- 1Markets, Trade and Institutions Unit, International Food Policy Research Institute (IFPRI), Nairobi, Kenya
- 2International Food Policy Research Institute, Washington, DC, United States
- 3Department of Agricultural and Food Economics, University for Development Studies (UDS), Tamale, Ghana
This paper introduces a new framework to quantify costs and benefits for resilience-related outcomes of agricultural innovations targeting smallholder farmers. The framework employs a non-unitary household model with expected utility to quantify welfare benefits associated with non-monetary outcomes that are important from a development perspective, such as improved consumption smoothing, empowerment, and changes in time use. We demonstrate the application of the framework using a case study of climate information services (CIS) in Ghana. We develop a set of individual bargaining weights based on the women’s empowerment in agriculture index, to demonstrate how benefits from CIS are distributed among men and women within households. We find that for the average risk-averse farmer, using CIS is associated with a 37-percent increase in expected utility, but male household heads benefit more than women living in male-headed households. Cost–benefit analyses that do not consider the intrahousehold distribution of benefits associated with agricultural innovations will overestimate benefits accruing to women with low bargaining power.
1. Introduction
Nearly three-fourths of the 2.1 billion people living in extreme poverty depend on agriculture as their main source of livelihood (Lele and Goswami, 2021). Increasing frequency of extreme weather events such as droughts, floods, heat waves, and tropical storms due to climate change however poses multiple threats to agriculture. Recent global estimates indicate that about 91 percent of the 7,255 major disasters between 1998 and 2017 were driven by extreme weather events (Wallemacq and House, 2018). Such events adversely affect crop and livestock productivity, as well as farmers’ willingness to experiment, invest, and innovate (Lybbert and Sumner, 2012). This jeopardizes food availability, incomes, and livelihoods, especially for the poor, smallholder subsistence farmers, and women (Tanner and Mitchell, 2008; Demetriades and Esplen, 2010; Thornton et al., 2014).
Governments and development agencies have sought to reduce the negative impacts of climate risks on vulnerable populations. They use a variety of approaches to manage weather risks ex ante, including the use of innovations aimed at building the resilience of the production systems against climate variability. Examples of these approaches include the provision of insurance against weather risks (Mahul and Stutley, 2010; OECD, 2015), promoting climate-smart agriculture (e.g., the use of sustainable and climate-resilient agricultural practices such as stress-tolerant varieties, conservation agriculture, and diversified farming), and climate information services [FAO (Food and Agriculture Organization of the United Nations), 2010; World Bank, 2011].
In developing countries, expenditure on agricultural innovation research increased by over 192 percent within the past four decades (Fuglie, 2016; Fuglie et al., 2020). Given that most developing countries have scarce resources, investments in agricultural innovations to build resilience compete for funds with other important areas of development such as social protection, health care, education, and physical infrastructure. Therefore, a better understanding of the different types of costs and benefits associated with developing and implementing these innovations will be critical to help justify, prioritize, and improve investments in them, as well as investments in the agricultural research-for-development that leads to these innovations.
Benefits associated with the innovations can be heterogeneous, and depend for instance on a user’s gender, social class, or marital status. It is important to quantify benefits for different groups of farmers (such as women and poorer subsistence farmers). This will help identify target groups where the greatest potential welfare gains can be achieved, as well as adjust programming to create greater benefits for those groups that development practitioners are aiming to benefit most. This paper provides a framework for analyzing the costs and benefits of investments in agricultural innovations that aim to enhance the resilience of smallholder farmers in developing economies, allowing the estimation of heterogeneous benefits for various groups of beneficiaries.
A growing literature has been devoted to studying the costs and benefits of investments in various agricultural innovations; for instance, climate-smart agriculture (Marta-Pedroso et al., 2007; Balmford et al., 2011; Sain et al., 2017; Nganga et al., 2017a,b; Wafula et al., 2018; Mutenje et al., 2019; CARE-Burundi, 2020; Williams et al., 2020), and country-level rought insurance (Clarke and Vargas Hill, 2013; Jensen et al., 2017; Kramer et al., 2020). Many of these studies focus on quantifying the expected Net Present Value (NPV) of current and future cash flows generated by these innovations over their lifecycle; often discounted at market interest rates to estimate the costs, benefits, and the subsequent payback period of the investments.
Although the NPV approach adequately captures changes in expected cash flows, the approach does not account for the benefits of reduced risk exposure for risk-averse farmers. Damba et al. (2021) and Smith et al. (2021) for instance acknowledge that while attempting to assess costs and benefits, they were confronted with challenges in measuring unintended outcomes as a result of the usage of a particular technology. Jensen et al. (2017) go a step further, by quantifying the welfare benefits from improved consumption smoothing and the benefits of reduced risk exposure for risk averse farmers. They employ an expected utility model to compare the benefits from agricultural insurance and unconditional cash transfers among livestock farmers in Kenya. While serving as an important starting point, this study does not identify benefits related to the impacts of insurance and cash transfers on time use, which is an area where this paper contributes.
Moreover, it is important to understand the intrahousehold gendered distribution of costs and benefits (Kabeer, 1992), since spouses are likely to have conflicting preferences [such those described in Haddad et al. (1998), Donni and Chiappori (2011), and Doss (2013)]. Mutenje et al. (2019) and CARE-Burundi (2020) study gender as part of their cost–benefit analyses but do not quantify welfare gains from improved gender outcomes such as women’s empowerment, which is another area where this paper aims to contribute. Most importantly, most existing gendered cost–benefit analyses are conducted at the household level, treating the household head’s gender as the main variable for gender-disaggregated analyses. Given that most women live in male-headed households, and the fact that bargaining power will vary among women living in male-headed versus female-headed households, focusing only on the household head will likely bias the gender distribution of cost and benefits of agricultural innovations.
To address these gaps, our toolkit employs an expected utility framework to map individual consumption under alternative weather scenarios into a measure of wellbeing (‘expected utility’), taking into consideration not only the expected level of consumption but also higher moments of its distribution, including its variance. In this way, the framework can capture the utility benefits associated with an improved ability to manage agricultural risks and smooth consumption, which is often an important objective for innovations developed to improve smallholder farmers’ resilience. We extend this model to include leisure, to capture an innovation’s benefits in terms of reducing labor burdens, or costs in terms of increasing workloads. Moreover, by using a non-unitary household model that allows for variations in individual bargaining power (Chiappori, 1988, 1992) we measure how the costs and benefits are differently distributed among men and women in the household. This will help in integrating the consequences of agricultural innovations related to gender equality and empowerment into a cost–benefit analysis.
To illustrate the use of the framework, we focus on climate information services (CIS) promoted through the Accelerating Impacts of CGIAR Climate Research for Africa (AICCRA) program. AICCRA is a three-year World Bank funded program that aims to scale innovations from international agricultural research in six countries in Africa: Ethiopia, Kenya, Ghana, Senegal, Mali, and Zambia.1 This study uses baseline data collected from 661 farmers recruited to participate in AICCRA’s CIS program in Ghana. We first study how the use of CIS is associated with agricultural outcomes (including investments in farm inputs, agricultural productivity, and off-farm labor incomes) under three weather conditions: normal, moderate, and severe2. We then estimate the expected utility with and without CIS for different household members, taking into consideration their individual beliefs and preferences. To shed light on the intrahousehold distribution of costs and benefits related to CIS, we analyze utility gains separately for three types of individuals: men in male-headed households (MHH), which are typically the male heads themselves; women in MHH, which are typically the spouses of the male heads; and women in female-headed households (FHH), including widows and never married female heads with no primary male-decision maker within the household.3
We find that under moderate weather conditions, CIS users invest significantly more in farm inputs than non-CIS users and agricultural payoffs are significantly higher under CIS production regime. On average, for a risk-averse farmer, using CIS increases the expected utility by 37 percent. Compared to women in MHH, men have higher bargaining power, which significantly increases their level of consumption, and thus their expected utility. Female household heads have higher levels of consumption than individuals in MHH under normal weather conditions but consume less under severe weather conditions. These findings suggest that although women in FHH have the bargaining power to make decisions, they might lack adequate access to resources to cope with severe climate events. Women in MHH are the least empowered, with significantly lower levels of consumption, and expected utilities, and they draw the least benefits from CIS. Whereas investing in CIS would create a utility gain that is equivalent to an increase in consumption of 93 USD for male household heads, the gains for women are 76 and 65 USD depending on whether they reside in a FHH or MHH, respectively. These findings show how household-level analysis can mask important details regarding the intrahousehold distribution associated with costs and benefits from agricultural innovation.
The remainder of the paper is structured as follows. In Section “Background on Climate Information Services (CIS)”, we provide background on CIS to contextualize the proposed framework to conduct cost–benefit analysis of resilience-enhancing agricultural innovations. This framework is introduced in Section “A new approach for cost-benefit analysis of resilience-enhancing innovations”, along with the data needed to apply this approach. Section “Empirical application of the new approach for cost-benefit analysis” presents our empirical case study, and Section “Discussion and Conclusions” concludes.
2. Background on climate information services
CIS delivers data, statistical analyses, tools, and other information resources about expected future climate conditions – including, among others, temperature and precipitation scenarios, and sea-level changes – and their potential impacts on livelihoods (USAID, 2013). People and organizations can use this information to reduce climate-related losses and in building resilience to future climate risks. In agriculture, CIS enables farmers to optimize many aspects of their production systems, including the timing of sowing, planting, fertilizer application, irrigation, pest and disease control, harvesting, and post-harvest handling (Balaji and Craufurd, 2011; Hansen et al., 2011; McKune et al., 2018), enabling them to improve their farm productivity and welfare (Naab et al., 2019; Vaughan et al., 2019; Nidumolu et al., 2020).
CIS have several desirable attributes that make them attractive to policymakers and farmers. First, CIS increase the accuracy and reliability of weather forecasts, enabling farmers to make timely production decisions (Asrar et al., 2020). As such, CIS can have important labor-and gender-related outcomes. For instance, early drought warnings and weather forecasts can help farmers optimize when to plant their crops, thereby reducing the labor burden of having to plant a second time in case the rains fail (Gumucio et al., 2020). Second, communication and dissemination of CIS can be conducted through a diverse suite of freely available or cheap channels such as mobile phones (SMS, phone calls, and internet), radio, television, printed media, extension officers (through demonstrations, training, and visits) and other channels like public meetings, farmer-to-farmer messaging, and workshops (Yegbemey and Egah, 2021). This can improve affordability and equity in reach among various groups of farmers. Third, CIS can be easily tailored to meet the needs of different groups of farmers, and compared to other risk-management strategies, the marginal cost of CIS dissemination and scaling is low (Tall et al., 2014; Guido et al., 2020).
Although these advantages are broadly acknowledged, and several studies have found that farmers have a high willingness to pay for CIS (Ouédraogo et al., 2018; Dinh, 2020; Gitonga et al., 2020; Antwi-Agyei et al., 2021a), in many developing countries, especially those in sub-Saharan Africa, CIS is either missing or it is available as short-term weather and seasonal forecasts rather than user targeted large-scale national and regional information that can support long-term planning (Hansen et al., 2011; World Meteorological Organization, 2014; Georgeson et al., 2017). Moreover, women and poor subsistence farmers—who are disproportionately vulnerable to the negative effects associated with climate risks— are more likely to be excluded from available CIS (Beuchelt and Badstue, 2013; Huyer et al., 2017; Diouf et al., 2019; Antwi-Agyei et al., 2021b). Although CIS, if reaching these populations, could contribute to their empowerment, the information might not reach them, and in fact, providing CIS could widen information gaps relative to the groups that are not reached by CIS. The disempowering effect of this widening information gap is one potential cost of CIS. Moreover, if households choose to expand their agricultural production in response to having access to CIS, the additional labor associated with that expansion might disproportionally fall on women. It is therefore important to document the unintended consequences of CIS for different household members’ work burdens as another potential cost of CIS.
The Accelerating Impacts of CGIAR Climate Research for Africa (AICCRA) program bridges these gaps by strengthening and facilitating the institutional capacity to develop and disseminate tailored and targeted CIS packages for small-scale farmers. The targeted CIS packages are expected to increase reach to previously excluded populations such as women and subsistence farmers. AICCRA also aims at promoting the delivery of longer-term forecasts that could help farmers in strategically planning their agricultural production activities. An important objective for AICCRA is also to document the impacts of these CIS packages, and their costs and benefits, including the costs associated with potential unintended consequences on for instance women’s economic empowerment and work burdens.
Figure 1 provides an overview of how access and use of CIS intersect with resilience to weather risks and the potential costs and benefits associated with CIS use. By gaining improved access to information about effective climate response measures, farmers will be able to better anticipate climate-related events and take preventive actions that can help safeguard productive activities, avoid catastrophic losses, and increase return on their investments in farm inputs, land, and labor. For instance, CIS can allow farmers to plant varieties that are optimal for the projected weather conditions, or they could increase their risk-taking capacity and invest in complementary inputs such as fertilizer, labor, and mechanization. These actions can directly impact farm productivity and incomes, thus improving household welfare. CIS could also shift labor burdens for women and men. For instance, CIS can reduce workloads via mechanization, better division of labor, or early planning, which can enhance individual and household welfare. This can also increase an individual’s bargaining power, but potentially at the expense of others’ say in how household resources are allocated. If CIS empower groups of individuals that are currently disempowered, net benefits from providing CIS are likely to be positive. But CIS could also empower already more powerful individuals, which may come at a cost of further disempowerment of marginalized individuals. Furthermore, a recommendation to apply fertilizer or grow new crop varieties may call for increased weeding and post-harvest processing, which can worsen labor burdens for household members (often women) who are tasked with these types of activities (Walker, 2013; Beuchelt, 2016). This would be a cost associated with the use of CIS.
3. A new approach for cost–benefit analysis of resilience-enhancing innovations
3.1. Theoretical framework
We model the costs and benefits associated with CIS from a farming household’s perceptive using a collective household utility framework (Chiappori, 1988, 1992; Browning et al., 2014), which we extend to include multiple states of the world, or weather realizations. Under this framework, household utility is defined as a weighted average of the set of (potentially unequal) levels of expected utility for individual members, aggregated using these family members’ bargaining weights (Sen, 1984). Most consumption takes place within the household, based on a common budget that is shared within the household.4 Certain goods, such as food consumption, are rival private goods, while others, such as house improvements, are non-rival public goods. When choosing to allocate a limited budget to various private and public goods, the household takes into consideration utility outcomes of each member, weighted by that member’s bargaining weight.
For simplicity, we model a two-person household with individuals , and assume that the household optimizes an aggregate of expected utility for both individuals, weighted by each individual’s negotiation power or bargaining weight , where (Manser and Brown, 1980; McElroy and Hornery, 1981; Chiappori, 1988),
where EU is expected utility aggregated at the family level, is individual ’s utility function, denotes whether the household uses CIS ( or not , is the level of consumption for individual , and is the amount of leisure that this person will enjoy.5 Expected utility for individual , is an aggregate of individual utilities across three possible states of the world or weather realizations, , using as the probability of realizing weather event to aggregate contemporaneous utilities for the separate states of the world. We consider normal ( ), moderate ( ), and severe ( ) weather events.
The household optimizes the level of consumption and leisure under weather realization for each family member , subject to the budget and time constraints specified in Equations (1) and (2):
The budget constraint, Equation (2), implies that an individual’s consumption is determined by three variables: net household income from agricultural production, , which is modeled as a function of both family members’ labor allocation to farm activities, , and household investments in farm inputs, ; the individual’s share of that income, which is determined by that individual’s bargaining weight, ; and the individual’s income from other activities, such as employment, casual work, and business, which is equal to the wage rate for those activities, , times the total number of hours worked on other activities, Zφk.6 The time constraint, Equation (3), specifies the total amount of leisure that individual enjoys as total available time minus any time spent on the farm, , on domestic work, , and other income-generating activities, .
Note that the relationship between agricultural income and inputs ( ) depends on whether the farmer uses CIS to optimize its farming practices and technologies, . Using CIS could improve the return on labor, on farm inputs, or on a combination of the two, and in our framework, that is the only mechanism through which CIS use can influence utility.7 In principle, one could also let the bargaining weight, , depend on whether the household uses CIS, given that information can be empowering and increase bargaining weights, but we abstract from doing so in this paper. As a result, we can quantify the utility gains from using CIS within this framework by defining a monetary equivalent of utility gains from using CIS. This equivalent is implicitly defined in Equation (4) as the amount by which individual ’s consumption would need to increase in the absence of CIS, , to match this same person’s expected utility when using CIS, :
To make the framework more flexible and easily adaptable, we will implement a numerical approach to identify this amount , instead of providing an explicit solution for the monetary equivalent of utility gains from using CIS. This numerical approach requires specifying a functional form for the utility function, for which we draw on Rupert et al. (1995):
Here, we define as the contribution of consumption to an individual’s utility (relative to the contribution of leisure), as the coefficient of relative risk aversion, and as the elasticity of substitution between consumption and leisure. This functional form may be a simplification of reality, as it assumes full separability and a constant elasticity of substitution (CES) between consumption and leisure, but it offers a useful starting point to quantify changes in expected utility when a program may affect both consumption and time use.8
3.2. Data requirements
To apply this framework, one needs data on the variables and parameters included in Equations (1)–(5). In this subsection, we outline what types of data one could collect to satisfy these data requirements. This includes various household and individual attributes such as the distribution of weather outcomes, farmers’ demographic and socio-economic characteristics, climate risks, CIS access and use, consumption, leisure, and time and risk preferences.
3.2.1. Probability of weather outcomes
The information on weather probabilities can be elicited by directly asking the respondents about their perception on the occurrence of severe, moderate, or normal weather condition within the next 10 years, such that the subjective probabilities of these events occurring sum to one, . The problem with this approach is that it is hypothetical, which will not always elicit truthful responses. It also provides merely a course measure, given that this method allows to construct probabilities only in increments of a decimal. In case researchers are interested in averages across a population, this will not necessarily be an issue, given that unsystematic biases in individual responses disappear when aggregating over larger numbers of survey respondents, and this will also provide more exact probabilities than the individual estimates with increments of at most a decimal (0, 0.1, 0.2, etc.). Alternatively, if an individual’s subjective beliefs are an important outcome measure on their own, one can use more granular and incentivized methods to elicit beliefs (see Attanasio, 2009, for an overview).
3.2.2. Distribution of consumption and leisure across weather scenarios
We define consumption as the expenditure incurred on goods and services (such as food, health, clothing, education, among others) that are used for the satisfaction of needs or wants (OECD, 2015). Current practices for collecting consumption data differ widely across types of surveys, between countries, and over time, which might compromise the comparability of the data and measurement (FAO and World Bank, 2018). One can measure consumption through an expenditure approach, but this is typically a time-consuming endeavor. Instead, in this study, where we needed to rely on a short survey, we assume that household and individual consumption growth is correlated very closely with expected income growth (Skinner, 1987; Jappelli and Pistaferri, 2000; Howe et al., 2009) and use projected incomes as a proxy for consumption. Several approaches to collecting income data have been outlined in the literature (for example, Sweeney et al., 2016). In this study, for each of the three alternative weather scenarios, we ask respondents to report the minimum and maximum projected incomes from agriculture, including crop production, livestock, and livestock activities ( in our framework), as well as from waged, salaried and trade activities ( in the framework). Income under each weather scenario is then estimated by averaging over the two values to reduce measurement error compared to using a single value response of projected income.
Following Alderman and Sahn (1993) and Aguiar and Hurst (2007), we define leisure as time spent away from market and domestic work to pursue other activities designed to yield direct utility, such as entertainment, socializing, active recreation, and general relaxation (excluding maintenance activities such as sleeping and eating). Leisure is an important aspect for individuals as it improves social well-being and long-term productivity (Beatty and Torbert, 2003; Wei et al., 2016). To estimate the share of time dedicated to leisure, it is important to understand how individuals divide their 24 h among various daily activities, for which we employ time-use survey guidelines from the Multinational Time Use Survey Project.9 We ask individuals to report the average time spent on various activities within a day, including paid work (such as full-time and part-time jobs, commuting to the workplace, school activities among others), domestic work (such as cooking, cleaning, caring for children and other family members, volunteering, shopping among others), farm work (such as planting, weeding, fertilizer application, harvesting, post-harvest handling, marketing among others), personal care (sleep, eating and drinking among others), and leisure (such as pursuing hobbies, watching television, socializing with friends and family, attending events, among others). One can deduce leisure time from the amount of time an individual engages in other non-leisure activities.
We ask respondents to provide their time use profile under bad, normal and good weather realizations, but when someone has longitudinal panel data with variation in weather realizations over time, one could use a 24-h time use profile, and estimate how this time use profile depends on realized weather outcomes, since a 24-h time use profile reduces measurement errors on time use responses.
3.2.3. Risk preferences
Risk preferences ( in our conceptual framework) are an important factor in individual decision making on investment, asset valuation, and life choices. For risk averse farmers, utility gains can be obtained not only from increases in the levels of consumption and leisure, but also from a reduction in the variability of these two variables. For a given consumption and leisure level, utility gains from reduced risk will be greatest among farmers with higher levels of risk aversion. It is therefore important to also capture risk preferences.10
To date, there have been several approaches used to assess individual degree of risk aversion. For instance, Binswanger (1980) uses lottery choices from field experiments to show that most farmers exhibit a significant degree of risk aversion. Other methods include a bidding and pricing task (Smith and Walker, 1993), and eliciting buying and/or selling prices for simple lotteries (Kachelmeier and Shehata, 1992). We adopt a risk preference elicitation method first introduced by Eckel and Grossman (2002) and validated by Falk et al. (2018) in the Global Preference Survey.11 In this approach, respondents make hypothetical choices between five lotteries with different levels of risk and expected payoffs versus a safe option. Measures of risk aversion are inferred from the levels of risk and expected payoffs in the lotteries at which respondents switch from preferring the lottery to the safe option.
3.2.4. Bargaining weights
An individual’s bargaining power, , will influence the share of rival household resources allocated to them, which will in turn influence their utility outcomes (see Equations 1 and 2). This implies that the intrahousehold distribution of rival resources will favor the preferences of the spouse with a stronger bargaining weight. Existing literature presents several ways to measure individual bargaining power, including the use of consumption ratios for different household members, income contribution, educational attainment, inheritance, and asset ownership rights (Blumberg and Coleman, 1989; Friedman-Sanchez, 2006; Anderson and Eswaran, 2009). Manser and Brown (1980) and McElroy and Hornery (1981) suggest that an individual’s bargaining power depends on a threat point, that is, when negotiating, spouses can threaten to walk away, and therefore bargaining power is related to how much utility an individual can achieve on their own. Friedberg and Webb (2006) however argue that several omitted factors in the previous empowerment measures could bias the outcomes. For instance, if a spouse earns more because they work more, this might reduce the time that is available for them to engage in daily decisions making activities, rendering them disempowered. They therefore argue that it is better to construct measures of bargaining power based on questions that directly ask respondents about how major decisions are made within their households.
To measure individual bargaining power, we follow the approach described in Friedberg and Webb (2006) and develop an index based on who has ‘the final say’ between a husband and wife (or opposite sex adult decision-makers) when major decisions are being made. We follow the bargaining power indicators outlined in the “input in productive decision-making” module of the Women’s Empowerment in Agriculture Index (WEAI; Alkire et al., 2013). In the module, respondents were asked whether they participated in decision making regarding various household activities, and if they participated, to what extent they had an input, and whether they could make the final decision. These household activities included seed selection, land allocation to various farm activities, harvesting, rearing of small and large livestock, household engagement in waged and salaried labor, and household spending. Our measure reveals whose preferences are reflected to a greater degree in household choices. By directly eliciting decision-making power, our analysis overcomes potential omitted variable bias problems arising in earlier studies.12
4. Empirical application of the new approach for cost–benefit analysis
4.1. Sampling and data collection
To demonstrate the applicability of our framework, we use survey data collected from the AICCRA intervention communities which constitute 6 regions in Ghana. The AICCRA intervention is a continuation of an earlier CIS program implemented by the CGIAR research program on Climate Change, Agriculture and Food Security (CCAFS). The data is from a baseline survey from 651 households conducted in November 2022. The sampling procedure involved a multi-stage purposive sampling criteria whereby six regions (namely, Bono East, Central, Greater Accra, Northern, Upper East, and Upper West) were selected for the study based on population size, agricultural activities, and climate risks. Using the same criteria, a minimum of one and maximum of three districts were chosen from each region, making a total of 11 districts. In total, 38 villages were randomly selected from all the districts. Sampling was clustered at the village level: in each village, a sampling frame was developed with the help of community leaders, from which households were randomly selected for inclusion in the study. The village also served as clusters for treatment randomization, whereby 19 villages were allocated to the treatment and 19 to control. The data used in this paper were collected as baseline, before the CIS intervention was rolled out in the 19 randomly selected treatment villages.
4.2. Summary statistics
4.2.1. Household characteristics
Table 1 provides descriptive statistics for the full sample (Columns 1–2) as well as for CIS users versus non-users (Columns 3–4 and 5–6, respectively), and the difference between the two (Column 7). In total, 61 percent of all households have been using CIS prior to the survey. The average age of the household head is 45 years and 67 percent of households are male headed. A significantly higher proportion of male-headed households used CIS. The average respondent has completed 3.63 years of formal schooling, with CIS users having significantly higher education levels than non-users. About 49 percent of the households belong to a farmer’s club and 15 percent are part of a savings group.
Households derive about 65 percent of their income from crop farming, indicating that this is their main but not only source of livelihood. However, CIS users depend significantly more on crop production than non-users, earning close to 70 percent of their income from crop farming. About 16 percent of the household income is derived from livestock farming. CIS non-users depend significantly more on livestock than CIS users. About 82 percent of farmers produced maize during the short rains of 2022, indicating the importance of maize to both CIS users and non-users. Other crops produced include cowpeas, potatoes, yams, pepper, and tomatoes. CIS users are significantly more likely to grow potatoes, yams, and pepper. In the first half of 2022, about half of the households in the sample experienced shocks that prevented them from sowing, or that prevented the germination of their seeds. Farmers also reported shocks that caused crop damage (44 percent), post-harvest losses (20 percent) and livestock deaths (17 percent). Most of these shocks are experienced equally often by CIS users and non-users, but households not using CIS are significantly more likely to experience post-harvest losses.
We also elicited the probability at which different types of weather scenarios occur, to be able to estimate expected utility by aggregating utilities for each of the three weather scenarios [ , see Equation (1)]. We did this by asking households to indicate how many of the next 10 years they expected to have severe, moderate, versus normal weather conditions. We then divided their responses to these questions by 10, in order to derive an individual’s estimate of the probability that the different types of weather conditions would occur. On average, households expect severe, moderate, and normal weather conditions with a probability of 37, 31, and 32 percent, respectively. Importantly, this did not vary between CIS users and non-users, and thus, when estimating expected utility, we will use the same beliefs for the two household types.
4.2.2. CIS use
Figure 2 shows the distribution of topics on which the 402 households using CIS received information through a CIS program. Information on expected rainfall, including intensity and duration of rainfall, is the most commonly used form of CIS, followed by information on recommended planting time, crop variety selection, and fertilizer application. Advisories on water management, field selection, weed management and soil management are used less frequently.
4.2.3. Bargaining power
Figure 3 shows the distribution of household bargaining power among the three gender categories. As discussed, in the theoretical model bargaining weights are distributes across the primary and secondary decision maker within the household such that Even in FHH, where there is only one decision maker on whom the survey was administered, other family members or community neighbors, may have influence in the decision-making process. A woman in a FHH will therefore not necessarily have a bargaining power that is equal to one. But given that we are defining at the household decision-making level, we maintain bargaining power for FHH at 1. In MHH, with both primary male and secondary female decision makers, Figure 3 shows that male household heads have substantially higher levels of bargaining power compared to their spouses . The lower levels of bargaining power among women living in MHH is an indicator of their level of disempowerment and shows how household negotiations and allocation of household public goods might not favor them.
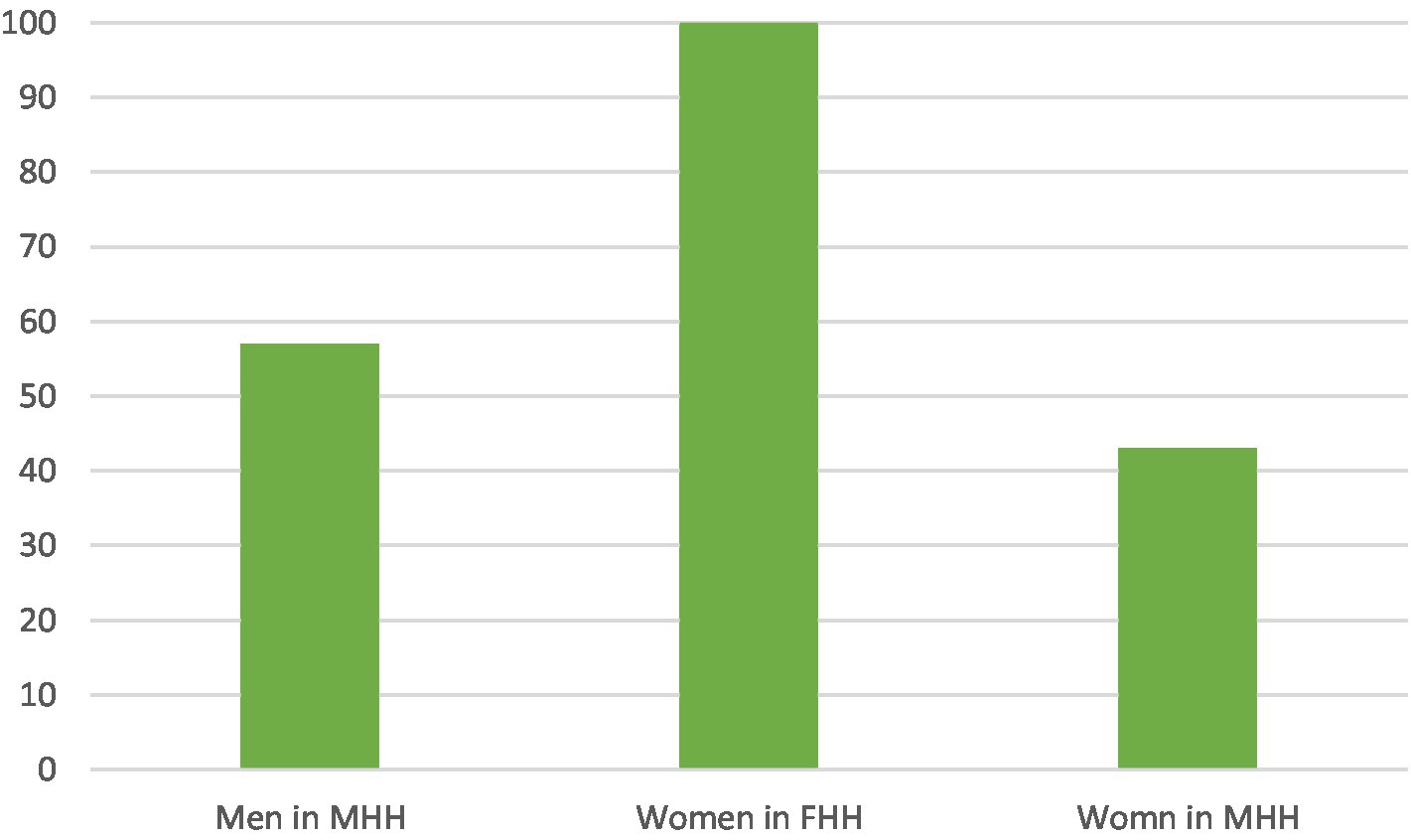
Figure 3. Distribution of household bargaining power. MHH and FHH stands for “Male Headed Households” and “Female Headed Households” respectively.
In theory, CIS could have impacts on bargaining power, as it can provide family members with information that increases their influence in household decision-making. However, we do not find significant differences in bargaining power between CIS users and non-users in either of the three groups (results not shown here but available upon request). This means that in the empirical application of our framework, we are unable to quantify utility gains related to married women’s increased bargaining power, but in other settings, where CIS users may enjoy increased bargaining weights, and a redistribution of resources within the household, our expected utility framework could be used to quantify the related utility costs and benefits.
4.3. Investments, labor allocation, and household incomes by weather scenario
In this section, we describe the main variables that enter the budget constraint (Equation 2), including net agricultural household income, household farm investment, and labor allocations under CIS and non-CIS production regimes. Table 2 indicates the average farm investment for CIS users and non-users in Ghanaian Cedi under different weather scenarios and the expected expenditure estimated as . The results show that the largest share of farm investment across the different weather outcomes is allocated toward fertilizer, hired human labor, seeds, herbicides, and mechanization. We find that when farmers are expecting severe weather outcomes, CIS users invest significantly more in pesticide, herbicides, machinery, and hired labor. CIS users also invest significantly more in seeds, fertilizer, pesticides, herbicides, machinery, and labor under moderate and/or normal weather conditions. Although farmers in both production regimes have low investment in animal traction and agricultural insurance, compared to CIS users, CIS non-users invest significantly more in animal traction under severe and moderate weather outcomes and they purchase significantly more agricultural insurance under moderate and normal weather conditions.13 Aggregating across the three weather scenarios, CIS users expect spending significantly more on fertilizer, pesticides, herbicides, machinery, and hired labor than CIS non-users. This is attributed to well informed CIS available at various stages of a production season and the need to respond to the demands of the services accessed.
Table 3 shows the average daily time allocation (in hours) in various household activities under different production regimes and weather scenarios, as well as the expected number of hours per day, aggregating over the three weather scenarios (for instance, in the case of on-farm labor). We include activities related to crop production (including grain production and production for high-value markets such as horticultural farming) and livestock production (including small and large animal farming, and aquaculture). Households also reported the amount of time they spend on non-farm domestic work (including time spent on cooking, fetching water and firewood, providing unpaid care work such as taking care of the elderly and children), market activities (including salaried and waged employment, and business), and leisure activities (including travel personal care, exercising, or socializing; but excluding maintenance activities such as sleeping and eating). The latter is used to construct the amount of leisure that individual household members experience ( in Equation).
Under the three weather conditions, we find that respondents from households not using CIS allocate significantly more time to livestock production and domestic activities while CIS users allocate significantly more time to market activities. There were no statistical differences in the time allocation to crop production and leisure between CIS users and non-users. Consistent with our theoretical assumption, the findings on significant differences in time allocation in domestic and market activities could signal that CIS can potentially free up time for users to pursue other market activities, but we do not replicate these findings in the labor domain.
We finally evaluate the differences in projected household income from agricultural activities, paid domestic work, waged and salaried employment, and other household businesses. Table 4 shows that projected agricultural incomes (both crop and livestock income) are highest under normal weather conditions and lowest under severe weather conditions. CIS users project earning significantly higher crop incomes under each of the three weather scenarios. This could be directly attributed to the preparedness that the CIS packages provide to the users which allows for planning of available resources and activities to mitigate negative climate events. At the same time, CIS users projected higher incomes from livestock and livestock activities which are significantly higher under moderate and normal weather scenarios. Consistent to time spent in market activities, projected business incomes are larger for CIS using households, however the differences are not statistically significant.
The results further show that CIS non-users project significantly higher levels of incomes from waged and salaried activities. Moreover, CIS non-users incomes from domestic activities are higher under all-weather scenarios, however the differences are not statistically significant. The findings on domestic and waged or salaried incomes suggest households that do not use CIS could be more diversified outside of farm production than their CIS counterparts. At the aggregate level, the difference in the expected level of income for CIS users versus non-users is 4,441 Cedis per season (387 USD). This is the increase in income that the typical CBA would report as the program benefit. Moving forward, we will analyze how this estimated benefit changes as we also consider time use and the intrahousehold distribution of benefits, and introduce a utility framework to analyze these benefits.
4.4. Consumption and leisure based on an individual’s bargaining power
We apply the utility model described in Section “A new approach for cost-benefit analysis of resilience-enhancing innovations” to estimate the costs and benefits associated with CIS and their subsequent intrahousehold distribution. We begin by estimating individual consumption based on Equation , assuming that this is equal to , agricultural income net of investments in agriculture ( ), multiplied by an individual’s bargaining weight ( , plus individual incomes from various market activities such that Figure 4 shows that for all the three gender categories, consumption is highest under normal weather conditions, and lowest under severe weather conditions. These results are not surprising considering the fact that farmers realize better agricultural payoffs under normal weather conditions even without CIS use. Households that use CIS under normal and moderate weather conditions realize significantly better (p < 0.01) consumption outcomes than their non-CIS counterparts. Although the consumption of CIS using households is marginally higher under severe weather conditions, the differences are not statistically different.
At the individual level, we find that under severe and moderate weather conditions, men heads in CIS using households have better consumption outcomes than women in both categories. Women heads in CIS using household experience better consumption outcome under normal weather conditions, however, women heads in both CIS production regime have the worst consumption outcomes under severe weather outcomes. These findings suggest that even though women in FHH have the autonomy of decision making in their household, they might lack adequate access to recourses that can help them in coping with severe climate events. At the aggregate level, we find that women in MHH have the least consumption outcomes, which is directly attributable to their lower degree of bargaining power. Collectively, these results demonstrate that by failing to account for intrahousehold distribution of agricultural innovation benefits, most studies overestimate the benefits accruing to women.
Next, we estimate the intrahousehold distribution of leisure under the different weather conditions and CIS production regimes. Figure 5 shows the amount of leisure enjoyed by CIS users and non-users. Within each group (e.g., Women in FHH who use CIS), there were no statistically significant differences in leisure time. However, CIS non-users in male heads of households enjoy significantly more leisure time than women (p < 0.05), while women in MHH, have the least amount of leisure under both production regimes. If CIS helps households free up time, as is often theorized (Gumucio et al., 2020), then it appears that in our study context, women did not use these time savings to enjoy more leisure time. Furthermore, the use of CIS did not decrease the labor burden borne by women living in MHH.
4.5. Estimating expected utility framework for different types of CIS users and non-users
To map these results into Equation (7), we first assume that the relative contribution of consumption and leisure to an individual’s utility are equal to one another, that is, In an extension, one could use data on a farmer’s willingness to substitute income for increased leisure (for which we have questions included in the survey described in Section “Data requirements”) to calibrate this parameter, but the current analyses adopt this assumption to simplify the empirical illustration of the framework. For similar reasons, we follow Lim and Lee (2021) and Choi et al. (2008) by also assuming imperfect substitution between consumption and leisure among all individuals, and fixing . We use farmers’ expectations of the frequency at which alternative weather conditions occur to estimate the probability of the occurrence of these weather scenarios, such that severe weather occurs with a probability , moderate weather with a probability , and normal weather with a probability . To evaluate how expected utility with and without CIS varies in risk preferences, we present results assuming that a decision-maker is risk averse ( ), risk neutral ( ), or risk loving ( ).
Table 5 shows the expected utility from consumption and leisure for the different groups of individuals under different weather scenarios for a risk averse farmer ( ). For all individuals under consideration, the expected utility from consumption and leisure is highest under normal weather conditions, and lowest under severe weather conditions. We find CIS users attain higher levels of utility from consumption and leisure than CIS non-users. The differences are statistically different under all-weather outcomes for household heads, and under severe and normal weather conditions for women in MHH. These results are consistent with the earlier findings that CIS use increases the level of consumption across all gender categories (Figure 6).
Comparing aggregate expected utilities across individuals in Columns (10)–(12), we find that on average, men heads of households under both CIS and non-CIS production regimes have the highest expected utility levels followed by women in FHH. On aggregate, women in MHH have the lowest levels of expected utility. Overall, we find that CIS use increases the expected utility from consumption and leisure by 37 percent.
Figure 6 shows the relationship between an individual’s risk aversion and the expected utility from consumption and leisure. Expected utility increases with an increase in the degree of risk-aversion. For risk averse farmers, production under CIS increases expected utility relative to non-CIS production. Although the expected utilities converge as farmers become more risk-taking, it is worth noting that even at higher levels of , that is, at values at which individuals become risk neutral or even risk taking, we find that CIS is associated with an increase in utility. The question, of course, is whether the increase in expected utility is sufficient to offset the costs associated with the program, and we will address this question in the next section.
4.6. Introducing costs for cost–benefit analysis
Thus far, we have focused primarily on the private costs and benefits associated with CIS. From a policy perspective, a government or NGO will be interested in understanding the cost implications of providing CIS services, whether these costs are small enough for the program costs to be smaller than the private benefits accrued to targeted beneficiaries, and whether alternative investments could achieve equal utility gains. To answer these questions, we consider a situation in which the government or a donor would increase the consumption of CIS non-users in order to provide them with equal expected utility as CIS users. This can also be interpreted as the monetary equivalent of the utility gains that CIS users achieve relative to non-users, that is, as the private benefit associated with introducing CIS.
Figure 7 shows the increase in consumption that each of the three groups of CIS non-users would need to achieve equal utility as the three groups of CIS users, or the monetary equivalent of utility gains associated with CIS. To achieve the same level of expected utility in one season, MHH from non-CIS households would require on average an increase in consumption of 1,100 Cedi (93 USD14), while the required increase in consumption for women in FHH is 900 CEDI (76 USD), and 780 Cedi (65 USD) for women in MHH. Put differently, investing in CIS would create a utility gain that is equivalent to an increase in consumption of 93 USD for male household heads, 76 USD for women in FHH, and 65 USD for women in MHH. If the total program cost per user is some number , the benefit–cost ratio can be defined as 76/ , 64/ , and 51/ for men, women heads and women in MHH, respectively.15 These benefit–cost ratios can be compared with other types of programs to prioritize investments.
Note that these estimated utility gains are substantially greater than the benefits in terms of increased expected income across respondents. The utility framework allowed us to quantify the gains from increased leisure enjoyed by CIS users. In future work, one could go a step further than presented in the current paper and decompose the difference in utility gains estimated using the two methods into a portion that is related to differences in the intrahousehold distribution of consumption, the introduction of risk preferences, and the introduction of leisure into the framework.
5. Discussion and conclusions
Governments and development agencies have invested millions of dollars in developing agricultural innovations to help farmers cope with climate risks ex ante. Understanding the costs and benefits of these innovations helps in justifying, prioritizing, and targeting the right packages to the right groups of farmers. Although previous studies have evaluated the costs and benefits of various innovations to enhance resilience in agriculture, most studies have focused on monetary outcomes at the household level, and typically do not quantify the benefits from gender-related outcomes such as increased women’s empowerment and bargaining power from providing women with access to better information, financial services, or production technologies. In addition, given that these studies are conducted at the household level, The findings might also not be generalizable in households where members do not share identical bargaining power and preferences. Finally, existing cost benefit analyses (CBA) typically do not consider the effects that agricultural innovations have on farmers’ risk exposure and ability to smooth consumption, which can have utility benefits for risk averse farmers even if the average level of consumption remains the same.
The aim of this study was to illustrate a new framework for the cost–benefit analysis of innovations in agriculture, particularly those aimed to enhance resilience. It contributes to the literature by quantifying a range of costs and benefits that have been previously ignored, while also accounting for how these outcomes are distributed among individuals within the household. To that end, we propose a non-unitary utility framework whereby individuals within the household are assumed to maximize utility from consumption—based on their share of payoffs from agricultural production, and the value of labor that they allocate in the market, determining their payoffs from off-farm activities—as well as leisure. Bargaining weights determine the share of agricultural output that is allocated to each individual within the household. Income realizations and associated decisions are analyzed under three weather outcomes: normal; moderate; and severe. We aggregate across these three weather scenarios by taking an individual’s expected utility, using subjective beliefs of the probability at which each weather scenario occurs. We analyze utility gains separately for three groups of farmers: men in male-headed households, women living in male-headed households, and women in female-headed households.
We illustrate our framework for cost–benefit analysis through an empirical application, focusing on baseline information collected in the context of a climate information service (CIS) that is being rolled out in Ghana with support from a World Bank-funded program on Accelerating Impacts of CGIAR Climate Research for Africa (AICCRA). We find that farmers using CIS invest more in their farms, especially under moderate weather conditions, consistent with the idea that resilience technologies promoted through CIS are particularly effective under those types of weather conditions. We also find that CIS use is positively correlated with agricultural incomes, which could be attributed to the high farm investment among users. Women living in male-headed households have lower bargaining weights, translating into lower consumption levels.
Combining these outcomes into our expected utility model, we find significant differences in expected utility between users and non-users of CIS. On average, CIS usage is associated with an increase in expected utility of 37 percent, and we find that the monetary equivalent of these utility gains is substantially greater than the benefits one would have derived under more common CBA approaches, which focus on changes in expected income levels and investments alone. Future work will explore in more detail what is driving these differences, and to what extent they are related to the focus on the intrahousehold distribution of benefits associated with CIS use, to integrating changes in leisure in the framework, versus the use of an expected utility framework in which households are assumed to be risk averse.
Moreover, we find that CIS usage is associated with increased utility for all three types of individuals, including men in male-headed households, women in female-headed households, and women in male-headed households; and that this finding is robust to changing the parameter of risk aversion assumed in the expected utility model. However, we find that the greatest utility gains from CIS accrue to household heads; the monetary equivalents of the estimated welfare gains correspond to 93 and 76 USD for male and female household heads respectively, versus a substantially smaller 65 USD for women in male-headed households. These differences in welfare gains are primarily related to the lower bargaining power and higher labor burdens of women, relative to the household heads. CIS usage was not associated with an improvement in these outcomes. If CIS were associated with an increase in bargaining power among women in male-headed households, or with lower labor burdens for this group of women, we would have expected to see greater utility gains.
Our study has a number of limitations. First, the theoretical model ignores time dynamics. It could be that resilience-enhancing innovations have costs in the present that result in long-term benefits, for instance in terms of increased productivity from soil conservation, or simply that there is a learning curve around adopting new technologies and practices, and that it takes time for the benefits associated with this adoption to materialize. For tractability, we abstained from bringing this time dimension into the current framework, but one could easily extend it to also incorporate future periods in the utility framework, along with discounting of future utility terms by using measures of an individual’s time preference.
In addition, in the empirical case study, we relied on a comparison of farmers using CIS versus those that have opted not to be using CIS. Any changes in outcomes for CIS users and non-users cannot be interpreted as causal, and the welfare benefits presented in this paper purely serve as an illustration of how the framework could be applied. Ideally, one would estimate gains in consumption and leisure under various weather conditions using more rigorous econometric methods, for instance by leveraging randomized controlled trials (RCTs) or quasi-experimental approaches. Moreover, instead of asking farmers to project their agricultural incomes, farming investments and time allocations under different weather scenarios, longer-term panel data collection could help estimate the effects of alternative weather conditions on these outcomes, which would provide more objective measures of the distribution of consumption and leisure. These limitations will be addressed in future stages of the AICCRA program, as the program is rolling out a set of RCTs in various settings and collecting additional rounds of data.
In conclusion, we find that the use of an expected utility framework can enrich cost–benefit analysis of innovations that have been developed with the aim of enhancing resilience. The framework helps quantify welfare benefits associated with non-monetary outcomes that are nonetheless important from a development perspective, such as improved consumption smoothing, changes in bargaining power, changes in labor allocations and time use, and, for a given set of bargaining weights, consumption levels and labor allocations, the distribution of benefits within a household. We show that it is important for cost–benefit analysis to move beyond quantifying the net present value of expected income gains, and consider a broader range of development objectives, as bringing in non-monetary outcomes can help quantify the outcomes that public sector investments are looking for.
Data availability statement
The raw data supporting the conclusions of this article will be made available by the authors, without undue reservation.
Ethics statement
The studies involving human participants were reviewed and approved by IFPRI. The patients/participants provided their written informed consent to participate in this study.
Author contributions
AT: questionnaire design, data cleaning, data analysis, and writing. BK: questionnaire design, data analysis, and writing. OD: data collection and writing. All authors contributed to the article and approved the submitted version.
Funding
This work was funded by the Accelerating Impacts of CGIAR Climate Research for Africa (AICCRA) program, which is supported by a grant from the International Development Association of the World Bank. The authors are grateful to Stellamaris Aju for research assistance, and to Steve Zebiak and Alison Rose from Columbia University for their support and guidance in implementing this work. All errors are our own.
Conflict of interest
The authors declare that the research was conducted in the absence of any commercial or financial relationships that could be construed as a potential conflict of interest.
The reviewers MM and SN declared a shared research partnership group (CGIAR) with the authors to the handling editor at the time of review.
Publisher’s note
All claims expressed in this article are solely those of the authors and do not necessarily represent those of their affiliated organizations, or those of the publisher, the editors and the reviewers. Any product that may be evaluated in this article, or claim that may be made by its manufacturer, is not guaranteed or endorsed by the publisher.
Footnotes
1. ^More details on the AICCRA program can be found here: https://aiccra.cgiar.org/
2. ^Normal: the weather outcome is above the medium historical ranges; Moderate: weather outcome within the medium of historical ranges. Severe; current weather significantly below or way above historical ranges, such as severe drought or floods.
3. ^For the analysis, we classify self-identifying female heads with a male partner who is a key decision maker within the household as women in MHH.
4. ^We abstract from household members having individual, personal incomes, that they hide from their household members. There are cases where individual keep separate finances, however, whenever they both contribute to a household public good, they are regarded as deciding consumption jointly (Fafchamps, 2005).
5. ^Utility is continuous, increasing ( , twice differentiable and strictly concave ( . Note that preferences can vary across family members, since individuals have separate utility functions.
6. ^Note that income from agricultural production is used to invest in agricultural inputs, which are considered household public goods, and the remaining is spent on individual consumption, which is considered a rival good.
7. ^Instead of specifying a functional form for the production function and estimating how CIS affects the return of net agricultural income, , on labor and farm inputs, we estimate gains from CIS directly from empirical observations of net agricultural income without and with CIS, not accounting for labor and farm input use. The framework could be extended in cases where rich panel data allow estimating production functions, and the Appendix conceptualizes one way to model the impacts of CIS. It suggests that under normal weather conditions, farmers are expected to do equally well regardless of whether they use recommendations made by CIS; during severe weather conditions, farmers are likely losing most of their income, regardless of whether they are following CIS recommendations; and only during moderate weather conditions, CIS may make a real difference, protecting farmers’ livelihoods from negative shocks.
8. ^Moreover, the numerical approach is sufficiently flexible for someone to specify an alternative utility function when applying the framework in cost–benefit analyses.
10. ^In our conceptual framework, we treat access to CIS as an exogenous parameter, but in reality, farmers’ willingness to use CIS and their ability to draw benefits from it will depend on their degree of risk aversion, offering another reason for why it is important to capture baseline levels of risk aversion.
11. ^https://www.briq-institute.org
12. ^The drawback of the model used, and its solution in which consumption out of household-level net income is equal to total income times the individual’s bargaining weight, is that bargaining weights need to sum to one. This means that an increase in bargaining power of one family member comes at the expense of reduced utility for another household member, unless the change in bargaining weights was accompanied by an increase in net income. This zero-sum game is against the idea of many empowerment programs that they are creating win-win solutions. Thus, we see the collective household bargaining model as a useful starting point, but future research could explore alternatives to circumvent the condition that bargaining weights need to sum up to one.
13. ^Farmers may purchase agricultural insurance even in seasons with normal weather conditions because enrollment windows typically close at the start of the agricultural season.
14. ^We use May, 2023 conversion rates where 1 USD = 11.85 Ghanian Cedi.
15. ^The comparison of CIS users and non-users draws on variation in whether households, are using any CIS, from a range of CIS that are available to them. The goal is to illustrate the use of the framework, not to provide an exact cost–benefit figure for a particular CIS program. We do not have information on the implementation costs of the alternative CIS that are available to farmers in the study population, and therefore leave open the exact cost figure.
References
Aguiar, M., and Hurst, E. (2007). Measuring trends in leisure: the allocation of time over five decades. Q. J. Econ. 122, 969–1006. doi: 10.1162/qjec.122.3.969
Alderman, H., and Sahn, D. E. (1993). Substitution between goods and leisure in a developing country. Am. J. Agric. Econ. 75, 875–883. doi: 10.2307/1243975
Alkire, S., Meinzen-Dick, R., Peterman, A., Quisumbing, A., Seymour, G., and Vaz, A. (2013). The Women’s empowerment in agriculture index. World Dev. 52, 71–91. doi: 10.1016/j.worlddev.2013.06.007
Anderson, S., and Eswaran, M. (2009). What determines female autonomy? Evidence from Bangladesh. J. Dev. Econ. 90, 179–191. doi: 10.1016/j.jdeveco.2008.10.004
Antwi-Agyei, P., Amanor, K., Hogarh, J. N., and Dougill, A. J. (2021a). Predictors of access to and willingness to pay for climate information services in North-Eastern Ghana: a gendered perspective. Environ. Dev. 37:100580. doi: 10.1016/j.envdev.2020.100580
Antwi-Agyei, P., Dougill, A. J., and Abaidoo, R. C. (2021b). Opportunities and barriers for using climate information for building resilient agricultural systems in Sudan savannah agro-ecological zone of North-Eastern Ghana. Clim. Serv. 22:100226. doi: 10.1016/j.cliser.2021.100226
Asrar, G., Ryabinin, V., and Detemmerman, V. (2020). Climate science and services: providing climate information for adaptation, sustainable development and risk management. Curr. Opin. Environ. Sustain. 4, 88–100. doi: 10.1016/j.cosust.2012.01.003
Attanasio, O. (2009). Expectations and perceptions in developing countries: their measurement and their use. Am. Econ. Rev. 99, 87–92. doi: 10.1257/aer.99.2.87
Balaji, V., and Craufurd, P. (2011). Using information and communication technologies to disseminate and exchange agriculture-related climate information in the indo Gangetic Plains. Copenhagen, Denmark: CCAFS.
Balmford, A., Fisher, B., Green, R. E., Naidoo, R., Strassburg, B., Turner, R. K., et al. (2011). Bringing ecosystem services into the real world: an operational framework for assessing the economic conse-quences of losing wild nature. Environ. Resour. Econ. 48, 161–175. doi: 10.1007/s10640-010-9413-2
Beatty, J., and Torbert, W. (2003). The false duality of work and leisure. J. Manag. Inq. 12, 239–252. doi: 10.1177/1056492603256340
Beuchelt, T. D., and Badstue, L. (2013). Gender, nutrition-and climate-smart food production: opportunities and trade-offs. Food Security 5, 709–721. doi: 10.1007/s12571-013-0290-8
Beuchelt, T. D. (2016). Gender, social equity and innovations in smallholder farming systems: Pitfalls and pathways. In Technological and institutional innovations for marginalized smallholders in agricultural development, 181–198.
Binswanger, H. (1980). Attitudes toward risk: experimental measurement in rural India. Am. J. Agric. Econ. 62, 395–407. doi: 10.2307/1240194
Blumberg, R. L., and Coleman, M. T. (1989). A theoretical look at the gender balance of power in the American couple. J. Fam. Issues 10, 225–250. doi: 10.1177/019251389010002005
Browning, C. R., Gardner, M., Maimon, D., and Brooks-Gunn, J. (2014). Collective efficacy and the contingent consequences of exposure to life-threatening violence. Dev. Psychol. 50, 1878–1890. doi: 10.1037/a0036767
CARE-Burundi, (2020). Costs and Benefits of Applying a Gender-Transformative Approach in Agriculture programming. Evaluation of the EKATA Model in Burundi. Available at: https://www.careevaluations.org/wp-content/uploads/Win-Win-Final-Cost-Benefit-Analysis.pdf.
Chiappori, P. A. (1988). Rational household labor supply. Econometrica 56, 63–90. doi: 10.2307/1911842
Chiappori, P. A. (1992). Collective labor supply and welfare. J. Polit. Econ. 100, 437–467. doi: 10.1086/261825
Clarke, D., and Vargas Hill, R. (2013). “Cost-benefit analysis of the African risk capacity facility.” IFPRI Discussion Paper 01292.
Choi, K. J., Shim, G., and Shin, Y. H. (2008). Optimal portfolio, consumption-leisure and retirement choice problem with CES utility. Math. Financ. 18, 445–472. doi: 10.1111/j.1467-9965.2008.00341.x
Damba, O. T., Kizito, F., Bonilla-Findji, O., Yeboah, S., Oppong-Mensah, B., Clottey, V., et al. (2021). Climate Smart Agriculture (CSA) -Climate Information Services (CIS) Prioritization in Ghana: Smartness Assessments and Outcomes. Accelerating Impacts of CGIAR Climate Research for Africa (AICCRA) Technical Report.
Demetriades, J., and Esplen, E. (2010). The gender dimensions of poverty and climate change adaptation. Social Dimensions of Climate Change. 133.
Dinh, D. T. (2020) Economic valuation of agricultural producers’ WTP for the weather information service – A case study in Central Vietnam. E3S web of conferences 203, 03016 (2020)
Diouf, N. S., Ouedraogo, I., Zougmoré, R., Ouedraogo, M., Partey, S., and Gumucio, T. (2019). Factors influencing gendered access to climate information services for farming in Senegal. Gend. Technol. Dev. 23, 93–110. doi: 10.1080/09718524.2019.1649790
Donni, O., and Chiappori, P. A. (2011). “Nonunitary models of household behavior: a survey of the literature” in Household economic behaviors. International series on consumer science. ed. J. Molina (New York, NY: Springer)
Doss, C. (2013). Intrahousehold bargaining and resource allocation in developing countries. World Bank Res. Obs. 28, 52–78. doi: 10.1093/wbro/lkt001
Eckel, C., and Grossman, P. (2002). Sex differences and statistical stereotyping in attitudes toward financial risk. Evol. Hum. Behav. 23, 281–295. doi: 10.1016/S1090-5138(02)00097-1
Falk, A., Becker, A., Dohmen, T., Enke, B., and Huffman, D. (2018). Global evidence on economic preferences. Q. J. Econ. 133, 1645–1692. doi: 10.1093/qje/qjy013
FAO (Food and Agriculture Organization of the United Nations). (2010). Climate-smart agriculture: Policies, practices and financing for food security, adaptation and mitigation, Rome, FAO
FAO and World Bank. (2018). Food data collection in household consumption and expenditure surveys: guidelines for low-and middle-income countries Rome: FAO; World Bank.
Friedberg, L., and Webb, A. (2006). Determinants and consequences of bargaining power in households. Center for retirement research at Boston University. Working paper, 2006–13.
Friedman-Sanchez, G. (2006). Assets in intra-household of bargaining among cut-flower workers in Colombia. Fem. Econ. 12, 247–269. doi: 10.1080/13545700500508551
Fuglie, K. (2016). The growing role of the private sector in agricultural research and development world-wide. Glob. Food Sec. 10, 29–38. doi: 10.1016/j.gfs.2016.07.005
Fuglie, K., Madhur, G., Aparajita, G., and William, F. (2020). Harvesting prosperity: Technology and productivity growth in agriculture. Washington, DC: World Bank.
Georgeson, L., Maslin, M., and Poessinouw, M. (2017). Global disparity in the supply of commercial weather and climate information services. Sci. Adv. 24:1602632. doi: 10.1126/sciadv.1602632
Gitonga, Z. M., Visser, M., and Mulwa, C. (2020). Can climate information salvage livelihoods in arid and semiarid lands? An evaluation of access, use and impact in Namibia. World Dev. Perspect. 20:100239. doi: 10.1016/j.wdp.2020.100239
Guido, Z., Knudson, C., Campbell, D., and Tomlinson, J. (2020). Climate information services for adaptation: what does it mean to know the context? Clim. Dev. 12, 395–407. doi: 10.1080/17565529.2019.1630352
Gumucio, T., Hansen, J., Huyer, S., and van Huysen, T. (2020). Gender-responsive rural climate services: a review of the literature. Clim. Dev. 12, 241–254. doi: 10.1080/17565529.2019.1613216
Haddad, L., Hoddinott, J., and Alderman, H. (1998). Intrahousehold resource allocation in developing countries: models, methods, and policies. Food Nutr. Bull. 19, 71–72. doi: 10.1177/156482659801900111
Hansen, J., Mason, S., Sun, L., and Tall, A. (2011). Review of seasonal climate forecasting for agriculture in sub-Saharan Africa. Exp. Agric. 47, 205–240. doi: 10.1017/S0014479710000876
Howe, L. D., Hargreaves, J. R., Gabrysch, S., and Huttly, S. R. A. (2009). Is the wealth index a proxy for consumption expenditure? A systematic review. J. Epidemiol. Community Health 2009, 871–877. doi: 10.1136/jech.2009.088021
Huyer, S., Hansen, J., Rose, A., Vaughan, C., and Huysen, T (2017) What we know about gender and rural climate services. CCAFS info note in agriculture programming: Evaluation of the EKATA model in Burundi
Jappelli, T., and Pistaferri, L. (2000). Using subjective income expectations to test for excess sensitivity of consumption to predicted income growth. Eur. Econ. Rev. 44, 337–358. doi: 10.1016/S0014-2921(98)00069-5
Jensen, N., Barrett, C., and Mude, A. (2017). Cash transfers and index insurance: a comparative impact analysis from northern Kenya. J. Dev. Econ. 129, 14–28. doi: 10.1016/j.jdeveco.2017.08.002
Kabeer, N. (1992). Evaluating cost-benefit analysis as a tool for gender planning. Dev. Chang. 23, 115–139. doi: 10.1111/j.1467-7660.1992.tb00448.x
Kachelmeier, J., and Shehata, M. (1992). Examining risk preferences under high monetary incentives: experimental evidence from the People’s republic of China. Am. Econ. Rev. 82, 1120–1141.
Kramer, B., and Ceballos, F. (2018). Enhancing adaptive capacity through climate-smart insurance: Theory and evidence from India. 30th International Conference of Agricultural Economists. Vancouver, Canada.
Kramer, B., Rusconi, R., and Glauber, J. (2020). Five years of regional risk pooling an updated cost-benefit analysis of the African risk capacity. IFPRI discussion paper 01965
Lele, U., and Goswami, S. (2021). Financing for sustainable structural transformation, food for all: International organizations and the transformation of agriculture. Oxford, 2021; online edn, Oxford Academic, 18 Nov. 2021.
Lim, B. H., and Lee, H. S. (2021). Household utility maximization with life insurance: a CES utility case. Japan J. Indust. Appl. Math. 38, 271–295. doi: 10.1007/s13160-020-00437-9
Lybbert, T. J., and Sumner, D. A. (2012). Agricultural technologies for climate change in developing countries: policy options for innovation and technology diffusion. Food Policy 37, 114–123. doi: 10.1016/j.foodpol.2011.11.001
Mahul, O., and Stutley, C. (2010). Government support to agricultural insurance challenges and options for developing countries. Washington, DC: The World Bank
Manser, M., and Brown, M. (1980). Marriage and household decision-making: a bargaining analysis. Int. Econ. Rev. 21, 31–44. doi: 10.2307/2526238
Marta-Pedroso, C., Domingos, T., Freitas, H., and de Groot, R. (2007). Cost–benefit analysis of the zonal program of Castro Verde (Portugal): highlighting the trade-off between biodiversity and soil conservation. Soil Tillage Res. 97, 79–90. doi: 10.1016/j.still.2007.08.010
McElroy, M., and Hornery, M. (1981). Nash-bargained household decisions: toward a generalization. Int. Econ. Rev. 22, 333–349. doi: 10.2307/2526280
McKune, S., Poulsen, L., Russo, S., Devereux, T., Faas, S., McOmber, C., et al. (2018). Reaching the end goal: do interventions to improve climate information services lead to greater food security? Clim. Risk Manag. 22, 22–41. doi: 10.1016/j.crm.2018.08.002
Mutenje, M. J., Farnworth, C. R., Stirling, C., Thierfelder, C., Mupangwa, W., and Nyagumbo, I. (2019). A cost-benefit analysis of climate-smart agriculture options in southern Africa: balancing gender and technology. Ecol. Econ. 163, 126–137. doi: 10.1016/j.ecolecon.2019.05.013
Naab, F., Abubakari, Z., and Ahmed, A. (2019). The role of climate services in agricultural productivity in Ghana: the perspectives of farmers and institutions. Clim. Serv. 13, 24–32. doi: 10.1016/j.cliser.2019.01.007
Nganga, S.K., Miller, V., Essegbey, G.O., Naaminong, K., Ansah, V., Nutsukpo, D., et al. (2017a). Cost and benefit analysis for climate-smart agricultural (CSA) practices in the coastal Savannah agro-ecological zone (AEZ) of Ghana. Uganda: Sub-Regional Office.
Nganga, S.K., Notenbaert, A., Mwungu, C.M., Mwongera, C., and Girvetz, E. (2017b). Cost and benefit analysis for climate-smart soil practices in Western Kenya, Kenya: Subregional Office for Africa.
Nidumolu, U., Lim-Camacho, L., Gaillard, E., Hayman, P., and Howden, M. (2020). Linking climate forecasts to rural livelihoods: mapping decisions, information networks and value chains. Weather Clim. Extrem. 27:100174. doi: 10.1016/j.wace.2018.06.001
Ouédraogo, M., Barry, S., Zougmoré, R. B., Partey, S. T., Somé, L., and Baki, G. (2018). Farmers’ willingness to pay for climate information services: evidence from cowpea and sesame producers in northern Burkina Faso. Sustainability. 10:3. doi: 10.3390/su10030611
Rupert, P., Rogerson, R., and Wright, R. (1995). Estimating substitution elasticities in household production models. Economic Theory 6, 179–193.
Sain, G., Ana María, A., Corner-Dolloff, C., Miguel, L., Andreea, L., Martínez-Baron, D., et al. (2017). Costs and benefits of climate-smart agriculture: the case of the dry corridor in Guatemala. Agric. Syst. 151, 163–173. doi: 10.1016/j.agsy.2016.05.004
Skinner, J. (1987). A superior measure of consumption from the panel study of income dynamics. Econ. Lett. 23, 213–216. doi: 10.1016/0165-1765(87)90042-5
Smith, H. E., Sallu, S. M., Whitfield, S., Gaworek-Michalczenia, M. F., Recha, J. W., Sayula, G. J., et al. (2021). Innovation systems and affordances in climate smart agriculture. J. Rural. Stud. 87, 199–212. doi: 10.1016/j.jrurstud.2021.09.001
Smith, L., and Walker, M. (1993). Rewards, experience, and decision costs in first price auctions. Econ. Inq. 31, 237–244. doi: 10.1111/j.1465-7295.1993.tb00880.x
Sweeney, S., Vassall, A., Foster, N., Simms, V., Ilboudo, P., Kimaro, G., et al. (2016). Methodological issues to consider when collecting data to estimate poverty impact in economic evaluations in low-income and middle-income countries. Health Econ. 25, 42–52. doi: 10.1002/hec.3304
Tall, A., Kristjanson, P.M., Chaudhury, M., McKune, S., and Zougmoré, R.B. (2014). Who gets the information? Gender, power and equity considerations in the design of climate services for farmers. CCAFS Working Paper Research Program on Climate Change, Agriculture and Food Security (CCAFS) Copenhagen, Denmark: CGIAR.
Tanner, T., and Mitchell, T. (2008). Entrenchment or Enhancement: Could Climate Change Adaptation Help Reduce Chronic Poverty?, Working Paper 106, Manchester: Chronic Poverty Research Centre.
Thornton, P. K., Ericksen, P. J., Herrero, M., and Challinor, A. J. (2014). Climate variability and vulnerability to climate change: a review. Glob Chang Biol. 20, 3313–3328. doi: 10.1111/gcb.12581
USAID (2013). Climate services. Better information for climate-resilient decision making. Washington: USAID
Vaughan, C., Muth, M. F., and Brown, D. P. (2019). Evaluation of regional climate services: learning from seasonal-scale examples across the Americas. Clim. Serv. 15:100104. doi: 10.1016/j.cliser.2019.100104
Wafula, J., Karimjee, Y., Tamba, Y., Malava, G., Muchiri, C., Koech, G., et al. (2018). Probabilistic assessment of investment options in honey value chains in Lamu county, Kenya. Front. Appl. Math. Stat. 4:2018. doi: 10.3389/fams.2018.00006
Walker, J. (2013). Time poverty, gender and well-being: lessons from the Kyrgyz Swiss Swedish health Programme. Dev. Pract. 23, 57–68. doi: 10.1080/09614524.2013.751357
Wallemacq, P., and House, R. (2018). Economic losses, poverty & disasters: 1998–2017. United Nations Office for disaster risk reduction (UNDRR) & Centre for Research on the epidemiology of disasters (CRED).
Wei, X., Qu, H., and Ma, E. (2016). How does leisure time affect production efficiency? Evidence from China, Japan, and the US. Soc. Indic. Res. 127, 101–122. doi: 10.1007/s11205-015-0962-1
Williams, P. A., Karanja Ng'ang'a, S., Crespo, O., and Abu, M. (2020). Cost and benefit analysis of adopting climate adaptation practices among smallholders: the case of five selected practices in Ghana. Clim. Serv. 20:100198. doi: 10.1016/j.cliser.2020.100198
World Meteorological Organization, (2014). Implementation plan of the global framework for climate services Geneva: World Meteorological Organization.
Yegbemey, R. N., and Egah, J. (2021). Reaching out to smallholder farmers in developing countries with climate services: a literature review of current information delivery channels. Clim. Serv. 23:100253. doi: 10.1016/j.cliser.2021.100253
Appendix
Figure A1 illustrates how CIS can benefit the farmer through increased agricultural payoffs. Payoffs depend on CIS use (which we denote by the symbol ) and weather conditions . The dashed line indicates payoffs for a farmer that is not using the recommendations provided by CIS ( , while the solid line indicates payoffs for a farmer that is implementing the recommendations by CIS ( . Under normal weather conditions, payoffs are at their maximum level, and farmers do not need CIS to attain this maximum payoff. When facing moderate weather conditions, , farmers are experiencing reduced payoffs under normal practices, as existing practices and technologies are not sufficient to address the stress imposed on crops and livestock. However, when following the recommendations provided by CIS, a farmer can mitigate some of these losses, and experience the adverse effects of climate risks only once weather conditions become more severe, . In years with extreme weather conditions, , payoffs tend to zero regardless of whether a farmer is using CIS. We will use this simple model for the relationship between payoffs from agricultural activities, weather conditions, and the adoption of CIS to structure our framework for cost–benefit analysis.
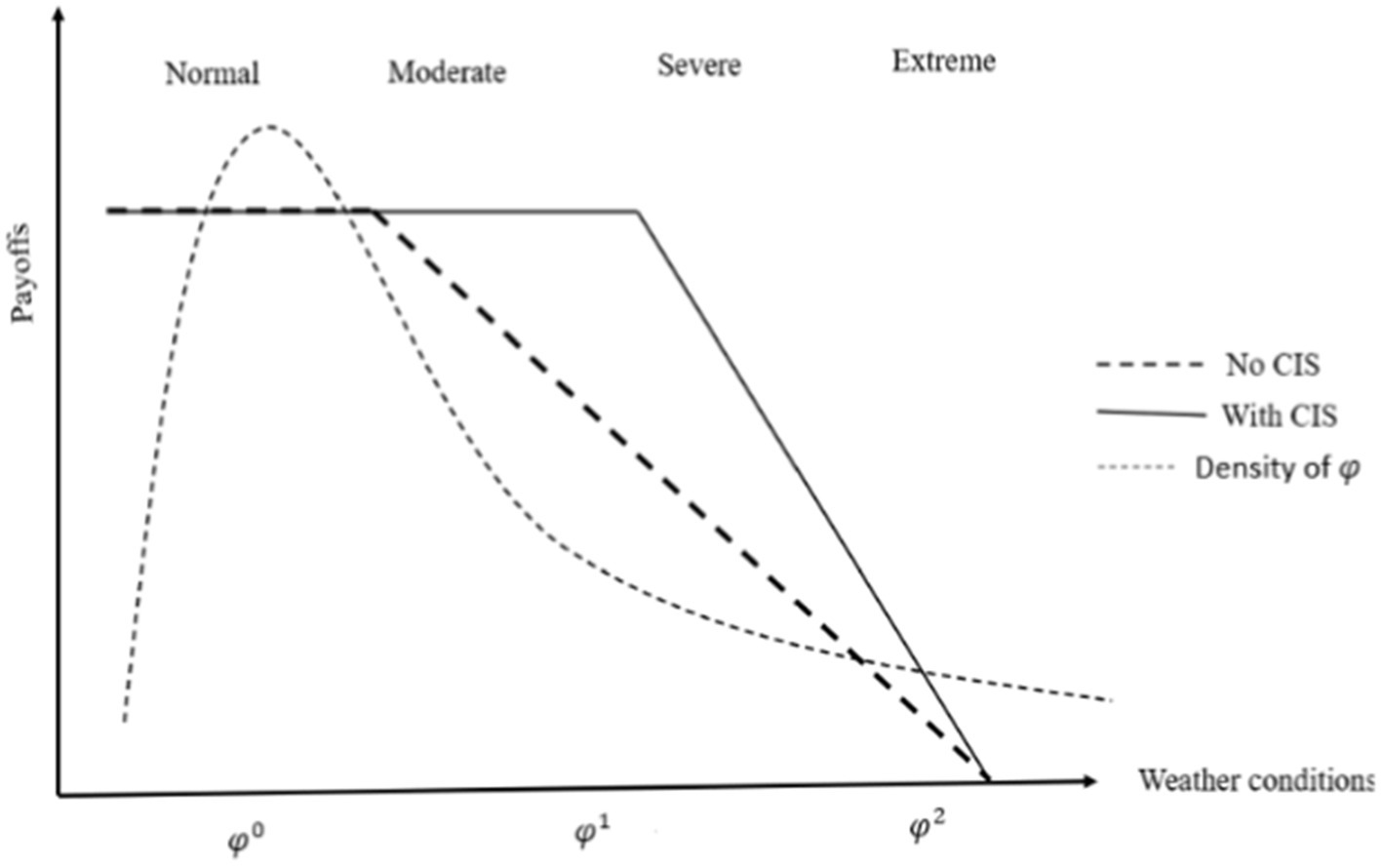
Figure A1. Density of Weather shocks and payoffs due to CIS (Adapted from Kramer and Ceballos, 2018).
Following Figure A1, we assume that for a given level of labor and agricultural investment, income from agricultural production does not depend on whether a farmer adopts CIS-recommended practices in normal or severe states of nature, but under moderate weather conditions, CIS-recommended practices shield agricultural payoffs from losses. Formally, .
Keywords: cost-benefit analysis, climate information services, women’s empowerment, climate resilience, Ghana
Citation: Kramer B, Timu AG and Damba O (2023) Estimating the intrahousehold costs and benefits of innovations to enhance smallholder farmers’ resilience. Front. Sustain. Food Syst. 7:1129419. doi: 10.3389/fsufs.2023.1129419
Edited by:
Julia Compton, Independent Researcher, Cardiff, United KingdomReviewed by:
Munyaradzi Junia Mutenje, International Maize and Wheat Improvement Centre (CIMMYT), ZimbabweStanley Karanja Ng'ang'a, International Center for Tropical Agriculture (CIAT), Colombia
Ruben G. Echeverria, Bill and Melinda Gates Foundation, United States
Copyright © 2023 Kramer, Timu and Damba. This is an open-access article distributed under the terms of the Creative Commons Attribution License (CC BY). The use, distribution or reproduction in other forums is permitted, provided the original author(s) and the copyright owner(s) are credited and that the original publication in this journal is cited, in accordance with accepted academic practice. No use, distribution or reproduction is permitted which does not comply with these terms.
*Correspondence: Anne G. Timu, QS50aW11QGNnaWFyLm9yZw==