- 1National Center for Applied Mathematics in Chongqing, Chongqing Normal University, Chongqing, China
- 2Department of Mathematics, Hangzhou Dianzi University, Hangzhou, China
Accurate recovery of Internet traffic data can mitigate the adverse impact of incomplete data on network task processes. In this study, we propose a low-rank recovery model for incomplete Internet traffic data with a fourth-order tensor structure, incorporating spatio-temporal regularization while avoiding the use of d-th order T-SVD. Based on d-th order tensor product, we first establish the equivalence between d-th order tensor nuclear norm and the minimum sum of the squared Frobenius norms of two factor tensors under the unitary transformation domain. This equivalence allows us to leave aside the d-th order T-SVD, significantly reducing the computational complexity of solving the problem. In addition, we integrate the alternating direction method of multipliers (ADMM) to design an efficient and stable algorithm for precise model solving. Finally, we validate the proposed approach by simulating scenarios with random and structured missing data on two real-world Internet traffic datasets. Experimental results demonstrate that our method exhibits significant advantages in data recovery performance compared to existing methods.
1 Introduction
Internet traffic data, characterized by distinct spatio-temporal attributes, are crucial for documenting information transmission volumes over specified time periods. It plays a vital role in network design and management [1–3]. However, in practice, uncontrollable factors often result in incomplete or corrupted traffic data, hindering its effective use. Therefore, accurately recovering original data from incomplete traffic data is of significant importance.
Since Zhang et al. [4] introduced the sparse regularized matrix factorization (SRMF) model for data recovery, while incorporating the unique characteristics of Internet traffic data, research in the field of Internet traffic data recovery has continued to advance. As higher-order generalizations of matrices, tensors are more effective at capturing the structural characteristics within the data. Consequently, many researchers have proposed various effective methods for Internet traffic data recovery based on different tensor decomposition techniques, such as the CANDECOMP/PARAFAC (CP) decomposition [5, 6], the tensor-train (TT) decomposition [7], and the third-order T-product factorization [8]. Given that low-rankness is a typical characteristic of incomplete data in scenarios with high levels of missing samples, low-rank modeling serves as another effective strategy beyond tensor decomposition. Candès et al. [9] were the pioneers in establishing a low-rank matrix data recovery model. To address its inherent non-convexity and NP hardness, they employed the nuclear norm of a matrix to convexly relax the model, enabling an effective solution. Unlike the definition of matrix rank, which is well-defined, the definition of tensor rank relies on specific decomposition method.
In 2014, based on the tensor singular value decomposition (T-SVD), Zhang et al. [10] proposed third-order tensor nuclear norm (TNN) and employed it to convexly relax the tubal rank of tensors, and they established a model for image restoration. On this basis, Li et al. [11] developed the SRTNN model for the recovery of Internet traffic data by incorporating spatial-temporal regularization with the nuclear norm of third-order tensors, which demonstrates significant advantages in terms of data recovery effectiveness. Meanwhile, to mitigate the computational burden associated with SVD, He et al. [12] proposed the equivalence between the so-called transformed tubal nuclear norm for a third-order tensor and the minimum of the sum of two factor tensors' squared Frobenius norms under a general invertible linear transform.
Currently, the recovery of Internet traffic data primarily relies on model construction based on third-order tensors. While these studies have achieved certain advancements, the effectiveness in restoring data with structural missingness remains limited. Addressing this challenge requires data recovery models to more thoroughly account for the intrinsic structural characteristics of the data. In the case of Internet traffic data, considering its complexity and multidimensionality, it can be organized in the form of a fourth-order tensor (, where N signifies network nodes, T is the daily recording times, and D indicates the recording days) to capture the internal structural features of the data more comprehensively. Therefore, exploring a low-rank fourth-order tensor model for Internet traffic data recovery is essential.
Notably, Martin et al. [13] have extended the third order T-product to d-th order T-product, which is explained in a recursive way but for computational speed is implemented directly using the fast Fourier transform. Inspired by this, Qin et al. [14] have generalized the multiplication in the discrete Fourier transform (DFT) domain to tensor product in the domain of general invertible transforms and introduced the d-th order tensor nuclear norm. They employed the d-th order TNN to perform convex relaxation on the fourth order tensor low-rank model, establishing the HTNN method for recovering visual data. However, for solving optimization problems involving nuclear norms, the thresholding operator is often employed, which involves performing SVD on a large number of matrices during the process, significantly increasing the computational time [11, 14]. Hence, a natural question arises about how to build a low-rank recovery model for a d-th order tensor without the need for SVD computation.
In this study, by arranging the observed Internet traffic data as a tensor , we enable unitary transformations on mode-3 and mode-4 to fully integrate the internal data of these modes. Meanwhile, a spatio-temporal regularization term is applied to modes-1 and-2, aiming to preserve the periodicity and similarity of the recovered data as much as possible, thereby enhancing the accuracy of data recovery. We develop a new low-rank model for the recovery of fourth-order Internet traffic data as follows:
where ||·||⋆, L means the tensor nuclear norm under the unitary transform L, and and are consisted by flow data of consecutive days and time points, respectively. Through the equivalence relationship between the d-th order tensor nuclear norm and the minimum sum of two factor tensors' squared Frobenius norms within the unitary transformation domain and under the d-th order T-product which is first established by us, we reformulate this model into the following version without relying on d-th order T-SVD:
We will give a detail explanation about these two models in Section 3.
The rest of this study is organized as follows. Some notions and preliminaries are listed in Section 2. In Section 3, we establish a low-rank Internet traffic data recovery model without d-th order tensor SVD and solve it using the alternating direction method of multipliers (ADMM), proposing a computational framework for solving the model. In Section 4, we conduct a convergence analysis of Algorithm 1, ensuring that it converges to a stationary point. Numerical experiments are presented in Section 5, where we apply the proposed method to two real-world Internet traffic datasets and simulate potential structural missingness scenarios in practice. The experimental results demonstrate that our method exhibits significant advantages, both in terms of recovery accuracy and computational efficiency. Finally, we give the conclusion of this study in Section 6.
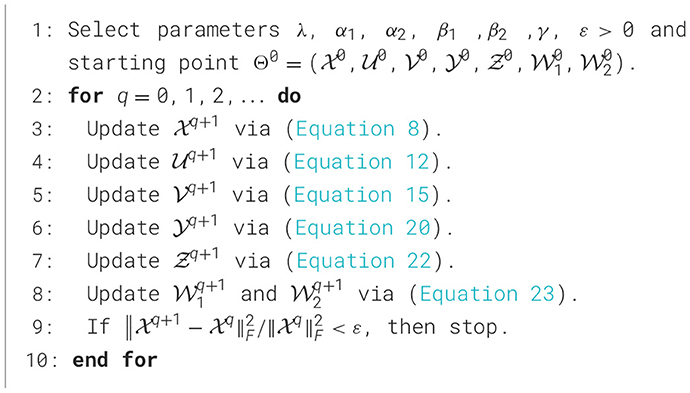
Algorithm 1. Tensor completion algorithm with d-th order tensor product decomposition and spatio-temporal regularization (SRdTPD).
2 Preliminary
In this study, the real number field and the complex number field are denoted by ℝ and ℂ, respectively. We use lowercase letters a, b, ⋯ to denote scalars, bold lowercase letters a, b, ⋯ to represent vectors, capital letters A, B, ⋯ to signify matrices, and calligraphic letters to denote tensors. For any positive integer n, we define the set [n]: = {1, 2, ..., n}. For A∈ℂm×n, AH denotes the conjugate transpose of A (when A is in the real number field, its transpose is A⊤), and its nuclear norm is denoted as , where σi is the i-th singular value of A. For a d-th order real tensor , its corresponding Frobenius norm is . The inner product between and is denoted as . Denote as a column vector formed by fixing all modes except for mode-l, and as the matrix slice along mode-p and mode-q. In particular, the matrix slice encompassing the first two modes is designated as , where j = (id−1)n3⋯nd−1+⋯+(i4−1)n3+i3, id∈[nd]. Denote
where J = n3⋯nd. Herein, elucidate the face-wise product for d-th order tensors.
Definition 1 (Face-wise product [14]). The face-wise product of two d-th order tensors and is the element of defined according to
For a given set of invertible transformation matrices denoted as , the linear transformation of a d-th order tensor under L is defined as , where the symbol × k represents the k-mode product of a tensor with a matrix defined in [15], and the inverse transformation of under L is denoted as . Thus, when the corresponding matrices of invertible linear transforms L are unitary matrices, we can obtain
Next, the clear definitions for the multiplication operation and related concepts of a d-th order tensor within the domain of general invertible transformations.
Definition 2 (d-th order T-product [14]). Let and . Then, the invertible linear transforms L based T-product is defined as
Definition 3 (d-th order tensor conjugate transpose [14]). The invertible linear transforms L based conjugate transpose of a tensor is the tensor , whose matrix slice encompassing the first two modes . We denote by the conjugate transpose of a tensor .
Definition 4 (d-th order TNN [14]). Let , the tensor nuclear norm of is defined as
where ρ > 0 is a positive constant determined by the invertible linear transforms L.
Remark 1. The constant ρ in the key definition and theorem arises when the corresponding matrices of invertible linear transforms L fulfill the given equation:
where ⊗ is the Kronecker product, I is the identity matrix, and n3n4⋯nd is its dimensions.
Based on Definition 4, we can draw the conclusion of the equivalence between d-th order tensor nuclear norm and the minimum of the sum of two factor tensors' squared Frobenius norms:
Theorem 1. If the corresponding matrices of invertible linear transforms L are unitary matrices, for , we have
Proof: By Definition 4, we have
where the proof for the second equality can be deduced from the proofs of Lemma 5.1 and Proposition 2.1 of [16], and the reason for the third equality holding is that are unitary matrices.
3 Model and algorithm
As mentioned in the introduction, Internet traffic data consist of network traffic records measured T times daily from N origin nodes to N destination nodes. Consequently, data collected over D consecutive days can be organized as a fourth-order tensor in ℝD×T×N×N (i.e., n1 = D, n2 = T, n3 = n4 = N), comprehensively capturing the spatio-temporal dynamics of the network traffic.
3.1 Design of model and transformation
Based on low-rank property of observed incomplete tensor , we construct a data recovery model for missing traffic data within the domain of unitary transformation (i.e., the corresponding matrices in L are unitary):
where α1, α2, and γ are positive parameters, is the Internet traffic tensor needed to be estimated, Ω is the index set corresponding to the observed entries of , and PΩ(·) is the linear operator that keeps known elements in Ω while setting the others to be zeros. Here, , where adjacent rows represent flow data measurements from consecutive days. And , where adjacent rows represent flow data measurements from consecutive time points. The regularization term is employed to ensure the boundedness of the sequence generated by the proposed algorithm. To better capture the periodicity of the data measured on each observation day and the similarity of the data obtained between adjacent observation time points within each day, we choose the temporal constraint matrix H = Toeplitz(0, 1, −1) of the size (n1−1) × n1 and K = Toeplitz(0, 1, −1) of size (n2−1) × n2, respectively, i.e.,
It is well-known that the Equation (Model) 3 requires the so-called SVD to compute the ||·||⋆, L minimization subproblem, which often takes much computing time for large-scale tensors. Therefore, with the employment of Theorem 1, we gainfully reformulate Equation (Model) 3 as the following tensor factorization version:
where . Clearly, such an optimization model no longer requires SVD to find its solution. Therefore, we call Equation (Model) 4 a SVD-free model. Moreover, to facilitate the solution of Equation (Model) 4, we introduce auxiliary variables . Thus, the Equation (Model) 4 is equivalently written as
3.2 Description of algorithm
We apply the alternating direction method of multipliers (ADMM) algorithm to solve Equation (Model) 5. The augmented Lagrangian function of Equation (Model) 5 is written as follows:
where and are the Lagrangian multipliers, and β1 and β2 are positive penalty parameters. Below, given the q-th iterate , we provide specific solutions for the updates of each variable.
▸ The -subproblem
We update by solving the following subproblem
The solution of Equation (Model) 7 is given by
▸ The -subproblem
We update by
Because the corresponding matrices in L are unitary, it holds that for j∈[n3n4],
Due to the separable structure of Equation 10 with respect to decision variables for j∈[n3n4], solving the Equation 9 is equivalent to solving
whose solution is given by
With the help of the obtained for j∈[n3n4], we can get by
▸ The -subproblem
We update by
Similarly to -subproblem, solving Equation 13 is equivalent to solving the following problem:
for every j∈[n3n4]. Clearly, the solution of Equation (Model) 14 is given by
for every j∈[n3n4]. Thus, can be obtained via
▸ The -subproblem
We update by
which is equivalent to solving
By the optimality condition of Equation (Model) 17, we have
which implies that the optimal solution of Equation (Model) 17 is
As a result, the optimal solution of Equation 16 is
where IMat1(·) is the inverse of Mat1(·).
▸ The -subproblem
We update by
Similarly to -subproblem, the optimal solution of Equation 21 is
where IMat2(·) is the inverse of Mat2(·).
▸ The and -subproblems
We update and by
Following the above analysis, the algorithmic framework for solving Equation (Model) 5 is presented at Algorithm 1.
Remark 2. In the and -subproblems, the inverses of two related matrices are computed directly. In the and -subproblems, we first utilize the special structure of H and K to perform Cholesky decomposition on the two matrices and , decomposing them into the product of two triangular matrices respectively, and then obtain their inverse matrices. This will significantly reduce the computational cost. In addition, considering that the inverses of the two related matrices remain constant in each round of solving the and -subproblems, we only need to compute the inverses once, and in the subsequent solving of the and -subproblems, we can simply call the inverse matrices directly.
4 Convergence analysis
In this section, we investigate the convergence properties of Algorithm 1. Although the convergence of ADMM algorithm for solving the general multi-block optimization cannot be guaranteed [17], we can still use the special structure of the Equation (Model) 5 to obtain the convergence of Algorithm 1, whose proofs are similar to the proofs in Section 3.2 of [18]. We first present the Karush-Kuhn-Tucker (KKT) conditions for Equation (Model) 5:
where Ωc represents the complement of the set Ω. Moreover, we call satisfying (Equations 24–31) a stationary point of Equation (Model) 5, where and are Lagrange multipliers associated with the constraints and , respectively. For any integer q≥0, denote .
Proposition 1. Let be the sequence generated by Algorithm 1. Then, we have
Proof: We consider three cases: (a) Ω = ∅, (b) ∅⊊Ω⊊[n1] × [n2] × [n3] × [n4], and (c) Ω = [n1] × [n2] × [n3] × [n4]. In case (a), the -subproblem Equation (Model) 7 becomes an unconstrained optimization problem, which is equivalent to , where
with , and . It is obvious that f1 is strongly convex with modulus at least λ+β1+β2. Consequently, by Theorem 5.24 in [19], it holds that
for any . Since is the optimal solution of , which means , from Equation (Inequality) 34, it holds that
which implies, together with the definition of , that Equation (Inequality) 32 holds.
In the case ∅⊊Ω⊊[n1] × [n2] × [n3] × [n4], the simple equality constraints in Equation (Model) 7 can be eliminated by substituting them into its objective function, and the corresponding -subproblem is converted into an unconstrained optimization problem, whose objective function is similar to the structure of f1, but , , , and are replaced by , , , and , respectively. Noticing , similar to the proof for the case (a), we know that Equation (Inequality) 32 still holds. In the case (c), since , the inequality [Equation (Inequality) 32] is obvious. We complete the proof.
Proposition 2. Let be the sequence generated by Algorithm 1. Then we have
and
Proof: For every j∈[n3n4] and integer q≥0, we define the function by
It is obvious that fjq is strongly convex with modulus at least 1. Similar to the proof for (a) of Proposition 1, we have
which implies, together with the fact (Equation 2), that
Moreover, by the definition of , we know that the first inequality in Equation (Inequality) 36 holds. The inequality [Equation (Inequality) 37] can be proved similarly.
Employing a proof analogous to that of Proposition 1, we can derive the following proposition.
Proposition 3. Let be the sequence generated by Algorithm 1. Then, we have
and
Denote
From Propositions 1, 2, and 3, we have the following theorem, which characterizes the sufficient decrease property and boundedness of the sequence .
Theorem 2. Let be the sequence generated by Algorithm 1. If ξ1>0 and ξ2>0, then the sequence satisfies
where and μ = (1/2)min1, λ+β1+β2, ξ1/β1, ξ2/β2. Furthermore, if and , then the sequence is bounded.
Proof: From Equations 6, 23, we have
Moreover, from Equations (Inequalities) 32, 36, 38, we have
Consequently, by Equation 41 and Equation (Inequality) 42, it holds that
On the other hand, it holds that
for every q≥0. Consequently, by Equation 44, we have
Similarly, we have
for every q≥0, which implies
Combining Equations (Inequalities) 43 with 45 and 47, we obtain
Finally, by the definition of μ, we know that Equation (Inequality) 40 holds.
Now, we prove the boundedness of . Because and for any q≥0, by Equation 6, we can obtain
for every q≥0. According to Equation (Inequality) 40, for any q≥0, it is clear that
Therefore, by the definition of f, as well as Equation 6 and Equation (Inequalities) 49, 50, it holds that for any q≥0,
Since η1>0, η2>0, we know that is bounded, which implies the boundedness of from Equations 44, 46. In addition, since for any q≥0, which from Equation (Inequality) 49, it follows that is also bounded. Based on the comprehensive analysis presented above, we have completed the proof.
Remark 3. Given the predefined dimensions of matrices H and K, the singular values of H⊤H and K⊤K can be computed. Consequently, we only need to select appropriate parameters that satisfy the hypotheses stated in Theorem 2.
Theorem 3. For the sequence generated by Algorithm 1. If the parameters satisfy the conditions in Theorem 2, then any cluster point Θ∞ of is a stationary point of Equation (Model) 5.
Proof: According to Theorem 2, the sequence is bounded. Let be a convergent subsequence such that . First, from Equation (Inequality) 50, we have
Taking the limit of both sides of the Equation 52 as i approaches ∞, it holds that
which means . Moreover, from the optimality conditions of -subproblem to -subproblem, as well as the update of and , it is easy to see that
Since and , it holds that from Equation (Inequality) 45. Similarly, we have from Equation (Inequality) 47. Consequently, by letting i → ∞ in Equation 54, we know that Θ∞ is a stationary point of Equation (Model) 5. We complete the proof.
5 Numerical experiments
In this section, we apply our approach (SRdTPD) to two authentic Internet traffic datasets: the GÉANT dataset [20], which logs Internet traffic data from 23 original nodes to 23 destination nodes every 15 min, and the Abilene1 dataset, which records Internet traffic data from 11 original nodes to 11 destination nodes every 5 min. For both datasets, we select data spanning a period of 7 days. The sizes of these two datasets, arranged in fourth-order tensor format, are 7 × 96 × 23 × 23 and 7 × 288 × 11 × 11, respectively. For each experiment situation, we reconstruct the Internet traffic data 5 times, and all experimental results presented are the average of five repetitions. As demonstrated by the recovery test results for synthetic experiments and many real-world applications [14], algorithms with the discrete Fourier transform not only exhibit similar recovery accuracy but also incur lower computational costs, compared to methods utilizing the discrete cosine transform or random orthogonal transform. It is well-known that the most basic Fast Fourier Transform (FFT) has a computational complexity of O(NlogN) [21]. Using this result, it is not difficult to see that the computational complexity of SRdTPD using FFT is O(n1+n2)n1n2+slog(n3n4)n3n4, which is significantly lower than that of methods using other transforms. Considering the potential computational cost advantage of SRdTPD equipped with FFT when processing large-scale data, we choose normalized discrete Fourier transform as L3 and L4 in L, i.e.,
with the . All the experiments are performed on a notebook computer (13th Gen Intel(R) Core(TM) i9-13900HX CPU @2.20 GHz with 24G memory) running a 64-bit Windows Operating System. The code of SRdTPD is released at https://github.com/Duan-Yuxuan/Traffic-data-recovery/tree/master.
To verify the performance of SRdTPD, we compare it with five existing approaches designed for data recovery, the first of which is a matrix completion method, and the remaining four are tensor completion methods:
• SRMF, which is a low-rank matrix completion with a spatio-temporal regularization [4];
• CPWOPT, which is a tensor CP factorization completion approach [22];
• T2C, which is a tensor filling approach that decomposes third-order tensors into three third-order low-rank tensors in a balanced manner [23];
• SRTNN, which is a tensor-based approach with spatio-temporal regularization terms [11];
• BGCP, which is a Bayesian Gaussian CP tensor decomposition approach [24].
We use the Normalized Mean Absolute Error (NMAE) to measure the quality of the recovered data by models and algorithms, where the NMAE is defined as follows
where and are original data and recovered data, respectively. Clearly, lower NMAE value means better quality of the recovered data. We first consider the recovery effectiveness of various approaches for missing data with data sample rates ranging from 10% to 90%. Under random missingness in the GÉANT dataset, we set α1 = 0.1, α2 = 200, β1 = 0.01, α2 = 10, λ = 0.001, γ = 0.01, while for the Abilene dataset, we choose α1 = β2 = 0.01, α2 = 10, β1 = λ = γ = 0.001. Figure 1 presents the NMAE values for missing data recovery using various methods at different sampling rates. It can be visually observed that SRdTPD outperforms other methods in terms of data recovery effectiveness, particularly as the missing data rate increases, where the superiority of our method's recovery performance becomes increasingly apparent.
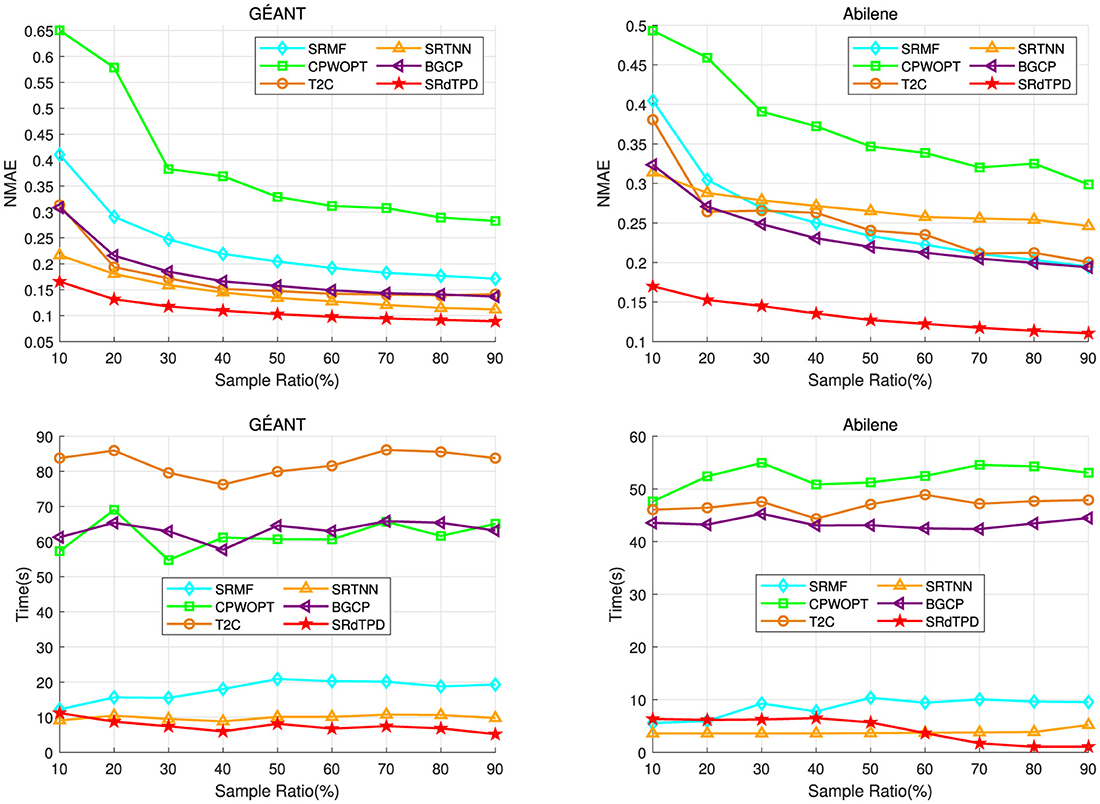
Figure 1. Numerical comparison on NMAE and computing time (seconds) with respect to different random sample ratios.
In addition to the recovery of data missing at random, we next consider the following four types of structural missingness scenarios, which are given in [4], thereby demonstrating that our method fully exploits the structural information within the data.
• xxTimeRandLoss This scenario simulates the phenomenon of structured data absence at specific time points influenced by certain factors, such as the utilization of unreliable data transmission equipment. Specifically, these data are missing at these specific time points in a certain random proportion. In our simulation, we randomly select xx% of the matrix slices along the last two modes of the Internet traffic tensor of size n1×n2×n3×n4, and subsequently, we further randomly delete q% of its elements in each selected matrix.
• xxElemRandLoss This scenario simulates the structured absence of data for specific OD (Origination-Destination) nodes under the influence of certain factors, such as the use of unreliable data transmission equipment. In this context, OD node data are missing in a certain random proportion. To simulate this phenomenon, we randomly select xx% of the matrix slices along the first two modes of the Internet traffic tensor of size n1×n2×n3×n4 and further randomly delete q% of the elements in each selected matrix.
• xxElemSyncLoss This scenario simulates the structured data missingness for specific OD nodes due to a uniform underlying cause, resulting in temporally synchronized missingness among these OD nodes. To emulate this condition, we randomly select xx% of the slices from the Internet traffic tensor of size n1×n2×n3×n4, which are matrix representations expanded along its first two modes. Subsequently, for each selected matrix, we randomly choose a common set of time indices (q%) and delete the corresponding elements at these indices.
• RowRandLoss With flow level measurements, data are collected by a router. If a router is unable to collect data for a period of time, all data collected during those specific time points will be missing. To simulate this scenario, we randomly select p% of the time points within a day and delete all data recorded at those time points across a 7-day period.
Under these scenarios of structural missingness, with β1 = γ = 0.01, the parameters for the first two structural missingness cases are identical within the same dataset (GÉANT: α1 = 0.1, α2 = 200, β2 = 10, λ = 0.001; Abilene: α1 = 0.1, α2 = 2, β2 = 1, λ = 0.001), while the parameters for the last two structural missingness cases are consistent within the same dataset (GÉANT: α1 = 0.01, α2 = 2, β2 = 1, λ = 0.01; Abilene: α1 = 0.01, α2 = 1, β2 = 0.1, λ = 0.01). Among these four types of structured missingness scenarios, we simulate the first three by considering the following 12 specific missingness cases: Missing Id 1-4: Set xx = 25 and, correspondingly, set q = 30, 50, 70, and 90, respectively; Missing Id 5-8: Set xx = 50 and, correspondingly, set q = 30, 50, 70, and 90, respectively; Missing Id 9-12: Set xx = 75 and, correspondingly, set q = 30, 50, 70, and 90, respectively. For the fourth type of structured missingness, we specifically select p = 15, 30, 45, 50, and 75.
The recovery performance and computational time of different methods under distinct structural missingness scenarios are comprehensively presented in Figures 2–4, Table 1. Experimental results demonstrate that the proposed SRdTPD approach achieves substantial improvements in handling structurally missing data. Regarding recovery accuracy, SRdTPD demonstrates superior performance compared to listed methods, attaining competitive results across all test cases. In terms of computational efficiency, SRdTPD maintains favorable time — while a moderate increase in computational time is observed under extreme missingness conditions (attributable to iterative process requirements), the runtime remains within practical thresholds for real-world applications. These findings collectively suggest that SRdTPD effectively balances recovery accuracy and computational demands, providing a robust solution for structural missing data recovery tasks.
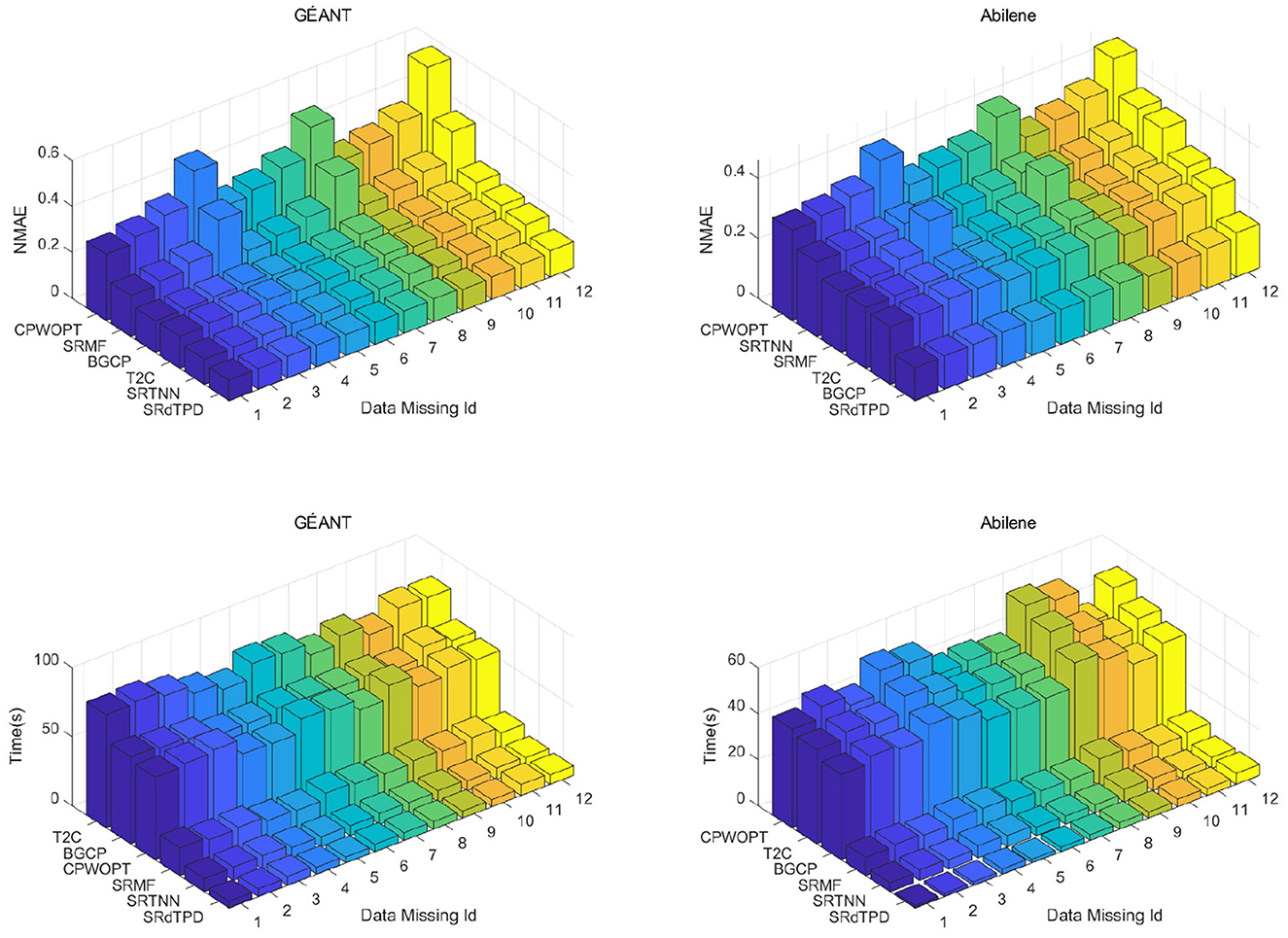
Figure 2. Numerical comparison on NMAE and computing time (seconds) with respect to different cases of xxTimeRandLoss.
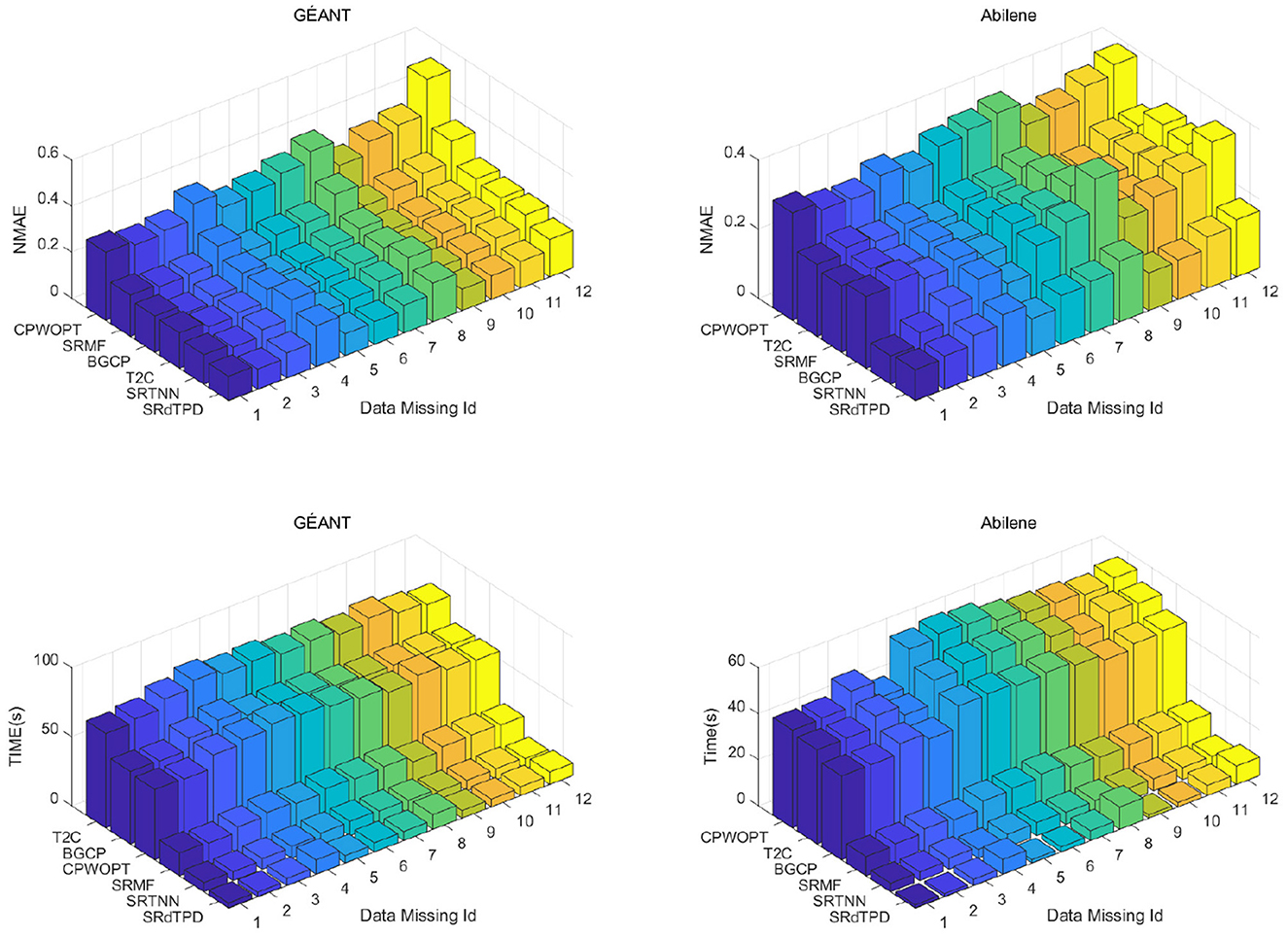
Figure 3. Numerical comparison on NMAE and computing time (seconds) with respect to different cases of xxElemRandLoss.
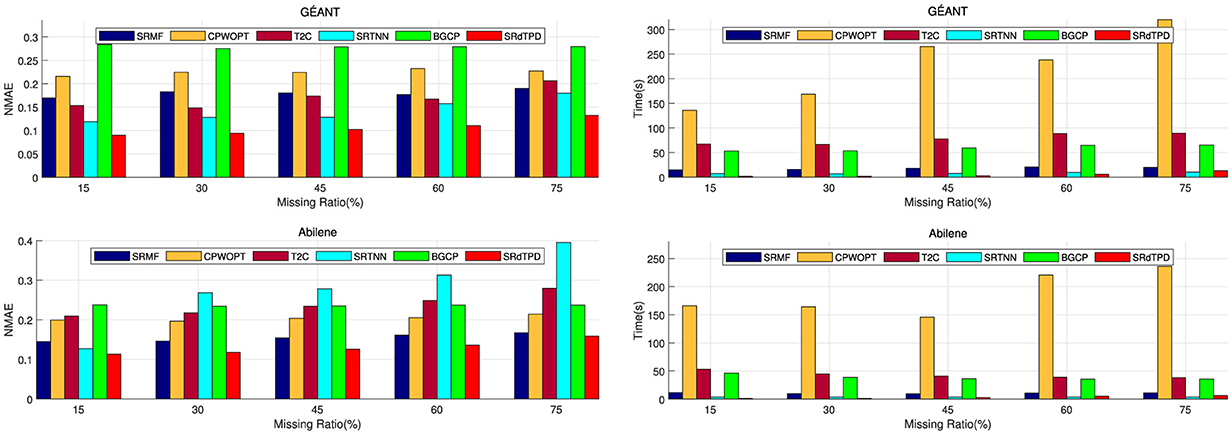
Figure 4. Numerical comparison on NMAE and computing time (seconds) with respect to diverse missing ratio of RowRandLoss.
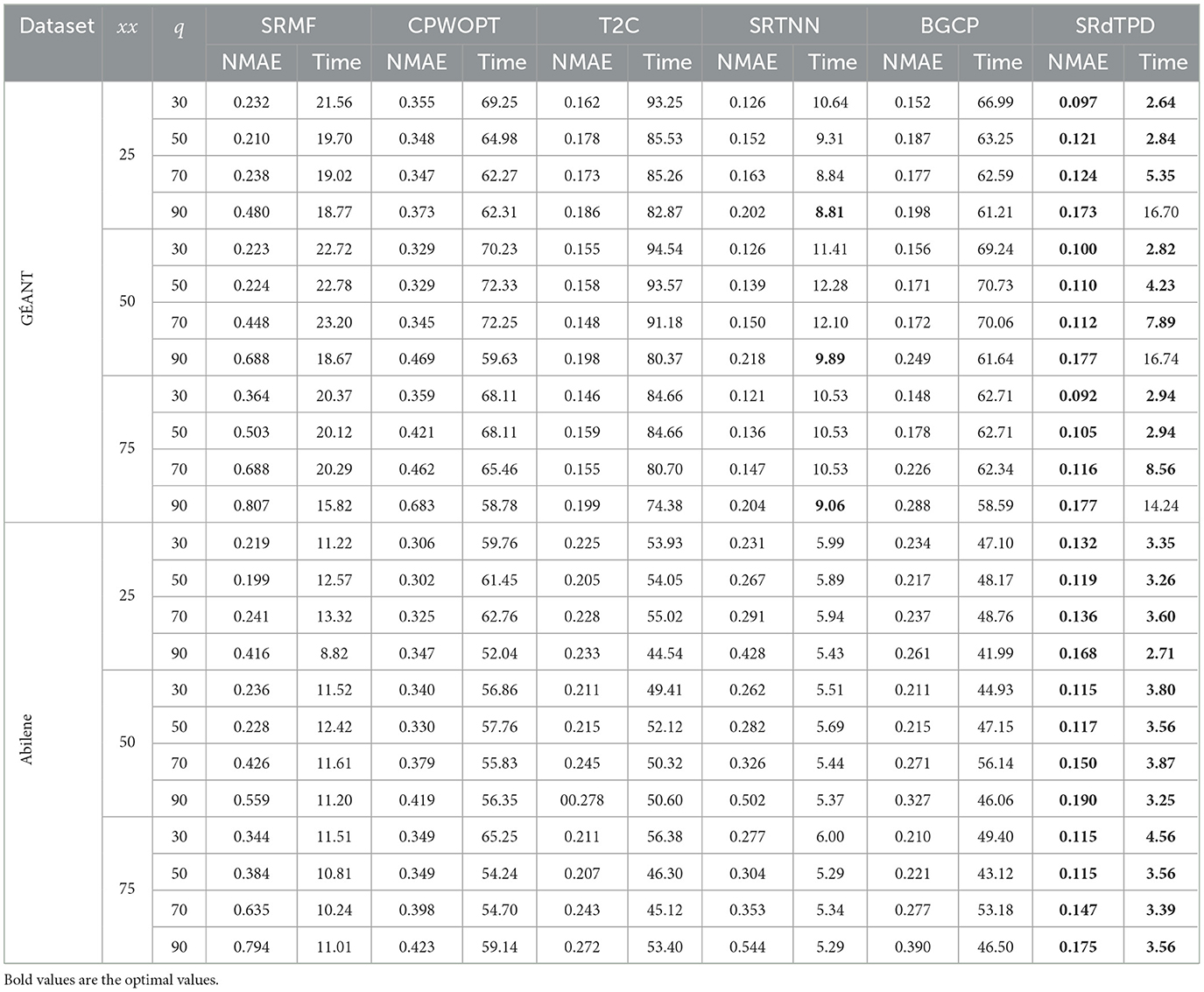
Table 1. Results on NMAE and computing time (seconds) with respect to diverse cases of xxElemSyncLoss.
6 Conclusion
This study establishes an equivalence relationship between the d-th order tensor nuclear norm (TNN) in the unitary transformation domain and the squared sum of the Frobenius norms of its two factorization factors. Based on this relationship, we constructed a novel low-rank recovery method for Internet traffic data that effectively incorporates the spatio-temporal characteristics of traffic data while significantly reducing computational time. Auxiliary variables are introduced into the model solution process, which is then solved using the ADMM. Numerical experiments demonstrate that the proposed method exhibits significant advantages in the recovery of Internet traffic data, particularly in cases of structural missingness.
Data availability statement
The raw data supporting the conclusions of this article will be made available by the authors, without undue reservation.
Author contributions
YD: Investigation, Methodology, Writing – original draft. CL: Validation, Writing – review & editing. JL: Validation, Writing – review & editing. XY: Validation, Writing – review & editing.
Funding
The author(s) declare that financial support was received for the research and/or publication of this article. CL's work was supported in part by National Natural Science Foundation of China (No. 11971138). JL's work was supported by the Science and Technology Research Program of Chongqing Municipal Education Commission (Grant No. KJZD-K202200506), by Chongqing Talent Program Lump-sum Project (No. cstc2022ycjh-bgzxm0040), and by the Foundation of Chongqing Normal University (22XLB005, ncamc2022-msxm02). XY's work was supported by the Major Program of National Natural Science Foundation of China (Nos. 11991020, 11991024).
Conflict of interest
The authors declare that the research was conducted in the absence of any commercial or financial relationships that could be construed as a potential conflict of interest.
Generative AI statement
The author(s) declare that no Gen AI was used in the creation of this manuscript.
Publisher's note
All claims expressed in this article are solely those of the authors and do not necessarily represent those of their affiliated organizations, or those of the publisher, the editors and the reviewers. Any product that may be evaluated in this article, or claim that may be made by its manufacturer, is not guaranteed or endorsed by the publisher.
Footnotes
References
1. Roughan M, Thorup M, Zhang Y. Traffic engineering with estimated traffic matrices. In: Proceedings of the 3rd ACM SIGCOMM Conference on Internet Measurement. Miami Beach, FL: Association for Computing Machinery (2003). p. 248–258.
2. Cahn RS Wide area network design: concepts and tools for optimization. IEEE Commun Mag. (2000) 38:28–30. doi: 10.1109/MCOM.2000.867843
3. Zhang Y, Ge Z, Greenberg A, Roughan M. Network anomography. In: Proceedings of the 5th ACM SIGCOMM conference on Internet Measurement. Berkeley, CA: USENIX Association (2005). p. 30.
4. Zhang Y, Roughan M, Willinger W, Qiu L. Spatio-temporal compressive sensing and internet traffic matrices. In: Proceedings of the ACM SIGCOMM 2009 Conference on Data Communication. Barcelona: Association for Computing Machinery (2009). p. 267–278.
5. Carroll JD, Chang JJ. Analysis of individual differences in multidimensional scaling via an N-way generalization of “Eckart-Young” decomposition. Psychometrika. (1970) 35:283–319. doi: 10.1007/BF02310791
6. Harshman RA, et al. Foundations of the PARAFAC procedure: Models and conditions for an “explanatory” multi-modal factor analysis. In: UCLA Work Papers Phonetics. Ann Arbor; Los Angeles, CA: University Microfilms (1970). p. 84.
7. Oseledets IV. Tensor-train decomposition. SIAM J Sci Comput. (2011) 33:2295–317. doi: 10.1137/090752286
8. Kilmer ME, Martin CD. Factorization strategies for third-order tensors. Linear Algebra Appl. (2011) 435:641–58. doi: 10.1016/j.laa.2010.09.020
9. Candès E, Recht B. Exact matrix completion via convex optimization. Found Comput Math. (2009) 9:717–72. doi: 10.1007/s10208-009-9045-5
10. Zhang Z, Ely G, Aeron S, Hao N, Kilmer M. Novel methods for multilinear data completion and de-noising based on tensor-SVD. In: 2014 IEEE Conference on Computer Vision and Pattern Recognition. Columbus, OH: IEEE (2014). p. 3842–3849.
11. Li C, Chen Y, Li D. Internet traffic tensor completion with tensor nuclear norm. Comput Optim Appl. (2024) 87:1033–57. doi: 10.1007/s10589-023-00545-5
12. He H, Ling C, Xie W. Tensor completion via a generalized transformed tensor T-product decomposition without t-SVD. J Sci Comput. (2022) 93:47. doi: 10.1007/s10915-022-02006-3
13. Martin CD, Shafer R, LaRue B. An order-p tensor factorization with applications in imaging. SIAM J Sci Comput. (2013) 35:A474–90. doi: 10.1137/110841229
14. Qin W, Wang H, Zhang F, Wang J, Luo X, Huang T. Low-rank high-order tensor completion with applications in visual data. IEEE Trans Image Process. (2022) 31:2433–48. doi: 10.1109/TIP.2022.3155949
15. Kolda TG, Bader BW. Tensor decompositions and applications. SIAM Rev. (2009) 51:455–500. doi: 10.1137/07070111X
16. Recht B, Fazel M, Parrilo PA. Guaranteed minimum-rank solutions of linear matrix equations via nuclear norm minimization. SIAM Rev. (2010) 52:471–501. doi: 10.1137/070697835
17. Chen C, He B, Ye Y, Yuan X. The direct extension of ADMM for multi-block convex minimization problems is not necessarily convergent. Math Program. (2016) 155:57–79. doi: 10.1007/s10107-014-0826-5
18. Popa J, Lou Y, Minkoff SE. Low-rank tensor data reconstruction and denoising via ADMM: algorithm and convergence analysis. J Sci Comput. (2023) 97:49. doi: 10.1007/s10915-023-02364-6
19. Beck A. First-Order Methods in Optimization. Philadelphia: SIAM. (2017). doi: 10.1137/1.9781611974997
20. Uhlig S, Quoitin B, Lepropre J, Balon S. Providing public intradomain traffic matrices to the research community. ACM SIGCOMM Comput Commun Rev. (2006) 36:83–6. doi: 10.1145/1111322.1111341
21. Cooley JW, Tukey JW. An algorithm for the machine calculation of complex Fourier series. Math Comp. (1965) 19:297–301. doi: 10.1090/S0025-5718-1965-0178586-1
22. Acar E, Dunlavy DM, Kolda TG, Mørup M. Scalable tensor factorizations for incomplete data. Chemom Intell Lab Syst. (2011) 106:41–56. doi: 10.1016/j.chemolab.2010.08.004
23. Chen X, He Z, Chen Y, Lu Y, Wang J. Missing traffic data imputation and pattern discovery with a Bayesian augmented tensor factorization model. Transp Res Part C: Emerg Technol. (2019) 104:66–77. doi: 10.1016/j.trc.2019.03.003
Keywords: Internet traffic data recovery, d-th order TNN, spatio-temporal regularization, ADMM algorithm, tensor completion
Citation: Duan Y, Ling C, Liu J and Yang X (2025) Internet traffic data recovery via a low-rank spatio-temporal regularized optimization approach without d-th order T-SVD. Front. Appl. Math. Stat. 11:1587681. doi: 10.3389/fams.2025.1587681
Received: 04 March 2025; Accepted: 07 April 2025;
Published: 06 May 2025.
Edited by:
Yannan Chen, South China Normal University, ChinaReviewed by:
Douglas Soares Goncalves, Federal University of Santa Catarina, BrazilCan Li, Honghe University, China
Xueli Bai, Guangdong University of Foreign Studies, China
Copyright © 2025 Duan, Ling, Liu and Yang. This is an open-access article distributed under the terms of the Creative Commons Attribution License (CC BY). The use, distribution or reproduction in other forums is permitted, provided the original author(s) and the copyright owner(s) are credited and that the original publication in this journal is cited, in accordance with accepted academic practice. No use, distribution or reproduction is permitted which does not comply with these terms.
*Correspondence: Jinjie Liu, amluamllLmxpdUBjcW51LmVkdS5jbg==