the sustainable production and consumption of vegetables will significantly address malnutrition and hidden hunger. Moreover, vegetable farming promotes income diversification in small-scale farming systems in sub-Saharan Africa, thereby improving livelihoods.
The level of crop production is context-dependent. Recent research has shown that scaling out sustainable agricultural intensification technologies to locations with biophysical and socio-economic conditions similar to their trial sites increases their adoption rate and reduces the risk of failure (Annicchiarico et al., 2005; Rubiano et al., 2016). Yields attained at the reference agronomic trials can be achieved in other areas with similar biophysical and socio-economic conditions. Land suitability assessment is applied to identify locations with similar conditions to reference trial sites. Land suitability assessment promotes design of better land management by supporting land use pattern that prevents or avoids environmental degradation through the segregation of competing land uses (AbdelRahman et al., 2016; Han et al., 2021). Land suitability assessment improves knowledge on the appropriateness of specific land use in a location and identifies the limitation(s) hindering land utilization. Mapping the suitability of crops to the prevailing biophysical and socio-economic context informs evidence-based agricultural investments and promotes the sustainable use of limited resources. Characterizing the biophysical context under which technologies performed successfully is vital for planning to scale out investments.
Geospatial data and tools are applied to generate recommendation domains for sustainable agricultural intensification technologies as they offer the speed, flexibility, and power to synthesize big data on land resources (Hyman et al., 2013; Usha and Singh, 2013; Muthoni et al., 2019). Spatial frameworks for mapping crop suitability follow a ‘top-down' or a ‘bottom-up' approach. The top-down approach utilizes a multi-criteria analysis to classify remote sensing data into clusters based on predetermined criteria or expert knowledge but without direct reference to the on-farm trial sites (e.g., Tesfaye et al., 2015; Muthoni et al., 2017). The bottom-up approach utilizes data from field trials as the benchmark of the suitable environmental conditions for agronomic technology, followed by a spatial search of other areas with a similar context in the wider geographical area (Rubiano et al., 2016; Muthoni et al., 2019). The top-down approach is the most applied to generate the spatial recommendation domains for agronomic technologies (Tesfaye et al., 2015; Notenbaert et al., 2016), primarily due to the increasing free availability of gridded biophysical and socio-economic layers.
The bottom-up approach is not applied widely because of the limited data availability from on-farm trials. Recent studies have shown the potential of the bottom-up approach for developing extrapolation domains of bundles of technology options using available on-farm trial data (Rubiano et al., 2016; Muthoni et al., 2019). The “bottom-up” approach is more specific because recommendations are generated from on-farm trials after validation in multiple locations or seasons. Muthoni et al. (2019) developed the extrapolation suitability index (ESI) for maize varieties and mineral fertilizers in Tanzania. They combined data from agronomic trials and remote sensing layers to generate maps on the suitability of technology packages beyond the trial sites to guide scaling-out operations. The ESI method apply an innovative extrapolation detection algorithm (Mesgaran et al., 2014) that factors the dissimilarity from the reference trial sites while also accounting for novel combination of environmental variables beyond those encountered in the trials. Rubiano et al. (2016) mapped the global out-scaling of water-efficient rice technologies using data from 220 pilot sites. Annicchiarico et al. (2005) showed that scaling out Algerian durum wheat (Triticum durum Desf.) on GIS-generated recommendation domains had an 11% yield advantage.
There are few spatially explicit recommendations domains for vegetable cultivars worldwide. For example, Jayasinghe and Machida (2008) developed a web-GIS tool utilizing soil, topography, climate, and land use data to map land suitability for tomatoes and cabbages in Sri Lanka. In Nepal, Thapa et al. (2020) mapped the suitability of cauliflower, and Baniya et al. (2009) developed spatial recommendations for the larger cardamom (Amomum subulatum Roxb.). Ji et al. (2018) mapped the the spatial-temporal variability of vegetable production in China. Mostafiz et al. (2021) applied a fuzzy membership method to map suitability of vegetables between different seasons in Bangladesh. Other studies had applied a top-down multi-criteria decision making to map suitability of vegetables without on-farm trial data (Mugo et al., 2016; Mostafiz et al., 2021; Rahmawaty et al., 2021; Yuniarti et al., 2022; Zakaria et al., 2022). Although multiple agencies in Tanzania promote sustainable vegetable farming at scale, detailed maps on the suitability of improved cultivars and related improved agronomic practices are lacking.
A recent study in Babati district of Tanzania analyzed on-farm trials data to identify promising vegetable cultivars and related management practices (Lukumay et al., 2018). The study compared the yield response and economic benefits of improved tomato (Lycopersicon esculentum; “Tengeru 2010”) and African eggplant (Solanum aethiopicum; “Tengeru White:) cultivars grown under improved management practices (IMPs) and standard farmer' practices (SFP's; control) (Lukumay et al., 2018). The IMPs comprised good quality seeds of improved cultivars, healthy seedlings, good agronomic practices (GAP's), and integrated pest management (IPM). The control plots used good-quality seeds and SFPs. Results from these on-farm trials revealed that growing the two cultivars using IMPs significantly increased yield for Tengeru 2010 tomato from 28 t ha−1 (control) to 64 t ha−1 and from 23.04 t ha−1 to 54 t ha−1 for African eggplant. Lukumay et al. (2018) further demonstrated that farmers who applied IMP's attained gross profit margins up to USD 18,300 and 9,600 ha−1 with benefit-cost ratios (BCR) of 8.5 and 4.5 for tomato and African eggplant, respectively. Evidently, the adoption of IMP in vegetable farming at scale could confer a broader societal impact in terms of yield and profits.
The question that emerged after the on-farm validation trials was where else should the package of two cultivars and IMP's be scaled-out with minimal risk of failure in Babati district of Tanzania? This study addresses the above question by conducting a spatially explicit characterization of biophysical and socio-economic contexts that match the trial sites where the improved vegetable technology packages returned significantly higher yield and income. We hypothesized that technological packages that show high yield potential in reference trial sites would also perform equally well in outlying areas with similar environmental conditions. The objective of our study is to produce a map of each cultivar's extrapolation suitability index (ESI) to identify areas where specific technological packages can be scaled-out with a potentially low risk of failure. Moreover, we generate a map showing the most important covariates (MIC) that highly limits each cultivar's suitability at every grid cell to guide the spatial targeting of appropriate remedial measures. The MIC analysis provides knowledge for localized targeting of management practices to improve the suitability of specific technologies. The spatially explicit framework presented in this paper will guide evidence-based land use planning for sustainable agricultural development in smallholder farming systems.
2. Materials and methods
2.1. Study area
The study area islocated in Babati district of Tanzania (Figure 1). Maize-legume intercropping is the main farming system in the Babati district, but vegetables are grown to supplement income and nutrition. Livestock keeping mostly under zero-grazing systems is common and provides milk and farmyard manure. This study focuses on two vegetable cultivars; the tomato (Lycopersicon esculentum; “Tengeru 2010”) and the African eggplant (Solanum aethiopicum; “Tengeru White”). The “Tengeru 2010” tomato is rated excellent for taste and high market acceptability and tolerate early blight, late blight, and tomato mosaic virus (Ojiewo et al., 2010; Minja et al., 2011). The “Tengeru White” African eggplant requires less water than tomatoes and prefers sunny conditions. It grows on a wide range of soils, including sandy loams but does not cope with waterlogging and intense shading. African eggplant is considered moderately sensitive to salinity and tolerant to acidity.
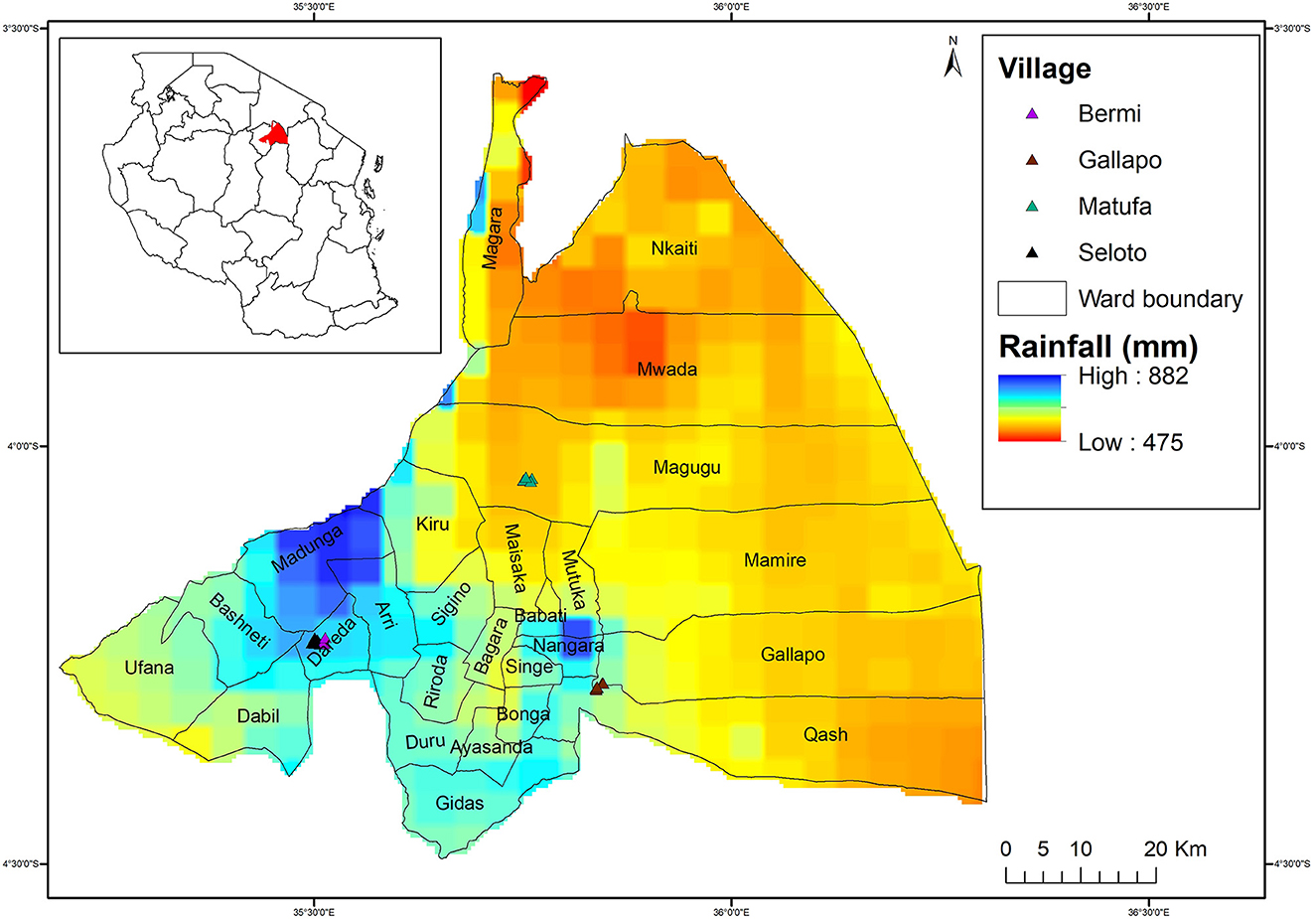
Figure 1. Location of Babati district and on-farm trial sites superimposed on the annual total rainfall obtained from TerraClimate database. This study utilizes knowledge gained from twenty-three (n = 23) on-farm trials conducted for two growing seasons (2013/14 and 2014/15).
The on-farm trials were implemented to validate the suitability of baskets of technologies comprising an elite tomato (“Tengeru 2010”) and African eggplant (“Tengeru White”) grown under IMP's in the Babati District of Tanzania. The on-farm trials in a randomized complete block experimental design were implemented in four villages (Figure 1) characterized by high (Bermi, Seloto), medium (Gallapo), and low (Matufa) rainfall zones (Lukumay et al., 2018). The trial sites were selected based on agroecological potential (rainfall and altitude), population density, and market access under the Africa RISING program (https://africa-rising.net/). The results from the on-farm trials compared the yield and net benefits (profit) between the standard farmer's practice (control) and the integrated management practice (IMP) for the two cultivars. Lukumay et al. (2018) showed that the yield advantage and income for the IMP treatments of the two cultivars were more than double compared to the control (Figure 2). The IMPs package emerged as the best-bet for eggplant and tomato. The IMPs package comprised good quality elite seeds of improved vegetable cultivars, healthy seedlings, good agronomic practices (GAPs) and integrated pest management (IPM). GAPs included the application of manure, mulching, proper spacing, stalking, irrigation, and weeding. For tomato and eggplant, respectively, the IMP package produced a yield of 2.3 times higher and increased income by 1.6 compared to standard farmers' practice (Figure 2).
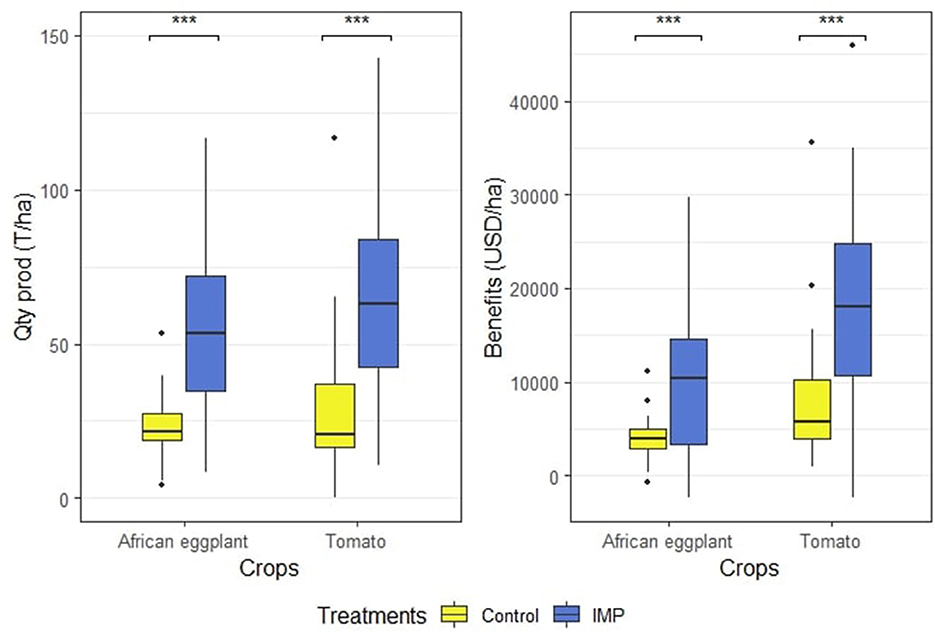
Figure 2. The yield and net benefits (income) for African eggplant (Tengeru White) and tomato (Tengeru 10) cultivars grown under standard farmers practice (control) and integrated management practices (IMP) in the Babati district of Tanzania.
2.2. Gridded biophysical and socio-economic datasets
We obtained a set of gridded biophysical and socio-economic layers that influence the suitability of the two vegetable cultivars from various sources (Table 1). The gridded datasets had different spatial resolutions ranging from 30 m (elevation) and 4 Km (climate). All gridded data was resampled to 30 meters resolution to avoid loss of high-resolution topographic details (Figure 3). The grid and vector layers representing the administrative boundaries were pre-processed, analyzed and visualized with the open-source R programming environment (R Core Team, 2023). The raster (Hijmans, 2023) and rasterVis (Lamigueiro and Hijmans, 2019) packages were mainly used for spatial analysis and visualization, respectively.
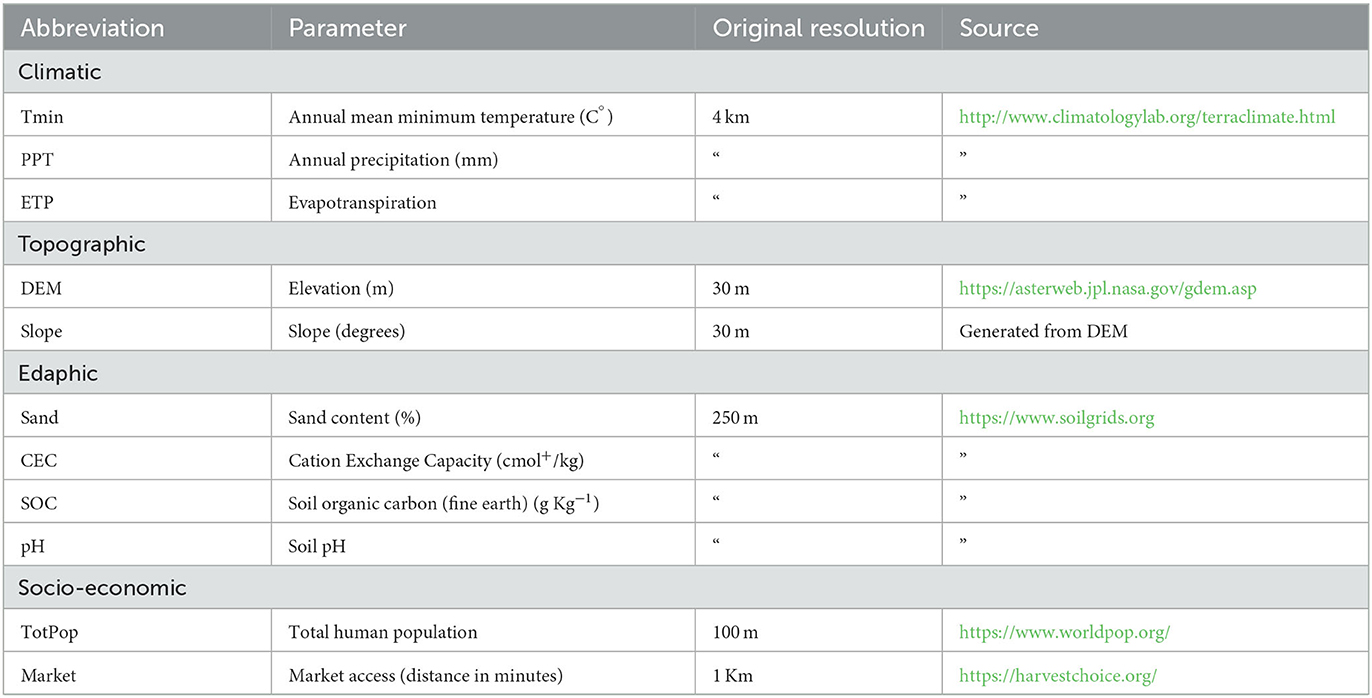
Table 1. Input variables for delineating extrapolation suitability index for vegetable technologies.
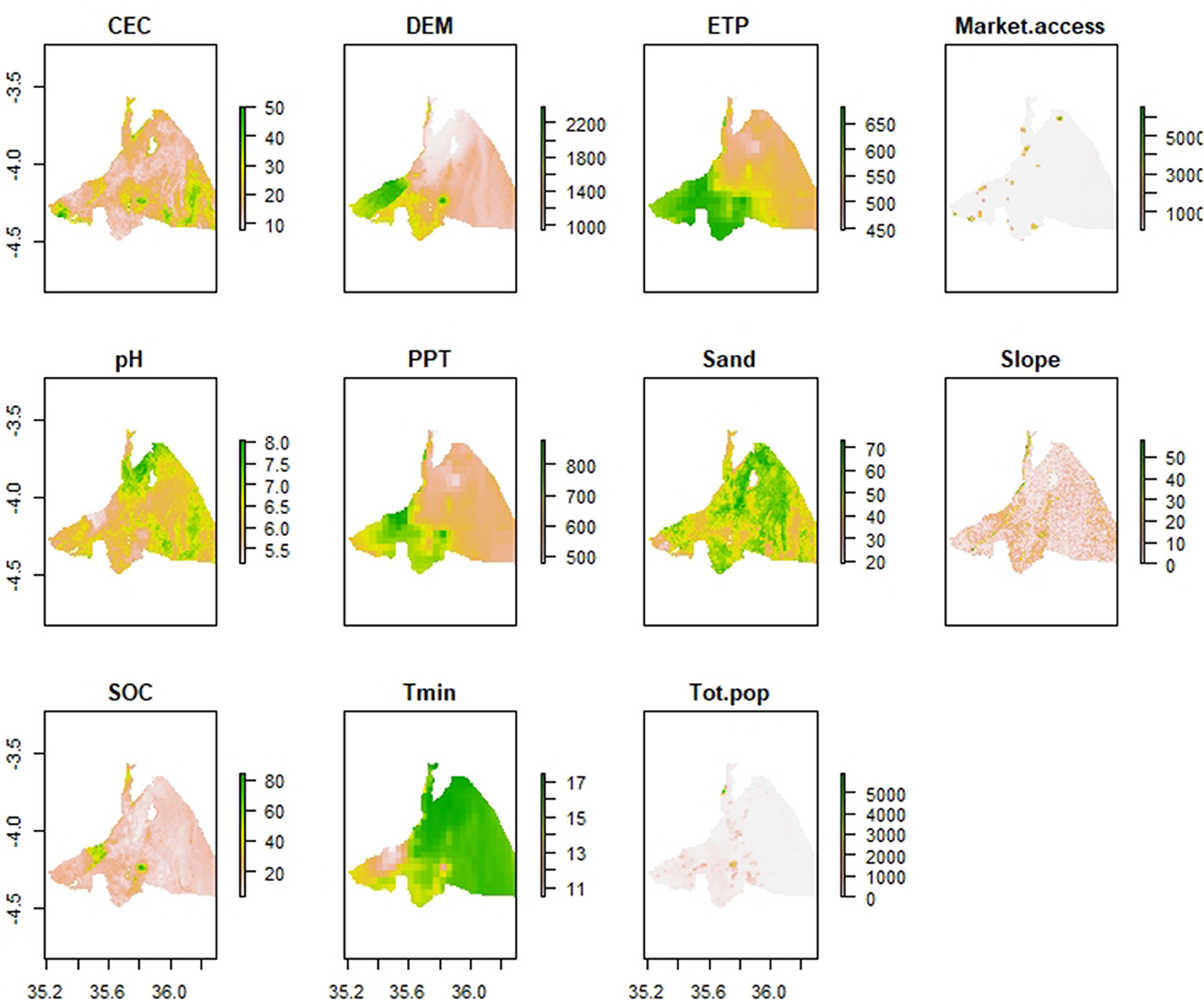
Figure 3. The input remote sensing layers representing the biophysical and socio-economic environment in Babati district. The abbreviations of the name are listed in Table 1.
2.3. Statistical analysis
2.3.1. Site characterization
Data collected from on-farm trials were used to generate a map of the extrapolation suitability index (ESI) of a tomato (“Tengeru 2010”) and the African eggplant (“Tengeru White”) grown using IMP. Compared to SFPs, the treatments with IMP had a higher yield advantage and incomes. Therefore, the trial plots for IMP for both cultivars were selected as the reference sites when delineating the extrapolating suitability maps. A principal components analysis (PCA) was performed on a data frame containing the gridded environmental layers listed in Table 2 to identify the primary gradient associated with each trial site. The optimal number of clusters for environmental variables was determined using “NbClust” R package (Charrad et al., 2014). The package provides 30 indices for assessing the number of clusters. It proposes the best clustering scheme to the user from different results obtained by varying combinations of clusters, distance measures, and clustering methods. In our case, we evaluated the complete method with Euclidean distance for cluster sizes ranging from 2 to 15. Hierarchical clustering on principal components (HCPC) function of “FactorMinoR” R package (Lê et al., 2008) was utilized to group the trial sites into an optimal number of clusters with relatively similar environmental conditions. The cluster of trial sites' that each cultivar showed the highest mean yield and net-benefits was selected as a reference for extrapolation. A 30 m buffer around the location of best-performing trials was generated and used to crop the reference environmental layers. However, different criteria for selecting the best-bet trial sites can be specified: i.e., the yield stability, longevity of production, and quality of seeds; depending on the production objective.
2.3.2. Generating extrapolation suitability index
The method proposed by Muthoni et al. (2019) was applied to generate the ESI maps of the two vegetable cultivars grown under IMP. The ESI method utilize data from agronomic trials and remote sensing layers to generate maps on the suitability of particular technology package beyond the trial sites. The input variables were the points around the best-performing trial sites for each two cultivar in Babati and 11 gridded biophysical and socio-economic variables or their reasonable proxies that are known to affect growing and marketability of vegetable cultivars (Table 1). The trial sites where particular vegetable cultivar showed good performance were utilized as reference training sites. The ESI maps were generated by calculating environmental dissimilarity between the reference trial sites and the outlying extrapolation area targeted for scaling out operations (Figure 1). This was accomplished by calculating novelty type-1 using the univariate extrapolation function in “ntbox” R package (Osorio-Olvera et al., 2020). The novelty type-1 ranges from 0 to negative infinity, with zero value representing the area that perfectly matches trial site conditions and, therefore, is the most suitable for scaling out. The most suitable zone in the projection domain that should be prioritized for scaling-out a particular agronomic technology package. This is the zone exhibiting the lowest univariate environmental dissimilarity compared to the conditions in the reference trial sites (novelty type-1 is equal or close to zero). The map of the novelty type-1 indicates the magnitude at which the environmental conditions at any particular location in the projection domains fall outside the range of values observed in the reference sites (Mesgaran et al., 2014). The most limiting covariate (MIC) was mapped using the ExeDet tool (Mesgaran et al., 2014). The most limiting factor at every location is the most extreme univariate value (minimum/maximum) compared to the reference sites' optimal conditions (Mesgaran et al., 2014).
3. Results
Three optimal clusters of environments were discriminated from the trial sites (Figure 4). The first two PCA axes explained 61.3% and 23.5% of the variance in environmental conditions, respectively. Cluster 1 represented trials in Matufa village characterized by lower agricultural potential due to warmer temperatures (high Tmin), sandy and alkaline soils (Figure 4). Cluster 2 had the highest trial plots (n = 12) located in Bermi and Seloto villages. The two villages have a high potential for agriculture as revealed by high elevation (DEM), precipitation (PPT), soil organic carbon (SOC), dense population (TotPop), and steeper slope; (Figure 4). Trials in cluster 3 were more correlated with the second PCA axis and represented trials in Galapo village characterized by high CEC and longer distance to the market.
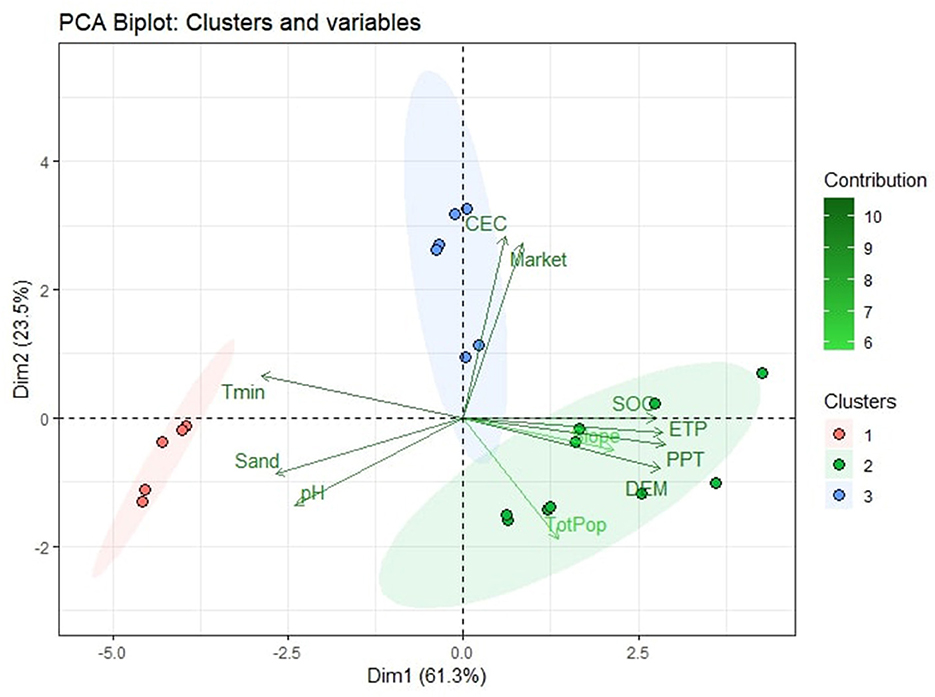
Figure 4. Characterization of the environmental conditions in the vegetable trials. Cluster 1 represents trials in Matufa village, cluster 2 are Bermi and Seloto and cluster 3 is Galapo. Trials in cluster 1 (Matufa village) and 3 (Galapo) showed the highest median yield (Qty Prod) and net benefit for the African eggplant and tomato, respectively (Figure 5). Therefore, trial plots located in the two best-performing clusters (1 and 3) were selected as the reference sites when delineating the extrapolating suitability maps for the two cultivars.
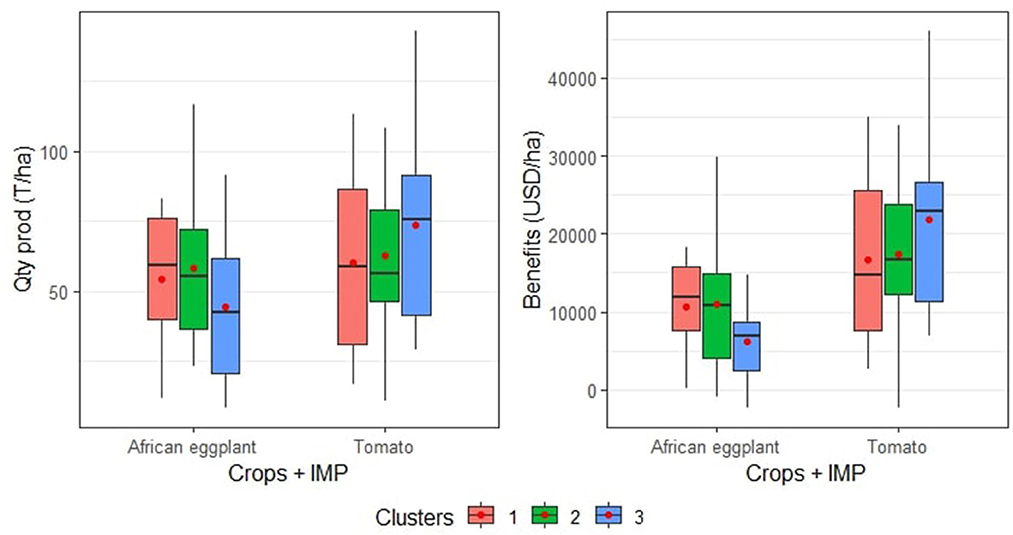
Figure 5. The yield (Qty prod) and net benefit variability for African eggplant (Tengeru White) and the tomato (Tengeru 2010) among the three environmental clusters. The red dots represent the mean of yield and benefits. Clusters 1 and 3 showed the highest yield and net benefit for African eggplant and Tengeru 2010 tomato, respectively, and were used as the reference for spatial extrapolation.
The ESI maps revealed that the Tengeru 2010 tomato is widely adapted but more suitable in the southern-central area of the Babati district (Figure 6A). The African eggplant was particularly unsuitable in the humid highlands in the southwestern of the district (Figure 6B). The central area around Matufa village was the most suitable for Tengeru White eggplant.
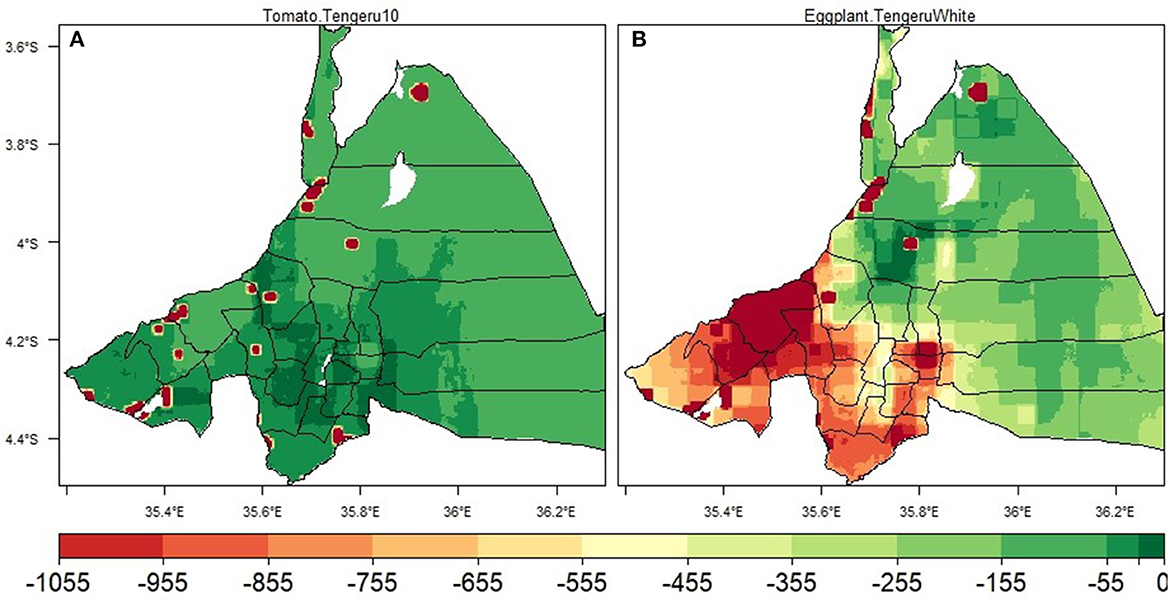
Figure 6. The extrapolation suitability index (ESI) for (A) tomato (Tengeru 2010) and (B) African eggplant (Tengeru White). ESI show the risk of scaling out vegetable cultivars grown under integrated management practices (IMP).
Tengeru 2010 tomato cultivar's suitability in over 80% of the Babati district was limited by evapotranspiration (ETP; Figure 7A). Figure 8A shows that the median ETP (545 mm) in the projection domain was lower than the reference trial sites (618 mm). The DEM was the MIC covering the second largest area for Tengeru 2010 cultivar (Figure 7A); the median elevation in this area was higher (1,800 m) compared to the reference trial sites at 1,300 m above sea level (Figure 8B). The Tengeru White eggplant was primarily limited by precipitation (PPT) in over 70% of the district and the market access in the rest of the area located in the east to southeast of the Babati district (Figure 7B). Figure 8C shows that the median PPT (620 mm) was higher and more variable than the reference trial sites (580 mm). The south-east section of the district showed very low market access due to the longer time taken to reach the nearest market (Figure 8D).
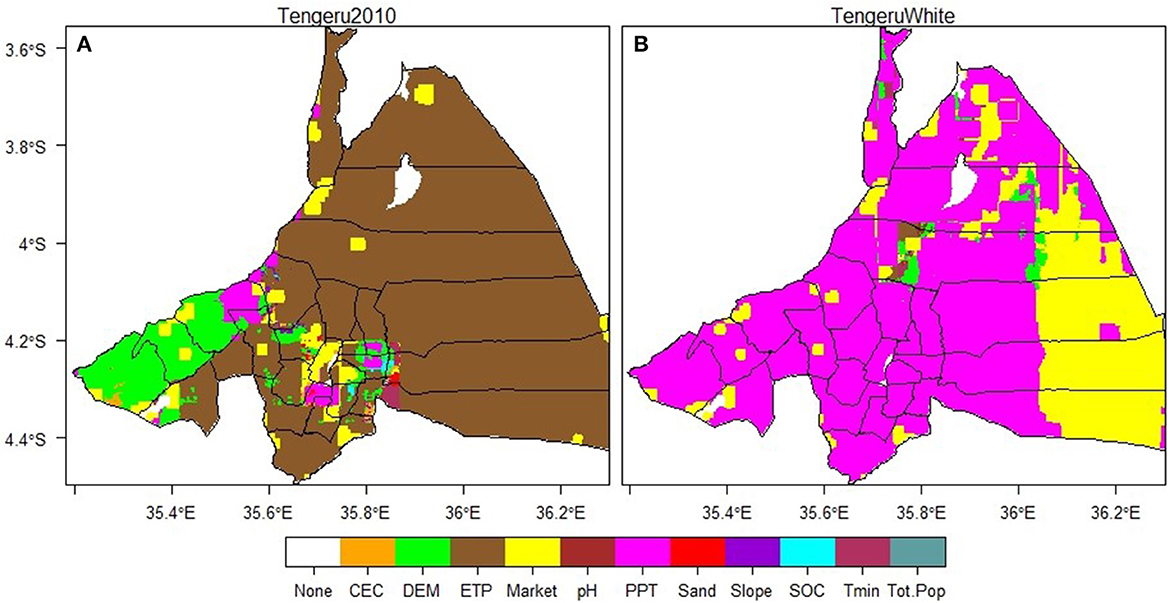
Figure 7. The spatial variation of the most important covariates (MIC) for two vegetable cultivars grown under improved management practices in Babati district. The zone where none of the covariates was identified as limiting represents vegetable cultivars' reference trial sites.
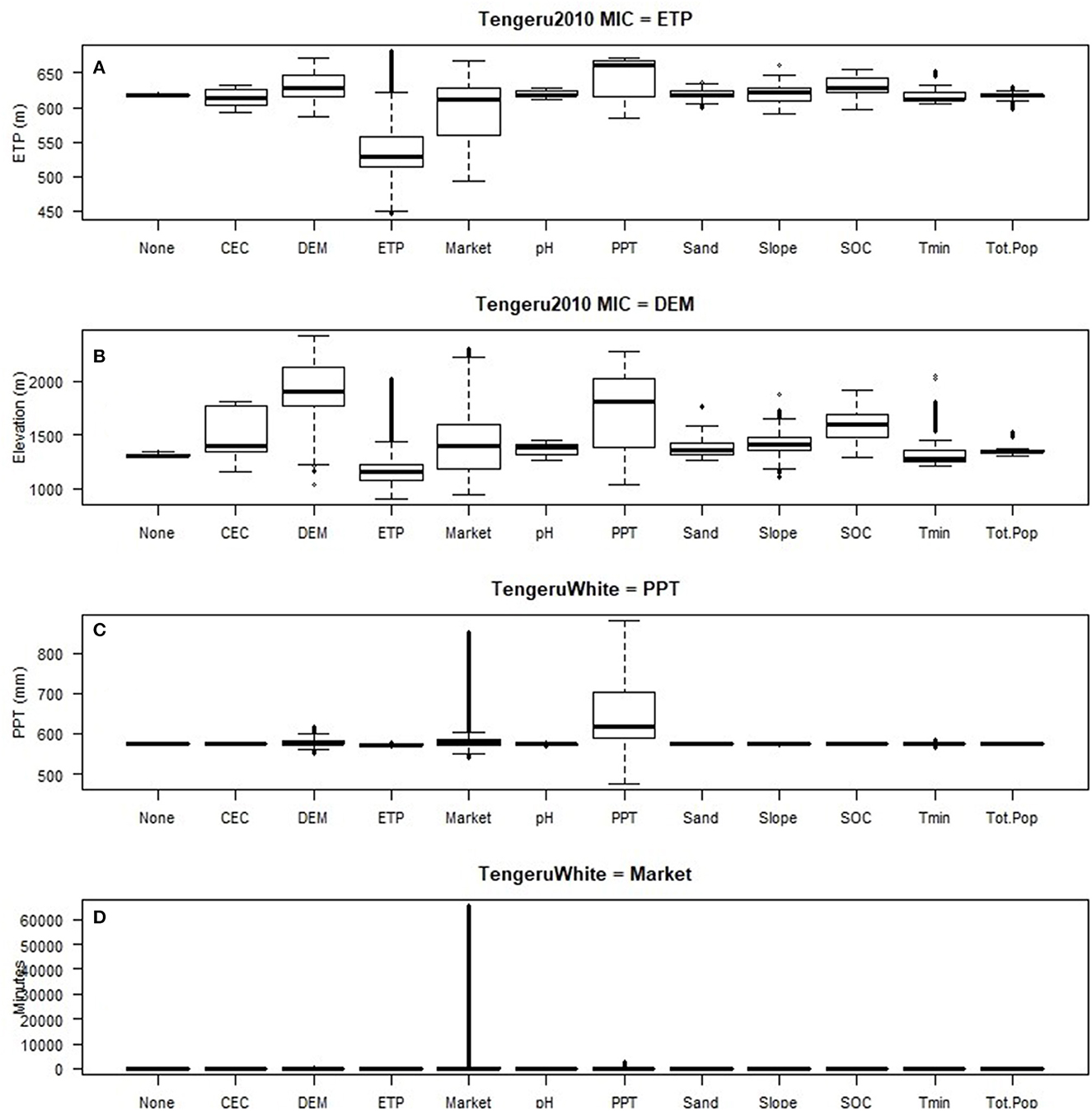
Figure 8. Boxplot of some of the most influential covariates (MIC) for the two vegetable cultivars in different Babati district zones. The MIC's for Tengeru 2010 are (A) evapotranspiration (ETP) and (B) elevation (DEM). MIC for Tengeru White eggplant are (C) precipitation (PPT) and (D) market access. The zone where none of the covariate was limiting represented the reference trial sites.
4. Discussions
Maps on the extrapolation suitability index (ESI) were developed to guide evidence-based spatial targeting of two improved vegetable cultivars grown under IMP's in Babati district of Tanzania. Data from agronomic trials, and remote sensing layers were utilized to generate ESI maps showing a technology package's suitability beyond the trial sites. The ESI maps highlighted the potential risk of scaling out the validated vegetable technologies outside the trial sites. The area with low ESI values (0–1) has a lower risk and is therefore identified as the highest priority recommendation domain for scaling out the two technology packages.
Characterizing the biophysical context under which technologies performed successfully is vital for planning the scaling out investments. It promotes the sustainable use of limited resources, e.g., targeting areas requiring minimal supplemental fertilizer and irrigation. Applying innovation presented in this paper in scaling-out programs is likely to reduce the risk of failure of technologies. Information presented in the ESI and MIC maps helps the extension and development agencies to rationalize investment given limited resources (Rubiano et al., 2016). The ESI map could help agro-input companies to target supplies to regions where their technologies are more suitable. The demand for improved seeds can be estimated before the planting season, thereby improving the input supply systems. The ESI maps can also guide agro-dealers to target suitable zones for disseminating specific agro-advisory services.
The approach leverages the increasing remote sensing data to drive evidence-based targeting of agricultural technologies in areas where long-term agronomic trials are lacking. The ESI and MIC maps can also be utilized to guide the setting up of multi-location trials to investigate the cultivars' local adaptations under different environments and management practices. A recent study in the same district identified the ability to adapt to local conditions as one of the critical drivers of adopting improved vegetables at scale (Gramzow et al., 2018). The maps on the ESI and the most limiting factor provide a factual basis for future surveys to unravel the socio-cultural conditions that limit technology adoption. These maps need continuous improvement as more data from on-farm trials and higher-resolution remote sensing data becomes available. Therefore, the ESI maps are regarded as “living maps” that are updated as more information from trials becomes available to improve agro-advisory. For example, the recently released very high resolution (30 m) on soil nutrients and physical conditions (Hengl et al., 2021) will improve mapping crop suitability at the plot level Since this study utilizes open-source remote sensing data and geospatial tools, our methodology is replicable in other geographies or crops, if geotagged crop trial data is available. Although several institutions or initiatives are running vegetable trials in Tanzania, sharing data from these trials is a challenge. Initiatives for harmonizing agronomic trials remain a critical area of improvement.
The ESI methodology can be adapted depending on the objective of the scaling operation. Different reference sites can be selected depending on the goal of the scaling initiative. We selected the reference trials in the villages that returned the highest yield and profit. Alternatively, a scaling operation can choose the sites with the lowest crop yield if the objective is to uplift productivity in most challenged farms. Similarly, the trials with the most stable yield across space and time could be selected even if the aim is to promote stability rather than increase crop productivity. Therefore, thorough site characterization, especially when setting the crop trials, is essential to ensure the representativeness of main environmental gradients.
The Tengeru 2010 tomato showed wide adaption to the Babati district's conditions, while the Tengeru White eggplant is unsuitable in southwest part of the district around Bermi and Seloto villages. The south-west area of Babati is characterized by humid highlands and high precipitation (Figure 1). African eggplant requires less water than tomatoes and prefers sunny conditions like the situation around Matufa village that recorded the highest yields. Higher precipitation and lower evapotranspiration compared to the reference trial sites emerged as the main limiting factors in the large area for eggplant and tomatoes, respectively. This reflects the importance of optimal moisture for vegetable cultivation. Lower evapotranspiration than the reference sites limited tomatoes' suitability in the largest area. Since evapotranspiration is directly proportional to soil moisture content, it suggests that lower than optimal moisture affected the suitability of the tomatoes. Our study concurs with recent studies that observed that deviations from optimal soil moisture highly limit land suitability for vegetable cultivation in the tropics (Widiatmaka, 2016). However, too much rainfall increase the instances of pest attack on tomatoes thereby increasing the production cost since more pesticides are applied (Yuniarti et al., 2022). Low market access was the main factor limiting the Tengeru White eggplant's suitability in the hilly and forested landscape in south-east of the district. Although south-east of Babati district showed moderate to high suitability for African eggplant, poor accessibility can hinder vegetable production investments. Vegetables are durable commodities that need good market access to avoid post-harvest losses and high transport costs that reduce profits. Ji et al. (2018) reported road density as among China's most important drivers of vegetable production. Poor accessibility in south-east of Babati district is confounded by the prevalent lack of appropriate post-harvest storage management practices that cause up to 50% of yield losses of vegetables between harvesting and consumption (Majubwa et al., 2015).
The maps on the most influential covariates provide an evidence-based tool for spatial targeting of appropriate remedial measures. Evapotranspiration (ETP) and precipitation (PPT) were the main limiting factors for the two cultivars revealing that enhancing soil water conservation measures or supplemental irrigation is likely to increase yield.
However, our study did not consider the socio-cultural aspects that may hinder the adoption of a technology package even in ecologically suitable locations. There is a need to improve the ESI approach to capture the spatial variations of socio-cultural aspects like the differences in resource endowments, level of awareness, production orientation, and gender norms known to influence the adoption of vegetable technologies. For example, Fischer et al. (2020) reported that cultural norms restrict the participation of women in public gatherings leading to lower access to extension services. These norms in turn prevents women from getting more involved in fruit vegetable production and make them focus on leafy vegetables, as their cultivation is perceived easier and less costly. Similarly, Mugiyo et al. (2021) observed that the exclusion of crucial social-economic variables, such as access to credit and extension services, limits the adoption of vegetable cultivars in areas with suitable biophysical criteria. In that regard, Notenbaert et al. (2016) suggested the inclusion of socio-cultural variables in developing recommendation domains for agricultural technologies through stakeholder engagement and consultations. Nevertheless, the ESI maps provide a solid basis for future surveys to unravel the socio-cultural conditions that limit vegetable technologies' suitability.
Most studies on mapping the suitability of vegetables primarily utilized the top-down multi-criteria decision-making approaches that are subject to expert opinions (e.g., Widiatmaka, 2016; Rahmawaty et al., 2020, 2021; Mugiyo et al., 2021; Zakaria et al., 2022). In these studies, the geospatial layers were first classified into suitability groups based on literature or expert knowledge. Our method substantially reduces subjectivity by incorporating the reference crop trial data.
Future developments will focus on designing a open-source web-GIS tool for mapping the suitability of vegetable cultivars and agronomic practices. The proposed tool should be user-friendly and straightforward so farmers and village extension staff can operate it with basic gadgets with or without an internet connection to provide on and off-line agro-advisories. A similar web-GIS open-source tool implemented in Sri Lanka proved to be helpful to rural farmers with limited IT knowledge (Jayasinghe and Machida, 2008). The tool's utility was supported by the establishment of information technology centers in rural areas under a government project called e-Sri Lanka. This could be replicated in the Babati district to foster the vast potential of vegetable development. The targeting tool can be integrated into mobile-based agro-advisory initiatives currently piloted in the district.
4.1. Policy implications
The government of Tanzania and development partners has identified adoption of sustainable agriculture intensification technologies as a strategy to improve access to nutritious food. This aimed to achieve the sustainable development goal (SDG) 2 i.e., “end hunger, achieve food security and improved nutrition, and promote sustainable agriculture.” The reduction of malnutrition, stunting, and wasting is a priority policy issue. Vegetables are rich in essential micro-nutrients (Ojiewo et al., 2010), therefore increased production, accessibility and consumption promotes healthy living by reducing the hidden hunger (malnutrition and stunting) especially in children under five and elderly (Khamis et al., 2019). Extension agencies in Tanzania promote growing elite varieties of fruits and vegetables to increase nutritional security and generate more income in smallholder farming systems (Ochieng et al., 2021). Moreover, promoting adoption of improved vegetables at scale is an essential policy toward increasing income for rural households. The ESI maps generated in this study enables the extension and development agencies to objectively identify the priority areas for better scaling out of improved vegetables.
5. Conclusion
This study presents maps on the extrapolation suitability index (ESI) and the most influential covariates (MIC) for two vegetable technology packages in the Babati District of Tanzania. These maps are a useful guide to extension and development partners on prioritizing sites for targeting scaling out intervention to increase yield, income and maintain environmental health. Maps generated from this study are further expected to reduce the risk of failure of improved vegetable packages and ultimately enhance adoption at scale. The information generated in this study will support decision making on allocation of land for diversified agricultural production to achieve food and nutrition security. The main advantage of the method is its replicability across space and time, low investment required, and generates easy to understand the maps. However, the accuracy of the maps is dependent on the precision of the input layers and therefore they need to be validated on the field before adoption by extension agencies.
Data availability statement
The agronomic data used in this manuscript is available upon request at https://doi.org/10.7910/DVN/GQGQR6. The remote sensing data applied in the manuscript are open source and are available in the repositories listed in Table 1.
Author contributions
PL and JO collected and curated agronomic data. FM and JD designed the study, collected remote sensing data, and analyzed the data. FM, JD, PL, and JO contributed to writing and review of the manuscript. All authors contributed to the article and approved the submitted version.
Funding
USAID funded this study through grant AID-BFS-G-11-00002 under the Feed the Future (FtF) initiative that supported the Africa RISING program. The funders had no role in the design of the study, in the collection, analyses, or interpretation of data; in the writing of the manuscript, or in the decision to publish the results.
Conflict of interest
The authors declare that the research was conducted in the absence of any commercial or financial relationships that could be construed as a potential conflict of interest.
Publisher's note
All claims expressed in this article are solely those of the authors and do not necessarily represent those of their affiliated organizations, or those of the publisher, the editors and the reviewers. Any product that may be evaluated in this article, or claim that may be made by its manufacturer, is not guaranteed or endorsed by the publisher.
References
AbdelRahman, M.A.E., Natarajan, A., and Hegde, R. (2016). Assessment of land suitability and capability by integrating remote sensing and GIS for agriculture in Chamarajanagar district, Karnataka, India. Egypt. J. Rem. Sens. Space Sci. 19, 125–141. doi: 10.1016/j.ejrs.2016.02.001
Annicchiarico, P., Bellah, F., and Chiari, T. (2005). Defining subregions and estimating benefits for a specific-adaptation strategy by breeding programs. Crop Sci. 45, 1741–1749. doi: 10.2135/cropsci2004.0524
Baniya, N., Böehme, M., and Baniya, S. (2009). Physical land suitability assessment for the large cardamom amomum subulatum Roxb. Cultivation in hills of kathmandu valley. Chin. J. Populat. Res. Environ. 7, 59–63. doi: 10.1080/10042857.2009.10684954
Charrad, M., Ghazzali, N., Boiteau, V., and Niknafs, A. (2014). NbClust: an R package for determining the relevant number of clusters in a data set. J. Stat. Software 61, 36. doi: 10.18637/jss.v061.i06
Fischer, G., Patt, N., Ochieng, J., and Mvungi, H. (2020). Participation in and gains from traditional vegetable value chains: a gendered analysis of perceptions of labour, income and expenditure in producers' and traders' households. Eur. J. Develop. Res. 32, 1080–1104. doi: 10.1057/s41287-020-00257-0
Gramzow, A., Sseguya, H., Afari-Sefa, V., Bekunda, M., and Lukumay, P.J. (2018). Taking agricultural technologies to scale: experiences from a vegetable technology dissemination initiative in Tanzania. Int. J. Agricult. Sustainabil. 16, 297–309. doi: 10.1080/14735903.2018.1473103
Han, C., Chen, S., Yu, Y., Xu, Z., Zhu, B., Xu, X., et al. (2021). Evaluation of agricultural land suitability based on RS, AHP, and MEA: a case study in Jilin Province, China. Agriculture 11, 370. doi: 10.3390/agriculture11040370
Hengl, T., Miller, M.A.E., Krizan, J., Shepherd, K.D., Sila, A., Kilibarda, M., et al. (2021). African soil properties and nutrients mapped at 30–m spatial resolution using two-scale ensemble machine learning. Scien. Reports 11, 6130. doi: 10.21203/rs.3.rs-120359/v1
Hijmans, R.J. (2023). Raster: Geographic Data Analysis and Modeling. R package version 3.6-14. Available online at: https://CRAN.R-project.org/package=raster (accessed January 23, 2023).
Hyman, G., Hodson, D., and Jones, P. (2013). Spatial analysis to support geographic targeting of genetypes to environments. Front. Physiol. 4, 40. doi: 10.3389/fphys.2013.00040
Jayasinghe, P.K.S.C., and Machida, T. (2008). Web-based GIS online consulting system with crop-land suitability identification. Agricult. Inform. Res. 17, 13–19. doi: 10.3173/air.17.13
Ji, L., You, L., See, L., Fritz, S., Li, C., Zhang, S., et al. (2018). Spatial and temporal changes of vegetable production in China. J. Land Use Sci. 13, 494–507. doi: 10.1080/1747423X.2018.1459908
Khamis, A.G., Mwanri, A.W., Ntwenya, J.E., and Kreppel, K. (2019). The influence of dietary diversity on the nutritional status of children between 6 and 23 months of age in Tanzania. BMC Pediatr. 19, 518. doi: 10.1186/s12887-019-1897-5
Kumssa, D.B., Joy, E.J.M., Ander, E.L., Watts, M.J., Young, S.D., Walker, S., et al. (2015). Dietary calcium and zinc deficiency risks are decreasing but remain prevalent. Sci. Rep. 5, 10974. doi: 10.1038/srep10974
Lamigueiro, O.P., and Hijmans, R. (2019). rasterVis R package version 0.51.5. Available: http://oscarperpinan.github.io/rastervis (accessed January 23, 2023).
Lê, S., Josse, J., and Husson, F. (2008). FactoMineR: an R package for multivariate analysis. 25, 18. doi: 10.18637/jss.v025.i01
Lukumay, P.J., Afari-Sefa, V., Ochieng, J., Dominick, I., Coyne, D., and Chagomoka, T. (2018). Yield response and economic performance of participatory evaluated elite vegetable cultivars in intensive farming systems in Tanzania. Acta Hortic. 1205, 75–86. doi: 10.17660/ActaHortic.2018.1205.9
Majubwa, R.O., Msogoya, T.J., and Maerere, A.P. (2015). Effects of local storage practices on deterioration of African eggplant (Solanum aethiopicum L.) fruits. Tanzania J. Agricult. Sci. 14, 106–111.
Mesgaran, M.B., Cousens, R.D., and Webber, B.L. (2014). Here be dragons: a tool for quantifying novelty due to covariate range and correlation change when projecting species distribution models. Divers. Distribut. 20, 1147–1159. doi: 10.1111/ddi.12209
Minja, R.R., Ambrose, J., Swai, I.S., and Ojiewo, C. (2011). Improved tomato varieties for Eastern Tanzania promising improved tomato varieties for Eastern Tanzania. Afr. J. Hort. Sci. 4, 24–30.
Mostafiz, R.B., Noguchi, R., and Ahamed, T. (2021). Calorie-based seasonal multicrop land suitability analysis for regional food nutrition security in Bangladesh. Asia-Pacific J. Reg. Sci. 5, 757–795. doi: 10.1007/s41685-021-00197-5
Mugiyo, H., Chimonyo, V.G.P., Sibanda, M., Kunz, R., Nhamo, L., Masemola, C.R., et al. (2021). Multi-criteria suitability analysis for neglected and underutilised crop species in South Africa. PLoS ONE 16, e0244734. doi: 10.1371/journal.pone.0244734
Mugo, J.W., Kariuki, P.C., and Musembi, D.K. (2016). Identification of suitable land for green gram production using GIS based analytical hierarchy process in Kitui County, Kenya. J Remote Sens. GIS 5, 170. doi: 10.4172/2469-4134.1000170
Muthoni, F.K., Baijukya, F., Bekunda, M., Sseguya, H., Kimaro, A., Alabi, T., et al. (2019). Accounting for correlation among environmental covariates improves delineation of extrapolation suitability index for agronomic technological packages. Geocarto Int. 34, 368–390. doi: 10.1080/10106049.2017.1404144
Muthoni, F.K., Guo, Z., Bekunda, M., Sseguya, H., Kizito, F., Baijukya, F., et al. (2017). Sustainable recommendation domains for scaling agricultural technologies in Tanzania. Land Use Policy 66, 34–48. doi: 10.1016/j.landusepol.2017.04.028
Notenbaert, A., Pfeifer, C., Silvestri, S., and Herrero, M. (2016). Targeting, out-scaling and prioritising climate-smart interventions in agricultural systems: lessons from applying a generic framework to the livestock sector in sub-Saharan Africa. Agric. Syst. doi: 10.1016/j.agsy.2016.05.017
Ochieng, J., Afari-Sefa, V., Muthoni, F., Kansiime, M., Hoeschle-Zeledon, I., Bekunda, M., et al. (2021). Adoption of sustainable agricultural technologies for vegetable production in rural Tanzania: trade-offs, complementarities, and diffusion. Int. J. Agricult. Sustain. 3, 1–19. doi: 10.1080/14735903.2021.1943235
Ojiewo, C.O., Tenkouano, A., and Yang, R. (2010). The role of AVRDC—The world vegetable centre in vegetable value chains. Af. J. Horticult. Sci. 3, 6130.
Osorio-Olvera, L., Lira-Noriega, A., Soberón, J., Peterson, A.T., Falconi, M., Contreras-Díaz, R.G., et al. (2020). Ntbox: an R package with graphical user interface for modelling and evaluating multidimensional ecological niches. Methods Ecol. Evolut. 11, 1199–1206. doi: 10.1111/2041-210X.13452
R Core Team (2023). R: A Language and Environment for Statistical Computing. Vienna, Austria: R Foundation for Statistical Computing. Available online at: https://www.R-project.org/ (accessed January 29, 2020).
Rahmawaty, F.S., Rauf, A., and Batubara, R. (2020). Land suitability assessment for Lansium domesticum cultivation on agroforestryland using matching methodand geographic information system. Biodiversitas 21, 3683–3690. doi: 10.13057/biodiv/d210835
Rahmawaty, Marpaung, R.M.E., Batubara, R., and Rauf, A. (2021). The limiting factor of land suitability for development of gambir cultivation (case-study in Sari Laba Jahe Village Deli Serdang North-Sumatra). IOP Conf. Series Earth Environ. Sci. 782, 032006. doi: 10.1088/1755-1315/782/3/032006
Rubiano, M.J.E., Cook, S., Rajasekharan, M., and Douthwaite, B. (2016). A Bayesian method to support global out-scaling of water-efficient rice technologies from pilot project areas. Water Int. 41, 290–307. doi: 10.1080/02508060.2016.1138215
Tesfaye, K., Jaleta, M., Jena, P., and Mutenje, M. (2015). Identifying potential recommendation domains for conservation agriculture in Ethiopia, Kenya, and Malawi. Environ. Manage. 55, 330–346. doi: 10.1007/s00267-014-0386-8
Thapa, D.M., Shrivastav, C.P., Shah, S.C., and Sah, K. (2020). Land suitability evaluation using GIS for vegetable crops at Sharadanagar, Chitwan, Nepal. Trop. Agrobiodiv. (TRAB) 1, 42–46. doi: 10.26480/trab.01.2020.42.46
Usha, K., and Singh, B. (2013). Potential applications of remote sensing in horticulture—A review. Sci. Hortic. 153, 71–83. doi: 10.1016/j.scienta.2013.01.008
Widiatmaka (2016). Integrated use of GIS, AHP and remote sensing in land use planning for tropical high altitude vegetable crops. J. Appl. Horticult. 18, 87–99. doi: 10.37855/jah.2016.v18i02.19
Yuniarti, W., Sumardjo, W., and Wibawa, W.D. (2022). Development of highland vegetable commodity areas through multi-criteria decision making (MCDM) analysis and geographic information systems. IOP Conf. Ser. Earth Environ. Sci. 950, 012074. doi: 10.1088/1755-1315/950/1/012074
Zakaria, Y.S., Shaibu, A.-G., and Baatuuwie, B.N. (2022). Assessment of physical suitability of soils for vegetable production in the libga irrigation scheme, Northern Region, Ghana using the analytic hierarchy process and weighted overlay analysis. Turk. J. Agricult. Food Sci. Technol. 10, 1395–1403. doi: 10.24925/turjaf.v10i8.1395-1403.5004
Keywords: digital agronomy, GIS, land suitability assessment, spatial targeting, recommendations domains, sustainable intensification, vegetable cultivars
Citation: Muthoni FK, Delore JM, Lukumay PJ and Ochieng J (2023) Extrapolation suitability index for sustainable vegetable cultivation in Babati district, Tanzania. Front. Sustain. Food Syst. 7:1047505. doi: 10.3389/fsufs.2023.1047505
Received: 18 September 2022; Accepted: 17 March 2023;
Published: 03 April 2023.
Edited by:
Francis Tetteh, Council for Scientific and Industrial Research (CSIR), GhanaReviewed by:
Msafiri Mkonda, Sokoine University of Agriculture, TanzaniaRahmawaty Rahmawaty, Universitas Sumatera Utara, Indonesia
Copyright © 2023 Muthoni, Delore, Lukumay and Ochieng. This is an open-access article distributed under the terms of the Creative Commons Attribution License (CC BY). The use, distribution or reproduction in other forums is permitted, provided the original author(s) and the copyright owner(s) are credited and that the original publication in this journal is cited, in accordance with accepted academic practice. No use, distribution or reproduction is permitted which does not comply with these terms.
*Correspondence: Francis Kamau Muthoni, ZmttdXRob25pQGdtYWlsLmNvbQ==