- Munich Center for Digital Sciences and AI (MUC.DAI), HM Hochschule München University of Applied Sciences, Munich, Germany
This study presents a comprehensive evaluation of the environmental cost of large language models (LLMs) by analyzing their performance, token usage, and CO2 equivalent emissions across 14 LLMs ranging from 7 to 72 billion parameters. Each LLM was tasked with answering 500 multiple-choice and 500 free-response questions from the MMLU benchmark, covering five diverse subjects. Emissions were measured using the Perun framework on an NVIDIA A100 GPU and converted through an emission factor of 480 gCO2/kWh. Our results reveal strong correlations between LLM size, reasoning behavior, token generation, and emissions. While larger and reasoning-enabled models achieve higher accuracy, up to 84.9%, they also incur substantially higher emissions, driven largely by increased token output. Subject-level analysis further shows that symbolic and abstract domains such as Abstract Algebra consistently demand more computation and yield lower accuracy. These findings highlight the trade-offs between accuracy and sustainability, emphasizing the need for more efficient reasoning strategies in future LLM developments.
1 Introduction
Artificial intelligence (AI) is transforming communication at all levels, from one-on-one interactions to organizational and societal exchanges, by enhancing speed, creativity, and personalization, while also raising challenges related to bias, privacy, and governance (Polak and Anshari, 2024; Sonni, 2025). As AI technologies permeate the communication domain, it becomes essential to quantify their environmental costs. Natural Language Processing (NLP) is a subfield of artificial intelligence focused on enabling computers to understand, generate, and interpret human language. In particular, the rapid development and widespread adoption of large language models (LLMs) have profoundly impacted NLP, communication research, and adjacent fields. LLMs are deep neural networks trained on large corpora of text data to learn statistical patterns of language, enabling them to generate and interpret human-like text. With new architectures and benchmarks emerging on the scale of weeks or months, LLM capabilities and applications are expanding at an unprecedented pace (Minaee et al., 2024; Movva et al., 2024). These applications include multilingual machine translation (Zhu et al., 2024), text summarization (Liu et al., 2024), question answering (Arefeen et al., 2024), and code generation (Jiang et al., 2024). Following the release of ChatGPT, the average daily rate of arXiv preprints mentioning “large language model” in their title or abstract rose from 0.40 to 8.58, highlighting the surge of interest in this domain (Zhao et al., 2024).
The growing adoption of LLMs has opened new research opportunities while amplifying concerns about data security, cultural bias, and societal impact. Recent studies reveal systemic issues of linguistic and cultural dominance, mainly driven by English-language training corpora, which threaten the inclusion of AI systems (Wang et al., 2024; LI et al., 2024). In response, researchers have proposed bias mitigation frameworks and culturally adaptive training methodologies to promote more equitable, globally representative LLMs (Wang et al., 2024).
These ethical and societal dimensions are increasingly reflected in publication trends. Between 2018–2022 and 2023, the Computers and Society subcategory on arXiv grew 20× faster in its share of articles related to LLMs than other subfields. Submissions on Applications of ChatGPT and Societal implications increased eight times and four times, respectively (Movva et al., 2024). Almost half (49.5%) of first authors in 2023 LLM papers and 38.6% of corresponding authors had no previous NLP publications, indicating a growing interdisciplinary research community exploring LLM applications in human–computer interaction, security, software engineering, and beyond (Movva et al., 2024).
Despite extensive attention to social and security issues, sustainability remains critically underexplored. Generative AI models, including LLMs, are estimated to consume ~29.3 TWh annually, comparable to Ireland's total energy consumption (de Vries, 2023), yet only a small fraction of LLM research addresses the carbon footprint or environmental impact. As model sizes grow, reaching hundreds of billions of parameters (Zhao et al., 2024), their sustainability implications intensify. A Scopus analysis shows that LLM-related publications increased by 82.7% from 2020 to January 2025, but only 1.82% and 0.64% of those papers explicitly address “carbon emissions” or “CO2” impacts, respectively (Elsevier, 2025). Moreover, existing work often relies on theoretical estimates rather than empirical measurements of energy consumption during training and inference.
2 Sustainability calculation for large language models
Accurate quantification of the environmental impact of a product requires a comprehensive Life Cycle Assessment (LCA), in which all environmental burdens are evaluated, from the extraction of raw materials to the end of life disposal (Klöpffer, 1997). For LLMs, this assessment encompasses both the manufacture of computing infrastructure (including raw material acquisition, processing, and fabrication of server components such as GPUs) and all subsequent lifecycle stages: dataset generation, data preprocessing, iterative experimentation, model training, deployment, and eventual retirement (Luccioni et al., 2023). Due to limited transparency across these phases, existing studies often rely on estimates of material and manufacturing impacts (Khowaja et al., 2024; de Vries, 2023) or focus on directly measurable quantities, notably energy consumption during training and inference (Luccioni et al., 2023; Liu and Yin, 2024; Faiz et al., 2024).
Standard analyses of LLM greenhouse gas (GHG) emissions typically focus on computing related impacts during deployment. However, a full LCA requires converting all GHGs, carbon dioxide (CO2), methane (CH4), and nitrous oxide (N2O), into carbon dioxide equivalents (CO2eq) by applying the global warming potential (GWP) of each gas relative to CO2 (Luccioni et al., 2023; Faiz et al., 2024; Strubell et al., 2020; Liu and Yin, 2024).
Although CO2eq is the de facto standard for reporting LLM sustainability, meaningful comparison across studies is impeded by methodological heterogeneity. Variations in system boundaries, estimation methods, functional units, model parameterizations, and architectural differences complicate direct benchmarking and the transferability of conclusions.
3 Method
In this study, we investigate the relationship between performance and carbon dioxide equivalent emissions (CO2eq) for various LLM families and evaluate multiple models with different numbers of parameters. The examined LLMs include Meta's Llama3.1 models (Grattafiori et al., 2024) with 8 billion and 70 billion parameters, as well as the Llama3.3 model (Grattafiori et al., 2024) with 70 billion parameters. Additionally, Alibaba's Qwen models (Bai et al., 2023) and the Qwen2.5 models (Qwen et al., 2025), each with 7 billion and 72 billion parameters, are used for comparison. Furthermore, two reasoning models developed by Deep Cogito, with 8 billion and 70 billion parameters, are included. These models operate in both standard text generation mode and reasoning mode. Also, Deepseek R1 models (DeepSeek-AI et al., 2025), specifically designed for reasoning, are included, featuring variants with 7 billion, 8 billion, and 70 billion parameters.
All models were tasked with answering the same 500 questions drawn from different subject areas. The questions and their correct answers were extracted from the Massive Multitask Language Understanding (MMLU) dataset (Hendrycks et al., 2021). The MMLU dataset evaluates multitask accuracy in diverse knowledge domains, comprising 15,908 multiple choice questions from 57 subjects including engineering, mathematics, humanities, and social sciences. The questions are sourced from publicly available practice exams and academic materials that span various educational levels and each question provides multiple choice answers, with correct responses derived from the original educational content created by experts (Hendrycks et al., 2021). For the purposes of our study, the five subjects Philosophy, High School World History, International Law, Abstract Algebra, and High School Mathematics were selected to ensure a comprehensive range of questions from general historical and legal knowledge to mathematical logic across educational levels. Each model answered 100 questions per subject and underwent two distinct testing phases.
In the first phase, the models received the question accompanied by four multiple choice options and were required to select the correct answer. For standard text-generation models such as Qwen, Llama, and Cogito (in their default text-generation mode), the output was limited to a single word, indicating the index of the chosen answer. This allowed for straightforward and objective comparison between the model's chosen answer and the correct answer from the MMLU dataset. However, for reasoning-based models, output word limits were not imposed, as these models require generating additional text to complete their reasoning processes.
In the second testing scenario, models received only the question as a prompt, without restrictions on output length. To assess the correctness of the responses, we used the fast, cost-effective OpenAI o4-mini reasoning model as an evaluator. This evaluator was provided with the question, the multiple choice options, the correct answer, and the model-generated answer as input, tasked with judging the correctness of the answer based on the specific subject and the known correct answer from the MMLU dataset.
All experiments were carried out on a local Nvidia A100 GPU with 80 GB of memory, allowing accurate measurement of energy consumption, memory usage, and response time during model evaluation. Measurements were performed using the Perun framework, designed for energy benchmarking of high-performance computing applications (Gutiérrez Hermosillo Muriedas et al., 2023). To calculate associated CO2eq emissions, an emission factor of 480 gCO2/kWh was applied. This emission factor was selected as it represents the latest global average. This value represents the global average emission factor, reflecting recent trends toward increased adoption of renewable energy, providing a realistic baseline to evaluate environmental impacts (Wiatros-Motyka et al., 2024).
4 Results
Before relating model behavior to energy consumption and the resulting CO2eq emissions, we first present the raw task performance that underpins all subsequent analyzes. Specifically, we quantify the precision of each model in the 500 MMLU questions in two scenarios: a constrained multiple-choice phase and a free-response phase, whose outputs are adjudicated by the OpenAI o4-mini evaluator. Figure 1 shows the number of correct responses each model achieves across the five MMLU subjects in both phases. By comparing success rates across knowledge domains and parameter scales from 7 billion to 72 billion parameters, we find that the largest models consistently lead. In the multiple-choice phase, the reasoning-enhanced Cogito 70B model tops the field with 91.0% correct answers, followed by the Deepseek R1 70B reasoning model at 85.0% and the Qwen 2.5 72B model at 80.2%. In the free-response phase, the same Cogito variant again ranks first with 78.8%, narrowly ahead of Cogito 70B in standard text mode (76.4%) and Qwen 2.5 72B (75.0%). Among the compact 7–8 billion-parameter models, Deepseek R1 8B attains 67.4% correct answers in the multiple-choice phase and 49.8% in the free-response phase. In contrast, the weakest compact model, Qwen 7B, answers only 41.4% in the multiple-choice phase and 24.4% in the free-response phase. Subject-wise, multiple-choice performance peaks on High School World History with an average of 76.3% correct answers per model and bottoms out on Abstract Algebra at 51.4% correct answers. In free-response mode, models score highest on High School Mathematics with 69.4 correct answers and lowest on Philosophy with 52.1% correct answers.
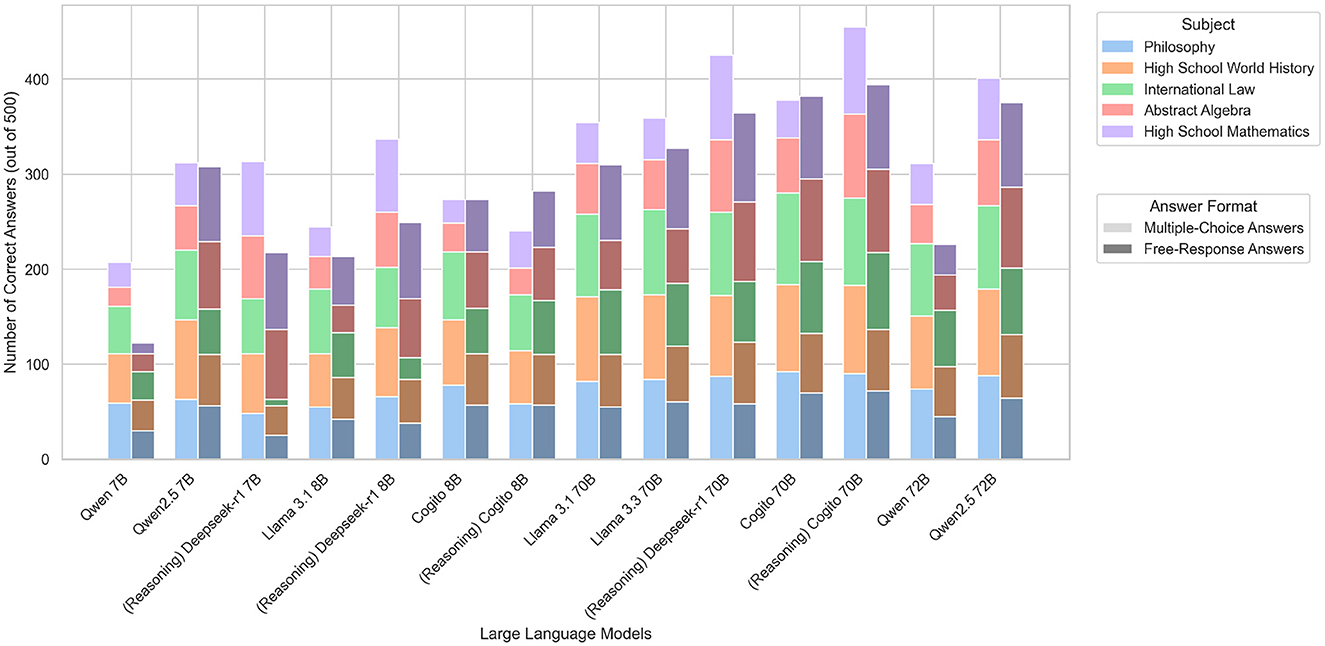
Figure 1. Comparison of the performance of tested LLMs on multiple-choice and free-response tasks, with individual subject performance displayed.
In addition to the accuracy of the different models, we also analyze the token footprints incurred by each model when answering the 500 MMLU questions. A token is a unit of text, such as a word, part of a word, or an individual character, that is converted into a numerical representation, so that an LLM can process it (Gastaldi et al., 2025). We distinguish between response tokens (the tokens comprising the model's final answer) and thinking tokens (the additional tokens generated by reasoning-enabled models before producing their answer). For each LLM, separate markers denote the average number of response tokens and, for reasoning-enabled variants, the average number of thinking tokens. In the multiple-choice setting the models generated 37.7 response tokens per question on average, while reasoning-enabled variants required an additional 543.5 thinking tokens. Subject-wise, High School Mathematics incurred the longest answers (83.3 tokens on average), while Abstract Algebra demanded the highest thinking overhead (865.5 tokens on average). In free-response mode, average response lengths increase to 435.2 tokens. The shortest free-text answers (two tokens) occur in the Qwen 2.5 72B model on Philosophy, while the longest single output (37.575 tokens) was generated by the Cogito 8B reasoning model on Abstract Algebra. Thinking tokens average 859.2 per reasoning run. Zero-token reasoning traces appear when no intermediate text is needed (e.g. Cogito 70B reasoning on certain History items), whereas the maximum reasoning burden (6.716 tokens) is observed for the Deepseek R1 7B model on an Abstract Algebra prompt. Figure 2 presents the distribution of token counts for every model in all five subjects in the free-response phase.
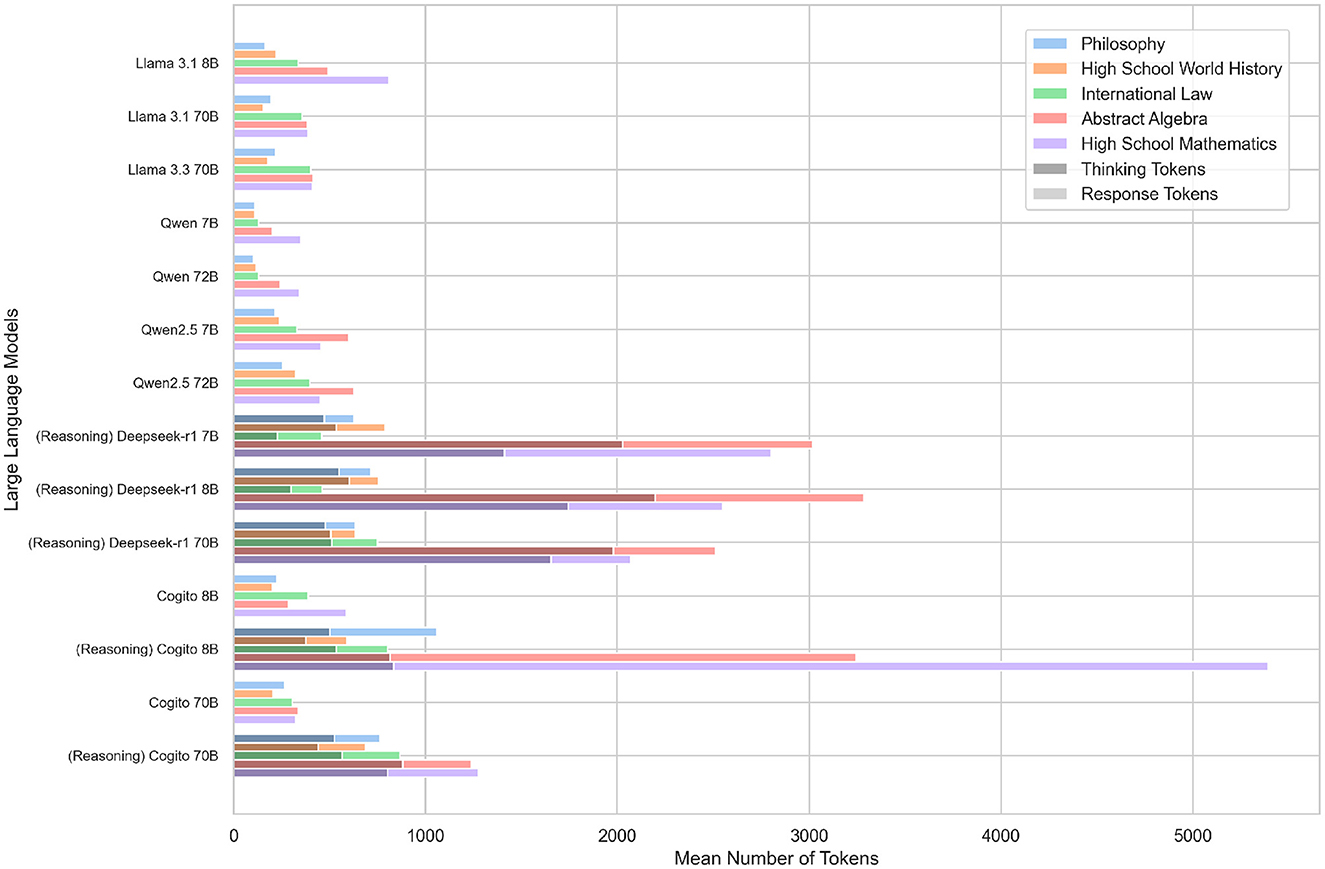
Figure 2. Average number of tokens required by each LLM to answer questions across different subjects. For reasoning models, the number of reasoning tokens generated prior to providing the final answer is indicated in a darker color.
The total CO2eq emissions, expressed in grams of CO2 equivalent, required to process the complete set of 500 MMLU questions in both the constrained multiple-choice and free-response phases are illustrated in Figure 3. In the multiple-choice phase, emissions spanned from just 1.25 g CO2eq for the default Cogito 8B model to 717.31 g CO2eq for the Deepseek-R1 70B model. Reasoning-enabled variants generated substantially more emissions than their standard counterparts (e.g. Cogito 70B reasoning: 411.72g vs. Cogito 70B default: 8.20g), and larger models (70–72B) uniformly consumed on the order of 100–700 g, whereas compact 7–8B systems emitted below 180g. In the free-response phase, the range expanded further, from a low of 26.28 g CO2eq for Qwen 7B to a high of 1,325.12 g CO2eq for Deepseek-R1 70B. Again, reasoning modes incurred a 4 × –6 × increase in emissions compared to text-only modes (e.g. Cogito 8B reasoning: 371.87g vs. Cogito 8B default: 56.30g), and high-parameter models (70–72B) emitted several hundred grams more CO2eq than their 7–8B counterparts (e.g. Qwen2.5 72B: 418.12g vs. Qwen2.5 7B: 60.63g). These trends underscore the environmental trade-off of scale and reasoning depth in large language models.
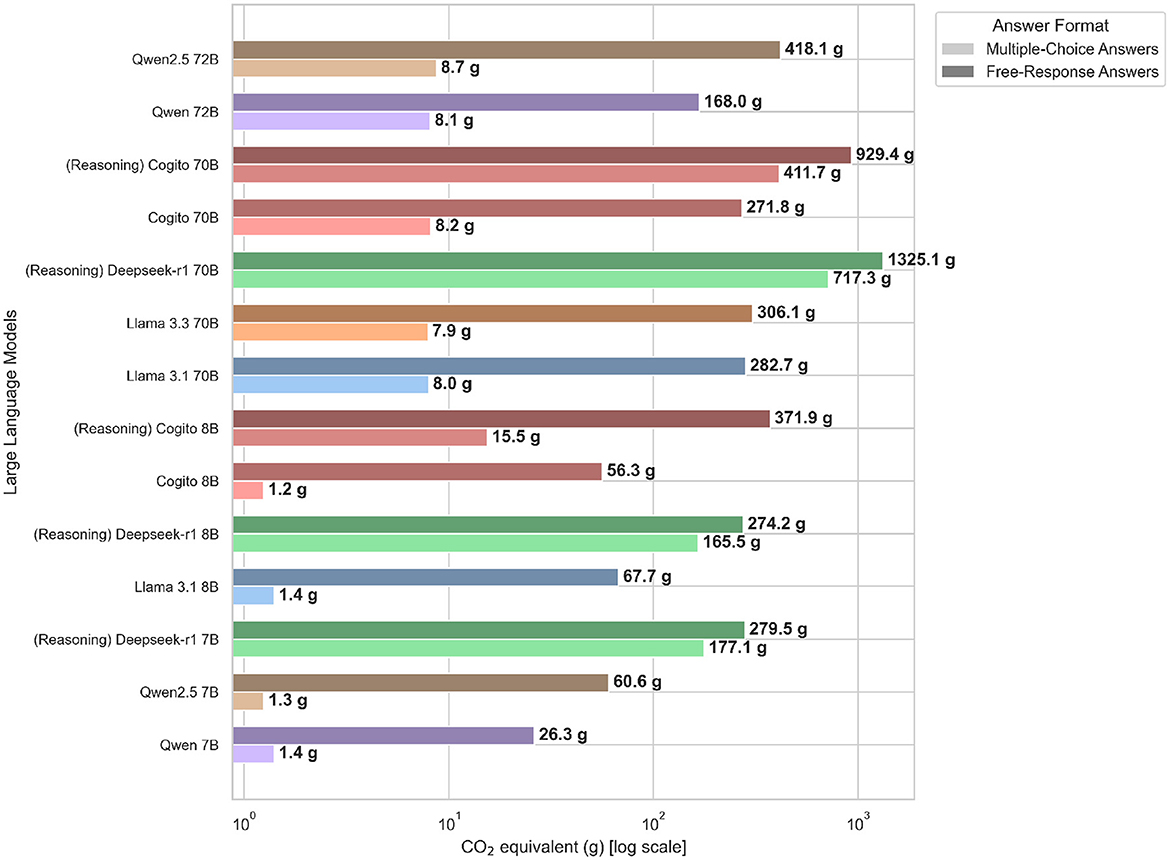
Figure 3. Total CO2 emissions (measured in grams) produced by each LLM when answering all 500 questions, reported separately for the multiple-choice and free-response scenarios.
When examining the combined CO2eq emissions and overall accuracy across all 1,000 questions (Figure 4), clear trade-offs emerge between model scale, reasoning depth, and environmental cost. The smallest model, Qwen 7B, emits only 27.7 g CO2eq, by far the lowest footprint, but achieves just 32.9% accuracy. Conversely, the largest reasoning model, Deepseek-R1 70B, incurs 2,042.4 g CO2eq and reaches 78.9% accuracy. Notably, 12 of the 14 evaluated systems require less than 500 g CO2eq, yet none of these exceeds 80% accuracy. In contrast, the reasoning-enabled Cogito 70B emits 1,341.1 g CO2eq, 34.3% less than Deepseek-R1 70B, while delivering 84.9% correct answers, representing a 7.6% improvement over its non-reasoning counterpart.
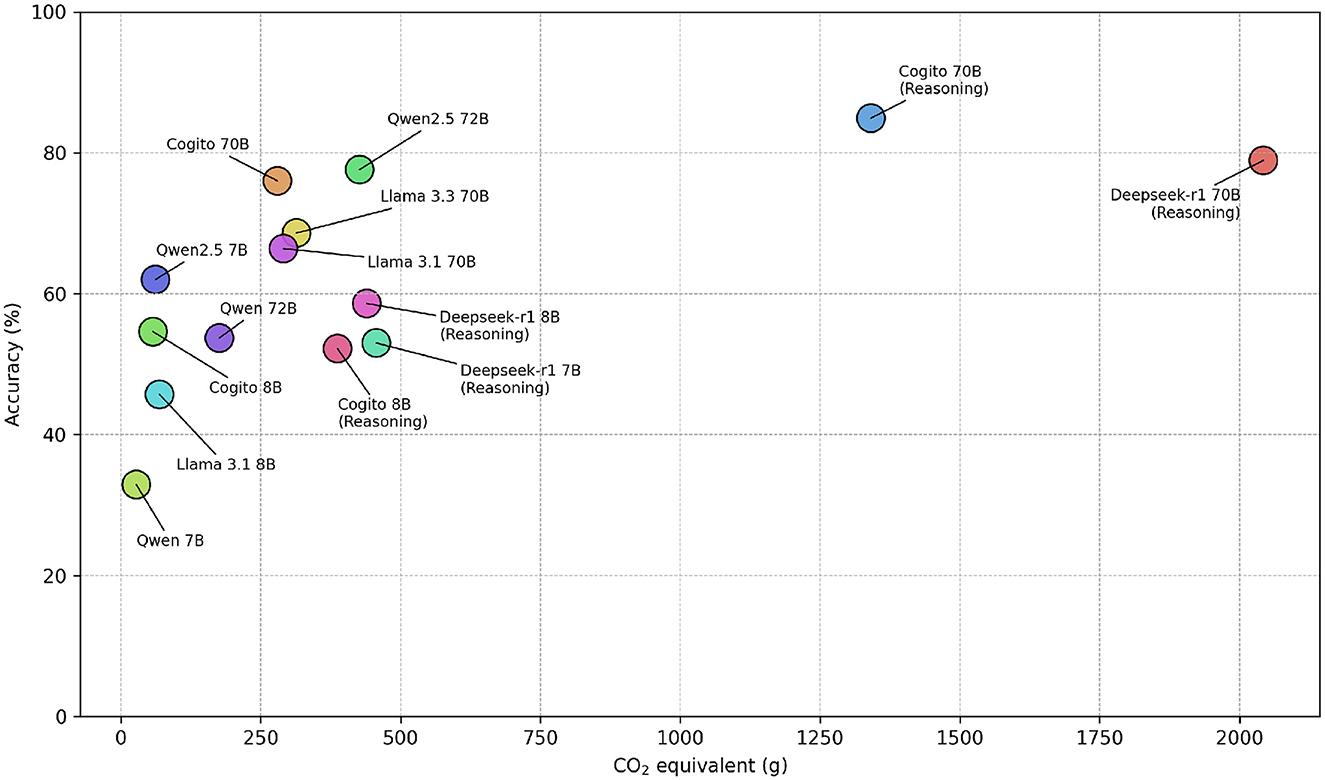
Figure 4. Combined CO2 eq emissions (measured in grams) and overall accuracy of each LLM across 1,000 questions. The results illustrate trade-offs between model size, reasoning depth, and environmental impact.
5 Discussion
The analysis of combined CO2eq emissions, accuracy, and token generation across all 1,000 questions reveals clear trends and trade-offs between model scale, reasoning complexity, and environmental impact. As model size increases, accuracy tends to improve. However, this gain is also linked to substantial growth in both CO2eq emissions and the number of generated tokens. The largest reasoning model, Deepseek-R1 70B, achieved 78.9% accuracy but emitted 2,042.4 g CO2eq, significantly surpassing smaller counterparts like Qwen 7B, which only consumed 27.7 g CO2eq but provided just 32.9% accuracy.
Notably, the reasoning-enabled Cogito 70B model demonstrates a superior performance-efficiency balance, achieving the highest accuracy of 84.9%, a relative improvement of 7.6 percentage points over the Deepseek-R1 70B, while emitting 34.3% less CO2eq (1,341.1 g). This suggests that adding a reasoning component to large models can substantially improve accuracy without proportionally escalating environmental impact.
Subject-wise analysis highlights significant variability in performance across different domains. Multiple-choice accuracy was consistently highest in High School World History, averaging 76.3% correct responses per model, likely due to the factual nature of the questions enabling easier recall or recognition. In contrast, Abstract Algebra posed the greatest challenge, averaging only 51.4% correct answers, reflecting its higher complexity and abstract conceptual demands. In the free-response phase, High School Mathematics was answered most successfully, averaging 69.4% correct responses per model, likely benefiting from explicit numerical computations. Conversely, Philosophy questions, requiring nuanced and subjective reasoning, presented substantial challenges with an average of only 52.1% correct responses.
The token generation analysis further underscores the computational cost of reasoning. On average, reasoning-enabled models required significantly more tokens in both testing phases. Particularly in the multiple-choice phase, reasoning models frequently struggled to produce concise answers, despite explicit prompts instructing them to only return the choice index. For instance, Deepseek-R1 7B generated up to 14,187 tokens on a single mathematical question, while standard models consistently produced minimal single-token responses. This trend persisted in the free-response phase, with the Cogito 8B reasoning model generating extremely verbose answers, such as a maximum of 37,575 tokens in Abstract Algebra, indicating an inherent challenge in controlling response verbosity when using reasoning prompts.
From an environmental perspective, reasoning models consistently exhibited higher emissions, driven primarily by their elevated token production. For instance, the Qwen 2.5 model with 72 billion parameters achieved strong performance with 77.6% accuracy while emitting only 426.8 g CO2eq, less than one-third of the emissions of Cogito 70B with reasoning. This efficiency is partly due to its response generation remaining concise. While Qwen 2.5 72B produced on average 1.0 word per answer for the multiple-choice test phase, compared to the Cogito 70B reasoning model, which required an average of 145.1 response tokens and 450.9 thinking tokens per question in mathematics alone. Such findings highlight how reasoning capability, while beneficial for accuracy, significantly increases emissions through longer outputs. The environmental footprint of the Cogito 70B reasoning model was substantially larger compared to non-reasoning models of similar scale, yet it maintained a favorable efficiency balance due to significantly higher accuracy.
In conclusion, while larger and reasoning-enhanced models significantly outperform smaller counterparts in terms of accuracy, this improvement comes with steep increases in emissions and computational demand. Optimizing reasoning efficiency and response brevity, particularly for challenging subjects like Abstract Algebra, is crucial for advancing more sustainable and environmentally conscious artificial intelligence technologies.
6 Limitations
Although this study compares a diverse range of language models with varying architectures, training datasets, parameter counts, and reasoning routines, the findings are not easily transferable to other model families. Due to these structural and architectural differences, generalizing the results to models with significantly different designs is limited. Moreover, the current findings do not allow for reliable conclusions about the behavior of much larger LLMs (several hundred billion parameters). To draw robust conclusions about the relationship between CO2eq emissions and accuracy at this scale, these models would need to be included in future analyses.
It should also be noted that all emissions were measured under a specific hardware and energy profile, namely, using an NVIDIA A100 80GB GPU and an emission factor of 480 gCO2/kWh. These values depend heavily on the chosen infrastructure and local energy grid, and results may vary significantly with different hardware setups or emission baselines. Therefore, the CO2eq results presented here cannot be directly extrapolated to other systems.
Future work could extend this investigation by including a broader range of models, including those fine-tuned for specific tasks across diverse domains. For instance, it would be valuable to analyze whether models specialized in code generation perform better on programming tasks, how many parameters are required to achieve high accuracy, and whether such specialization leads to lower CO2eq emissions compared to general-purpose LLMs.
Data availability statement
The original contributions presented in the study are included in the article/supplementary material, further inquiries can be directed to the corresponding author.
Author contributions
MD: Writing – original draft, Writing – review & editing. GS: Writing – original draft, Writing – review & editing.
Funding
The author(s) declare that no financial support was received for the research and/or publication of this article.
Conflict of interest
The authors declare that the research was conducted in the absence of any commercial or financial relationships that could be construed as a potential conflict of interest.
Generative AI statement
The author(s) declare that Gen AI was used in the creation of this manuscript. Generative AI was used to check for typos and wrong spelling.
Publisher's note
All claims expressed in this article are solely those of the authors and do not necessarily represent those of their affiliated organizations, or those of the publisher, the editors and the reviewers. Any product that may be evaluated in this article, or claim that may be made by its manufacturer, is not guaranteed or endorsed by the publisher.
References
Arefeen, M. A., Debnath, B., and Chakradhar, S. (2024). Leancontext: cost-efficient domain-specific question answering using llms. Nat. Lang. Process. J. 7:100065. doi: 10.1016/j.nlp.2024.100065
Bai, J., Bai, S., Chu, Y., Cui, Z., Dang, K., Deng, X., et al. (2023). Qwen technical report. arXiv [Preprint]. arXiv:2309.16609. doi: 10.48550/arXiv.2309.16609
de Vries, A. (2023). The growing energy footprint of artificial intelligence. Joule 7, 2191–2194. doi: 10.1016/j.joule.2023.09.004
DeepSeek-AI, Guo, D., Yang, D., Zhang, H., Song, J., Zhang, R., et al. (2025). Deepseek-r1: incentivizing reasoning capability in LLMs via reinforcement learning. arXiv [Preprint] arXiv:2501.12948. doi: 10.48550/arXiv:2501.12948
Elsevier (2025). Scopus. Available online at: https://www.scopus.com (accessed January 01, 2025).
Faiz, A., Kaneda, S., Wang, R., Osi, R. C., Sharma, P., Chen, F., et al. (2024). “LLM carbon: Modeling the end-to-end carbon footprint of large language models,” in The Twelfth International Conference on Learning Representations. Available online at: https://openreview.net/forum?id=aIok3ZD9to (accessed March 10, 2025).
Gastaldi, J. L., Terilla, J., Malagutti, L., DuSell, B., Vieira, T., and Cotterell, R. (2025). The foundations of tokenization: statistical and computational concerns. arXiv [Preprint]. arXiv:2407.11606. doi: 10.48550/arXiv.2407.11606
Grattafiori, A., Dubey, A., Jauhri, A., Pandey, A., Kadian, A., Al-Dahle, A., et al. (2024). The llama 3 herd of models. arXiv [Preprint]. arXiv:2407.21783. doi: 10.48550/arXiv.2407.21783
Gutiérrez Hermosillo Muriedas, J. P., Flügel, K., Debus, C., Obermaier, H., Streit, A., and Götz, M. (2023). “Perun: benchmarking energy consumption of high-performance computing applications,” in Euro-Par 2023: Parallel Processing, eds. J. Cano, M. D. Dikaiakos, G. A. Papadopoulos, M. Pericàs, and R. Sakellariou (Cham: Springer Nature Switzerland), 17–31. doi: 10.1007/978-3-031-39698-4_2
Hendrycks, D., Burns, C., Basart, S., Zou, A., Mazeika, M., Song, D., and Steinhardt, J. (2021). Measuring massive multitask language understanding. arXiv [Preprint]. arXiv:2009.03300. doi: 10.48550/arXiv.2009.03300
Jiang, J., Wang, F., Shen, J., Kim, S., and Kim, S. (2024). A survey on large language models for code generation. arXiv [Preprint]. arXiv:2406.00515. doi: 10.48550/arXiv.2406.00515
Khowaja, S. A., Khuwaja, P., Dev, K., Wang, W., and Nkenyereye, L. (2024). Chatgpt needs spade (sustainability, privacy, digital divide, and ethics) evaluation: a review. Cogn. Comput. 16, 2528–2550. doi: 10.1007/s12559-024-10285-1
Klöpffer, W. (1997). Life cycle assessment. Environ. Sci. Pollut. Res. 4, 223–228. doi: 10.1007/BF02986351
Li, C., Chen, M., Wang, J., Sitaram, S., and Xie, X. (2024). “CultureLLM: incorporating cultural differences into large language models,” in The Thirty-eighth Annual Conference on Neural Information Processing Systems. Available online at: https://openreview.net/forum?id=sIsbOkQmBL (accessed March 30, 2025).
Liu, V., and Yin, Y. (2024). Green ai: exploring carbon footprints, mitigation strategies, and trade offs in large language model training. Discov. Artif. Intell. 4:49. doi: 10.5772/intechopen.111293
Liu, Y., Shi, K., He, K., Ye, L., Fabbri, A., Liu, P., et al. (2024). “On learning to summarize with large language models as references,” inProceedings of the 2024 Conference of the North American Chapter of the Association for Computational Linguistics: Human Language Technologies (Volume 1: Long Papers), eds. K. Duh, H. Gomez, and S. Bethard (Mexico City: Association for Computational Linguistics), 8647–8664. Available online at: https://aclanthology.org/2024.naacl-long.478/ (accessed March 18, 2025).
Luccioni, A. S., Viguier, S., and Ligozat, A.-L. (2023). Estimating the carbon footprint of bloom, a 176b parameter language model. J. Mach. Learn. Res. 24, 1–15. doi: 10.5555/3648699.3648952
Minaee, S., Mikolov, T., Nikzad, N., Chenaghlu, M., Socher, R., Amatriain, X., et al. (2024). Large language models: a survey. arXiv [Preprint]. arXiv:2402.06196. doi: 10.48500/arXiv.2402.06196
Movva, R., Balachandar, S., Peng, K., Agostini, G., Garg, N., Pierson, E., et al. (2024). “Topics, authors, and institutions in large language model research: trends from 17K arXiv papers,” in Proceedings of the 2024 Conference of the North American Chapter of the Association for Computational Linguistics: Human Language Technologies (Volume 1: Long Papers), eds. K. Duh, H. Gomez, and S. Bethard (Mexico City: Association for Computational Linguistics), 1223–1243. doi: 10.18653/v1/2024.naacl-long.67
Polak, P., and Anshari, M. (2024). Exploring the multifaceted impacts of artificial intelligence on public organizations, business, and society. Palgrave Commun. 11, 1–3. doi: 10.1057/s41599-024-03913-6
Qwen, Yang, A., Yang, B., Zhang, B., Hui, B., Zheng, B., et al. (2025). Qwen2.5 technical report. arXiv [Preprint]. arXiv:2412.15115. doi: 10.48550/arXiv.2412.15115
Sonni, A. F. (2025). Digital transformation in journalism: mini review on the impact of ai on journalistic practices. Front. Commun. 10:1535156. doi: 10.3389/fcomm.2025.1535156
Strubell, E., Ganesh, A., and McCallum, A. (2020). Energy and policy considerations for modern deep learning research. Proc. AAAI Conf. Artif. Intell. 34, 13693–13696. doi: 10.1609/aaai.v34i09.7123
Wang, W., Jiao, W., Huang, J., Dai, R., Huang, J.-t., Tu, Z., et al. (2024). “Not all countries celebrate thanksgiving: On the cultural dominance in large language models,” in Proceedings of the 62nd Annual Meeting of the Association for Computational Linguistics (Volume 1: Long Papers), eds. L.-W. Ku, A. Martins, and V. Srikumar (Bangkok: Association for Computational Linguistics), 6349–6384. doi: 10.18653/v1/2024.acl-long.345
Wiatros-Motyka, M., Fulghum, N., Jones, D., Altieri, K., Black, R., Broadbent, H., et al. (2024). Global Electricity Review 2024. Creative Commons ShareAlike Attribution Licence (CC BY-SA 4.0). Available online at: https://ember-climate.org (accessed March 28, 2025).
Zhao, W. X., Zhou, K., Li, J., Tang, T., Wang, X., Hou, Y., et al. (2024). A survey of large language models. arXiv [Preprint]. arXiv:2303.18223. doi: 10.48550/arXiv.2303.18223
Keywords: sustainability, energy costs, CO2 emission, CO2 equivalent, large language model (LLM)
Citation: Dauner M and Socher G (2025) Energy costs of communicating with AI. Front. Commun. 10:1572947. doi: 10.3389/fcomm.2025.1572947
Received: 07 February 2025; Accepted: 30 April 2025;
Published: 19 June 2025.
Edited by:
Tobias Eberwein, Austrian Academy of Sciences (OeAW), AustriaReviewed by:
Patrick Murphy, Temple University, United StatesLucas Greif, Karlsruhe Institute of Technology (KIT), Germany
Copyright © 2025 Dauner and Socher. This is an open-access article distributed under the terms of the Creative Commons Attribution License (CC BY). The use, distribution or reproduction in other forums is permitted, provided the original author(s) and the copyright owner(s) are credited and that the original publication in this journal is cited, in accordance with accepted academic practice. No use, distribution or reproduction is permitted which does not comply with these terms.
*Correspondence: Maximilian Dauner, bWF4aW1pbGlhbi5kYXVuZXIwQGhtLmVkdQ==
†ORCID: Maximilian Dauner orcid.org/0009-0001-1756-7914
Gudrun Socher orcid.org/0000-0001-7211-7724