- 1Research Institute of Organic Agriculture (FiBL), Frick, Switzerland
- 2Earth and Life Institute, University of Louvain, Louvain-la-Neuve, Belgium
- 3Stanford Doerr School of Sustainability, Stanford University, Stanford, CA, United States
A key strategy of chocolate manufacturers is the promotion of sustainable farming practices amongst their supplying cocoa producers. A growing body of micro-economic literature has analysed factors influencing the adoption of such practices, yet broadly disregarded value chain factors. Information on how factors within single value chains increase the adoption of sustainable farming practices can help direct chocolate companies’ investments and increase return of investments in sustainability. The objective of this study was to understand: (a) how important value chain factors are, relative to farmer and farm factors, for cocoa farmers’ implementation of sustainable farming practices and (b) through which mechanisms value chain factors influence sustainable farming practices implementation. By integrating the practice adoption with sustainable supply chain management literature, we contribute to closing an important research gap. We collected data from 394 cocoa farmers in Ecuador and Uganda and analysed the determinants of implementation sustainable farming practices, testing quantitatively whether value chain factors with variation within single value chains are significantly associated with practice implementation. These factors included information factors (farmers’ access to training; advisory service through the value chain) and structural factors (value chain organisation and persistence; farmers’ dependency on this value chain). We selected 11 sustainable farming practices or indicators across three sustainability dimensions, i.e., environmental, social, and economic. We found that value chain factors are comparable to farmer and farm factors in explaining the implementation of sustainable farming practices across dimensions. Both capacity building and stable relationships were significantly related with the implementation of certain sustainable farming practices. Yet these results were weaker than expected, indicating that their potential was not fully exploited within our case study value chains. Through their value chain sustainability initiatives, chocolate companies should disseminate knowledge, address inhibitors to sustainable farming practices implementation beyond knowledge, and align sustainability goals with all value chain actors.
1. Introduction
Global demand for sustainable chocolate is rising and with it, the pressure on chocolate companies to source sustainably produced cocoa. This has motivated several sustainability initiatives in the cocoa sector. Companies are increasingly sourcing certified cocoa or implementing their own in-house sustainability schemes (Thorlakson, 2018; Fountain and Huetz-Adams, 2020; Perez et al., 2020). A key strategy of both certification and corporate schemes is the promotion of sustainable farming practices or indicators (SFPI) amongst their upstream producers. We define SFPI to cover production practices that contribute to the performance in all three dimensions of sustainability (i.e., environmental, social, and economic).
A growing body of micro-economic literature has looked into factors that influence the adoption of such practices, which is summarised in several recent review studies (e.g., Meijer et al., 2015; Mozzato et al., 2018; Foguesatto et al., 2020; Takahashi et al., 2020). These conclude that the SFPI adoption literature has largely focussed on basic and extrinsic characteristics of the farmer and the farm, such as structure and resource endowments. Additionally, value chain factors, such as relationships between actors, are largely disregarded in existing frameworks (Mozzato et al., 2018). In contrast, the literature on value chain sustainability indicates that desirable (environmental) outcomes can be influenced by value chain partners (Hansson et al., 2019); through information sharing and economic dependencies (Grimm et al., 2014); and through strong and persistent relationships between actors – referred to as “stickiness” (Reis et al., 2020). There is thus a research gap in evaluating the influence of value chain related factors on farmers’ adoption of SFPI (Mozzato et al., 2018; Candemir et al., 2021) and on how value chain partners can best increase knowledge and adoption of SFPI amongst small-scale farmers (Liverpool-Tasie et al., 2020). Furthermore, research on adoption of SFPI in cocoa has looked into environmental practices (e.g., Aneani et al., 2012; Djokoto et al., 2016; Ali et al., 2018), with less attention paid to the social and economic dimensions of sustainability (e.g., Nkamleu and Kielland, 2006; Amfo and Ali, 2020). These questions are important in the cocoa sector as the role of voluntary sustainability standards in transforming the food system towards more sustainability is ever more questioned (Meemken et al., 2021) and as influential downstream value chain actors are increasingly involved and invest in value chain sustainability. Particularly, knowing how factors within single value chains might increase the adoption of sustainable cocoa production practices can help direct investments and improve the cost-effectiveness of investments in sustainable value chains.
The objective of this study was to better understand the relationship between value chain factors and SFPI implementation, specifically aiming to identify if and how value chains influence farming practice adoption. In this study, we define value chain factors as those that describe information flow towards farmers as well as the organisation between and dependency of farmers and other value chain actors and thus contribute to the adoption literature. We posed two main research questions: (1) Relative to farmer- and farm-level factors, how important are value chain factors for cocoa farmers’ implementation of sustainable farming practices?; and (2) Through which mechanisms do value chain factors influence SFPI implementation? Using our existing data set from two samples of cocoa farmers in Ecuador and Uganda, we analyse the determinants of implementation of 11 practices across three sustainability dimensions, testing quantitatively whether value chain factors within single value chains are significantly associated with practice implementation. We do this whilst controlling for farmer and farm factors known to influence practice implementation. By testing the role of value chain factors beyond the environmental dimension of sustainability in two very different cases, we aim to gain an indication of their broader significance and thus global value. This manuscript first provides an overview of relevant literature and the theoretical framework developed for this study, followed by a description of the case studies and selected analytical approaches. We then present the results and discuss them in light of our research questions and existing literature before providing concluding remarks.
2. Conceptual framework
Our conceptual framework combines selected SFPI, i.e., outcome variables, with covariates that might explain their implementation on farms. It is informed by underlying theory and literature on the adoption of SFPI amongst farmers. The conceptual framework for this study is based on the technology adoption and the sustainable value chain literature that links downstream actors with upstream sustainability outcomes. We chose the term practice “implementation” over “adoption,” as many SFPI in cocoa production are traditional production practices and partly a legacy of past management instead of new practices that farmers actively decided to adopt on their farms.
2.1. Sustainable farming practices
Based on our existing identical data set from two producer groups, we identified practices from the environmental, social, and economic sustainability dimensions considered important for sustainable cocoa production (Table 1). We are aware that we do not cover all aspects of sustainability, like gender equality, phytosanitary measures, or living incomes. This was largely due to data limitations, as this study was conceptualised after primary data collection, and maintaining comparability across farms. For example, indicators such as gender equality could only be compared on a sub-sample of farms where both male and female employees were present, and thus was excluded from the analysis. Similarly, we did not identify the practice of “appropriate work for children” as an issue in the Ecuadorian case study, as children on sampled farms were hardly engaged in hazardous work. We recognise potential trade-offs between selected SFPI, such as potential negative effects of pesticide-free production on cocoa yields.
2.2. Theoretical considerations
Our conceptual framework is informed by the expected utility theory (Schoemaker, 1982) and the theory of planned behaviour (Ajzen, 1991). The expected utility theory adopts an economic rationale in which decision-making is based on greatest expected utility. It is considered relevant in the context of economically-constrained farmers who need to manage risk to secure their livelihoods (Meijer et al., 2015). Farmers’ participation in sustainability initiatives and the compliance with respective codes of conduct might be a way to access better prices and thus maximise utility. Yet decision-making is not always purely rational and often influenced through social-psychological pathways, which is what the theory of planned behaviour aims to understand. According to this theory, attitude, subjective norms, and perceived behavioural control shape an individual’s behavioural intentions, which again is considered the closest determinant of behaviour. Participation in sustainability initiatives and the training sessions offered to farmers within these initiatives, and gaining first-hand experiences with SFPI might influence farmers’ attitude towards them and ultimately shape farmers’ intention to implement them. With these theories as a basis, our conceptual framework builds on the agricultural technology adoption literature and the sustainable supply chain literature that links downstream actors with upstream sustainability outcomes.
2.2.1. Agricultural technology adoption literature
A growing body of micro-economic literature has looked into factors that influence farmers’ adoption of sustainable farming practices, mainly regarding agro-environmental practices. The large number of recent review studies is evidence of this trend, each proposing different frameworks based on reviewed studies (Meijer et al., 2015; Liu et al., 2018; Mozzato et al., 2018; Arslan et al., 2020; de Oca Munguia and Llewellyn, 2020; Foguesatto et al., 2020; Piñeiro et al., 2020). Most frameworks distinguish between factors within and factors beyond the farm.
Factors within the farm most commonly include farmers’ socio-demographic factors like age and education level. Past studies have shown a mixed influence of farmer factors on practice adoption. In line with the theory of planned behaviour, farmers with higher education levels or higher awareness about environmental threats, for example, might be more informed about sustainability issues and thus more likely to implement SFPI across all sustainability dimensions (Nkamleu and Kielland, 2006; Boadi-Kusi et al., 2016; Amfo and Ali, 2020). Contrarily, female farm managers might be less likely to implement or adopt a new technology as they might face structural inequalities, such as lower access to education or production factors (Djokoto et al., 2016).
Additionally, farm factors are included in most SFPI adoption frameworks, including farm structure and management. Past studies found that farms’ economic situation positively influences the adoption of environmental, social, and economic practices as a result of greater access to resources and necessary inputs. For example, more resource endowed farmers have shown to be more likely to hire labour and thus involve less children in hazardous tasks (Berlan, 2013; Busquet et al., 2021). Furthermore, a lack of labour can reduce the implementation of labour-intensive environmental practices (Andres et al., 2016). Secure land tenure can furthermore have a positive effect on SFPI adoption and investments in green practices (Useche and Blare, 2013; Yang et al., 2022). Finally, the cocoa variety has been shown to strongly influence environmental practices, as hybrid varieties, such as the Ecuadorian CCN-51, require higher inputs and tolerate less shade (Rueda et al., 2018).
Factors beyond the farm-level include the biophysical, spatial, socio-economic, and policy environment in which farms operate. Proximity to urban centres and markets might increase farmers’ access to information and inputs and thus increase SFP implementation (Foguesatto et al., 2020). Furthermore, social norms and networks can influence SFPI adoption (Liu et al., 2018). Finally, the practice characteristics themselves are important influencing factors. For example, cost-intensive practices might obstruct their adoption (de Oca Munguia and Llewellyn, 2020) especially on low-income smallholder farms. Labour-constrained households might be more willing to adopt labour-saving technologies (Arslan et al., 2020).
2.2.2. Sustainable supply chain literature
Commercial partners within value chains can influence practice adoption amongst farmers (Hansson et al., 2019), for example through incentives or regulatory measures (Piñeiro et al., 2020). Examples include performance-based price premiums and sustainability certification. Cocoa value chains are characterised by a highly diffuse producer base of many small-scale producers and a concentrated downstream actor level, with increasing power (Thorlakson, 2018).
Past research in the value chain literature has focused on compliance of upstream partners with sustainability requirements of downstream companies. Grimm et al. (2014) identified critical factors for achieving supplier compliance, grouping them into factors within and beyond downstream companies. The former includes top management support, which positively influences companies’ commitment and available resources for value chain sustainability mechanisms. The latter includes information sharing and commitment between value chain partners. A large body of literature has shown the importance of information sharing through training and extension for practice adoption in cocoa production systems (e.g., Andres et al., 2016; Denkyirah et al., 2016; Okoffo et al., 2016), often provided by downstream value chain actors.
A recent study linked persistent relationships in value chains with sustainability outcomes under the concept of “stickiness” and showed that more persistent soy value chains in Brazil are more effective in creating change in sustainability performance amongst upstream producers (Reis et al., 2020). Furthermore, upstream actors’ economic dependence might increase compliance with downstream companies’ sustainability codes of conduct (Grimm et al., 2014). As such, farmers with a higher economic dependency on cocoa for their livelihoods, a greater dependency on one buyer with certain sustainability codes of conduct, and generally fewer cocoa buyers might implement more SFPI.
2.3. Selected covariates
As the literature review displays, a great range of factors have been shown to influence practice implementation or adoption. The selection of groups of factors and specific factors to include in our analysis was restricted by our existing data set. To assess the importance of value chain factors for the adoption and implementation of sustainable cocoa production practices, we controlled for other factors known to influence their implementation. We organised factors influencing SFPI into two groups of internal and external factors (Figure 1). An overview of factors and rationale for their inclusion can be found in the supplementary materials.
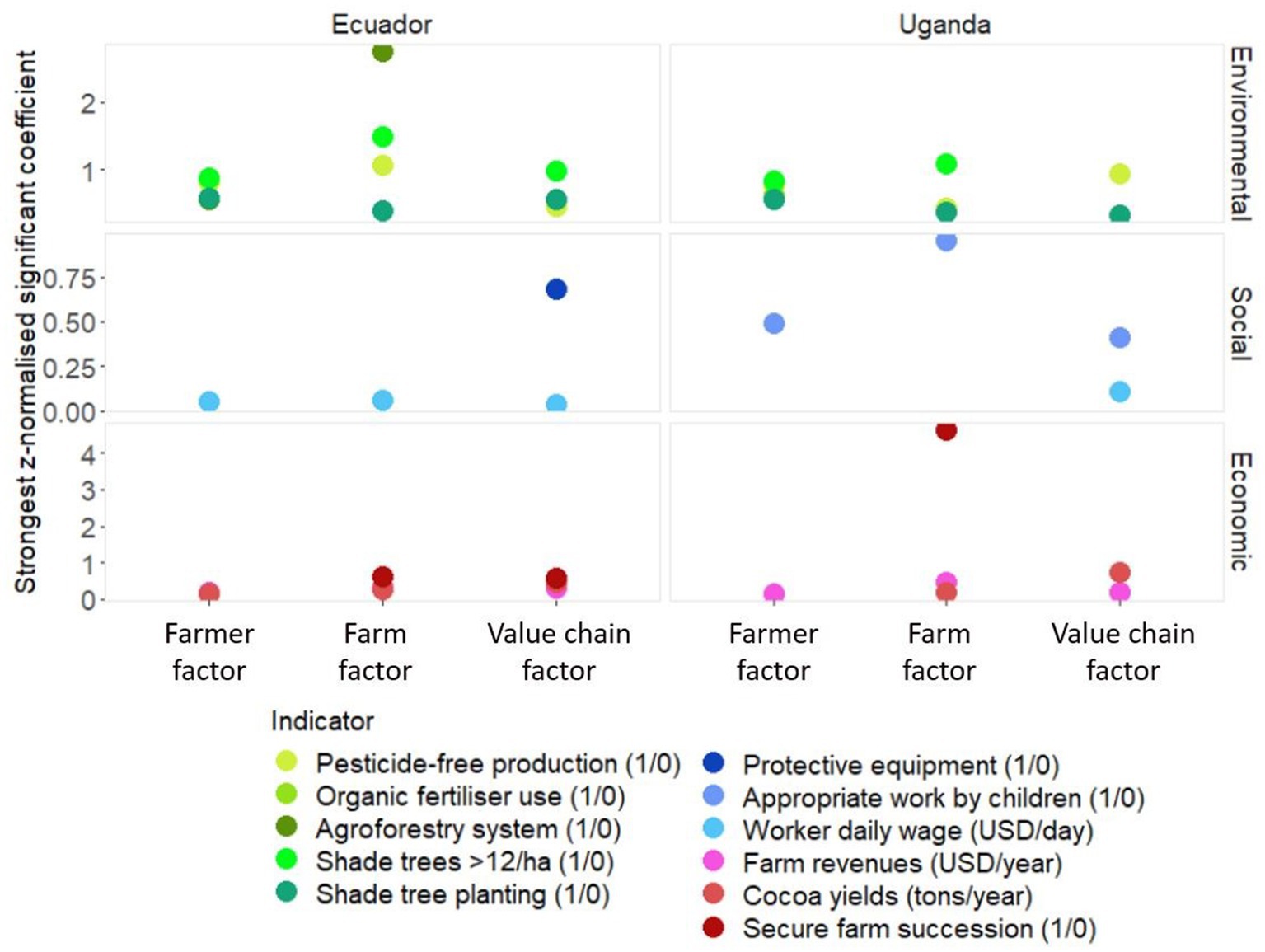
Figure 1. Comparison of strongest z-normalised significant coefficient among farm, farmer, and value chain factors for each sustainable farming practice and country.
2.3.1. Internal farmer factors
The set of farmer factors controlled for included farmers’ age, gender, and formal education years. We additionally incorporated farmers’ expressed commitment to sustainability (dummy) and knowledge about climate change (dummy). Given the literature shown above, we hypothesise that farmer factors, specifically farmers’ knowledge and conviction, are highly important for the decision to implement SFPI in our case studies (Hypothesis 1).
2.3.2. Internal farm factors
Incorporated farm factors comprised multiple indicators for farms’ economic endowment, including farm and cocoa plot size (hectares), land ownership (dummy), and livestock units owned (number). Labour availability on farm was covered by the number of family workers. In the Ecuadorian case study, we added the hybrid cocoa variety CCN-51 as a predictor (dummy). Given the mixed picture presented in past studies, we assume that farm factors are less important for SFPI implementation (Hypothesis 2).
2.3.3. Value chain factors
We considered both information sharing and organisation as important value chain variables. Within information factors, we included the number of training days farmers participated in (days/year) and farmers’ perceived access to extension services (dummy). These two variables account for farmers’ access to private training and advisory service organised by downstream value chain actors. Additionally, we considered factors that describe the value chain organisation and persistence, adapting the stickiness definition by Reis et al. (2020) to our cross-sectional data. We included farmers’ economic dependency on cocoa (share of gross farm revenue from cocoa) and their main customer (share of gross farm revenue from main buyer). Furthermore, we included the number of cocoa buyers per farmer and the years of relationship with the main cocoa buyer. Based on first evidence reviewed above, we hypothesise that downstream value chain actors have several mechanisms available with which they can generate a change in SFPI implementation at farm level, thus value chain factors are important for SFPI implementation (Hypothesis 3). We specifically tested two mechanisms: (a) Information factors, covering farmers’ access to capacity building through the value chain, which we presume to be a suitable mechanism for downstream value chain actors to increase SFPI implementation amongst their suppliers (Hypothesis 4); and (b) Structural factors, including value chain organisation and persistence as well as farmers’ dependency on this value chain. Our final hypothesis follows the assumption that the mechanism of establishing long-term and stable relationships along value chains create trust and thus increase SFPI implementation (Hypothesis 5).
3. Materials and methods
3.1. Case study description
We addressed our research questions and related hypotheses with a comparative case study approach. In order to get comprehensive insights into the cocoa sector, we selected two diverse value chains. They connect cocoa farmers in Ecuador and Uganda to downstream Swiss chocolate companies.
3.1.1. Ecuador
Ecuador is the world’s fifth largest producer of cocoa, with almost 330,000 tonnes produced in 2020 (FAO, 2022a), and is the largest producer of fine flavour cocoa, known in Ecuador as Cacao Nacional (Anecacao, n.d.). The majority of the 527,347 ha of land used for cocoa cultivation in Ecuador in 2020 (FAO, 2022b) was located in the coastal area (INEC, 2020), which is also the location of the sampled farmers in this case study.
The Swiss chocolate company at the downstream end of this cocoa value chain is a large multinational chocolate manufacturer, which sources cocoa from Ecuador through an in-house sustainability programme. This programme was introduced in the country in 2014 and has gradually increased in size, including almost 6,000 independent farmers in 2019. Farmers are grouped around intermediaries in the closest town, which also form part of the sustainability programme. Finally, a large multinational trading company buys cocoa from intermediaries and exports it to Europe. The exporter is also in charge of implementing the sustainability programme, mainly focussing on cocoa traceability, farmer training, in-kind premium distribution, and community development. Farmers receive “normal” market prices for their cocoa, dependent on its quality and humidity content. They additionally receive in-kind premiums, which included mineral fertilisers, fungicides, or tools. Programme farmers are not contractually obliged to sell their cocoa to programme intermediaries, yet no longer receive premiums if they frequently sell elsewhere. Farmers in the programme undergo several training modules with a strong focus on good agricultural practices and environmental protection. Each intermediary group, ranging in size from 100 up to 600 farmers, generally has one farmer trainer. Most trainings sessions are held at the intermediary shop in town and farmers are motivated to participate in these sessions as they are combined with the distribution of premiums. Farmer trainers rarely pay additional visits on individual farms given the high number of farmers.
3.1.2. Uganda
In comparison to Ecuador, Uganda had a much smaller cocoa production in 2020 of 35,000 tonnes, harvested from 70,809 ha (FAO, 2022b). National production quantities and export values, however, have been increasing steadily (FAO, 2022b). Major cocoa producing areas in Uganda are Bundibugyo in the Western and Mukono in the Central Region, the latter being the location of the cocoa producers in our second case study value chain.
In this case study, around 500 independent farmers in Mukono District have been converting to certified organic production for three years at the time of data collection. In 2017, a national export company searching to increase its supplier base in Mukono District recruited farmers based on a door-to-door method asking for their willingness to comply with organic regulation in exchange for higher cocoa prices. This export company started the certification process with the promise to buy farmers’ cocoa and vanilla with a price premium once certified. For organic certification, the export company established an internal control system, which includes yearly controls on farms to ensure their compliance with the organic standard. In order to provide knowledge about certification and organic production practices, a training programme with four modules was initiated. Farmers were invited to participate in trainings. The export company hired two farmer trainers to cater to the group of farmers in Mukono District, who are in charge of the trainings and compliance control. The downstream Swiss chocolate brand is relatively young and small, and caters to a niche market of sustainable chocolate consumers. The owners personally know the lead farmer and the conditions in which farmers in Mukono District live and operate.
3.2. Farmer sampling and data collection
Farmer sampling followed a randomised approach in both case studies, targeting sample sizes of around 200 farmers, which was feasible within the project framework. We selected a random sample of farmers within each case study. In Ecuador, we selected eight intermediary groups in four provinces of north-western Ecuador and then randomly selected a subsample of 25 farmers per group, totalling 190 farmers. In Uganda, we made a random selection of 204 farmers across the entire farmer group of around 450 farmers.
Trained enumerators and the lead author visited the selected farms between July and September 2019 in Ecuador and February and March 2020 in Uganda to undertake face-to-face interviews with farm managers [more details in Tennhardt et al. (2022)]. At each farm, we applied the SMART-Farm Tool (Schader et al., 2016, 2019) as described in Tennhardt et al. (2022), to derive a large indicator pool on farm management and sustainability indicators. In addition, we collected contextual data on the farmer, farm, and the cocoa supply chain. We collected all information for the reference years of 2018 in Ecuador and 2019 in Uganda. Data collection was performed in accordance with all relevant institutional and national ethical guidelines. It followed free and informed consent by farmers, which was obtained orally from respondents and documented with a signature in a participation list.
3.3. Data analysis
We ran multivariate mixed regression models for each case study, using individual practices as dependent variables and the set of predictors including value chain factors as explanatory variables. Dependent variables were binary and continuous (Table 1) and thus required different types of regression models. In each model, we checked for multicollinearity using variance inflation factors and deleted predictors with values >3. We z-normalised all predictors to facilitate comparison between predictors with differing scales (Bruce et al., 2020).
We approached the estimation of value chain factors’ importance for SFPI implementation being aware that certain predictors might be endogenous to dependent variables and reverse causality might exist, potentially leading to biased estimates. Thus, we refrained from interpreting any result as impacts and any mention of an effect refers to a change in probability, not to a causal effect. Additionally, we do not use data to predict adoption but rather explain current implementation.
We controlled for a potential “village bias,” i.e., a potential correlation amongst close-by farms due to information exchange amongst neighbours, locally-specific training offers, or local conditions such as topography or road infrastructure. Due to the uneven distribution of farms per village, especially in the Ugandan case, not all mixed models converged when adding the village as a random effect. Therefore, we developed a standard procedure, which was applied to all models: First, we fit each model with only village as a random effect and without other covariates. If the simple model converged, we added all covariates as fixed effects and kept village as a random effect. If the full model converged, we kept it and interpreted the results. If the full model did not converge after the second step, we introduced the village as a fixed effect instead of as a random effect and checked if the village had a significant effect. If the village showed a significant effect, we kept it and interpreted the results. If the village did not show a significant effect, we removed it as a fixed effect and interpreted the estimates of the model without village. If the simple model did not converge after the first step, we introduced the village as a fixed effect instead of as a random effect and checked if the village had a significant effect. If the village was significant, we kept it and interpreted the results. If the village was not significant, we removed it as a fixed effect and interpreted the estimates of the model without village. This approach was considered the best middle ground between accounting for a potential village bias where possible and simplifying the models where necessary.
All statistical analyses were performed in R (vers. 4.1.0, R Project for Statistical Computing, RRID:SCR_001905), via RStudio (vers. 2022.02.01 + 461, RStudio, Q19 RRID:SCR_000432). The analysis was implemented in RStudio’s RMarkdown script format, which integrates analysis, reporting, and export functions for highly reproducible research reports (Baumer and Udwin, 2015). Data and code are available here.1
3.3.1. Binary dependent variables
Most of the SFPI in our database had binary response options, i.e., were applied (=1) or not applied on a farm (=0). This dichotomous division is typical in studies that aim at modelling the adoption of agricultural practices (Foguesatto et al., 2020) and allows for a clear differentiation between farms that implemented and farms that did not implement a certain practice. We modelled the effects of predictors on binary SFPI using generalised linear mixed models (GLMM) in case the model converged with the village variable introduced as a random effect, and generalised linear model (GLM) otherwise, after confirming the insignificance of the fixed variable “village” (see supporting information). We used glmmTMB() function of the R package glmmTMB for GLMM including village as random effect, glm() function of the R package stats for GLM, and Anova() from the R package car to test for significance of “village” as fixed effect.
3.3.2. Continuous dependent variables
We employed linear mixed regression models (LMM) for continuous dependent variables and log-transformed dependent variables due to skewed data. In those cases where the mixed models did not converged with the village variable as a random effect, we applied Ordinary Least Square (OLS) regression. We used the lmer() function of the R package lme4 for LMM including “village” as random effect and the lm() function of the R package stats for OLS models.
3.3.3. Simultaneous models
Some SFPI selected in this study are related and not entirely independent of other selected SFPI. As an example, the dependent variable cocoa yields might influence the dependent variable farm revenues. Not accounting for these interdependencies generates a potential bias (Grovermann et al., 2023). In order to test for this bias and verify the robustness of the results of the isolated models, we ran simultaneous models. We estimated multivariate probit models for binary dependent variables with interdependency (i.e., agroforestry systems, shade tree planting, and shade tree density). Furthermore, we estimated seemingly unrelated models for continuous dependent variables with interdependency (i.e., gross farm revenues and cocoa yields). The results of these models are presented in the supplementary materials.
4. Results
4.1. Descriptive statistics results
Table 2 shows the descriptive results of the dependent variables analysed in the environmental, social, and economic dimensions of sustainability as well as the independent farmer, farm, and value chain variables. Most dependent and independent variables show a distribution suitable for further analyses with regression models, with some exceptions: organic fertiliser use in Ecuador and engagement of children in hazardous work was low and further regression analyses were not carried out. Similarly, the use of protective equipment was low and growing cocoa in agroforestry systems was high in Uganda. All farms in the Ecuadorian sample owned some sort of livestock and we excluded this predictor from the regression models for this case study.
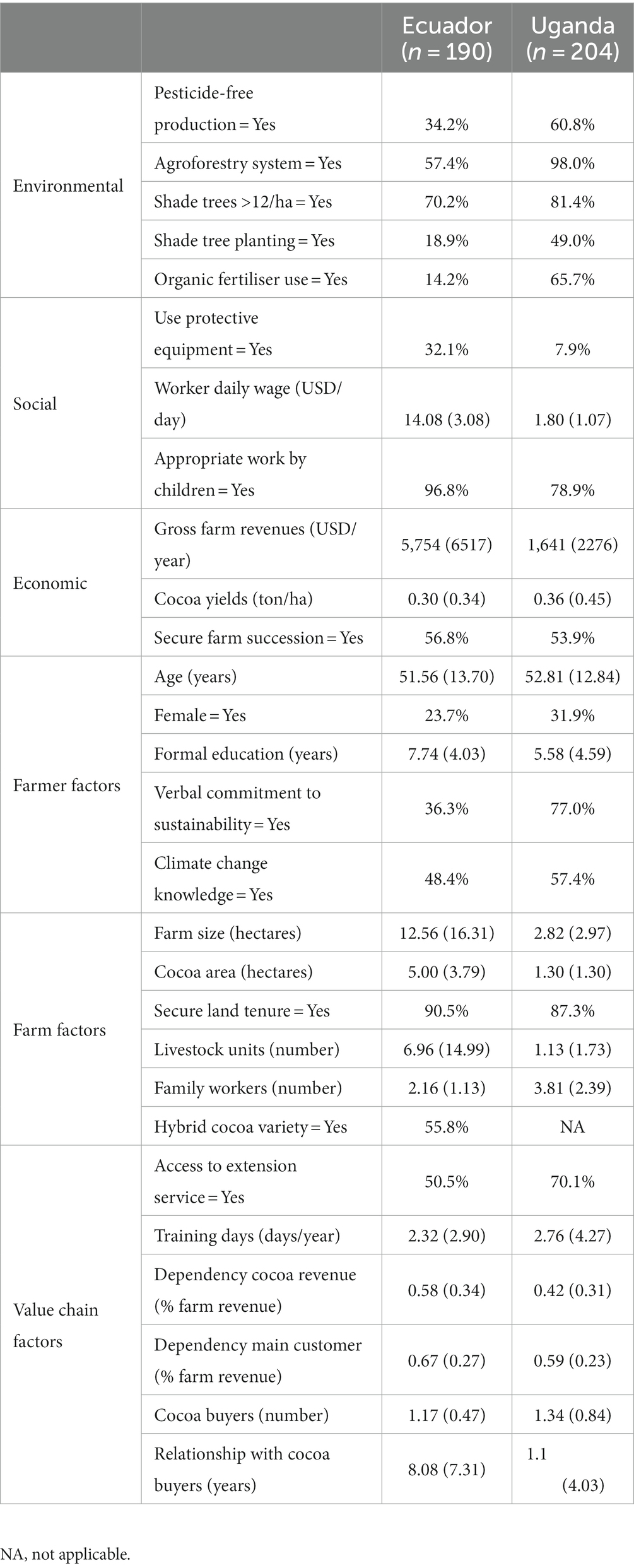
Table 2. Mean values (standard deviation) and percentages of dependent variables and predicting factors for sustainable practice implementation in cocoa.
4.2. Relative importance of value chain factors (hypotheses 1, 2, and 3)
The results of the different regression models testing the influence of value chain variables on SFPI implementation in the environmental dimension for the Ecuadorian and Ugandan case studies are presented in Tables 3, 4. The results for the social and economic dimensions for the Ecuadorian and Ugandan case studies are shown in Tables 5, 6. Furthermore, the strongest coefficient (β) of significant predictors per factor group and SFPI are shown in Figure 2.
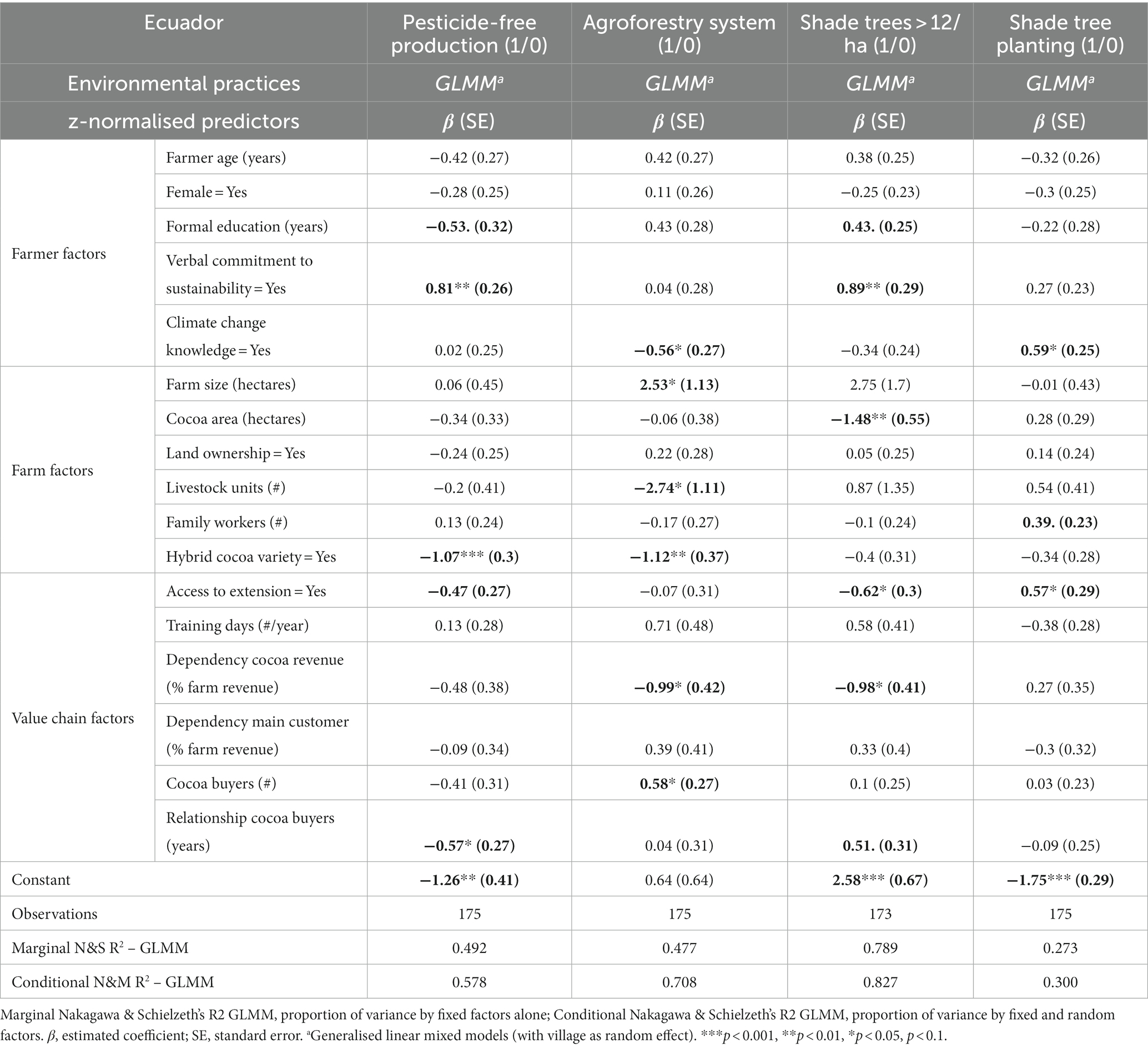
Table 3. Multivariate mixed regression models for sustainable farming practices and indicators in cocoa production systems in Ecuador – results for practices in the environmental dimension.
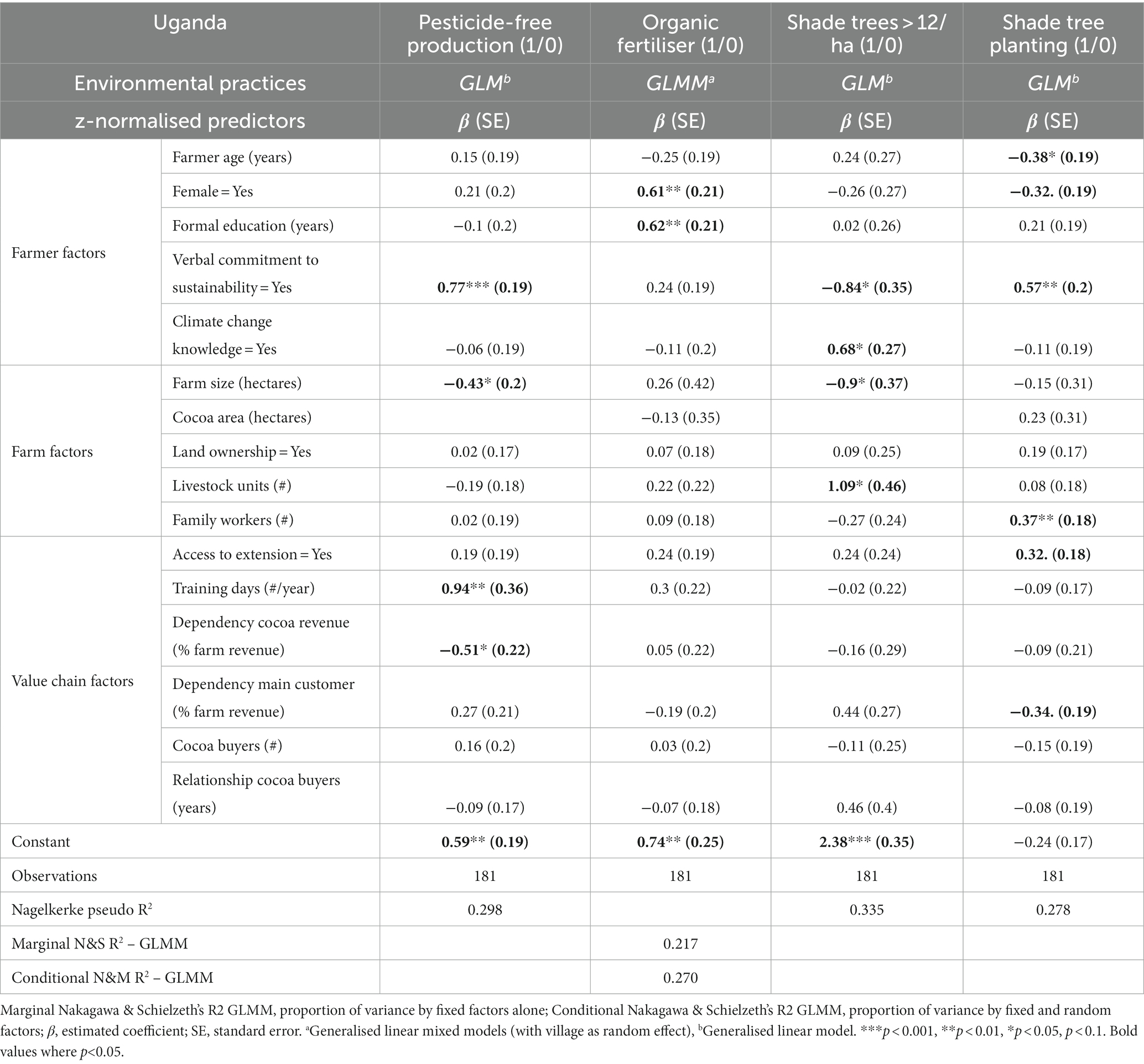
Table 4. Multivariate mixed regression models for sustainable farming practices and indicators in cocoa production systems in Uganda – results for practices in the environmental dimension.
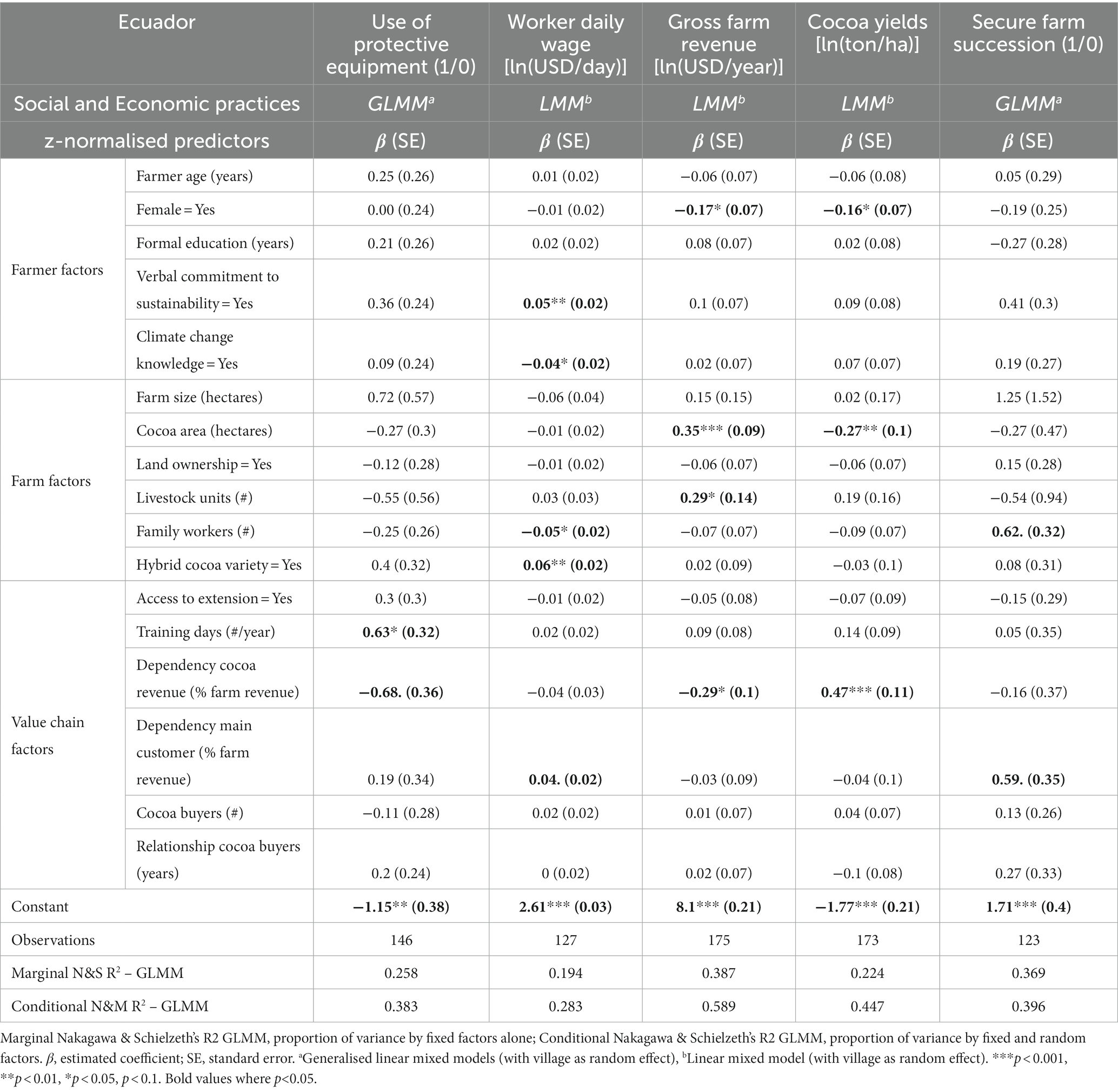
Table 5. Multivariate mixed regression models for sustainable farming practices and indicators in cocoa production systems in Ecuador – results for practices in the social and economic dimensions.
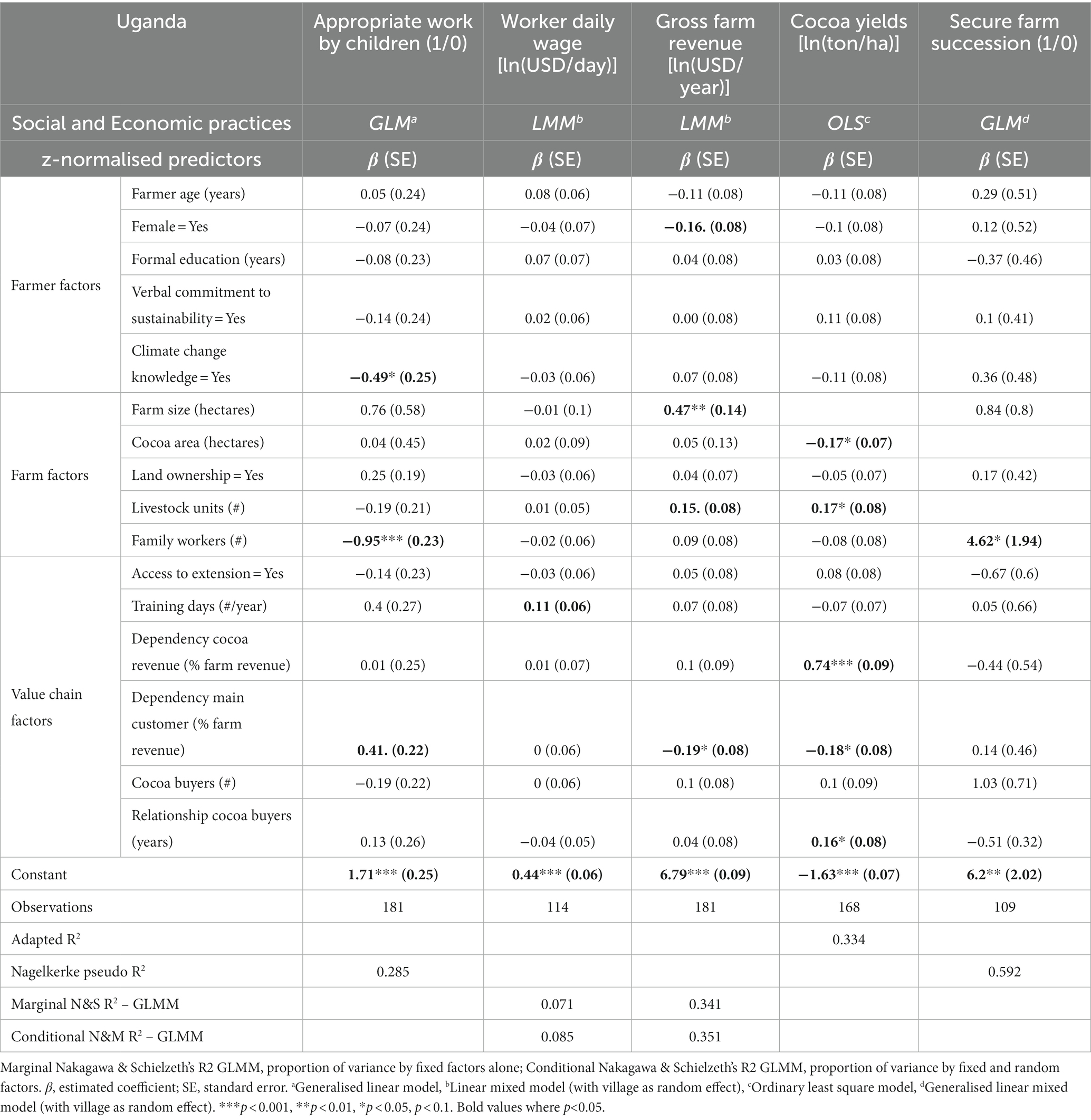
Table 6. Multivariate mixed regression models for sustainable farming practices and indicators in cocoa production systems in Uganda – results for practices in the social and economic dimensions.
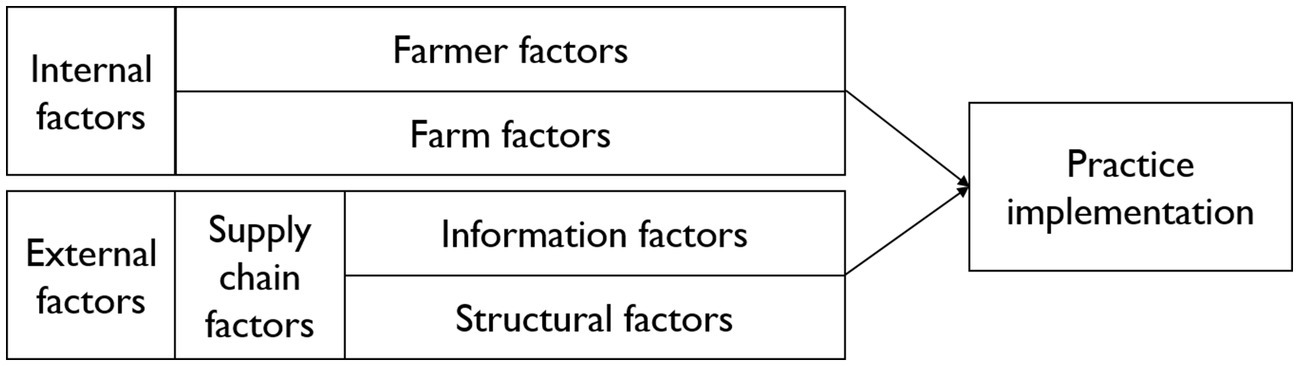
Figure 2. Conceptual framework of factors influencing sustainable practice implementation on cocoa farms.
4.2.1. Practices within the environmental dimension
Within the Ecuadorian case study, factors from all three groups of predictors showed significant relationships with environmental SFPI (Table 3). We found strong predictors amongst the group of farm factors (Figure 2). Farm size showed a significant and positive relationship with growing cocoa in agroforests (GLMM, β = 2.53, n = 175, p < 0.05), yet larger cocoa plots were significantly and negatively associated with shade tree density (GLMM, β = -1.48, n = 173, p < 0.01). Growing the hybrid cocoa variety CCN-51 was significantly and negatively related to growing cocoa in agroforestry systems (GLMM, β = −1.12, n = 175, p < 0.01) and pesticide-free production (GLMM, β = −1.07, n = 175, p < 0.001). Holding all other variables constant, the odds for farms growing hybrid cocoa to practice pesticide-free cocoa production were 66% lower than for farmers not growing hybrid cocoa. The farmer factor farmers’ commitment to sustainability also showed a significant and positive association with pesticide-free production (GLMM, β = 0.81, n = 175, p < 0.01) and shade tree density (GLMM, β = 0.89, n = 175, p < 0.01). The strongest value chain factor with a coefficient of −0.99 was farmers’ dependency on cocoa revenues, which showed a significant negative relationship with agroforestry systems (GLMM, n = 173, p < 0.05).
Also in the Ugandan case study, all three groups of predictors showed significant relationships with environmental SFPI (Table 4). The number of training days represented the strongest value chain factor, which was significantly and positively associated with pesticide-free production (GLM, β = 0.94, n = 182, p < 0.01). This indicates that with an increase of 4.3 training days and holding all other variables constant, the odds of producing pesticide-free cocoa increased by 156%. The farmer factor commitment to sustainability showed a significant and positive relationship with pesticide-free production (GLM, β = 0.77, n = 182, p < 0.001) and shade tree planting (GLM, β = 0.57, n = 182, p < 0.01), yet a negative relationship with shade tree density (GLM, β = −0.84, n = 182, p < 0.05).
4.2.2. Practices within the social dimension
Our covariates showed rather weak predicting power for the implementation of social SFPI amongst sampled Ecuadorian farmers (Table 5). Farmers reported that they had received some personal protective equipment, such as rubber boots, through the sustainability programme. Training participation was significantly and positively associated with the use of protective equipment (GLMM, β = 0.63, n = 146, p < 0.05). The average daily wage for a farm worker in this case study was 14 USD. As reported by farmers, wages partly depend on the tasks (e.g., manual weeding receives a lower wage than using an electric grass cutter due to the higher risk involved), the gender of the worker with women often receiving lower daily wages, and farmers’ ability to pay. Farmers’ commitment to sustainability showed a significant and positive relationship with wages (LMM, β = 0.05, n = 127, p < 0.05).
Workers on farms in the Ugandan sample received on average 1.8 USD per day. Ugandan farmers mostly pay workers per task, requiring an estimation of the associated workload. Training days were significantly and positively associated with paid wages (LMM, β = 0.11, n = 114, p < 0.1). Furthermore, most farms in this case study included children up to 16 years of age in farming activities. For the vast majority of children, farm work did not impair their school assistance or performance as they engaged during school holidays or the weekend. However, on 21% of sampled farms, children were involved in hazardous work like using sharp tools or spraying pesticides. The number of family workers as farm factor showed the strongest relationship with this outcome variable (GLM, β = −0.95, n = 181, p < 0.001). Farmers’ dependency on their main customer, contrarily, showed significant and positive relationships with appropriate work by children (GLM, β = 0.41, n = 181, p < 0.1).
4.2.3. Practices within the economic dimension
All factor groups showed significant relationships with economic SFPI within our Ecuadorian sample (Table 5; Figure 2). Female farmers had significantly lower gross farm revenues and cocoa yields (LMM, β = −0.17 and − 0.16, n = 173, p < 0.05). Whilst the number of livestock units and cocoa plot size showed a significant and positive relationship with gross farm revenues (LMM, β = 0.29 and 0.35, n = 175, p < 0.1), cocoa plot size showed a significant and negative relationship with yields (LMM, β = −0.3, n = 175, p < 0.05). Finally, farmers’ dependency on cocoa revenues showed a significant and negative relationship with gross farm revenues (LMM, β = −0.29, n = 175, p < 0.01), yet a significant and positive relationship with cocoa yields (LMM, β = 0.47, n = 173, p < 0.001). This indicates that, holding all other variables constant, with a 34% increase in the share of farm revenues from cocoa, cocoa yields increase by 60%.
The results of the Ugandan case study mirror several findings from the Ecuadorian data (Table 6). As such, the share of revenues generated by cocoa showed a significant and positive relationship with cocoa yields (LMM, β = 0.74, n = 164, p < 0.001), female farmers generated lower gross farm revenues (LMM, β = −0.16, n = 164, p < 0.1), and farm size and the number of livestock units were significantly and positively related to gross farm revenues (LMM, β = 0.47 and 0.15, n = 164, p < 0.1).
In summary, we found the largest number of significant relationships within our models estimating SFPI implementation in the environmental dimension, with lowest explanatory power of our models estimating SFPI implementation in the social dimension (Figure 2). The relative importance of value chain factors to explain SFPI implementation was similar to the importance of intrinsic farmer and farm factors within all sustainability dimensions.
4.3. Value chain mechanisms that influence SFPI implementation (hypotheses 4 and 5)
We tested the relationship of two value chain factor subgroups, i.e., mechanisms of value chain influence, and SFPI implementation at farm level. These were information and organisational factors.
4.3.1. Information factors
Within both samples, few information factors were significantly associated with the implementation of SFPI. Amongst Ecuadorian farmers, training days showed a significant and positive relationship with the use of protective equipment (GLMM, β = 0.63, n = 173, p < 0.05, Table 5) and access to advisory service was negatively associated with pesticide-free cocoa production (GLMM, β = −0.47, n = 173, p < 0.1, Table 3). Amongst Ugandan farmers, training days were significantly and positively associated with pesticide-free production (GLM, β = 0.94, n = 182, p < 0.01, Table 4). In Ecuador (GLMM, β = 0.57, n = 173, p < 0.05, Table 3) and Uganda (GLM, β = 0.32, n = 181, p < 0.1, Table 4), farmers with access to extension were more likely to plant shade trees in their cocoa plots. In conclusion, we found limited evidence for hypothesis 4 that information sharing and capacity building along value chains increases the implementation of a large number of SFPI at farm level.
4.3.2. Organisation factors
Organisation factors showed some important significant relationships with SFPI implementation. The most significant predictor was farmers’ dependency on cocoa revenues, which showed several significant relationships. In the Ecuadorian case, farms with greater dependency on cocoa were less likely to have a shade tree density of above 12 trees per hectare (GLMM, β = −0.98, n = 173, p < 0.05, Table 3), grow cocoa in agroforestry (GLMM, β = −0.99, n = 173, p < 0.05), use personal protective equipment (GLMM, β = −0.68, n = 146, p < 0.1, Table 5), and had lower gross farm revenues (LMM, β = −0.29, n = 175, p < 0.01), yet higher cocoa yields (LMM, β = 0.47, n = 173, p < 0.001). This trend was partly mirrored in the Ugandan case, where dependency on cocoa revenues was significantly and positively associated with cocoa yields (OLS, β = 0.74, n = 168, p < 0.001, Table 6) and showed a significant negative relationship with pesticide-free production (GLM, β = −0.51, n = 182, p < 0.05), whilst dependency on the main customer was significantly and negatively associated with shade tree planting (GLM, β = −0.34, n = 181, p < 0.1, Table 4). Long-term relationships with cocoa buyers were significantly and negatively associated with pesticide-free production in Ecuador (GLMM, β = −0.57, n = 175, p < 0.05, Table 3) but showed a significant positive relationship with cocoa yields in the Ugandan case (OLS, β = 0.16, n = 168, p < 0.05, Table 6). These results indicate that value chain organisation and stability can influence SFPI implementation at farm level providing evidence for hypothesis 5.
5. Discussion
Our study tested the relative importance of external value chain factors compared to internal farmer and farm factors in explaining SFPI implementation on cocoa farms across the environmental, social, and economic dimensions of sustainability. It furthermore examined two specific mechanisms within value chains for their relationships with SFPI implementation, namely information provision through and organisation of the value chain. We observed strong differences across practices and sustainability dimensions in terms of which factors influenced SFPI implementation. Despite some differences between case studies, we also identified a few reoccurring important factors. This suggests that the factors influencing adoption of SFPI are partly context dependent and cannot be generalised across the board. As a result, the supporting evidence for the various hypotheses was more nuanced and contextual. All three groups of factors showed significant relationships with the implementation of SFPI across sustainability dimensions, yet were strongest for environmental SFPI. We discuss these results in detail in relation to our original hypotheses below.
5.1. Relative importance of value chain factors
5.1.1. Intrinsic farmer factors are important for SFPI implementation (Hypothesis 1)
From the farmer factor group, significantly associated predictors mainly cover farmers’ intrinsic motivation and go beyond SFPI implementation in the environmental dimension. These results confirm the importance of intrinsic and attitudinal factors for SFPI implementation (Meijer et al., 2015; Bijani et al., 2017; Dessart et al., 2019; Bazrafkan et al., 2022).
5.1.2. Farm factors show mixed results for SFPI implementation (Hypothesis 2)
Our models included multiple factors that represent farmers’ economic endowment, which have been shown to be positively associated with practices that require financial investments (Arslan et al., 2020). We observed some important relationships in our results, such as farm and cocoa plot size and the number of livestock units, which were positively related to gross farm revenue generation in both case studies. Remarkably, no significant relationship was identified between farm economics and the use of personal protective equipment, as previously found by Boadi-Kusi et al. (2016), Okoffo et al. (2016), and Owombo et al. (2014). This indicates that farmers’ economic situation is not solely responsible for SFPI implementation. Our results for Uganda furthermore support the notion that the relationship between farms’ economic endowment and hazardous child labour is not unidirectional and more complex (Berlan, 2013; Busquet et al., 2021). In our case, larger households with more members involved in farm work were more likely to involve children in hazardous tasks, which is contrary to past findings from Côte d’Ivoire (Nkamleu and Kielland, 2006). Finally, cocoa yields decreased with increasing cocoa plot sizes in both case studies, in line with past findings (Bymolt et al., 2018).
Current demographic changes are affecting cocoa farming systems, as farmers report ageing managers and labour shortage (Mithöfer et al., 2017). This especially affects SFPI that are considered labour intensive, such as good cocoa management practices (Armengot et al., 2019) or agroforestry systems (Armengot et al., 2016). Farms with more family workers in our samples were more likely to implement the laborious task of shade tree planting and have a secure farm succession. This is in line with studies from Atube et al. (2021), who found that larger household sizes were positively associated with the adoption of labour intensive farming practices in Uganda. More generally, however, and in light of recent demographic changes in cocoa growing communities, the relationship between labour availability and SFPI implementation should receive additional attention.
Finally, our results mirror prior research on the role of the hybrid cocoa variety CCN-51, which is becoming more common amongst Ecuadorian cocoa farmers due to its relative disease resistance and higher productivity (Boza et al., 2014). Farmers with the hybrid cocoa variety CCN-51 were more likely to apply synthetic pesticides and grow cocoa in full-sun systems compared to the fine flavour cocoa variety, in line with past findings (Bentley et al., 2004; Blare and Useche, 2013; Middendorp et al., 2018; Rueda et al., 2018). Contrasting these past findings, growing hybrid cocoa was not accompanied by economic co-benefits in our case study.
5.1.3. Value chain factors are comparatively important for SFPI implementation (Hypothesis 3)
Our results highlight the importance of all predictor groups for SFPI implementation in the environmental dimension, supporting past research on the importance of value chains for environmental decision-making at farm level (Hansson et al., 2019; Liverpool-Tasie et al., 2020). Our results also highlight a similar importance of value chain factors and intrinsic farm and farmer factors in the social and economic dimensions, which was not known before.
5.2. Value chain mechanisms that influence SFPI implementation
5.2.1. Information can be a relevant factor for SFPI implementation (Hypothesis 4)
In general, value chain factors regarding information flow showed few relationships with SFPI implementation, and these could be explained based on existing farmer training programmes. In the Ecuadorian case, the sustainability programme promoted the use of personal protective equipment and these were handed to farmers during trainings. Past studies also found clear relationships between access to extension service and the use of protective equipment amongst cocoa farmers in Ghana, Nigeria, and Cameroon (Owombo et al., 2014; Boadi-Kusi et al., 2016; Okoffo et al., 2016). In this value chain, the Swiss chocolate company’s in-house sustainability programme is the only source of advisory service or training on cocoa production for most of the sampled farmers. Governmental advisory services were scarce and farmers’ level of organisation for joint commercialisation was low, as is the case amongst Ecuadorian cocoa farmers in general (Huetz-Adams et al., 2016). This highlights the important role of corporate capacity building in delivering services to farmers that are underprovided by the state.
In the Ugandan case, farmers who had participated in more training days were more likely to produce pesticide-free cocoa despite our observations that all farmers had access to agro-shops and thus pesticides. This is in line with farmers’ conversion to organic certification at the time of data collection as the restriction of synthetic pesticides was an important topic in the export company’s trainings to prepare farmers for the upcoming audit. However, since training participation is voluntary, causality could be reversed due to positive selection bias, with farmers who used no or few pesticides more eager to take part in trainings. Voluntary training participation and the delay in payment of price premiums might also explain that almost 40% of sampled farmers were not complying with organic regulation. Low compliance rates have been reported from certified cocoa producers in Ghana, pointing to the need to further invest in capacity building and compliance verification (Ansah et al., 2020; Schader et al., 2021). Regardless, our results suggest that farmer training can be an important aspect for SFPI adoption, in line with past studies that identified positive effects of training or cooperative membership on practice implementation (e.g., Effendy et al., 2019; Piñeiro et al., 2020; Adebayo et al., 2021; Musafiri et al., 2022).
Our results did not show a relationship between training and advisory service and other SFPI, despite Ecuadorian farmers receiving training on topics such as cocoa management practices to increase productivity and growing cocoa in agroforestry systems for product diversification. This indicates implementation constraints beyond knowledge. For example, Useche and Blare (2013) found that Ecuadorian farmers who prioritised a fast economic return rather grew cocoa in monoculture systems.
5.2.2. The value chain organisation can be an important factor for SFPI implementation (Hypothesis 5)
The relationships between value chain organisation factors and SFPI implementation observed in our samples were weaker than expected and sometimes even negative. In the Ecuadorian case, long-term relationships with intermediaries reduced the likelihood of pesticide-free cocoa production. This could in part be due to the double agency role of intermediaries, as both buyers of cocoa and sellers of inputs. Reports from farmers suggest that long-standing relationships with local intermediaries facilitated the receipt of loans or inputs, which farmers repay with the next cocoa harvest. For pest or disease management, many Ecuadorian cocoa farmers seek advice from input providers (Blare and Useche, 2014), highlighting their importance for reducing synthetic pesticide use in cocoa. Reis et al. (2020) demonstrated that stable relationships between value chain partners can improve the sustainability performance by comparing between value chains. Our results indicate that long-term relationships within single value chains can also influence SFPI implementation, yet only when all actors involved share the same sustainability goals. In our two cases, downstream chocolate manufacturers aimed to reduce pesticide residues in cocoa and thus their use on farms, whilst input-supplying intermediaries distributed synthetic pesticides and provided loans for them.
Our results also highlighted significant relationships regarding farmers’ economic dependency. In the Ecuadorian case, farms’ dependency on cocoa revenues was negatively associated with gross farm revenues. At the time of data collection, cocoa prices in Ecuador were relatively low and some farmers had already or planned to switch to more attractive commodities, such as passion fruit. In Uganda, however, cocoa prices were higher than those for traditional commodities, such as coffee. A higher dependence on cocoa revenues and thus specialisation, however, increased farms’ cocoa yields across cases, yet at the expense of shade tree density and planting. These results support the notion that income diversification strategies, especially when cocoa prices are low, are important for smallholder livelihoods and resilience (Aneani et al., 2011; Cerda et al., 2014), despite potentially reducing downstream companies’ power to enforce rules for sustainable cocoa production (Grimm et al., 2014). Ecuadorian farmers in our sample who diversify their cocoa buyers too much are sanctioned by no longer receiving in-kind premiums, a threat commonly associated with sustainability initiatives (Grabs and Carodenuto, 2021). Thus, promoting diverse agroforestry systems and income diversification might require a balancing act from downstream companies to ensure living incomes and resilient systems for supplying farmers whilst not losing too much leverage to generate change in cocoa farming practices.
Farm workers’ wages have recently received attention with potential implications for value chains. Farmers in our case studies paid their workers 70% of the national minimum wage (Ecuador) and 18% of the estimated living wage (Uganda) (Global Living Wage Coalition, 2021; Wage Indicator, 2022). Low wages are generally perceived as a result of commercial value chain practices with uneven value distribution and low prices paid by buyers (Lebaron, 2021), following which farmers are not able to pay adequate wages. Meemken et al. (2019) assessed the effect of Fairtrade certification on cocoa farm workers’ annual wages in Côte d’Ivoire and concluded that given current payment modalities, smallholder farmers were not incentivised to pay higher wages without clear rules and their monitoring.
5.3. Limitations and future research directions
In this study, we show several significant relationships between value chain factors and SFPI, whilst controlling for numerous confounding factors. This illustrates the importance of value chain factors despite the lack of attention paid to them in the literature thus far. However, some caveats are required in the interpretation of results due to several limitations in our study design. First, the results are largely exploratory and causal inference between value chain factors and sustainability outcomes should be avoided due to potential endogeneity issues. Future studies on the adoption of SFPI should therefore consider an experimental approach that controls for endogeneity, potentially with instrumental variables. Second, our farmer sample might not represent a sample of “typical” Ecuadorian and Ugandan cocoa farmers but rather farmers from the “sustainable cocoa” segment and part of specific value chains with sustainability orientation. Thus, our results are generalisable only to a certain extent. Third, we were limited to the data set available and several potentially important groups of factors were thus not included in our analysis, such as the broader economic or climatic situation. The importance of attitudinal and social aspects for practice adoption is increasingly recognised (Meijer et al., 2015; Dessart et al., 2019; Arslan et al., 2020), which we were only partly able to cover. Our study furthermore did not cover the role of (financial) incentives, which can be a strong element in farmers’ decision making (Piñeiro et al., 2020). Also the role of additional contextual factors like the broader economic situation or climatic conditions. In the future, different value chain characteristics including incentives could be combined with an in-depth analysis of farmers’ social setting and motivation to implement or adopt certain practices to determine their importance.
6. Conclusion
Chocolate companies are increasingly investing in value chain sustainability initiatives, highly focusing on sustainable practice uptake amongst upstream producers. Yet little is known about if and how value chain factors influence practice implementation, especially within the social and economic dimension of sustainability. Our results from two cocoa value chains connecting cocoa producers in Ecuador and Uganda with Swiss chocolate brands revealed the most important conclusion: value chain factors can have a substantial influence on the implementation of SFPI amongst cocoa producers. Whilst observed relationships were weaker than hypothesised, our results indicate that value chain factors are just as important as farmer and farm factors for SFPI across sustainability dimension. Whilst this study was rather exploratory in nature, it provides evidence that future studies on farmers’ adoption of sustainable practices should integrate value chain characteristics into their conceptual frameworks.
Our results highlight that chocolate brands have various levers within their own value chains to improve the sustainability performance of their supplying farmers and assure the long-term supply of sustainably produced cocoa. However, their potential was underexploited in both case studies. Capacity building is an important mechanism for downstream actors to increase SFPI implementation at farm level, especially when training and extension target specific practices. Training and extension service organised by downstream companies should therefore address specific practices beyond agro-environmental practices. In addition, resource transfer might provide farmers with the necessary resources to enact change. In our case studies, this is currently done through in-kind premium distribution and promised price premiums, yet could be extended to address inhibiting factors for practice implementation beyond knowledge. Our results furthermore suggest that stable and long-term relationships between value chain actors can influence SFPI implementation on farms, yet require a common definition of sustainability goals. Chocolate manufacturers should clearly define and communicate their sustainability goals along the entire value chain and align all actors in order to avoid contradictory agendas from single parties. In order to fully exploit the potential to generate change, chocolate companies need to continuously invest and establish strong collaborations along their entire value chain.
Data availability statement
The datasets presented in this study can be found in online repositories. The names of the repository/repositories and accession number(s) can be found at: https://figshare.com/s/d25ecd539209287d7b1e.
Ethics statement
The studies involving human participants were reviewed and approved by Ethics committee of the Department of Food Systems Sciences, Research Institute of Organic Agriculture (FiBL). Written informed consent for participation was not required for this study in accordance with the national legislation and the institutional requirements.
Author contributions
LT, EL, MC, and CS contributed to conception and design of the study. LT performed the investigation, statistical analysis, and wrote the first draft of the manuscript. All authors contributed to manuscript revision, read, and approved the submitted version.
Funding
This work was supported by the Swiss National Science Foundation (grant number 407340_172451).
Acknowledgments
The authors would like to thank all farmers who participated in this study, all extension officers and company staff assisting in the organisation, as well as the two participating chocolate companies. Our warmest appreciation also goes to the data collection teams in Ecuador and Uganda (Moritz Egger, Elio Palmero, Rainer Weisshaidinger, Brian Ssebunya, Kagimu Martin, Mwesigwa Lauben, Kawere Brasio Kigongo, Lilian Victor Nakirigya, Ajio Florence, Nathan Ruhiiga, and David Derrick Mubiru). The authors also thank Gianna Lazzarini, Goedele Van den Broeck, and Miet Maertens for their valuable comments and feedback during the development of this manuscript and the analyses.
Conflict of interest
The authors declare that the research was conducted in the absence of any commercial or financial relationships that could be construed as a potential conflict of interest.
Publisher’s note
All claims expressed in this article are solely those of the authors and do not necessarily represent those of their affiliated organizations, or those of the publisher, the editors and the reviewers. Any product that may be evaluated in this article, or claim that may be made by its manufacturer, is not guaranteed or endorsed by the publisher.
Footnotes
References
Abdulai, I., Hoffmann, M. P., Jassogne, L., Asare, R., Graefe, S., Tao, H.-H., et al. (2020). Variations in yield gaps of smallholder cocoa systems and the main determining factors along a climate gradient in Ghana. Agric. Syst. 181:102812. doi: 10.1016/j.agsy.2020.102812
Adebayo, S. T., Oyawole, F. P., Sanusi, R. A., and Afolami, C. A. (2021). Technology adoption among cocoa farmers in Nigeria: What drives farmers’ decisions? Forests Trees Livelihoods, 1–12. doi: 10.1080/14728028.2021.2011789
Ajzen, I. (1991). The theory of planned behavior. Organ. Behav. Hum. Decis. Process. 50, 179–211. doi: 10.1016/0749-5978(91)90020-T
Ali, E. B., Awuni, J. A., and Danso-Abbeam, G. (2018). Determinants of fertilizer adoption among smallholder cocoa farmers in the Western region of Ghana. Cogent. Food Agricult. 4:1538589. doi: 10.1080/23311932.2018.1538589
Amfo, B., and Ali, E. B. (2020). Climate change coping and adaptation strategies: how do cocoa farmers in Ghana diversify farm income? Forest Policy Econ. 119:102265. doi: 10.1016/j.forpol.2020.102265
Andres, C., Comoé, H., Beerli, A., Schneider, M., Rist, S., and Jacobi, J. (2016). “Cocoa in monoculture and dynamic agroforestry” in Sustainable Agriculture Reviews. ed. E. Lichtfouse , vol. 19 (Cham: Springer International Publishing)
Aneani, F., Anchirinah, V. M., Owusu-Ansah, F., and Asamoah, M. (2011). An analysis of the extent and determinants of crop diversification by cocoa (Theobroma cacao) farmers in Ghana. Africal J. Agricult. Res. 6, 4277–4287. doi: 10.5897/AJAR10.1083
Aneani, F., Anchirinah, V., Owusu-Ansah, F., and Asamoah, M. (2012). Adoption of some cocoa production technologies by cocoa farmers in Ghana. Sustain. Agricult. Res. 1:103. doi: 10.5539/sar.v1n1p103
Anecacao . (n.d.). Cacao Nacional. Available at: http://www.anecacao.com/es/quienes-somos/cacao-nacional.html (Accessed January 08, 2021)
Ansah, E. O., Kaplowitz, M. D., Lupi, F., and Kerr, J. (2020). Smallholder participation and procedural compliance with sustainable cocoa certification programs. Agroecol. Sustain. Food Syst. 44, 54–87. doi: 10.1080/21683565.2019.1579776
Armengot, L., Barbieri, P., Andres, C., Milz, J., and Schneider, M. (2016). Cacao agroforestry systems have higher return on labor compared to full-sun monocultures. Agron. Sustain. Dev. 36:70. doi: 10.1007/s13593-016-0406-6
Armengot, L., Ferrari, L., Milz, J., Velásquez, F., Hohmann, P., and Schneider, M. (2019). Cacao agroforestry systems do not increase pest and disease incidence compared with monocultures under good cultural management practices. Crop Prot. 130:105047. doi: 10.1016/j.cropro.2019.105047
Arslan, A., Floress, K., Lamanna, C., Lipper, L., Asfaw, S., and Rosenstock, T. (2020). The adoption of improved agricultural technologies. A meta-analysis for Africa. IFAD Research Series Vol. 63.
Atube, F., Malinga, G. M., Nyeko, M., Okello, D. M., Alarakol, S. P., and Okello-Uma, I. (2021). Determinants of smallholder farmers’ adaptation strategies to the effects of climate change: evidence from northern Uganda. Agricult. Food Security 10:6. doi: 10.1186/s40066-020-00279-1
Baumer, B., and Udwin, D. (2015). R Markdown. WIREs Computational Statistics 7, 167–177. doi: 10.1002/wics.1348
Bazrafkan, K., Valizadeh, N., Khannejad, S., Kianmehr, N., Bijani, M., and Hayati, D. (2022). What drives farmers to use conservation agriculture? Application of mediated protection motivation theory. Front. Psychol. 13:1323. doi: 10.3389/fpsyg.2022.991323
Bentley, J. W., Boa, E., and Stonehouse, J. (2004). Neighbor trees: shade, intercropping, and cacao in Ecuador. Hum. Ecol. 32, 241–270. doi: 10.1023/B:HUEC.0000019759.46526.4d
Berlan, A. (2013). Social sustainability in agriculture: an anthropological perspective on child labour in cocoa production in Ghana. J. Dev. Stud. 49, 1088–1100. doi: 10.1080/00220388.2013.780041
Bijani, M., Ghazani, E., Valizadeh, N., and Fallah Haghighi, N. (2017). Pro-environmental analysis of farmers' concerns and behaviors towards soil conservation in central district of Sari County, Iran. Int. Soil Water Conserv. Res. 5, 43–49. doi: 10.1016/j.iswcr.2017.03.001
Blare, T., and Useche, P. (2013). Competing objectives of smallholder producers in developing countries: examining cacao production in northern Ecuador. Environ. Econom. 4, 71–79.
Blare, T., and Useche, P. (2014). What does it mean to be socially responsible? Case study on the impact of the producer-plus-program on communities, women, and the environment in Ecuador. Modernizing Extension and Advisory Services (MEAS).
Blaser, W. J., Oppong, J., Hart, S. P., Landolt, J., Yeboah, E., and Six, J. (2018). Climate-smart sustainable agriculture in low-to-intermediate shade agroforests. Nat. Sustain. 1, 234–239. doi: 10.1038/s41893-018-0062-8
Boadi-Kusi, S. B., Hansraj, R., Mashige, K. P., and Ilechie, A. A. (2016). Factors associated with protective eyewear use among cocoa farmers in Ghana. Inj. Prev. 22, 365–369. doi: 10.1136/injuryprev-2014-041531
Boza, E. J., Motamayor, J. C., Amores, F. M., Cedeño-Amador, S., Tondo, C. L., Livingstone, D. S., et al. (2014). Genetic characterization of the cacao cultivar CCN 51: its impact and significance on global cacao improvement and production. Journal of the American Society for Horticultural Science. J. Amer. Soc. Hort. Sci. 139, 219–229. doi: 10.21273/JASHS.139.2.219
Bruce, P., Bruce, A., and Gedeck, P. (2020). Practical statistics for data scientists: 50+ essential concepts using R and Python. Sebastopol, CA: O’Reilly Media.
Busquet, M., Bosma, N., and Hummels, H. (2021). A multidimensional perspective on child labor in the value chain: the case of the cocoa value chain in West Africa. World Dev. 146:105601. doi: 10.1016/j.worlddev.2021.105601
Bymolt, R., Laven, A., and Tyszler, M. (2018). Demystifying the cocoa sector in Ghana and Côte d’Ivoire. Netherlands: Royal Tropical Institute.
Candemir, A., Duvaleix, S., and Latruffe, L. (2021). Agricultural cooperatives and farm sustainability – a literature review. J. Econ. Surv. 1–27. doi: 10.1111/joes.12417
Cerda, R., Deheuvels, O., Calvache, D., Niehaus, L., Saenz, Y., Kent, J., et al. (2014). Contribution of cocoa agroforestry systems to family income and domestic consumption: looking toward intensification. Agrofor. Syst. 88, 957–981. doi: 10.1007/s10457-014-9691-8
de Bon, H., Huat, J., Parrot, L., Sinzogan, A., Martin, T., Malézieux, E., et al. (2014). Pesticide risks from fruit and vegetable pest management by small farmers in sub-Saharan Africa. A review. Agronomie 34, 723–736. doi: 10.1007/s13593-014-0216-7
De Oca Munguia, O. M., and Llewellyn, R. (2020). The adopters versus the technology: which matters more when predicting or explaining adoption? Appl. Econ. Perspect. Policy 42, 80–91. doi: 10.1002/aepp.13007
Denkyirah, E. K., Okoffo, E. D., Adu, D. T., Aziz, A. A., Ofori, A., and Denkyirah, E. K. (2016). Modeling Ghanaian cocoa farmers’ decision to use pesticide and frequency of application: the case of Brong Ahafo region. Springerplus 5:1113. doi: 10.1186/s40064-016-2779-z
Dessart, F., Barreiro-Hurlé, J., and Van Bavel, R. (2019). Behavioural factors affecting the adoption of sustainable farming practices: a policy-oriented review. Eur. Rev. Agric. Econ. 46, 417–471. doi: 10.1093/erae/jbz019
Djokoto, J. G., Owusu, V., and Awunyo-Vitor, D. (2016). Adoption of organic agriculture: evidence from cocoa farming in Ghana. Cogent. Food Agricult. 2:1242181. doi: 10.1080/23311932.2016.1242181
Dormon, E. N. A., Van Huis, A., Leeuwis, C., Obeng-Ofori, D., and Sakyi-Dawson, O. (2004). Causes of low productivity of cocoa in Ghana: farmers' perspectives and insights from research and the socio-political establishment. NJAS 52, 237–259. doi: 10.1016/S1573-5214(04)80016-2
Effendy,, Pratama, M. F., Pratama, M. F., Rauf, R. A., Antara, M., Basir-Cyio, M., et al. (2019). Factors influencing the efficiency of cocoa farms: a study to increase income in rural Indonesia. PLoS One 14:e0214569. doi: 10.1371/journal.pone.0214569
FAO . (2022a). Countries by commodity. Available at: http://www.fao.org/faostat/en/#rankings/countries_by_commodity (Accessed April 13, 2022)
FAO . (2022b). FAOSTAT crops. Rome, Italy. Available at: http://www.fao.org/faostat/en/#data/QC (Accessed January 30, 2023)
Foguesatto, C. R., Borges, J. A. R., and Machado, J. A. D. (2020). A review and some reflections on farmers' adoption of sustainable agricultural practices worldwide. Sci. Total Environ. 729:138831. doi: 10.1016/j.scitotenv.2020.138831
Global Living Wage Coalition . (2021). Living wage for rural Uganda. Available at: https://www.globallivingwage.org/living-wage-benchmarks/living-wage-for-lake-victoria-basin-uganda/ (Accessed February 02, 2022)
Grabs, J., and Carodenuto, S. L. (2021). Traders as sustainability governance actors in global food supply chains: a research agenda. Bus. Strateg. Environ. 30, 1314–1332. doi: 10.1002/bse.2686
Grimm, J. H., Hofstetter, J. S., and Sarkis, J. (2014). Critical factors for sub-supplier management. Int. J. Prod. Econ. 152, 159–173. doi: 10.1016/j.ijpe.2013.12.011
Grovermann, C., Rees, C., Beye, A., Wossen, T., Abdoulaye, T., and Cicek, H. (2023). Uptake of agroforestry-based crop management in the semi-arid Sahel – analysis of joint decisions and adoption determinants. Front. Sustain. Food Syst. 7:2551. doi: 10.3389/fsufs.2023.1042551
Hansson, H., Thompson, B., Manevska, G., Toma, L., and Leduc, G. (2019). LIFT – deliverable D2.1: drivers of farmers’ up-take of ecological approaches – a conceptual framework with a behavioural focus.
Huetz-Adams, F., Huber, C., Knoke, I., Morazán, P., and Mürlebach, M. (2016). Strengthening the competitiveness of cocoa production and improving the income of cocoa producers in West and Central Africa. Bonn, Germany: SÜDWIND e.V.
ILO . (n.d.). What is child labour. International labour association. Available at: https://www.ilo.org/ipec/facts/lang--en/index.htm (Accessed April 11, 2022)
INEC . (2020). ECUADOR – Encuesta de Superficie y Producción Agropecuaria Continua 2015. Abuja: INEC.
Kuyah, S., Whitney, C. W., Jonsson, M., Sileshi, G. W., Öborn, I., Muthuri, C. W., et al. (2019). Agroforestry delivers a win-win solution for ecosystem services in sub-Saharan Africa. A meta-analysis. Agron. Sustain. Dev. 39:47. doi: 10.1007/s13593-019-0589-8
Lebaron, G. (2021). Wages: an overlooked dimension of business and human rights in global supply chains. Bus. Human Rights J. 6, 1–20. doi: 10.1017/bhj.2020.32
Liu, T., Bruins, R. J. F., and Heberling, M. T. (2018). Factors influencing farmers’ adoption of best management practices: a review and synthesis. Sustainability 10:432. doi: 10.3390/su10020432
Liverpool-Tasie, L. S. O., Wineman, A., Young, S., Tambo, J., Vargas, C., Reardon, T., et al. (2020). A scoping review of market links between value chain actors and small-scale producers in developing regions. Nat. Sustain. 3, 799–808. doi: 10.1038/s41893-020-00621-2
Meemken, E.-M., Barrett, C. B., Michelson, H. C., Qaim, M., Reardon, T., and Sellare, J. (2021). Sustainability standards in global agrifood supply chains. Nature Food 2, 758–765. doi: 10.1038/s43016-021-00360-3
Meemken, E.-M., Sellare, J., Kouame, C. N., and Qaim, M. (2019). Effects of Fairtrade on the livelihoods of poor rural workers. Nat. Sustain. 2, 635–642. doi: 10.1038/s41893-019-0311-5
Meijer, S. S., Catacutan, D., Ajayi, O. C., Sileshi, G. W., and Nieuwenhuis, M. (2015). The role of knowledge, attitudes and perceptions in the uptake of agricultural and agroforestry innovations among smallholder farmers in sub-Saharan Africa. Int. J. Agric. Sustain. 13, 40–54. doi: 10.1080/14735903.2014.912493
Middendorp, R. S., Vanacker, V., and Lambin, E. F. (2018). Impacts of shaded agroforestry management on carbon sequestration, biodiversity and farmers income in cocoa production landscapes. Landsc. Ecol. 33, 1953–1974. doi: 10.1007/s10980-018-0714-0
Mithöfer, D., Roshetko, J. M., Donovan, J. A., Nathalie, E., Robiglio, V., Wau, D., et al. (2017). Unpacking ‘sustainable’ cocoa: do sustainability standards, development projects and policies address producer concerns in Indonesia, Cameroon and Peru? Int. J. Biodiver. Sci. Ecosyst. Serv. Manag. 13, 444–469. doi: 10.1080/21513732.2018.1432691
Mozzato, D., Gatto, P., Defrancesco, E., Bortolini, L., Pirotti, F., Pisani, E., et al. (2018). The role of factors affecting the adoption of environmentally friendly farming practices: can geographical context and time explain the differences emerging from literature? Sustainability 10:3101. doi: 10.3390/su10093101
Musafiri, C. M., Kiboi, M., Macharia, J., Ng'etich, O. K., Kosgei, D. K., Mulianga, B., et al. (2022). Adoption of climate-smart agricultural practices among smallholder farmers in Western Kenya: do socioeconomic, institutional, and biophysical factors matter? Heliyon 8:e08677. doi: 10.1016/j.heliyon.2021.e08677
Nkamleu, G. B., and Kielland, A. (2006). Modeling farmers' decisions on child labor and schooling in the cocoa sector: a multinomial logit analysis in Côte d'Ivoire. Agric. Econ. 35, 319–333. doi: 10.1111/j.1574-0862.2006.00165.x
Okoffo, E. D., Mensah, M., and Fosu-Mensah, B. Y. (2016). Pesticides exposure and the use of personal protective equipment by cocoa farmers in Ghana. Environ. Syst. Res. 5:17. doi: 10.1186/s40068-016-0068-z
Owombo, P. T., Idumah, F. O., and Afolayan, A. F. (2014). Assessing factors affecting adherence to safety precautions in pesticides use among cocoa farmers in Nigeria. Ethiopian J. Environ. Stud. Manag. 7, 810–820. doi: 10.4314/ejesm.v7i2.1S
Perez, M., Lopez-Yerena, A., and Vallverdú-Queralt, A. (2020). Traceability, authenticity and sustainability of cocoa and chocolate products: a challenge for the chocolate industry. Crit. Rev. Food Sci. Nutr., 62, 1–15. doi: 10.1080/10408398.2020.1819769
Piñeiro, V., Arias, J., Dürr, J., Elverdin, P., Ibáñez, A. M., Kinengyere, A., et al. (2020). A scoping review on incentives for adoption of sustainable agricultural practices and their outcomes. Nat. Sustain. 3, 809–820. doi: 10.1038/s41893-020-00617-y
Reis, T. N. P. D., Meyfroidt, P., zu Ermgassen, E. K. H. J., West, C., Gardner, T., Bager, S., et al. (2020). Understanding the stickiness of commodity supply chains is key to improving their sustainability. One Earth 3, 100–115. doi: 10.1016/j.oneear.2020.06.012
Rueda, X., Paz, A., Gibbs-Plessl, T., Leon, R., Moyano, B., and Lambin, E. F. (2018). Smallholders at a crossroad: intensify or fall behind? Exploring alternative livelihood strategies in a globalized world. Bus. Strateg. Environ. 27, 215–229. doi: 10.1002/bse.2011
Schader, C., Baumgart, L., Landert, J., Muller, A., Ssebunya, B., Blockeel, J., et al. (2016). Using the sustainability monitoring and assessment routine (SMART) for the systematic analysis of trade-offs and synergies between sustainability dimensions and themes at farm level. Sustainability 8:274. doi: 10.3390/su8030274
Schader, C., Curran, M., Heidenreich, A., Landert, J., Blockeel, J., Baumgart, L., et al. (2019). Accounting for uncertainty in multi-criteria sustainability assessments at the farm level: improving the robustness of the SMART-farm tool. Ecol. Indic. 106:105503. doi: 10.1016/j.ecolind.2019.105503
Schader, C., Heidenreich, A., Kadzere, I., Egyir, I., Muriuki, A., Bandanaa, J., et al. (2021). How is organic farming performing agronomically and economically in sub-Saharan Africa? Glob. Environ. Chang. 70:102325. doi: 10.1016/j.gloenvcha.2021.102325
Schoemaker, P. J. H. (1982). The expected utility model: its variants, purposes, evidence and limitations. J. Econ. Lit. 20, 529–563.
Takahashi, K., Muraoka, R., and Otsuka, K. (2020). Technology adoption, impact, and extension in developing countries’ agriculture: a review of the recent literature. Agric. Econ. 51, 31–45. doi: 10.1111/agec.12539
Tennhardt, L., Lazzarini, G., Weisshaidinger, R., and Schader, C. (2022). Do environmentally-friendly cocoa farms yield social and economic co-benefits? Ecol. Econ. 197:107428. doi: 10.1016/j.ecolecon.2022.107428
Thorlakson, T. (2018). A move beyond sustainability certification: the evolution of the chocolate industry's sustainable sourcing practices. Bus. Strateg. Environ. 27, 1653–1665. doi: 10.1002/bse.2230
Useche, P., and Blare, T. (2013). Traditional vs. modern production systems: Price and nonmarket considerations of cacao producers in northern Ecuador. Ecol. Econ. 93, 1–10. doi: 10.1016/j.ecolecon.2013.03.010
UTZ . (2017). UTZ and shade trees. Findings from literature review by VU Center for International Cooperation. Available online: https://utz.org/wp-content/uploads/2018/01/Key-findings-on-shade-trees.pdf
Van Vliet, J. A., and Giller, K. E. (2017). “Chapter five – mineral nutrition of cocoa: a review” in Advances in agronomy. ed. D. L. Sparks (Cambridge, MA: Academic Press)
Van Vliet, J. A., Slingerland, M. A., Waarts, Y. R., and Giller, K. E. (2021). A living income for cocoa producers in Côte d'Ivoire and Ghana? Front. Sustain. Food Syst. 5:732831. doi: 10.3389/fsufs.2021.732831
Waarts, Y., Janssen, V., Ingram, V., Slingerland, M., Van Rijn, F., Beekman, G., et al. (2019). A living income for smallholder commodity farmers and protected forests and biodiversity: How can the private and public sectors contribute? Netherlands: Wageningen Economic Research.
Wage Indicator . (2022). Minimum wage – Cocoa plantations, including selected cocoa for export. Available at: https://wageindicator.org/salary/minimum-wage/ecuador/3854-agriculture-and-plantations/3867-cocoa-plantations-including-selected-cocoa-for (Accessed February 02, 2022)
Keywords: cocoa, value chain, sustainability, practice adoption, sustainable supply chain management, capacity building, Ecuador, Uganda
Citation: Tennhardt LM, Lambin EF, Curran M and Schader C (2023) Implementation of sustainable farming practices by cocoa farmers in Ecuador and Uganda: the influence of value chain factors. Front. Sustain. Food Syst. 7:1167683. doi: 10.3389/fsufs.2023.1167683
Edited by:
Valerien Pede, International Rice Research Institute (IRRI), PhilippinesReviewed by:
Aminou Arouna, Africa Rice Center (CGIAR), Côte d'IvoireNaser Valizadeh, Shiraz University, Iran
Copyright © 2023 Tennhardt, Lambin, Curran and Schader. This is an open-access article distributed under the terms of the Creative Commons Attribution License (CC BY). The use, distribution or reproduction in other forums is permitted, provided the original author(s) and the copyright owner(s) are credited and that the original publication in this journal is cited, in accordance with accepted academic practice. No use, distribution or reproduction is permitted which does not comply with these terms.
*Correspondence: Lina M. Tennhardt, bGluYS50ZW5uaGFyZHRAZmlibC5vcmc=