- 1Department of Mechanical and Aerospace Engineering, New York University Tandon School of Engineering, Brooklyn, NY, United States
- 2Department of Ecology, Evolution and Behavior, The Hebrew University of Jerusalem, Jerusalem, Israel
- 3Department of Technology Management and Innovation, New York University Tandon School of Engineering, Brooklyn, NY, United States
Dynamic group coordination facilitates adaptive division of labor in response to group-level changes. Yet, little is known about how it can be operationalized in online collaborations among individuals with limited information about each other. We hypothesized that simple social information about the task distribution of others can elicit emergent task allocation. We conducted an online experiment where participants analyze images of a polluted canal by freely switching between two tasks: creating keyword-based tags for images and categorizing existing tags. During the task execution, we presented experimentally manipulated information about the contrasting group-level task distributions. Participants did not change the effort allocation between the tasks when they were notified that the group is deficient in workers in the task they intrinsically prefer. By contrast, they allocated more effort to the less preferred task than they would intrinsically do when their intrinsic effort allocation counterbalances the current distribution of workers in the group. Such behavioral changes were observed more strongly among those with higher skills in the less preferred task. Our results demonstrate the possibility of optimizing group coordination through design interventions at the individual level that lead to spontaneous adaption of division of labor at the group level. When participants were provided information about the group-level task distribution, they tend to allocate more effort to the task against their intrinsic preference.
1. Introduction
In groups, division of labor often emerges through task specialization, which constitutes an efficient way to perform collective tasks (Smith, 1776). However, groups composed of specialists may not function efficiently in dynamically varying social environments, where the distribution of workers across tasks may change over time. An economical solution to efficient division of labor may be afforded by coordinated task allocation in response to changes in the operational environment, considering that adaptability is one of the most fundamental prerequisites for effective teamwork (Burke et al., 2006). Team adaptation can be realized through team situational awareness (Salas et al., 1995), which emerges from shared understanding of the current situation within teams. Combined with shared mental models of task requirement and responsibility (Salas et al., 2001) and mutual performance monitoring (McIntyre and Salas, 1995), members could spontaneously adjust their roles to meet the need of others, and consequently, teams could operationalize adaptively in the new environment (Serfaty et al., 1993; Entin and Serfaty, 1999). Thus, information on the group's needs is expected to elicit coordinated task allocation toward the balanced division of labor.
A key factor for adaptive group performance is communication (Burke et al., 2006; Mastrogiacomo et al., 2014). Through communication, groups can construct shared knowledge about the expertise of members, called transactive memory (Stewart and Stasser, 1995). With transactive memory, group members can allocate their responsibilities among tasks based on their expertise and adaptively coordinate task allocation in response to changes in the environment, based on a shared mental model (Stasser et al., 1995). For example, group members with higher skills in a task may be more likely to switch to that task when there is a deficit of people working on this task to facilitate the achievement of the collective goal. Once transactive memory is developed in a group, members can coordinate task allocation through the existing transactive memory even without further communication (Wegner et al., 1991; Wittenbaum et al., 1996, 1998; Hollingshead, 2000). For example, Littlepage et al. (2008) found no effect of communication on group performance when members already had transactive memory. However, transactive memory would be difficult to develop in the first place in groups with limited communication tools, such as online communities, or short-lived groups that do not have time to do so. Presently, it is not clear whether these groups are still able to coordinate without communication or transactive memory in response to changes in the operational environment when provided only with social information about the current task allocation.
Crowdsourcing, an online practice of enlisting a large number of people to perform micro-tasks, offers a unique experimental setting to systematically investigate group coordination. Recent studies have explored collective tasks in crowdsourcing projects, where participants spontaneously allocate themselves among different activities (Luther et al., 2009; Willett et al., 2011; Valdes et al., 2012). Common tasks performed in crowdsourcing projects are content creation and content curation. In content creation, participants act as distributed sensors to gather information. In ARTigo (www.artigo.org), for example, people tag images of artwork with the associated words, such as style and emotions, toward building a search engine for artwork. In content curation, participants act as distributed processors to extract semantic information from the large amount of data created by automated monitoring systems or human participants. In Snapshot Serengeti (www.snapshotserengeti.org), people categorize images of wildlife into species online, which helps understand the distribution of wildlife. When participants share an objective in conducting crowdsourcing projects with multiple activities, they may coordinate their task allocation as a virtual group, and consequently, overall output of the projects may increase (Dissanayake et al., 2015).
The use of crowdsourcing has been shown to be particularly advantageous in performing hypothesis-driven studies on human behavior in socio-technical settings, where computer interface can be used as a customizable and controllable stimulus to identify determinants of behavior. For example, crowdsourcing has been used to investigate risk preference (Eriksson and Simpson, 2010), performance changes with group size (Mao et al., 2016), and social dilemma in networks (Suri and Watts, 2011). These efforts have contributed to validating the high-degree of reproducibility of crowdsourcing-based behavioral studies (Rand, 2012) in agreement with traditional studies (Paolacci et al., 2010; Behrend et al., 2011; Mason and Suri, 2012; Crump et al., 2013). Thus, using crowdsourcing enables the experimenter to control the social signals provided to participants and precisely identify the specific activities associated with the labor.
Beyond its potential value in social behavior, addressing group coordination in crowdsourcing has compelling implications for our capacity to design efficient collective tasks in online communities. Just as the specific motivational factors contributing to the division of labor are presently unclear, the role of social information on group coordination in crowdsourcing remains elusive. An improved understanding of these aspects could inform design interventions for collective tasks (Feigh et al., 2012; Nov et al., 2014). Such interventions could modulate social signals to elicit the dynamic assignment of people to selected tasks toward optimized collaboration in a shared effort.
Here, we investigated the roles of social information and individual differences (Nov et al., 2013) in skill levels on spontaneous task allocation when people collaborate toward a shared objective in a crowdsourcing effort as a short-lived virtual group without the possibility of communication. Resting on the premises that humans are social animals that exhibit high behavioral plasticity in response to changes in social environments (Aronson, 1972) and that task specialization is an efficient way to perform collective tasks (West et al., 2015), we hypothesized that workers in projects that are aimed at a collective goal will distribute themselves into tasks to balance the distribution of workers across the tasks. Specifically, when the number of workers on a certain task is perceived to be deficient, there should be an increased tendency of others to switch to that task. Further, we hypothesized that people who display higher skill levels in the deficient task would show stronger behavioral tendency to choose that task without knowing skill levels of others. We tested our hypotheses by providing experimentally manipulated social information about the distribution of workers between contrasting tasks (creating or curating content), using a crowdsourcing project (Laut et al., 2014) as the experimental setup.
2. Materials and Methods
2.1. Infrastructure
The Brooklyn Atlantis citizen science project was designed to monitor the environmental health of the Gowanus Canal (Brooklyn, NY, USA), one of the most polluted bodies of water in the U.S. On the web-based platform, participants access the images taken by an aquatic robot and can create tags on any notable objects in the images. We modified the Brooklyn Atlantis infrastructure to allow participants to perform two tasks: an image tagging task and a tag categorization task (Figure 1). In the image tagging task, participants are presented with an image, and are instructed to select any notable object using a mouse and type the description using a keyboard. They are allowed to create as many image tags as they want on the same image. In the tag categorizing task, participants are presented with an existing tag associated with a particular image, created by other participants. When participants hover the mouse over the tag, they are displayed a portion of the image associated with the tag text. To assign a category to a tag, participants drag the tag text to one of the categories presented at the bottom of the screen, such as “nature,” “waste/debris,” and “human-made structure.” Each tag can belong to only one category.
The platform records the number of images participants tagged and categorized. Detailed instructions for each task are included on the task screens. Additionally, the application displays to participants the number of other people currently working each of the two tasks.
2.2. Experiment
We recruited U.S. participants from Amazon Mechanical Turk. A flow chart in Figure 2 summarizes the experimental procedure. First, participants were presented with the collective objective: a short paragraph was displayed to them, explaining that the images and tags come from an environmental monitoring project and that participation will aid the project's effort in assembling an image repository. This was aimed at increasing participants' motivations by framing the crowdsourcing effort as a scientific activity with societal benefits, and laying a collective objective to be accomplished through both the tagging and categorization tasks. The participants were informed that monetary compensation did not depend on either the individual's or the group's output. Then, participants were asked to complete a 4-min online tutorial on both the tagging and categorizing tasks (Figure 1). After completing the tutorial, participants proceeded by clicking a button to a 3-min trial session, in which they familiarized themselves with the application. During this session, participants were allowed to switch freely between the two tasks by clicking “Switch Tasks” or continue to the next image by clicking “Next.”
After the trial session, participants proceeded by clicking a button to the main experimental session in which they were instructed to work on their tasks of choice for 8 min, during which they could freely switch between tasks or continue working on the same task. The first task displayed on the screen was randomized in the trial and main sessions, which controlled for any bias caused by participants simply continuing in the first task they encountered during the experiment.
To investigate spontaneous task allocation in response to the task choices of others, we displayed experimentally manipulated information about the number of other participants performing the tagging and categorizing tasks throughout the main session (Table 1). Each participant was randomly assigned to one of four experimental conditions: In the control condition, participants were given no social information on how many people were engaged in either task. In the “categorizers deficient” condition, participants were informed that 14 people were tagging and 1 person was categorizing. In the “taggers deficient” condition, they were informed that 1 person was tagging and 14 people were categorizing. In the “balanced” condition, they were informed that seven people were tagging and seven people were categorizing. The displayed numbers included the participant. When the participant switched the task, the numbers were adjusted accordingly to keep the total number of other participants constant throughout the main session.
Participants were automatically logged out of the system when they were inactive for 10 min in the main session or for 30 min in the entire experiment. As a result, we had 94 participants in the control condition, 121 participants in the “categorizers deficient” condition, 57 participants in the “balanced” condition, and 109 participants in “taggers deficient” condition, totaling 381 participants. Upon finishing the whole session, participants were paid $2.50. Each participant was allowed to participate in the experiment only once.
This study was exempt from an institutional review board and did not require an approval by an ethics committee, as the methods and experimental protocols fell under the category of exempt research by University Committee on Activities Involving Human Subjects. The informed consent was obtained from all subjects to participate in the study.
2.3. Analysis
First, we investigated whether the social information presented to participants changed their choice of tasks. We used a generalized linear model with treatment (control, “categorizers deficient,” “balanced,” and “taggers deficient”) as a predictor variable and the numbers of images tagged and categorized by each participant as response variables, specifying binomial errors with a logit link [R package lme4 v.1.1-12 (Bates et al., 2015)]. The effect of each treatment was compared to the control condition using a Wald test, whose statistic is equal to a z-score. The statistical significance was adjusted for multiple comparisons using Dunnett's test [R package multcomp v.1.4-6 (Hothorn et al., 2008)]. Participants who did not switch the task during the entire session (i.e., the trail and main sessions) were excluded from the analysis to eliminate the possibility that they did not understand the functionality of the platform (n = 13 in control, 24 in “categorizers deficient,” 8 in “balanced,” and 15 in “taggers deficient”). Subsequently, the analysis was conducted using 321 participants in total, with 81 participants in the control condition, 97 participants in the “categorizers deficient” condition, 49 participants in the “balanced” condition, and 94 participants in the “taggers deficient” condition.
Next, we investigated whether the spontaneous task allocation was influenced by the participant's skill levels of performing one task relative to the other. We assessed individual skill levels as the speed of tagging relative to that of categorizing, considering that time is a resource that is limited equally to all participants during the task. Specifically, using the data in the trial session, we calculated the proportion of the speed of tagging per image over the sum of the speeds of tagging and categorizing for each individual. The relative speed of tagging ranges between 0 and 1, with large values indicating that participants perform the tagging task faster than the categorizing task per image. We also checked the consistency of the relative speed of tagging over time by comparing between the trial session and the main session in the control condition (i.e., no social information on others) using Pearson's correlation test. To test the effect of the relative speed of tagging on task allocation, we used a generalized linear model, specifying binomial errors with a logit link. As predictor variables, we specified treatment and participant's relative speed of tagging as main effects and the interaction of the two. Significance of the interaction term was checked using a likelihood ratio test by comparing the fit of the model with and without the interaction term. Significance of each coefficient was checked using a Wald test with Bonferroni correction for multiple comparisons (Zuur et al., 2009).
Finally, we explored the rationale of the potential influence of the relative skill levels on spontaneous task allocation without a possibility of communication. Specifically, we investigated whether the relative skill level of an individual was associated with the absolute skill level of the individual among all participants. We tested for correlation between the relative speed of tagging and the absolute speed of tagging among all participants in the trial session using Pearson's correlation test.
3. Results
In the 3-min trial session, where no social information was provided, participants (n = 321) created 10.3 ± 5.5 (mean ± standard deviation) tags and categorized 25.7 ± 14.7 tags. They spent 87.6 ± 35.5 s on tagging and 92.4 ± 35.5 s on categorizing. As a result, participants created 0.12 ± 0.05 tags/s and categorized 0.27 ± 0.09 tags/s, spending 32.4 ± 18.6% of time on tagging. In the 8-min main session, participants in the control condition (n = 81) created 29.8 ± 16.8 tags in 209.7 ± 97.7 s and categorized 83.4 ± 40.5 tags in 270.3 ± 97.7 s. As a result, they created 0.14 ± 0.05 tags/s and categorized 0.31 ± 0.09 tags/s.
In the 8-min main session, the social information presented to participants influenced the choice of task (Figure 3). When participants did not receive any social information, they performed the categorizing task more often than the tagging task (73.7% on average, z = 43.445, p < 0.001), indicating that participants were intrinsically biased in task choice toward categorizing. However, when participants received social information that taggers were deficient in the group (“taggers deficient” condition), participants changed their actions to counterbalance the distribution of workers by performing a tagging task more often compared to the control condition (z = 10.855, p < 0.001). Similarly, when participants were informed that others were distributed evenly between the tasks in the group (“balanced” condition), they changed from the intrinsic task choice that were biased toward categorizing and performed a tagging task more often compared to the control condition (z = 3.702, p < 0.001). By contrast, when participants were notified that group distribution between the tasks was strongly biased against the intrinsic task choice (“categorizers deficient” condition), they did not change their actions compared to the control condition (z = −1.203, p = 0.485).
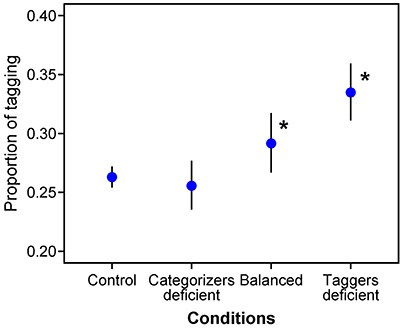
Figure 3. Proportion of the number of tagging acts over the total number of tagging + categorizing acts in different conditions: control condition (no social information), “categorizers deficient” condition (14 taggers and 1 categorizer), “balanced” condition (7 taggers and 7 categorizers), and “taggers deficient” condition (1 tagger and 14 categorizers). Asterisks indicate significant difference (p < 0.05) compared to the control condition. Vertical lines indicate 95% confidence intervals.
Participants' relative speed of tagging was consistent across trials (Figure 4). The relative speed of tagging was positively correlated between the trial session and the main session in the control condition (Person's correlation test, r = 0.368, t = 3.522, df = 79, p < 0.001). Further, the relative speed of tagging within a participant was highly correlated with the absolute speed of tagging among all participants in the trial session (r = 0.511, t = 10.614, df = 319, p < 0.001).
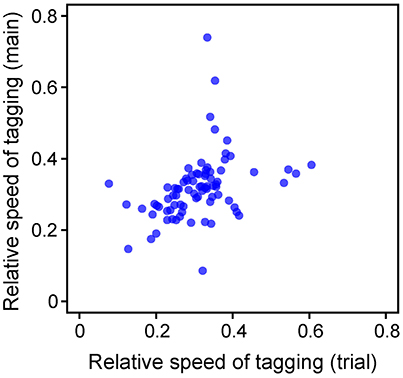
Figure 4. Participant's relative speed of tagging in the trial session and the main session. Relative speed of tagging was calculated as the proportion of tagging speed over the total speed (tagging and categorizing).
Spontaneous task allocation was determined by both the treatment and participant's relative speed of tagging (Figure 5). Overall, we found a significant interaction between treatment and the relative speed of tagging (Likelihood ratio test, , adjusted p < 0.001), indicating that relationship between task choice and relative speed of tagging depended on the treatments. When the “categorizers deficient” condition was compared to the control condition, there was no significant interaction between conditions and the relative speed of tagging (z = −0.446, adjusted p > 0.999). By contrast, when the “balanced” condition was compared to the control condition, the relative speed of tagging showed a positive interaction with condition (z = 4.539, adjusted p < 0.001), indicating that participants with higher speeds of tagging relative to categorizing were more likely to perform the tagging task, compared to the participants in the control condition. A similar relationship was found in the “taggers deficient” condition, where participants with higher speeds of tagging were more likely to switch to a tagging task (z = 7.015, adjusted p < 0.001).
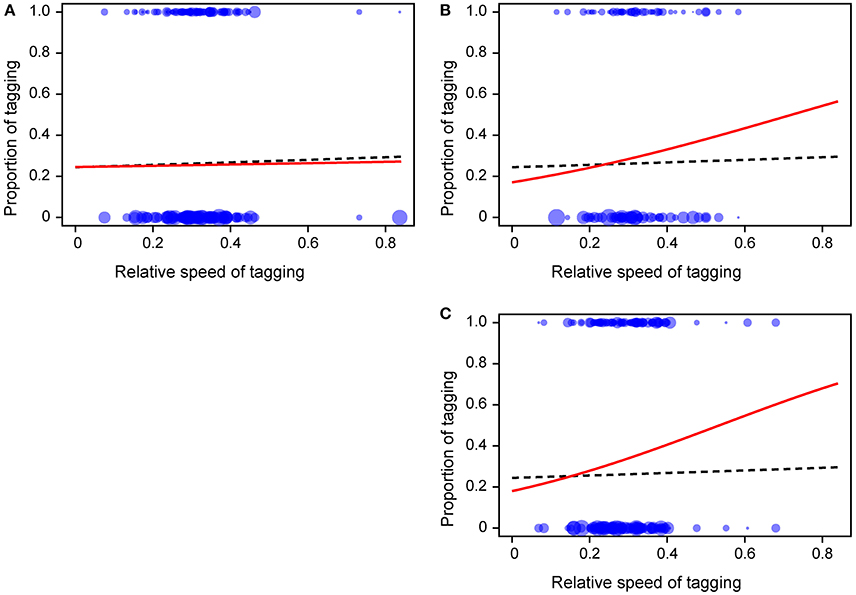
Figure 5. Effects of the relative speed of tagging on task choice. (A) “Categorizers deficient” condition, (B) “balanced” condition, (C) “taggers deficient” condition. Dashed lines represent the control condition, and solid lines represent the treatment conditions with some form of social information. Each point represents the number of images tagged (top) and categorized (bottom) by each individual. The point size is proportional to the number.
4. Discussion
The results confirm our hypothesis that people spontaneously allocate their tasks toward balancing the division of labor in response to social information about the worker distribution when they perform a collective task. Specifically, when people were notified that the group deficits workers in the categorizing task (i.e., the “categorizers deficient” condition), they did not change their intrinsic task choice, which was biased toward categorizing. By contrast, they allocated more effort to the less preferred task than they would intrinsically do when their intrinsic task choice counterbalances the current distribution of workers in the group (i.e., the “taggers deficient” and “balanced” conditions). Therefore, our results suggest that the social information elicits spontaneous task allocation when people are working toward a collective goal.
Further, in agreement with our expectations, the findings revealed that the extent of the observed behavioral changes depended on the participant's skill levels. In both the “taggers deficient” and “balanced” conditions, a stronger behavioral change toward tagging against their intrinsic inclination to categorizing was found in participants who exhibited higher skill levels in tagging compared to categorizing (i.e., faster speed of tagging relative to categorizing). By contrast, participants with low tagging speed were less likely to change their task choice from their intrinsic inclination to categorizing in the same situations. In our study, it is plausible that participants with low tagging speed exhibited limited changes in task allocation toward tagging as they assumed that they would contribute less to the group output if they changed their behavior. Therefore, people working as a group toward a collective goal are likely to use their skill level in each task in deciding task allocation, possibly to optimize group performance when direct communication is not possible.
Naturally, the amount of information curated should be similar to the amount of information created toward maximizing group output, and participants in a collective task changed their task choices to the expected direction. When they were not able to communicate to assess their own skill levels relative to others, participants used their skill levels of one task relative to the other as a reference for changing their actions. This is seemingly counterintuitive, since knowledge of the relative skills of a participant should not constitute a valid appraisal of the absolute skills of the participant in a group. However, the relative speed of tagging of each participant was highly correlated with the absolute speed of tagging among other participants, suggesting that self-assessment may offer an efficient indicator for the contribution to the group in collective tasks. Thus, in agreement with our hypothesis, participants with high skill levels in a specific task tended to contribute more to it as a compensatory strategy to increase group output (Salas et al., 2015). Further study is needed to test whether participants would exhibit more adaptive behavioral responses to the social information when provided with information about the absolute skill levels of participants within a group.
Our results show that the social information elicited behavioral change even in the “balanced” condition, where people performed the tagging task more often than they would intrinsically do, such that the efforts were evenly distributed between the tasks at a group level. However, social information on the task distribution might exert a weaker influence in larger groups, as people could feel negligible contributions of changing their behavior to the group. Responses to social information would also hinge on the rewarding schemes. In contrast to our experiment, rewarding group performance might facilitate behavioral changes against the individual preference toward a more balanced division of labor, whereas rewarding individual performance might hinder such behavioral changes by eliciting selfish behavior.
Our study was grounded in the use of crowdsourcing as an experimental platform to investigate changes in division of labor in virtual groups. This platform was pivotal in testing our hypothesis, by providing the possibility to systematically select social interactions, precisely identify tasks, and present the social information in real time. Beyond these methodological advantages, crowdsourcing allows to reach a diverse background of participants and optimizes cost- and time-effectiveness, in comparison with more traditional experimental settings (Mason and Suri, 2012). However, the use of crowdsourcing could also raise concerns about the generalizability of our findings, which are based on experiments where social influences occurred only in a virtual setting and the users were paid to participate. While it could be proposed that these factors might change motivations of participants, potentially eliciting more selfish behavior (Kohn, 1993), recent studies have indicated that paid participants in crowdsourcing exhibit the same heuristics in behavior as those in traditional methods (Paolacci et al., 2010) and that data quality is not affected by the amount of payment (Mason and Watts, 2009). These concerns are further assuaged by our findings that participants indeed exhibited spontaneous task allocation in response to social information and expertise to the expected direction as adaptive groups would do, in a similar way that group members lend support to one another if necessary (Porter et al., 2003).
Although our results demonstrate that people can utilize simple social information to balance the division of labor, they do not undermine the importance of communication in adaptive group coordination. Numerous studies demonstrate that communication enhances group performance by allowing group members to identify expertise and knowledge of others (Stasser et al., 1995; Argote and Olivera, 1999) and allocating task responsibilities in such a way to utilize their expertise (Hackman, 1987; Littlepage et al., 1995, 1997; Stempfle et al., 2001). Further, communication can enhance adaptive group coordination in the dynamic environment by helping members to learn from each other and foster team situational awareness (West, 2004). Thus, communication is an important factor for groups to capitalize the synergetic potential. However, laying the foundation for direct communication is challenging in online communities with limited communication tools. In these communities, performance could be enhanced by implementing information sharing mechanisms, in which people can share their knowledge (Kankanhalli et al., 2005; Espinosa et al., 2007; Robert et al., 2008) and engage in discussion (Alavi and Leidner, 1999). When it is practically difficult or impossible to implement these systems, due to technical and privacy-related issues, it may still be possible to enhance group performance by providing the information about the past actions of others, considering that people can infer expertise of others from it (Wegner et al., 1991). Further study is needed to scrutinize the influences of different types of information on group dynamics (Stieglitz and Dang-Xuan, 2013), with a metric that accurately assesses group performance.
Our study shows that, overall, participants modified their contributions to balance the task distribution in the group. Such group awareness in a virtual setting is also seen in many other large-scale online environments, such as Wikipedia and Stack Overflow, where people help each other as virtual groups with no seeming reward (McLure-Wasko and Faraj, 2000, 2005; Forte et al., 2009). Our findings suggest the possibility of enhancing group output through design interventions that address individual behavioral plasticity to ultimately modulate spontaneous adaption of collective dynamics (Feigh et al., 2012; Barg-Walkow and Rogers, 2016). For example, by exposing workers to social information about a distribution of other workers, the collective task designer or administrator could dynamically influence workers' task choice in a desired direction. Considering that groups often perform better when they are given autonomy in decision making (Hackman and Oldham, 1980), such design interventions can help enhance group management and output.
Author Contributions
DD contributed to designing the study, conducting the experiment, and revising the manuscript. SN contributed to analyzing the data, drafting the manuscript, and revising the manuscript. JH and GB contributed to revising the manuscript. MP and ON contributed to designing the study and revising the manuscript.
Funding
This work was supported by the National Academies Keck Futures Initiative under grant CB9 and the National Science Foundation under grant numbers BCS 1124795, CBET 1547864, CMMI 1644828, and IIS 1149745.
Conflict of Interest Statement
The authors declare that the research was conducted in the absence of any commercial or financial relationships that could be construed as a potential conflict of interest.
References
Alavi, M., and Leidner, D. E. (1999). Knowledge management systems: issues, challenges, and benefits. Commun. Assoc. Inform. Syst. 1, 1–28.
Argote, L., and Olivera, F. (1999). “Orgnizational learning and new product development: CORE processes,” in Shared Cognition in Organization: The Management of Knowledge, eds L. L. Thompson, J. M. Levine, and D. M. Messick (Mahwah, NJ: Erlbaum), 297–325.
Barg-Walkow, L. H., and Rogers, W. A. (2016). The effect of incorrect reliability information on expectations, perceptions, and use of automation. Hum. Factors 58, 242–260. doi: 10.1177/0018720815610271
Bates, D., Mächler, M., Bolker, B., and Walker, S. (2015). Fitting linear mixed-effects models using lme4. J. Stat. Softw. 67, 1–48. doi: 10.18637/jss.v067.i01
Behrend, T. S., Sharek, D. J., Meade, A. W., and Wiebe, E. N. (2011). The viability of crowdsourcing for survey research. Behav. Res. Methods 43, 800–813. doi: 10.3758/s13428-011-0081-0
Burke, C. S., Stagl, K. C., Salas, E., Pierce, L., and Kendall, D. (2006). Understanding team adaptation: a conceptual analysis and model. J. Appl. Psychol. 91, 1189–1207. doi: 10.1037/0021-9010.91.6.1189
Crump, M. J., McDonnell, J. V., and Gureckis, T. M. (2013). Evaluating Amazon's Mechanical Turk as a tool for experimental behavioral research. PLoS ONE 8:e57410. doi: 10.1371/journal.pone.0057410
Dissanayake, I., Zhang, J., and Gu, B. (2015). Task division for team success in crowdsourcing contests: resource allocation and alignment effects. J. Manag. Inform. Syst. 32, 8–39. doi: 10.1080/07421222.2015.1068604
Entin, E. E., and Serfaty, D. (1999). Adaptive team coordination. Hum. Factors 41, 312–325. doi: 10.1518/001872099779591196
Eriksson, K., and Simpson, B. (2010). Emotional reactions to losing explain gender differences in entering a risky lottery. Judgm. Decis. Making 5, 159–163.
Espinosa, J., Slaughter, S., Kraut, R., and Herbsleb, J. (2007). Team knowledge and coordination in geographically distributed software development. J. Manag. Inform. Syst. 24, 135–169. doi: 10.2753/MIS0742-1222240104
Feigh, K. M., Dorneich, M. C., and Hayes, C. C. (2012). Toward a characterization of adaptive systems: a framework for researchers and system designers. Hum. Factors 54, 1008–1024. doi: 10.1177/0018720812443983
Forte, A., Larco, V., and Bruckman, A. (2009). Decentralization in wikipedia governance. J. Manag. Inform. Syst. 26, 49–72. doi: 10.2753/MIS0742-1222260103
Hackman, R. J. (1987). “The design of work groups,” in Handbook of Organizational Behavior, ed J. W. Lorsch (Englewood Cliffs, NJ: Prentice Hall), 315–339.
Hollingshead, A. B. (2000). Perceptions of expertise and transactive memory in work relationships. Group Process. Intergr. Relat. 3, 257–267. doi: 10.1177/1368430200033002
Hothorn, T., Bretz, F., and Westfall, P. (2008). Simultaneous inference in general parametric models. Biometr. J. 50, 346–363. doi: 10.1002/bimj.200810425
Kankanhalli, A., Tan, B. C. Y., and Wei, K.-K. (2005). Contributing knowledge to electronic knowledge repositories: an empirical investigation. MIS Q. 29, 113–143. doi: 10.2307/25148670
Laut, J., Henry, E., Nov, O., and Porfiri, M. (2014). Development of a mechatronics-based citizen science platform for aquatic environmental monitoring. IEEE/ASME Trans. Mechatr. 19, 1541–1551. doi: 10.1109/TMECH.2013.2287705
Littlepage, G., Robison, W., and Reddington, K. (1997). Effects of task experience and group experience on group performance, member ability, and recognition of expertise. Organ. Behav. Hum. Decis. Process. 69, 133–147. doi: 10.1006/obhd.1997.2677
Littlepage, G. E., Hollingshead, A. B., Drake, L. R., and Littlepage, A. M. (2008). Transactive memory and performance in work groups: specificity, communication, ability differences, and work allocation. Group Dyn. 12, 223–241. doi: 10.1037/1089-2699.12.3.223
Littlepage, G. E., Schmidt, G. W., Whisler, E. W., and Frost, A. G. (1995). An input-process-output analysis of influence and performance in problem-solving groups. J. Pers. Soc. Psychol. 69, 877–889. doi: 10.1037/0022-3514.69.5.877
Luther, K., Counts, S., Stecher, K. B., Hoff, A., and Johns, P. (2009). “Pathfinder: an online collaboration environment for citizen scientists,” in Proceedings of the 27th International Conference on Human Factors in Computing Systems - CHI '09 (New York, NY: ACM Press), 239.
Mao, A., Mason, W., Suri, S., and Watts, D. (2016). An experimental study of team size and performance on a complex task. PLoS ONE 11:e0153048. doi: 10.1371/journal.pone.0153048
Mason, W., and Suri, S. (2012). Conducting behavioral research on Amazon's Mechanical Turk. Behav. Res. Methods 44, 1–23. doi: 10.3758/s13428-011-0124-6
Mason, W. A., and Watts, D. J. (2009). “Financial incentives and the performance of crowds,” in Proceedings of the ACM SIGKDD Workshop on Human Computation (New York, NY), 77–85. doi: 10.1145/1600150.1600175
Mastrogiacomo, S., Missonier, S., and Bonazzi, R. (2014). Talk before it's too late: reconsidering the role of conversation in information systems project management. J. Manag. Inform. Syst. 31, 44–78. doi: 10.2753/MIS0742-1222310103
McIntyre, R. M., and Salas, E. (1995). “Measuring and managing for team performance: emerging principles from complex environment,” in Team Effectiveness and Decision Making in Organizations, eds R. Guzzo and E. Salas (San Francisco, CA: Jossey-Bass), 9–45.
McLure-Wasko, M., and Faraj, S. (2000). “It is what one does”: why people participate and help others in electronic communities of practice. J. Strat. Inform. Syst. 9, 155–173. doi: 10.1016/S0963-8687(00)00045-7
McLure-Wasko, M., and Faraj, S. (2005). Why should I share? Examining social capital and knowledge contribution in electronic networks of practice. MIS Q. 29, 35–57. doi: 10.2307/25148667
Nov, O., Arazy, O., and Anderson, D. (2014). Scientists@home: what drives the quantity and quality of online citizen science participation? PLoS ONE 9:e90375. doi: 10.1371/journal.pone.0090375
Nov, O., Arazy, O., López, C., and Brusilovsky, P. (2013). “Exploring personality-targeted UI design in online social participation systems,” in Proceedings of the SIGCHI Conference on Human Factors in Computing Systems - CHI '13 (New York, NY: ACM Press), 361–370. doi: 10.1145/2470654.2470707
Paolacci, G., Chandler, J., and Ipeirotis, P. G. (2010). Running experiments on Amazon Mechanical Turk. Judgm. Decis. Making 5, 411–419.
Porter, C. O., Hollenbeck, J. R., Ilgen, D. R., Ellis, A. P., West, B. J., and Moon, H. K. (2003). Backing up behaviors in teams: the role of personality and legitimacy of need. J. Appl. Psychol. 88, 391–403. doi: 10.1037/0021-9010.88.3.391
Rand, D. G. (2012). The promise of Mechanical Turk: how online labor markets can help theorists run behavioral experiments. J. Theor. Biol. 299, 172–179. doi: 10.1016/j.jtbi.2011.03.004
Robert, L. P., Dennis, A. R., and Ahuja, M. K. (2008). Social capital and knowledge integration in digitally enabled teams. Inform. Syst. Res. 19, 314–334. doi: 10.1287/isre.1080.0177
Salas, E., Cannon-Bowers, J., Fiore, J. A., and Stout, R. J. (2001). “Cue-recognition training to enhance team situation awareness,” in New Trends in Cooperative Activities: Understanding System Dynamics in Complex Environments, eds M. McNeese, E. Salas, and M. Endsley (Santa Monica, CA: Human Factors and Ergonomics Society), 169–190.
Salas, E., Grossman, R., Hughes, A. M., and Coultas, C. W. (2015). Measuring team cohesion: observations from the science. Hum. Factors 57, 365–374. doi: 10.1177/0018720815578267
Salas, E., Prince, C., Baker, D. P., and Shrestha, L. (1995). Situation awareness in team performance: implications for measurement and training. Hum. Factors 37, 123–136. doi: 10.1518/001872095779049525
Serfaty, D., Entin, E. E., and Volpe, C. (1993). “Adaptation to stress in team decision-making and coordination,” in Proceedings of the Human Factors and Ergonomics Society, (Santa Monica, CA: Human Factors and Ergonomics Society), 1228–1232. doi: 10.1177/154193129303701806
Stasser, G., Stewart, D. D., and Wittenbaum, G. M. (1995). Expert roles and information exchange during discussion: the importance of knowing who knows what. J. Exp. Soc. Psychol. 31, 244–265. doi: 10.1006/jesp.1995.1012
Stempfle, J., Hubner, O., and Badke-Schaub, P. (2001). A functional theory of task role distribution in work groups. Group Process. Intergr. Relat. 4, 138–159. doi: 10.1177/1368430201004002005
Stewart, D. D., and Stasser, G. (1995). Expert role assignment and information sampling during collective recall and decision making. J. Pers. Soc. Psychol. 69, 619–628. doi: 10.1037/0022-3514.69.4.619
Stieglitz, S., and Dang-Xuan, L. (2013). Emotions and information diffusion in social media—sentiment of microblogs and sharing behavior. J. Manag. Inform. Syst. 29, 217–248. doi: 10.2753/MIS0742-1222290408
Suri, S., and Watts, D. J. (2011). Cooperation and contagion in web-based, networked public goods experiments. PLoS ONE 6:e16836. doi: 10.1371/journal.pone.0016836
Valdes, C., Ferreirae, M., Feng, T., Wang, H., Tempel, K., Liu, S., et al. (2012). “A collaborative environment for engaging novices in scientific inquiry,” in Proceedings of the 2012 ACM International Conference on Interactive Tabletops and Surfaces - ITS '12 (New York, NY: ACM Press), 109.
Wegner, D. M., Erber, R., and Raymond, P. (1991). Transactive memory in close relationships. J. Pers. Soc. Psychol. 61, 923–929. doi: 10.1037/0022-3514.61.6.923
West, M. A. (2004). Effective Teamwork: Practical Lessons From Organizational Research. Oxford: Blackwell.
West, S. A., Fisher, R. M., Gardner, A., Kiers, E. T., and Mccutcheon, J. P. (2015). Major evolutionary transitions in individuality. Proc. Natl. Acad. Sci. U.S.A. 112, 10112–10119. doi: 10.1073/pnas.1421402112
Willett, W., Heer, J., Hellerstein, J., and Agrawala, M. (2011). “CommentSpace: structured support for collaborative visual analysis,” in Proceedings of the 2011 Annual Conference on Human Factors in Computing Systems - CHI '11 (New York, NY: ACM Press), 3131.
Wittenbaum, G. M., Stasser, G., and Merry, C. J. (1996). Tacit coordination in anticipation of small group task completion. J. Exp. Soc. Psychol. 32, 129–152. doi: 10.1006/jesp.1996.0006
Wittenbaum, G. M., Vaughan, S. I., and Stasser, G. (1998). “Coordination in task-performning groups,” in Theory and Reserch on Small Groups, eds R. S. Tindale, L. Heath, J. Edwards, E. J. Posavac, F. B. Bryant, J. Myers, Y. Suarez-Balcazar, and E. Henderson-King (New York, NY: Plenum Press), 177–204.
Keywords: behavioral plasticity, citizen science, collective behavior, content creation, content curation, crowdsourcing, division of labor
Citation: Nakayama S, Diner D, Holland JG, Bloch G, Porfiri M and Nov O (2018) The Influence of Social Information and Self-expertise on Emergent Task Allocation in Virtual Groups. Front. Ecol. Evol. 6:16. doi: 10.3389/fevo.2018.00016
Received: 20 December 2017; Accepted: 06 February 2018;
Published: 21 February 2018.
Edited by:
Simon Garnier, New Jersey Institute of Technology, United StatesReviewed by:
Mehdi Moussaid, Max Planck Institute for Human Development (MPG), GermanyTakao Sasaki, Arizona State University, United States
Copyright © 2018 Nakayama, Diner, Holland, Bloch, Porfiri and Nov. This is an open-access article distributed under the terms of the Creative Commons Attribution License (CC BY). The use, distribution or reproduction in other forums is permitted, provided the original author(s) and the copyright owner are credited and that the original publication in this journal is cited, in accordance with accepted academic practice. No use, distribution or reproduction is permitted which does not comply with these terms.
*Correspondence: Maurizio Porfiri, bXBvcmZpcmlAbnl1LmVkdQ==
Oded Nov, b25vdkBueXUuZWR1