- 1Urban Nature Research Center, Natural History Museum of Los Angeles County, Los Angeles, CA, United States
- 2The Nature Conservancy, Los Angeles, CA, United States
- 3The Nature Conservancy, San Diego, CA, United States
A lack of information about urban habitats, and a lack of professionally-collected species occurrence data are often cited as major impediments to completing bioassessments in urban landscapes. We developed an urban biodiversity assessment framework that addresses these challenges. The proposed framework combines a customized hierarchical urban habitat classification scheme with citizen science-generated species occurrence data, such as iNaturalist and eBird. It integrates publicly available data on the physical and anthropogenic environment with species occurrence information and serves as a novel method for conducting urban biodiversity assessments. This framework provides insights into how species occurrences within an urban landscape are associated with spatial variation in the physical and anthropogenic environment. It can also yield information useful for planning and conservation management aimed at maintaining and enhancing the abundance and diversity of native and other desirable species in urban areas. This framework requires minimal taxonomic expertise on the part of those who employ it, and it can be implemented in urban areas worldwide, wherever adequate data exist. We demonstrate the application of this framework in the highly urbanized portion of Los Angeles County, California, USA. Our demonstration used 18 physical and anthropogenic variables to classify our study area into nine urban habitat types. We then assessed relationships between these urban habitat types with species occurrences using research-grade data from iNaturalist. This analysis detected significant differences in distributions of some species between these nine urban habitat types and demonstrated that the proposed framework can be used to conduct urban biodiversity assessments. With increasing availability of remote sensing data and publicly-generated biodiversity data, this framework may be used for analysis of urban areas around the globe.
Introduction
As the percentage of people living in urban areas continues to grow, and the extent of urbanized lands continues to expand (United Nations Department of Economic Social Affairs Population Division, 2014), understanding and protecting biodiversity in cities where large numbers of people live is of global conservation relevance (Kaplan et al., 1998). Importantly, making cities more welcoming to nature can provide large portions of the populace with greater chances for regular contact with, and appreciation for, biodiversity (Parker, 2015). However, developing a widely adoptable methodology to assess, plan for, and conserve urban biodiversity remains a challenge (Margules and Pressey, 2000; Ferrier, 2002). This is largely attributable to the lack of information about wildlife habitat and species occurrence data in urban areas and a lack of understanding about the role that spatial variation in anthropogenic factors (i.e., factors related to human social structure or the built environment) may play in species distributions across urban areas. These information gaps often stem from limited access to private property to collect such data, as well as the tendency among those conducting regional biodiversity assessments to classify urbanized areas using a small number of general, “developed” land cover types (e.g., Figure 1), and to assume that all of these land cover types have little or no biodiversity value (Pickett et al., 2001).
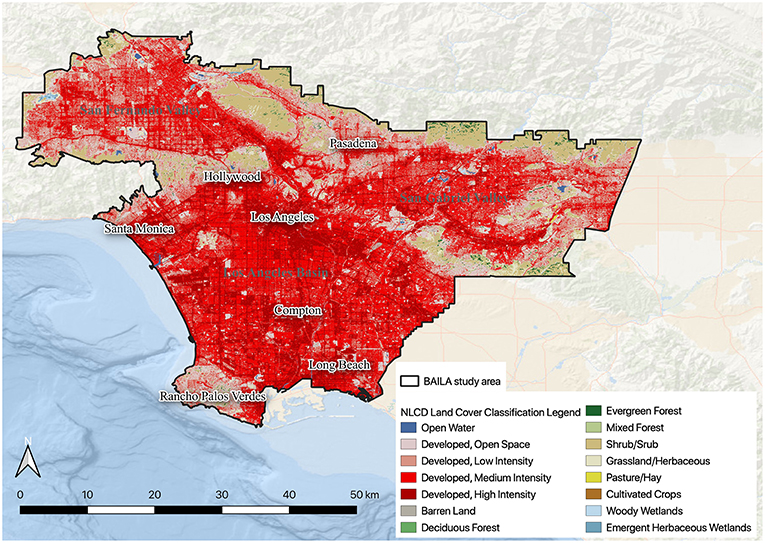
Figure 1. Study area with National Land Cover Data (NLCD) classification demonstrating that much of the study area is developed with only a few NLCD classes.
Species' abundances and distributions are highly dependent on fine-scale environmental variation (Blair and Launer, 1997; Williams et al., 2009). Thus, conducting biodiversity assessments in large urban areas requires spatially explicit characterizations of their heterogeneity (Pickett and Cadenasso, 1995; Stein et al., 2014). However, existing methods to quantify urban heterogeneity differ in how they combine biophysical and anthropogenic components (Grimm et al., 2000) and in the spatial scales at which they are applied (Wu and Loucks, 1995). Coarse-scale ecosystem classifications based on physical, climatic, and biological conditions, such as the Anderson or Anderson-derived classification systems (Anderson et al., 1976) or the Multi-Resolution Land Characteristics classification system (Wickham et al., 2014) often fail to capture the dynamic social processes and varied built environments that typify urban areas and that may greatly influence species distributions (Pickett and Cadenasso, 1995). Fine-scale approaches like HERCULES (Cadenasso et al., 2007) and “ecotopes” (Ellis et al., 2000; Chan and Paelinckx, 2008), while highly detailed, combine social use and biophysical parameters within a single patch, but each of the resulting urban types/ecotopes are independent of each other, thereby obscuring how they are related in terms of physical features and ecological functions (Wiens et al., 1993; Steenberg et al., 2015). Although a growing number of studies have conducted site-specific analyses to elucidate how urban heterogeneity structures biodiversity (e.g., Kinzig et al., 2005; Hand et al., 2016), the methods used are often too complex and costly to scale up to the level of entire cities or metropolitan regions (Goddard et al., 2010).
Heterogeneity in both natural and urban ecosystems is relative and scale-dependent (Klijn and de Haes, 1994; Grimm et al., 2000); for example, patches at a particular scale (e.g., blocks) can be aggregated into larger patches (e.g., neighborhoods) and are often themselves composed of smaller patches (e.g., home lots). Thus, an urban ecosystem classification is best structured as a nested hierarchy. A hierarchical approach to urban ecosystem classification captures the scale-dependent nature of ecosystems and facilitates understanding (Wu, 1999). This approach has rapidly gained ground in urban ecology, due to its ability to incorporate biophysical and anthropogenic components (Wu and Loucks, 1995). Hierarchical approaches have been adopted for a variety of research and management purposes (e.g., Nielsen-Pincus et al., 2015; Steenberg et al., 2015; Jackson-Smith et al., 2016). However, to our knowledge, no hierarchical classification has been developed with a focus on understanding how the distribution of urban biodiversity is related to urban habitat heterogeneity, and specifically on how species' distributions in urban areas are structured by variation in the biophysical landscape, the built environment, and the social structure of the region.
Urban biodiversity assessments have also been hampered by a lack of species occurrence data (Ferrier, 2002). However, the explosive growth in citizen science projects, and the use of platforms such as eBird and iNaturalist, have greatly enhanced the amount and availability of species occurrence data from urban areas (Silvertown, 2009; Spear et al., 2017). Although citizen science data have been critiqued for being gathered with non-standardized survey methods, similar biases and errors often exist in surveys conducted by professional biologists, even in some of the most commonly used species occurrence datasets (Devictor et al., 2010). Occurrence data gathered by citizen scientists in urban areas are proving to be particularly valuable because these same urban landscapes are typically under-surveyed by traditional, professional survey methods (Ballard et al., 2017). Citizen science has become an established method for advancing scientific knowledge in urban areas, including tracking population trends and distributions of species (e.g., Gardiner et al., 2012; Border et al., 2017; Spear et al., 2017), researching animal behaviors (e.g., Bonier et al., 2007; Boydston et al., 2018; Pesendorfer et al., 2018), and identifying and prioritizing urban conservation and management actions (e.g., Gregory et al., 2005; Crall et al., 2010). Likewise, data gathered by citizen science programs have served as the basis for thousands of peer-reviewed publications (Sullivan et al., 2009).
We developed an urban biodiversity assessment framework to address the challenges associated with the lack of information about urban habitats and the lack of professionally-collected species occurrence data available for urban areas. Our urban biodiversity assessment framework has two main components (Figure 2): a customized hierarchical urban habitat classification scheme that uses physical and anthropogenic factors to systematically differentiate habitat types within an urban landscape; and the use of citizen science-generated species occurrence data to examine species distributions across these habitat types. Serving as a novel and broadly applicable approach for conducting urban biodiversity assessments, this framework aims to integrate and make the best possible use of available environmental, and species information. This framework allows users to investigate: (1) how different species use different types of urban habitats, (2) which species have been observed only in certain types of urban habitats, and (3) community compostion differences across different urban habitat types. Additionally, such pattern exploration can help users generate hypotheses to further investigate the underlying drivers of urban biodiversity patterns. These analyses may also reveal particular areas or urban habitat types that have been undersampled and where new citizen science projects might be targeted. We demonstrate this framework in the highly urbanized portion of Los Angeles County, California, USA, and we term this demonstration Biodiversity Analysis in Los Angeles (BAILA). With increasing availability of remote sensing data and publicly-generated biodiversity data, the proposed framework can be adopted globally, and provide information useful for urban planning and conservation management aimed at maintaining and enhancing the abundance and diversity of native and desirable species in urban areas.
Methods
Study Area
The first step in developing an urban classification scheme is to specify the boundaries of the study area (Figure 2). To demonstrate our framework, we selected the urbanized portion of Los Angeles County, California, USA as our study area (Figure 1). The study area is situated in the California Floristic Province, one of the world's 36 recognized biodiversity hotspots (Myers et al., 2000). It covers 3,208 km2, including 80 incorporated cities and 69 unincorporated neighborhoods, and is home to more than 9 million people. It contains several different biophysical environments, and three major landscapes: the Los Angeles Basin, the San Fernando Valley, and the San Gabriel Valley. Bordered to the west and south by the Pacific Ocean and to the north and east by mountains and hills, the coastal Los Angeles Basin is generally cooler in the summer and milder in the winter but receives less rainfall than the inland San Fernando and San Gabriel Valleys. Daytime temperatures can vary as much as 20°C (36°F) between the coastal Los Angeles Basin and the San Fernando Valley or San Gabriel Valley. Although 86% of our study area has been heavily modified through the development of residential, commercial, industrial, and transportation infrastructure (Figure 1), recent studies and iNaturalist data demonstrate that it nonetheless contains a broad array of species (e.g., Clarke et al., 2013; Hartop et al., 2015; Allen et al., 2016). However, there is little understanding of how different taxa are distributed across the study area and of the factors that drive those distributions.
Identifying the Geographic Unit for the Urban Classification Scheme
The second step in developing an urban classification scheme is to select a basic geographic unit for analysis (Figure 2). The geographic unit should be selected based on the scale at which users wish to apply the resulting classification scheme, as well as the scale at which relevant data are available within the study area. The geographic unit may be based on ecosystem-based boundaries (e.g., subwatersheds or climate zones), jurisdictional boundaries (e.g., cities or neighborhoods), demographic boundaries (e.g., U.S. Census Tracts or U.S. Census Block Groups), or artificial grids that divide a study area into equally-sized cells. The decision about the geographic unit should take into consideration the future applicability and usability of the classification. For example, an artificial grid might be less useful for those focused on urban conservation planning and management, whereas jurisdictional and/or demographic boundaries may be more familiar and more easily used by decision-makers.
In the case of BAILA, to balance the trade-offs between the resolution of the available datasets, the need to ensure our results would be useful for city and county-level planning processes and conservation management programs, and to address computational limitations, we chose the U.S. Census Block Group (hereafter referred to as “BG”) as our basic unit of classification. A BG is a geographic unit that is intermediate in size between the Census Tract and the Census Block. It represents a cluster of Census Blocks (often the same as or similar to city blocks). BGs are generally bounded by roads, natural features, or political boundaries, and often approximate neighborhood boundaries recognized by local residents. Generally, within a BG, biophysical factors (such as microclimate and elevation), socioeconomic status, housing development type, and landscaping are relatively homogenous (Geronimus and Bound, 1998). BGs are available in Geographic Information System (GIS) format across the entire U.S. (and can be downloaded at: http://www.census.gov/cgi-bin/geo/shapefiles/index.php). Many other countries have similar census units. BGs have been widely used in U.S. urban landscape classification studies as an appropriate unit to quantify heterogeneity across large urban areas (Grove et al., 2006). Within the BAILA study area, there were 6,040 BGs ranging in size from 0.03 km2 to 23.65 km2, with an average size of 0.53 km2.
Variable Selection for the Urban Classification Scheme
To effectively understand how fine-scale environmental variation shapes urban biodiversity, an urban habitat classification scheme must include variables that represent three key elements of an urban region: the biophysical landscape, the built environment, and the human social structure. However, the inclusion of variables can vary based on data availability within the study area and data resolution compatibility. We suggest that users who adopt our framework first identify variables that have demonstrated or suspected direct effects on shaping biodiversity. This can be achieved through literature review or by consulting with local experts who have on-the-ground knowledge of the factors that shape local biodiversity. The next step is to inventory whether the proposed variables have suitable spatial datasets. Generally, local county GIS portals, Natural Earth Data (https://www.naturalearthdata.com/), USGS Earth Explorer (https://earthexplorer.usgs.gov/), US Census Bureau (https://factfinder.census.gov/faces/nav/jsf/pages/index.xhtml), NASA's Socioeconomic Data and Applications Center (https://sedac.ciesin.columbia.edu/), and Esri Open Data (https://hub.arcgis.com/pages/open-data) are reputable places to search for free, remotely sensed and GIS-based data. We also encourage potential users to contact local biodiversity experts, researchers, and conservation agencies for input on sources of available and suitable data. Fortunately, remotely sensed and GIS-based environmental data are becoming increasingly available worldwide. Finally, users must refine the list of candidate variables and develop a final set to be used in the analysis. In this step, users first should ensure that the data are of similar resolution and fully cover the study area. Users may also address collinearity between variables, either by extracting features using Principle Component Analysis (PCA) (Wold et al., 1987; Jackson-Smith et al., 2016), or by running correlation analyses among variables, and removing highly correlated variables based on knowledge about which variable has a weaker mechanistic relationship with urban biodiversity. The advantage of using correlation analyses is that they are easier to interpret than PCA.
In BAILA, through discussions with experts and literature review, we first identified 48 candidate variables relevant to the distribution of biodiversity in our study area. Those variables represent the biophysical, built environmental, and social aspects of the urban landscape. After data screening, 31 variables were kept whose data resolution was suitable for BG level classification and had full coverage of the study area. We further narrowed down those 31 variables to 18 based on reducing collinearity between variables. Specifically, when variables were highly correlated (r > 0.6), we kept variables that had the most direct impact on biodiversity. For example, we excluded land use (e.g., commercial, residential, industrial, etc.) and housing type (e.g., single-family housing, multi-family housing) from the framework because these attributes are highly correlated with percentage of imperviousness and greenness, population density, and traffic density (Appendix S1 in Supplementary Material); further, it is these latter factors, and not land use and housing type, that are the more direct drivers of urban biodiversity (Luck and Wu, 2002). Notably, we did not include a variety of socio-economic variables, such as education, income, property value, and ethnicity. Although some studies have identified correlations between socio-economic factors and urban vegetation (Luck and Wu, 2002), the relationship between socioeconomic factors and urban biodiversity, independent of other biophysical and built environment attributes, is not well-understood. In addition, we performed a sensitivity analysis by removing those highly-correlated variables (e.g., number of housing units, BG size, and nighttime light) one at a time to evaluate whether the final classification outcome changed dramatically and meaningfully in a way that was consistent with local knowledge of the region. We kept 18 variables for which there are strong empirical or theoretical grounds for presuming that they affect urban biodiversity and for which there are appropriate datasets for the analysis (Table 1). They include temperature, precipitation, terrain, landcover, greenness, distance to natural areas, population, and traffic noise and density. As it happens, these 18 variables are all well studied and known to have effects on biodiversity in urban areas around the world. These 18 variables may serve as examples for variable selection globally.
All 18 variables were calculated for each of the 6,040 BGs based on the definitions provided in Table 1. Given that the 18 variables have different units of measurement, we standardized each variable to range from 0 to 1. Data extraction and consolidation were performed in R 3.4.2 utilizing sf and tidyverse packages.
Conducting the Hierarchical Cluster Analysis
A hierarchical clustering algorithm is recommended for users to develop a customized urban habitat classification. Hierarchical clustering starts by treating each unit (in our BAILA case study, the units are the BGs) as a separate cluster, then repeatedly merges the two most similar clusters (Wilks, 2011). This continues until all the clusters are merged together, resulting in a nested hierarchical structure of the clusters (Wilks, 2011). Thus, it is an ideal method to reveal the hierarchical structure of complex urban environments. Hierarchical clustering can be performed with either a distance matrix or raw data. When raw data are provided, the algorithm requires a specified distance method to convert it to a distance matrix. Another feature of a hierarchical clustering algorithm is that the user decides how many final types the classification will identify (e.g., 5 types, 9 types, or 40 types). This grants users the flexibility to classify urban areas into a few, generally distinctive types, or numerous types that have more subtle differences. There is no definitive method for determining the optimal number of clusters in an analysis. A simple and frequently used solution consists of visually inspecting the dendrogram produced by hierarchical cluster analysis to see if it suggests a particular number of clusters (Bridges, 1966; Köhn and Hubert, 2006). Gap statistic is another method to estimate the optimal number of clusters by identifying at which point the rate of increase of the gap statistic begins to slow (Tibshirani et al., 2002). Whatever approach is used, we suggest that users check the final classification results to ensure that they are consistent with local knowledge. Decisions about the final number of clusters should also take into consideration the potential application and usability of the final urban habitat classification.
In BAILA, we used hierarchical cluster analysis to categorize the 6,040 BGs based on variation in the 18 input variables. We used Euclidean distance to measure dissimilarity between each pair of BGs and Ward's minimum variance method to measure dissimilarity between clusters of BGs. All analyses were performed in R 3.4.2 using fastcluster, dendextend, and tidyverse packages. We used visual inspection of the dendrogram and the gap statistic to estimate the optimal number of clusters. The gap statistic was performed with 30 bootstraps and a maximum of 15 clusters. The R script used to perform the hierarchical cluster analysis and gap statistic can be found at: https://github.com/enjieli/BAILA. Last, we inspected the final results of the classfication to verify that each of those urban habitat types were consistent with our local knowledge of the study area.
Citizen Scientist-Generated Species Occurrence Data
Owing to growth in citizen science efforts, there is an increasing availability of species occurrence data for urban areas (Spear et al., 2017). Some citizen science platforms/programs are focused on a single taxon (e.g., eBird; https://ebird.org/home), while others are focused on diverse groups of organisms (e.g., iNaturalist; https://www.inaturalist.org). Generally, citizen scientist-generated species occurrence data are free and publicly accessible, and many programs/platforms also provide easy online access to download and use data. In addition, the Global Biodiversity Information Facility (GBIF, https://www.gbif.org/en/) is a global data repository where, through a single portal, users can access biodiversity data from diverse sources including museum specimen records and citizen science observations.
For BAILA, we used iNaturalist observations as our source of species occurrence data. iNaturalist has gained great popularity in Los Angeles County due to a series of ongoing citizen science projects managed by the Natural History Museum of Los Angeles County (Ballard et al., 2017). Also, the iNaturalist database contains species occurrence records for a broad suite of taxa, making it ideal for biodiversity assessments. We used 59,842 observations (2,281 species) of species-level, research-grade iNaturalist observations spanning a variety of taxa (e.g., birds, plants, insects, reptiles, mammals, gastropods, arachnids, fungi, etc.) reported between 1 January 2010 and 15 September 2017 (GBIF.org, 2017) to assess biodiversity patterns across our study area. iNaturalist data can be downloaded through a data export tool (https://www.inaturalist.org/observations/export) or through GBIF. Research-grade observations are defined by iNaturalist as observations with a photo voucher, locality, date, and community-supported identification, and they cannot be cultivated or captive organisms. Importantly, depending on the research questions, users might want to include cultivated or captive organisms as these may be important components of the flora and fauna in some urban areas. Such information is also downloadable on iNaturalist. Our case study focuses on wild biodiversity; therefore, we did not include cultivated or captive organisms. Additionally, we gathered information on the provenance (native/introduced status) of these species from the California Department Fish and Wildlife, Calflora, the California Bird Records Committee, and iNaturalist. We were able to categorize 857 species as native to California and 434 as introduced. An additional 990 species (mostly insects) lacked provenance information.
Coupling Species Occurrences With the Urban Habitat Classification
Using species occurrences in parallel with an urban habtiat classification, our framwork can offer insights on the distribution of local urban biodiversity, facilitating the exploration of a variety of ecological questions. Using BAILA as an example, our framework can be used to explore the number of observations and species observed within each urban habitat type, the numbers of native and introduced species across taxa and urban habitat types, as well as aspects of the urban habitat that could be modified to make the area more welcoming to certain species. Further, our framework can investigate the shared species among urban habitat types, and the suites of species unique to specific urban habitat types. Lastly, our framework can be used to examine community dissimilarity across the urban habitat types. Due to the non-standardized survey method of citizen science-generated biodiversity data, we suggest that users develop criteria for data inclusion to address questions of interest. For example, when analyzing species found only in certain types of urban environments, a minimum number of observations can be set to ensure that those species have been commonly observed and therefore, that the observations are unique to that urban habitat type(s). However, such a cutoff is subjective, will depend on the total number of occurrences and the desired accuracy of the assesment, and may sharply limit the number of species and occurrences available for analysis. We suggest users set a cutoff based on inspection of the histogram or percentile rank of the numbers of observations per species.
In the case of BAILA, when analyzing the shared species among urban habitat types, and the suites of species unique to each urban habitat type, many species were observed only a few times within our entire study area, making it difficult to distinguish whether they were unique to a certain urban habitat type, or whether they were simply difficult to observe or under-sampled. Therefore, we only included species with at least 5 observations (945 out of 2,281 species) in our analysis. In the BAILA dataset, there are 59,842 observations of 2,281 species. The median number of observations per species is 3, the 60th percentile is 5, and the 75th percentile is 13. While using the 75th percentile cutoff (species with at least 13 observations) would surely increase the confidence of finding unique species in each urban habitat type, it would significantly reduce the number of species to be included in the analysis. In this case, only 587 out of 2,281 (about 26%) species would be kept for further analysis, which would dramatically reduce the representation of the biodiversity of the region. Therefore, to ensure we have some representation of the diverse species in the region, we choose the 60th percentile as the cutoff, which is five observations per species.
We used Non-metric Multidimensional Scaling (NMDS; Kruskal, 1964) to examine community dissimilarity across the urban habitat types. For ordination analyses, we treated each BG containing iNaturalist observations as a sample. Within each sample, the iNaturalist observations were treated as sampling units. Because it is mathematically difficult to calculate community dissimilarity with 2,281 species, especially when sites share few species, we analyzed only the 100 most frequently observed species using BGs that contained at least 30 observations. As a result, 160 BGs with 24,571 observations of the 100 most observed species were retained for ordination analyses. All 9 of our urban habitat types were included within the 160 BGs. We used the Jaccard coefficient, treating species with observations as “present” and those without observations as “absent,” to construct similarity matrices of those 160 BGs. This reduces the noise caused by uneven and biased sampling efforts in the iNaturalist dataset and heightens the signal of species distribution patterns. The fit (or stress) of an NMDS ordination was evaluated at both 2- and 3-dimensions with 1,000 iterations. We also performed a Permutational Multivariate Analysis of Variance (PerMANOVA) to test whether the community compositions among the various urban habitat types were significantly different based on 1,000 permutations of the data. Both NMDS analysis and PerMANOVA were performed using the vegan package in R 3.4.2.
Results
A Typology for Urban Biodiversity
The gap statistic (Figure 3) indicated the study area could be optimally divided into three (gap3 = 1.5211; Figure 3) distinct categories which are the less urbanized habitat types (Types 1–4), and two categories of more urbanized types: Valley urban habitat types (Types 5–6), and Basin urban habitat types (Types 7–9; Figures 3, 4). We also identified 9 distinct urban habitat types nested within these three different categories based on visual inspection of the dendrogram (Figures 3, 4). Each of these nine urban habitat types was given a name that reflects its geographic location and/or an additional distinctive feature to improve communication (Appendix S2 in Supplementary Materials). It should be noted however, that each of these nine urban habitat types was delineated by a cluster analysis of 18 variables, and the names that we assigned these urban habitat types are at best a shorthand that allows us to more readily communicate about them, but which in no way fully describes how they differ from one another.
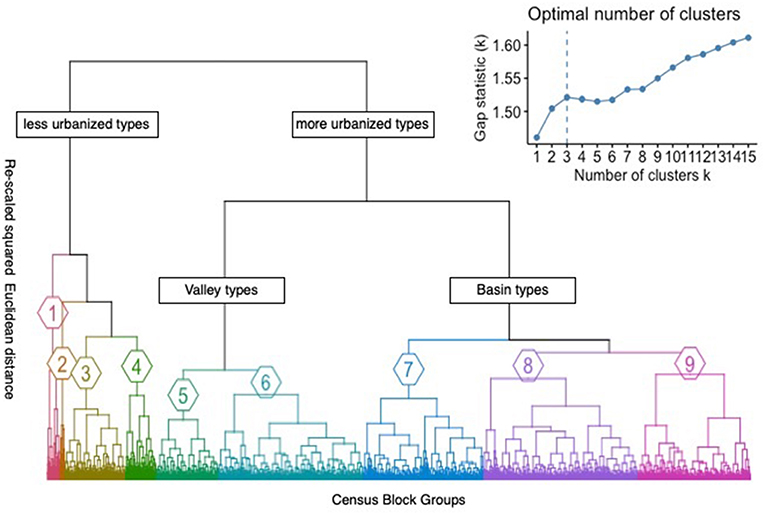
Figure 3. Dendrogram showing the hierarchical clustering of Census Block Groups and the distance among clusters in multivariate data space. The gap statistic (upper right) showing the total within-cluster variation for different values of k; the number of optimal clusters was determined by identifying at which point the rate of increase of the gap statistic began to slow down. Refer to Figure 4 for definitions of the nine urban habitat types.
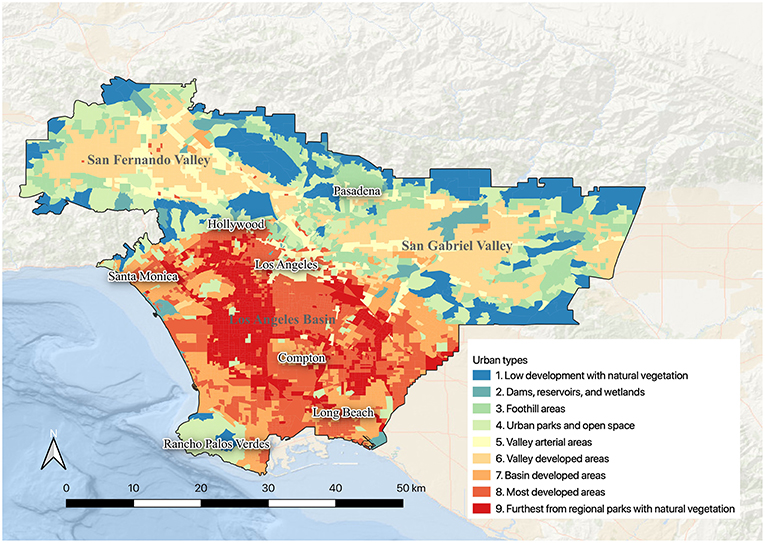
Figure 4. Spatial layout of the nine urban habitat types in the study area. These nine urban habitat types were identified from hierarchical clustering of 18 variables.
Overall, there were significant differences between the less urbanized and the more urbanized habitat types in percentage of impervious surface (M = 32.78, SD = 13.46 vs. M = 64.78, SD = 11.04; t(6038) = 2.89, p < 0.001), percentage of tree canopy cover (M = 4.78, SD = 4.51 vs. M = 64.78, SD = 1.76; t(6038) = 62.46, p < 0.001), and greenness (M = 0.22, SD = 0.05 vs. M = 0.14, SD = 0.04; t(6038) = 52.90, p < 0.001). The dendrogram (Figure 3) also indicated that there was great variation among the four less urbanized habitat types, while variation among the 5 more urbanized habitat types was not quite as strong.
Differences among the more urbanized habitat types in climatic factors, such as temperature and precipitation, were largely explained by geography, with Types 7 (Basin developed areas), 8 (Most developed areas), and 9 (Furthest from regional parks with natural vegetation) occurring largely in the more coastal Los Angeles Basin, and Types 5 (Valley arterial areas), and 6 (Valley developed areas) occurring largely in the San Gabriel and San Fernando Valleys to the north. The Basin urban habitat types had significantly cooler mean annual temperatures (M = 23.05°C, SD = 1.05 vs. M = 25.68°C, SD = 0.58; t(5104) = 52.90, p < 0.001), and less rainfall (M = 347.94 mm, SD = 25.95 vs. M = 421.27 mm, SD = 32.77; t(5104) = 62.46, p < 0.001) than the Valley urban habitat types.
Patterns of iNaturalist Observations
The iNaturalist observations were unevenly distributed across the study area (See Table 2 and Appendix S3 in Supplementary Materials for species accumulation curves for each urban habitat type). There were more observations in the less urbanized habitat types (Types 1–4; n = 40,122) than in the more urbanized habitat types (Types 5–9; n = 19,720) (Table 2). For BGs that have iNaturalist observations, the average number of observations, number of species observed, and the density of observations were higher in the less urbanized habitat types (Table 2). Types 1 (Low development with natural vegetation), 2 (Dams, reservoirs, and wetlands), and 4 (Urban parks and open space), where the majority of the public lands are located, had the most observations per BG (139, 164, and 53 respectively), highest species richness per BG (51, 64, 23, respectively), and highest species richness per unit area (35/km2, 102/km2, and 28/km2, respectively), while Type 5 (Valley arterial areas), which contains busy and loud traffic areas, had the fewest number of observations (2,421) and the fewest species (523) observed.
Insects, birds, and flowering plants (Magnoliophyta) constituted the majority of observations across all 9 urban habitat types (Figure 5). Type 1 (Low development with natural vegetation) had the greatest diversity of observed native flowering plant species (207). However, even in the highly urbanized areas, such as Types 5 (Valley arterial areas), and 9 (Furthest from regional parks with natural vegetation), more than 50 native flowering plant species were observed. Overall, we found 26,824 observations of 772 of California native species in the less urbanized habitat types (Types 1 through 4) and 9,679 observations of 539 native species in the more urbanized habitat types (Types 5 through 9). Native species accounted for the majority of occurrences (67%) in the less urbanized habitat types. Type 4 (Urban parks and open space) had the highest number of both native species (559 species) and introduced species (247 species).
The observed abundance and richness of introduced species varied depending on taxon and urban habitat type, and they were not dominant in any of the 9 urban habitat types (Table 2 and Figure 5). Across all types, 83% (Type 5; Valley arterial areas) to 89% (Type 2; Dams, reservoirs, and wetlands) of the bird species occurrences reported were native to California; fewer than 10% (12–14 species) were introduced species (Figure 5). For mammals, we found that there were more occurrences of native species than introduced species in areas with lower human population densities and less human activity, such as Types 1 (Low development with natural vegetation), 2 (Dams, reservoirs, and wetlands), and 4 (Urban parks and open space), whereas in areas with higher human populations and more activity, such as Types 3 (Foothill areas), and 5 (Valley arterial areas) through 9 (Furthest from regional parks with natural vegetation), introduced mammal occurrences were reported twice as often as native mammals (Figure 5). This result was largely driven by observations of a single species, the Eastern Fox Squirrel (Sciurus niger), which is the focus of a museum-led citizen science project.
The distribution of species (with at least 5 observations) varied across the 9 urban habitat types. A total of 185 species (20%) were found in all nine urban habitat types, including the Western Fence Lizard (Sceloporus occidentalis), Eastern Fox Squirrel, European Honey Bee (Apis mellifera), Brown Garden Snail (Cornu aspersum), Monarch Butterfly (Danaus plexippus), House Sparrow (Passer domesticus), and House Finch (Haemorhous mexicanus). Those 185 commonly observed species included 61 bird species, 50 of which were native, and 45 flowering plant species, of which only one third (15 species) were native, and 28 were introduced. Seventeen species were exclusively observed in only one of the 9 urban habitat types. Most of these were native plants in Type 1 (Low development with natural vegetation) and shorebirds and freshwater birds in Types 2 (Dams, reservoirs, and wetlands), and 4 (Urban parks and open space). In addition, 120 species were unique to the less urbanized habitat types (Types 1 through 4), the most common of which were California Broomsage (Lepidospartum squamatum), California bordered plant bug (Largus californicus), Phainopepla (Phainopepla nitens), Rock Wren (Salpinctes obsoletus), and White-breasted Nuthatch (Sitta carolinensis). 20 species were found exclusively in the more urbanized habitat types (Types 5 through 9), with 7 being introduced, 4 native, and 9 of unknown provenance, including: the slug Deroceras invadens, Spotted Lady Beetle (Adalia bipunctata), and Common House Spider (Parasteatoda tepidariorum).
Ordination Analysis Using the 100 Most Commonly Observed Species
For the 160 BGs included in the ordination analysis, Types 1–9 had 40, 16, 22, 33, 8, 13, 10, 13, and 5 BGs, respectively. Thus, we had samples in each of the nine urban habitat types. There were more than 4 times more observations from the less urbanized habitat types (19,748) than from the more habitat urbanized types (4,823). We used a 3-dimensional solution for the NMDS (Appendix S4 in Supplementary Materials), because we could not find a 2-dimensional solution for convergence. Overall goodness-of-fit was good (stress = 0.161). Results of PerMANOVA suggested that observed species composition differed between the urban habitat types (r2 = 0.15743, p = 0.001).
Pairwise comparisons showed that Type 1 (Low development with natural vegetation) and Type 2 (Dams, reservoirs, and wetlands) had significantly different species compositions from the remaining 7 urban habitat types (Table 3). The 4 less urbanized habitat types had very distinct community compositions from each other, with the exception of Types 4 (Dams, reservoirs, and wetlands) and 2 (Urban parks and open space), which were relatively similar to one another (F = 2.434, r2 = 0.049, p = 0.004, and padjust = 0.144). This is not surprising, considering some urban parks contain lakes and ponds. However, with the exception of the pair of Type 5 (Valley arterial areas) and Type 8 (Most developed areas), we did not find statistically significant differences in species composition amongst the 5 most urbanized habitat types (Types 5 through 9) (Table 3).
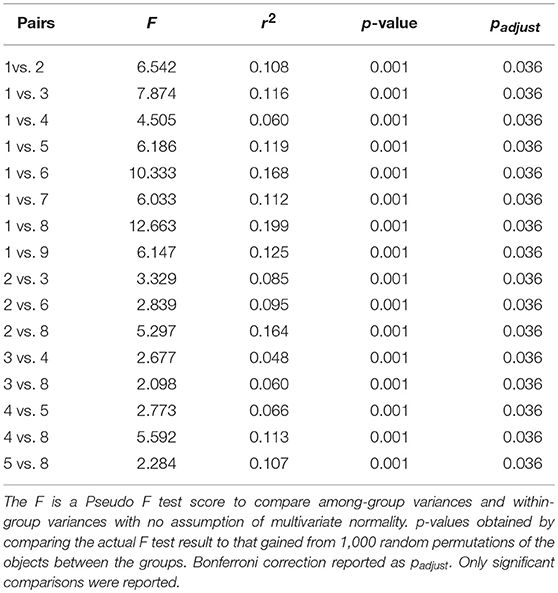
Table 3. Results of PerMANOVA pairwise comparisons of community composition among the nine urban habitat types. Refer to Figure 4 for definitions of the nine urban habitat types.
Discussion
BAILA Case Study
We found quantifiable environmental and biological differences within the BAILA study area. Instead of treating the urban area as one mass region with low biodiversity potential, our hierarchical clustering analyses distinguished nine urban habitat types that differed in extent of urbanization (i.e., percentage of impervious surface cover, human population density, percentage of urban area cover), climate, and other variables including traffic density and traffic noise. Species were distributed across these types in various ways, with some species being found across all types and others that were more restricted to one or a subset of types. Each of the 9 urban habitat types, support a variety of organisms, albeit with different levels of observed species richness and overall abundance (Table 2 and Figure 5). For example, more than 200 native species were observed in Type 5, one of the more urbanized habitat types, and one of the types covering the smallest total area. This also indicates that native species can be found not only in large urban green spaces but also within commercial, industrial, and residential districts (Rudd et al., 2002; Blair, 2004; Acar et al., 2007). This in turn indicates there is potential to enhance biodiversity in all of the urban habitat types with strategic interventions.
The variables used for this classification span the biophysical, built, and social landscapes, and urbanization was found to be the main factor driving the basal divergence in the hierarchical classification (less urbanized types vs. more urbanized types; Figure 4). Ordination analysis also showed that there were significant differences in community composition associated with differences between the urban habitat types (Table 3). This finding corroborates other studies that have shown that urban heterogeneity affects species distributions (Stein et al., 2014; Norton et al., 2016). Although we did not find significant differences in observed species distributions among the 5 most urbanized habitat types (Types 5 through 9) (Table 3), this might have resulted from our use of only the 100 most commonly observed species in the ordination analysis. While analyzing commonly observed species increased the statistical confidence of the community composition analysis, it might have caused us to overlook species that are truly unique to just one or a few of the more urbanized types. On the other hand, this finding might be indicative of “biotic homogenization” within the more urbanized types across our study area. Many studies indicate that urban areas can be biotically homogenous, containing a suite of “cosmopolitan,” generalist species (Blair, 2004; McKinney, 2006; McDonald et al., 2013; Leong and Trautwein, 2019). Collection of additional occurrence data in the 5 more urbanized types would help answer questions about whether they support distinctive suites of species or are “biotically homogenized.”
Our study demonstrates that the iNaturalist platform provides taxonomically diverse biodiversity data that can be used for spatially explicit urban biodiversity studies (see also Cooper et al., 2007; Bonney et al., 2009; Spear et al., 2017). About 60% of the land within our study area is privately owned, making it difficult to access and survey using traditional approaches. Datasets of professionally gathered species occurrence data, such as the California Natural Diversity Database (CNDDB), and other state Natural Heritage Program and national Conservation Data Center databases, contain few records for urban areas. For example, CNDDB had 956 occurrence records for only 154 species within our study area, and most of those species are rare and/or of special conservation concern, with little information about common and/or introduced species and with even less information from the more urbanized areas. Further, the number of records in such databases is growing only slowly, especially for heavily urbanized areas. In contrast, iNaturalist contained more than 59,842 observations of a diverse assortment of 2,281 species, including birds, plants, insects, reptiles, mammals, gastropods, arachnids, and fungi across all 9 urban habitat types, and those numbers are growing rapidly as more and more people participate in citizen science projects and as more and more people use iNaturalist.
Our case study could also provide insights for on-the-ground conservation management and planning in the Los Angeles area. There is increasing interest in enhancing native plant biodiversity within our study area, and other urban areas, through urban habitat restoration. Our analysis has generated a list of native plants that naturally occur and are commonly found across all 9 urban types [e.g., Eastern Mojave buckwheat (Eriogonum fasciculatum var. foliolosum), laurel sumac (Malosma laurina), toyon (Heteromeles arbutifolia), willow baccharis (Baccharis salicina), black sage (Salvia mellifera), California brittlebush (Encelia californica), western sycamore (Platanus racemosa), Southern California black walnut (Juglans californica), California poppy (Eschscholzia californica), and Coulter's Matilija poppy (Romneya coulteri)], even in areas with high percentages of impermeable cover (i.e., buildings and pavement). This offers practical suggestions for the implementation of urban habitat enhancement projects that intend to incorporate plants. In addition, our analyses found that while introduced species occurred in all urban habitat types, introduced mammals and spiders were observed more frequently in the more urbanized habitat types (Figure 5). These results suggest that efforts to detect and track introduced species should be concentrated in more urbanized areas, where citizen science approaches can be especially effective at overcoming the challenges of private property access for detecting the arrival and spread of introduced species.
Merits and Shortcomings of This Framework
Biodiversity assessments require information on both environmental variation and spatial distributions of the organisms within a study area. On the one hand, habitat classification derived from remotely sensed environmental data (such as climate, terrain, soil, landcover, etc.) have been widely used as surrogate information in assessing biodiversity. However, mapped habitat types may or may not correspond with actual biological differences. The potential for mismatches between assumed vs. real species-habitat relationships is high. On the other hand, species occurrence data are often sparse or unevenly sampled. Despite the abundant amount of citizen science-generated species occurrence data, it is still challenging to detect the geographical patterns, particularly in relation to environmental variation. By using an urban habitat classification in tandem with species distribution data, our framework serves as a useful tool to better evaluate whether mapped habitat types are meaningful in predicting biodiversity, as well as to detect species distribution patterns in relation to environmental variation. The combination of an urban habitat classification and species distribution data allows users to generate hypotheses to futher investigate the underlying drivers of the observed biodiversity patterns.
Remote sensing and GIS have progressed over the past decade with inexpensive, fine-resolution, and easily available data, as well as advanced analytical techniques that allow for the development of urban habitat classification systems tailored to urban biodiversity. An urban habitat classification generated using our framework will differ depending on the urban area evaluated, the variables used, the scale at which the classification is done, and the number of clusters chosen. In the future, data for many of our 18 variables are likely to exist at a finer resolution across our study area than those available today. Data on other variables may also become available, allowing further improvements to our analyses. We identified several other variables that may have been particularly useful for our study but for which data were unavailable or were not available at a fine enough scale across our study area to warrant inclusion. Examples include GIS data of soil types, street tree species, vegetation types, and irrigation system presence and use. When adopting this framework for other regions, we encourage users to carefully select variables based on availability of data, socio-ecological context of the locale, species of interest, and specific objectives of the analysis. While we developed this framework to study broad patterns in biodiversity, it could be easily modified to address other questions, such as how certain taxa use particular urban environments. In that case, the variables used for the urban classification should be key drivers that affect the distribution of the taxa of interest.
Citizen science-generated biodiversity data provide taxonomically diverse information in urban areas that are historically under-sampled by professionals. Our framework has several advantages, especially in urban areas where the lack of professionally-collected species occurrence data have historically limited opportunities for such analyses. Among these benefits are the incorporation of more data gathered on privately-owned lands, educational and other societal benefits related to involving volunteer citizen scientists in the gathering of data (Ballard et al., 2017), and avoiding the expense involved in employing professional biologists for field or lab work. Biodiversity assessments typically require taxonomic experts to carry out surveys or identify specimens in existing collections; however, this taxonomic expertise can now be crowdsourced to the online iNaturalist community (which includes taxonomic experts), such that the input dataset can be made up of high-quality occurrence records for which the species identity has a high level of confidence.
Our framework may also serve as a useful tool for identifying gaps in citizen science-generated species occurrence data. An analysis of the locations of the iNaturalist observations across the entire study area reveals specific areas (i.e., specific BGs) and specific urban habitat types that apparently have not been sampled by community scientists or where surveys for specific taxa have not yet been undertaken, thus revealing specific locales that could be targeted for future surveys. More observations from unsampled and under-sampled areas will improve our understanding of how different species utilize different urban habitats. In particular, such information will be useful to guide future citizen science projects to fill in such data gaps, and to improve the appreciation of and engagement with nature in communities located in currently under-sampled BGs. Furthermore, there are BGs that have no iNaturalist records at all. Using our framework, we can predict which species might occur in an under-sampled BG based on those that have been observed in other BGs of the same urban habitat type. Citizen science projects that focus on data collection in these BGs can be used to test these predictions.
In order to generate a meaningful urban habitat classification and be able to detect geographic patterns of local urban biodiversity, our framework requires a large amount of environmental and species occurrence data. Although the hierarchical classification could be performed with a minimum of one input variable, the outcome would be less comprehensive in terms of representing the full spectrum of the biophysical, built environmental, and social aspects of the urban environment. For example, for areas with a lack of social data, one could generate an urban habitat classification with only biophysical and built environment data. However, such an urban habitat classification might be weak in interpreting the effects of social variables on urban biodiversity. One can also generate an urban classification solely using social factors, but the resulting classification might not provide direct insights into how physical features shape local biodiversity. In general, we recommend that users select a suite of variables spanning the biophysical, built environmental, and social aspects of urban environments to achieve a more comprehensive urban habitat classification. However, it is not necessary to overload the classification model with variables, as many of the variables are correlated. On the other hand, the more species occurrence data incorporated, the more rigorous the biodiversity assessment can be. For areas lacking in species occurrence data, we encourage users to promote citizen science projects that gather occurrence records for use in future urban biodiversity studies. In addition, where citizen science-generated species occurrences data are growing rapidly, we encourage users to take advantage of the increasing availability of data to continuously improve their understanding of biodiversity. For example, in BAILA, we used 59,842 iNaturalist observations collected between 1 January 2010 and 15 September 2017. As of 28 June 2019, an additional 114,295 research grade (twice as many) observations were added in our study area. We are confident that with such significant growth in species occurrence data, some intricate and complex patterns of urban biodiversity could be revealed in our study area. To sum up, with continuous growth and development in GIS, remote sensing, and citizen science projects, many urban areas around the globe now have, or will soon have, both the environmental data and the species occurrence records needed to conduct robust urban biodiversity assessments.
Conclusion
The goal of this study was to generate an urban biodiversity assessment framework that is relatively simple to undertake, uses data from public sources and citizen science efforts, and is broadly applicable to other urban areas around the world. The novelty of this framework is that it combines urban habitat information with citizen science-generated species occurrence data and offers an improved understanding of urban biodiversity patterns that neither an urban habitat classification nor species occurrence information alone could reveal. With continuing advancement in GIS and remote-sensing, and the exponential growth of citizen science-generated species occurrence records, we believe that our framework will provide even more robust knowledge of urban biodiversity over time as the data used in the analyses improve. We hope our pioneering case demonstrates the importance of citizen science-generated species occurrence records such as those available from iNaturalist, and inspires and encourages other urban areas to promote citizen science projects that gather occurrence records for use in future urban biodiversity studies.
Data Availability
Publicly available datasets were analyzed in this study. iNaturalsit species occurrence data can be found here: https://doi.org/10.15468/dl.vnpfxd. Data and R script used for generating the urban habitat classification can be found here: https://github.com/enjieli/BAILA.
Author Contributions
SP, JR, GP, and BB developed the long-term collaboration that led to this manuscript, including securing funding for this project. All authors helped design and revise this methodology. EL acquired the appropriate data layers, with assistance from BC. EL performed the analyses and drafted the manuscript, with significant input from SP, JR, and GP. All authors edited and revised the manuscript and gave final approval for publication.
Conflict of Interest Statement
The authors declare that the research was conducted in the absence of any commercial or financial relationships that could be construed as a potential conflict of interest.
Acknowledgments
We thank our BAILA Science Advisory Group members: Katy Delaney, Joseph Decruyenaere, Naomi Fraga, Kat Superfisky, Jann Vendetti, and Eric Wood, as well as members of the Natural History Museum of Los Angeles County's Urban Nature Research Center and Community Science Office for their efforts promoting community science and for comments on an earlier draft. We also thank MaLisa Martin and others at the National Park Service and Shona Ganguly of The Nature Conservancy for their assistance in managing our BAILA Stakeholders Group, which includes representatives of 87 agencies, organizations, institutions, businesses, and offices of elected officials in the Los Angeles area, all of whom provided valuable input, guidance, and recommendations as our analyses took shape. Stakeholder participation is invaluable as we apply this work to landscape planning and urban conservation efforts. We are grateful to Bill and Katie Garland and the Bain & Company Conservation Innovation Grant for their generous financial support of this project, and to The Nature Conservancy and the Natural History Museum for their support of the BAILA partnership, and particularly to Jill Sourial, Charlotte Pienkos, Shona Ganguly, Jason Pelletier, Scott Morrison, and Luis Chiappe. Finally, we recognize the developers of iNaturalist and more than 10,000 citizen/community scientists, whose observations made our analyses possible; their efforts are revolutionizing urban biodiversity research.
Supplementary Material
The Supplementary Material for this article can be found online at: https://www.frontiersin.org/articles/10.3389/fevo.2019.00277/full#supplementary-material
References
Acar, C., Acar, H., and Eroglu, E. (2007). Evaluation of ornamental plant resources to urban biodiversity and cultural changing: a case study of residential landscapes in Trabzon city (Turkey). Build. Environ. 42, 218–229. doi: 10.1016/j.buildenv.2005.08.030
Allen, L. W., Garrett, K. L., and Wimer, M. C. (2016). LA County Breeding Bird Atlas. Los Angeles: Los Angeles Audubon Society. Available online at: https://www.laaudubon.org/shop/la-country-breeding-bird-atlas
Anderson, J. R., Hardy, E. E., Roach, J. T., and Witmer, R. E. (1976). A Land Use and Land Cover Classification System for Use With Remote Sensor Data. Geological Survey Professional Paper 964. Washington, DC: U.S. Government Printing Office.
Ballard, H. L., Robinson, L. D., Young, A. N., Pauly, G. B., Higgins, L. M., Johnson, R. F., et al. (2017). Contributions to conservation outcomes by natural history museum-led citizen science: examining evidence and next steps. Biol. Conserv. 208, 87–97. doi: 10.1016/j.biocon.2016.08.040
Blair, R. B. (2004). The effects of urban sprawl on birds at multiple levels of biological organization. Ecol. Soc. 9:2. doi: 10.5751/ES-00688-090502
Blair, R. B., and Launer, A. E. (1997). Butterfly diversity and human land use: species assemblages along an urban grandient. Biol. Conserv. 80, 113–125. doi: 10.1016/S0006-3207(96)00056-0
Bonier, F., Martin, P. R, and Wingfield, J. C. (2007). Urban birds have broader environmental tolerance. Biol. Lett. 3, 670–673. doi: 10.1098/rsbl.2007.0349
Bonney, R., Cooper, C. B., Dickinson, J., Kelling, S., Phillips, T., Rosenberg, K. V., et al. (2009). Citizen science: a developing tool for expanding science knowledge and scientific literacy. Bioscience 59, 977–984. doi: 10.1525/bio.2009.59.11.9
Border, J. A., Newson, S. E., White, D. C. J., and Gillings, S. (2017). Predicting the likely impact of urbanisation on bat populations using citizen science data, a case study for Norfolk, UK. Landsc. Urban Plan. 162, 44–55. doi: 10.1016/j.landurbplan.2017.02.005
Boydston, E. E., Abelson, E. S., Kazanjian, A., and Blumstein, D. T. (2018). Canid vs. canid: insights into coyote–dog encounters from social media. Human Wildlife Interact. 12, 233–242. doi: 10.26077/ab4z-t030
Bridges, C. C. (1966). Hierarchical cluster analysis. Psychol. Rep. 18, 851–854. doi: 10.2466/pr0.1966.18.3.851
Cadenasso, M. L., Pickett, S. T. A., and Schwarz, K. (2007). Spatial heterogeneity in urban ecosystems: reconceptualizing land cover and a framework for classification. Front. Ecol. Environ. 5, 80–88. doi: 10.1890/1540-9295(2007)5[80:SHIUER]2.0.CO;2
Chan, J. C.-W., and Paelinckx, D. (2008). Evaluation of random forest and adaboost tree-based ensemble classification and spectral band selection for ecotope mapping using airborne hyperspectral imagery. Remote Sens. Environ. 112, 2999–3011. doi: 10.1016/j.rse.2008.02.011
Clarke, L. W., Jenerette, G. D., and Davila, A. (2013). The luxury of vegetation and the legacy of tree biodiversity in Los Angeles, CA. Landsc. Urban Plan. 116, 48–59. doi: 10.1016/j.landurbplan.2013.04.006
Cooper, C. B., Dickinson, J., Phillips, T., and Bonney, R. (2007). Citizen science as a tool for conservation in residential ecosystems. Ecol. Soc. 12:11. doi: 10.5751/ES-02197-120211
Crall, A. W., Newman, G. J., Jarnevich, C. S., Stohlgren, T. J., Waller, D. M., and Graham, J. (2010). Improving and integrating data on invasive species collected by citizen scientists. Biol. Invasions 12, 3419–3428. doi: 10.1007/s10530-010-9740-9
Devictor, V., Whittaker, R. J., and Beltrame, C. (2010). Beyond scarcity: citizen science programmes as useful tools for conservation biogeography. Divers. Distribut. 16, 354–362. doi: 10.1111/j.1472-4642.2009.00615.x
Ellis, E. C., Li, R. G., Yang, L. Z., and Cheng, X. (2000). Long-term change in village-scale ecosystems in china using landscape and statistical methods. Ecol. Appl. 10, 1057–1073. doi: 10.1890/1051-0761(2000)010[1057:LTCIVS]2.0.CO;2
Ferrier, S. (2002). Mapping spatial pattern inbiodiversity for regional conservation planning: where to from here? System. Biol. 51, 331–363. doi: 10.1080/10635150252899806
Gardiner, M. M., Allee, L. L., Brown, P. M., Losey, J. E., Roy, H. E., and Smyth, R. R. (2012). Lessons from lady beetles: accuracy of monitoring data from US and UK citizen-science programs. Front. Ecol. Environ. 10, 471–476. doi: 10.1890/110185
Geronimus, A. T., and Bound, J. (1998). Use of census-based aggregate variables to proxy for socioeconomic group: evidence from national samples. Am. J. Epidemiol. 148, 475–486. doi: 10.1093/oxfordjournals.aje.a009673
Goddard, M. A., Dougill, A. J., and Benton, T. G. (2010). Scaling up from gardens: biodiversity conservation in urban environments. Trends Ecol. Evol. 25, 90–98. doi: 10.1016/j.tree.2009.07.016
Gregory, R. D., van Strien, A., Vorisek, P., Gmelig Meyling, A. W., Noble, D. G., Foppen, R. P. B., et al. (2005). Developing indicators for European birds. philosophical transactions of the royal society of London. Series B Biol. Sci. 360, 269–288. doi: 10.1098/rstb.2004.1602
Grimm, N. B., Grove, J. M., Pickett, S. T. A., and Redman, C. L. (2000). Integrated approaches to long-term studies of urban ecological systems: urban ecological systems present multiple challenges to ecologists—pervasive human impact and extreme heterogeneity of cities, and the need to integrate social and ecological approaches, concepts, and theory. Bioscience 50, 571–584. doi: 10.1641/0006-3568(2000)050[0571:IATLTO]2.0.CO;2
Grove, J. M., Troy, A. R., O'Neil-Dunne, J. P. M., Burch, W. R., Cadenasso, M. L., and Pickett, S. T. A. (2006). Characterization of households and its Implications for the vegetation of urban ecosystems. Ecosystems. 9, 578–597. doi: 10.1007/s10021-006-0116-z
Hand, K. L., Freeman, C., Seddon, P. J., Stein, A., and van Heezik, Y. (2016). A novel method for fine-scale biodiversity assessment and prediction across diverse urban landscapes reveals social deprivation-related inequalities in private, not public spaces. Landsc. Urban Plan. 151, 33–44. doi: 10.1016/j.landurbplan.2016.03.002
Hartop, E. A., Brown, B. V., and Disney, R. H. L. (2015). Opportunity in our ignorance: urban biodiversity study reveals 30 new species and one new nearctic record for Megaselia (Diptera: Phoridae) in Los Angeles (California, USA). Zootaxa 3941, 451–484. doi: 10.11646/zootaxa.3941.4.1
Jackson-Smith, D. B., Stoker, P. A., Buchert, M., Endter-Wada, J., Licon, C. V., Cannon, M. S., et al. (2016). Differentiating urban forms: a neighborhood typology for understanding urban water systems. Cities Environ. 9:5. Available online at: https://digitalcommons.lmu.edu/cate/vol9/iss1/5
Kaplan, R., Kaplan, S., and Ryan, R. (1998). With People in Mind: Design and Management of Everyday Nature. Washington, DC: Island Press.
Kinzig, A. P., Warren, P., Martin, C., Hope, D., and Katti, M. (2005). The effects of human socioeconomic status and cultural characteristics on urban patterns of biodiversity. Ecol. Soc. 10:23. doi: 10.5751/ES-01264-100123
Klijn, F., and de Haes, H. A. U. (1994). A hierarchical approach to ecosystems and its implications for ecological land classification. Landsc. Ecol. 9, 89–104. doi: 10.1007/BF00124376
Köhn, H., and Hubert, L. J. (2006). Hierarchical Cluster Analysis. Hoboken, NJ: Wiley StatsRef; Statistics Reference Online.
Kruskal, J. B. (1964). Multidimensional scaling by optimizing goodness of fit to a nonmetric hypothesis. Psychometrika 29, 1–27. doi: 10.1007/BF02289565
Leong, M., and Trautwein, M. (2019). A citizen science approach to evaluating US cities for biotic homogenization. PeerJ. 7:e6879. doi: 10.7717/peerj.6879
Luck, M., and Wu, J. (2002). A gradient analysis of urban landscape pattern: a case study from the Phoenix metropolitan region, Arizona, USA. Landsc. Ecol. 17, 327–339. doi: 10.1023/A:1020512723753
Margules, C. R., and Pressey, R. L. (2000). Systematic conservation planning. Nature 405, 243–253. doi: 10.1038/35012251
McDonald, R. I., Marcotullio, P. J., and Güneralp, B. (2013). “Urbanization and global trends in biodiversity and ecosystem services,” in Urbanization, Biodiversity and Ecosystem Services: Challenges and Opportunities: A Global Assessment, eds T. Elmqvist, M. Fragkias, J. Goodness, B. Güneralp, P. J. Marcotullio, R. I. McDonald, et al. (Dordrecht: Springer Netherlands, 31–52.
McKinney, M. L. (2006). Urbanization as a major cause of biotic homogenization. Biol. Conserv. 127, 247–260. doi: 10.1016/j.biocon.2005.09.005
Myers, N., Mittermeier, R. A., Mittermeier, C. G., da Fonseca, G. A. B., and Kent, J. (2000). Biodiversity hotspots for conservation priorities. Nature 403, 853–858. doi: 10.1038/35002501
Nielsen-Pincus, M., Ribe, R. G., and Johnson, B. R. (2015). Spatially and socially segmenting private landowner motivations, properties, and management: a typology for the wildland urban interface. Landsc. Urban Plan. 137, 1–12. doi: 10.1016/j.landurbplan.2014.11.020
Norton, B. A., Evans, K. L., and Warren, P. H. (2016). Urban biodiversity and landscape ecology: patterns, processes and planning. Curr. Landscape Ecol. Rep. 1, 178–192. doi: 10.1007/s40823-016-0018-5
Parker, S. S. (2015). Incorporating critical elements of city distinctiveness into urban biodiversity conservation. Biodivers. Conserv. 24, 683–700. doi: 10.1007/s10531-014-0832-1
Pesendorfer, M. B., Dickerson, S., and Dragoo, J. W. (2018). Observation of tool use in striped skunks: how community science and social media help document rare natural phenomena. Ecosphere 9:e02484. doi: 10.1002/ecs2.2484
Pickett, S. T. A., and Cadenasso, M. L. (1995). Landscape ecology: spatial heterogeneity in ecological systems. Science 269, 331–334. doi: 10.1126/science.269.5222.331
Pickett, S. T. A., Cadenasso, M. L., Grove, J. M., Nilon, C. H., Pouyat, R. V., Zipperer, W. C., et al. (2001). Urban ecological systems: linking terrestrial ecological, physical, and socioeconomic components of metropolitan areas. Annu. Rev. Ecol. Syst. 32, 127–157. doi: 10.1146/annurev.ecolsys.32.081501.114012
Rudd, H., Vala, J., and Schaefer, V. (2002). Importance of backyard habitat in a comprehensive biodiversity conservation strategy: a connectivity analysis of urban green spaces. Restorat. Ecol. 10, 368–375. doi: 10.1046/j.1526-100X.2002.02041.x
Silvertown, J. (2009). A new dawn for citizen science. Trends Ecol. Evol. 24, 467–471. doi: 10.1016/j.tree.2009.03.017
Spear, D. M., Pauly, G. B., and Kaiser, K. (2017). Citizen science as a tool for augmenting museum collection data from urban areas. Front. Ecol. Evolut. 5:86. doi: 10.3389/fevo.2017.00086
Steenberg, J. W. N., Millward, A. A., Duinker, P. N., Nowak, D. J., and Robinson, P. J. (2015). Neighbourhood-scale urban forest ecosystem classification. J. Environ. Manage. 163, 134–145. doi: 10.1016/j.jenvman.2015.08.008
Stein, A., Gerstner, K., and Kreft, H. (2014). Environmental heterogeneity as a universal driver of species richness across taxa, biomes and spatial scales. Ecol. Lett. 17, 866–880. doi: 10.1111/ele.12277
Sullivan, B. L., Wood, C. L., Iliff, M. J., Bonney, R. E., Fink, D., and Kelling, S. (2009). eBird: a citizen-based bird observation network in the biological sciences. Biol. Conserv. 142, 2282–2292. doi: 10.1016/j.biocon.2009.05.006
Tibshirani, R., Guenther, W., and Trevor, H. (2002). Estimating the number of clusters in a data set via the gap statistic. J. R. Statist. Soc. Series B 63, 411–423. doi: 10.1111/1467-9868.00293
United Nations Department of Economic Social Affairs Population Division (2014). World Urbanization Prospects The 2014 Revision. Available online at: http://esa.un.org/unpd/wup/CD-ROM/Default.aspx
Wickham, J., Homer, C., Vogelmann, J., McKerrow, A., Mueller, R., Herold, N., et al. (2014). The multi-resolution land characteristics (MRLC) consortium-−20 years of development and integration of USA national land cover data. Remote Sensing 6, 7424–7441. doi: 10.3390/rs6087424
Wiens, J. A., Chr, N., Van Horne, B., and Ims, R. A. (1993). Ecological mechanisms and landscape ecology. Oikos. 66, 369–380. doi: 10.2307/3544931
Wilks, D. S. (2011). “Chapter 15 - cluster analysis,” in International Geophysics, ed. D. S. Wilks (Academic Press), 603–616.
Williams, N. S., Schwartz, M. W., Vesk, P. A., McCarthy, M. A., Hahs, A. K., Clemants, S. E., et al. (2009). A conceptual framework for predicting the effects of urban environments on floras. J. Ecol. 97, 4–9. doi: 10.1111/j.1365-2745.2008.01460.x
Wold, S., Esbensen, K., and Geladi, P. (1987). Principal component analysis. Chemometrics Intel. Lab. Syst. 2, 37–52. doi: 10.1016/0169-7439(87)80084-9
Wu, J. (1999). Hierarchy and scaling: extrapolating information along a scaling ladder. Canadian J. Remote Sens. 25, 367–380. doi: 10.1080/07038992.1999.10874736
Keywords: urban biodiversity assessment, citizen science, urban habitat classification, landscape planning, conservation management, iNaturalist, Los Angeles
Citation: Li E, Parker SS, Pauly GB, Randall JM, Brown BV and Cohen BS (2019) An Urban Biodiversity Assessment Framework That Combines an Urban Habitat Classification Scheme and Citizen Science Data. Front. Ecol. Evol. 7:277. doi: 10.3389/fevo.2019.00277
Received: 11 April 2019; Accepted: 04 July 2019;
Published: 17 July 2019.
Edited by:
Sonja Knapp, Technische Universität Berlin, GermanyReviewed by:
Loren B. Byrne, Roger Williams University, United StatesFrederick R. Adler, The University of Utah, United States
Copyright © 2019 Li, Parker, Pauly, Randall, Brown and Cohen. This is an open-access article distributed under the terms of the Creative Commons Attribution License (CC BY). The use, distribution or reproduction in other forums is permitted, provided the original author(s) and the copyright owner(s) are credited and that the original publication in this journal is cited, in accordance with accepted academic practice. No use, distribution or reproduction is permitted which does not comply with these terms.
*Correspondence: Enjie Li, amxpQG5obS5vcmc=