- 1Department of Microbiology and Immunology, Montana State University, Bozeman, MT, United States
- 2Department of Infectious Disease Epidemiology, Faculty of Medicine, School of Public Health, Imperial College London, St. Mary's Hospital Campus, London, United Kingdom
- 3Center for the Ecology of Infectious Disease, University of Georgia, Athens, GA, United States
Environmental persistence of zoonotic pathogens is a key trait that influences the probability of zoonotic spillover. Pathogen survival outside of the host determines the window available for contact with the new recipient host species and the dose of pathogen available to that host. The longer a pathogen survives in the environment, the more disconnected the reservoir and recipient hosts can be in space and time, and the more likely that an infective dose will be available to recipient hosts. Therefore, environmental persistence is a key parameter for mechanistic models needed to predict pathogen spillover. Avian influenza can be transmitted from wildlife to poultry and people in part due to its ability to persist in the environment. Considerable work has been done to quantify trends in avian influenza persistence across environmental conditions, often published in separate studies with separate datasets. In this paper, we quantify the trends and variability of avian influenza viral persistence across environmental conditions by collating disjoint experimental data on viral particle persistence in water across many studies and a range of environmental conditions. The collated data represent 120 estimates from three different studies of the decay rates of highly pathogenic avian influenza H5N1 (90 estimates from Asia and 30 from Europe) in response to temperature, pH, and salinity. We analyzed these data with a Bayesian model to control for biases with random effects and used experimental replicates and R2 estimates of the publication's regression procedures as statistical weights. We found temperature significantly decreases persistence of H5N1 virus in water, and this effect is stronger than that of salinity alone. Salinity interacts with temperature and probably drives the most contrasting persistence scenarios between cold-saline and warm-saline water bodies, where highest and lowest persistence times could occur respectively. Our work provides needed parameters for models that examine the risk of spillover of avian influenza viruses.
Introduction
The capacity of microorganisms to survive in the environment has fundamental consequences for their ecology, epidemiology, and ability to transmit between individuals and species (Ewald, 1987; Kuris et al., 2014; Plowright et al., 2017). For instance, the time over which organisms can remain infectious and available for transmission can be influenced by environmental characteristics (Ewald, 1987; Walther and Ewald, 2004; Martin et al., 2015, 2017; Plowright et al., 2017). Environmental persistence times can drive temporal patterns in epidemics (Lipsitch and Viboud, 2009; Shaman and Kohn, 2009; Shaman et al., 2010; Satterfield et al., 2017), the duration and magnitude of epidemics (Walther and Ewald, 2004; Rohani et al., 2009), and virulence in reservoir hosts (Walther and Ewald, 2004).
Avian influenza viruses (AIV, Orthomyxoviridae: Inlfuenzavirus A) form a diverse group with a very broad host range spanning taxonomic orders and classes, including humans, in which these viruses have caused pandemics. Among the different genera of influenza viruses, only Influenza A viruses have had the potential to become pandemic in humans (Taubenberger and Kash, 2010). Most influenza subtypes pathogenic to humans originate in birds and swine. The latter are considered an ideal recombination vessel for influenza variants of diverse origins (Webster et al., 1992). In aquatic birds (Anseriformes and Charadriiformes), AIV replicate in and are shed from the digestive tract allowing waterborne transmission (Webster et al., 1978; Hinshaw and Webster, 1982; Markwell and Shortridge, 1982). In humans, swine and other mammal models, influenza viruses replicate in the upper or lower respiratory tract, owing to the presence of the necessary sialic acid receptors, favoring airborne transmission (Kuiken et al., 2006; Taubenberger and Kash, 2010; Lipsitch et al., 2016). For this reason, studies of the environmental persistence of influenza viruses pathogenic to humans tend to focus on airborne transmission (Weber and Stilianakis, 2008; Shaman and Kohn, 2009). However, the environmental factors that are more relevant for AIV spillover and consequent adaptation to poultry, swine and humans are more likely to be those affecting waterborne survival.
The most important zoonotic subtypes of AIV belong to the highly pathogenic lineage, more specifically the subtypes with hemagglutinin proteins 5 and 7. These viruses are thought to originate in low and mildly pathogenic AIV from aquatic birds after several passages in domestic poultry (Swayne and Suarez, 2000; Swayne and Pantin-Jackwood, 2006). Gallinaceous birds experience pathogenic systemic infections, whereas aquatic birds infected with these viruses remain asymptomatic (Swayne and Thomas, 2009). Of the two known highly pathogenic subtypes (H5 and H7), all fatal human cases but one, have been caused by the H5N1 Eurasian lineage (Swayne and Thomas, 2009). High-mortality zoonotic outbreaks of H5N1 have occurred after close interactions between poultry and humans (Perdue et al., 2000; Peiris et al., 2004; Steensels et al., 2005; Woo et al., 2006; Abulreesh et al., 2007; Park and Glass, 2007).
While low-pathogenic AIV infect both the gastrointestinal and respiratory tract of water birds, highly pathogenic AIV have adapted to bind and replicate in the respiratory tract of its recipient hosts, a critical characteristic for zoonotic transmission (Lipsitch et al., 2016). Regardless of their adaptations for direct transmission among less aquatic hosts (Brown et al., 2009), highly pathogenic AIV are capable of spilling back to and spreading among wild aquatic birds (Ellis et al., 2004; Gaidet et al., 2008). Such re-adaptation to aquatic birds whilst retaining high pathogenicity in poultry has been observed in the Eurasian lineage of H5N1 (Buisch et al., 1984; Swayne et al., 1999). The capacity to re-establish in wild aquatic bird populations facilitates zoonotic outbreaks and spread in areas otherwise less accessible to domestic poultry (Gilbert et al., 2006). For this reason, activities such as bird feeding and swimming in water inhabited by aquatic birds may increase the risk of H5N1 spillover (Artois et al., 2009; Bright et al., 2016). Consequently, better understanding the processes that facilitate transmission of influenza A viruses within avian hosts and to humans is critical for global health (Stallknecht et al., 2010; Lipsitch et al., 2016).
Previous attempts to quantify the environmental stability of AIV in water have shown that temperature, salinity, and pH are important characteristics that determine how long AIV remain infectious (Stallknecht et al., 1990a,b; Brown et al., 2007, 2009; Domanska-Blicharz et al., 2010; Dalziel et al., 2016). However, there is still poor consensus as to whether these variables have similar effects on environmental persistence across AIV subtypes or strains. Reasons for the lack of consensus might be as follows: (1) persistence times among strains of the same subtype vary as much as persistence times among subtypes (e.g., Stallknecht et al., 1990a, 2010; Brown et al., 2014); (2) biases exist in the studied subtypes and environmental conditions represented in laboratory studies (Dalziel et al., 2016); and (3) statistical estimates and laboratory settings are inadequately reported for the majority of environmental persistence studies (Dalziel et al., 2016). Of the zoonotic influenza A subtypes, H5N1 has been studied more frequently owing to high mortality events in eastern and southeast Asia, and thus there is better knowledge of the variability of responses to different environmental factors. Meta-analyses of studies involving a number of influenza strains have examined effects of water temperature, salinity, pH and between study variability on low pathogenicity AIV subtypes (Dalziel et al., 2016); the effects of water temperature, salinity and pH on disease dynamics within natural reservoirs (Rohani et al., 2009); or have focused on airborne persistence and infectivity (Shaman and Kohn, 2009; Myatt et al., 2010; Shaman et al., 2010) on fomites (Thomas et al., 2008; Dublineau et al., 2011). The latter two analyses, however, are more relevant for viruses that have already adapted to human hosts. As a result, the broader problem of identifying common drivers of environmental persistence across potentially zoonotic influenza strains remains (Horm et al., 2012).
Here we present a meta-analysis on the joint effects of temperature, pH, and salinity on the environmental persistence of many strains of highly pathogenic AI H5N1 virus from multiple avian hosts. Using published data, we present the first attempt to identify general trends of virus-environment interactions in a unified model for one of the most relevant potentially zoonotic AI viruses. Our model also identifies how abiotic characteristics influence viral transmission opportunities and spillover processes.
Methods
We analyzed the environmental persistence of zoonotic AIV from the published literature. To be included in the analysis, publications had to contain data from survival experiments of zoonotic AI in an aqueous medium; experimental conditions had to be recorded (characteristics of the substrate like temperature, salinity, and pH; titration method; number of replicates; and number of titrations in time) or available from authors; statistical analyses had to have estimated decay rates (proportion of virus inactivated per unit time, equivalent to slope coefficient in equation 1 below) at each of the different experimental conditions; and results of these analyses had to be adequately reported (contain number of experimental replicates and number of titrations in time, type of transformation of the response data such as natural or base 10 logarithm, and R2 estimates). To include a zoonotic subtype in our analyses, data needed to be available from more than one publication. To identify the zoonotic AIV subtypes, we searched the CDC weekly mortality reports and performed a search in Google Scholar with the terms “influenza human infection,” “zoonotic influenza subtypes,” and “human pandemic influenza subtypes.” With the zoonotic subtypes identified we searched for the published material on AIV environmental persistence experiments. With the above inclusion criteria, we identified three publications from Google Scholar with appropriate data on AI environmental persistence: Domanska-Blicharz et al. (2010), Zhang et al. (2014), and Brown et al. (2014). Further details on the search results are given in the Results and Supplementary Materials. From these publications, we analyzed the relationships between the slope regression coefficient a (see equation 1 below) from each publication as functions of experimental temperature (°C), pH, and salinity (parts per thousand, ppt).
One exception was Brown et al. (2014), which only reported Rt-values (resistance time, or time elapsed until inactivation of 90% of the initial virus titer); hence, we obtained estimates of a (alog 10 from equation 1 in ln scale) using the following rationale. Because Rt is the time elapsed until 90% of the virus dies, in an exponential setting we have:
Hence:
where S = 0.1 (10% of the initial titer), the remaining fraction of the virus. Therefore:
Which leads to:
To transform regression coefficients from the remaining publications from log10 scale to ln scale, we multiplied each coefficient by ln(10):
All data were then analyzed in the same ln scale.
We also homogenized the scale of salinity data. Domanska-Blicharz et al. (2010) and Brown et al. (2014) reported salinity in ppt, whereas Zhang et al. (2014) reported salinity as conductivity (S·m−1, Siemens per meter). To transform Zhang et al. (2014) data to ppt, we transcribed the Fortran code of the Cond2Sal algorithm of the UNESCO technical papers in marine sciences (Fofonoff and Millard, 1983) to R code. The algorithm uses the following inputs: pressure (result of the depth at which the water sample was taken), temperature of collection, and salinity in S·m−1. For these calculations, we assumed zero depth, hence pressure of 0 (Zhang et al., 2014 did not report the depth, although zero depth is a reasonable assumption), and used the reported temperature of collection. This algorithm is most reliable for salinity conversion values between 2 and 42 ppt; however, the UNESCO report (Fofonoff and Millard, 1983) mentions that unless estimates are <0.05 ppt, values between 0.05 and 2 are plausible. Hence, we omitted the truncation included in the original Fortran code for such range of values.
Our compiled dataset consisted of estimates of a, temperature, salinity, pH, source of the data, titration method, if experiments were performed in filtered or unfiltered medium, the H5N1 strain used in the laboratory analyses, sample size, approximate number of titrations through time, and R2 of each linear regression. These data comprised of 120 records of the decay rate a, and were analyzed with a Bayesian model. These data cover a range of temperatures (4, 10, 16, 17, 20, and 28°C; all approximately evenly represented, full dataset in the Supplementary Materials) and salinities, from 0 to 30 ppt (parts per thousand) with relatively evenly distributed intermediate values of 6.4, 15, and 25.1 ppt. As for pH, available data only covered a narrow range of 6.9–8.9. All the factors that might affect the dispersion of a were included as random effects, and factors affecting the reliability of the data were used as weights (sample size and R2, Dalziel et al., 2016). Brown et al. (2014) did not report R2 of the regressions used to estimate Rt; however, we extracted their raw data from graphs in log10 scale with the precision of one decimal to recreate R2. For this procedure, we assumed the closest point to the regression line corresponded to the data used for that regression. We acknowledge this could inflate R2; however, this is unlikely to result in major differences as the overall weight of each observation in that study was smaller compared with the other two studies (Domanska-Blicharz et al., 2010; Zhang et al., 2014) due to their considerably larger sample sizes.
We next modeled a as a function of temperature, salinity, and pH using distinct functional forms (linear, quadratic, and with interactions). To find the optimal fixed effects structure of the model, we formulated separate models for a(Temp, pH), a(Temp, Sal) and for a(Sal, pH). For the models of a as a function of temperature and pH, we allowed for a bimodal response to pH, in which a decreases from its value at low or acid pH to a minimum at neutral pH values (7.2–7.4), and then increases as pH becomes alkaline. We did this by setting interactions of temperature and salinity with parameters of the expression (Brown et al., 2007, 2009):
Interactions with each parameter allow temperature and salinity to regulate the size effect of pH on virus inactivation rates (interaction with b), the width of the peak effect (parameter c), and the optimal pH for persistence (parameter d). Given the available narrow range of pH data (6.8–8.9), we were unable to find a joint effect of pH and temperature on any of the features described above; we therefore only show the temperature-salinity model.
The relationship between temperature, salinity, and survival is simpler than with pH; thus we only included simple linear terms and interactions between both variables. The form of the Bayesian model was:
Here ai is the coefficient data in ln scale, βi is a vector of the effects of temperature and salinity and its interactions, γi are the random effects per observation, is the weighted mean, N is the number of experimental replicates, and titrations is the number of virus titrations through time in each experimental replicate. To select the optimum model, we modified the structure of the model matrix X to include only the single linear terms or their interactions and with or without an intercept. The final model was selected upon the minimization of the deviance information criterion (DIC, Spiegelhalter et al., 2002), compliance with normality of residuals, and by consideration of the effects of salinity found in other publications that specifically tested its effect in controlled conditions in several influenza subtypes and strains (Stallknecht et al., 1990a,b; Brown et al., 2007, 2009, 2014). We established significance of statistical effects (inferred parameters) if parameter posteriors did not contain 0 within the range of 95% of samples. The other monitored parameter during MCMC sampling was the Bayesian R2; for more details on how this was calculated, we refer the reader to the JAGS (Plummer, 2003) code in the Supplementary Materials.
With the resulting model for a, we calculated the specific effect of temperature and salinity on Rt by finding the required temperature or salinity change for doubling the estimated Rt. First, if Rt = –ln 0.1/a, then Rt would double when a halves in response to temperature or salinity. Hence to find how a halves in response to temperature and salinity changes, we found da/dTemp and da/dSal. We solved these derivatives for dTemp (or dSal) when da = –a/2 (see Supplementary Materials for more details).
Results
Data Collection
With our systematic search, we identified the following zoonotic influenza subtypes: H1N1, H3N2, H2N2 (Webster, 1998), H5N1 (Webby and Webster, 2001; CDC, 2003; Peiris et al., 2004), H9N2 (Peiris et al., 1999), H7N2 (Wentworth et al., 1997), H7N7 (Fouchier et al., 2004; Koopmans et al., 2004), and H7N3 (Tweed et al., 2004). Of these subtypes, we only found environmental persistence experiments for H1N1 (Brown et al., 2009; Dublineau et al., 2011; Shigematsu et al., 2014) H3N2 (Brown et al., 2009), H5N1 (Paek et al., 2010; Dublineau et al., 2011; Brown et al., 2014; Shigematsu et al., 2014; Zhang et al., 2014), H9N2 (Zhang et al., 2014) and H7N3 (Brown et al., 2007). We found other publications with environmental persistence experiments; however, these focused on airborne transmission and are listed in the Supplementary Materials. Only three publications, Domanska-Blicharz et al. (2010), Zhang et al. (2014) and Brown et al. (2014), met the criteria for inclusion in our analyses (Liberati et al., 2009). Two of the studies that met most criteria for inclusion were Paek et al. (2010) and Shigematsu et al. (2014); however, we were unable to obtain the necessary data from the authors upon request (e.g., medium salinity, number of replicates, and R2 estimates of regressions) for inclusion. The final sample size was 120 different estimates of the slope coefficient (or decay rate) a (Equation 1): 30 estimates from Domanska-Blicharz et al. (2010), 24 estimates from Zhang et al. (2014), and 66 estimates from Brown et al. (2014). Further details on the number of replicates, titrations through time, R2 estimates, and strains are available in the full dataset in the Supplementary Materials.
Our dataset contains potential bias through the number of replicates per strain: four experimental replicates in Domanska-Blicharz et al. (2010) with close to 17 measurements through time with only one H5N1 strain (30 estimates); three experimental replicates and three to four titrations through time in Zhang et al. (2014, 24 estimates) with only one H5N1 strain; and no experimental replicates with two to five titrations through time in Brown et al. (2014) with 11 H5N1 strains (66 estimates). We tried to control for these differences between publications by including random effects and using sample sizes as weights in the regression. While some strains might be overrepresented, their overall contribution to our models are likely weak given that the total number of records per publication is also uneven and because the weight of the data points also depends on how well its models explained the regressed data (R2 estimates, Dalziel et al., 2016). The distributions of estimates of a per publication are given in Figure 1.
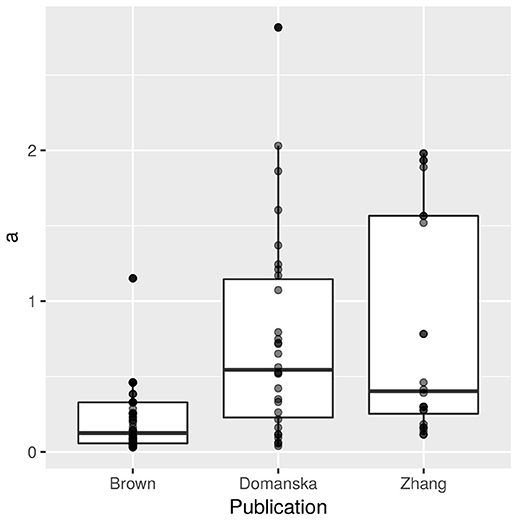
Figure 1. Decay rates a (N = 120) extracted from three publications (66 from Brown et al., 2014, 30 from Domanska-Blicharz et al., 2010, and 24 from Zhang et al., 2014). Data points have been made transparent to allow visualization of overlap.
The Model
All models of the joint effect of temperature and pH failed to show an effect of pH, probably due to the narrow range of pH values and the dispersion of the available data among experiments for H5N1. However, the wider range of salinity levels and its stronger effect on survival facilitated its inclusion in the analyses. The final model was simple (Equation 3) and showed a positive effect of temperature and a negative effect of salinity on a; the interaction between temperature and salinity also had a positive effect on a (Table 1):
The DIC of model from equation 3 (267.8) was <3 DIC units larger than a more complex model (DIC 267.77; the same terms as equation 3 but with an additional parameter, β0, as the intercept). Therefore, the set of fixed effects in equation 3 was the most parsimonious. Further, the resulting credible intervals for the model with the lowest DIC were unrealistically large and exceeded the observed range of a. Parameter estimates of the reported model are shown in Table 1. The selected model's Bayesian R2 was 0.278 (0.154–0.392, 95% Cr. I).
Of the random effects that could be included in the model, we only retained the experimental medium (substrate; e.g., filtered or unfiltered water and allantoic fluid), as no other random effects (source of the data and strain) had a significant effect (95% credible intervals of posterior samples did not include zero). In addition, removing random effects of publication source helped reduce the model's DIC and improved normality of residuals. Another reason was that the experimental medium was correlated with publication source, effectively addressing possible pseudoreplication and bias. The models which included publication source as a random effect instead of culture medium had the same parameter estimates and led to the selection of the same model reported here. The effect of the experimental medium was significant in all cases (γ estimates are provided in Table 1), and the lowest a estimates occurred in allantoic fluid (Brown et al., 2014), followed by filtered and unfiltered water (Domanska-Blicharz et al., 2010; Zhang et al., 2014).
Model Estimates
As temperature increased, the decay rate, a, increased, meaning that temperature decreases survival of H5N1 strains given that all data were experimental. Salinity as an isolated term had a negative effect, but the interaction between temperature and salinity was positive. This results in the decay rate, a, increasing in response to salinity at high temperatures and decreasing at low temperatures (Figure 2). The distribution of a by the model produced the resistance times (time to death of 90% of the initial virus) provided in Figure 3, using the formula Rt = –ln(0.1)/a.
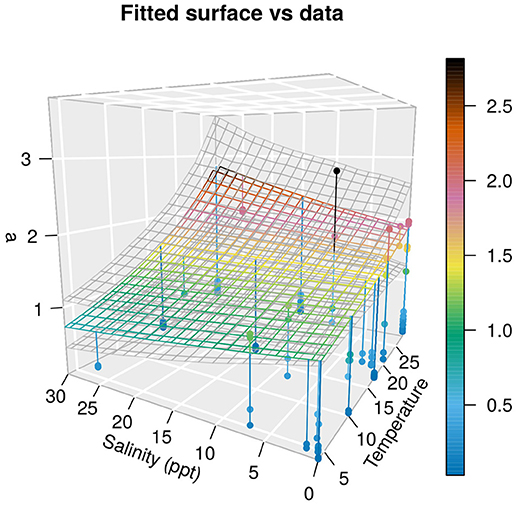
Figure 2. Surface plot of the model with credible intervals (gray planes above and below the median of model estimates in color). Color code indicates the observed (data points) and estimated (plane) value of decay rates a. The vertical lines show the distance between data points and the plane (without random effects) estimated by the model.
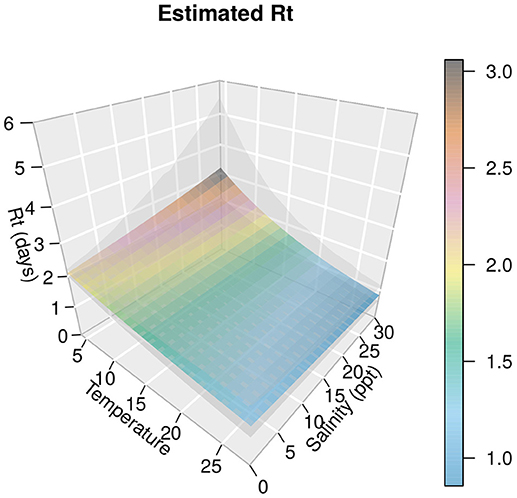
Figure 3. Resistance time in days (Rt) until 90% of the virus is inactivated in response to temperature and salinity. Color scale is for reference and indicates the estimated Rt with 95% credible intervals obtained with the posterior parameter samples (gray planes above and below the color plane).
A representation of the effects of temperature, salinity, and time on H5N1 decay in the environment is given in Figure 4. Based on the final model for a, the changes in temperature and salinity necessary to double Rt can be identified using:
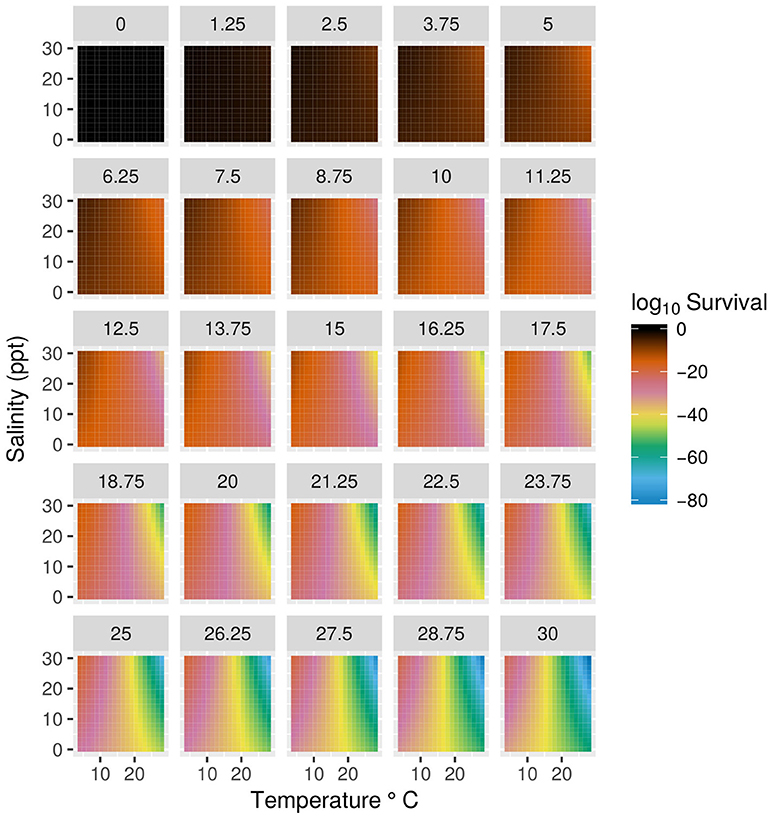
Figure 4. Color scale indicates the log10 of the proportion of viable H5N1 during a lapse of 30 days (cutoff in time in the gray panels). Each time snapshot includes the range of temperature (x-axis) and salinity (y-axis) over which viability was evaluated.
We note that some obtained estimates are unlikely to be biologically feasible. For instance, Rt can double when temperature decreases by 22.11°C (13.15–41.74°C95% Cr. I.) at salinity 0 ppt and decreases to 8.77°C (6.39–12.25°C95% Cr. I.) at 30 ppt (Figure 5). Whereas, with respect to salinity, Rt could both increase or decrease at any observed temperature, especially around 17° (Figure S1, Supplementary Materials). Ambiguity is due to the negative effect of salinity alone in the model (β2 < 0, P < 0.05, Table 1) and the positive effect of its interaction with temperature (β3 > 0, P > 0.05, Table 1). Furthermore, the range of salinity changes needed to double Rt are infeasible based on the credible intervals. According to our model, doubling Rt would occur when there are more parts of salt per parts of water (salinity −2,500–2,000 ppt). Thus, salinity would rarely drive strong changes of influenza H5N1 survival. Instead, such changes are predicted to occur more frequently in response to temperature changes in saline water bodies.
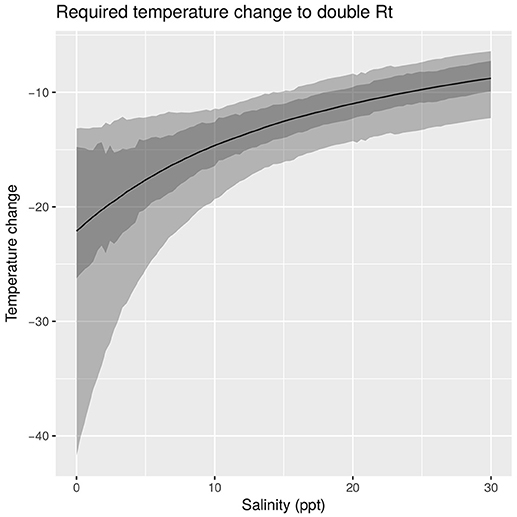
Figure 5. Temperature change in response to increasing salinity required to double resistance time. Note the negative scale of temperature, indicating that at higher salinity (parts per thousand) the temperature decrease required to double the time to inactivate 90% of the initial virus titer is less in average than at lower salinity levels. Shades of gray indicate the 95 and 68% credible intervals.
Discussion
We provide estimates of the joint effect of salinity and temperature on the rates of decay of several strains of influenza H5N1, showing a stronger effect of temperature on environmental viability compared to salinity. The stronger effect of temperature occurred in both types of experimental substrates included in the analyses: studies that tightly controlled substrate conditions in the laboratory (Brown et al., 2014) and studies that used water samples from natural water bodies (Domanska-Blicharz et al., 2010; Zhang et al., 2014). The estimated rates of decay identified here are consistent with estimates for other influenza subtypes in response to salinity and temperature (Stallknecht et al., 1990a; Brown et al., 2007) and with those of low pathogenicity AIV (Dalziel et al., 2016).
The most significant effect of salinity on H5N1 viability was through its interaction with temperature: the decrease of temperature required to double the resistance time (Rt) is smaller at higher salinity (Figure 5). Thus, longer persistence could occur across a wide range of salinity levels, especially at low temperatures, whereas small changes in temperature have non-linear effects on virus persistence. The same trend is present Brown et al. (2007) experiments, and the same pattern is apparent in that some H5N1 strains react differently to higher salinity and temperature combinations (Brown et al., 2014). The random effects included in our model to control for experimental substrate indicate that a was lower in the data from Brown et al. (2014); therefore, it is unclear how the estimates of salinity effects relate to persistence in natural water bodies. Other studies have found similar effects of salinity, pH, and temperature between viability in distilled water and samples from natural water bodies in the same strain of H6N2 (Stallknecht et al., 1990a; Brown et al., 2007) and in highly pathogenic H5N1 (Horm et al., 2012). Hence, it is possible that the trends we found occur among various influenza subtypes when facing different salinity levels.
One of the variables we tried to model was pH; however, the narrow range of pH values hampered its inclusion in our analyses. Regardless of the ubiquitous non-linear response of AIV to pH (Stallknecht et al., 1990a; Brown et al., 2009), it remains unclear how the non-linear responses are relevant in the context of water bodies where AIV occur naturally. For instance, Brown et al. (2009) performed survival experiments with 12 subtypes (none were H5N1) in a pH range of 5.8–8.6, while the water from natural bodies used for the experiments included in these analyses had pH extremes of 6.9 (Domanska-Blicharz et al., 2010) to 8.9 (Zhang et al., 2014). There is not a clear effect of pH within the range of existing pH data on the different H5N1 strains. Nevertheless, the possibility to further study the effects of a wider range of pH in several different subtypes and strains could be important to understand the relationship between inactivation pH and zoonotic potential (Lipsitch et al., 2016) and should therefore be a research priority.
During the model fitting process, we found that strain as a random effect was not significant and its inclusion in the model increased DIC. One possible explanation was that Domanska-Blicharz et al. (2010) and Zhang et al. (2014) only used one strain, while Brown et al. (2014) used 11 strains, none of which had experimental replicates or measurements in different substrates. However, the large difference of strain numbers between studies translates into limitations and potential biases. An approach to test the limitations of this study could be a qualitative comparison with the results of new experiments of untested H5N1 strains. Likewise, the lower persistence estimated with the model compared with Brown et al. (2014) might be a product of our statistical weights. Because statistical effects estimates from larger sample sizes are deemed more reliable than estimates from studies with small sample sizes (Garamszegi, 2014), weights here increased the importance of the experiments from Domanska-Blicharz et al. (2010) and Zhang et al. (2014) given their larger sample sizes (Figure 2). The inclusion of statistical weights in meta-analyses is common as is the inclusion of random effects where specific experimental or observational settings might influence effect sizes (Hedges et al., 1999; Deeks et al., 2008).
Studies of low-pathogenic AIV dynamics in wild aquatic birds have concluded that environmental transmission in response to water temperature and pH is important for the initiation and duration of epidemics (Rohani et al., 2009). The influenza persistence data used by Rohani et al. (2009) are similar to the Brown et al. (2014) data used here, which had the highest estimates of Rt likely from the tightly controlled experimental substrate conditions. Hence, it is probable that in light of the probable lower persistence of AIV in natural water bodies (data from Domanska-Blicharz et al., 2010; Zhang et al., 2014), the contribution of environmental persistence of AIV to epidemic takeoff and duration in aquatic birds is lower than that found in the simulation studies of Rohani et al. (2009). Furthermore, in a broader context, many other factors contribute to the effect that environmental persistence of AIV has on its transmission dynamics among hosts. For example, cross reactivity between subtypes and strains could affect transmissibility of closely related strains circulating in a population (Lipsitch et al., 2016). Similarly, the adaptive changes necessary to establish infection in terrestrial hosts derived from the different available binding receptors (Aubin et al., 2005; Gabriel et al., 2005; Lipsitch et al., 2016); the reassortment of Hemagglutinin and Neuraminidase types (de Vries et al., 2015); and the development of pathogenicity through novel cleavage sites (Wright, 2007) all introduce variability in environmental persistence, and result in trade-offs that affect viral fitness (Beauchemin and Handel, 2011; Park et al., 2013; Gog et al., 2015). As a result our model should be interpreted as a trend among highly pathogenic H5N1 strains. However, we argue that the mentioned complexities that affect the relevance of environmental persistence of AIV toward its transmission dynamics among reservoir hosts are overcome in the context of zoonotic transmission to influenza-naive recipient hosts (Plowright et al., 2017).
Finally, we emphasize the need to further investigate the pH inactivation dynamics of influenza viruses due to their non-monotonic nature. This characteristic of decay responses, in addition to its potential to indicate zoonotic risk (Lipsitch et al., 2016), could help identify optimal water characteristics for zoonotic transmission to humans and domestic animals. Given that the ability of viruses to persist in the environment is an important component of whether or not pathogens can spill over (Plowright et al., 2017), future work on pH inactivation of AIV will be important to prevent future pandemics.
Conclusions
With these analyses, we provide estimates for parameters that will be useful for holistic models that assess or predict risk of zoonotic AIV spillover. Temperature has a significant effect on the environmental persistence of H5N1 AIV. The effect of salinity, however, might depend on its interaction with temperature. We therefore infer that AIV could persist in both high salinity warm water and high salinity cold water. These two abiotic conditions might represent contrasting environmental contexts for determining AIV spillover. Further studies are required to understand the effect of salinity and its interaction with temperature within the geographical areas where AIV strains originate, such as the effects of the local environmental conditions and reservoir host species on overall resistance. Moreover, more work is needed to reconcile data on viral persistence from field and laboratory experiments. Finally, there is a need to further study how pH affects viral persistence, as pH may be an important characteristic that determines optimal conditions for spillover.
Author Contributions
GM Performed the strategic search, designed selection criteria, extracted data, performed the analyses and wrote the manuscript. DB Identified the requirements for the research to be framed as a meta-analysis, helped design and refine the statistical analysis and helped in manuscript preparation. RP Obtained the funding, developed the main ideas for research and helped in manuscript preparation.
Conflict of Interest Statement
The authors declare that the research was conducted in the absence of any commercial or financial relationships that could be construed as a potential conflict of interest.
Acknowledgments
This research was developed with funding from the Defense Advanced Research Projects Agency (DARPA; D16AP00113), the National Science Foundation DEB-1716698, the National Institute of General Medical Sciences of the National Institutes of Health under Award Number P20GM103474 and P30GM110732, and SERDP RC-2633. The views, opinions and/or findings expressed are those of the author and should not be interpreted as representing the official views or policies of the Department of Defense or the U.S. Government. We thank Alex Washburne for helpful feedback on earlier versions of the manuscript, and the reviewers whose contributions helped improve the quality of this paper.
Supplementary Material
The Supplementary Material for this article can be found online at: https://www.frontiersin.org/articles/10.3389/fevo.2018.00131/full#supplementary-material
References
Abulreesh, H. H., Goulder, R., and Scott, G. W. (2007). Wild birds and human pathogens in the context of ringing and migration. Ringing Migr. 23, 193–200. doi: 10.1080/03078698.2007.9674363
Artois, M., Bicout, D., Doctrinal, D., Fouchier, R., Gavier-Widen, D., Globig, A., et al. (2009). Outbreaks of highly pathogenic avian influenza in Europe: the risks associated with wild birds. Rev. Sci. Tech. 28, 69–92. doi: 10.20506/rst.28.1.1854
Aubin, J.-T., Azebi, S., Balish, A., Banks, J., Bhat, N., Bright, R. A., et al. (2005). Evolution of H5N1 avian influenza viruses in asia. Emerg. Infect. Dis. 11, 1515–1521. doi: 10.3201/eid1110.050644
Beauchemin, C. A., and Handel, A. (2011). A review of mathematical models of influenza A infections within a host or cell culture: lessons learned and challenges ahead. BMC Public Health 11:S7. doi: 10.1186/1471-2458-11-S1-S7
Bright, P., Langenberg, J., Morrisey, J. K., and Tizard, I. (2016). Association of avian veterinarians wild bird feeding : the pros and the cons. J. Avian Med. Surg. 20, 124–127. doi: 10.1647/1082-6742(2006)20[124:WBFTPA]2.0.CO;2
Brown, J., Stallknecht, D., Lebarbenchon, C., and Swayne, D. (2014). Survivability of Eurasian H5N1 highly pathogenic avian influenza viruses in water varies between strains. Avian Dis. 58, 453–457. doi: 10.1637/10741-120513-ResNote.1
Brown, J. D., Goekjian, G., Poulson, R., Valeika, S., and Stallknecht, D. E. (2009). Avian influenza virus in water: infectivity is dependent on pH, salinity and temperature. Vet. Microbiol. 136, 20–26. doi: 10.1016/j.vetmic.2008.10.027
Brown, J. D., Swayne, D. E., Cooper, R. J., Burns, R. E., Stallknecht, D. E., et al. (2007). Persistence of H5 and H7 avian influenza viruses in water. Avian Dis. 51(1 Suppl.), 285–289. doi: 10.1637/7636-042806R.1
Buisch, W. W., Hall, A. E., and McDaniel, H. A. (1984). “1983-1984 Lethal avian influenza outbreak,” in Proceeding of the Annual Meeting-United States Animal Health Association (Richmond, VA).
CDC (2003). Update: influenza activity – United States and worldwide, 2002–03 Season, and composition of the 2003–04 influenza vaccine. Morb. Mortal. Wkly Rep. 52, 109–112.
Dalziel, A. E., Delean, S., Heinrich, S., and Cassey, P. (2016). Persistence of low pathogenic influenza A virus in water: a systematic review and quantitative meta-analysis. PLoS ONE 11:e0161929. doi: 10.1371/journal.pone.016192
de Vries, E., Guo, H., Dai, M., Rottier, P. J., van Kuppeveld, F. J., and de Haan, C. A. (2015). Rapid emergence of highly pathogenic avian influenza subtypes from a subtype H5N1 hemagglutinin variant. Emerg. Infect. Dis. 21, 842–846. doi: 10.3201/eid2105.141927
Deeks, J. J., Altman, D. G., and Bradburn, M. J. (2008). “Statistical methods for examining heterogeneity and combining results from several studies in meta-analysis,” in Systematic Reviews in Health Care, eds M. Egger, G. D. Smith, and D. G. Altman (London, UK: Wiley-Blackwell), 285–312.
Domanska-Blicharz, K., Minta, Z., Smietanka, K., and Marché, S. (2010). H5N1 high pathogenicity avian influenza virus survival in different types of water. Avian Dis. 54, 734–737. doi: 10.1637/8786-040109-ResNote.1
Dublineau, A., Batéjat, C., Pinon, A., Burguière, A. M., Leclercq, I., and Manuguerra, J. C. (2011). Persistence of the 2009 pandemic influenza a (H1N1) virus in water and on non-porous surface. PLoS ONE 6:e28043. doi: 10.1371/journal.pone.0028043
Ellis, T. M., Bousfield, R. B., Bissett, L. A., Dyrting, K. C., Luk, G. S., Tsim, S. T., et al. (2004). Investigation of outbreaks of highly pathogenic H5N1 avian influenza in waterfowl and wild birds in Hong Kong in late 2002. Avian Pathol. 33, 492–505. doi: 10.1080/03079450400003601
Ewald, P. W. (1987). Transmission modes and evolution of parasitism. NY. Acad. Sci. 503, 295–306. doi: 10.1111/j.1749-6632.1987.tb40616.x
Fofonoff, N. P., and Millard, R. C. Jr. (1983). Unesco Technical Papers in Marine Science 44: Algorithms for Computation of Fundamental Properties of Seawater. Paris: UNESCO.
Fouchier, R. A., Schneeberger, P. M., Rozendaal, F. W., Broekman, J. M., Kemink, S. A., Munster, V., et al. (2004). Avian influenza A virus (H7N7) associated with human conjunctivitis and a fatal case of acute respiratory distress syndrome. Proc. Natl. Acad. Sci. U.S.A. 101, 1356–1361. doi: 10.1073/pnas.0308352100
Gabriel, G., Dauber, B., Wolff, T., Planz, O., Klenk, H. D., and Stech, J. (2005). The viral polymerase mediates adaptation of an avian influenza virus to a mammalian host. Proc. Natl. Acad. Sci. U.S.A. 102, 18590–18595. doi: 10.1073/pnas.0507415102
Gaidet, N., Cattoli, G., Hammoumi, S., Newman, S. H., Hagemeijer, W., Takekawa, J. Y., et al. (2008). Evidence of infection by H5N2 highly pathogenic avian influenza viruses in healthy wild waterfowl. PLoS Pathog. 4:e1000127. doi: 10.1371/journal.ppat.1000127
Garamszegi, L. Z. (2014). “Uncertainties due to within-species variation in comparative studies: measurement errors and statistical weights,” in Modern Phylogenetic Comparative Methods and Their Application in Evolutionary Biology, ed L. Z. Garamszegi (Seville: Springer), 157–199.
Gilbert, M., Xiao, X., Domenech, J., Lubroth, J., Martin, V., and Slingenbergh, J. (2006). Anatidae migration in the western Palearctic and spread of highly pathogenic avian influenza H5NI virus. Emerg. Infect. Dis. 12, 1650–1656. doi: 10.3201/eid1211.060223
Gog, J. R., Pellis, L., Wood, J. L., McLean, A. R., Arinaminpathy, N., and Lloyd-Smith, J. O. (2015). Seven challenges in modeling pathogen dynamics within-host and across scales. Epidemics 10, 45–48. doi: 10.1016/j.epidem.2014.09.009
Hedges, L. V., Gurevitch, J., and Curtis, P. S. (1999). The meta-analysis of response ratios in experimental ecology. Ecology 80, 1150–1156. doi: 10.1890/0012-9658(1999)080[1150:TMAORR]2.0.CO;2
Hinshaw, V. S., and Webster, R. G. (1982). The natural history of influenza A viruses. Basic Appl. Influ. Res. 79–104.
Horm, V. S., Gutiérrez, R. A., Nicholls, J. M., and Buchy, P. (2012). Highly pathogenic influenza A (H5N1) virus survival in complex artificial aquatic biotopes. PLoS ONE 7:e34160. doi: 10.1371/journal.pone.0034160
Koopmans, M., Wilbrink, B., Conyn, M., Natrop, G., van der Nat, H., Vennema, H., et al. (2004). Transmission of H7N7 avian influenza A virus to human beings during a large outbreak in commercial poultry farms in the Nederlands. Lancet 363, 587–593. doi: 10.1016/S0140-6736(04)15589-X
Kuiken, T., Holmes, E. C., McCauley, J., Rimmelzwaan, G. F., Williams, C. S., and Grenfell, B. T. (2006). Host species barriers to influenza virus infections. Science 312, 394–397. doi: 10.1126/science.1122818
Kuris, A. M., Lafferty, K. D., and Sokolow, S. H. (2014). Sapronosis: a distinctive type of infectious agent. Trends Parasitol. 30, 386–393. doi: 10.1016/j.pt.2014.06.006
Liberati, A., Altman, D. G., Tetzlaff, J., Mulrow, C., Gøtzsche, P. C., Ioannidis, J. P., et al. (2009). The PRISMA statement for reporting systematic reviews and meta-analyses of studies that evaluate health care interventions: explanation and elaboration. PLoS Med. 6:e1000100. doi: 10.1371/journal.pmed.1000100
Lipsitch, M., Barclay, W., Raman, R., Russell, C. J., Belser, J. A., Cobey, S., et al. (2016). Viral factors in influenza pandemic risk assessment. Elife 5, 1–38. doi: 10.7554/eLife.18491
Lipsitch, M., and Viboud, C. (2009). Influenza seasonality: lifting the fog. Proc. Natl. Acad. Sci. U.S.A. 106, 3645–3646. doi: 10.1073/pnas.0900933106
Markwell, D. D., and Shortridge, K. F. (1982). Possible waterborne transmission and maintenance of influenza viruses in domestic ducks. Appl. Environ. Microbiol. 43, 110–115.
Martin, G., Plowright, R., Chen, C., Kault, D., Selleck, P., and Skerratt, L. (2015). Hendra virus survival does not explain spillover patterns and implicates relatively direct transmission routes from flying foxes to horses. J. Gen. Virol. 96(Pt 6), 1229–1237. doi: 10.1099/vir.0.000073
Martin, G., Webb, R. J., Chen, C., Plowright, R. K., and Skerratt, L. F. (2017). Microclimates might limit indirect spillover of the bat borne zoonotic hendra virus. Microb. Ecol. 74:106. doi: 10.1007/s00248-017-0934-x
Myatt, T. A., Kaufman, M. H., Allen, J. G., MacIntosh, D. L., Fabian, M. P., and McDevitt, J. J. (2010). Modeling the airborne survival of influenza virus in a residential setting: the impacts of home humidification. Environ. Health 9:55. doi: 10.1186/1476-069X-9-55
Paek, M. R., Lee, Y. J., Yoon, H., Kang, H. M., Kim, M. C., Choi, J. G., et al. (2010). Survival rate of H5N1 highly pathogenic avian influenza viruses at different temperatures. Poult. Sci. 89, 1647–1650. doi: 10.3382/ps.2010-00800
Park, A. W., and Glass, K. (2007). Dynamic patterns of avian and human influenza in east and southeast Asia. Lancet Infect. Dis. 7, 543–548. doi: 10.1016/S1473-3099(07)70186-X
Park, M., Loverdo, C., Schreiber, S. J., and Lloyd-Smith, J. O. (2013). Multiple scales of selection influence the evolutionary emergence of novel pathogens. Philos. Trans. R. Soc. B Biol. Sci. 368:20120333. doi: 10.1098/rstb.2012.0333
Peiris, J. S., Yu, W. C., Leung, C. W., Cheung, C. Y., Ng, W. F., Nicholls, J. M., et al. (2004). Re-emergence of fatal human influenza A subtype H5N1 disease. Lancet 363, 617–619. doi: 10.1016/S0140-6736(04)15595-5
Peiris, M., Yuen, K. Y., Leing, C. W., Chan, K. H., Ip, P. L., Lai, R. W., et al. (1999). Human infection with influenza H9N2. Lancet 354, 917–918. doi: 10.1016/S0140-6736(99)03311-5
Perdue, M. L., Suarez, D. L., and Swayne, D. E. (2000). Avian influenza in the 1990s. Poult. Avian Biol. Rev. 11, 1–20.
Plowright, R. K., Parrish, C. R., McCallum, H., Hudson, P. J., Ko, A. I., Graham, A. L., et al. (2017). Pathways to zoonotic spillover. Nat. Rev. Microbiol. 15, 502–510. doi: 10.1038/nrmicro.2017.45
Plummer, M. (2003). “JAGS: A program for analysis of Bayesian graphical models using Gibbs sampling,” in Proceedings of the 3rd International Worshop on Distributed Statistical Computing (Vienna).
Rohani, P., Breban, R., Stallknecht, D. E., and Drake, J. M. (2009). Environmental transmission of low pathogenicity avian influenza viruses and its implications. Proc. Natl. Acad. Sci. U.S.A. 106, 10365–10369. doi: 10.1073/pnas.0809026106
Satterfield, D. A., Altizer, S., Williams, M. K., and Hall, R. J. (2017). Environmental persistence influences infection dynamics for a butterfly pathogen. PLoS ONE 12:e0169982. doi: 10.1371/journal.pone.0169982
Shaman, J., and Kohn, M. (2009). Absolute humidity modulates influenza survival, transmission, and seasonality. Proc. Natl. Acad. Sci. U.S.A. 106, 3243–3248. doi: 10.1073/pnas.0806852106
Shaman, J., Pitzer, V. E., Viboud, C., Grenfell, B. T., and Lipsitch, M. (2010). Absolute humidity and the seasonal onset of influenza in the continental United States. PLoS Biol. 8:e1000316. doi: 10.1371/journal.pbio.1000316
Shigematsu, S., Dublineau, A., Sawoo, O., Batéjat, C., Matsuyama, T., Leclercq, I., et al. (2014). Influenza A virus survival in water is influenced by the origin species of the host cell. Influenza Other Respir. Viruses 8, 123–130. doi: 10.1111/irv.12179
Spiegelhalter, D. J., Best, N. G., Carlin, B. P., and van der Linde, A. (2002). Bayesian measures of model complexity and fit. J. R. Soc. Stat. 64, 583–639. doi: 10.1111/1467-9868.00353
Stallknecht, D. E., Goekjian, V. H., Wilcox, B. R., Poulson, R. L., and Brown, J. D. (2010). Avian Influenza virus in aquatic habitats: what do we need to learn? Avian Dis. 54, 461–465. doi: 10.1637/8760-033109-Reg.1
Stallknecht, D. E., Kearney, M. T., Shane, S. M., and Zwank, P. J. (1990a). Effects of pH, temperature, and salinity on persistence of avian influenza viruses in water. Avian Dis. 34, 412–418.
Stallknecht, D. E., Shane, S. M., Kearney, M. T., and Zwank, P. J. (1990b). Persistence of avian influenza viruses in water. Avian Dis. 34, 406–411.
Steensels, M., Van Borm, S., and Van den Berg, T. P. (2005). Avian influenza: mini-review, European control measures and current situation in Asia. Curr. Opin. Infect. Dis. 68, 103–120.
Swayne, D. E., Beck, J. R., Garcia, M., and Stone, H. D. (1999). Influence of virus strain and antigen mass on efficacy of H5 avian influenza inactivated vaccines. Avian Pathol. 28, 245–255. doi: 10.1080/03079459994731
Swayne, D. E., and Pantin-Jackwood, M. (2006). Pathogenicity of avian influenza viruses in poultry. Dev. Biol. (Basel). 124, 61–67.
Swayne, D. E., and Suarez, D. L. (2000). Highly pathogenic avian influenza. Rev. Sci. Tech. 19, 463–482.
Swayne, D. E., and Thomas, C. (2009). “Trade and food safety aspects for avian influenza viruses,” in Avian Influenza, ed. D. E. Swayne (Athens, GA: Wiley-Blackwell), 499–512.
Taubenberger, J. K., and Kash, J. C. (2010). Influenza virus evolution, host adaptation, and pandemic formation. Cell Host Microbe 7, 440–451. doi: 10.1016/j.chom.2010.05.009
Thomas, Y., Vogel, G., Wunderli, W., Suter, P., Witschi, M., Koch, D., et al. (2008). Survival of influenza virus on banknotes. Appl. Environ. Microbiol. 74, 3002–3007. doi: 10.1128/AEM.00076-08
Tweed, S. A., Skowronski, D. M., David, S. T., Larder, A., Petric, M., Lees, W., et al. (2004). Human illness from avian influenza H7N3, British Columbia. Emerg. Infect. Dis. 10, 2196–2199. doi: 10.3201/eid1012.040961
Walther, B. A., and Ewald, P. W. (2004). Pathogen survival in the external environment and the evolution of virulence. Biol. Rev. 79, 849–869. doi: 10.1017/S1464793104006475
Webby, R. J., and Webster, R. G. (2001). Emergence of influenza A viruses. Philos. Trans. R. Soc. Lond. B. Biol. Sci. 356, 1817–1828. doi: 10.1098/rstb.2001.0997
Weber, T. P., and Stilianakis, N. I. (2008). Inactivation of influenza A viruses in the environment and modes of transmission: a critical review. J. Infect. 57, 361–373. doi: 10.1016/j.jinf.2008.08.013
Webster, R. G. (1998). Influenza: an emerging disease. Emerg. Infect. Dis. 4, 436–441. doi: 10.3201/eid0403.980325
Webster, R. G., Bean, W. J., Gorman, O. T., Chambers, T. M., and Kawaoka, Y. (1992). Evolution and ecology of influenza A viruses. Microbiol. Rev. 56, 152–79.
Webster, R. G., Yakhno, M., Hinshaw, V. S., Bean, W. J., and Murti, K. G. (1978). Intestinal influenza: replication and characterization of influenza viruses in ducks. Virology 84, 268–278. doi: 10.1016/0042-6822(78)90247-7
Wentworth, D. E., McGregor, M. W., Macklin, M. D., Neumann, V., and Hinshaw, V. S. (1997). Transmission of swine influenza virus to humans after exposure to experimentally infected pigs. J. Infect. Dis. 175, 7–15.
Woo, P. C., Lau, S. K., and Yuen, K. (2006). Infectious diseases emerging from Chinese wet-markets: zoonotic origins of severe respiratory viral infections. Curr. Opin. Infect. Dis. 19, 401–407. doi: 10.1097/01.qco.0000244043.08264.fc
Wright, P. F. (2007). “Orthomyxovriuses,” in Fields Virology, eds N. Bernard, D. M. Knipe, and P. M. Howley (Philadelphia, PA: Wolters Kluwer Health; Lippincott Williams and Wilkins).
Keywords: influenza, H5N1, environmental persistence, zoonotic pathogens, temperature, salinity, meta-analysis
Citation: Martin G, Becker DJ and Plowright RK (2018) Environmental Persistence of Influenza H5N1 Is Driven by Temperature and Salinity: Insights From a Bayesian Meta-Analysis. Front. Ecol. Evol. 6:131. doi: 10.3389/fevo.2018.00131
Received: 18 October 2017; Accepted: 16 August 2018;
Published: 07 September 2018.
Edited by:
Sabir Bin Muzaffar, United Arab Emirates University, United Arab EmiratesReviewed by:
Diann J. Prosser, United States Geological Survey, United StatesAbdessamad Tridane, United Arab Emirates University, United Arab Emirates
Copyright © 2018 Martin, Becker and Plowright. This is an open-access article distributed under the terms of the Creative Commons Attribution License (CC BY). The use, distribution or reproduction in other forums is permitted, provided the original author(s) and the copyright owner(s) are credited and that the original publication in this journal is cited, in accordance with accepted academic practice. No use, distribution or reproduction is permitted which does not comply with these terms.
*Correspondence: Gerardo Martin, Z2VyYXJkb21tY0BnbWFpbC5jb20=