- 1International Institute of Tropical Agriculture, IITA, Ibadan, Nigeria
- 2Department of Plant Breeding and Seed Technology, Federal University of Agriculture, Abeokuta, Nigeria
Maize production in sub-Saharan Africa (SSA) faces challenges due to the damage caused by the parasitic weed, Striga hermonthica (Del.) Benths and low soil nitrogen. To address these constraints and improve food security and nutrition, this study assessed the combining ability of 47 inbred lines and four testers, grouped them into heterotic groups, identified effective testers, and determined the stability of the lines in hybrid combinations under contrasting research conditions. The study was conducted at Mokwa and Abuja during the 2019 and 2020 growing seasons. One hundred and ninety-six hybrids comprising 188 testcrosses, 6 hybrids derived by intermating the four testers, and two commercial checks were evaluated using a 14 × 14 lattice design with two replicates. Results revealed that under Striga infestation, the best quality protein maize (QPM) hybrid, TZEEQI 468 × TZEEQI 321, outyielded the best check, TZEEQI 342 × TZEEQI 7, by 24%. Under low-N, QPM hybrid, TZEEQI 515 × TZEEQI 321 outyielded the best check, TZEEQI 507 × TZEEQI 7 by 11% while under optimal conditions the best QPM hybrid, TZEEQI 506 × TZEEQI 321 outyielded the best check, TZEEQI 342 × TZEEQI 7 by 2%. General combining ability (GCA) and specific combining ability (SCA) significantly influenced grain yield and other measured traits across the test environments. These indicated the importance of both additive and non-additive genetic variances in trait inheritance. GCA was more important than SCA for grain yield and most traits in contrasting environments. Four inbred lines had significant and positive GCA effects for grain yield under Striga-infested conditions, while three lines had similar GCA effects under low-nitrogen conditions. These lines demonstrated outstanding potential for developing Striga-resistant and low-nitrogen-tolerant hybrids. The study identified four heterotic groups using the heterotic grouping method based on the general combining ability of multiple traits (HGCMAT). Inbred lines TZEEQI 490 and TZEEQI 460 were identified as testers. The QPM hybrid TZEEQI 515 × TZEEQI 321 exhibited outstanding yield and stability across contrasting environments, highlighting the need for extensive on-farm trials to confirm its superiority and potential for commercialization in SSA.
1. Introduction
Maize is the third most important stable cereal in the world, and the second major stable food crop in sub-Saharan Africa (SSA) (Ekpa et al., 2018; FAO, 2021). Over 300 million Africans rely on maize as a primary staple food, making it crucial for food security in the region (Thorne, 2002; VIB, 2017). More than two-thirds of the maize production constitutes over 20% of the food calorie intake for half of the population in SSA (Shiferaw et al., 2011). The poor population relies heavily on maize as a daily staple food as it serves as their primary source of calories, proteins, vitamins, and minerals in their diets (Badu-Apraku et al., 2015). However, normal endosperm maize lacks sufficient protein due to its low lysin and tryptophan content. These two amino acids are essential in human and livestock nutrition (Huang et al., 2004; Wu et al., 2010). As a result, efforts have been made to develop, disseminate, and encourage the adoption of various quality protein maize (QPM) varieties in West and Central Africa (WCA). The opaque-2 (o2) gene and modifiers that contain double the lysine (>4.0%) and tryptophan (>0.8%) contents compared to normal endosperm maize have been incorporated into the QPM varieties. This development has played a crucial role in reducing malnutrition in the region by enhancing the levels of quality protein in maize-based diets (Krivanek et al., 2007; Badu-Apraku et al., 2016b).
Maize production is predicted to be the highest in developing countries by the year 2025, and this demand is expected to double by 2050 (CIMMYT and IITA, 2010). However, maize productivity in SSA is faced with three major stresses, namely, low soil nitrogen, Striga infestation, and drought. These factors greatly limit the productivity of maize in SSA and are primarily attributed to the limited capacity of smallholder farmers to adapt to the effects of climate change (Mulungu and Ng’ombe, 2019).
Maize is a crop that thrives in fertile soils for optimal yield (Uribelarrea et al., 2009). However, soil fertility in the savannas is inherently low due to weathering and leaching, resulting in deficiencies in essential nutrients such as nitrogen (Edmeades et al., 1996; Koné et al., 2008). Nitrogen plays a vital role in stimulating vegetative growth, kernel initiation, and kernel set (Below, 1997). The average fertilizer use in SSA is reported to be 5 to 10 kg ha-1, which falls below the target of at least 50 kg ha-1 by 2015 set by the Fertilizer for the African Green Revolution for African Union Member States (African Development Bank, 2006; FAOSTAT, 2017). The increasing demand for higher grain yield to sustain the growing population, coupled with decreasing fertilizer use, poses a great challenge to food security in SSA. This therefore necessitates improved nitrogen use efficiency (Cassman et al., 2002; Zhang et al., 2015). Developing maize varieties through breeding for both high nitrogen-use efficiency and high yield potential offers a sustainable and economically viable solution to support the rapidly growing population, and address the needs of smallholder farmers in the sub-region.
Striga hermonthica is a parasitic weed that poses a significant threat to staple food crops such as maize, sorghum, and cowpea, among others. It is the primary factor limiting maize production in SSA (Ejeta, 2007; Parker, 2012). The weed is particularly prevalent in the savannas, which are known for their high maize yield potential (Yallou et al., 2009; Khan et al., 2014). Severe infestation by Striga can result in yield losses ranging from 10 to 100% (Lagoke et al., 1991; Odhiambo and Woomer, 2005; Abdulmalik et al., 2017). In extreme cases, farmers are compelled to abandon their fields due to total crop failure caused by Striga infestation (Ejeta, 2007; Atera et al., 2012). Several methods have been employed to control Striga, including handpicking, crop rotation, trap and cash crops, fertilizer application, fallowing, and seed treatments. However, these approaches have proven ineffective (Bebawi et al., 1984; Odhiambo and Ransom, 1994; Shaxson and Riches, 1998; Kountche et al., 2016). Host plant resistance or tolerance is therefore considered the most efficient, cost-effective, and sustainable strategy, especially for resource-limited farmers (Odhiambo and Ransom, 1994; Shaxson and Riches, 1998; Mandumbu et al., 2019). The savannah regions of West and Central Africa suffer from low soil nitrogen levels, as farmers apply minimal or no fertilizer, aggravating the problem of Striga infestation in maize farms (Badu-Apraku et al., 2018). Oswald and Ransom (2001) reported that the combined effects of Striga and low nitrogen can lead to yield losses of up to 100%. Consequently, there is a pressing need to breed and cultivate maize varieties with dual resistance to low nitrogen and Striga to meet the increasing maize demand driven by population growth in SSA countries.
Combining ability analysis of different maize inbred lines is a highly effective tool for identifying superior inbred combiners for the development of maize hybrids. It enhances the understanding of the genetic basis of different traits, thereby enabling the selection of appropriate parental lines based on their performance in crosses (Sprague and Tatum, 1942). Combining ability have been widely employed by breeders to select the best crossing pairs for hybrid development. Additionally, knowledge of gene action in maize inbred lines is important for devising suitable breeding strategies in breeding programs (Kamara et al., 2014).
Several authors have investigated the combining ability and gene action controlling the inheritance of grain yield under Striga resistance and low-N tolerance in maize inbreds. Some findings affirmed the preponderance of additive gene action in the inheritance of grain yield, Striga damage syndrome ratings, and Striga emergence (Ifie et al., 2015; Badu-Apraku et al., 2020; Oyekale et al., 2020; Abu et al., 2021) under Striga-infested conditions. Other authors affirmed the dominance of non-additive gene action in the expression of these traits (Badu-Apraku et al., 2016b; Akaogu et al., 2019; Annor et al., 2019). There has been conflicting information regarding how gene action influences grain yield under low soil N. Some authors affirmed that additive gene action was more important than non-additive gene action for grain yield and most associated traits under low soil N conditions (Tamilarasi et al., 2010; Makumbi et al., 2011; Badu-Apraku et al., 2016b; Obeng-Bio et al., 2019; Ribeiro et al., 2020). Other studies reported that non-additive gene action largely influenced the inheritance of grain yield under low soil nitrogen (Meseka et al., 2006; Ndhlela, 2012; Badu-Apraku et al., 2016b; Mafouasson et al., 2017). The conflicting findings among these researchers may have stemmed from variations in the levels of N stress under which the genotypes were tested (Mosisa et al., 2007). Most of these studies focused on white endosperm, intermediate, and early maturing maize inbred lines. To optimize the use of available extra-early quality protein maize inbred lines for breeding low N-tolerant and Striga-resistant hybrids at IITA, a true understanding of the combining ability of these inbred lines and the gene actions controlling the stresses is expedient. This will help to identify the inbred combiners that best exploit heterosis and to devise the best breeding strategy to be employed in breeding low-N tolerant and Striga-resistant maize hybrids.
Newly developed hybrids are often evaluated in multi-environment trials (MET) including favorable and unfavorable conditions. This results in a genotype by environment interaction (GEI), which is the differential performance of genotypes with respect to environmental variations (Alves et al., 2021). Genotype × environment interaction causes variability in grain yield outcomes across various environments, thereby complicating the identification of superior genotypes (Miah et al., 2016). Yield stability is therefore a paramount criterion for identifying newly developed hybrids with potential for commercialization. An important goal of a maize breeder is to develop and select hybrids that have high and stable yields across varying environmental conditions.
This study aimed to gather information regarding the combining ability and gene action that governs the inheritance of low soil nitrogen tolerance and Striga resistance in extra-early maturing QPM inbred lines. The findings will contribute to the development of maize cultivars suitable for farmers in SSA for cultivation under the conditions of low soil fertility and significant Striga infestation. The objectives of this study were as follows: (a) to determine the gene action that influences grain yield and other agronomic traits of extra-early white QPM inbred lines under low-nitrogen and Striga infestation, as well as optimal growing conditions; (b) to classify the inbred lines into heterotic groups using the heterotic grouping method based on the combining ability of multiple traits (HGCAMT) and identify suitable inbred testers; and (c) to assess the performance of the QPM inbred lines.
2. Materials and methods
2.1. Plant materials
The study involved 47 new inbred lines (Table 1), four standard IITA inbred testers, and two hybrid checks with combined resistance to Striga, tolerance to drought, and low soil N. The inbred lines were derived from F1 hybrids of nine bi-parental crosses involving crosses among extra-early white QPM inbred testers and early maturing white QPM inbred testers. The testers and the checks were extracted from Striga-resistant populations. The F1 hybrids were taken through a cycle of backcrossing to the extra-early inbred testers to recover the earliness. The BC1F1 with desirable agronomic characteristics were selected using the pedigree selection method from each backcrossed population and advanced through repeated inbreeding to the S8 generation. The QPM inbred lines were screened under a lightbox for desirable endosperm modification ranging from 25 to 50%. The QPM inbred line testers were identified as having positive and significant general combining ability from previous studies (Badu-Apraku et al., 2016b; Badu-Apraku and Fakorede, 2017). The 47 inbred lines were crossed with four standard IITA testers, TZEEQI 7, TZEEQI 134, TZEEQI 321, and TZEEQI 294, to generate 188 testcrosses. The four testers were intercrossed to obtain six hybrids which together with the two hybrid checks, TZEEQI 314 × TZEEQI 134 and TZEEQI 342 × TZEEQI 7, totaling 196 hybrids.
2.2. Experimental sites and field layout
The 196 hybrids were evaluated under low soil N, Striga-infested, and optimum conditions in 2019 and 2020. Evaluation under Striga-infested conditions was conducted in Mokwa (9o 18’N and 5o 04′E, 457 m asl, 1,100 mm annual rainfall) and Abuja (9o 15’N and 7o 20′E, 300 m asl, 1,700 mm annual rainfall) during the 2020 planting season. Evaluation under low N conditions was conducted in Mokwa in 2019 and 2020, while the optimum experiments were conducted in Mokwa in 2019 and 2020 and in Abuja in 2020. The experiments were laid out in a 14 × 14 lattice design with two replications. Each entry was evaluated in a one-row plot, 3 m long, spaced 0.75 m apart with 0.4 m between hills. Three maize seeds were sown per hill and thinned to two plants per hill 2 weeks after planting, to obtain a final population density of 66,666 plants per ha. For the low soil nitrogen tolerance experiment, the experimental field was depleted of nitrogen over several years by repeatedly planting maize crops and removing the plant biomass after each harvest. Soil samples were collected annually before planting in the test environments. These soil samples were then analyzed for nitrogen content at the IITA soil laboratory located in Ibadan.
The total N in the soils was determined by Kjeldahl digestion using the colorimetric method of Technician AAII Autoanalyzer (Bremner and Mulvaney, 1982). Fertilizer was applied to bring the total available N to 30 kg ha−1. Also, single superphosphate (P2O5) and muriate of potash (K2O) were applied to the low N plots at the rate of 60 kg N ha−1. Fertilizer was applied at the rate of 90 kg N ha−1, 60 kg ha−1 each of P2O5, and K2O at 3 WAP and an additional 60 kg N ha−1 was top-dressed as urea at 5 WAP on the optimum experiment. The trials were kept weed-free with the application of pre-emergence (Primextra Gold) and post-emergence (Gramoxone) herbicides supplemented with manual weeding.
For the Striga-infested condition, each plot was artificially infested with approximately 5,000 germinable S. hermonthica seeds/hill. The Striga infestation method developed by IITA Maize Improvement Programme was adopted to ensure uniform Striga infestation with no escapes (Kim, 1991; Kim and Winslow, 1991). Approximately 30 kg of nitrogen fertilizer per hectare (ha-1) was applied, with the application split into two stages. The first application was deliberately delayed until 21 days after planting, creating stress conditions to stimulate the production of strigolactones in the maize plants. This strategy aimed to enhance the germination of Striga seeds and promote the attachment of Striga plants to the roots of maize plants. A top dressing of fertilizer was done 35 days after planting. To maintain effective weed control, manual removal of weeds other than Striga was constantly done by hand.
2.3. Data collection
Data were collected for grain yield and other agronomic traits under Striga, low-N and optimal research conditions as shown in Table 2.
2.4. Data analysis
Plot means were subjected to analysis of variance (ANOVA) under each research condition (low soil N, Striga-infested, and optimal environments) for the hybrids and the checks using general linear model procedure in Statistical Analysis System (SAS Institute, 2012 version 9.3). Data on traits common to the three research conditions were pooled for combined ANOVA. Using a RANDOM statement with the TEST option where block was nested within replicate × environment and replicate nested within environment. Environments were random effects while genotypes were fixed effects. Line × tester analysis was carried out following the procedure of Singh and Chaudhary (1977). General combining ability (GCA) and specific combining ability (SCA) and their standard errors were computed for grain yield and other measured traits under each research condition. The variation among hybrids was partitioned into sources due to lines, testers, and line × testers. The main effects of line and tester were represented by GCA-line and GCA-tester respectively, while the main effect of line × tester was represented by SCA. The GCA of a tester (male) was obtained based on its performance in F1 hybrid combinations with all possible lines (females). Also, the GCA of each line was determined based on the performance of the F1 hybrid with the four testers.
GCA and SCA effects were determined for each trait under each research condition and across research conditions. The general linear model for line by tester mating design is:
where Uijbkl = observed value of the progeny between the ith line and the jth tester in the bth block within the kth replication in the lth environment, μ = population mean, Al = average effect due to environment, Æb(k) = effect of block nested within replication, Γk(l) = effect of replication nested within environment, gi = general combining ability (GCA) of the ith line, gj = general combining ability (GCA) effect of the jth tester, sij = specific combining ability (SCA) of the ijth testcross, (Av)ijl = effect of interaction between the ijth testcross and lth location, and Eijbkl = residual effect.
Broad-sense heritability values were estimated for each trait under each research condition as follows:
where is the genotype x environment interaction, is the genotypic variance, is the error variance, is the number of replicates per environment, and is the number of environments (Fehr, 1991).
The proportionate contribution of GCA to SCA sum of squares for grain yield and other agronomic traits was determined using the ratio proposed by Baker (1978). The inbred lines were classified into heterotic groups based on GCA effects of multiple traits (HGCAMT) (Badu-Apraku et al., 2013). The grouping was achieved by standardizing the GCA effects with a mean of zero and standard deviation of 1 for traits measured that had significant genotypic mean squares and GCA across test environments using the following statistical model:
where Y is HGCAMT, representing the genetic value that measured the relationship among genotypes using the GCA of multiple traits i to n; is the individual GCA effects of genotypes for trait i, is the mean of GCA effects across genotypes for trait i; s is the standard deviation of the GCA effects of trait i, and is the residual of the model associated with the combination of inbred i and j.
Identification of inbred testers was carried out using the criteria proposed by Pswarayi and Vivek (2008), which states that an inbred line should (i) belong to a heterotic group, (ii) have significant and positive GCA effects across the test conditions, and (iii) have a high per se yield performance. To identify desirable hybrids across test conditions, the multiple trait base index (MI) proposed by Badu-Apraku et al. (2011a,b, 2016b) was calculated. The MI integrated grain yield, number of ears per plant, plant aspect, ear aspect, stay green characteristic, Striga damage ratings at 8 and 10 WAP as well as number of emerged Striga plants at 8 and 10 WAP as follows:
where: YLD = grain yield across conditions; EPP = number of ears per plant across conditions; EASP = Ear aspect across conditions; PASP = Plant aspect across environments; SGR = Stay green characteristic across low-N environments; SDR8 and SDR10 = Striga damage rating at 8 and 10 WAP across Striga environments; ESP8 and ESP10 = Number of emerged Striga plants at 8 and 10 WAP across Striga environments.
A set of 20 best hybrids and five worst hybrids and the two QPM checks were thereafter selected and subjected to a GGE biplot to determine the stability of performance across test environments. The “mean vs. stability” view of the GGE biplot was employed to identify hybrids with high grain yield and stability across the Striga-infested, low-nitrogen, and optimal environments. The GGE biplot model equation used was as follows:
where: Yij is the average yield of genotype i in environment j; Yj is the average yield across the genotypes in environment j; λ1 and λ2 are the singular values for PC1 and PC2, respectively; ξ1 and ξ2 are the PC1 and PC2 scores, respectively, for genotype i, ηj1 and ηj2 are the PC1 and PC2 scores, respectively for environment j; βj is the mean of all combinations involving trait j; dj is the standardization value (with mean of zero and standard deviation of 1); and εij is the residual of the model associated with the genotype I in environment j.
3. Results
3.1. Performance of hybrids under Striga-infested, low N, and optimal conditions
Under Striga-infested conditions (Table 3), analysis of variance (ANOVA) revealed significant (p < 0.05) environment variance for measured traits except for ear aspect. Significant differences (p < 0.05) for the hybrids were also observed for the measured traits except for the anthesis-silking interval and number of ears per plant. Hybrid × environment interaction variance was not significant for most of the measured traits except plant and ear height (p < 0.05/0.01). Using the line × tester analysis to partition the genotype variances into its GCALine, GCATester, and SCA components, significant (p < 0.05/0.01) GCALINE variance was observed for measured traits except for grain yield, Striga damage syndrome rating at 8 WAP, and number of ears per plant. GCATester variance was significant for measured traits except for Striga damage syndrome ratings at 10 WAP and number of ears per plant while SCA variance was significant for only emerged Striga count at 8 WAP and ear aspect. Similarly, environment × GCALine variance was significant only for emerged Striga count at 8 WAP, emerged Striga count at 10 WAP, and anthesis-silking interval (p < 0.05), while the environment × GCATester variances were significant for Striga damage syndrome ratings at 8 WAP, emerged Striga count at 10 WAP, days to 50% anthesis, days to 50% silking, and anthesis-silking interval. Environment × SCA variance was only significant for ear height (p < 0.01). The broad sense heritability (H) ranged from 9% for emerged Striga count to 79% for days to 50% anthesis.
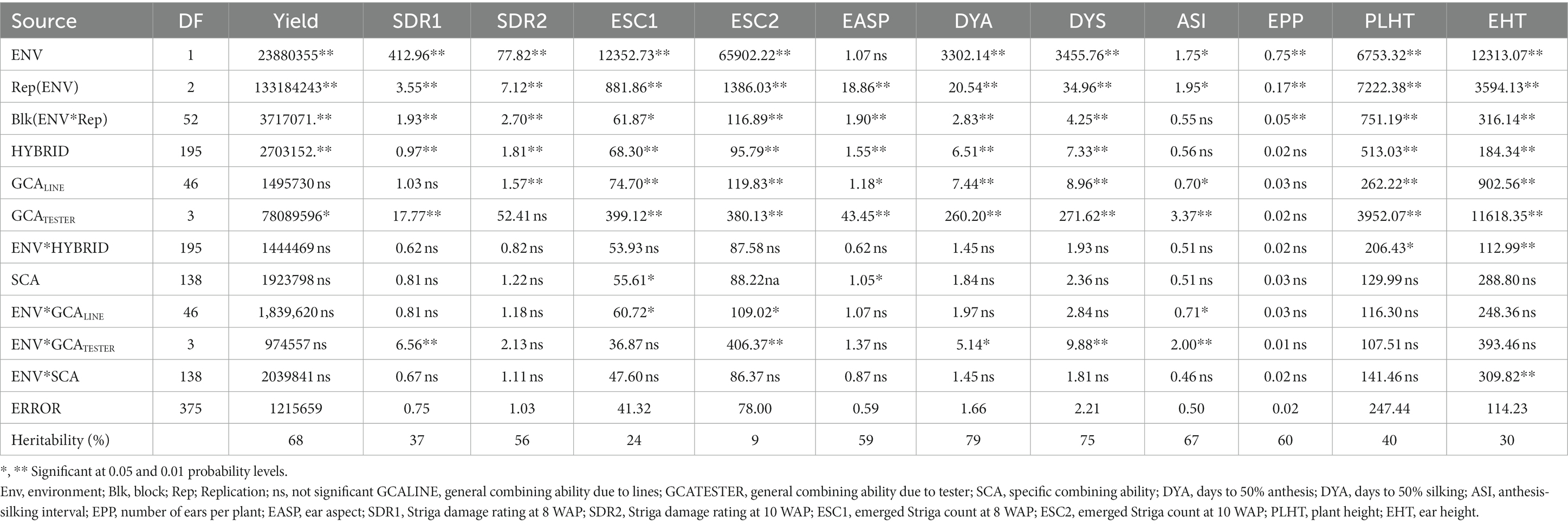
Table 3. Mean squares of grain yield and other agronomic traits of 188 extra-early maturing quality protein maize hybrids and 8 checks evaluated across Striga-infested conditions in Mokwa and Abuja, 2020.
Under low N conditions (Table 4), the environment was significant for most measured traits except for the stay-green characteristic, ear aspect, number of ears per plant, and plant height. Hybrid was significant for all measured traits except ears per plant, anthesis-silking interval, plant height, and ear height. Environment × hybrid was significant for only grain yield, days to 50% anthesis, and number of ears per plant. GCALine variance was significant for grain yield, plant aspect, stay-green characteristics, days to 50% anthesis, days to 50% silking, number of ears per plant, and plant height. GCATester variance was significant for all measured traits except ear aspect, anthesis-silking interval, and ear height while the SCA variance was significant for only grain yield and number of ears per plant. Similarly, environment × GCALine was significant for only the number of ears per plant while environment × GCATester variance was significant for grain yield, days to 50% anthesis, days to 50% silking, and number of ears per plant. Also, environment × SCA variance was significant for only the number of ears per plant. Broad sense heritability (H) estimates of the studied traits ranged from 4% for the number of ears per plant to 55% for ear aspect.
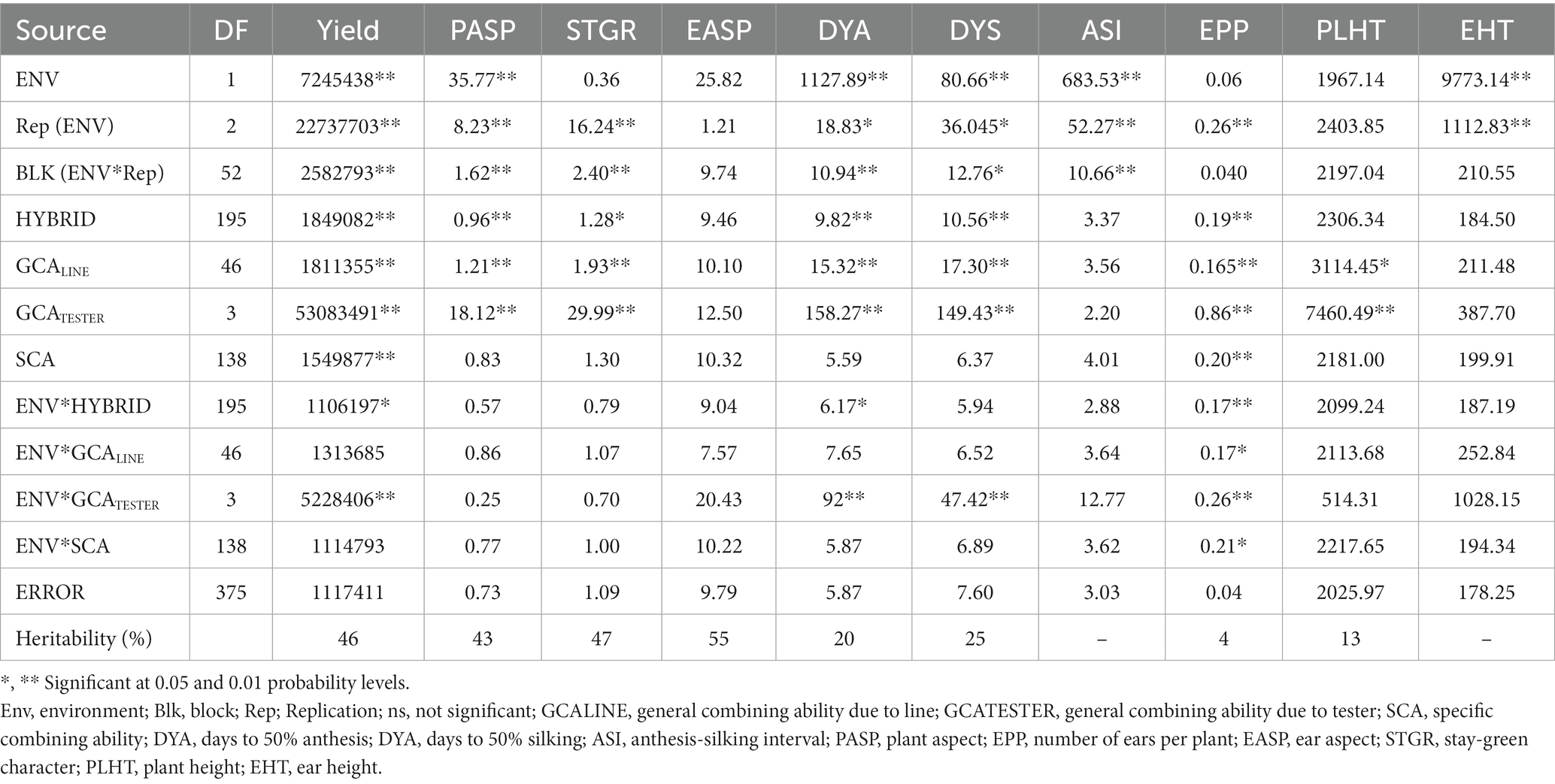
Table 4. Mean squares of grain yield and other agronomic traits of 188 extra-early maturing quality protein maize hybrids and 8 checks evaluated across low soil N conditions in Mokwa, 2019 and 2020.
Under optimal conditions (Table 5), environment was significant (p < 0.01) for all measured traits, while hybrid mean squares was significant for measured traits except the anthesis-silking interval. Similarly, the environment × hybrid was significant for grain yield, days to 50% anthesis, days to 50% silking, plant height, and ear height. GCALine was significant for all measured characters except for anthesis-silking and the number of ears per plant. GCATester variance was significant for all measured traits and SCA variance was significant for only days to 50% anthesis, days to 50% silking, plant height, and ear height. Environment × GCALine was significant for days to 50% anthesis, days to 50% silking, plant height, and ear height. Significant environment × GCATester variance was observed for most of the measured traits except for days to 50% silking and the number of ears per plant. There was significant environment × SCA interaction for the measured traits days to 50% anthesis, days to 50% silking, and plant height. Broad sense heritability estimates ranged from 29% for ears per plant to 78% for ear aspect.
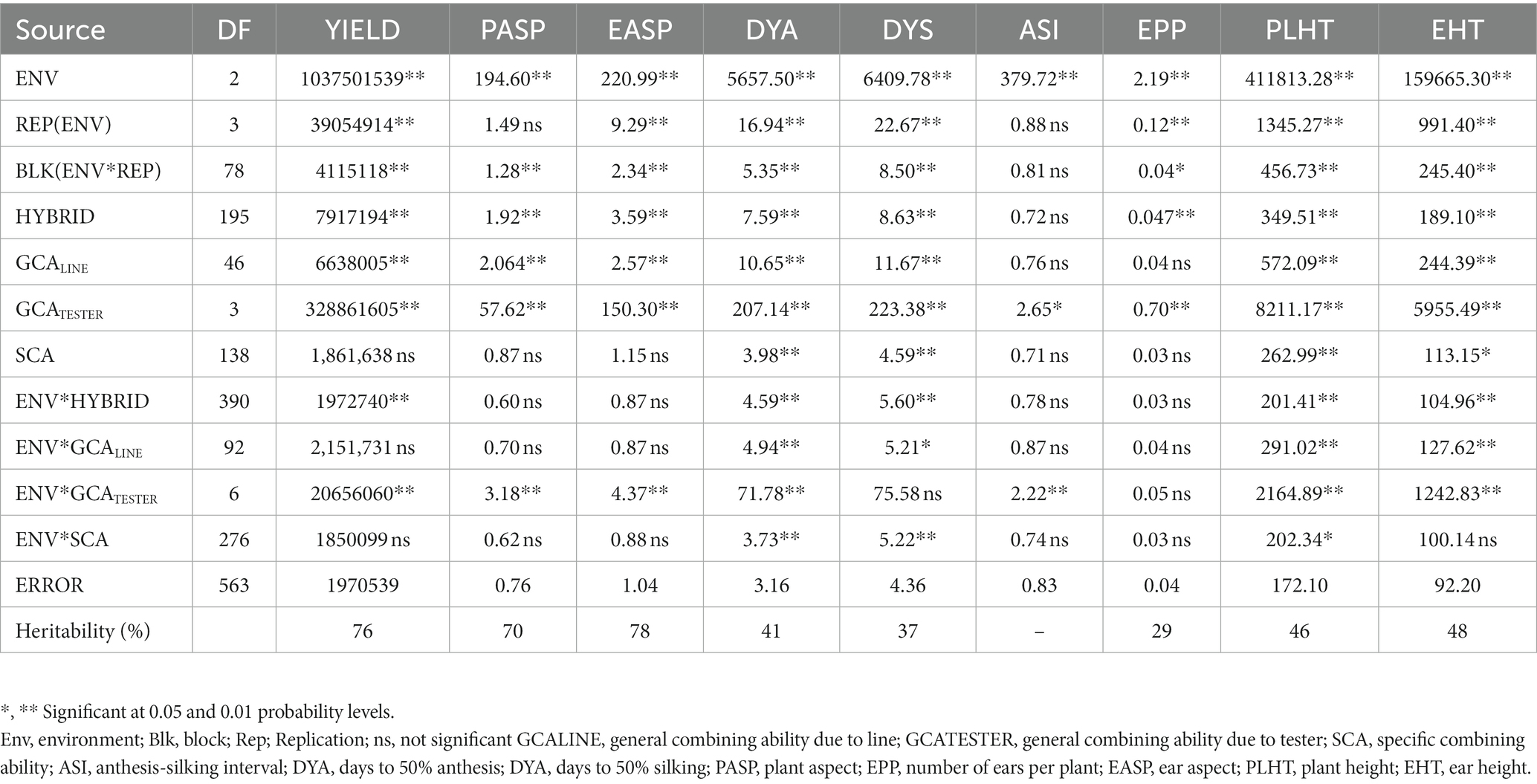
Table 5. Mean squares of grain yield and other agronomic traits of 188 extra-early maturing quality protein maize hybrids and 8 checks evaluated across optimal conditions in Mokwa 2019 and 2020 and Abuja, 2020.
3.2. Relative contributions of combining ability effects across Striga-infested, low N, optimal, and research environments
The relative importance of GCA and SCA variances was determined as the ratio of GCA variance to the total genetic variances using the sum of squares. The closer the ratio is to unity, the greater the predictability based on GCA (Baker, 1978). The percentage contribution of GCA to the overall genotypic sum of squares for grain yield was greater than that of SCA under Striga-infested, low-N, optimal, and across research conditions (Table 6). Under Striga-infested environments, the overall contributions of GCA effects to the total genotypic variation among hybrids ranged from 26.29% for the number of ears per plant to 81.53% for days to 50% anthesis, while the SCA variance ranged from 18.46% for days to 50% anthesis to 73.71% for the number of ears per plant. GCA variance accounted for 60.60% of the variations in grain yield, 47.48% for Striga damage at 8WAP, 57.60% for Striga damage rating at 10WAP, and 37.65 and 35.34% for the number of emerged Striga plants at 8 and 10 WAP, respectively. The percentage contribution of SCA for Striga damage rating at 8 WAP was 52.52%, for Striga damage rating at 10 WAP was 42.40%, emerged Striga plants at 8 WAP was 62.35%, emerged Striga plants at 10 WAP was 64.66%, the anthesis-silking interval was 62.46%, and the number of ears per plant was 73.71%. The percentage contribution of GCA variance for Striga damage rating at 10 WAP (57.60%), ear aspect (55.92%), days to 50% anthesis (81.52%), days to 50% silking (79.02%), plant height (65.71%), and ear height (57.14%) were greater than that of SCA.
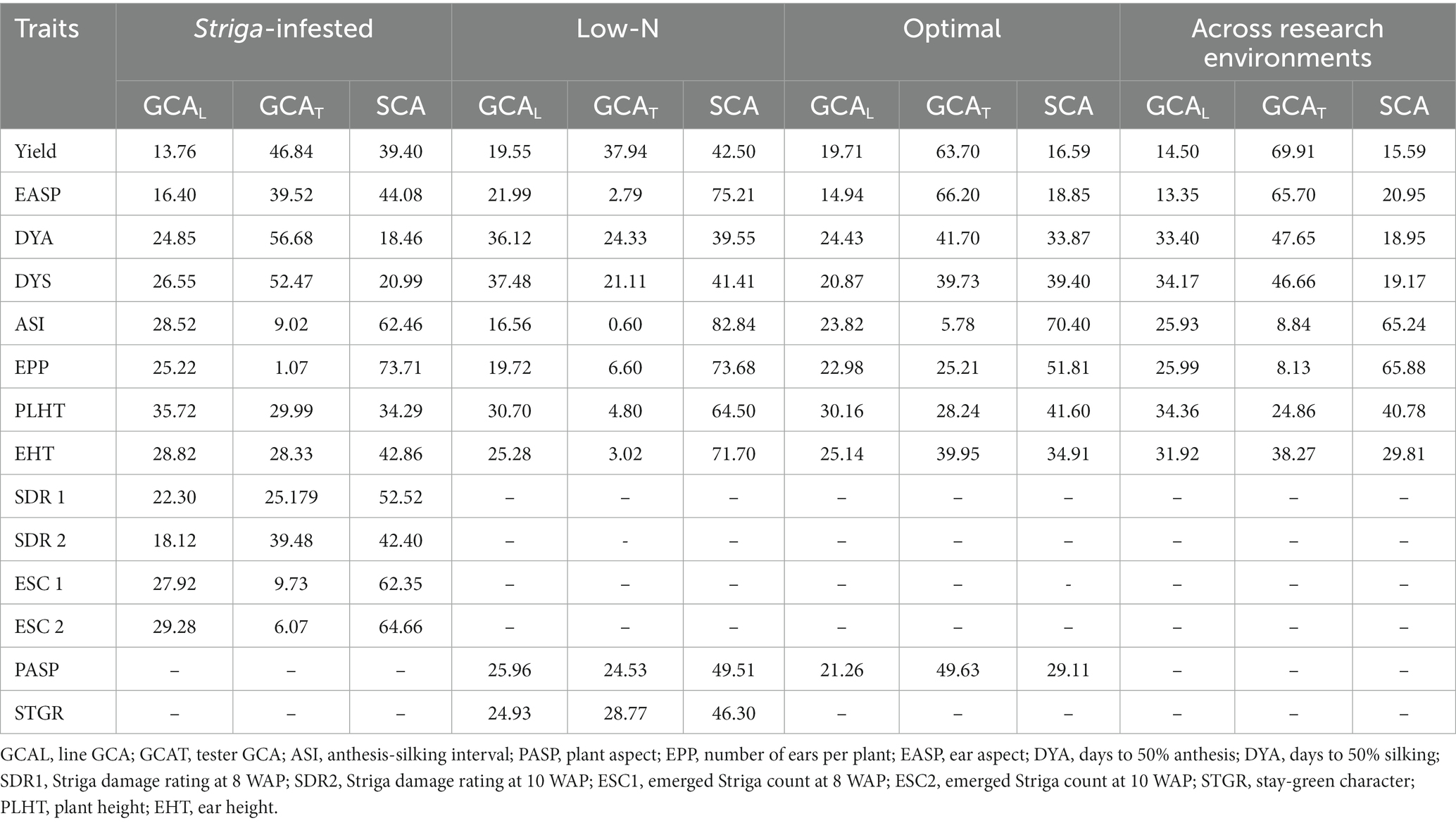
Table 6. Proportion of the sums of squares attributable to general combining ability (GCA) and specific combining ability (SCA) for grain yield and other agronomic traits of extra-early QPM inbred lines under Striga-infested, low-N, optimal, and across research environments.
Under low soil N environments, the percentage contribution of GCA to the total variance ranged from 17.16% for the anthesis-silking interval to 60.45% for days to 50% anthesis, while SCA contributions varied from 39.55% for days to 50% anthesis to 82.84% for the anthesis-silking interval.
The percentage contribution of GCA for grain yield (57.50%), stay-green characteristic (53.70%), plant aspect (50.49%), days to 50% anthesis (60.45%), and days to 50% silking (58.59%) were higher than SCA, while SCA contributions for ear aspect (75.21%), anthesis-silking interval (82.84%), number of ears per plant (73.68%), plant height (64.50%), and ear height (71.70%) were higher than the corresponding GCA.
Across optimal research conditions, the percentage contribution of GCA to the total variation varied from 29.60% for anthesis-silking interval to 83.41% for grain yield, while SCA varied from 16.59 to 70.40% for grain yield and ASI, respectively. Percentage contributions of GCA was higher for ear aspect (81.15%), plant aspect (70.89%), days to 50% anthesis (66.13%), days to 50% silking (60.60%), plant height (58.40%), and ear height (65.09%), while the percentage contribution of SCA was 51.81% for the number of ears per plant and 70.40% for the anthesis-silking interval.
Across research conditions, the percentage contribution of GCA varied from 34.12% for the number of ears per plant to 84.41% for grain yield, while the SCA varied from 15.59% for grain yield and 65.88% for the number of ears per plant. The percentage variation due to GCA for grain yield was 84.41, 79.05% for ear aspect, 81.05% for days to 50% anthesis, 80.83% for days to 50% silking, 59.22% for plant height, and 70.19% for ear height. However, the percentage variation due to SCA was higher for the anthesis-silking interval (65.24%) and the number of ears per plant (65.88%).
3.3. General combing ability effects for grain yield and other tolerance/resistance indicator traits under Striga infestation, low N, and optimal conditions
The estimates of the GCA effects of the 47 inbred lines evaluated under Striga-infested conditions are presented in Table 7. The GCA effects for grain yield ranged from-592.73 for TZEEQI 498 to 744.26 for TZEEQI 468. Significant and positive GCA effects were observed for inbreds TZEEQI 428, TZEEQI 468, TZEEQI 490, and TZEEQI 506, while TZEEQI 425, TZEEQI 451, and TZEEQI 498 had significant and negative GCA effects for grain yield. The GCA effects of TZEEQI 436 and TZEEQI 451 were significant and positive for Striga damage ratings at 8 WAP, while TZEEQI 490 had significant and negative GCA effects. Inbreds TZEEQI 436, TZEEQI 451, and TZEEQI 470 had significant and positive GCA effects for Striga damage ratings at 10 WAP, while TZEEQI 489 had significant and negative GCA effects. Also, significant, and positive GCA effects were observed for TZEEQI 425 and TZEEQI 481 for the number of emerged Striga plants at 8 and 10 WAP. However, a significant and negative GCA effect was observed for TZEEQI 456 for emerged Striga plants at 8 WAP, and for TZEEQI 468, TZEEQI 475, and TZEEQI 498 for Striga plants at 10 WAP.
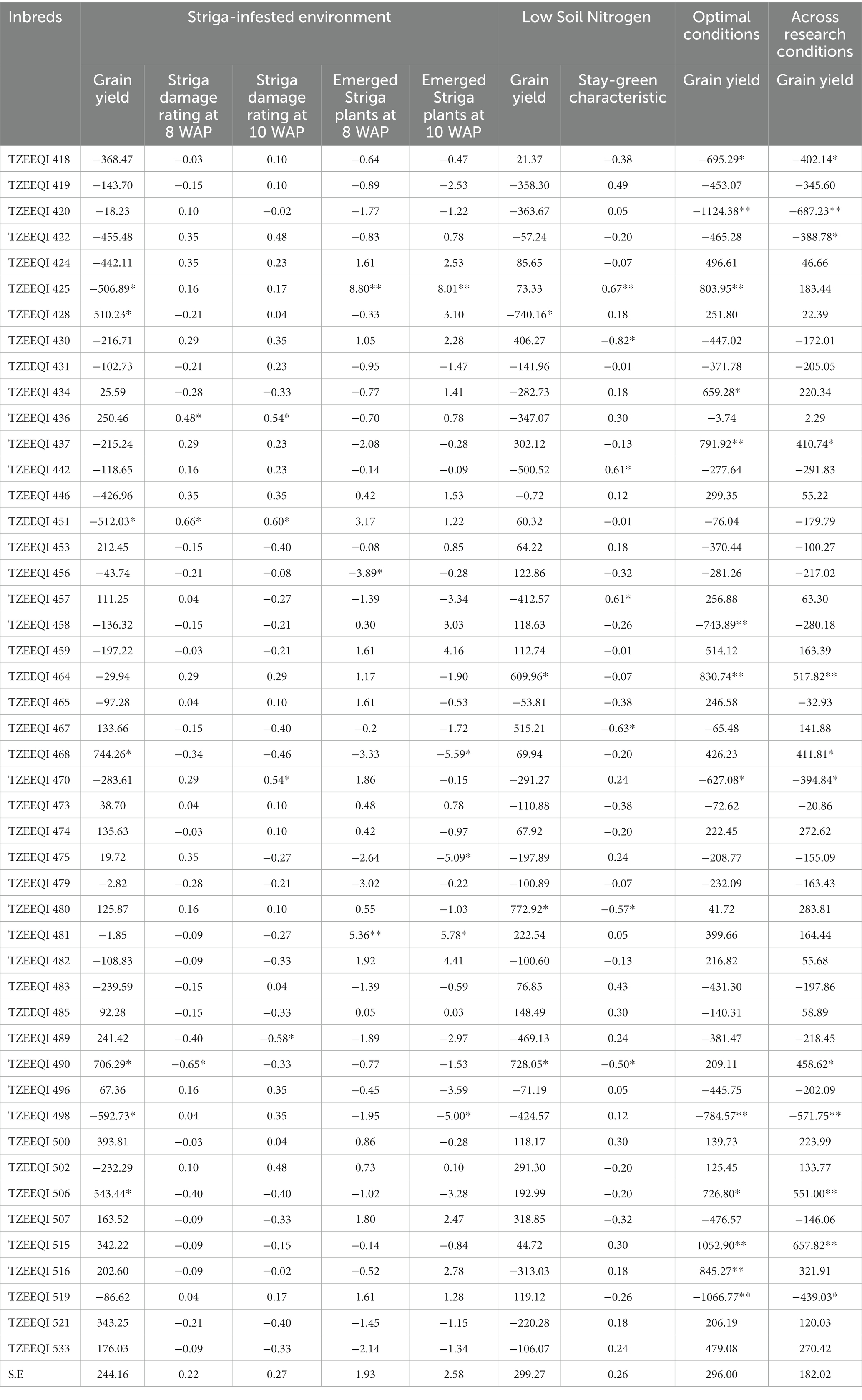
Table 7. GCA effects of the 47 extra-early QPM inbred lines for grain yield and other agronomic traits evaluated under Striga-infested, low-N, optimal, and across research environments.
The GCA effects of grain yield of the 47 extra-early maturing QPM inbreds under low soil N ranged from-740.16 for TZEEQI 428 to 772.92 for TZEEQI 480. Inbreds TZEEQI 490, TZEEQI 480 and TZEEQI 464 had significant and positive GCA effects, while TZEEQI 428 had significant and negative GCA effects for grain yield. Significant and positive GCA effects for the stay-green characteristic were observed for inbreds TZEEQI 425, TZEEQI 442, and TZEEQI 457, while inbreds TZEEQI 430, TZEEQI 467, TZEEQI 490, and TZEEQI 480 had significant and negative GCA effects for the stay green characteristic.
The GCA effects for grain yield for optimum conditions ranged from −1124.38 for TZEEQI 420 to 1054.9 for TZEEQI 515. Significant and positive GCA effects were recorded for TZEEQI 425, TZEEQI 434, TZEEQI 437, TZEEQI 464, TZEEQI 506, TZEEQI 515 and TZEEQI 516, while inbreds TZEEQI 418, TZEEQI 420, TZEEQI 458, TZEEQI 470, TZEEQI 498 and TZEEQI 519 had significant and negative GCA effects.
Across the research conditions, GCA effects for grain yield ranged from −687.23 for TZEEQI 420 to 657.82 for TZEEQI 515. Inbreds TZEEQI 437, TZEEQI 464, TZEEQI 490, and TZEEQI 515 had significant and positive GCA effects for grain yield, while TZEEQI 418, TZEEQI 420, TZEEQI 470, TZEEQI 498, and TZEEQI 519 had significant and negative GCA effects.
3.4. Heterotic grouping of inbred lines across research conditions
A dendrogram constructed based on HGCAMT grouping across research conditions is presented in Figure 1. The 47 inbreds and four testers fell into four heterotic groups at a 40% level of similarity. In total, 22 inbred lines (TZEEQI 418, TZEEQI 470, TZEEQI 422, TZEEQI 442, TZEEQI 436, TZEEQI 496, TZEEQI 451, TZEEQI 483, TZEEQI 498, TZEEQI 419, TZEEQI 453, TZEEQI 479, TZEEQI 423, TZEEQI 446, TZEEQI 473, TZEEQI 507, TZEEQI 430, TZEEQI 431, TZEEQI 519, TZEEQI 456, TZEEQI 489, TZEEQI 458, TZEEQI 475, TZEEQI 456), including tester TZEEQI 134, made up the first group. The second group consisted of two lines TZEEQI 420, including tester TZEEQI 7, and the third group comprised 14 inbred lines (TZEEQI 425, TZEEQI 458, TZEEQI 516, TZEEQI 468, TZEEQI 533, TZEEQI 500, TZEEQI 480, TZEEQI 515, TZEEQI 434, TZEEQI 482, TZEEQI 521, TZEEQI 428, TZEEQI 474), including tester TZEEQI 321, while 10 inbred lines (TZEEQI 437, TZEEQI 502, TZEEQI 457, TZEEQI 467, TZEEQI 485, TZEEQI 464, TZEEQI 481, TZEEQI 490, TZEEQI 294 and TZEEQI 506), including tester TZEEQI 294 made up the fourth group (Table 8).
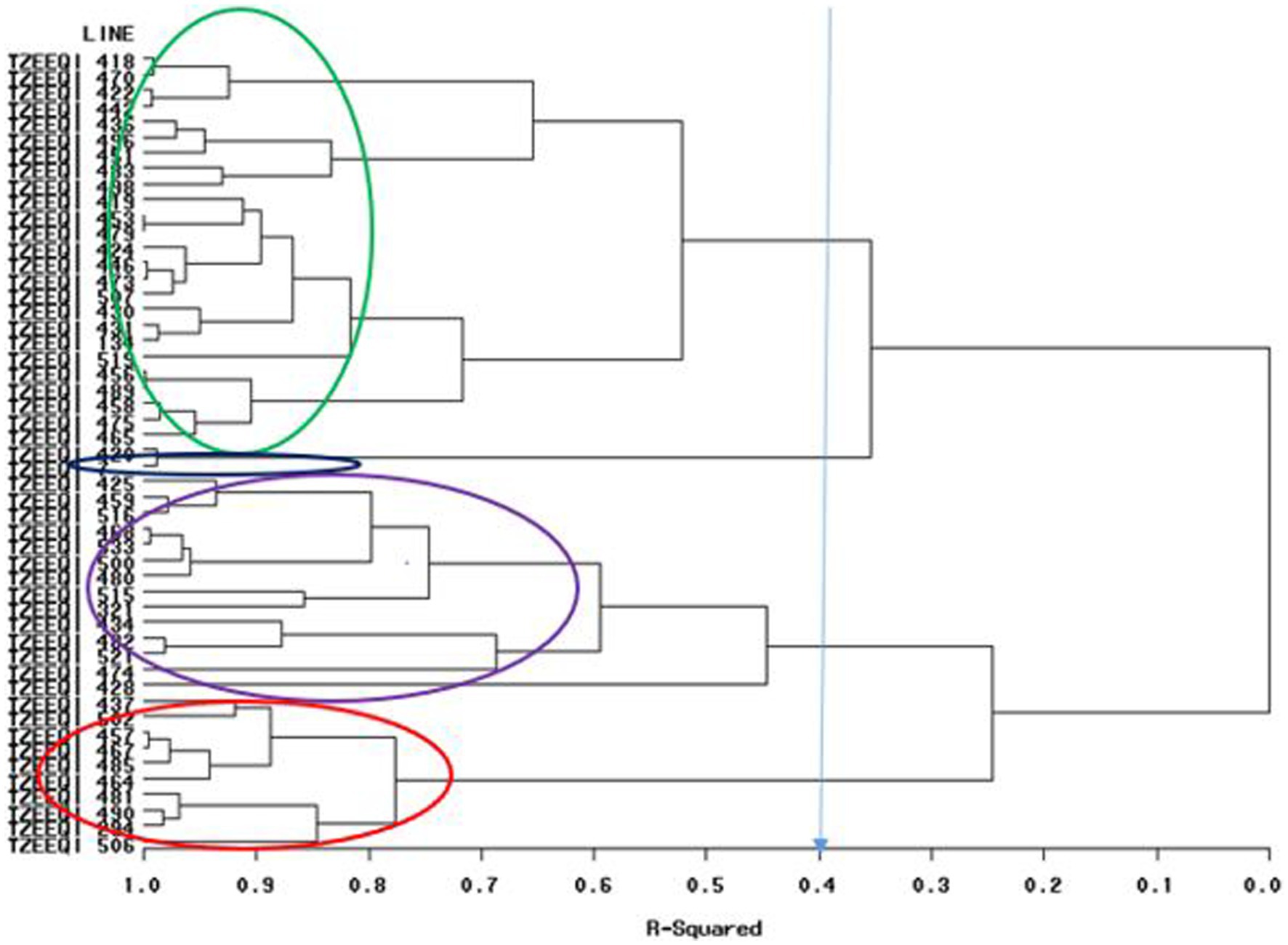
Figure 1. Heterotic grouping based on general combining ability of 47 extra-early quality protein maize across research environments in Mokwa 2019 and 2020 and Abuja, 2020.
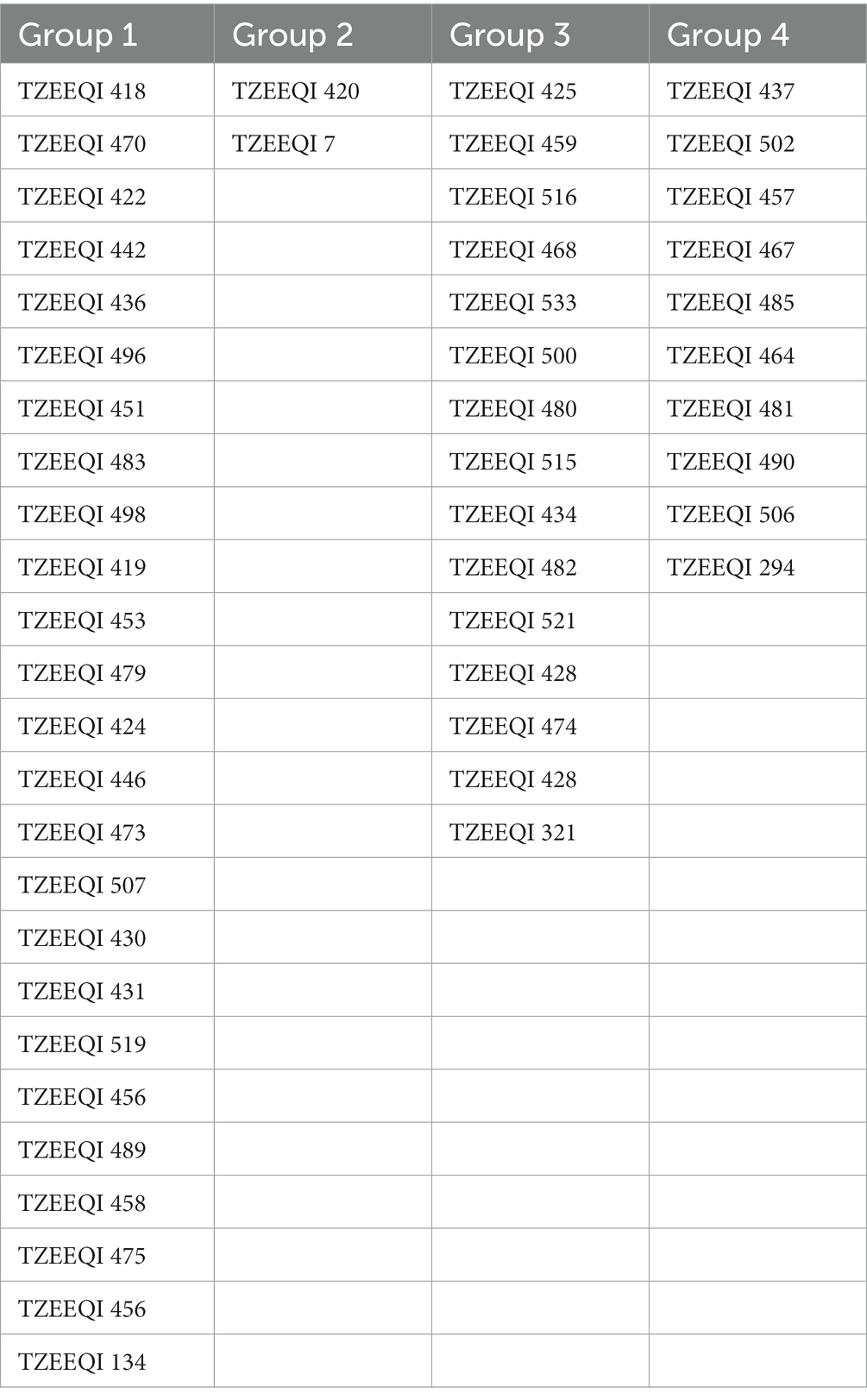
Table 8. Heterotic grouping of the 47 inbred lines evaluated across stress and non-stress research environments at Mokwa and Abuja.
3.5. Identification of inbred testers under Striga infestation
Inbred and single cross testers were identified using the criteria established by Pswarayi and Vivek (2008). TZEEQI 490 and TZEEQI 460 had positive and significant GCA effects for grain yield as well as for per se high grain yield under Striga-infested conditions and were classified into heterotic groups. Inbred lines TZEEQI 490 and TZEEQI 460 with these characteristics were identified as suitable testers under Striga-infested conditions.
3.6. Grain yield of 47 extra-early quality protein maize hybrids across Striga-infested, low N, and optimal conditions
Under Striga-infested conditions, grain yield ranged from 1796 kg ha−1 for the check TZEEQI 342 × TZEEQI 7 to 5,383 kg ha−1 for TZEEQI 468 × TZEEQI 321 with a mean yield of 4,764 kg ha−1. The best hybrid TZEEQI 468 × TZEEQI 321 outyielded the best check by 24% (Table 9). The grain yield of the QPM hybrids under low-nitrogen conditions ranged from 1,640 kg ha−1 for TZEEQI 507 × TZEEQI 7 to 4,788 kg ha−1 for TZEEQI 515 × TZEEQI 321 with a mean of 2,765 kg ha−1. The best hybrid outyielded the highest-yielding check by 11%. Under optimal conditions, the grain yield ranged from 2,805 kg ha−1 for TZEEQI 507 × TZEEQI 7 to 7,923 kg ha−1 for TZEEQI 506 × TZEEQI 321 with a mean of 5,454 kg ha−1.
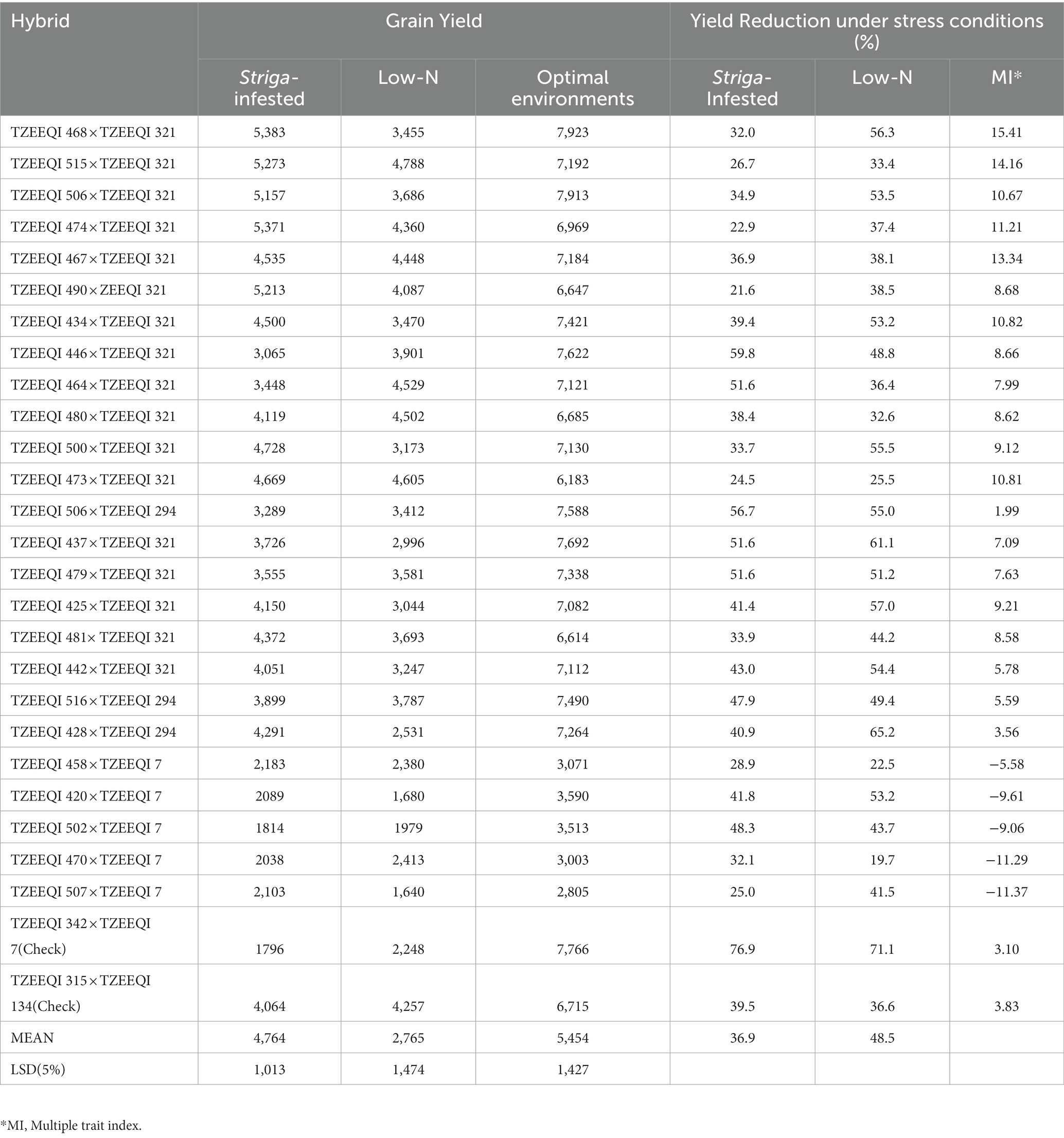
Table 9. Grain yield under Striga-infestation in Mokwa and Abuja 2020, low-N in Mokwa 2019 and 2020, and optimal conditions at Mokwa 2019, 2020 and Abuja 2020.
3.7. Grain yield stability of hybrids across research environments in Mokwa in 2019 and 2020 and Abuja in 2020
The 20 best hybrids and 10 worst hybrids plus two checks were subjected to GGE biplot analysis. In the polygon view, the vertex entries represented the highest-yielding hybrids in the sector (Figure 2). The hybrids’ distance from the biplot origin served as a measure of their performance differences and how they deviated from the average yields of other hybrids. Therefore, the vertex hybrids within each sector were more responsive to the conditions compared to those located within the polygon or near the biplot origin. The polygon view revealed that the research conditions fell into 2 of 8 sectors, resulting in the identification of 2 mega-environments. The first mega-environment comprised Mokwa infested 2020 (MKWSTR20), Abuja infested 2020 (ABSTR20), Mokwa Low N 2019 (MKLN19), and Mokwa Low N 2020 (MKLN20) while Abuja optimal 2020 (ABOPT20), Mokwa optimal 2020 (MKOPT20), and Mokwa optimal 2019 (MKOPT19) constituted the second mega-environment. Hybrids 1(TZEEQI 468 × TZEEQI 321) and 8 (TZEEQI 506 × TZEEQI 321) were vertex hybrids in this sector which consisted of MKLN19, MKLN20, MKSTR20, and ABSTR20 in Striga-infested and low soil N environments. Mega-environment 2 had no vertex hybrid, therefore no outstanding hybrids were identified in these mega-environments. Hybrids 22, 29, and 6 were also vertex hybrids but did not fall under any environment.
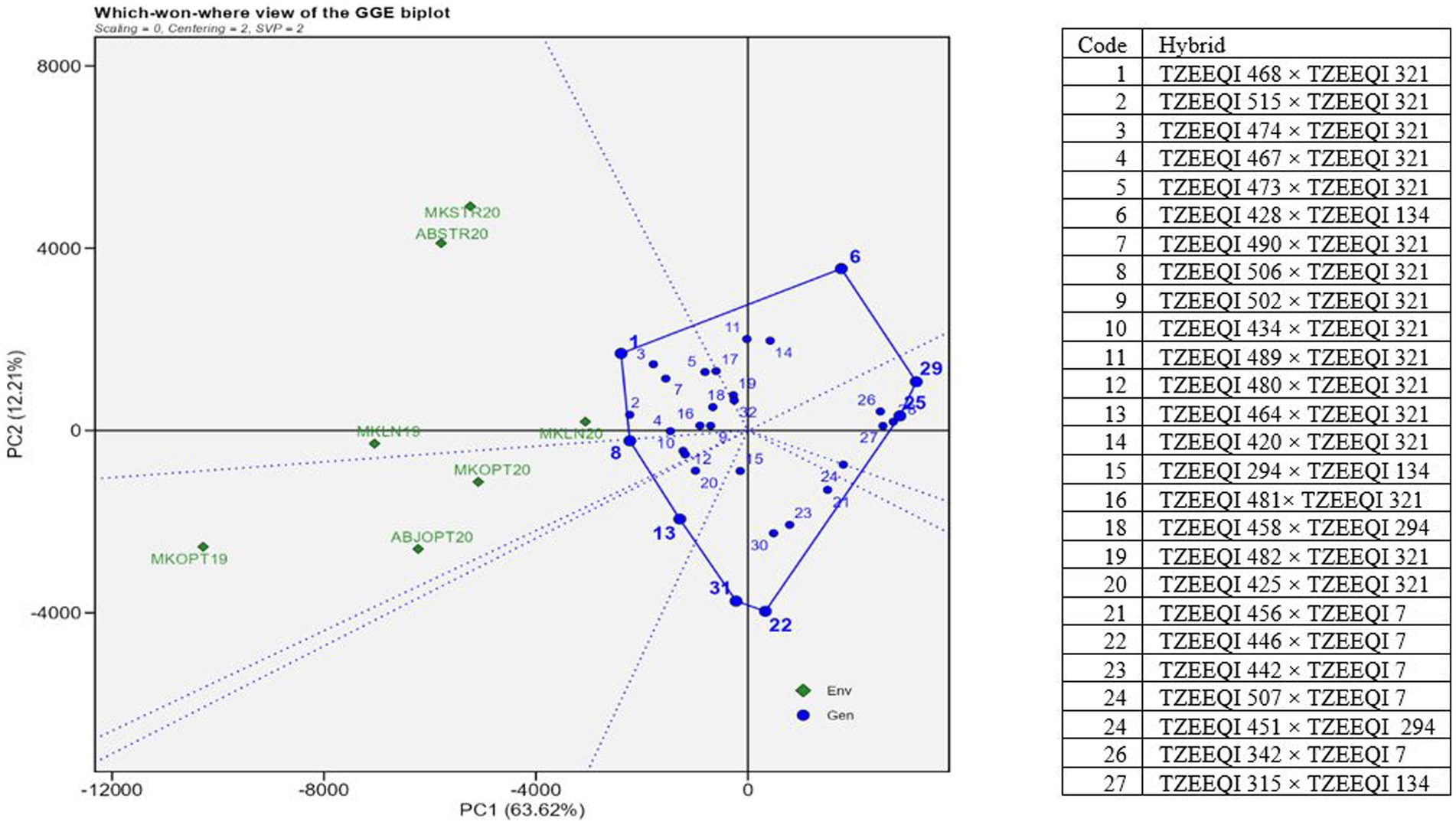
Figure 2. A “which won where” genotype plus genotype by environment interaction biplot of grain yield of selected 25 extra-early QPM hybrids plus two checks based on Striga infested and the low-nitrogen base index. The hybrids were evaluated across Striga-infested, low N and optimal environments in 2019 and 2021. MKLN2019, Mokwa under low-nitrogen environment, 2019; MKLN20, Mokwa under low-nitrogen environment, 2020; MKOPT19, Mokwa under optimal environment, 2019; MKOPT20, Mokwa optimal environment, 2020; MKSTR20, Mokwa Striga-infested environment, 2020; ABJSTR20, Abuja Striga-infested environment, 2020; ABJOPT20, Abuja optimal environment, 2020.
The “mean versus stability” (Figure 3) of the GGE biplot view showed the mean performance and stability of 32 extra-early white QPM hybrids. The average coordinates of the test environments are represented by a small circle in the biplot. The average environment axis (AEA), depicted as a single-arrowed line passing through the origin of the biplot and the average environment, indicates the direction of increasing performance of the genotypes. The AEA points towards the direction of increasing performance of the genotypes.
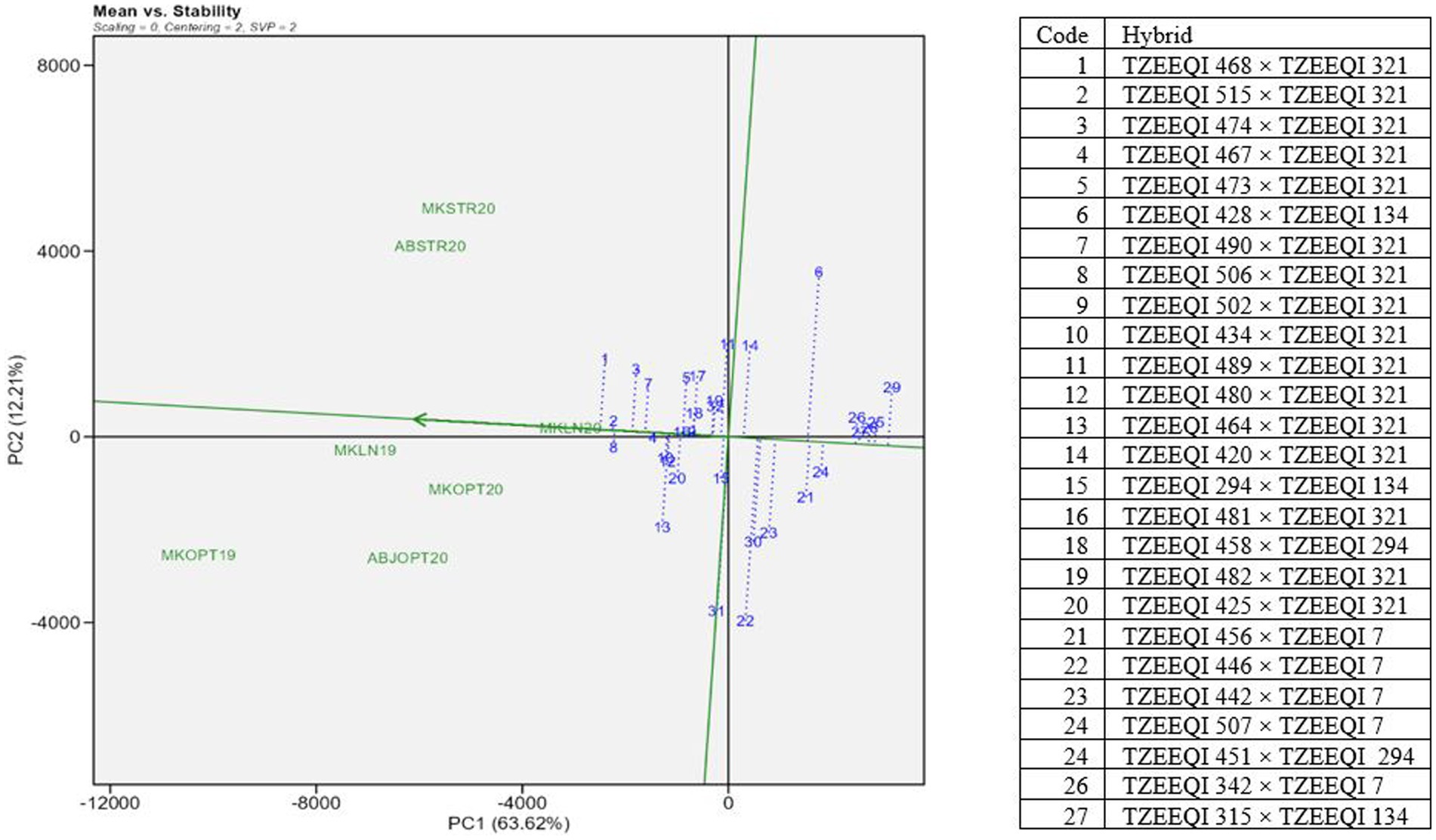
Figure 3. A “mean versus stability” view genotype plus genotype by environment interaction biplot of grain yield of selected 25 extra-early QPM hybrids plus two checks based on Striga infestation and the low-nitrogen base index evaluated across Striga-infested, low N and optimal environments in 2019 and 2021. MKLN2019, Mokwa under low-nitrogen environment, 2019; MKLN20, Mokwa under low-nitrogen environment, 2020; MKOPT19, Mokwa under optimal environment, 2019; MKOPT20, Mokwa optimal environment, 2020; MKSTR20, Mokwa Striga-infested environment, 2020; ABJSTR20, Abuja Striga-infested environment, 2020; ABJOPT20, Abuja optimal environment, 2020.
Hybrid TZEEQI 468 × TZEEQI 321 (1) was the highest-yielding hybrid in all environments. TZEEQI 515 × TZEEQI 321 (2), TZEEQI 506 × TZEEQI 321 (8), TZEEQI 474 × TZEEQI 321 (3), and TZEEQI 490 × TZEEQI 321 (7) constituted the five best-performing hybrids. The worst-performing hybrids were TZEEQI 507 × TZEEQI 7 (29), TZEEQI 470 × TZEEQI 294 (23), TZEEQI 442 × TZEEQI 7 (28), TZEEQI 446 × TZEEQI 7 (27), and TZEEQI 456 × TZEEQI 7 (26). The stability of the genotypes is assessed by their projection onto the AEC ordinate. The length of the genotype’s projection on the AEA axis indicates its contribution to the genotype by environment interaction (GEI) and its level of stability across different test environments. A longer projection signifies a higher contribution to GEI and lower stability across test environments (Yan et al., 2007). Thus, the most stable hybrids across the test environments were TZEEQI 502 × TZEEQI 321 (9) and TZEEQI 481 × TZEEQI 321 (16), followed by TZEEQI 458 × TZEEQI 294 (18) and TZEEQI 515 × TZEEQI 321 (2). Thus, TZEEQI 515 × TZEEQI 321 (2) is the most desirable in terms of grain yield and stability across test environments.
4. Discussion
The presence of significant variation in grain yield and other traits under conditions of Striga infestation, low nitrogen (N), and optimal growth indicated the existence of substantial genetic diversity among the hybrids. This finding suggested that significant advancements could be achieved through the selection of important agronomic traits under both stress and non-stress conditions.
The non-significant G × E interaction for grain yield and other traits across Striga-infested conditions implied that the measured traits responded to the two stresses the same way. This corroborates the results reported by Akinwale et al. (2013) who found no G × E interaction for grain yield and most traits observed under Striga infestation. This disagrees with the findings of Menkir et al. (2010), Abu et al. (2021), and Makinde et al. (2023), who observed significant G x E mean square for grain yield and other Striga resistance indicator traits for late, early, and extra-early maturing maize hybrids, respectively. Oyekale et al. (2020) attributed the non-significant G × E interactions in extra-early PVA inbred lines to the existence of limited genetic variation among the hybrids in the test locations. The significant G × E interaction observed for grain yield, days to silking, and the number of ears per plant across low soil N conditions implied that the responses of the hybrids varied with the individual environments. Similar findings were reported by Makumbi et al. (2011), Mebratu et al. (2019), and Dosho et al. (2022) who found significant G × E for grain yield and few measured traits under low-N soil conditions.
The non-significant general combining ability (GCA) and specific combining ability (SCA) mean square observed for grain yield across Striga-infested environments implied that there was no variation in the performance of the inbred parents when combined in hybrid combinations. A similar observation was made by Oyetunde et al. (2020) in their study on early maturing white maize, where non-significant GCA effects were found for both inbred lines and testers, but significant SCA effects were detected. However, significant GCA and SCA mean square was observed for the number of emerged Striga plants at 8 WAP and 10 WAP, indicating the involvement of both additive and non-additive gene actions in the expression of Striga infestation. This suggested that breeding strategies such as backcrossing, hybridization, and recurrent selection could be employed to enhance Striga tolerance/resistance.
Significant GCA and SCA mean square was observed for grain yield and ears per plant under low soil N conditions, indicating the influence of both additive and non-additive gene actions in the inheritance of these traits. This suggested that recurrent selection and hybridization can be employed for the genetic improvement of these traits. Similar findings were reported by Wegary et al. (2013) and Owusu et al. (2023) using the diallel mating method, where both additive and non-additive gene actions were found to be important for the inheritance of grain yield among QPM inbreds under low-N conditions. Meseka et al. (2006), Afolabi et al. (2019), Abu et al. (2021), and Makinde et al. (2023) also observed the significance of both additive and non-additive gene actions in the expression of grain yield under low-N conditions. However, the non-significant SCA variances for the stay-green characteristic and other measured traits in this study suggested that the inheritance of these traits was not influenced by non-additive gene action. This finding aligns with the results reported by Ribeiro et al. (2020) in elite maize inbred lines, where non-significant SCA effects were observed for the stay-green characteristic and other traits, except for grain yield and ears per plant. Mafouasson et al. (2017), Ifie et al. (2015), and Badu-Apraku et al. (2013) similarly observed non-significant SCA effects for the stay-green characteristic.
Under optimal conditions, the significant GCA observed for grain yield and other traits except anthesis-silking interval and ears per plant, suggested that additive genetic variances dominated the expression of these traits. This is consistent with the observation of Abu et al. (2021) and Makinde et al. (2023) for grain yield. Thus, these traits could be improved through recurrent selection schemes during the selection stages of inbred lines (Ertiro et al., 2017).
Significant GCATester × E and GCALine × E were observed for the number of emerged Striga plants at 8 WAP and 10 WAP, and anthesis-silking interval across Striga-infested environments, which indicated that the additive gene action was not consistently observed from environment to environment. The lack of significant GCALine × E and SCA × E for grain yield and most measured traits under Striga-infested and low-N conditions indicated that the GCA and SCA gene actions for the parental lines were consistent across these environments. The significant GCATester × E, GCALine × E and SCA × E observed for ears per plant across low soil N environments indicated that the performance of parental lines and hybrid combinations varied from environment to environment.
A larger proportion of GCA relative to SCA was observed for grain yield, Striga damage ratings at 10 WAP, ear aspect, days to silking, days to anthesis, plant height, and ear height, which is an indication that the inheritance of these traits was governed by additive genetic variances and that the genetic variation observed among the 196 hybrids was more influenced by the GCA component. This indicated that selecting for S. hermonthica resistance solely based on GCA predictions would be highly effective, especially in the early generations of a breeding program. These findings are supported by Badu-Apraku et al. (2011a,b), Badu-Apraku et al. (2013), Ifie et al. (2015), Konaté et al. (2017), Adu et al. (2022), and Adewale et al. (2023) for grain yield under Striga-infestation. Contrary to these findings, Annor et al. (2019) found that non-additive gene action played a more significant role in the expression of grain yield. Non-additive genetic variance played a more significant role in the expression of Striga damage ratings at 8 WAP, number of emerged Striga plants at 8 and 10 WAP, anthesis-silking interval, and ears per plant under Striga parasitism. Akanvou et al. (1997), Badu-Apraku et al. (2016a), and Annor et al. (2019) also found that non-additive gene action played a larger role in determining the inheritance of Striga damage syndrome rating and the number of emerged Striga plants. Contrarily, Yallou et al. (2009), Badu-Apraku and Oyekunle (2012), Badu-Apraku et al. (2021), and Adewale et al. (2023) found the inheritance of emerged Striga plants to be governed by additive gene action under Striga infestation.
The larger proportion of GCA to SCA observed for grain yield, stay-green characteristic, plant aspect, days to silking, and days to anthesis indicated that additive gene action was predominant in the expression of these traits and that GCA was the major component accounting for the genetic variations among the hybrids evaluated. These results are consistent with the observations of Owusu et al. (2023), Bhadmus et al. (2021), Ribeiro et al. (2020), Ertiro et al. (2017), Ifie et al. (2015), Badu-Apraku et al. (2013), and Tamilarasi et al. (2010), who also found additive genetic variance to be dominant for grain yield expression under low N conditions. In contrast, reports by Makinde et al. (2023), Mafouasson et al. (2017), Ndhlela (2012), Makumbi et al. (2011), Worku et al. (2008), Meseka et al. (2006), and Betran et al. (2003) affirmed the preponderance of non-additive gene action in the genetic control of grain yield under similar conditions. The differences in these findings and those of other authors could be attributed to the different genetic materials and environmental conditions used in the respective studies. However, non-additive gene action dominated the inheritance of ear aspect, anthesis-silking interval, ears per plant, plant, and ear heights. Similarly, the proportion of GCA was larger for grain yield and most measured traits across optimum conditions, an indication that additive gene action was more important in the inheritance of these traits. The studies conducted by Ifie et al. (2015) and Oyetunde et al. (2020) also supported these findings.
Heritability estimates play a crucial role in breeding programs as the effectiveness and success of such programs rely on the amount of genotypic variation that can be observed across different genetic populations, particularly when multi-environment trials (MET) are carried out. The moderate to high heritability estimates obtained for grain yield (68%), Striga damage syndrome rating at 10 WAP (56%), days to anthesis (79%), days to silking (75%), anthesis-silking interval (67%), and number of ears per plant (60%) indicated that selection for these traits will be stable and reliable across different stress environments (Menkir et al., 2012; Shayanowako et al., 2018; Badu-Apraku et al., 2020). However, the moderately low heritability estimates observed for Striga damage ratings at 8 WAP (37%), Striga emergence counts at 8WAP (24%) and 10 WAP (9%) suggested that the expression of the traits was influenced by the environment (Badu-Apraku, 2007). Moderate heritability estimates were recorded for grain yield (46%), plant aspect (43%), stay-green characteristic (47%), and ear aspect (55%) under low soil N. This suggested that the expression of these traits was influenced by research conditions.
The general combining ability effects were positive and significant for inbreds TZEEQI 428, TZEEQI 468, TZEEQI 490, and TZEEQI 506 for grain yield under Striga infestation indicating that these lines can function as good combiners and had favorable alleles for grain yield under Striga-infested environments. The inbreds TZEEQI 456, TZEEQI 468, TZEEQI 475, and TZEEQI 498 had significant and negative GCA effects for emerged Striga plants, a Striga tolerance indicator trait. This suggested that the lines could be used as parents for Striga tolerance breeding. TZEEQI 489 and TZEEQI 490 had negative and significant GCA effects for Striga damage ratings, an indicator trait for Striga resistance. These lines could be used as parents for Striga resistance breeding.
Significant and positive GCA effects were observed for grain yield of the inbreds TZEEQI 464, TZEEQI 480, and TZEEQI 490 under low soil N, suggesting their potential for improvement of grain under this growing condition. The stay-green characteristic is associated with delayed leaf senescence, and it is described as a productivity enhancing character in plants (Thomas and Howarth, 2000). The negative and significant GCA effects displayed by TZEEQI 430, TZEEQI 467, TZEEQI 480, and TZEEQI 490 for the stay-green characteristic is an indication that these inbreds possessed genes for delayed leaf senescence which could be passed on to their progenies when used as parents for low-N tolerance improvement.
Under optimal conditions, TZEEQI 425, TZEEQI 437, TZEEQI 464, TZEEQI 506, TZEEQI 515, and TZEEQI 516 had positive and significant GCA effects for grain yield and would transfer favorable alleles for grain yield to their progenies under this growing condition.
TZEEQI 490 had favorable alleles for grain yield and Striga damage syndrome under Striga infestation, and favorable alleles for grain yield and stay-green characteristic under low N conditions. This suggested that the inbred could be used for recurrent selection and generation of new QPM inbred lines with tolerance/resistance to both stresses.
The HGCAMT grouping method classified the 47 extra-early QPM inbred lines including the four testers into four heterotic groups. The identified heterotic groups would increase the prospect of developing QPM hybrids and synthetics that possess traits such as extra-early maturity, Striga resistance, and low-nitrogen tolerance. This will facilitate their commercialization in SSA.
The choice of potential lines as testers to classify and evaluate new inbred lines was based on the display of positive GCA effects, classification into heterotic groups, and high per se grain yield (Pswarayi and Vivek, 2008). The inbred lines TZEEQI 490 and TZEEQI 460 exhibited these desired attributes and were identified as suitable testers specifically for Striga-infested environments. This suggested that these inbred lines possessed good combining abilities, making them viable options either as parental lines for developing high-yielding hybrids or for grouping other inbred lines under Striga-infested conditions.
The most outstanding hybrids were revealed using the mean performances of the hybrids across individual research conditions and across research conditions. Hybrid TZEEQI 468 × TZEEQI 321 was outstanding across Striga-infested conditions, optimum conditions, and across all research conditions. The QPM hybrid TZEEQI 515 × TZEEQI 321 was outstanding across low soil-N conditions. These hybrids showed great potential as extra-early, Striga resistant, and low-N tolerant QPM hybrids. They should therefore be tested extensively for commercialization in SSA.
The GGE biplot is a statistical tool that has been employed to identify exceptional genotypes by evaluating their performance in specific and diverse environments. It aids in detecting environments that are most suitable for the genotypes and serve as representative test environments (Badu-Apraku et al., 2020). In this study, the seven environments were categorized into two mega-environments. The first mega-environment encompassed stress environments, which are Mokwa infested 2020 (MKWSTR20), Abuja infested 2020 (ABSTR20), Mokwa Low N 2019 (MKLN19), and Mokwa Low N 2020 (MKLN20), where hybrids TZEEQI 468 × TZEEQI 321 and TZEEQI 502 × TZEEQI 321 displayed exceptional performance. Among the tested hybrids, TZEEQI 515 × TZEEQI 321 had the highest performance and stability across the various test environments, including the optimal, Striga-infested, and low-N environments. This hybrid demonstrated great potential for production and should undergo a thorough evaluation in extensive on-farm trials for possible commercialization in SSA.
5. Conclusion
Additive genetic variance was dominant in the inheritance of grain yield and key agronomic traits under both stress and optimal environments. The inbred lines TZEEQI 480, TZEEQI 490, and TZEEQI 464 showed positive and significant GCA effects for grain yield under low soil N. Also, the inbred lines, TZEEQI 428, TZEEQI 468, TZEEQI 490, and TZEEQI 506 showed positive and significant GCA effects for grain yield under Striga infestation. These inbred lines could be used for the development of hybrids and synthetic varieties with tolerance to Striga and low N. TZEEQI 490 and TZEEQI 460 were identified as inbred testers. These lines could be used as parents in the production of high-yielding hybrids in Striga-infested environments. The QPM hybrid TZEEQI 515 × TZEEQI 321 showed high yield and stability across research environments. This hybrid should be tested extensively in multi-location trials and promoted for commercialization in SSA.
Data availability statement
The datasets presented in this study can be found in online repositories. The names of the repository/repositories and accession number(s) can be found at: The datasets used in the present study have been deposited at the IITA CKAN repository http://data.iita.org.
Author contributions
BB-A and GO: conceptualization, methodology, and project administration. BB-A, GO, and OA: validation. GO: formal analysis and investigation, data curation, writing – original draft preparation, and visualization. BB-A: resources and funding acquisition. BB-A, OA, and MA-V: writing – review and editing and supervision. All authors contributed to the article and approved the submitted version.
Funding
This research was conducted through funding support from the Bill and Melinda Gates Foundation (OPP1134248) under the Stress Tolerant Maize for Africa (STMA) and the Accelerated Genetic Gain in Maize and Wheat (AGG) Projects. We are also grateful for the funding support from the World Bank Center of Excellence in Agricultural Development and Sustainable Environment, Federal University of Agriculture, Abeokuta. The funding body played no role in the design of this study, collection, analysis, and interpretation of data as well as the writing of the manuscript.
Acknowledgments
The authors also acknowledge the IITA Maize Improvement Program (MIP) for providing technical support.
Conflict of interest
The authors declare that the research was conducted in the absence of any commercial or financial relationships that could be construed as a potential conflict of interest.
Publisher’s note
All claims expressed in this article are solely those of the authors and do not necessarily represent those of their affiliated organizations, or those of the publisher, the editors and the reviewers. Any product that may be evaluated in this article, or claim that may be made by its manufacturer, is not guaranteed or endorsed by the publisher.
References
Abdulmalik, R. O., Menkir, A., Meseka, S. K., Unachukwu, N., Ado, S. G., Olarewaju, J. D., et al. (2017). Genetic gains in grain yield of a maize population improved through marker assisted recurrent selection under stress and non-stress conditions in West Africa. Front. Plant Sci. 8:841. doi: 10.3389/fpls.2017.00841
Abu, P., Badu-Apraku, B., Ifie, B. E., Tongoona, P., Ribeiro, P. F., Obeng-Bio, E., et al. (2021). Genetics of extra-early-maturing yellow and orange quality protein maize inbreds and derived hybrids under low soil nitrogen and Striga infestation. Crop Sci. 61, 1052–1072. doi: 10.1002/csc2.20384
Adewale, S. A., Badu-Apraku, B., and Akinwale, R. O. (2023). Assessing the suitability of stress tolerant early-maturing maize (Zea mays) inbred lines for hybrid development using combining ability effects and DArTseq markers. Plant Breed. 142, 223–237. doi: 10.1111/pbr.13077
Adu, G. B., Badu-Apraku, B., Akromah, R., and Awuku, F. J. (2022). Combining abilities and heterotic patterns among early maturing maize inbred lines under optimal and Striga-infested environments. Genes 13:2289. doi: 10.3390/genes13122289
Afolabi, M. S., Salami, A. E., and Agbowuro, G. O. (2019). Genetic studies of grain yield and other agronomic traits of low-n maize (Zea mays L.) using a diallel cross under nitrogen fertilizer levels. IJPBCS 6, 569–574.
African Development Bank (2006). Abuja declaration. Abuja declaration on fertilizer for the African green revolution. Available at: https://www.afdb.org/en/topics-and-sectors/initiativespartnerships/african-fertilizer-financingmechanism/abujadeclaration/ (accessed April 5, 2018).
Akanvou, L., Doku, E. V., and Kling, J. (1997). Estimates of genetic variances and interrelationships of traits associated with Striga resistance in maize. Afr. Crop. Sci. J. 5, 1–8. doi: 10.4314/acsj.v5i1.27864
Akaogu, I. C., Badu-Apraku, B., Tongoona, P., Cellabos, H., Gracen, V., Offei, S. K., et al. (2019). Inheritance of Striga hermonthica adaptive traits in an early-maturing white maize inbred line containing resistance genes from Zea diploperennis. Plant Breed. 138, 546–552. doi: 10.1111/pbr.12707
Akinwale, R. O., Badu-Apraku, B., and Fakorede, M. A. B. (2013). Evaluation of Striga-resistant early maize hybrids and test locations under Striga-infested and Striga-free environments. Afr. Crop. Sci. J. 21, 1–19.
Alves, F. C., Galli, G., Matias, F. I., Vidotti, M. S., Morosini, J. S., and Fritsche-Neto, R. (2021). Impact of the complexity of genotype by environment and dominance modeling on the predictive accuracy of maize hybrids in multi-environment prediction models. Euphytica 217:37. doi: 10.1007/s10681-021-02779-y
Annor, B., Badu-Apraku, B., Nyadanu, D., Akromah, R., and Fakorede, M. A. (2019). Testcross performance and combining ability of early maturing maize inbreds under multiple-stress environments. Sci. Rep. 9, 13809–13811. doi: 10.1038/s41598-019-50345-3
Atera, E. A., Itoh, K., Azuma, T., and Ishii, T. (2012). Farmers' perspectives on the biotic constraint of Striga hermonthica and its control in western Kenya. Weed Biol. Manag. 12, 53–62. doi: 10.1111/j.1445-6664.2012.00435.x
Badu-Apraku, B. (2007). Genetic variances and correlations in an early tropical white maize population after three cycles of recurrent selection for Striga resistance. Maydica 52:205.
Badu-Apraku, B., Annor, B., Oyekunle, M., Akinwale, R. O., Fakorede, M. A. B., Talabi, A. O., et al. (2015). Grouping of early maturing quality protein maize inbreds based on SNP markers and combining ability under multiple environments. Field Crop Res. 183, 169–183. doi: 10.1016/j.fcr.2015.07.015
Badu-Apraku, B., and Fakorede, M. A. B. (2017). Advances in genetic enhancement of early and extra-early maize for sub-Saharan Africa. Springer International Publishing AG New York
Badu-Apraku, B., Fakorede, M. A. B., Oyekunle, M., and Akinwale, R. O. (2011b). Selection of extra-early maize inbreds under low N and drought at flowering and grain-filling for hybrid production. Maydica 56, 29–41.
Badu-Apraku, B., Fakorede, M. A. B., Talabi, A. O., Oyekunle, M., Aderounmu, M., Lum, A. F., et al. (2020). Genetic studies of extra-early provitamin-a maize inbred lines and their hybrids in multiple environments. Crop Sci. 60, 1325–1345. doi: 10.1002/csc2.20071
Badu-Apraku, B., Fakorede, M. A. B., Talabi, A. O., Oyekunle, M., Akaogu, I. C., Akinwale, R. O., et al. (2016a). Gene action and heterotic groups of early white quality protein maize inbreds under multiple stress environments. Crop Sci. 56, 183–199. doi: 10.2135/cropsci2015.05.0276
Badu-Apraku, B., Obisesan, O., Olumide, O. B., and Toyinbo, J. (2021). Gene action, heterotic patterns, and inter-trait relationships of early maturing pro-vitamin a maize inbred lines and performance of testcrosses under contrasting environments. Agronomy 11:1371. doi: 10.3390/agronomy11071371
Badu-Apraku, B., and Oyekunle, M. (2012). Genetic analysis of grain yield and other traits of extra-early yellow maize inbreds and hybrid performance under contrasting environments. Field Crop Res. 129, 99–110. doi: 10.1016/j.fcr.2012.01.018
Badu-Apraku, B., Oyekunle, M., Akinwale, R. O., and Lum, A. F. (2011a). Combining ability of early-maturing white maize inbreds under stress and nonstress environments. Agron. J. 103, 544–557. doi: 10.2134/agronj2010.0345
Badu-Apraku, B., Oyekunle, M., Fakorede, M. A. B., Vroh, I., Akinwale, R. O., and Aderounmu, M. (2013). Combining ability, heterotic patterns, and genetic diversity of extra-early yellow inbreds under contrasting environments. Euphytica 192, 413–433. doi: 10.1007/s10681-013-0876-4
Badu-Apraku, B., Talabi, A. O., Ifie, B. E., Chabi, Y. C., Obeng-Antwi, K., Haruna, A., et al. (2018). Gains in grain yield of extra-early maize during three breeding periods under drought and Rainfed conditions. Crop Sci. 58, 2399–2412. doi: 10.2135/cropsci2018.03.0168
Badu-Apraku, B., Yallou, C. G., Alidu, H., Talabi, A. O., Akaogu, I. C., Annor, B., et al. (2016b). Genetic improvement of extra-early maize cultivars for grain yield and Striga resistance during three breeding eras. Crop Sci. 56, 2564–2578. doi: 10.2135/cropsci2016.02.0089
Baker, R. J. (1978). Issues in diallel analysis. Crop Sci. 18, 533–536. doi: 10.2135/cropsci1978.0011183X001800040001x
Bebawi, F. F., Eplec, R. E., and Harris, C. E. and Norris, R. S. (1984). Longevity of witchweed (Striga 12 asiatica) seed. Weed Science 32, 494–497.
Below, F. E. (1997). Growth and productivity of maize under nitrogen stress. In Proceedings of a symposium, El Batan, Mex. (Mexico), 25–29 CIMMYT.
Betran, F. J., Beck, D., Bänziger, M., and Edmeades, G. O. (2003). Genetic analysis of inbred and hybrid grain yield under stress and non-stress environments in tropical maize. Crop Sci. 43, 807–817. doi: 10.2135/cropsci2003.8070
Bhadmus, O. A., Badu-Apraku, B., Adeyemo, O. A., and Ogunkanmi, A. L. (2021). Genetic analysis of early white quality protein maize Inbreds and derived hybrids under low-nitrogen and combined drought and heat stress environments. Plan. Theory 10:2596. doi: 10.3390/plants10122596
Bremner, J. M., and Mulvaney, C. S. (1982). Nitrogen-total. In: a.L. page, R.H. miller, and D.R. Keeney, editors, methods of soil analysis. Part 2. Agronomy 9, 595–624. doi: 10.2134/agronmonogr9.2.2ed.c31
Cassman, K. G., Dobermann, A., and Walters, D. T. (2002). Agroecosystems, nitrogen-use efficiency, and nitrogen management. AMBIO J. Hum. Environ. 31, 132–140. doi: 10.1579/0044-7447-31.2.132
CIMMYT and IITA (2010). Maize alliance for improving food security and the livelihoods of the resource poor in the developing world draft proposal submitted by CIMMYT and IITA to the CGIAR consortium board, El Batan, Mexico, 9. Available at: http://hdl.handle.net/10883/670
Dosho, B. M., Ifie, B. E., Asante, I. K., Danquah, E. Y., and Zeleke, H. (2022). Genotype-by-environment interaction and yield stability for grain yield of quality protein maize hybrids under low and optimum soil nitrogen environments. J. Crop. Sci. Biotechnol. 25, 437–450. doi: 10.1007/s12892-022-00143-7
Edmeades, G. O., Bänziger, M., Mickelson, H. R., and Peña-Valdivia, C. B. (1996). Drought-and low N-tolerant maize. In Proceedings of a symposium (25–29).
Ejeta, G. (2007). Breeding for Striga resistance in sorghum: exploitation of an intricate host–parasite biology. Crop Sci. 47:S-216. doi: 10.2135/cropsci2007.04.0011IPBS
Ekpa, O., Palacios-Rojas, N., Kruseman, G., Fogliano, V., and Linnemann, A. R. (2018). Sub-Saharan African maize-based foods: technological perspectives to increase the food and nutrition security impacts of maize breeding programmes. Glob. Food Sec. 17, 48–56. doi: 10.1016/j.gfs.2018.03.007
Ertiro, B. T., Beyene, Y., Das, B., Mugo, S., Olsen, M., Oikeh, S., et al. (2017). Combining ability and testcross performance of drought-tolerant maize inbred lines under stress and non-stress environments in Kenya. Plant Breed. 136, 197–205. doi: 10.1111/pbr.12464
FAO. Food and agriculture Organization of the United Nations. FAOSTAT database. Rome: FAO. (2021). Available at: http://www.fao.org/faostat/en/#home (accessed February 15, 2021).
FAOSTAT, (2017) Food and agricultural Organization of the United Nations (FAO), FAO statistical database, 2016. Available at: http://faostat3.fao.org
Huang, S., Adams, W. R., Zhou, Q., Malloy, K. P., Voyles, D. A., Anthony, J., et al. (2004). Improving nutritional quality of maize proteins by expressing sense and antisense zein genes. J. Agric. Food Chem. 52, 1958–64. doi: 10.1021/jf0342223
Ifie, B. E., Badu-Apraku, B., Gracen, V., and Danquah, E. Y. (2015). Genetic analysis of grain yield of IITA and CIMMYT early maturing maize inbreds under Striga-infested and low soil nitrogen environments. Crop Sci. 55, 610–623. doi: 10.2135/cropsci2014.07.0470
Kamara, M. M., El-Degwy, I. S., and Koyama, H. (2014). Estimation combining ability of some maize inbred lines using line x tester mating design under two nitrogen levels. Aust. J. Crop. Sci. 8, 1336–1342.
Khan, Z. R., Midega, C. A., Pittchar, J. O., Murage, A. W., Birkett, M. A., Bruce, T. J., et al. (2014). Achieving food security for one million sub-Saharan African poor through push–pull innovation by 2020. Philos. Trans. R. Soc. Lond., B, Biol. Sci. 369:20120284. doi: 10.1098/rstb.2012.0284
Kim, S. K. (1991). Breeding maize for Striga tolerance and the development of a field infestation technique. Proceedings of the International Workshop. IITA, 22-24 August 1988. Ibadan, Nigeria: IITA
Kim, S. K., and Winslow, M. D. (1991). “Progress in breeding maize for Striga-tolerance/resistance at IITA 1991” in Proceeding of 5th international symposium. Parasitic weeds. ed. J. K. Ransom (Nairobi: IITA), 494–499.
Konaté, L., Baffour, B. A., and Traoré, D. (2017). Combining ability and heterotic grouping of early maturing provitamin a maize inbreds across Striga-infested and optimal environments. J. Agric. Environ. Int. Dev. 111, 157–173. doi: 10.12895/jaeid.20171.572
Koné, A. W., Tondoh, J. E., Angui, P. K., Bernhard-Reversat, F., Loranger-Merciris, G., Brunet, D., et al. (2008). Is soil quality improvement by legume cover crops a function of the initial soil chemical characteristics? Nutr. Cycl. Agroecosyst. 82, 89–105. doi: 10.1007/s10705-008-9172-4
Kountche, B. A., Al-Babili, S., and Haussmann, B. I. (2016). “Striga: a persistent problem on millets” in Biotic stress resistance in Millets. eds. I. K. Das and P. G. Padmaja (Cambridge, MA: Academic Press), 173–203.
Krivanek, A. F., De Groote, H., Gunaratna, N. S., Diallo, A. O., and Friesen, D. K. (2007). Breeding and disseminating quality protein maize (QPM) for Africa. Academic Press. Cambridge, MA
Lagoke, S. T. O., Parkinson, V., and Agunbiade, R. M. (1991). “Parasitic weed control methods in Africa” in Proceedings in international workshop (IITA, ICRISAT and IDRC): Combating Striga in Africa, Ibadan, Nigeria 22–24 august 1998. ed. S. K. Kim (Ibadan: IITA), 3–14.
Mafouasson, A. H. N., Kenga, R., Gracen, V., Yeboah, A. M., Mahamane, L. N., Tandzi, N. L., et al. (2017). Combining ability and gene action of tropical maize (Zea mays L.) inbred lines under low and high nitrogen conditions. J. Agric. Sci. 9, 222–235. doi: 10.5539/jas.v9n4p222
Makinde, S. A., Badu-Apraku, B., Ariyo, O. J., and Porbeni, J. B. (2023). Combining ability of extra-early maturing pro-vitamin a maize (Zea mays L.) inbred lines and performance of derived hybrids under Striga hermonthica infestation and low soil nitrogen. PLoS One 18:e0280814. doi: 10.1371/journal.pone.0280814
Makumbi, D., Betran, J. F., Banziger, M., and Ribaut, J. M. (2011). Combining ability, heterosis and genetic diversity in tropical maize (Zea mays L.) under stress and non-stress conditions. Euphytica 180, 143–162. doi: 10.1007/s10681-010-0334-5
Mandumbu, R., Mutengwa, C., Mabasa, S., and Mwenje, E. (2019). Challenges to the exploitation of host plant resistance for Striga management in cereals and legumes by farmers in sub-Saharan Africa: a review. Acta. Agric. Scand. B Soil Plant. Sci. 69, 82–88. doi: 10.1080/09064710.2018.1494302
Mebratu, A., Wegary, D., Mohammed, W., Teklewold, A., and Tarekegne, A. (2019). Genotype× environment interaction of quality protein maize hybrids under contrasting management conditions in eastern and southern Africa. Crop Sci. 59, 1576–1589. doi: 10.2135/cropsci2018.12.0722
Menkir, A., Adetimirin, V. O., Yallou, C. G., and Gedil, M. (2010). Relationship of genetic diversity of inbred lines with different reactions to Striga hermonthica (Del.) Benth and the performance of their crosses. Crop Sci. 50, 602–611. doi: 10.2135/cropsci2009.05.0247
Menkir, A., Makumbi, D., and Franco, J. (2012). Assessment of reaction patterns of hybrids to Striga hermonthica (Del.) Benth. Under artificial infestation in Kenya and Nigeria. Crop Sci. 52, 2528–2537. doi: 10.2135/cropsci2012.05.0307
Meseka, S. K., Menkir, A., Ibrahim, A. E. S., and Ajala, S. O. (2006). Genetic analysis of performance of maize inbred lines selected for tolerance to drought under low nitrogen. Maydica 51:487. Available at: https://hdl.handle.net/20.500.12478/3074
Miah, M., Ahmed, S., and Uddin, M. (2016). Assessment of yield stability of maize inbred lines in multi-environment trials. Bangladesh J. Sci. Ind. 51:61. doi: 10.3329/bjsir.v51i1.27064
Mosisa, W., Bänziger, M., Erley, G. S. A. M., Friesen, D., Diallo, A. O., and Horst, W. J. (2007). Nitrogen uptake and utilization in contrasting nitrogen efficient tropical maize hybrids. Crop Sci. 47, 519–528. doi: 10.2135/cropsci2005.05.0070
Mulungu, K., and Ng’ombe, J. N. (2019). “Climate change impacts on sustainable maize production in sub-Saharan Africa: a review” in Maize - Production and Use. ed. A. Hossain, vol. 47-58 (London: IntechOpen)
Ndhlela, T. (2012). Improvement strategies for yield potential, disease resistance and drought tolerance of Zimbabwean maize inbred lines (dissertation). University of the Free State, South Africa, 295.
Obeng-Bio, E., Badu-Apraku, B., Ifie, B. E., Danquah, A., Blay, E. T., and Annor, B. (2019). Genetic analysis of grain yield and agronomic traits of early provitamin a quality protein maize inbred lines in contrasting environments. J. Agric. Sci. 157, 413–433. doi: 10.1017/S0021859619000753
Odhiambo, G. D., and Ransom, J. K. (1994). “Long term strategies for Striga control,” in Maize research for stress environments. proc. 4th eastern and Southern Africa Regional Maize Conference. ed. D. C. Jewell (CIMMYT, Mexico: Harare, Zimbabwe), 263–26.
Odhiambo, G., and Woomer, P. L. (2005). “Striga emergence and seed bank dynamics under different maize management practices in Western Kenya” in Proceedings of the African crop science conference. eds. J. S. Tenywa, E. Adipala, P. Nampala, G. Tusiime, P. Okori, and W. Kyamuhangire, vol. 7 (Kampala: African Crop Science Society), 473–477.
Oswald, A., and Ransom, J. K. (2001). Striga control and improved farm productivity using crop rotation. Crop Prot. 20, 113–120. doi: 10.1016/S0261-2194(00)00063-6
Owusu, G. A., Abe, A., and Ribeiro, P. F. (2023). Genetic analysis and heterotic grouping of quality protein maize (Zea mays L.) inbred lines and derived hybrids under conditions of low soil nitrogen and drought stress. Euphytica 219:29. doi: 10.1007/s10681-023-03159-4
Oyekale, S. A., Badu-Apraku, B., and Adetimirin, V. O. (2020). Combining ability of extra-early biofortified maize inbreds under Striga infestation and low soil nitrogen. Crop Sci. 60, 1925–1945. doi: 10.1002/csc2.20195
Oyetunde, O. A., Badu-Apraku, B., Ariyo, O. J., and Alake, C. O. (2020). Efficiencies of heterotic grouping methods for classifying early maturing maize inbred lines. Agronomy 10:1198. doi: 10.1016/j.fcr.2013.10.015
Parker, C. (2012). Parasitic weeds: a world challenge. Weed Sci. 60, 269–276. doi: 10.1614/WS-D-11-00068.1
Pswarayi, A., and Vivek, B. S. (2008). Combining ability amongst CIMMYT’s early maturing maize (Zea mays L.) germplasm under stress and non-stress conditions and identification of testers. Euphytica 162, 353–362. doi: 10.1007/s10681-007-9525-0
Ribeiro, P. F., Badu-Apraku, B., Gracen, V., Danquah, E. Y., Afriyie-Debrah, C., Obeng-Dankwa, K., et al. (2020). Combining ability and testcross performance of low N tolerant intermediate maize inbred lines under low soil nitrogen and optimal environments. J. Agric. Sci. 158, 351–370. doi: 10.1017/S0021859620000702
Shaxson, L., and Riches, C. (1998). Where once there was grain to burn: a farming system in crisis in eastern Malawi. Outlook Agric. 27, 101–105. doi: 10.1177/003072709802700
Shayanowako, A. I., Shimelis, H., Laing, M. D., and Mwadzingeni, L. (2018). Variance components and heritability of traits related to Striga asiatica resistance and compatibility to fusarium oxysporum F. Sp. Striga in maize. Maydica 63:8. Available at: https://journals-crea.4science.it/ind
Shiferaw, B., Prasanna, B. M., Hellin, J., and Bänziger, M. (2011). Crops that feed the world 6. Past successes and future challenges to the role played by maize in global food security. Food Security 3, 307–327. doi: 10.1007/s12571-011-0140-5
Singh, R. K., and Chaudhary, B. D. (1977). Biometrical methods in quantitative genetic analysis. Kalyani Publishers. Ernakulam
Sprague, G. F., and Tatum, L. A. (1942). General vs combining ability in single crosses of corn. Agronomy 34, 923–932. doi: 10.2134/agronj1942.00021962003400100008x
Tamilarasi, P. M., Arumugachamy, S., Anantha, M. S., and Utharasu, S. (2010). Identification of nitrogen (N) stress tolerant maize (Zea mays L.) inbred lines for low nitrogen input condition. Indian. J. Agric. Res. 44, 53–57.
Thomas, H., and Howarth, C. J. (2000). Five ways to stay green. J. Exp. Bot. 51, 329–337. doi: 10.1093/jexbot/51.suppl_1.329
Thorne, P. J. (2002). Maize as food, feed, and fertilizer in intensifying crop-livestock Systems in East and Southern Africa: An ex-ante impact assessment of technology interventions to improve 840 smallholder welfare. ILRI (aka ILCA and ILRAD). Nairobi, Kenya
Uribelarrea, M., Crafts-Brandner, S. J., and Below, F. E. (2009). Physiological N response of field-grown maize hybrids (Zea mays L.) with divergent yield potential and grain protein concentration. Plant Soil 316, 151–160. doi: 10.1007/s11104-008-9767-1
VIB. Maize in Africa. VIB international plant biotechnology outreach. Fact series. Ghent: VIB (2017). Available at: http://www.vib.be/en/aboutvib/Documents/VIB_MaizeInAfrica_EN_2017.pdf (accessed September 17, 2020).
Wegary, D., Vivek, B., and Labuschagne, M. (2013). Association of parental genetic distance with heterosis and specific combining ability in quality protein maize. Euphytica 191, 205–216. doi: 10.1007/s10681-012-0757-2
Worku, M., Banziger, M., Friesen, D., Schulte, G., Horst, W. J., and Vivek, B. S. (2008). Relative importance of general combining ability and specific combining ability among tropical maize (Zea mays L.) inbreds under contrasting nitrogen environments. Maydica 53, 279–288.
Wu, Y. R., Holding, D. R., and Messing, J. (2010). γ-Zein are essential for endosperm modification in quality protein maize. Proc. Natl. Acad. Sci. U. S. A. 107, 12810–12815. doi: 10.1073/pnas.1004721107
Yallou, C. G., Menkir, A., and Adetimirin, V. O. (2009). Combining ability of maize inbred lines containing genes from Zea diploperennis for resistance to Striga hermonthica (Del.) Benth. Plant Breed. 128, 143–148. doi: 10.1111/j.1439-0523.2008.01583
Yan, W., Kang, M. S., Ma, B., Woods, S., and Cornelius, P. L. (2007). GGE biplot vs. AMMI analysis of genotype-by-environment data. Crop Sci. 47, 643–653. doi: 10.2135/cropsci2006.06.0374
Keywords: maize, QPM inbred lines, Striga , low-N, genetics, stability
Citation: Okunlola G, Badu-Apraku B, Ariyo O and Ayo-Vaughan M (2023) The combining ability of extra-early maturing quality protein maize (Zea mays) inbred lines and the performance of their hybrids in Striga-infested and low-nitrogen environments. Front. Sustain. Food Syst. 7:1238874. doi: 10.3389/fsufs.2023.1238874
Edited by:
Matteo Balderacchi, Independent Researcher, Piacenza, ItalyReviewed by:
Chikkappa G. Karjagi, Indian Council of Agricultural Research (ICAR), IndiaJiban Shrestha, Nepal Agricultural Research Council, Nepal
Copyright © 2023 Okunlola, Badu-Apraku, Ariyo and Ayo-Vaughan. This is an open-access article distributed under the terms of the Creative Commons Attribution License (CC BY). The use, distribution or reproduction in other forums is permitted, provided the original author(s) and the copyright owner(s) are credited and that the original publication in this journal is cited, in accordance with accepted academic practice. No use, distribution or reproduction is permitted which does not comply with these terms.
*Correspondence: Baffour Badu-Apraku, b.badu-apraku@cgiar.org