Marketing performance and factors influencing farmers choice for agricultural output marketing channels: the case of garden pea (Pisum sativum) in India
- 1Department of Business Management, Dr. Yashwant Singh Parmar University of Horticulture and Forestry, Solan, India
- 2Department of Agribusiness Management, Acharya Narendra Deva University of Agriculture and Technology, Ayodhya, India
- 3Department of Humanities and Social Sciences, National Institute of Technology, Hamirpur, Himachal Pradesh, India
- 4Department of Silviculture and Agroforestry, Dr. Yashwant Singh Parmar University of Horticulture and Forestry, Solan, Himachal Pradesh, India
- 5Department of Agricultural Economics, Acharya Narendra Deva University of Agriculture and Technology, Kumarganj, India
- 6Department of Geography, Miranda House, University of Delhi, New Delhi, India
- 7Department of Agricultural Economics and Extension, School of Agriculture, Lovely Professional University, Phagwara, India
- 8Department of Mathematics, College of Science, Jazan University, Jizan, Saudi Arabia
- 9Department of Plant Pathology, Chaudhary Charan Singh Haryana Agricultural University, Hisar, India
- 10College of Agriculture, Rewa, (M.P.), Jawaharlal Nehru Krishi Vishwa Vidyalaya, Jabalpur, India
Agriculture is the backbone of Indian economy and farmers play significant role in sustaining the sector. However, farmers face several challenges such as poor market access and limited access to market information which adversely affect their marketing performance. Therefore, this study aimed to examine the marketing performance and factors influencing farmers choice for agricultural output marketing channels in garden pea in the Indian state of Himachal Pradesh. The study utilized primary data collected through structured questionnaires administered via the interview method to a sample size of 400 farmers selected through a multistage stratified random sampling technique. The data collected were analyzed using descriptive statistics and acharya approach to study the marketing performance. Further, a multinomial logistic regression model was used to determine the factors that influence farmers marketing choice of marketing channels. Additionally, the constraints experienced by farmers during marketing were investigated using garret ranking. The study revealed that farmers who sold their pea directly to consumer had higher marketing performance than those who sold through market intermediaries such as local traders, commission agents and wholesalers. Further, the results indicated that farm income, farm experience, distance to the market and market information were significant determinants of farmers choice for marketing channels. Whereas, lack of market consultancy, high commission chargers and poor transportation were the major constraints faced by farmers during marketing of pea. The findings of this study highlight the need for policymakers to develop and implement interventions that enhance farmers marketing skill, facilitate access to markets, improve negotiation power which can lead to higher incomes for farmers, improve their livelihoods, better quality produce for consumers and a more efficient and sustainable food system.
1 Introduction
The garden pea (Pisum sativum) is one of the oldest cultivated leguminous vegetables in the world, commonly known as pea or green pea. Pea has a significant global value as a food and nutritional source for millions of people. The pea is in a good position to meet the rising demand for high-protein foods around the world and to serve as a leguminous break-up crop in a variety of farming systems. It is one of the extensively cultivated vegetable crops grown for commercial purposes (Swaminathan, 2002; Singla et al., 2006; Sidhu et al., 2011). The annual global production of pea is around 21.77 million tons. China is a top producer of green peas with total production 12.2 million tons, followed by India with 4.8 million tons (FAO, 2022). In India, major pea-growing states include Uttar Pradesh, Punjab, Madhya Pradesh and Himachal Pradesh (MOAFW, 2022). Along with other agricultural produce, peas are essential for farmers and market participants for employment opportunities, income generation and food security. Recognizing the significance of peas in human life and diet, it is crucial to focus on increasing production levels while strengthening the marketing system (Maspaitella et al., 2018). The vision of commercialized agriculture can only be realized when farmers are encouraged to engage in agribusiness and participate actively in the market (Panda and Sreekumar, 2012; Thakur et al., 2022a,b). Marketing exerts a stimulating influence on the production and distribution of agricultural output. Thus, agricultural marketing plays a vital role in promoting growth and development of the agriculture sector (Chand and Singh, 2016; Dawit, 2016; Olutumise, 2022). Therefore, enhancing market participation will facilitate agricultural modernization and encourage increased investment in agriculture (Lapar et al., 2003; Akrong et al., 2021). Apart from this, market participation plays a crucial role in meeting consumer demand at the right time, in the right place, in the right quantity and at an affordable price (Lenne and Ward, 2010; Dessie et al., 2019; Owusu and Iscan, 2020).
In developing countries, strategies aimed at reducing poverty rely on improving agricultural production and providing farmers with access to formal markets (Kumar et al., 2004; Narayanan, 2014; Olutumise, 2020). However, the agricultural output market in developing nations is characterized by a number of difficulties including far-flung markets, poor road systems, lack of market participants, weak market information system and high marketing costs that prevent farmers from entering new markets and restrict the advantages they can obtain from market participation (Dhaka and Poonia, 2010; Yaregal, 2018; Aliyi et al., 2021). Farmers are unable to sell their agricultural produce in an organized manner and do not receive a fair share of consumer prices (Baba et al., 2010; Mishra et al., 2014; Chand et al., 2020, 2021). Himachal Pradesh is one of the hilly states with highest area and production under pea. Pea cultivation holds immense significance in the agrarian landscape of the state, playing a pivotal role in bolstering the local economy and sustaining the livelihoods of countless farmers. The cultivation of peas translates into substantial economic gains for the region. This, in turn, leads to a steady inflow of income, crucial for the financial stability of farming households. Additionally, pea cultivation generates substantial employment opportunities, encompassing activities from sowing to harvesting and subsequent processing. This not only reduces unemployment in rural areas but also fosters a sense of economic empowerment among the local population. The state has adverse climatic conditions divided into four agroclimatic zones which shows substantial potential for pea cultivation. Peas are widely grown by farmers in the high hills wet temperate and mid hills sub-temperate zones during the kharif and rabi seasons, serving as a means to enhance family income, achieve food security and improve livelihoods (Bala et al., 2011; JICA, 2021). The choice of an output marketing channel is a crucial decision for farmers, as it has a significant impact on farm profitability (Gelaw et al., 2016; Pham et al., 2019; Lee et al., 2020). However, understanding marketing channels for garden peas in Himachal Pradesh is of paramount importance for several compelling reasons. Firstly, it directly influences the income of farmers. A well-organized marketing channel ensures that farmers receive fair prices for their produce, preventing exploitation and guaranteeing a sustainable livelihood. This, leads to increased economic stability for farming communities. Moreover, effective marketing channels have far-reaching implications for food security. By facilitating the efficient distribution of garden peas, it ensures a steady and reliable supply of this nutritious crop to both local and distant markets. This contributes significantly to the overall food security of the region, safeguarding against potential shortages and fluctuations in availability. Additionally, the presence of robust marketing channels has a profound impact on the agricultural sector at large. It encourages investment, innovation and modernization, bolstering the sector's overall growth and productivity.
In Himachal Pradesh, farmers sell their pea produce through formal and informal markets. Informal markets are unorganized they are typically local roadside markets while formal markets are organized and provide better market spaces for farmers. Formal markets offer better prices to farmers for their agricultural produce (Manjunath and Girish, 2016; Singh et al., 2020; Thakur et al., 2023). Therefore, farmers who choose to participate in formal markets have an opportunity to maximize their profits from pea marketing. However, the level of participation in formal markets by farmers in Himachal Pradesh is low. Moreover, there is a lack of recent empirical data that validates the market performance and factors influencing farmers choices to participate in the agricultural output marketing channels for pea crops. Analyzing market performance and choice is important for decision making in agricultural produce marketing, yet policy analysis has not been done in this area. It is therefore difficult to know what motivates or hinders farmers of the area to participate in particular output market. To this end, the present research sought to analyze the determinants that influence farmers choice to participate in marketing channel. As a result, taking this into account, this study was carried out to examine and fill the existing research gap. The study proffered answers to the following questions: (1) what are the existing agricultural output marketing channels in the study area? (2) what is the scenario of market performance and who get more benefit along agricultural marketing channels? (3) what are the factors influencing pea producer choices in the study area? (4) what are the constraints faced by the pea farmers in the study area? This present research offers substantial contributions to both academic literature and practical policymaking in the agricultural sector. In terms of academic contributions, the study provides a comprehensive evaluation of the marketing performance of garden pea farmers, filling a potential gap in the existing body of knowledge specific to this region. Moreover, by employing advanced econometric techniques, the research offers a methodologically robust approach to understanding the factors influencing farmers choice of marketing channels. This methodological rigor enhances the academic credibility of the study. Further, the investigation into the constraints faced by farmers during the marketing process provides valuable empirical insights that can inform further scholarly inquiries and contribute to a more nuanced understanding of agricultural marketing dynamics in the region. The results obtained from the research would be helpful in drafting appropriate policies regarding institutional development and creation of systematic agricultural market infrastructure which could benefit the farmers of the India.
The remainder of this paper is organized as follows: The next section overview the theoretical background and empirical evidences followed by data and methodology. In this section nature and sources of data, research design, various stages of analysis and technique are discussed. The results and discussion are presented in the following section. The last sections present the conclusion, suggestion and policy implications.
2 Background and empirical evidences
This section presents the theoretical background and empirical evidence related to marketing channel choice and marketing performance in the agricultural output marketing sector. The primary objective is to review previous studies and identify the indicators or proxies used by researchers to analyze marketing channel choice and performance in this sector. A secondary purpose is to identify the main methodologies employed in previous studies focusing on marketing channel choice and market performance. Several researchers have studied different types of output marketing channels for vegetable crops (Sidhu et al., 2010, 2011; De and Rahaman, 2014); Mishra et al., 2014). Agricultural output marketing channels refer to the market institutions through which agricultural commodities, goods, or services are transferred from original producers (farmers) to end users or consumers (Coughlan et al., 2001; Chopde, 2019; Kala, 2020; Mondal et al., 2020). Based on the reviewed studies, it can be concluded that agricultural output marketing channels create employment opportunities for farmers, village traders, commission agents, wholesalers and retailers. Further, research suggests that establishing a modern market infrastructure through public-private partnerships can improve agricultural marketing channels (Jalalzadeh et al., 2014; Krafft et al., 2015; Naveen et al., 2015; Routroy and Behera, 2017; Ozor and Nwankwo, 2018). A significant portion of the literature, including Park et al. (2014), Park (2015), Gebrehiwot et al. (2018) and Park et al. (2018) focuses exclusively on the impact of direct marketing on farm sales, comparing farms that participate in direct marketing to those that do not. To analyze the market performance of agricultural marketing channels for vegetable crops, researchers commonly use indicators such as marketing cost, marketing margins, marketing efficiency, price spread and the producer's share in the consumer rupee method (LeRoux et al., 2010; Bala et al., 2011; Bukar et al., 2015; Mutayoba and Ngaruko, 2015; Hailegiorgis and Hagos, 2016; Abdulai et al., 2017). According to the performance studies, selling vegetables directly to consumers resulted in the highest overall gross marketing margin and profit margin, while selling crops through intermediaries across the channel yielded the lowest margins (Mmasa et al., 2013; Osondu et al., 2014; Negasi, 2015). Moreover, Baba et al. (2010), Dastagiri et al. (2013), Devkota and Sharma (2014), Chand et al. (2020) found that the producer-to-consumer channel was the most efficient among all the marketing channels adopted by farmers for their specific vegetable produce. Additionally, Kumar et al. (2019), Devi et al. (2020), Thakur et al. (2023) identified several major constraints in the agricultural marketing system, including poor product handling and packaging, ineffective pricing system, lack of transparency in market information, limited market availability, high number of middlemen and lack of coordination among producers. The choice of marketing channel is a crucial decision for farm households as it significantly impacts farmers income and livelihood (Jari and Fraser, 2009; Mafukata, 2015; Harrizon et al., 2016; Marine et al., 2016). Farmers take various factors into consideration when selecting a marketing channel (Makhura et al., 2001; Manjunath and Girish, 2016; Marine et al., 2016). Previous studies have consistently found that household factors have a significant influence on farmers' participation in output markets (Wiersinga et al., 2007; Argade and Laha, 2018; Donkor et al., 2018; Fan and Garcia, 2018; Siddique et al., 2018; Anh and Bokelmann, 2019). Whereas, Tsourgiannis et al. (2008) observed that the choice of marketing outlet is influenced by factors such as sales price, payment speed and loyalty. According to a study by Blandon et al. (2009) and Adanacioglu (2017) farmers have strong preferences for new supply chains that involve pre-arranged prices and quantities with buyers, but they still prefer traditional spot markets for certain attributes such as the absence of produce grading, cash payments, lack of delivery schedules, the ability to sell at the farm gate and the ability to sell individually. Many researchers, including Chirwa (2009), Xaba and Masuku (2013), Edoge (2014), Geoffrey et al. (2015), Soe et al. (2015), Gelaw et al. (2016), Pham et al. (2019), Chiv et al. (2020), Lee et al. (2020) have used multinomial logistic regression analysis to investigate the factors that influence farmers marketing channel choices. Farmer's age, quantity of produce, education, farm size, farming experience, storage facilities, access to information, distance to the market, and output price/payment at the time of sale have all been identified as significant determinants of farmers decisions to sell their farm produce through a particular marketing channel (Maina, 2015; Harrizon et al., 2016; Ntimbaa and Akyoob, 2017; Nxumalo et al., 2019; Ihli et al., 2021). Factors such as farmers age, distance from the farm to the market, marketing agreements and membership in farmer associations have played important roles in choosing a wholesale market channel over other market channels (Mafukata, 2015; Zhang et al., 2017). Whereas study conducted by Soe et al. (2015) concluded that farmers with access to market information, storage, transportation and larger quantities are more likely to choose remunerative direct marketing channels, such as selling directly to rice mills. Another study by Cheng et al. (2016) focusing on consumer choice found that traditional marketing information outlets such as television, radio and well-educated consumers, preferred the internet as a decision-making tool. On the basis of the theoretical background and empirical evidences under this section, it can be concluded that important factors influencing farmers agricultural output marketing channel choices are education, income, farming experience, storage facility, source of market information, time of sale, type of payment and distance to market.
3 Material and methods
3.1 Study area
The study was carried out in Himachal Pradesh, India, which is situated in the foothills of the North-Western Himalayas and located between latitudes of 30 22′ 40′′ to 33 12′ 20′′ N and longitudes of 75 45′ 55′′ to 79 04′ 20′′ E. Its altitude ranges 350 to 6,975 meters above mean sea level (amsl) and has a wide variety of climate niches. The State of Himachal Pradesh has been divided into four agro-climatic zones, characterized by varying elevations ranging from below 650 to over 2200 meters above mean sea level (amsl). The selection of sampled household was done from two agro-climatic zones of the state, mid hills sub humid, Zone-II and high hills temperate wet, Zone-III based on maximum area under vegetable cultivation (JICA, 2021). Hence, the study area was stratified into these two strata (Figure 1).
3.2 Sampling procedure
3.2.1 Farmers sampling
The sample farmers households were selected using a multistage stratified random sampling method. In the first stage of sampling, a list of blocks in the selected agro-climatic zones was compiled, and five blocks were chosen based on the maximum cultivated area under vegetables. Next, a list of all Gram Panchayats in the chosen blocks was created, and four Gram Panchayats were randomly selected from each chosen block. Finally, 10 farmers were randomly selected from each Gram Panchayat to create a sample size of 400 farmers. The use of stratification and randomization helped to ensure that the sample was representative of the target population in the study area.
3.2.2 Selection market intermediaries
The study determined the sample size of market functionaries on the basis of information obtained from the agricultural produce market committee office. As a result, two main markets were chosen in each selected agro-climatic zone. In the mid-hills sub-humid Zone-II, the solan and mandi markets, while in the high hills temperate wet Zone-III, the shimla and theog markets were selected purposively. Further, to investigate different aspects of pea output marketing, a total sample size of 80 traders was formed by randomly selecting 5 local traders, 5 commission agents, 5 wholesalers and five retailers from each market in each agro-climatic zone.
3.2.3 Data source and collection
In present research study, both quantitative and qualitative data were collected from primary as well as secondary data sources. Primary data were collected by researcher with the help of self-structured questionnaire by interviewing the farmers and different market functionaries. The process of designing the self-structured questionnaires for this study began with a thorough review of existing literature, particularly focusing on agricultural marketing. This literature review served as the cornerstone for identifying key variables and established measures relevant to the research objectives. To ensure the accuracy and effectiveness of data collection, several critical steps were taken. Each identified variable was meticulously defined to guarantee conceptual clarity and consistency. Questions were thoughtfully formulated to directly address these variables, emphasizing clarity, specificity, and freedom from ambiguity to facilitate accurate responses. Before the main data collection phase, a pilot test was conducted with a small sample of farmers. This exercise provided valuable feedback, which was instrumental in refining and fine-tuning the questionnaire. The questionnaire aimed to gather information from pea farmers regarding their production, marketing activities, and market preferences. Additionally, data on marketing costs, market margins and market efficiency were collected to assess the performance of pea marketing channels. Data on household factors, vegetable production, sales and sources of market information were also collected to evaluate their influence on farmers' choices of agricultural output market channels. Further, relevant information was collected to examine the various constraints faced by the farmers in marketing of pea. Moreover, ethical considerations were addressed within the questionnaire, ensuring respondents were informed about the study's purpose, their voluntary participation and the confidentiality of their responses. By adopting this meticulous approach to questionnaire design, the researcher aimed to collect accurate and reliable data that effectively addressed the variables of interest. The use of a self-structured questionnaire, informed by a comprehensive review of existing literature, ensured that the instrument was tailored to the specific context of garden pea cultivation in Himachal Pradesh, ultimately contributing to the validity and robustness of the research findings. Whereas, secondary data pertinent to the study were obtained from diverse sources such as books, journals, and university reports and also publications from government departments such as horticulture, agriculture, directorate of statistics and land records.
3.3 Analytical framework for marketing performance
The evaluation of market performance involves analyzing the costs and margins associated with marketing agents across various channels. A frequently utilized method to assess performance is by examining measures such as marketing costs, market margins, price spreads and market efficiency (Chand et al., 2020; Aliyi et al., 2021).
3.3.1 Marketing cost
The marketing costs were calculated by combining the costs incurred by each marketing functionaries participating in the supply chain process of pea produce. The amount of money spent on marketing differed depending on various factors such as the type of specific marketing activities, the type of marketing institutions and the location of marketing. The intermediaries marketing costs comprised cost for packaging materials, fees for loading and unloading, transportation costs, commission charges and taxes (Acharya and Agarwal, 2016).
The calculation involved determining the overall cost of marketing activities as follows:
Where,
TCm = The total cost associated with marketing of pea produce
Cg = The cost incurred by the farmer in the marketing of pea produce
MCi = The marketing costs incurred by ith middleman.
3.3.2 Marketing margin
Marketing margin analysis involves comparing prices at different levels of the marketing chain within the same timeframe. It assesses the portion of the final selling price that a specific agent in the marketing chain captures and is expressed as a percentage of the final price or the price paid by the end consumer (Thakur et al., 2023). Therefore, marketing margins were utilized in this study as an important indicator for evaluating market performance (Ghorbani, 2008). The marketing margin is the disparity between the price paid to the initial seller, often known as the farm-gate price and the price paid by the ultimate buyer, commonly referred to as the retail price (Abankwah et al., 2010).
In the present research study, marketing margins were calculated by determining the absolute margin. This involves the cost price (purchase price and marketing cost) that was subtracted from the selling price of pea by a market agent. The length of the marketing chain, the number of economic activities involved during marketing and the profit expectations of each marketing agency are some of the factors that affect the size of the marketing margins in different agricultural output marketing channels for pea. To determine the percentage of marketing margins obtained by each intermediary involved in the marketing of pea produce, the following formula as mentioned by Acharya and Agarwal (2016) was used.
Where,
Ami = Absolute margin of middlemen
PRi = Total value of receipts per unit (price of sale)
Ppi = Purchase price per unit of goods
Cmi = Marketing cost incurred per unit.
Where, TGMM represents the total gross marketing margin. Additionally, it is beneficial to consider the concept of producer's gross margin (GMMP) which denotes the proportion of the consumer price that is received by the producer. The calculation for the producer's margin is as follows:
Where, GMMp = the producer's share in consumer price.
Whereas, the net marketing margin (NMM) refers to the portion of the final price received by intermediaries as their net income, taking into account the deduction of their marketing costs.
The proportion of net income that can be categorized as pure profit, indicating the return on capital, varies depending on factors such as the intermediaries' own costs (including working capital). The equation reveals that a higher marketing margin reduces the share of the producer, and conversely, a lower marketing margin increases their share. This equation also offers insights into the distribution of welfare among production and marketing agents. A higher net marketing margin (NMM) or profit for the marketing intermediaries indicates a decrease in downward and unfair income distribution. This situation can lead to a discouraging market participation for smallholders.
3.3.3 Price spread
Price spread refers to the disparity between the price paid by the consumer and the price received by the producer. The calculation of price spread was performed using the formula recommended by Acharya (1988).
Where,
PS = Producer's share in consumer's rupee
PF = Price of produce received by farmer and PC = Price of produce paid by consumer.
3.3.4 Marketing efficiency
Marketing efficiency focuses on the efficient transfer of goods from producers to consumers, aiming to achieve the lowest possible cost while providing the desired services to consumers. In the study area, the marketing efficiency of different channels was assessed using Acharya's approach, as suggested by Acharya (2016), Acharya and Agarwal (2016).
Where,
FP = Price received by the farmer
MC = Total marketing cost
MM = Net market margins.
3.4 Analytical framework for factors influencing choice
3.4.1 Factors influencing farmers choice
The Random Utility Model is a framework that can be applied to analyze the marketing channels that influence farmers decisions on selling their agricultural produce. Several studies, such as those by Mtimet and Albisu (2006), Geoffrey et al. (2015), Ma and Abdulai (2016) and Espinosa-Goded et al. (2021) have utilized this model in their research. The model assumes that farmers choose their marketing channels based on the expected value and that the decision to participate in a specific channel is based on maximizing a utility function (Greene, 2003; Train, 2009). In other words, farmers weigh the expected gains, costs, and advantages of each channel and choose the one that provides the highest overall utility. The utility gained from selling through a specific channel, denoted as Uij. The utility is defined as a linear function of the channel-specific parameter vector (βj), the characteristics of that option (Xij) and a stochastic error component (eij) for each farmer selecting a specific option.
The farmer's decision on which output channel to use is based on the utility which is the difference between the benefit and cost. This utility cannot be directly observed, but the decision made by the farmer indicates which output channel offers the most utility (Greene, 2012). If the expected utility from selling the agricultural produce through a particular output channel is greater than that of all other options, the farmer will choose that channel. The probability of selecting a specific option depends on the likelihood that its utility will be higher or at least equal to the utilities of every other alternative in the decision set (Mariel et al., 2022). The farmer will select the j=k market channel for agricultural output if the utility of that channel is greater than the utility of all other channels as expressed in equation (2).
The random utility associated with the market channel j=k is denoted as Uij while the index function β(j = k)Xij represents the average utility of the producer associated with this choice. The second term eij represents a random error specific to the producer's utility choice, as mentioned by Louviere et al. (2000), Tafesse et al. (2020). Moreover, based on the empirical evidences presented in the theoretical background section, Figure 2 illustrates the conceptual framework of the dependent variables that affect farmers choices regarding the channels they choose for marketing their pea output. The choice of market channels described the farmers decision to sell the pea produce to retailers, local traders, commission agents, wholesalers and consumers. Whereas, determinants of choice are four categories which includes household factors (education, farm income), vegetable production factors (farming experience, storage facilities, distance to market), vegetable sales factors (financial urgency, payment in advance, payment at the time of sale, slow sale) and source of market information factors (word of mouth/relative/friends, government department/tv radio).
3.4.2 Multinomial logit regression model
We used a multinomial logistic regression model to assess farmers preferences for agricultural output marketing channel. We employed this technique because farmers in the study regions have more than two options for marketing their farm produce. The model plays significant role, because through this choice method has the ability to value multiple marketing channels. To examine the factors influencing their choice of marketing channels, a Multinomial Logit Regression (MNL) model was employed. The MNL model is commonly utilized when there are several alternatives for the variable being explored (Bardhan et al., 2012; Martey et al., 2012; Delong et al., 2019; Asante and Weible, 2020; Goncalves et al., 2022). This technique is suitable for analyzing responses that are not ordered and involve more than two options (Chung et al., 2011; Mgale and Yunxian, 2020; Olutumise, 2022). Furthermore, based on the conceptual framework and previous empirical research on market channel selection, several relevant explanatory variables with potential impact on the choice of marketing channels were identified and incorporated into the multinomial logit analysis (Panda and Sreekumar, 2012; Gelaw et al., 2016; Pham et al., 2019; Thakur et al., 2022a,b).
According to Greene (2003), Gujarati and Porter (2009) we make an assumption regarding the probability(Pij) that the ith farmer will choose the jth agricultural output marketing channel among four available options. Consequently, the multinomial logistic regression model was used to estimate the probability of a farmer selecting a specific alternative j in the following manner:
Where, xi is a distinct characteristic of the ith farmer, while βj represents a set of estimated regression parameters associated with the jth alternative. It is important to note that there are four agricultural output market channels available for selection in the choice set.
In the multinomial logit model, the coefficients of the independent variables for the reference or omitted category are assumed to be zero. To determine the probability of selecting the base category, the following equation is employed:
The probabilities of the ith farmer belonging to the remaining three categories (where j = 2, 3, or 4) can be computed using the following approach:
To assess the impact of various characteristics on the likelihood, the marginal effects can be determined by differentiating equation (3) with respect to the covariates in the following manner:
Pj represents the probability that the pea producer will choose marketing channel j,
while
βj is a vector of regression parameter estimates specifically associated with option j,
The empirical Multinomial Logit Regression model incorporates the following variables that influence the farmers' selection of marketing channels for their pea produce:
Pij= 1n (Pi/Pj) = β0+ β1 Education + β2 Farm Income + β3 Farming experience + β4 Storage Facility + β5 Distance + β6 Financial Urgency + β7 Payment in advance + β8 Payment at the time of sale + β9 Slow sale. + β10 Market information through word of mouth + β11 Market Information T.V/Social-Media/Govt. Agency.
The variables that need to be estimated in the model are β0……………. β11.
Pij represents the probability that farmer i choosing marketing channel j for their agricultural output,
Where, j= 1 for producer → retailer → consumer, j= 2 for producer → commission agent → retailer → consumer, j=3 for producer → local trader → wholesaler → retailer → consumer j= 4 for producer → wholesaler → retailer → consumer. In the present research study, we used STATA-12 software to estimate the empirical model.
3.5 Analytical framework for marketing constraints
In order to investigate the constraints faced by pea growers in marketing, researchers utilized Garrett's ranking method (Chand et al., 2020; Thakur et al., 2023). This method offers a significant advantage over a basic frequency distribution as it allows constraints to be ranked based on their perceived severity from the farmers perspective. Farmers were requested to assign a rank to each category of constraints presented to them using this approach. The per cent position for each rank was then calculated using the following formula:
Where:
Rij = Rank given to ith position by the jth individual
Nj = Numbers of problems ranked by jth individual
The per cent position was converted into scores using the reference table suggested by Garrett and Woodworth (1969). Subsequently, the scores of each respondent were summed and divided by the total number of respondents who provided scores. These average scores for each factor were then arranged in descending order, allowing for the identification of the most influential factors through the assigned ranks. The factors with the highest mean value score were considered the most severe problems encountered by farmers in the study area (Karthick et al., 2013; Kenjit et al., 2021).
4 Results and discussion
4.1 Agricultural output marketing channels of pea crop
It can be observed from Table 1 and Figure 3 that the sampled farmers in the research area utilized five different output marketing channels to sell their pea produce. The most preferred channel, accounting for 50.5 per cent of the total quantity transacted among the channels was Producer-Commission Agent-Retailer-Consumer (Channel-C). This was followed by 20.5 per cent in Producer-Local Trader-Wholesaler-Retailer-Consumer (Channel-D) and Producer-Wholesaler-Retailer-Consumer (Channel-E). A smaller percentage, 6.25 per cent was transacted through Producer-Retailer-Consumer (Channel-B) and the minimum quantity of 2.25 per cent was sold through Producer-Consumer (Channel-A). These results shows that Channel-C, which involves selling through a commission agent to a retailer who then sells to consumers, can have advantages for farmers. Commission agents may help farmers find buyers for their crops, negotiate prices and handle the logistics of transporting the crops produce to market. Whereas, retailers may provide a consistent market for the crops and consumers benefit from having access to fresh, locally-grown produce. However, each marketing channel has its own advantages and disadvantages and farmers may choose different channels based on factors such as crop quality, market demand, transportation costs and competition from other farmers. Therefore, it is important for farmers to consider all their options and choose the marketing channel that best meets their needs and maximizes their profits.
4.2 Marketing costs and margins of various market functionaries
The data presented in Table 2 provides information on the marketing cost and margins of different market participants engaged in various marketing channels for pea produce in the study area. In Channel-A, where producers directly sold pea produce to consumers, the total marketing cost for producers amounted to rupees (Rs.) 61 per quintal. In Channel-B, where pea produce was supplied to retailers, the marketing cost for producers was Rs. 70 per quintal. For Channel-C, where pea produce was marketed to commission agents, the marketing cost for producers was Rs. 112.50 per quintal. Producers who sold their pea output to local traders in Channel-D paid marketing costs of Rs. 85 per quintal, while producers who sold their produce to wholesalers in Channel-E paid Rs. 90 per quintal. The packaging, loading/unloading fee, transportation costs, commission fee and Mandi tax are included in the marketing cost borne by the pea growers in the research area. However, these results shows that the marketing costs incurred by farmers is lower when selling directly from farmer to consumer compared to other marketing channels. This is because in direct sales, there are typically fewer intermediaries involved in the transaction which can reduce costs associated with transportation, loading/unloading and commission charges. Additionally, direct sales do not require as much transportation charges as sales to other market functionaries which can also reduce costs.
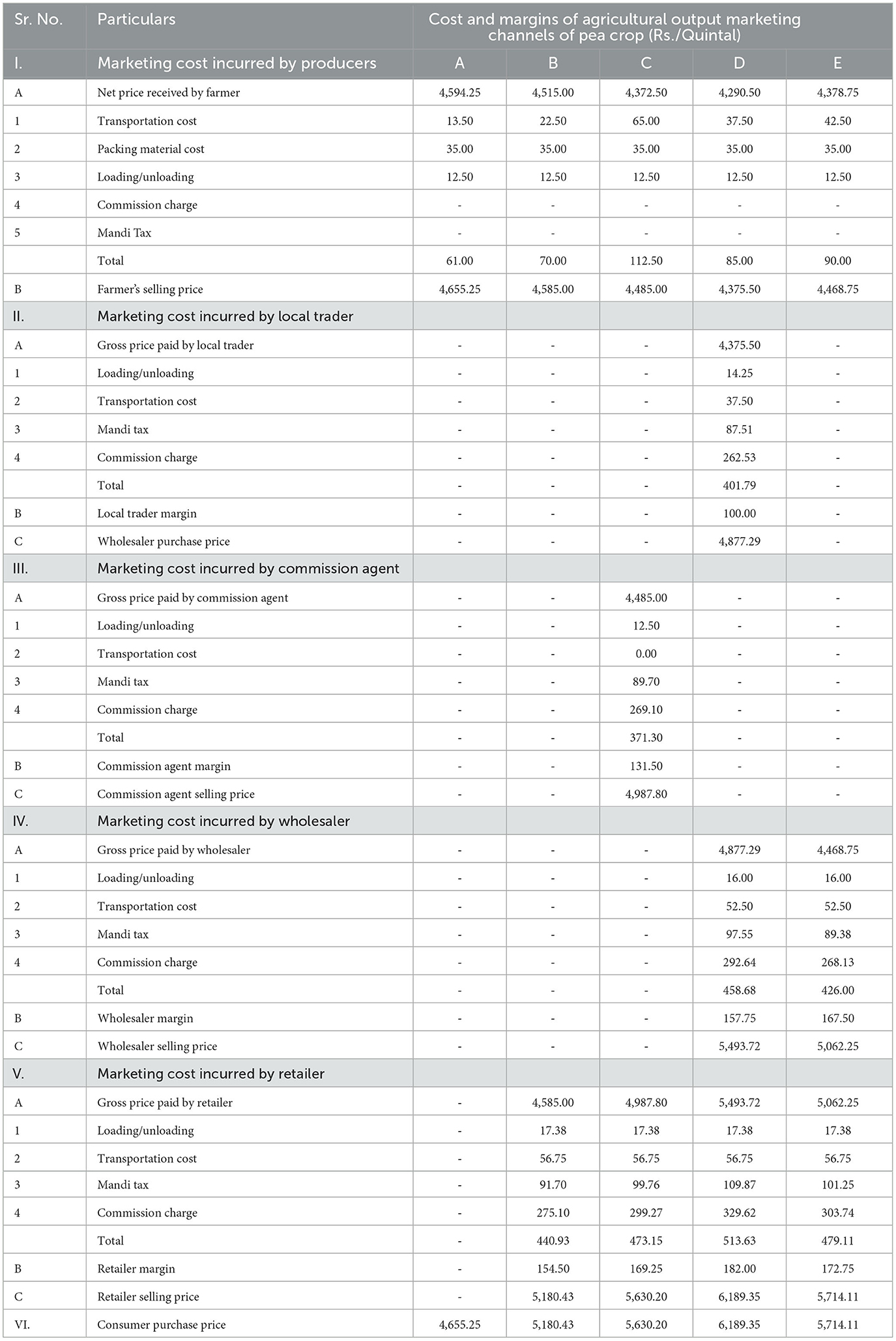
Table 2. Marketing costs and margins of various functionaries in the different marketing channels of pea crop.
The retailers can be observed across four different marketing channels in the study area, these are Channels B, C, D and E respectively. Aside from the producer, the retailer was the sole market participant selling produce directly to consumers. The significant marketing cost they incurred included commission fees, transportation costs, loading and unloading costs and Mandi tax. It has been determined that retailers in Channels B, C, D and E paid a total cost of Rs. 440.93, Rs. 473.15, Rs. 513.63 and Rs. 479.11 per quintal, respectively. Further, retailer margin per quintal in Channel B (Rs. 154.50), Channel-C (Rs. 169.25) and Channel-E (Rs. 172.75) respectively. However, retailer margin in Channel D (Rs. 182) was found to be the highest margin per quintal among all the functionaries. This means that the retailer is able to charge a higher price per quintal of pea crop compared to the other intermediaries in the channel which suggests that the retailer has a greater level of market power and is able to extract more value from the transaction. It is worth noting that the high retailer margin may also reflect higher costs incurred by the retailer such as commission charges, transportation and handling costs. However, retailers are the last point of contact between the agricultural produce and the consumer. As a result, they have more bargaining power and can charge higher prices for the produce. Moreover, retailers often add value to the product by providing services such as product display, marketing and customer service.
In the marketing Channel-C, the commission agent was discovered to be an important market functionary. The primary factors contributing to marketing costs have been identified as the commission charges, transportation cost, loading and unloading costs and Mandi tax. The total marketing cost spent by commission agent was Rs. 371.30, while market margin was Rs. 131.50 per quintal. The results reveals that the commission agent plays a significant role in the marketing of pea produce in Channel-C. Commission charges are fees paid to the commission agent for their services in facilitating the sale of goods. Loading/unloading costs are expenses incurred in the transportation of goods to and from the market. Mandi tax is a tax levied on the sale of goods in certain markets in India. By incurring these costs, the commission agent is able to provide valuable services to both buyers and sellers in the market. The commission agent helps buyers to find the goods they need, and helps sellers to find buyers for their goods. The commission agent also helps to ensure that the market operates efficiently by facilitating transactions and providing important market information to buyers and sellers. Further, the local trader had only been found in output marketing Channel-D. The local trader spent Rs 401.79 per quintal on marketing cost overall. Furthermore, the local trader engaged in the sale of the produce to the wholesaler. However, research did not find any evidence of local trader selling directly to the consumer. The results reveals that farmers sell their pea produce to local traders who act as intermediaries between the farmers and other market participants such as wholesalers and retailers. These local traders offer services such as transportation, storage and market information to farmers which help them to sell their produce more efficiently.
According to the data in Table 2, wholesalers were one of the crucial market participants that have been found in the marketing Channel-D and Channel-E. Commission fees, Mandi tax, and transportation were determined to be the main components of the wholesalers marketing costs which were found to be Rs. 458.68 and Rs. 426 per quintal respectively, in these channels. The data also indicated that in Channel-E, the wholesalers directly purchase from the farmers, whereas in Channel-D, the pea produce was bought through the local trader. However, the pea produce was sold to the retailer through both channels. Thus, wholesalers play an important role in the agricultural output marketing of pea by providing a link between farmers and other market functionaries, ensuring that the peas produce are available to other stakeholders in the marketing chain and helping to distribute the peas to retailers and other businesses.
4.3 Price spread and marketing efficiency
The perusal of data in Table 3 reveals that the producer's price for the pea crop displayed variation across different output marketing channels in the research area. Channel-D had the lowest producer's price at Rs. 4,290.50, while Channel-C recorded Rs. 4,372.50, Channel-E had Rs. 4,378.75, Channel-B had Rs. 4,515 and the highest price was observed in Channel-A at Rs. 4,594.25. In terms of gross marketing margin, Channel-D had the highest overall margin at 30.68 per cent followed by Channel-E (23.37%), Channel-C (22.34%), Channel-B (12.85%) and Channel-A (1.31%). The producer's share of the consumer's rupee was most significant in Channel-A (98.69%) when the producer directly acted as a retailer. This was followed by Channels-B (87.15%), Channel-C (77.66%), Channel-E (76.63%) and Channel-D having the lowest percentage at 69.39 per cent. The marketing margins varied across the channels with Channel-A having a margin of 0.00 per cent followed by Channel-B (2.98%), Channel-C (5.34%), Channel-E (7.53%) and Channel-D (11.98%). The cost of marketing differed as well with Channel-A having the lowest percentage at 1.31 per cent followed by Channel-B (19.86%), Channel-C (17%), Channel-E (17.41%), and Channel-D (23.57%).
It can be observed from the Table 3 that Channel-A (75.32%) was most efficient channel followed by Channel- B (6.79%), Channel-C (3.48%), Channel-E (3.01%) and Channel-D (1.81%) respectively. Thus, it was found that Channel-A was most efficient channel among all five output marketing channels. However, this channel was not prominent because it sold less quantities of pea produce than other channels. The possible explanation is that selling directly from farmer to consumer can be a more efficient marketing channel in terms of costs, but it may not always be the most practical option for larger quantities of produce. Direct sales from the farmer to the consumer typically involve smaller volumes and may require more effort on the part of the farmer to market their pea produce such as setting up a roadside stand or participating in farmers markets. This can be a viable option for small-scale or specialty producers, but may not be practical for larger quantities of produce.
Moreover, Channel-A was determined to be the most efficient from the remaining four channels because consumer prices (Rs. 4,655.25) were the lowest and farmer prices (Rs. 4,594.25) were the highest. Other marketing channels such as selling to retailers, wholesalers, local traders commission agents, may involve higher marketing costs but can facilitate the sale of larger quantities of produce. These channels often have established distribution networks and access to a wider customer base which can help to increase the volume of produce transacted. Ultimately, the choice of marketing channel depends on a variety of factors including the scale of production, market demand and available distribution networks. A combination of different marketing channels may be the most effective approach to maximize sales and profits for farmers.
4.4 Factors affecting farmers choice of agricultural output marketing in pea crop
Table 4 presents descriptive statistics and definitions of the variables that influence farmers choice of agricultural output marketing channels for the pea crop. These variables were utilized in the multinomial logit regression analysis. Factors like household (education, farm income), vegetable production factors (farming experience, storage facilities), vegetable sales factors (financial urgency, payment in advance, payment at the time of sale, slow sale) and source of market information factors (word of mouth/relatives, friends, Govt. dept/TV/Radio) are considered as independent variable. The coefficients of variation (CV) revealed that more volatility was noticed in storage facility (161.48) whereas less in education (41.26) variable which was used in the model.
4.5 Maximum likelihood estimates
The data presented in Table 5 shows the various factors that influence the decision of pea farmers in Himachal Pradesh when choosing agricultural output marketing channels. A multinomial logistic regression analysis was employed to examine this. We identified five marketing channels for pea crop and these channels were grouped into five outcome categories. To analyze the likelihood of selecting one market channel over another, we used Channel-A as the base or reference category. The coefficients presented in the first, second, third and fourth columns represent the coefficients and significance of choosing Channel-B (Producer-Retailer-Consumer), Channel-C (Producer-Commission Agent-Retailer-Consumer), Channel-D (Producer-Local Trader-Wholesaler-Retailer-Consumer) and Channel-E (Producer-Wholesaler-Retailer-Consumer) instead of Channel-A (Producer—Consumer). The log likelihood ratio test indicates that the overall model is statistically significant. According to the chi-squared test statistic, which is significant at a 1% level, the estimated multinomial logit model tends to provide a reasonable regression and as a result the independent variables in the model adequately explain the variation in the dependent variable. Regarding the characteristics of the households, the education variable was found to be significant in influencing farmers choices to join agricultural output marketing Channel-B and Channel-C. On the other hand, farm income affects the farmers decision to participate in output marketing Channel-C, D and E, respectively. Whereas, factors related to pea crop production such as farming experience, were found to influence farmers choices to sell their pea produce through output Channel-B and E. Similarly, the distance to the market also affects farmers options in Channel-B, C and E. Moreover, the availability of storage facilities was found to have a negative significance in Channel-C and E. With regard to vegetable sales factor, the payment in advance was significant in farmers choice to select output marketing Channel-B, whereas payment at the time of sale was found to be significant in Channel-C. Slow sale was also significant in Channel-B and Channel-C. Furthermore, the source of market information factors such as word of mouth/relatives/friends, significantly impacted farmers preferences to join Channel-B, additionally government departments/TV/radio also influenced producers desire to market their pea produce through Channel-C and D.
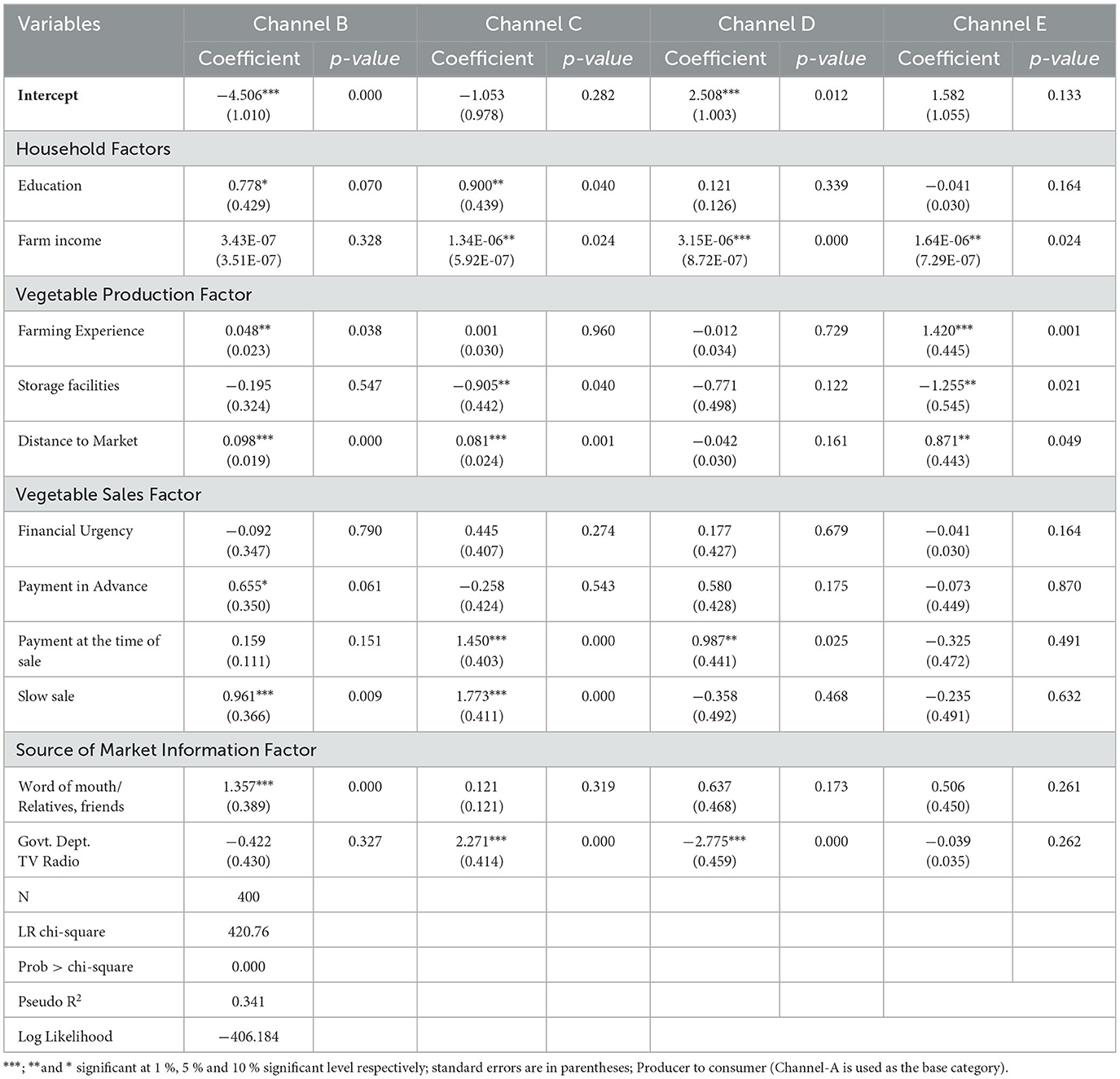
Table 5. Maximum likelihood estimates for factors affecting choice of agricultural output marketing channel of pea crop.
4.6 Marginal effects estimates
The marginal effects (ME) demonstrate how each unit increase in an independent variable, increases or decreases the probability of selecting an alternative marketing channel. Table 6 presents the estimated marginal effects of the multinomial logit model, focusing on the factors that influence the choice of agricultural output marketing channels for pea in the study area. Education plays a crucial role in reducing the cost and time associated with searching for information and processing it effectively (Pingali et al., 2005; Cheng et al., 2016). According to Table 6, education was found to be positively significant at 5% significant level in determining farmers choices to sell pea produce through agricultural output marketing channel producer-wholesaler-retailer-consumer (Channel-E). The education factor increased the probability of choosing marketing Channel-E by 7.6%. This can be attributed to the fact that as farmer households acquire more education, they gain marketing skills and knowledge that enable them to sell peas in lucrative markets with higher returns, such as output marketing Channel-E. Moreover, farmers with higher levels of education possess greater knowledge to adopt effective farm business management practices, utilize new technologies, adhere to production standards and implement management measures for their pea crops, which subsequently meets the marketing standards (Mariano et al., 2012; Alam, 2015). The farm income was found to be positively significant at the 1% significant level in output marketing Channel-C and at the 10% significant level in Channel-E, influencing farmers choices to participate in agricultural output marketing channels. Conversely, farm income was negatively significant at the 1% level in Channel-B. An increase in farm income by one unit has significantly increased the probability of selling to Channel-C and E by 0.1% (P-value 0.000 and 0.091) per unit increase in farm income. This can be attributed to the fact that output marketing Channel-C and E are capable of purchasing pea produce in bulk. The marginal effect on the other hand shows that a unit increase in farm income results in a 0.1% reduction in the likelihood of selecting Channel-B when compared to the base category. These findings align with studies conducted by Barrett (2008) and Mzoughi (2011) which indicate that farmers with higher farm income tend to sell their crops through modern marketing channels (such as Channel-C and E) that can accommodate their larger crop outputs, thus allowing them to engage in other farm activities. Farmers with significant farming income are more likely to afford necessary farm inputs, enabling them to achieve the required quality for the market.
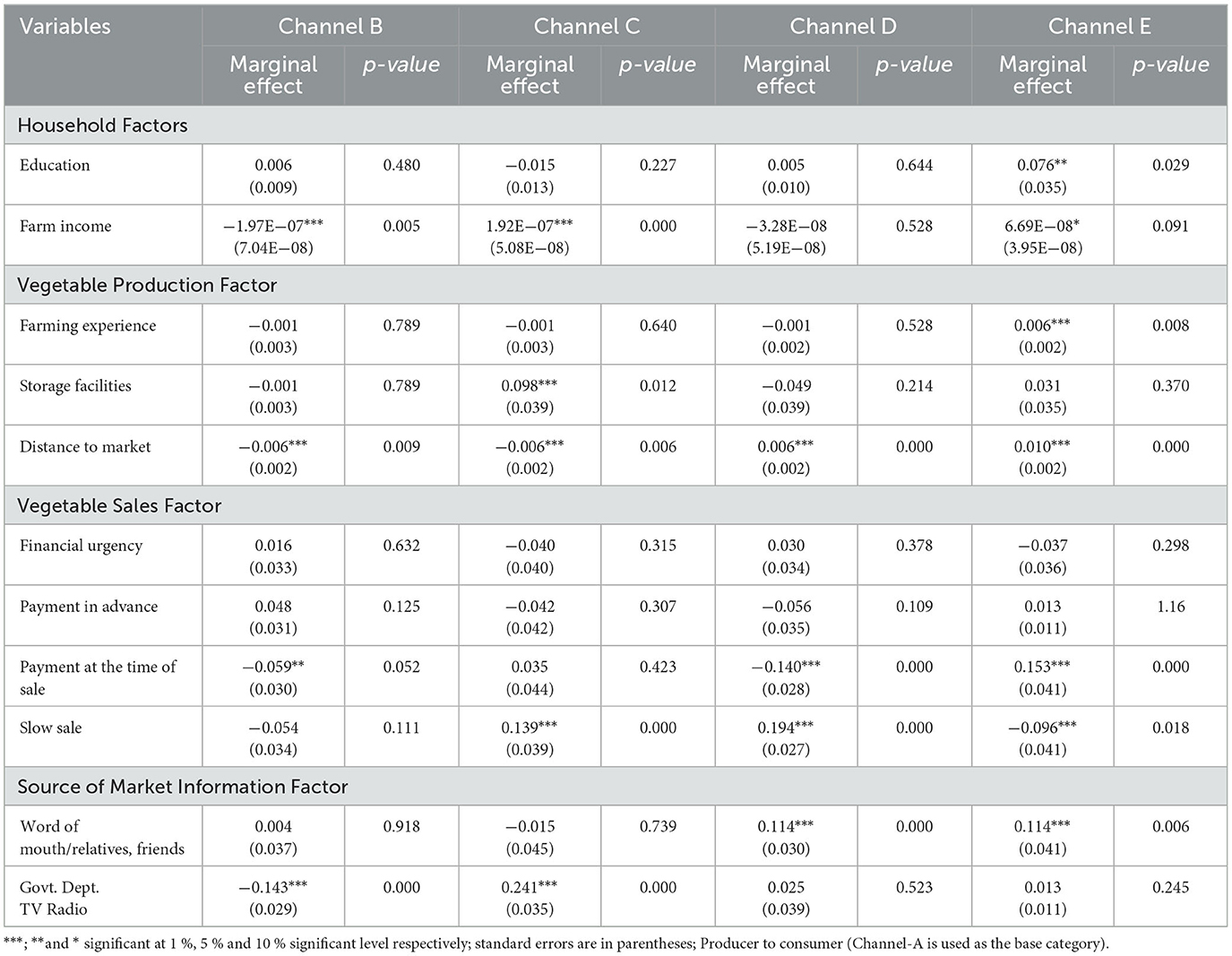
Table 6. Marginal effects estimate for factors affecting choice of agricultural output marketing channel of pea crop.
With respect to vegetable production factors, Table 6 shows a significant positive relationship between farmers choice of marketing channels and farming experience at the 1% significant level in output marketing Channel-E. The marginal effects show that as compared to the base category, farming experience increases the likelihood of choosing output marketing Channel-E by 0.6%. Farming experience directly relates to farmers bargaining power and marketing network. The long-term relationships built over the years between farmers and market intermediaries may contribute to farmers preferring output marketing Channel-E. Previous studies by Deressa et al. (2009), Hisali et al. (2011), Barrett et al. (2012), and Alam (2015) have also highlighted the strong effect of farming experience on farmers decision-making. Moreover, the possession of storage facilities is significantly positive at a 1% significant level in output marketing Channel-C. A unit increase in storage facility leads to a 9.8% increase in the probability of farmers choosing Channel-C compared to the base category. This positive relationship indicates that if a farmer has access to storage facilities, the likelihood of intending to sell through Channel-C increases. The proximity to the nearest market emerged as a significant factor influencing the selection of agricultural output marketing channels. Table 6 shows that farmers who live far from the market are more likely to sell their pea produce through agricultural output marketing channels like Channel-B, C, D and E rather than the base category. The distance to the market exhibited a positive significance at the 1% significant level in Channel-D and E, while it showed a negative significance at the 1% level in output marketing Channel-B and Channel-C. The marginal effects, indicate that compared to the base category, the probability of selecting output marketing Channel-D and E increased by 0.6% and 1.0% respectively, while the likelihood of selecting Channel-B and C decreased by 0.6% each. Similar findings were observed by Mmbando et al. (2015) who revealed that when farmers have a large quantity of crop output for sale, they tend to prioritize selling their crop output in the market, even if it requires traveling to a distant market.
Farmer's choice of pea output marketing channel can change based on the maximization of benefits. Factors related to pea sales directly impact production choices and crop yields, thereby influencing farmers' decision-making. One such sales factor is the payment received at the time of sale, has a significant relationship with the choice of output marketing channel. In output marketing Channel-E, there was a significant positive connection at the 1% significant level. Furthermore, the marginal effects show that the probability of choosing output marketing Channel-E with payment at the time of sale increased by 15.3% in comparison to the base case. However, payment received at the time of sale showed a negative significance at the 1% and 5% levels for choosing output marketing Channel-D and B, respectively. For instance, compared to the base category, a unit increase in payment at the time of sale resulted in a 14.0% and 5.9% decrease in the likelihood of selecting output marketing Channels D and B, respectively. The empirical results presented in Table 6 indicate that sales factors related to vegetables, such as slow sales, directly lead to lower income for farmers. The findings reveal that slow sales of pea crops in marketing Channel-A influence farmers decisions to participate in other marketing channels. Slow sales were found to be positively significant at the 1% significant level in agricultural output marketing Channel-C and D, while they showed a negative significance at the 1% significant level in Channel-E. Further, the marginal effects indicate that slow sales of pea crop significantly increase the probability of choosing output marketing Channel-C and Channel-D by 13.9% and 19.4% respectively. Moreover, the marginal effects also indicate a decrease in the probability of choosing Channel-E by 9.6%.
It is evident from Table 6 that source of market information factors are important variables which influence farmers choice to participate in agricultural output marketing channel. Access to market information through word of mouth, relatives and friends was found to have a positive significance at the 1% significant level, influencing farmers' decision to select marketing Channel-D and E. The results reveal that word of mouth, relatives, and friends increase the probability of farmers selling their produce through both output marketing Channel-D and E by 11.4%. This suggests that farmers are less likely to travel to the market center to sell their pea produce through marketing Channel-A if they acquire market information through informal networks. Instead, they prefer to sell through marketing Channel-D and E. Access to marketing information plays a crucial role in encouraging farmers to explore new innovations (Zhang et al., 2017). Farmers who receive market information through government departments, TV and radio show a positive significance at the 1% significant level. Farmers are 24.1% more likely to select and sell their pea produce through output marketing Channel-C if they have better access to information about market from these sources. This outcome is crucial since pea growers sometimes lack access to marketing information when deciding how to sell their crop. Marketing Channel-C which involves a Producer-Commission Agent-Retailer-Consumer structure, uses advanced pricing with fixed prices. On the other hand, in marketing Channel-A, where the producer directly sells to consumers at farmers' markets, traditionally allows for variable and higher prices. Therefore, farmers may choose to sell their pea produce through output marketing Channel-A instead of Channel-C to increase their income. These findings are consistent with the previous studies conducted by Magesa et al. (2014), Fan and Garcia (2018) and Pham et al. (2019). These studies emphasize that timely access to market information enables farmers to make informed choices about crop selection, quantities and determining the most suitable markets for selling their agricultural produce.
4.7 Constraints faced by farmers
Table 7 presents the data pertaining to the constraints encountered by farmers in the marketing of the pea crop. It is evident from the table that the foremost constraint reported by the farmers in the study area was the lack of market consultancy services with the average percent score of garrett ranking was 62.68. Due to this farmer not have access to the latest information on market trends, pricing and consumer preferences which can lead to inefficient marketing strategies and lower profits. However, market consultancy services can provide farmers with valuable insights into market conditions such as supply and demand dynamics, market trends and price fluctuations. This information can help farmers make informed decisions about when and where to sell their crops as well as how to package and promote their farm produce to appeal to consumers. Moreover, market consultancy services can also provide farmers with guidance on quality control and standards compliance which are critical factors in ensuring that their crops meet the requirements of buyers and consumers. This can help farmers to avoid rejection or lower prices for their farm produce due to quality issues. The second major constraint was high commission charges with average percent score of garrett ranking was 62.68. In the study area farmers sell their crops through middlemen and local traders, they are charged a commission fee or a markup on the sale price which reduce their profits. The commission charges are too high leading farmers to seek out other marketing channels or sell their crops at lower prices to avoid these charges. This can ultimately affect their bottom line and limit their ability to invest in their farms and improve their productivity. Moreover, the lack of transparency around commission charges and other fees can make it difficult for farmers to negotiate better terms with buyers or to make informed decisions about which marketing channels to use.
The third major constraint was vehicle not available in time (per cent score 61.74). The present results reveals that farmers are unable to transport their crops to market in a timely manner, they experience delays that can reduce the quality and value of their pea produce. Transportation delays occur due to a variety of factors including inadequate road infrastructure, poor weather conditions and lack of available vehicles. In some cases, farmers also face challenges in coordinating with transport providers or negotiating fair prices for transportation services. Further, the marketing constraints such lack of all- weather roads (per cent score 59.15) ranked fourth in study area. The roads are not properly maintained or are impassable during certain seasons, farmers faced challenges in transporting their crops to market in a timely and cost-effective manner. This can lead to delays, spoilage and lower prices for their pea produce. Whereas exploitative practices by intermediaries (53.15) ranked fifth in constraints by the farmers in the study area. In the study area farmers sell their crops through local traders and middlemen they experience unfair or exploitative practices such as price manipulation, delayed payments, false grading and weighing of produce. These practices can have a significant impact on farmer's incomes and livelihoods, as they may result in lower prices, reduced profits and losses. It may be possible that farmers forced to sell their crops at prices that are significantly lower than market rates and to accept unfavorable terms. These conditions can limit their ability to negotiate better prices. Thus, it may have been stated that these emerging constraints requires a serious overlook and immediate concern by the state government and pertinent agencies resulting to bring better an ease of doing marketing and other allied activities for the farming community at large.
5 Conclusions
This study examines the marketing performance and factors influencing farmers choice of agricultural output marketing channels in pea crop. According to our findings, the marketing channel with the highest preference for pea produce was Producer-Commission Agent-Retailer-Consumer (Channel-C). Thus, it can be concluded that this marketing channel may have advantages such as providing a streamlined distribution process that enables the producer to focus on crop cultivation while leaving the marketing to intermediaries and ensuring a steady supply of pea produce for the retailer and consumer. The combinations of these factors make this channel the most attractive option for farmers. However, it may also result in higher prices for the end consumer due to the commissions charged by the commission agent. Our findings indicates that producer-to-consumer output marketing channels had the lowest overall marketing cost incurred by farmers. It implies that when farmers sell their pea produce directly to consumers, there are typically fewer middlemen involved in the transaction which reduces the cost of transportation, loading/unloading and commission charges. Therefore, direct sales can be a cost-effective way for farmers to market their farm produce and can help them earn a higher profit margin. Additionally, it was observed that the retailer incurred the highest total cost within Channel-C. Whereas, the retailer margin in this channel was found to be the highest margin per quintal among all the functionaries. This may be due to the fact that the retailer in this channel is able to charge a higher price to the consumer for the produce they are selling which helps them earn a higher profit margin, despite the higher costs they incur in the supply chain. The findings of the study further revealed that the producer-to-consumer channel in agricultural output marketing is considered the most efficient as it allows farmers to sell their produce directly to consumers without involving intermediaries, thereby reducing transaction costs and increasing profits for farmers. But this channel is not used by farmers due to the lower quantity of produce sold as compared to other channels. Therefore, our result suggests that there is need to create demand for locally produced and marketed farm produce. This can be accomplished through marketing and promotional campaigns that highlight the benefits of buying local such as supporting the local economy, reducing carbon footprints and getting fresher and healthier produce. Governments can also promote the use of local products in their procurement policies such as in schools mess, colleges mess and hospitals kitchen which can create a stable and reliable market for farmers. Another solution is to facilitate the aggregation of smallholder farmers produce through collective marketing arrangements such as cooperatives, farmer groups and associations. By pooling their resources and negotiating as a group, smallholder farmers can access larger markets and sell larger quantities of produce, while still benefiting from the efficiencies of the producer-to-consumer channel.
Further, the empirical results indicate that farm income, farming experience, storage facilities, distance to market, payment at the time of sale and market information through word of mouth, government department affects farmers choice of agricultural output marketing channels. Therefore, it can be concluded that farmers with higher incomes may be able to afford to transport their pea produce to more distant markets where they can sell them at a better price. Similarly, they may have the resources to store their crops until market conditions are favorable. Whereas, distance to market influences the logistics and transportation costs associated with selling pea produce. The present results revealed that, if the market is relatively close to the farm, farmers may have more options for marketing their pea produce including selling directly to consumers through farmers markets, community-supported agriculture programs, selling to local traders or retailers. Moreover, if the market is farther away, farmers may need to rely on longer-distance transportation methods such as trucking or pickup to get their peas to market. This can add significant costs to the process as well as increase the risk of spoilage and damage during transit. In such cases, farmers may choose to sell their pea produce through larger intermediaries such as commission agents and wholesalers who have more experience and resources for handling long-distance transportation. Further, the study indicates that when sales are slow farmers may need to explore alternative marketing channels and adjust their sales strategies in order to ensure that they are able to sell their crop and avoid losses. Moreover, it can be concluded from the results that farmers often rely on information and advice from their social networks when making decisions about how and where to sell their crops. If a farmer hears from a trusted source that a particular marketing channel is offering good prices for pea produce, they may be more likely to choose that channel over others. Similarly, if a farmer hears about negative experiences with a particular marketing channel, they may be more likely to avoid that channel in the future. Whereas, market information market information disseminated by government departments as well as through TV and radio programs information can provide farmers with valuable insights into market trends, pricing information and consumer demand which can help them make informed decisions about how and where to sell their crop. This information can help farmers assess different marketing channels and choose the one that is best suited to their needs and goals.
The results further revealed that lack of market consultancy services was the major constraints faced by farmers. Thus, it is concluded that it can limit the potential for agricultural growth and development, as farmers may miss out on opportunities to expand their markets, increase their profits and improve the quality of their farm produce. Whereas, the other marketing constraints are high commission charges, vehicle not available in time, exploitative practices by intermediaries and lack of all-weather roads. It can be concluded that all-weather roads are critical for enabling farmers to transport their crops to market throughout the year and regardless of weather conditions. When roads are properly maintained and designed to withstand rain, mud and other weather-related obstacles, farmers are better able to access markets and sell their produce at competitive prices. However, it is important to address all these constraints for ensuring that farmers are able to efficiently and effectively market their pea crops and to support the growth and development of the agricultural sector more broadly.
6 Policy implications
Among all the agricultural output marketing channels in the present study area, the producer-to-consumer channel is regarded as the most efficient. But this channel is not be preferred by farmers due to the lower quantity of produce sold as compared to other channels. Therefore, farmers need to be informed about the benefits of selling their produce through this channel such as higher prices, better quality control and reduced transaction costs. This can be done through various means such as workshops, training programs and awareness campaigns. Whereas, adequate infrastructure such as transportation, storage and processing facilities, needs to be developed to support the efficient channel. This will enable farmers to access markets that are farther away from their farms and also allow for value addition and quality control of their produce. However, governments can provide price support to farmers who sell their produce through the producer-to-consumer channel. This can be in the form of subsidies, direct payments and can help offset the higher costs associated with this channel. Moreover, establishing farmers markets and online marketplaces can facilitate the connection between farmers and consumers. Further, the regulatory environment needs to be conducive to the producer-to-consumer channel with clear rules and regulations that enable farmers to sell their produce directly to consumers. This may require changes to existing regulations as well as the development of new ones to support this channel. In the present study, the retailer market margin was highest when they receive pea produce through local trader channel. Thus, higher retailer margins can lead to higher prices for consumers which may affect demand for the produce. However, if the cost of marketing and distribution is too high then it may limit the ability of small-scale farmers to participate in the market which leads to an inequitable distribution of benefits across the supply chain. Therefore, it is important for policymakers and market participants to consider the overall impact of marketing channels on the economic viability of small-scale farmers and the affordability of produce for consumers, while also ensuring that the costs and benefits are fairly distributed across the supply chain.
The study further stressed upon that, it is important for governments, non-governmental organizations and private sector actors to invest in providing market consultancy services to farmers, in order to support their efforts to improve their agricultural output marketing channels. Whereas, to address the other constraints, governments and related stakeholders in the agricultural sector may consider implementing those initiatives that promote greater transparency, infrastructure and competition in agricultural markets. Moreover, implementing policies such as price supporting systems, market information systems, regulations to limit commission charges and other fees can promote greater transparency and competition in agricultural markets. Additionally, improving access to alternative marketing channels such as direct sales and cooperative marketing could provide farmers with more choices for selling their pea crop and reduce their reliance on middlemen and traders who charge high commission fees. It is also suggested that building and improving roads play significant role in increasing the availability of public and private transportation providers that offer affordable and reliable services to farmers. Moreover, improving communication and coordination between farmers and transportation providers could help to ensure that transportation services are available when and where they are needed. However, to address the constraint such as lack of all-weather roads, the governments and other related stakeholders may consider investing in road infrastructure and maintenance programs that are specifically designed to meet the needs of farmers. This can be done by building all-weather roads as well as providing ongoing maintenance and repair services to ensure that roads remain passable throughout the year. Moreover, to promote greater transparency and fairness in agricultural markets, the significant measures such as regulations to prevent price manipulation and other exploitative practices, initiatives to improve communication between farmers and intermediaries can be implemented. The study emphasis that providing training and support to farmers can improve their bargaining power and negotiating skills which can also help to mitigate the impact of exploitative practices. By implementing these policy implications, governments and other stakeholders can help to increase farmers choice for the efficient channel in agricultural output marketing.
Data availability statement
The raw data supporting the conclusions of this article will be made available by the authors, without undue reservation.
Ethics statement
Ethical review and approval was not required for the study on human participants in accordance with the local legislation and institutional requirements. Written informed consent from the participants was not required to participate in this study in accordance with the national legislation and the institutional requirements.
Author contributions
PT: Conceptualization, Data curation, Formal analysis, Investigation, Methodology, Software, Validation, Writing—original draft, Writing—review & editing. PM: Conceptualization, Methodology, Supervision, Validation, Visualization, Writing—review & editing. CD: Validation, Visualization, Writing—review & editing. PS: Validation, Visualization, Writing—review & editing. KS: Conceptualization, Validation, Visualization, Writing—review & editing. SY: Conceptualization, Validation, Visualization, Writing—review & editing. PL: Methodology, Conceptualization, Formal analysis, Validation, Visualization, Writing—review & editing. YR: Methodology, Conceptualization, Validation, Visualization, Writing—review & editing. PK: Conceptualization, Validation, Visualization, Writing—review & editing. PM: Methodology, Conceptualization, Validation, Visualization, Writing—review & editing.
Funding
The author(s) declare that no financial support was received for the research, authorship, and/or publication of this article.
Acknowledgments
The authors are grateful to the Head of the Department of Business Management, Dr Y.S. Parmar, University of Horticulture and Forestry, Solan (HP), India, for providing the necessary facilities during the study. Authors are also thankful to the farmers, officials of the Department of Agriculture Horticulture, agricultural produce marketing committee and government of Himachal Pradesh, India who have provided valuable information. We are also thankful to reviewers for their valuable suggestions and comments on the overall improvement of the manuscript.
Conflict of interest
The authors declare that the research was conducted in the absence of any commercial or financial relationships that could be construed as a potential conflict of interest.
Publisher's note
All claims expressed in this article are solely those of the authors and do not necessarily represent those of their affiliated organizations, or those of the publisher, the editors and the reviewers. Any product that may be evaluated in this article, or claim that may be made by its manufacturer, is not guaranteed or endorsed by the publisher.
References
Abankwah, V., Aidoo, R., and Tweneboah-Koduah, B. (2010). Margins and economic viability of fresh coconut marketing in the kumasi metropolis of Ghana. J. Dev. Agric. Econ. 2, 432–440.
Abdulai, J., Nimoh, F., Darko-Koomson, S., and Kassoh, K. F. S. (2017). Performance of vegetable production and marketing in peri-Urban Kumasi, Ghana. J. Agri. Sci. 9, 202–218. doi: 10.5539/jas.v9n3p202
Acharya, S. S. (1988). Agricultural Production, Marketing and Price Policy in India. Delhi: Mittal Publications.
Acharya, S. S. (2016). Policy analysis-what and how: A case of agricultural price and marketing policies in India. Indian J. Agri. Mark. 30, 26–47. doi: 10.5958/0974-0279.2017.00017.9
Acharya, S. S., and Agarwal, N. L. (2016). Agricultural Marketing in India. New Delhi: Oxford and IBH Publishing Corporation Private Limited.
Adanacioglu, H. (2017). Factors affecting farmers' decisions to participate in direct marketing: A case study of cherry growers in the Kemalpasa District of Izmir, Turkey. Renewable Agri. Food Syst. 32, 291–305. doi: 10.1017/S1742170516000193
Akrong, R., Mbogoh, S. G., and Irungu, P. (2021). What factors influence access to and the level of participation in high value mango markets by smallholder farmers in Ghana? Heliyon 7, e06543. doi: 10.1016/j.heliyon.2021.e06543
Alam, K. (2015). Farmer's adaptation to water scarcity in drought-prone environments: a case study of Rajshahi District, Bangladesh. Agri. Water Manage. 148, 196–206. doi: 10.1016/j.agwat.2014.10.011
Aliyi, I., Faris, A., Ayele, A., Oljirra, A., and Bayessa, M. (2021). Profitability and market performance of smallholder vegetable production: evidence from Ethiopia. Heliyon 7, e08008. doi: 10.1016/j.heliyon.2021.e08008
Anh, H. N., and Bokelmann, W. (2019). Determinants of smallholders' market preferences: The case of sustainable certified coffee farmers in Vietnam. Sustainability 11, 2897. doi: 10.3390/su11102897
Argade, A., and Laha, A. K. (2018). Marketplace options in an emerging economy local food marketing system: Producer's choices, choice determinants and requirements. In: Working Paper No. 2018-01-01. Ahmedabad, India: Indian Institute of Management.
Asante, A. C., and Weible, D. (2020). Is there hope for domestically produced poultry meat? A choice experiment of consumers in Ghana. Agribusiness 36, 281–298. doi: 10.1002/agr.21626
Baba, S. H., Wani, M. H., Wani, S. A., and Yousuf, S. (2010). Marketed surplus and price spread of vegetables in Kashmir valley. Agri. Econ. Res. Rev. 23, 115–128. Available online at: https://citeseerx.ist.psu.edu/documentrepid=rep&type=pdf&doi=cc416041f1f148307270088a6f7ed02c76ed3ef4
Bala, B., Sharma, N., and Sharma, R. K. (2011). Cost and return structure for the promising enterprise of off-season vegetables in Himachal Pradesh. Agri. Econ. Res. Rev. 24, 141–148.
Bardhan, D., Sharma, M. L., and Saxena, R. (2012). Market participation behaviour of smallholder dairy farmers in Uttarakhand: a disaggregated analysis. Agri. Econ. Res. Rev. 25, 243–254.
Barrett, C. B. (2008). Smallholder market participation: concepts and evidence from eastern and southern Africa. Food Policy 33, 299–317. doi: 10.1016/j.foodpol.2007.10.005
Barrett, C. B., Bachke, M. E., Bellemare, M. F., Michelson, H. C., Narayanan, S., and Walker, T. F. (2012). Smallholder participation in contract farming: comparative evidence from five countries. World Dev. 40, 715–730. doi: 10.1016/j.worlddev.2011.09.006
Blandon, J., Henson, S., and Islam, T. (2009). Marketing preferences of small-scale farmers in the context of new agrifood systems: a stated choice model. Agribusiness 25, 251–267. doi: 10.1002/agr.20195
Bukar, U., Mohammed, D., Wakawa, R., Shettima, B. G., and Muhammad, S. T. (2015). Analysis of market structure, conduct and performance for pepper in Borno state, Nigeria: a review. J. Agri. Econ. Environ. Soc. Sci. 1, 181–190.
Chand, K., Kumar, S., Suresh, A., and Dastagiri, M. B. (2020). Marketing efficiency of vegetables in developing economies: evidences for critical intervention from Rajasthan, India. Indian J. Agri. Sci. 90, 55–63. doi: 10.56093/ijas.v90i8.105908
Chand, K., Suresh, A., Dastagiri, M. B., Kumar, S., and Mandal, S. (2021). Fruit marketing, its efficiency and supply chain constraints in India: a case study. Indian J. Agri. Sci 91, 1146–1150. doi: 10.56093/ijas.v91i8.115800
Chand, R, and Singh, J. (2016). Agricultural Marketing and Farmer Friendly Reforms Across Indian States and UTs. Study Report. New Delhi: National Institution for Transforming India.
Cheng, L., Jiang, S., Zhang, S., You, H., Zhang, J., Zhou, Z., et al. (2016). Consumers' behaviors and concerns on fresh vegetable purchase and safety in Beijing urban areas, China. Food Control 63, 101–109. doi: 10.1016/j.foodcont.2015.11.024
Chirwa, E. W. (2009). “Determinants of marketing Channels among Smallholder maize farmers in Malawi,” in Working Paper No. 2009/03. Malawi: Chancellor College, University of Malawi.
Chiv, R., Nie, F., Wu, S., and Tum, S. (2020). Analysis of Factors Influencing Marketing channel choices by smallholder farmers: a Case Study of paddy product in wet and dry season of Prey Veng Province, Cambodia. J. Sust. Dev. 13, 15–34. doi: 10.5539/jsd.v13n4p15
Chopde, K. D. (2019). Price spread for capsicum in Akola district of Maharashtra. J. Econ. Manage. Trade 25, 1–7. doi: 10.9734/jemt/2019/v25i430202
Chung, C., Boyer, T., and Han, S. (2011). How many choice sets and alternatives are optimal? Consistency in choice experiments. Agribusiness 27, 114–125. doi: 10.1002/agr.20252
Coughlan, A. T. E., Stern, A. L., and Ansary, A. I. (2001). Marketing Channels. New Jersey: Prentice Hall.
Dastagiri, M. B., Chand, R., Immanuelraj, T. K., Hanumanthaiah, C. V., Paramsivam, P., Sidhu, R. S., et al. (2013). Indian vegetables: Production trends, marketing efficiency and export competitiveness. Am. J. Agri. Forest. 1, 1–11. doi: 10.11648/j.ajaf.20130101.11
Dawit, S. H. (2016). Structure and performance of vegetable marketing in east Shoa zone, Oromia region, Ethiopia. J. Market. Consum. Res. 2016, 26.
De, S. K., and Rahaman, S. M. W. (2014). Economics of production and marketing of cabbage in Bankura district of West Bengal. J. Crop and Weed 10, 101–106.
Delong, K. L., Jensen, K. L., Griffith, A. P., and McLeod, E. (2019). Beef cattle farmer's marketing preferences for selling local beef. Agribusiness 35, 343–357. doi: 10.1002/agr.21579
Deressa, T. T., Hassan, R. M., Ringler, C., Alemu, T., and Yesuf, M. (2009). Determinants of farmer's choice of adaptation methods to climate change in the Nile Basin of Ethiopia. Global Environmental Change 19, 248–255. doi: 10.1016/j.gloenvcha.2009.01.002
Dessie, A. B., Koye, T. D., Koye, A. D., and Abltew, A. A. (2019). Analysis of red pepper marketing: evidence from northwest Ethiopia. J. Econ. Struct. 8, 1–14. doi: 10.1186/s40008-019-0156-0
Devi, N., Raina, K. K., and Sharma, R. (2020). Constraints perceived by the farmers of Himachal Pradesh in organic farming. Econ. Affairs 65, 213–218. doi: 10.46852/0424-2513.2.2020.12
Devkota, R., and Sharma, K. D. (2014). Conduct and performance of vegetable marketing system in Kangra district of Himachal Pradesh in India. Int. Agri. Innovat. Res. 3, 737–744. Available online at: https://www.ijair.org/administrator/components/com_jresearch/files/publications/IJAIR_1021_Final.pdf
Dhaka, J. M., and Poonia, B. S. (2010). Identification of constraints encountered by the farmers in production and marketing of vegetables in Bundi district of Rajasthan. Indian J. Agri. Mark. 24, 20–25.
Donkor, E., Onakuse, S., Boguen, J., and de los Ríos Carmenado, I. (2018). Determinants of farmer participation in direct marketing channels: A case study for cassava in the Oyo State of Nigeria. Spanish J. Agri. Res. 16, 1–3. doi: 10.5424/sjar/2018162-12076
Edoge, E. D. (2014). Determinants of choice of distribution channels by fish farmers in Ughelli north local government area of Delta state, Nigeria. Asian J. Agri. Food Sci. 2, 409–419.
Espinosa-Goded, M., Rodriguez-Entrena, M., and Salazar-Ordóñez, M. (2021). A straightforward diagnostic tool to identify attribute non-attendance in discrete choice experiments. Econ. Anal. Policy 71, 211–226. doi: 10.1016/j.eap.2021.04.012
Fan, Q., and Garcia, V. B. S. (2018). Information access and smallholder farmer's market participation in Peru. J. Agri. Econ. 69, 476–494. doi: 10.1111/1477-9552.12243
Garrett, H. E., and Woodworth, R. S. (1969). Statistics in Psychology and Education. Bombay, Vakils: Feffer and Simons Pvt Ltd.
Gebrehiwot, N. T., Azadi, H., Taheri, F., and Van, P. S. (2018). How participation in vegetables market affects livelihoods: empirical evidence from Northern Ethiopia. J. Int. Food Agribusin. Mark. 30, 107–131. doi: 10.1080/08974438.2017.1402725
Gelaw, F., Speelman, S., and Van, H. G. (2016). Farmer's marketing preferences in local coffee markets: Evidence from a choice experiment in Ethiopia. Food Policy 61, 92–102. doi: 10.1016/j.foodpol.2016.02.006
Geoffrey, S. K., Hillary, B. K., Jonah, K. K., and Timothy, O. O. (2015). Factors influencing the choice of marketing outlets among small-scale pineapple farmers in Kericho county, Kenya. Int. J. Regional Dev.2, 1–11. doi: 10.5296/ijrd.v2i2.6237
Ghorbani, M. (2008). The efficiency of saffron marketing channel in Iran. World Appl. Sci. J. 4, 523–527.
Goncalves, T., Lourenço-Gomes, L., and Pinto, L. M. C. (2022). The role of attribute non-attendance on consumer decision-making: theoretical insights and empirical evidence. Econ. Analy. Policy. 76, 788-805. doi: 10.1016/j.eap.2022.09.017
Hailegiorgis, D. S., and Hagos, F. (2016). Structure and performance of vegetable marketing in East Shoa zone, Oromia Region, Ethiopia. J. Mark. Cons. Res.26, 7–16.
Harrizon, K., Benjamin, M. K., Lawrence, K. K., Patrick, K. R., and Anthony. (2016). Determinants of tea marketing channel choice and sales intensity among smallholder farmers in Kericho district, Kenya. J. Econ. Sust. Dev. 7, 105–114.
Hisali, E., Birungi, P., and Buyinza, F. (2011). Adaptation to climate change in Uganda: Evidence from micro level data. Global Environm. Change 21, 1245–1261. doi: 10.1016/j.gloenvcha.2011.07.005
Ihli, H., Seegers, R., Winter, E., Chiputwa, B., and Gassner, A. (2021). Preferences for tree fruit market attributes among smallholder farmers in eastern Rwanda. Agri.Econ. 2021, 1–17. doi: 10.1111/agec.12673
Jalalzadeh, M., Zamanabadi, S. H. N., and Kalantari, K. (2014). Investigating the marketing channels of agricultural crops in west Azerbaijan province, Iran. Int. J.Mark. Stud 6, 148–154. doi: 10.5539/ijms.v6n3p148
Jari, B., and Fraser, G. C. G. (2009). An analysis of institutional and technical factors influencing agricultural marketing amongst smallholder farmers in the Katriver valley, Eastern Cape Province, South Africa. Rhodes University, South Africa. Afr. J. Agric. Res. 4, 1129–1137.
JICA (2021). The Preparatory Survey of Himachal Pradesh Crop Diversification Promotion Project Phase-II (HPCDP-II) in Republic of India. Washington, D.C.: Japan International Cooperation Agency (JICA).
Kala, S. (2020). An economic analysis of marketing and constraints for green chilli in Jaipur district of Rajasthan. Econ. Affairs 65, 33–52. doi: 10.46852/0424-2513.4.2020.19
Karthick, V., Alagumani, T., and Amarnath, J. S. (2013). Resource use efficiency and technical efficiency of turmeric production in Tamil Nadu: a stochastic frontier approach. Agri. Econ. Res. Rev. 26, 109–114.
Kenjit, T., Singh, Y. C., and Singh, O. K. (2021). A study on production and marketing constraints of french bean (Phaseolus vulgaris L.) growers in Bishnupur district of Manipur. Asian J. Agricultural Extens. Econ. Sociol. 39, 37–41. doi: 10.9734/ajaees/2021/v39i930639
Krafft, M., Goetz, O., Mantr Barrett, M., Sotgiu, F., and Tillmanns, S. (2015). The evolution of marketing channel research domains and methodologies: An integrative review and future directions. Journal of Retailing 91, 569–585. doi: 10.1016/j.jretai.2015.05.001
Kumar, A., Sumit Yadav, M. K., and Rohila, A. K. (2019). Constraints faced by the farmers in production and marketing of vegetables in Haryana. Indian J. Agri. Sci. 89, 153–160. doi: 10.56093/ijas.v89i1.86198
Kumar, G. B., Pramanik, S. C., and Shakila, N. (2004). Economics of Production and Marketing of vegetables in the Andaman andNicobar Islands. Indian J. Agri. Sci. 18, 16–22.
Lapar, M. L., Holloway, G., and Ehui, S. (2003). Policy options promoting market participation among smallholder livestock producers: a case study from the Philippines. Food Policy 28, 187–211. doi: 10.1016/S0306-9192(03)00017-4
Lee, B., Liu, J. Y., and Chang, H. H. (2020). The choice of marketing channel and farm profitability: Empirical evidence from small farmers. Agribusiness 36, 402–421. doi: 10.1002/agr.21640
Lenne, J. M., and Ward, A. F. (2010). Improving the efficiency of domestic vegetable marketing systems in east Africa constraints and opportunities. Outlook Agric. 34, 225–232.
LeRoux, M. N., Schmit, T. M., Roth, M., and Streeter, D. H. (2010). Evaluating marketing channel options for small-scale fruit and vegetable producers. Renew. Agri. Food Syst. 25, 16–23. doi: 10.1017/S1742170509990275
Louviere, J. J., Hensher, D. A., and Swait, J. (2000). Stated Choice Methods: Analysis and Application. Cambridge: Cambridge University Press, 1–30.
Ma, W., and Abdulai, A. (2016). Linking farmers to markets: determinants and impacts of marketing contracts in China. China Agri. Econ. Rev. 8, 1–21. doi: 10.1108/CAER-04-2015-0035
Mafukata, M. A. (2015). Factors having the most significance on the choice and selection of marketing channels amongst communal cattle farmers in Vhembe District, Limpopo Province. J. Human Ecol. 49, 77–87. doi: 10.1080/09709274.2015.11906826
Magesa, M. M., Michael, K., and Ko, J. (2014). Access to agricultural market information by rural farmers in Tanzania. Int. J. Inform. Commun. Technol. Res. 4, 264–273.
Maina, C. M. (2015). Effect of transaction costs on choice of mango marketing channel and income of small-scale farmers in Makueni County, Kenya. IOSR J. Agri. Vet. Sci. 8, 54–62.
Makhura, M., Kirsten, J., and Delgado, C. (2001). “Transaction costs and smallholder participation in the maize market in the Northern Province of South Africa,” in Proceedings of the Seventh Eastern and Southern Africa Regional Conference. Pretoria: IEEE.
Manjunath, V., and Girish, M. R. (2016). An analysis of private vegetable market vis-à-vis public vegetable market in Karnataka-a study in Kolar district of Karnataka state. Agri. Update 11, 173–177. doi: 10.15740/HAS/AU/11.2/173-177
Mariano, M. J., Villano, R., and Fleming, E. (2012). Factors influencing farmer's adoption of modern rice technologies and good management practices in the Philippines. Agric. Syst. 110, 41–53. doi: 10.1016/j.agsy.2012.03.010
Mariel, P., Khan, M. A., and Meyerhoff, J. (2022). Valuing individuals' preferences for air quality improvement: evidence from a discrete choice experiment in South Delhi. Econ. Anal. Policy 74, 432–447. doi: 10.1016/j.eap.2022.03.006
Marine, S. C., Martin, D. A., Adalja, A., Mathew, S., and Everts, K. L. (2016). Effect of market channel, farm scale, and years in production on mid-Atlantic vegetable producer's knowledge and implementation of Good Agricultural Practices. Food Control 59, 128–138. doi: 10.1016/j.foodcont.2015.05.024
Martey, E. K., Annin Wiredu, A. N., and Attoh, C. (2012). Does access to market information determine the choice of marketing channel among smallholder yam farmers in the Brong Ahafo region of Ghana? A Multinomial logit regression analysis. J. Econ. Sust. Dev. 3, 18–28.
Maspaitella, M., Garnevska, E., Siddique, M. I., and Shadbolt, N. (2018). Towards high value markets: a case study of smallholder vegetable farmers in Indonesia. Int. Food Agribus. Manag. Rev. 21, 73–88. doi: 10.22434/IFAMR2017.0011
Mgale, Y. J., and Yunxian, Y. (2020). Marketing efficiency and determinants of marketing channel choice by rice farmers in rural Tanzania: evidence from Mbeya region, Tanzania. Aust. J. Agri. Res. Econ. 64, 1239–1259. doi: 10.1111/1467-8489.12380
Mishra, S., Singh, R., and Singh, O. P. (2014). Economic analysis of marketing of major vegetables in varanasi district of Uttar Pradesh, India. Econ. Affairs 59, 649–652. doi: 10.5958/0976-4666.2014.00038.2
Mmasa, J. J., Elibariki, E. M., and Melchion, M. (2013). Performance of various marketing channels for sweet potato value added products. J. Agric. Econ. Dev. 2, 65–76.
Mmbando, F. E., Wale, E. Z., and Baiyegunhi, L. J. (2015). Determinants of smallholder farmer's participation in maize and pigeon pea markets in Tanzania. Agrekon 54, 96–119. doi: 10.1080/03031853.2014.974630
MOAFW (2022). Agricultural Statistics at a Glance 2022. Government of India Ministry of Agriculture and Farmers Welfare Department of Agriculture and Farmers Welfare Economics and Statistics Division.
Mondal, M., Ali, H., and Bera, B. K. (2020). A study on economics of marketing of brinjal (Solanum melongena L.) in Nadia district of West Bengal. Curr. J. Appl. Sci. Techn. 39, 89–95. doi: 10.9734/cjast/2020/v39i4831203
Mtimet, N., and Albisu, L. M. (2006). Spanish wine consumer behavior: A choice experiment approach. Agribusiness 22, 343–362. doi: 10.1002/agr.20090
Mutayoba, V., and Ngaruko, D. (2015). Market performance and farmers' choice of marketing channels of high value crops in Tanzania. Int. J. Econ. Commerce Manage. Kingdom 3, 276–289.
Mzoughi, N. (2011). Farmer's adoption of integrated crop protection and organic farming: Do moral and social concerns matter. Ecol. Econ. 70, 1536–1545. doi: 10.1016/j.ecolecon.2011.03.016
Narayanan, S. (2014). Profits from participation in high value agriculture: evidence of heterogeneous benefits in contract farming schemes in Southern India. Food Policy 44, 142–157. doi: 10.1016/j.foodpol.2013.10.010
Naveen, B., Jayaram, M., Swamy, P. D., Ramesh, G., and Raghavendra, D. (2015). Marketing channels and price spread of banana in Chikkaballapur district of Karnataka. Int. Res. J. Agricult. Econ. Statis. 6, 18–22. doi: 10.15740/HAS/IRJAES/6.1/18-22
Negasi, M. Y. (2015). Marketing system analysis of vegetables and fruits in Amhara regional State: survey evidence from Raya Kobo and Harbu Woredas. Ethiop. J. Econ. 24, 1–41.
Ntimbaa, G. J., and Akyoob, A. M. (2017). Factors influencing choice decision for marketing channels by coffee farmers in Karagwe district, Tanzania. Global J. Biol. Agri. Health Sci. 6, 1–10. doi: 10.24105/gjbahs.6.2.1701
Nxumalo, K. K. S., Oduniy, O. S., Antwi, M. A., and Tekana, S. S. (2019). Determinants of market channel choice utilised by maize and sunflower farmers in the North West province, South Africa. Cogent Social Sci. 5, 1678451. doi: 10.1080/23311886.2019.1678451
Olutumise, A. I. (2020). Transportation system and output market participation nexus among yam producers in Southwest region of Nigeria. Econ. Eng. Agricu. Rural Dev. 20, 365–379.
Olutumise, A. I. (2022). Determinants of market participation and preference for production of pepper (Capsicum spp.) among farmers in southwest, Nigeria. Heliyon 8, e10585. doi: 10.1016/j.heliyon.2022.e10585
Osondu, C. K., Nwadike, F. C., Ijioma, J. C., Udah, S. C., and Ugboaja, C. I. (2014). Marketing performance of salad vegetables: the case of cabbage marketing in Abia state, Nigeria. Int. J. Agri. Sci. Res. Techn. Extens. Educ. Syst. 4, 151–162.
Owusu, O., and Iscan, T. B. (2020). Drivers of farm commercialization in Nigeria and Tanzania. Agric. Econ. 52, 265–299. doi: 10.1111/agec.12618
Ozor, M. U., and Nwankwo, T. N. (2018). A comparative analysis of the market structure of two varieties of maize (Zea mays) in South-east, Nigeria. Int. J. Agri. Forest. 8, 150–154.
Panda, R. K., and Sreekumar. (2012). Marketing channel choice and marketing efficiency assessment in agribusiness. J. Int. Food Agribusiness Mark. 24, 213–230. doi: 10.1080/08974438.2012.691812
Park, T. (2015). Direct marketing and the structure of farm sales: An unconditional quantile regression approach. J. Agri. Res. Econ. 40, 266–284.
Park, T., Mishra, A., and Wozniak, S. (2014). Do farm operators benefit from direct to consumer marketing strategies? Agri. Econ. 45, 213–224. doi: 10.1111/agec.12042
Park, T., Paudel, K., and Sene, S. (2018). Sales impacts of direct marketing choices: treatment effects with multinomial selectivity. Eur. Rev. Agri. Econ. 45, 433–453. doi: 10.1093/erae/jbx038
Pham, T. T., Theuvsen, L., and Otter, V. (2019). Determinants of smallholder farmer's marketing channel choice: evidence from the Vietnamese rice sector. Asian Econ. J. 33, 281–300. doi: 10.1111/asej.12187
Pingali, P., Khwaja, Y., and Meijer, M. (2005). Commercializing small farms: Reducing transaction costs. In: The Future of Small Farms: Proceedings of a Research Workshop. Washington, DC: International Food Policy Research Institute. (2005), 61–74.
Routroy, S., and Behera, A. (2017). Agriculture supply chain: a systematic review of literature and implications for future research. J. Agribusiness Dev. Emerg. Econ. 7, 275–302. doi: 10.1108/JADEE-06-2016-0039
Siddique, M. I., Garnevska, E., and Marr, N. E. (2018). Factors affecting marketing channel choice decisions of smallholder Citrus growers. J. Agribusiness Dev. Emerg. Econ. 8, 426–453. doi: 10.1108/JADEE-03-2016-0014
Sidhu, R. S., Kumar, S., Vatta, K., and Singh, P. (2010). Supply chain analysis of onion and cauliflower in Punjab. Agri. Econ. Res. Rev. 23, 445–454.
Sidhu, R. S., Sidhu, M. S., and Singh, J. M. (2011). Marketing efficiency of green peas under different supply chains in Punjab. Agri. Econ. Res. Rev. 24, 267–274.
Singh, N., Sharma, R., and Kayastha, R. (2020). Economic analysis of pea (Pisum sativum) in Himachal Pradesh. Econ. Affairs 65, 191–195. doi: 10.46852/0424-2513.2.2020.9
Singla, R., Chahal, S. S., and Kataria, P. (2006). Economics of Production of Green Peas (Pisum sativum L.) in Punjab. Agri. Econ. Res. Rev. 19, 237–250.
Soe, W. P. P., Moritaka, M., and Fukuda, S. (2015). An analysis of the factors influencing marketing channel choice by paddy rice farmers in Myanmar. J. Faculty Agri. Kyushu Univers. 60, 535–542. doi: 10.5109/1543425
Swaminathan, M. S. (2002). “Food groups and balance diet: recommended dietary allowances,” in Essentials of Food and Nutrition — An Advanced Textbook. Bangalore: The Bangalore Printing and Publishing Co. Ltd.
Tafesse, A., Megerssa, G. R., and Gebeyehu, B. (2020). Determinants of agricultural commercialization in Offa district, Ethiopia. Cogent Food Agri. 6, 1–4. doi: 10.1080/23311932.2020.1816253
Thakur, P., Mehta, P., Guleria, A., Divyanshu, S. P., and Sharma, P. (2022a). Farmers' choice for output marketing channels of cauliflower in himachal pradesh, india: a multinomial logit model analysis. Econ. Affairs 67, 731–738. doi: 10.46852/0424-2513.5.2022.6
Thakur, P., Mehta, P., Guleria, A., Divyanshu, S. P., and Sharma, P. (2023). Study on marketing performance and constraints of pea (pisum sativum) output in high hills wet temperate zone of Himachal Pradesh, India. Indian Journal of Ecology 50, 129–135.
Thakur, P., Mehta, P., Sharma, P., and Divyanshu. (2022b). Determinants of farmers' adoption behaviour towards farm business management practices for vegetable farming in mid-hills of Himachal Pradesh, India. Economic Affairs 67, 117–122. doi: 10.46852/0424-2513.2.2022.19
Train, K. E. (2009). Discrete Choice Methods With Simulation. 2nd ed. Cambridge: Cambridge University Press.
Tsourgiannis, L., Eddison, J., and Warren, M. (2008). Factors affecting the marketing channel choice of sheep and goat farmers in the region of east Macedonia in Greece regarding the distribution of their milk production. Small Ruminant Res. 79, 87–97. doi: 10.1016/j.smallrumres.2008.07.005
Wiersinga, R. C., Wijk van, M. S., and Luyen, C.H. (2007). Marketing channel choice and marketing timing of peri-urban vegetable growers in Vietnam. Acta Hortic. 762, 373–380. doi: 10.17660/ActaHortic.2007.762.36
Xaba, G. B., and Masuku, M. B. (2013). Factors affecting the choice of marketing channel by vegetable farmers in Swaziland. Sust. Agri. Res. 2, 2016–2087. doi: 10.5539/sar.v2n1p112
Yaregal, T. (2018). Structure, conduct and performance of potato marketing in Sekela district west Gojjam zone, amhara region, Ethiopia. Int. J. Res. Stud. Agric. Sci. 4, 2454–6224.
Keywords: marketing channels, performance, efficiency, factors, choice, multinomial logistic regression, marketing constraints
Citation: Thakur P, Mehta P, Devi C, Sharma P, Singh KK, Yadav S, Lal P, Raghav YS, Kapoor P and Mishra P (2023) Marketing performance and factors influencing farmers choice for agricultural output marketing channels: the case of garden pea (Pisum sativum) in India. Front. Sustain. Food Syst. 7:1270121. doi: 10.3389/fsufs.2023.1270121
Received: 31 July 2023; Accepted: 20 November 2023;
Published: 08 December 2023.
Edited by:
Monika Thakur, Amity University, IndiaReviewed by:
Therese Mwatitha Gondwe, Alliance Bioversity International and CIAT, KenyaNilhari Neupane, International Water Management Institute, Nepal
Copyright © 2023 Thakur, Mehta, Devi, Sharma, Singh, Yadav, Lal, Raghav, Kapoor and Mishra. This is an open-access article distributed under the terms of the Creative Commons Attribution License (CC BY). The use, distribution or reproduction in other forums is permitted, provided the original author(s) and the copyright owner(s) are credited and that the original publication in this journal is cited, in accordance with accepted academic practice. No use, distribution or reproduction is permitted which does not comply with these terms.
*Correspondence: Pankaj Thakur, pankajthakur.abm@gmail.com; Priyanka Lal, priyanka.lal6@gmail.com