- 1College of Mechanical Engineering, Guizhou University, Guiyang, China
- 2Engineering Training Center, Guizhou Minzu University, Guiyang, China
The COVID-19 pandemic has severely disrupted the global food supply chain through various interventions, such as city closures, traffic restrictions, and silent management. Limited research has been conducted on the design of emergency regional food supply chains (ERFSC) and its labor demand forecasting under government-mandated interventions. This paper applies emergency supply chain management theory to analyze the business processes of the ERFSC and proposes a multi-level ERFSC network tailored to different risk levels. Additionally, a food demand forecasting model and a mathematical model for stochastic labor demand planning are constructed based on the development trend of regional epidemics. An empirical analysis is presented using Huaguoyuan, Guiyang, China, as an example. The results demonstrate that the proposed ERFSC design and its labor demand forecasting model can achieve secure supply and accurate distribution of necessities in regions with different risk levels. These findings have important policy and research implications for the government and practitioners to take interventions and actions to ensure food supply for residents in the context of city closure or silent management. This study serves as a pilot study that will be further extended by the authors from geographical and policy perspectives.
1. Introduction
During the COVID-19 pandemic, many countries have implemented extensive lockdowns, economic interventions, and health system measures to mitigate the spread of the virus (Ivanov and Dolgui, 2020; Hale et al., 2021). Several recent studies have concluded that the lockdown has posed a threat to food security, leading to reduced yields, disruptions in food supply chains, restricted trade flows, and reduced dietary choices (Devereux et al., 2020; Fan et al., 2020). The interventions implemented to contain COVID-19 have significantly impacted the food value chain, resulting in difficulties in purchasing necessities and insecurity of basic needs for the population in the outbreak region (Hobbs, 2020; Narayanan et al., 2020). In urban areas that rely on external supplies to meet their needs, sudden disruptions in food supply and panic buying behavior caused prices to soar and triggered social panic (Davila et al., 2021). On the supply side, the lockdown may lead to a reduction in vegetable production and also lengthen the food distribution cycle, particularly impacting some perishable commodities such as fruit, meat products, and fresh vegetables (Harris et al., 2020; Yu et al., 2020; Huang et al., 2021). On the demand side, the outbreak of the COVID-19 crisis may cause social panic and potential hoarding of food, leading to two extremes of over-buying and under-buying that can impact the basic livelihood security of people quarantined at home (Goddard, 2020; Nicomedes and Avila, 2020; Zhang et al., 2020).
The pandemic has exposed the vulnerabilities of global food supply chains and disrupted the flow of food from producers to consumers (Christiaensen et al., 2021; Yin et al., 2021; Alabi and Ngwenyama, 2023). The regional food supply chains are becoming a viable alternative for food security due to easier access to local food (Cristiano, 2021; Thilmany et al., 2021). Regional food supply chains are based on local food production and demand and are characterized by fewer intermediaries, shorter distribution times, greater agility, and more sustainability in economic, environmental, and social terms than conventional food production (Berti and Mulligan, 2016). They are also favored by consumers for reasons such as fresher, safer, more nutritious food supplies and support for local economic development (Schnell, 2013; Feldmann and Hamm, 2015). The efforts are underway to reconfigure and innovate the current food supply chain and strengthen the urban-rural integration of food supply to build a more stable, resilient, and sustainable food supply chain (Mollenkopf et al., 2021; Sharma et al., 2021). The Food and Agriculture Organization of the United Nations has emphasized the importance of regional supply chains in countering large-scale disruptions in food supply chains caused by the COVID-19 crisis (Food and Organization, 2020; Rosenzweig et al., 2020). Strengthening regional food supply chains is also being considered as a viable option for dealing with the impact of uncertainty (Mahajan and Tomar, 2021). Singh et al. (2021) proposed a public distribution system consisting of a central warehouse, state warehouse, district warehouse, and fair price shop to quickly recover the food supply in the region.
The COVID-19 crisis has resulted in labor shortages in the food supply chains due to lockdowns, movement restrictions, quarantines, and illnesses (Hobbs, 2020; Saul et al., 2020). As labor is a crucial input for the functioning of every supply chain network, this can lead to increased costs, lower profits for firms, higher prices for consumers, and unfulfilled demand (Bhattarai and Reiley, 2020). To cope with sudden labor demands during an outbreak, redundancy within the supply chain system or finding new alternatives can be a way forward (Coopmans et al., 2021). Nagurney (2021) developed a supply chain network optimization framework that explicitly includes labor as a variable in the economic activities of supply chain networks, such as production, transportation, storage, and distribution. Community organizations are using local information, networks, and relationships to distribute food to community residents during the pandemic (Aday and Aday, 2020). In emergency supply chain management, the establishment of self-organization with efficient management and transparency of information is crucial (Zebrowski, 2019; Banerjee et al., 2021; Mutebi et al., 2021). Shareef et al. (2019) argue that volunteers play an important role in emergency disaster rescue and provide access to a government-run network of volunteer requisitioners. Some studies suggest that an integrated system of simultaneous truck and drone distribution in high-risk zones can efficiently meet demand distribution without close contact (Jeong et al., 2019; Das et al., 2020). In addition, outdoor spaces are being designated in different parts of the city as alternative locations for traders and farmers to conduct sales transactions during retail closures (Singh et al., 2021). Therefore, the rapid recovery or reconstruction of the food supply chain in the region during public health emergencies requires the consideration of the population, distribution location, risk area division, interchange state (IS) setting, operator requirements and end-delivery strategy, which is a complex system project.
By analysing the literature, resilience and sustainability were found to be the most critical themes, and the application of various innovative technologies such as digital twins, artificial intelligence, blockchain and the Internet of Things in the management of supply chains suffering from sudden disruptions was more studied (Moosavi et al., 2021, 2022; Montoya-Torres et al., 2023). However, it was discovered that there has not been sufficient discussion on how to quickly construct an ERFSC, particularly in the context of a logistics park outbreak and city-wide silent management. Although researchers have proposed several ideas for restoring the food supply chain during a pandemic, the practicality of these ideas is limited due to different premises considered in previous studies (Fan et al., 2020; Chitrakar et al., 2021; Dixon et al., 2021; Huang et al., 2021). This research gap includes the lack of food supply chain network design, end-delivery services strategies, and labor demand forecasting models under lockdown conditions. To address this gap, we present a multi-level regional food supply chain system solution adapted to different risk levels, which is being introduced for the first time. Finally, we construct an ERFSC framework of agricultural suppliers, distribution centres (DCs), ISs, and residents as an example, focusing on Huaguoyuan in Guiyang City, China, to achieve secure supply and accurate distribution of necessities in areas with varying risk levels. This study makes important contributions to the field, including:
(i) It proposed a more practical strategy for safeguarding the supply of necessities to regional residents during a regional pandemic outbreak, namely a regional food supply chain design based on dynamic demand for essential supplies, labor demand forecasting and end-delivery strategies to achieve risk management and emergency response during emergencies such as food supply chain disruptions.
(ii) It studied the development of a demand forecasting model for necessities and a labor forecasting model for regional food supply chain operation requirements and end-delivery services strategy based on the risk trend of the epidemic in the region under silent management interventions, which improved the efficiency and quality of ERFSC management.
(iii) The proposal of an ERFSC labor demand forecasting algorithm that takes into account uncertain parameters and fully considers the random distribution properties of these parameters.
Our study serves as an important theoretical foundation for future research on ERFSC reconfiguration and labor demand forecasting during emergency situations. Additionally, it provides valuable recommendations for government agencies, businesses, and social organizations involved in emergency response.
2. ERFSC network design
The ERFSC is a temporary alliance formed by the government in response to emergencies to integrate various advantageous resources of society. In addition to the characteristics of the general regional food supply chain, the ERFSC has variations in the operation items of each activity link in the supply chain and temporary cooperation and coordination among them. This is also the key factor determining the operational efficiency of the ERFSC (Shah Alam Khan, 2008; Dwivedi et al., 2018). An effective emergency regional supply chain should be able to make rapid assessments and decisions based on actual dynamic demand (Dwivedi et al., 2018).
There are several issues in emergency supply chain operations, such as mapping existing emergency supply chains, demand forecasting and assessment, procurement, inventory management, logistics management, and relief distribution (Wang and Zhang, 2016). Therefore, it is necessary for the government to set up a supply assurance team to optimize the distribution of resources to transport agricultural products to designated farmers' markets, retailers, or sell them directly to residents.
Generally, food supply chains consist of a three-tier structure of supplier-DC-retailer, and the farmer-consumer model is also more common in regional food supply chains (Thilmany et al., 2021). During an outbreak in an urban area, interventions such as city closures, movement restrictions, and home quarantines can lead to the city being divided into smaller zones for management, such as administrative boundaries, communities, and neighborhoods. In such cases, DCs become the main source of supplies for the city's residents. To achieve full coverage and precise distribution of food while complying with epidemic prevention policies that reduce movement, we propose an ERFSC network with DCs as the source, as depicted in Figure 1. Here, each DC is responsible for a certain range of retailers/ISs, and each retailer/IS is responsible for supplying food to residents in a designated zone.
During the epidemic, unexpected transport restrictions and labor shortages disrupted the urban food supply chain (Hobbs, 2020; Sukhwani et al., 2020). Availability of labor, including loading and unloading, delivery, sorting, and processing labor, as well as smooth logistics, became the crucial factors in maintaining the food supply chain. For some companies, the inability of employees to return to work became a bottleneck (Singh et al., 2021; Tarra et al., 2021). Therefore, the goal of the ERFSC is to distribute the necessary supplies in infected areas with minimum labor, within a reasonable time and cost.
2.1. Description and analysis of the ERFSC
To optimize the allocation of labor in the ERFSC, we have developed a scenario as shown in Figure 2. Our analysis focuses on the optimal allocation of labor in the ERFSC in urban areas, specifically from the DCs to the residents. The DCs receive goods from outside, distribute them based on the downstream retailers' demand, and arrange vehicles or engage third-party logistics to transport them to each retailer. The retailers receive shipments from DCs, sort and prepack the food according to customer requirements, and hand them over to riders or carriers for delivery to residents. Residents get their food via self-purchase, pick-up or home delivery, depending on the risk level of their zone, as shown in Figure 3.
2.1.1. Market demand forecast
Forecasting models play a crucial role in precision marketing, aiding in the comprehension and fulfillment of customer needs and expectations (You et al., 2015). Various statistical analysis techniques, such as time-series analysis and regression analysis, have been employed for demand forecasting in supply chain management (Wang et al., 2016). The utilization of AI techniques, such as artificial neural networks and evolutionary computation, has become prevalent in demand forecasting due to the advancements in computing technologies (Lin et al., 2018). In addition, big data analysis in supply chain management is receiving increasing attention (Ali et al., 2017; Nguyen et al., 2018). As data for the parameters in the model were readily available, we chose to use statistical analysis to forecast food demand.
Normally, the total food demand in a region is mainly determined by the number of people and remains relatively stable when the population changes little. However, during an outbreak, the total food demand in the region fluctuates due to changes in the risk zones. Let N0 denote the number of permanent residents in the region and d0 denote the normal daily per capita food requirement. If the number of people classified as high and medium risk zones is nhr and nmr, respectively, then the total food demand D(t) in the region during an outbreak can be expressed as
Here, α and β are the perturbation factors for the demand for food by people in high and medium risk zones, respectively, which can be interpreted as the shopping cycle.
2.1.2. Efficiency of end-delivery services
Convenience plays a crucial role in consumers' food purchasing choices (Morganosky and Cude, 2000). Traditional grocery stores have implemented various methods to offer convenient delivery options to their customers, such as online shopping, door-step home deliveries, and drive-through pick-ups (Raison and Jones, 2020). Additionally, studies have shown that consumers are willing to pay a small fee for enhanced convenience, like home delivery services (Anesbury et al., 2015). During an epidemic, it is crucial for ERFSCs to devise a delivery strategy that ensures residents have access to food while minimizing the risk of mutual exposure. To achieve an efficient and safe end-delivery strategy, we propose a differentiated approach based on the level of risk zones, which is depicted in Figure 3. In low-risk zones, residents can opt for offline shopping, visiting physical stores to purchase their food. For those in medium-risk zones, a convenient option would be to have their food delivered to designated locations where they can pick it up in an orderly manner. Finally, residents in high-risk zones should receive home delivery, ensuring minimal contact and maximum safety. The efficiency of the end-delivery service is defined as the weight of goods delivered per unit time from retailers/ISs to residents according to the demand list. Generally, the delivery time for high-risk zones is longer than for medium-risk zones. Therefore, the end-delivery service efficiency can be expressed as
where AW is the average weight of goods per delivery and follows a uniform distribution U(a, b); thr and tmr are the time required to deliver a batch of goods to high-risk and medium-risk zones, respectively, and follow normal distributions N(μ, δ2).
2.1.3. Labor efficiency and transport efficiency
labor is a crucial element in the functioning of any supply chain network, and the efficiency of work directly affects the overall effectiveness of the network (Jaillet et al., 2019; Bhattarai and Reiley, 2020). In general, labor efficiency can be represented by the time required for each task and the number of tasks completed within a certain period of time. During an epidemic, it becomes imperative to choose the appropriate type and size of vehicles to achieve transport efficiency and meet supply chain objectives. Larger vehicles may decrease the frequency of transportation, but they also have a lower turnover rate for perishable goods, which can lead to increased spoilage. On the other hand, smaller trucks have a lesser carrying capacity but are more agile, making them suitable for emergency situations where quick transport dispatch is essential (Marusak, 2021). Assuming that the time required for each task follows a normal distribution, we can derive the average and standard deviation of labor efficiency for each task from historical data. Therefore, we can represent the average labor efficiency of unloading, prepacking, loading and transportation tasks of goods in the food supply chain by UL, Prep, L, Tr and follow some random distribution.
2.1.4. Supply chain delivery window
The supply chain delivery window is the time required from the supply side of raw materials to delivery to the consumer. It has received a lot of focus from researchers, particularly in the areas of production planning and delivery routing optimisation (da Silveira and Arkader, 2007; Benjamin and Beasley, 2010; Yeung et al., 2011). Uniquely, this paper aims to study the issue of supply chain delivery windows from a different perspective, with a particular focus on the allocation of labor in the food supply chain. Generally, the relief supplies dispatch centres cannot distribute all the relief supplies to the affected areas at once, and successive multi-batch are a time-saving supply method, as depicted in Figure 4. Suppose there are n nodes in the supply chain, each with an operating time ti, and let tes(j, i) denote the earliest start time of batch j at node i. Then we can calculate the earliest start time of batch j at node i as follows
Recursively, the supply chain delivery window for successive j batches are
Here, ti_max is the maximum value in the sequence ti.
3. Mathematical modeling of labor demand forecasting
Humanitarian relief efforts are critical during disasters, such as providing food, water, and medical care. It is essential to distribute relief supplies quickly, fairly, and accurately to rescue scenes (Starr and Van Wassenhove, 2014; Çankaya et al., 2019). The COVID-19 pandemic and quarantine interventions have disrupted the labor market and made it difficult for existing food supply chains to operate effectively. Despite labor being a key input in the food industry, it is often overlooked in food supply chain assessments (Wijnands and Ondersteijn, 2006). However, forecasting the workforce required for the operation of the ERFSC is crucial, as it is a vital input to the operation. In an ERFSC scenario that involves labor changes in the DCs, Details, and Deliveries, a mathematical model has been developed to optimize the allocation of the labor force, ensuring that the dispatch and distribution of the demanded food quantity are completed within the specified time period.
3.1. Assumptions
Considering the complexity of the model, the following assumptions were made:
(1) Only a few essential foodstuffs (rice, pulses, non-perishable vegetables, etc.) were studied and were well stocked.
(2) The total demand of the population is based on the resident population and is calculated according to the healthy dietary provisions per capita.
(3) Prepackaging processing and preserving residents' food demand orders to be delivered by weight.
(4) Government-authorized green passes are given to vehicles, and there are no restrictions on vehicle transport.
(5) Logistics vehicles and drivers are adequate and have uniform vehicle sizes.
(6) The completion time of the labor force is subject to a certain random distribution.
(7) Successive operations at each activity node are achieved through shift changes.
(8) The food supply chain is designed using lean logistics ideas, i.e. inventory is not considered here.
To facilitate the narrative, the symbols and their descriptions are presented as follows.
D(t): Total daily food demand in the region (kg).
d0: Daily average food requirement per capita (kg).
na0: Number of available workforce per shift in the DC.
nr0: Number of available workforce per shift in retail stores.
nd0: Number of available workforce per shift in end-delivery.
nhr: Number of people in high-risk areas within the supply range.
nmr: Number of people in medium-risk areas within the supply range.
α: Perturbation factor for food demand in high-risk areas.
β: Perturbation factor for food demand in medium-risk areas.
V: Maximum capacity of logistics vehicles (kg).
ρ: Transportation batch.
EDEhr: End-delivery efficiency in high-risk areas (kg/h).
EDEmr: End-delivery efficiency in medium-risk areas (kg/h).
UL: Unloading efficiency (kg/h).
Prep: Pre-packing efficiency (kg/h).
L: Loading efficiency (kg/h).
Tr: Transportation efficiency (kg/h).
T0: Demand cycle (h).
na: Number of personnel required in the DC.
nr: Number of personnel required in retail stores.
nd: Number of personnel required in end-delivery.
3.2. Modeling
In the model, the objective function (6) is the minimum labor demand to ensure the normal operation of the ERFSC; constraint (6-1) is the time to complete the full supply of regional food within a specified period of time; constraint (6-2) is the total dynamic demand for regional food; constraint (6-3) is the determination of transport batches based on total food demand and logistics vehicle capacity; Constraint (6-4) is the longest time spent by a node in the ERFSC; and constraints (6-5), (6-6), and (6-7) are the labor constraints at each activity node of the ERFSC.
3.3. Scenario design
The decrease in operators and increase in demand for food distribution following a regional outbreak has created difficulties in the operation of the ERFSC. Based on an analysis of the ERFSC operation description, it was found that DCs and retailers require workers with higher skills, while end-delivery services requires fewer skills. Therefore, to alleviate the labor shortage problem, volunteers can be recruited for end-delivery services work, thus shifting the bottleneck factor of labor shortage to the end-delivery services. In other words, when all available personnel are involved in the supply chain operation but the task cannot be completed, we lift the restriction on the number of end-delivery services workers and, in practice, solve the staff shortage by recruiting volunteers or community residents. The solution design technology roadmap is shown in Figure 5.
3.4. Solution
In optimising food supply chains, the main objectives generally include minimum transportation cost, minimum wastage rate, and minimum cycle time. To achieve this, the problem is usually modelled and solved using different machine learning techniques, including genetic algorithms, simulated annealing algorithms, ant colony algorithms, neural network algorithms, and mixed integer programming (Tarhan and Grossmann, 2008; Peidro et al., 2009; Govindan and Cheng, 2018; Chan et al., 2020; Altun et al., 2022). However, the complexity of the optimisation problem increases with the spatial and temporal scale of the supply chain, involving numerous participants and different operation cycles, as well as uncertainties such as policies, natural disasters, wars, and epidemics. To address this, complex supply chain design and optimisation have been made possible by the development of computing performance and various optimisation algorithms (Conti et al., 2009; Scott et al., 2013).
The mathematical model presented in Equation (6) reflects the changes in total demand and distribution schedules due to changes in personnel at risk in the region. The optimal matching of the number of laborers at each node of the food supply chain is required in order to meet the supply of basic household goods to the residents of the region within a specified time frame, which is a dynamic integer non-linear programming problem that can be solved by Algorithm 1.
The primary objective of this algorithm is to ensure the smooth operation of the emergency regional food supply chain, particularly during public health emergencies. It aims to determine the minimum labor force required for essential stages such as DC, retail, and end-delivery. The input parameters encompass personnel count, changes in the number of at-risk individuals, vehicle capacity, labor efficiency, transportation efficiency, and delivery efficiency. These data can be derived from historical and dynamically updated data analysis, making the algorithm user-friendly. The primary output is the labor demand for each operational node, which varies due to the random distribution of input parameters. To address this issue, we employ multiple iterations and average calculations to forecast labor requirements.
The fmincon function is a powerful optimization tool widely used for nonlinear constrained optimization problems. Its flexibility and versatility enable users to customize settings based on specific problem characteristics, resulting in effective optimization outcomes (Chuan et al., 2014). However, due to the limited research on labor demand forecasting in ERFSC, further data is needed to verify the validity of our proposed model across different scenarios.
In summary, the algorithm presents a viable solution for forecasting the minimum labor requirements in the main stages of ERFSC. Its readily available input parameters contribute to its broad applicability. Moreover, the algorithm can be customized for various scenarios, such as forecasting quantities for additional stages by including corresponding constraints in the model. However, the algorithm's repeated use of nested loops increases time and space complexity, signaling areas for further improvement in future studies. Additionally, reliance on the quality and accuracy of input data is an important consideration. Implementing techniques like data cleaning, preprocessing, multi-source data fusion, domain expertise application, error handling, and fault tolerance mechanisms can enhance the algorithm's reliability and robustness.
4. Case study
4.1. Background of the case
The Huaguoyuan region of Guiyang, China, which is known as the Asia's largest community, covers over 6,000 acres of land, with 12 main municipal roads, 10 shopping malls, and 27 subdivisions with 220 high-rise buildings. The area has a population of 430,000 residents and 1 million daily transients. In September 2022, an outbreak occurred between September 1 and September 30, 2022, making the Huaguoyuan region a representative and significant case study.
4.2. ERFSC network design
To address the challenge of supplying necessities to the residents of Huaguoyuan, a “multi-level distribution and pick-up” strategy was proposed. The strategy involves establishing a temporary DC in Huaguoyuan, adding additional ISs based on zone division and retailer operation status, and setting up buffer areas by buildings to form a straight-through organizational structure for the ERFSC. The DC location plays a crucial role in supply chain efficiency for aggregating and distributing products (Ge et al., 2022). The agricultural products DC is positioned on the primary traffic road and in the centre of Huaguoyuan to facilitate the flow of goods and allow only government-authorized vehicles and labor for supply assurance.
The ISs are placed close to the community to reduce the frequency and distance of residents moving outward. The buffer areas are situated in the open area directly adjacent to buildings and are managed by community managers or volunteers for order pick-up and/or home delivery. Figure 6 shows the design of the ERFSC, and Figure 7 demonstrates the workflow for the operation of the regional food distribution network in Huaguoyuan.
4.3. Labor demand forecasting model development and optimization
4.3.1. Data collection and analysis
Following the outbreak in Huaguoyuan, the local government implemented silent management and city closure interventions, and the total population of the region remained relatively constant at 430,000. The number of people at high and medium risk in the Huaguoyuan region was collected using various methods, such as telephone consultations with the community and internet searches, as shown in Table 1.
Other parameters derived from historical data from DCs, logistics companies, retailers, and delivery staff are presented in Table 2.
4.3.2. Mathematical modelling
To develop the labor demand forecasting model, it is important to consider the surge in demand for necessities during the epidemic, which led to residents stockpiling food due to the lack of a well-established food supply chain. The total demand for necessities in an area is proportional to the resident population, and fluctuations caused by the epidemic can be eliminated by designing an efficient food supply chain and delivery cycle. Assuming disturbance coefficients of 3 and 2 for food demand by residents in high and medium risk zones, respectively, and a required delivery cycle of 1 time per day, the model is developed as follows.
4.3.3. Model solution
The problem is a stochastic programming problem, and the optimal solution is characterized by stochasticity and instability due to the multiple stochastic parameters. To tackle this, it is proposed to use a sampling average approximation to obtain the expected value of the optimal solution, which will demonstrate the validity of the model and forecast the demand for labor at each activity node. This will provide an auxiliary scientific decision for practical work. First, by optimising the solution according to the available labor, i.e. with restrictions on na, nr and nd, which determines whether volunteers need to be recruited. Secondly, if there is a shortage of labor, the restriction on nd is removed and the number of additional volunteers needed is solved optimally. The problem is solved using Algorithm 1, and a sample of 1000 random optimal solutions are selected to calculate the mean value. The results are shown in Tables 3, 4.
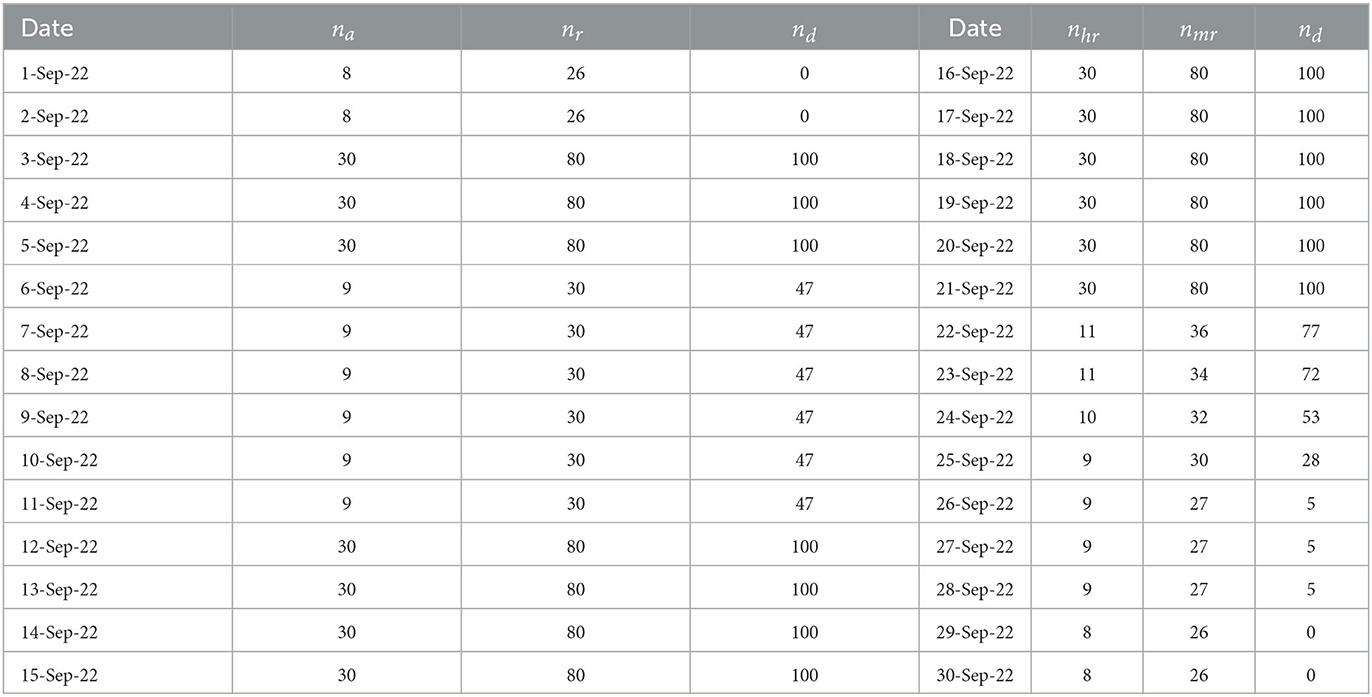
Table 3. Labor demand forecasting for the ERFSC in Huaguoyuan under the constraints of na, nr and nd (persons/shift).
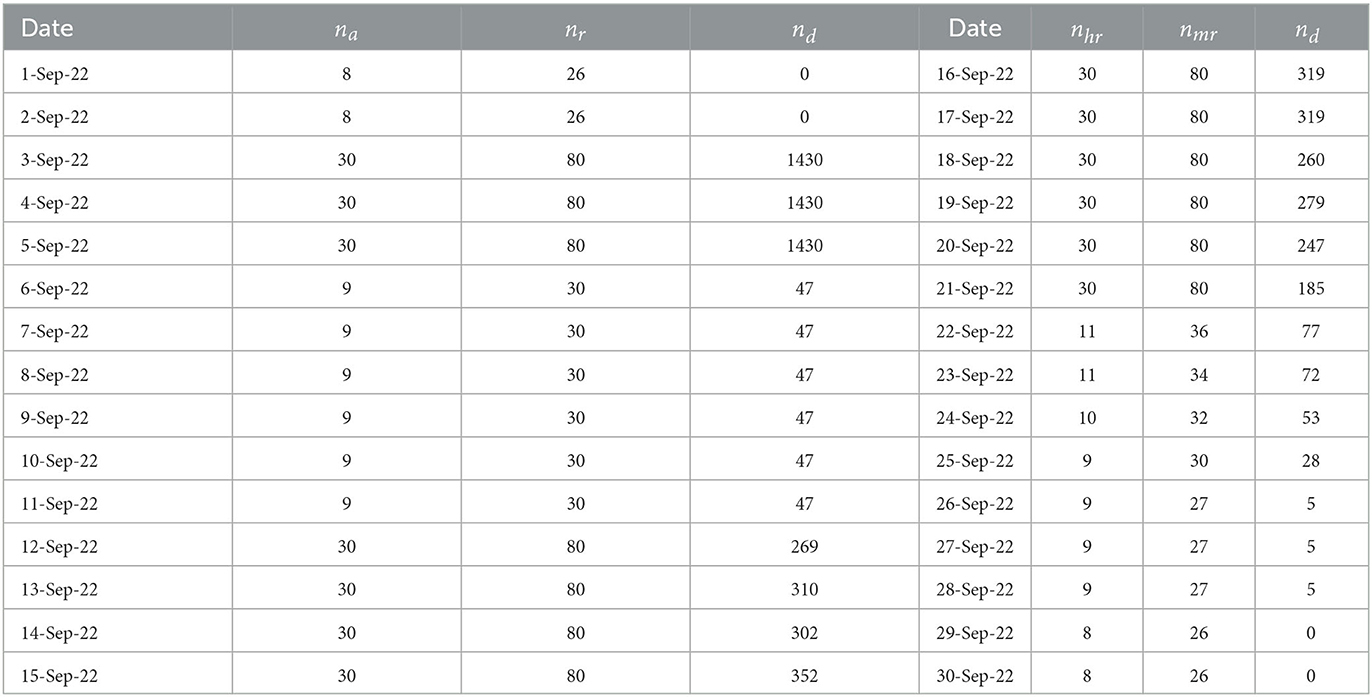
Table 4. Labor demand forecasting for the ERFSC in Huaguoyuan with the removal of nd restrictions (persons/shift).
4.3.4. Results
4.3.4.1. Content analysis
It can be seen from Tables 3, 4 that the demand for labor in the DCs, retailers and end-delivery services nodes of ERFSC changes with variations in the number of high- and medium-risk zones in the region. The demand for end-delivery services labor is particularly more sensitive to such changes, as illustrated in Figure 8. When there are no high- or medium-risk zones in the region, there is no need for additional end-delivery services staff. However, during periods of city-wide silent management, the demand for labor at each activity node of the ERFSC reaches its maximum, and the demand for end-delivery services staff increases dramatically, making it difficult for the existing end-delivery services system to cope, leading to delayed deliveries.
As the silent management interventions are lifted, residents in low-risk zones can shop offline, thereby reducing the demand for labor at each activity node of the ERFSC. In Table 3, when na, nr and nd all reach a threshold value, it indicates that the current labor force cannot complete the required task and must be increased. Table 4 provides the amount of labor required per shift, which can serve as a basis for adjusting the total labor demand for multiple shifts while planning staff requirements. Overall, the results of the analysis demonstrate the validity of the developed model and provide a scientific basis for decision-making in practical work. The use of the sampling average approximation technique enables the estimation of the expected value of the optimal solution, taking into account the stochastic nature of the problem and the instability caused by multiple parameters, thereby providing valuable insights for the management of labor resources in ERFSC.
4.3.4.2. Correlation analysis
To investigate the influence of the number of individuals in the risk area on the number of staff required at each node of the ERFSC, this study adopted Pearson correlation analysis combined with Tables 1, 4 to provide a quantitative description. Pearson correlation analysis is a statistical method used to measure the closeness of the linear relationship and the direction of correlation between two variables (Asuero et al., 2006; Sedgwick, 2012). Based on the calculations, as shown in Table 5, without controlling for variables, we found that nhr had correlation coefficients of 0.77, 0.971, and 0.996 with na, nr, and nd, respectively. This indicates a strong positive correlation between nhr and these three variables, all of which are statistically significant at a level below 0.01 (p-values: 0.000). Thus, we can reject the null hypothesis and conclude that there is a strong positive correlation between nhr and na, nr, and nd.
Similarly, we found that nlr had correlation coefficients of -0.835, -0.973, and -0.926 with na, nr, and nd, respectively. This suggests a strong negative correlation between nlr and these three variables, all of which are statistically significant at a level below 0.01 (p-values: 0.000). Therefore, we reject the null hypothesis and conclude that there is a strong negative correlation between nlr and na, nr, and nd. However, for the correlation between nmr and na, nr, and nd, we found correlation coefficients of 0.309, 0.215, and 0.063 respectively, with corresponding p-values of 0.097, 0.253, and 0.742. This indicates that in the specific context of this study, we cannot reject the null hypothesis and conclude that there is no correlation between nmr and na, nr, and nd. This conclusion may contradict our intuition. We speculate that this may be due to the smaller β values set in the model. However, the setting of β values must align with the actual situation and cannot be arbitrarily increased. Therefore, in the specific scenario of this study, our conclusion is valid.
The correlation analysis results mentioned above hold significant academic implications for studying the impact of the number of personnel in high-risk areas on the personnel requirements at various nodes of the emergency food supply chain. By exploring the strong positive correlation between nhr and na, nr, and nd, as well as the strong negative correlation between nlr and na, nr, and nd, we gain a better understanding of how changes in personnel numbers affect different nodes of the emergency food supply chain. Additionally, although no correlation was found between nmr and na, nr, and nd, this provides guidance and inspiration for further research to delve into the complex relationships among these variables.
In general, the analysis results confirm the efficacy of the developed model and provide scientific basis for decision-making in practical work. Given the stochastic nature of the problem and the instability caused by multiple parameters, a sampling average approximation method can be used to estimate the expected value of the optimal solution (Kleywegt et al., 2002; Verweij et al., 2003), providing valuable insights for labor resource management in the ERFSC.
These research findings hold academic significance as they demonstrate the potential of the model in managing food supply chains during emergencies. Moreover, the reliability and accuracy of the model serve as the foundation for further improvement and development by researchers. Future research can explore ways to optimize the parameter settings of the model in order to enhance its predictive performance and application scope.
5. Discussion
The outbreak of a public health emergency requires the establishment of an emergency food supply chain to ensure the basic needs of the population. In this study, we focused on constructing an ERFSC and distribution network to meet both the requirements of epidemic prevention and food supply guarantee. We emphasized the importance of labor force planning and assignment in the food supply chain, especially during times of crisis such as the outbreak of a pandemic. The scarcity of labor force in the food supply chain was identified as a critical challenge that needed to be addressed (Luckstead et al., 2021; Nagurney, 2021).
To overcome this challenge, we proposed to accurately forecast labor demand in each activity of the ERFSC by establishing labor demand forecasting models, which could provide valuable insights for companies to effectively manage and allocate their labor resources, ensuring the normal operation of the food supply chain and guaranteeing food security in society.
Furthermore, we highlighted the need for diversification in agricultural suppliers and the establishment of long-term strategic cooperation agreements with agricultural provinces and import agents. Additionally, involving local farmers and farms as suppliers can not only ensure regular food supply but also cater to the demand during emergencies. The role of supply and marketing cooperatives as local aggregation centers for high-quality locally grown products was also emphasized to strengthen the link between urban and rural food supply.
The optimization of layout distribution centers and connection points was identified as a necessary measure to be taken in case of disruptions in the existing food supply chain. Moreover, community engagement and mobilization of residents to participate in end-delivery were suggested as a practical solution when there is a shortage of labor force. In the long run, enhancing the development of the necessities industry chain and the construction of an information platform will promote the sharing of emergency material information resources and facilitate integration with the national emergency platform.
6. Conclusions
In this study, we have presented a comprehensive approach to address the disruptions in food supply chains during public health emergencies. Our research focuses on the design of ERFSC and the forecasting of labor demand, aiming to ensure the provision of necessities to affected areas.
To achieve this goal, we have proposed a multi-level ERFSC framework that can effectively adapt to different risk levels. The framework leverages local manufacturing and nearby sourcing strategies to enhance the resilience and sustainability of the supply chain (Alhawari et al., 2021; Boehme et al., 2021; Burgos and Ivanov, 2021). By utilizing local resources and optimizing logistics and supply chain infrastructure, the framework enables the rapid establishment of a coordinated ERFSC network. This network can dynamically adjust the food supply according to changes in the regional risk level, ensuring the continuous operation and efficient distribution of necessities such as food and protective equipment.
To accurately forecast food demand, we have developed a model that incorporates the trend of regional outbreaks. This model enables us to forecast the required food quantities for different risk level regions. By aligning the supply chain operations with the predicted demand, we can effectively meet the needs of disaster-affected populations for necessities.
Furthermore, we have formulated a stochastic planning model to determine the labor demand in the food supply chain during emergencies. This model allows for the swift allocation of the required workforce for the distribution of emergency food supplies. It ensures that the labor force is properly allocated based on the fluctuating demands, guaranteeing the timely delivery of relief food.
To validate the effectiveness of our proposed approach, we conducted a case study in the Huaguoyuan area of Guiyang, China. The results demonstrated that our models and frameworks are practical and effective in ensuring the provision and accurate distribution of necessities in regions with varying risk levels. The significance of this research rests in its contribution to the field of emergency management by providing a systematic and practical solution for the construction of ERFSC. By combining local resources and optimizing supply chain networks, our approach effectively addresses the challenges of food security and precise distribution during public health emergencies. Government agencies and practitioners can utilize our findings as a theoretical foundation for informed decision-making in developing food security measures and action plans.
While we have made important strides in this study, there are areas for future research. One such aspect is the need to further expand and refine our food demand forecasting model, particularly by considering additional factors such as geographical variations and policy frameworks. Moreover, the scalability and adaptability of our approach should be thoroughly examined in different geographical contexts and under various emergency scenarios.
In summary, our research provides valuable insights and practical guidance for designing ERFSC during public health emergencies. The proposed models and frameworks offer an effective means to ensure the continuous provision and efficient distribution of necessities This study contributes to the existing body of knowledge in emergency management and holds promise for practical applications.
Data availability statement
The datasets presented in this study can be found in online repositories. The names of the repository/repositories and accession number(s) can be found below: https://figshare.com/s/be2fc1e2039086f76444.
Author contributions
YM contributed to study concepts, data acquisition, manuscript revision/review, and manuscript final version approval. ST contributed to study design, literature research, experimental studies, data acquisition, data analysis/interpretation, statistical analysis, manuscript preparation, manuscript editing, and manuscript final version approval. All authors contributed to the article and approved the submitted version.
Funding
This research received partial support from the Growth Project of Young Scientific and Technological Talents in Colleges and Universities of Guizhou Province (Grant Qian Jiao he KY Zi [2021] 113). Additionally, it was supported by the Science and Technology Projects of Guizhou Province (QKHJCZK2022YB195, QKHJCZK2023YB143, and QKHPTRCZCKJ2021007), as well as the Natural Science Research Project of the Education Department of Guizhou Province (QJJ2023061 and QJJ2023012).
Acknowledgments
The authors would like to thank the management of the companies involved and the people who took time to attend interviews and complete questionnaires.
Conflict of interest
The authors declare that the research was conducted in the absence of any commercial or financial relationships that could be construed as a potential conflict of interest.
Publisher's note
All claims expressed in this article are solely those of the authors and do not necessarily represent those of their affiliated organizations, or those of the publisher, the editors and the reviewers. Any product that may be evaluated in this article, or claim that may be made by its manufacturer, is not guaranteed or endorsed by the publisher.
References
Aday, S., and Aday, M. S. (2020). Impact of covid-19 on the food supply chain. Food Qual. Safety 4, 167–180. doi: 10.1093/fqsafe/fyaa024
Alabi, M. O., and Ngwenyama, O. (2023). Food security and disruptions of the global food supply chains during covid-19: building smarter food supply chains for post covid-19 era. Br. Food J. 125, 167–185. doi: 10.1108/BFJ-03-2021-0333
Alhawari, O., Bhutta, K., and Muzzafar, A. (2021). Supply chain emerging aspects and future directions in the age of covid-19: a systematic review. Uncertain Supply Chain Manag. 9, 429–446. doi: 10.5267/j.uscm.2021.1.007
Ali, M. M., Babai, M. Z., Boylan, J. E., and Syntetos, A. A. (2017). Supply chain forecasting when information is not shared. Eur. J. Oper. Res. 260, 984–994. doi: 10.1016/j.ejor.2016.11.046
Altun, A.Köklü, M., et al. (2022). Optimizing the learning process of multi-layer perceptrons using a hybrid algorithm based on mvo and sa. Int. J. Ind. Eng. Comput. 13, 617–640. doi: 10.5267/j.ijiec.2022.5.003
Anesbury, Z., Nenycz-Thiel, M., Dawes, J., and Kennedy, R. (2015). How do shoppers behave online? an observational study of online grocery shopping. J. Consumer Behav. 15, 1566. doi: 10.1002/cb.1566
Asuero, A. G., Sayago, A., and González, A. (2006). The correlation coefficient: an overview. Crit. Rev. Anal. Chem. 36, 41–59. doi: 10.1080/10408340500526766
Banerjee, I., Warnier, M., Brazier, F. M., and Helbing, D. (2021). Introducing participatory fairness in emergency communication can support self-organization for survival. Sci. Rep. 11, 7209. doi: 10.1038/s41598-021-86635-y
Benjamin, A. M., and Beasley, J. E. (2010). Metaheuristics for the waste collection vehicle routing problem with time windows, driver rest period and multiple disposal facilities. Comp. Operat. Res. 37, 2270–2280. doi: 10.1016/j.cor.2010.03.019
Berti, G., and Mulligan, C. (2016). Competitiveness of small farms and innovative food supply chains: the role of food hubs in creating sustainable regional and local food systems. Sustainability 8, 616. doi: 10.3390/su8070616
Bjattarai, A., and Reiley, L. (2020, March 13). The companies that feed America brace for labor shortages and worry about restocking stores as coronavirus pandemic intensifies. The Washington Post.
Boehme, T., Aitken, J., Turner, N., and Handfield, R. (2021). Covid-19 response of an additive manufacturing cluster in australia. Int. J. Supply Chain Manag. 26, 767–784. doi: 10.1108/SCM-07-2020-0350
Burgos, D., and Ivanov, D. (2021). Food retail supply chain resilience and the covid-19 pandemic: a digital twin-based impact analysis and improvement directions. Transp. Res. Part C Emerg. 152, 102412. doi: 10.1016/j.tre.2021.102412
Çankaya, E., Ekici, A., and Özener, O. Ö. (2019). Humanitarian relief supplies distribution: An application of inventory routing problem. Ann. Oper. Res. 283, 119–141. doi: 10.1007/s10479-018-2781-7
Chan, F. T., Wang, Z., Goswami, A., Singhania, A., and Tiwari, M. K. (2020). Multi-objective particle swarm optimisation based integrated production inventory routing planning for efficient perishable food logistics operations. Int. J. Product. Res. 58, 5155–5174. doi: 10.1080/00207543.2019.1701209
Chitrakar, B., Zhang, M., and Bhandari, B. (2021). Improvement strategies of food supply chain through novel food processing technologies during covid-19 pandemic. Food Control 125, 108010. doi: 10.1016/j.foodcont.2021.108010
Christiaensen, L., Rutledge, Z., and Taylor, J. E. (2021). The future of work in agri-food. Food Policy 99:101963. doi: 10.1016/j.foodpol.2020.101963
Chuan, W., Lei, Y., and Jianguo, Z. (2014). “Study on optimization of radiological worker allocation problem based on nonlinear programming function-fmincon,”? in 2014 IEEE International Conference on Mechatronics and Automation. Tianjin: IEEE, 1073–1078. doi: 10.1109/ICMA.2014.6885847
Conti, S., Held, H., Pach, M., Rumpf, M., and Schultz, R. (2009). Shape optimization under uncertaintya stochastic programming perspective. SIAM J. Optimizat. 19, 1610–1632. doi: 10.1137/070702059
Coopmans, I., Bijttebier, J., Marchand, F., Mathijs, E., Messely, L., Rogge, E., et al. (2021). Covid-19 impacts on flemish food supply chains and lessons for agri-food system resilience. Agric. Syst. 190, 103136. doi: 10.1016/j.agsy.2021.103136
Cristiano, S. (2021). Organic vegetables from community-supported agriculture in italy: Emergy assessment and potential for sustainable, just, and resilient urban-rural local food production. J. Clean. Prod. 292, 126015. doi: 10.1016/j.jclepro.2021.126015
da Silveira, G. J., and Arkader, R. (2007). The direct and mediated relationships between supply chain coordination investments and delivery performance. Int. J. Oper. Prod. Manag. 27, 140–158. doi: 10.1108/01443570710720595
Das, D. N., Sewani, R., Wang, J., and Tiwari, M. K. (2020). Synchronized truck and drone routing in package delivery logistics. IEEE Trans. Intellig. Transp. Syst. 22, 5772–5782. doi: 10.1109/TITS.2020.2992549
Davila, F., Bourke, R. M., McWilliam, A., Crimp, S., Robins, L., Van Wensveen, M., et al. (2021). Covid-19 and food systems in pacific island countries, papua new guinea, and timor-leste: Opportunities for actions towards the sustainable development goals. Agric. Syst. 191, 103137. doi: 10.1016/j.agsy.2021.103137
Devereux, S., Béné, C., and Hoddinott, J. (2020). Conceptualising covid-19s impacts on household food security. Food Security 12, 769–772. doi: 10.1007/s12571-020-01085-0
Dixon, J. M., Weerahewa, J., Hellin, J., Rola-Rubzen, M. F., Huang, J., Kumar, S., et al. (2021). Response and resilience of asian agrifood systems to covid-19: an assessment across twenty-five countries and four regional farming and food systems. Agric. Syst. 193, 103168. doi: 10.1016/j.agsy.2021.103168
Dwivedi, Y. K., Shareef, M. A., Mukerji, B., Rana, N. P., and Kapoor, K. K. (2018). Involvement in emergency supply chain for disaster management: a cognitive dissonance perspective. Int. J. Product. Res. 56, 6758–6773. doi: 10.1080/00207543.2017.1378958
Fan, S., Si, W., and Zhang, Y. (2020). How to prevent a global food and nutrition security crisis under covid-19? China Agric. Econ. Rev. 12, 471–480. doi: 10.1108/CAER-04-2020-0065
Feldmann, C., and Hamm, U. (2015). Consumers perceptions and preferences for local food: a review. Food Qual. Prefer. 40, 152–164. doi: 10.1016/j.foodqual.2014.09.014
Food and Organization (2020). Urban Food Systems and COVID-19: The Role of Cities and Local Governments in Responding to the Emergency. Available online at: https://www.fao.org/policy-support/tools-and-publications/resources-details/en/c/1271238/ (accessed December 2, 2022).
Ge, H., Goetz, S. J., Cleary, R., Yi, J., and Gómez, M. I. (2022). Facility locations in the fresh produce supply chain: an integration of optimization and empirical methods. China Agric. Econ. Rev. 249, 108534. doi: 10.1016/j.ijpe.2022.108534
Goddard, E. (2020). The impact of covid-19 on food retail and food service in canada: Preliminary assessment. Can. J. Agricult. Econ 68, 157. doi: 10.1111/cjag.12243
Govindan, K., and Cheng, T. (2018). Advances in stochastic programming and robust optimization for supply chain planning. Comp. Operat. Res. 100, 262–269. doi: 10.1016/j.cor.2018.07.027
Hale, T., Angrist, N., Goldszmidt, R., Kira, B., Petherick, A., Phillips, T., et al. (2021). A global panel database of pandemic policies (oxford covid-19 government response tracker). Nature Human Behav. 5, 529–538. doi: 10.1038/s41562-021-01079-8
Harris, J., Depenbusch, L., Pal, A. A., Nair, R. M., and Ramasamy, S. (2020). Food system disruption: initial livelihood and dietary effects of covid-19 on vegetable producers in india. Food Security 12, 841–851. doi: 10.1007/s12571-020-01064-5
Hobbs, J. E. (2020). Food supply chains during the covid-19 pandemic. Can. J. Agric. Econ. 68, 171–176. doi: 10.1111/cjag.12237
Huang, X., Yang, S., and Wang, Z. (2021). Optimal pricing and replenishment policy for perishable food supply chain under inflation. Comp. Industrial Eng. 158, 107433. doi: 10.1016/j.cie.2021.107433
Ivanov, D., and Dolgui, A. (2020). Viability of intertwined supply networks: extending the supply chain resilience angles towards survivability. a position paper motivated by covid-19 outbreak. Int. J. Product. Res. 58, 2904–2915. doi: 10.1080/00207543.2020.1750727
Jaillet, P., Loke, G. G., and Sim, M. (2019). Strategic Manpower Planning Under Uncertainty. Rochester, NY: Social Science Electronic Publishing.
Jeong, H. Y., Song, B. D., and Lee, S. (2019). Truck-drone hybrid delivery routing: Payload-energy dependency and no-fly zones. Int. J. Prod. Econ. 214:220–233. doi: 10.1016/j.ijpe.2019.01.010
Kleywegt, A. J., Shapiro, A., and Homem-de Mello, T. (2002). The sample average approximation method for stochastic discrete optimization. SIAM J. Optimizat. 12, 479–502. doi: 10.1137/S1052623499363220
Lin, L., He, Z., and Peeta, S. (2018). Predicting station-level hourly demand in a large-scale bike-sharing network: a graph convolutional neural network approach. Transp. Res. Part C Emerg. 97, 258–276. doi: 10.1016/j.trc.2018.10.011
Luckstead, J., Nayga Jr, R. M., and Snell, H. A. (2021). Labor issues in the food supply chain amid the covid-19 pandemic. Appl. Econ. Perspect. Policy 43, 382–400. doi: 10.1002/aepp.13090
Mahajan, K., and Tomar, S. (2021). Covid-19 and supply chain disruption: Evidence from food markets in india. Am. J. Agric. Econ. 103, 35–52. doi: 10.1111/ajae.12158
Marusak, A., Sadeghiamirshahidi, N., Krejic, C., Mittal, A., Beckwit, S., Cantu, J., et al. (2021). Resilient regional food supply chains and rethinking the way forward: Key takeaways from the covid-19 pandemic. Agric. Syst. 190, 1. doi: 10.1016/j.agsy.2021.103101
Mollenkopf, D. A., Ozanne, L. K., and Stolze, H. J. (2021). A transformative supply chain response to covid-19. J. Serv. Manag. 32, 190–202. doi: 10.1108/JOSM-05-2020-0143
Montoya-Torres, J. R., Mu noz-Villamizar, A., and Mejia-Argueta, C. (2023). Mapping research in logistics and supply chain management during covid-19 pandemic. Int. J. Logistics Res. Appl. 26, 421–441. doi: 10.1080/13675567.2021.1958768
Moosavi, J., Fathollahi-Fard, A. M., and Dulebenets, M. A. (2022). Supply chain disruption during the covid-19 pandemic: recognizing potential disruption management strategies. Int. J. Disaster Risk Reduct. 75, 102983. doi: 10.1016/j.ijdrr.2022.102983
Moosavi, J., Naeni, L. M., Fathollahi-Fard, A. M., and Fiore, U. (2021). Blockchain in supply chain management: a review, bibliometric, and network analysis. Environm. Sci. Pollut. Res. 3, 1–15. doi: 10.1007/s11356-021-13094-3
Morganosky, M. A., and Cude, B. J. (2000). Consumer response to online grocery shopping. Int. J. Retail & Distribut. Manage. 28, 17–26. doi: 10.1108/09590550010306737
Mutebi, H., Muhwezi, M., and Munene, J. C. K. (2021). Self-organisation and supply chain agility: empirical evidence from humanitarian relief operations in a developing country. Int. J. Emerg. Servi. 10, 390–411. doi: 10.1108/IJES-07-2020-0044
Nagurney, A. (2021). Optimization of supply chain networks with inclusion of labor: Applications to covid-19 pandemic disruptions. Int. J. Prod. Econ. 235, 108080. doi: 10.1016/j.ijpe.2021.108080
Narayanan, L., Pandit, M., Basu, S., Karmakar, A., Bidhan, V., Kumar, H., et al. (2020). Impact of Lockdown due to COVID-19 Outbreak: Lifestyle Changes and Public Health Concerns in India.
Nguyen, T., Zhou, L., Spiegler, V., Ieromonachou, P., and Lin, Y. (2018). Big data analytics in supply chain management: A state-of-the-art literature review. Comp. Operat. Res. 2018, 98. doi: 10.1016/j.cor.2017.07.004
Nicomedes, C. J. C., and Avila, R. M. A. (2020). An analysis on the panic during covid-19 pandemic through an online form. J. Affect. Disord. 276:14–22. doi: 10.1016/j.jad.2020.06.046
Peidro, D., Mula, J., Poler, R., and Lario, F.-C. (2009). Quantitative models for supply chain planning under uncertainty: a review. Int. J. Adv. Manufact. Technol. 43, 400–420. doi: 10.1007/s00170-008-1715-y
Raison, B., and Jones, J. (2020). Virtual farmers markets: a reflective essay on a rural Ohio project. J. Agric. Food Syst. 9, 299–310. doi: 10.5304/jafscd.2020.094.020
Rosenzweig, C., Mbow, C., Barioni, L. G., Benton, T. G., Herrero, M., Krishnapillai, M., et al. (2020). Climate change responses benefit from a global food system approach. Nature Food 1, 94–97. doi: 10.1038/s43016-020-0031-z
Saul, J., Dowsett, S., and Baertlein, L. (2020). Western Supply Chains Buckle as Coronavirus Lockdowns Spread. London: Reuters.
Schnell, S. M. (2013). Food miles, local eating, and community supported agriculture: putting local food in its place. Agric. Human Values 30, 615–628. doi: 10.1007/s10460-013-9436-8
Scott, P., Thiébaux, S., Van Den Briel, M., and Van Hentenryck, P. (2013). “Residential demand response under uncertainty,”? in Principles and Practice of Constraint Programming: 19th International Conference, CP 2013, Uppsala, Sweden, September 16-20, 2013. Cham: Springer, 645–660.
Shah Alam Khan, M. (2008). Disaster preparedness for sustainable development in bangladesh. Disaster Prev. Manag. 17, 662–671. doi: 10.1108/09653560810918667
Shareef, M. A., Dwivedi, Y. K., Mahmud, R., Wright, A., Rahman, M. M., Kizgin, H., et al. (2019). Disaster management in bangladesh: developing an effective emergency supply chain network. Ann. Operat. Res. 283, 1463–1487. doi: 10.1007/s10479-018-3081-y
Sharma, D., Singh, A., Kumar, A., Mani, V., and Venkatesh, V. (2021). Reconfiguration of food grain supply network amidst covid-19 outbreak: an emerging economy perspective. Ann. Operat. Res. 2021, 1–31. doi: 10.1007/s10479-021-04343-2
Singh, S., Kumar, R., Panchal, R., and Tiwari, M. K. (2021). Impact of covid-19 on logistics systems and disruptions in food supply chain. Int. J. Product. Res. 59, 1993–2008. doi: 10.1080/00207543.2020.1792000
Starr, M. K., and Van Wassenhove, L. N. (2014). Introduction to the special issue on humanitarian operations and crisis management. Prod. Operat. Manage. 23, 925–937. doi: 10.1111/poms.12227
Sukhwani, V., Deshkar, S., and Shaw, R. (2020). Covid-19 lockdown, food systems and urban-rural partnership: Case of nagpur, india. Int. J. Environ. Res. Public Health 17, 5710. doi: 10.3390/ijerph17165710
Tarhan, B., and Grossmann, I. E. (2008). A multistage stochastic programming approach with strategies for uncertainty reduction in the synthesis of process networks with uncertain yields. Comp. Chem. Eng. 32, 766–788. doi: 10.1016/j.compchemeng.2007.03.003
Tarra, S., Mazzocchi, G., and Marino, D. (2021). Food system resilience during covid-19 pandemic: The case of roman solidarity purchasing groups. Agriculture 11, 156. doi: 10.3390/agriculture11020156
Thilmany, D., Canales, E., Low, S. A., and Boys, K. (2021). Local food supply chain dynamics and resilience during covid-19. Appl. Econ. Perspect. Policy 43, 86–104. doi: 10.1002/aepp.13121
Verweij, B., Ahmed, S., Kleywegt, A. J., Nemhauser, G., and Shapiro, A. (2003). The sample average approximation method applied to stochastic routing problems: a computational study. Comput. Optim. Appl. 24, 289–333. doi: 10.1023/A:1021814225969
Wang, G., Gunasekaran, A., Ngai, E. W. T., and Papadopoulos, T. (2016). Big data analytics in logistics and supply chain management: Certain investigations for research and applications. Int. J. Prod. Econ. 176, 98–110. doi: 10.1016/j.ijpe.2016.03.014
Wang, J., and Zhang, J. (2016). Big data analytics for forecasting cycle time in semiconductor wafer fabrication system. Int. J. Prod. Res. 54, 7231–7244. doi: 10.1080/00207543.2016.1174789
Wijnands, J., and Ondersteijn, C. J. (2006). Quantifying the agri-food supply chain: overview and new research directions. Frontis 2006, 1–10. doi: 10.1007/1-4020-4693-6
Yeung, W.-K., Choi, T.-M., and Cheng, T. (2011). Supply chain scheduling and coordination with dual delivery modes and inventory storage cost. Int. J. Prod. Econ. 132, 223–229. doi: 10.1016/j.ijpe.2011.04.012
Yin, S., Bai, L., and Zhang, R. (2021). Prevention schemes for future fresh agricultural products (faps) supply chain: mathematical model and experience of guaranteeing the supply of faps during the covid-19 pandemic. J. Sci. Food Agric. 101, 6368–6383. doi: 10.1002/jsfa.11308
You, Z., Si, Y. W., Zhang, D., Zeng, X. X., Leung, S. C. H., and Li, T. (2015). A decision-making framework for precision marketing. Expert Syst. Appl. Int. J. 42, 3357–3367. doi: 10.1016/j.eswa.2014.12.022
Yu, X., Liu, C., Wang, H., and Feil, J.-H. (2020). The impact of covid-19 on food prices in china: evidence of four major food products from beijing, shandong and hubei provinces. China Agricult. Econ. Rev. 12, 445–458. doi: 10.1108/CAER-04-2020-0054
Zebrowski, C. (2019). Emergent emergency response: speed, event suppression and the chronopolitics of resilience. Security Dialogue 50, 148–164. doi: 10.1177/0967010618817422
Keywords: COVID-19, emergency regional food supply chain (ERFSC), public health emergencies, necessities, end-delivery services, labor demand forecasting, interchange state
Citation: Tian S and Mei Y (2023) Emergency regional food supply chain design and its labor demand forecasting model: application to COVID-19 pandemic disruption. Front. Sustain. Food Syst. 7:1189451. doi: 10.3389/fsufs.2023.1189451
Received: 19 March 2023; Accepted: 07 September 2023;
Published: 27 September 2023.
Edited by:
Mirgissa S. Kaba, Addis Ababa University, EthiopiaReviewed by:
Janpriy Sharma, Dr. B. R. Ambedkar National Institute of Technology Jalandhar, IndiaShruti Mohapatra, Sri Sri University, India
Copyright © 2023 Tian and Mei. This is an open-access article distributed under the terms of the Creative Commons Attribution License (CC BY). The use, distribution or reproduction in other forums is permitted, provided the original author(s) and the copyright owner(s) are credited and that the original publication in this journal is cited, in accordance with accepted academic practice. No use, distribution or reproduction is permitted which does not comply with these terms.
*Correspondence: Yi Mei, eW1laUBnenUuZWR1LmNu