- 1School of Natural Resources and the Environment, University of Arizona, Tucson, AZ, United States
- 2FRB-CESAB, Montpellier, France
- 3Electrical Engineering and Computer Science, Oregon State University, Corvallis, OR, United States
- 4Department of Fisheries & Wildlife, Oregon State University, Corvallis, OR, United States
- 5Vermont Center for Ecostudies, White River Junction, VT, United States
- 6Department of Biology, University of Ottawa, Ottawa, ON, Canada
- 7Canada Research Chair on Northern Biodiversity, Centre for Northern Studies and Quebec Center for Biodiversity Science, Université du Québec á Rimouski, Rimouski, QC, Canada
- 8Insectarium, Montreal Space for Life, Montreal, QC, Canada
Species distributions, abundance, and interactions have always been influenced by human activity and are currently experiencing rapid change. Biodiversity benchmark surveys traditionally require intense human labor inputs to find, identify, and record organisms limiting the rate and impact of scientific enquiry and discovery. Recent emergence and advancement of monitoring technologies have improved biodiversity data collection to a scale and scope previously unimaginable. Community science web platforms, smartphone applications, and technology assisted identification have expedited the speed and enhanced the volume of observational data all while providing open access to these data worldwide. How to integrate and leverage the data into valuable information on how species are changing in space and time requires new best practices in computational and analytical approaches. Here we integrate data from three community science repositories to explore how a specialist herbivore distribution changes in relation to host plant distributions and other environmental factors. We generate a series of temporally explicit species distribution models to generate range predictions for a specialist insect herbivore (Papilio cresphontes) and three predominant host-plant species. We find that this insect species has experienced rapid northern range expansion, likely due to a combination of the range of its larval host plants and climate changes in winter. This case study shows rapid data collection through large scale community science endeavors can be leveraged through thoughtful data integration and transparent analytic pipelines to inform how environmental change impacts where species are and their interactions for a more cost effective method of biodiversity benchmarking.
Introduction
Biodiversity benchmarking is fundamental to both basic and applied ecological research offering insights into the biological processes shaping species and their interactions. Benchmarking is a labor intensive endeavor, often limited by participation and training. Recent advances in sensing technology and communication have led to a diverse and plentiful data landscape coordinating and improving biodiversity community science efforts at scale so that they can be used in meaningful ways for benchmarking efforts (e.g., Sullivan et al., 2009; Prudic et al., 2017). Observational web platforms and smartphone applications, automated camera arrays, and machine learning-assisted identifications have also changed how biodiversity data is collected, processed, and verified (e.g., Sullivan et al., 2009; Prudic et al., 2017) although challenges remain (Bonney et al., 2009). These technologies have expedited the rate of understanding and changed the research focus to exciting new areas where an informatics toolkit is now a necessity (Feng et al., 2020). One new aspect of benchmarking biodiversity is to evaluate where species are and which species they co-occur with, or species distributions and their changing interactions (e.g., Bueno de Mesquita et al., 2016; Palacio and Girini, 2018).
Species distributions are known to be greatly influenced by climate (Brown et al., 2016). Climate-related range shifts have been and are continuing to be documented globally across taxa and systems: terrestrial (Parmesan and Yohe, 2003), marine (Poloczanska et al., 2013), and aquatic (Rahel and Olden, 2008). With current changes in global climate, species range shifts (Parmesan et al., 1999) and extensions in both altitude and latitude are being observed (Roth et al., 2014; Kerr et al., 2015). While many studies have examined the ongoing changes in climate and their effects on biodiversity and species ranges, most consider only abiotic factors in their analyses, missing the potential importance of local interspecific interactions once a species moves into a novel environment beyond its previous range (Blois et al., 2013; HilleRisLambers et al., 2013; Wisz et al., 2013).
Several interspecific interactions are known to play important roles in shaping range boundaries including competition (Connell, 1961; Huey et al., 2009; Stanton-Geddes et al., 2012), mutualism (Chalcoff et al., 2012; Moeller et al., 2012), facilitation (Bader et al., 2007; Stueve et al., 2011; Ettinger and HilleRisLambers, 2017) and natural enemies (Freeman et al., 2003; Speed et al., 2010). When a species extends into a new local environment, there are a few main scenarios it can encounter (Holt, 2003; Urban et al., 2007; Sexton et al., 2009): (1) ecological conditions are similar enough to previous conditions that there is little immediate effect on fitness and population growth rate, (2) the new local environment may possess biotic or abiotic conditions that differ from the original local environment and can accelerate (e.g., competitive or predatory release; or (3) decelerate (e.g., nutrient or nesting limitation) range expansion.
For insect herbivores, climate change can influence abundance and distribution through direct mechanisms (physiological impacts on growth, development and reproduction that impact fitness) and indirect mechanisms (impacting biotic factors such as host plant quality or predator abundance) (Bale et al., 2002; Deutsch et al., 2008; Kingsolver et al., 2011; Robinson et al., 2017). How and when climate change will affect herbivorous insect dynamics has received considerable attention generating a diversity of observed responses, especially in the pest management literature (Porter et al., 1991; Cannon, 1998; Harrington et al., 2001; Altieri et al., 2015; Castex et al., 2018). Some species are expanding in ranges and abundance (Battisti et al., 2005; Robinet and Roques, 2010; Robinson et al., 2017) while others are retracting and decreasing in numbers (Robinet and Roques, 2010; Zvereva et al., 2016; Sánchez-Bayo and Wyckhuys, 2019). Host plant abundance and distribution play a key role in generating these patterns as herbivorous insects are often limited by larval food resources (Dempster and Pollard, 1981; Pearson and Knisley, 1985; Ylioja et al., 1999). Exactly how host-availability translates into patterns of distribution, abundance, and range shifts for insect herbivores is still contentious and particularly complex when combined with direct effects on physiology (Louthan et al., 2015; Lany et al., 2018). Our understanding of the determinants regulating species distributions are becoming more nuanced as we begin to incorporate information on species’ dispersal capacity, population abundance trends, and climatic variables into our models (Elith and Leathwick, 2009).
In this study, we investigate the role of host availability and climatic variables on the range expansion of the specialist giant swallowtail butterfly (Papilionidae: Papilio cresphontes) in northeast North America over the last 60 years (1959-2018), with an emphasis on the perceived accelerated expansion of the last 18 years. We combine evidence from raw occurrence data with a series of species distribution models for P. cresphontes and associated host plants to evaluate the rate and direction of range changes in relation to both abiotic and biotic factors. While other studies have incorporated biotic variables as model inputs (Bueno de Mesquita et al., 2016; Palacio and Girini, 2018), our approach was to model the distribution of the insect herbivore and host plants separately and using these independent models to make post hoc inferences and comparisons of ranges. Because both this insect and its primary larval host plants (the common prickly ash [Rutaceae: Zanthoxylum americanum], southern prickly ash [Rutaceae: Zanthoxylum clava-herculis] and common hop tree [Rutaceae: Ptelea trifoliata]) are conspicuous, they are often reported in systematic biological surveys and museum collections. In this study, we bring together a combination of museum collection, survey, and citizen science data to understand how host plant availability, climate changes, and butterfly abundance are influencing the rapid expansion of an herbivorous insect as a case study. This study is one of few to demonstrate the interplay of both climate change and biotic interactions in shaping range limits while focusing on the ecologically important role of herbivores.
Materials and Methods
Study Region and Time Interval
We focused on eastern North America (study area bounded by −94° and −65° longitude and 25° and 55° latitude) where Papilio cresphontes has been reported to be expanding rapidly (Finkbeiner et al., 2011; Breed et al., 2012) and data are readily available for both P. cresphontes and larval host plants, (Zanthoxylum americanum, Zanthoxylum clava-herculis and Ptelea trifoliata). Though records of P. cresphontes exist further west than −94°, we set this cutoff to minimize complications of misidentification and complex species boundaries with its congener P. rumiko. We categorized and compared two time periods: T1 (1959-1999) representing the period prior to the beginning of the rapid range expansion and T2 (2000-2018) as the period when the rapid range expansion to the north began. This cutoff point was determined from raw occurrence data (Figure 1).
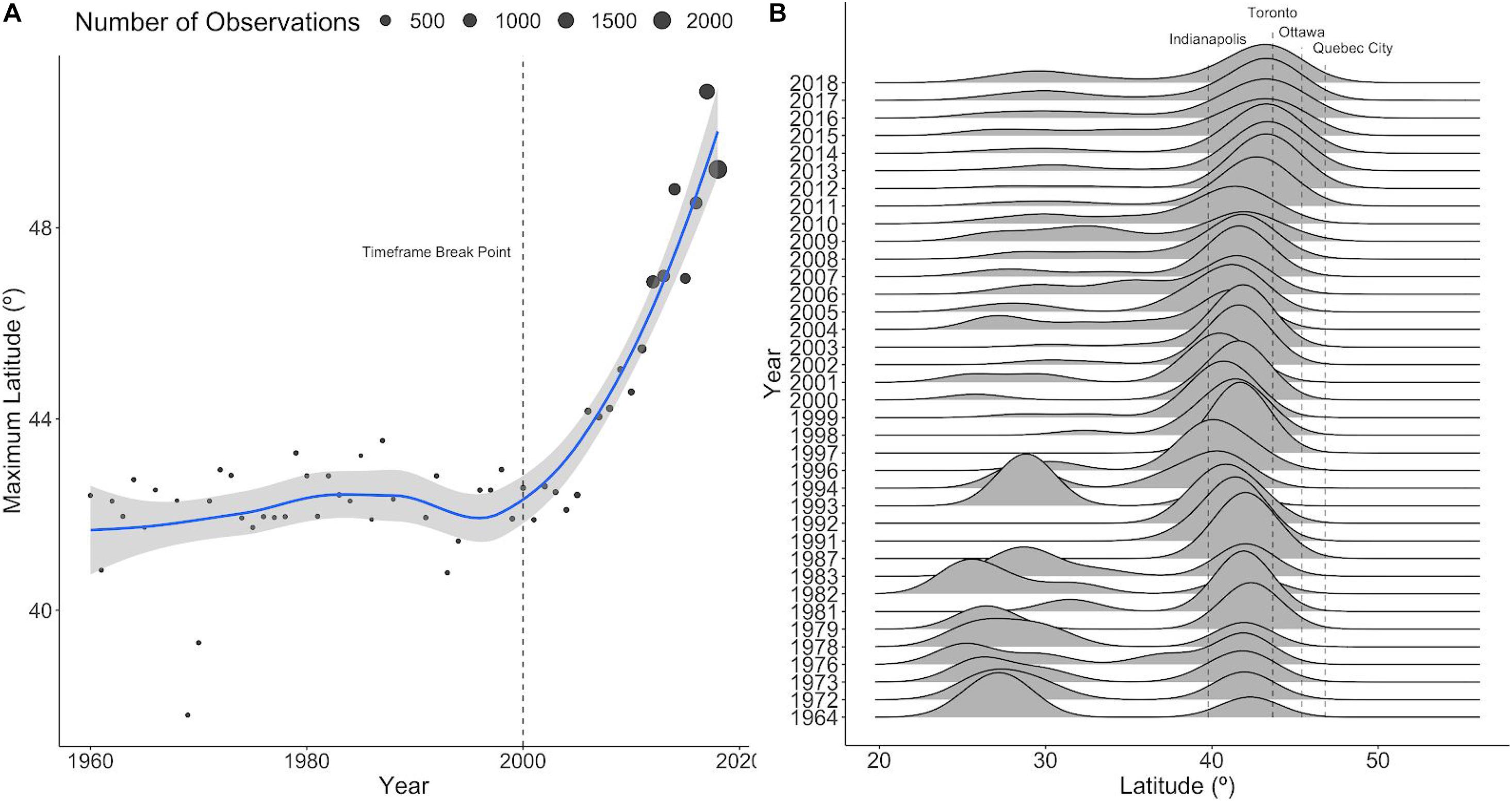
Figure 1. Evidence of northward range shift of P. cresphontes from raw occurrence data. (A) The maximum latitude of a recorded occurrence of P. cresphontes by year. Larger circles indicate years with higher numbers of occurrence records (high numbers in more recent years are due to increased citizen-science activity). The dashed lines represent the breakpoint between T1 and T2. Three years with extremely low maximum latitudes (<35) were omitted for clarity. The blue line and gray bar represent the loess smoothing curve and 95% confidence interval. (B) A ridge-plot of kernel density estimates of occurrences for P. cresphontes. Vertical dashed lines represent latitudes of major cities within the range. Years with <5 occurrence records were removed from plotting.
Data Sources
Butterfly and Host Plant Data
Papilio cresphontes (Papilionidae) is a sub-tropical butterfly widely distributed across North America. P. cresphontes and host plant occurrence data were obtained from a variety of sources: iNaturalist1, n = 3,007, Global Biodiversity Information Facility (GBIF2), n = 14,181, the Maine Butterfly Atlas3, n = 11, the Maritime Canada Butterfly Atlas4, n = 6, Massachusetts Butterfly Club, n = 512, Butterflies and Moths of North America5, n = 1,188, and eButterfly6, n = 3,083. Data from iNaturalist and GBIF were downloaded using the spocc package for R (Chamberlain et al., 2016). We filtered iNaturalist data to include only research-grade records before combining with other data sets. Combined data were filtered for time frame, duplicates, and study area extent (see below) before further analysis and model building. In total, we used 8,051 occurrence records for P. cresphontes and 2,697 occurrence records (combined) for all three host plant species.
Environmental Data
We used the TerraClimate data set (Abatzoglou et al., 2018), a 4 km × 4 km resolution gridded set of monthly climatological data from 1958 to 2017 (at the time of writing this) to generate environmental predictor variables for modeling. We calculated a set of yearly summaries of 19 bioclimatic variables (Fick and Hijmans, 2017), frequently used in species distribution modeling, using the dismo package in R (Hijmans et al., 2017) for each year in each time period (T1 and T2) and then averaged these summaries across each time period to provide temporally appropriate climate summary for each set of models. We included all 19 bioclimatic variables as predictors for modeling.
Species Distribution Models
Distributions of P. cresphontes and host plants were estimated using MaxEnt 3.4.0, a machine learning algorithm based on the principle of maximum entropy (Phillips and Dudík, 2008; Elith et al., 2011; Phillips et al., 2017). MaxEnt is a presence-background method, which is considered to perform well when modeling climatic niches across a variety of sample sizes (Wisz et al., 2008). We used the ENMeval package for model-building, testing, and tuning (Muscarella et al., 2014), ultimately building 8 total models (P. cresphontes and three host species for each time period).
We used a combination of geographically structured and regular k-fold cross validation for model testing and tuning. We generated 10,000 random background points per species-time period combination (within the geographic extent outlined by the occurrences across both time periods – a rectangle defined by the minimum and maximum latitude and longitudes of occurence points) per model and used the blockCV package (Valavi et al., 2019) to divide our study area into 400 km × 400 km blocks. Blocks were randomly assigned to folds 1-5 over 250 iterations to determine a block design that maximized evenness of occurence and background points spread across all folds. This procedure was repeated for every model (8 times in total). Occurrence and background points from folds 1-4 were used as training data for MaxEnt cross-validation and tuning, while fold 5 was reserved as a set of out-of-sample test data for final model evaluation. Throughout the manuscript, we refer to these data as test data. We used another set of random fivefold cross validation within the training data to tune model parameters (within the ENMeval package). Throughout the manuscript, we refer to these data as validation data. We tested linear, quadratic and hinge features (and all combinations) as well as a set of regularization multipliers (0.5-4 in 0.5-step increments). We examined models using a range of evaluation metrics (Supplementary Figures 1–8), but eventually chose the model with the highest area under the receiver operating characteristic curve (AUC) on validation data. All evaluation metrics were reported for the separate set of spatially explicit test data generated by blockCV (Table 1). AUC values typically range between 0.5 and 1, and can be used for relative comparisons between models with the same data (with higher values closer to 1 indicating models with better predictive capacity (Lobo et al., 2008). Once the optimal parameters for a given species and time-frame were determined, we built full models using all available occurrence data to generate predictions for subsequent visualizations and analyses. We mapped the “cloglog” MaxEnt output, which can be interpreted as probability of occurrence under the assumption that the species presence or absence at nearby sites are independent (Phillips and Dudík, 2008; Elith et al., 2011; Phillips et al., 2017). Importance of predictors was assessed using the permutation contribution metrics generated when building full models. These metrics are built as MaxEnt steps through modifications of coefficients for single features. For each variable, values are randomly permuted on training data and a model is reevaluated on the permuted data. Then, the resulting drop in AUC scores are tracked and normalized to percentages (Phillips et al., 2006). Thresholds for binary presence-absence maps and presence distributions were generated using the maximum test specificity plus sensitivity (Liu et al., 2005). For all models, we used species-specific (but not time-specific) geographic extents during model building and tuning, as well as making predictions for graphical outputs. Kernel density plots are used to show latitudinal distributions of model predictions and northern range limits.
MaxEnt has become a popular modeling resource because of its predictive power, ease of use, and a well-detailed literature to get researchers started (Phillips and Dudík, 2008; Elith et al., 2011; Phillips et al., 2017). However, this framework has also received criticism, with researchers advocating for more explicit examinations of tuning parameters, evaluation metrics, and the incorporation of tools to deal with sampling bias (Radosavljevic and Anderson, 2014). Recent software additions have addressed some of these challenges, and opened up the “black-box” of MaxEnt (Phillips et al., 2017), though issues remain, particularly in the transparency of researchers’ hyperparameter tuning and evaluation (Morales et al., 2017). To this end, we implemented recently developed tools (ENMeval and blockCV packages in R; (Muscarella et al., 2014; Valavi et al., 2019) to explicitly outline tuning (Supplementary Figures 1–8), and to incorporate a spatially independent evaluation design to minimize overfitting (along with the built-in regularization in MaxEnt).
Northern Range Limits
We calculated the distance between the northern limit modeled for P. cresphontes for T1 and T2 using a longitude class approach (Leroux et al., 2013). For each 4-km longitude class (i.e., each “column” of 4 km of longitude across the entire study area), we determined the latitude of the northernmost grid cell where the species was predicted to be present during T1 and T2. We selected the latitude-pairs (pairs of data for a single latitude at T1 and T2) for which we had grid cells with occurrence for P. cresphontes in both time periods for each longitude class and tested whether the average northern limit distribution of P. cresphontes differed between T1 and T2, using a paired t-test. We used similar methods to determine differences between northern range limits of P. cresphontes and Z. americanum for both time periods.
Results
Evidence of Northward Range Shift of P. cresphontes From Raw Occurrence Data
Patterns of occurrence (as opposed to the predictive outputs from species distribution models) indicate a strong trend of a rapid and recent northward range expansion in P. cresphontes since the earliest recorded records of the species in our dataset (1959). The butterfly’s highest recorded latitude in a given year has increased dramatically since 2000 (Figure 1A), and the predicted suitability has shifted from low to high in many cities close to the current northern edge of the range (Figure 1B).
Predictive Accuracy of Species Distribution Models
Maxent models with optimal complexity settings were chosen via hyperparameter tuning, and a variety of evaluation metrics were calculated (Supplementary Figures 1–8), but ultimately the feature classes and regularization multiplier of the model with the highest average validation AUC was used for each species-time period pair. Once the final parameter set was chosen, models were evaluated on spatially explicit out-of-sample test data created by blockCV. Overall, models had high predictive accuracy on test data, with AUC scores ranging from 0.871 to 0.957 (Table 1). Generally, models were complex and incorporated combinations of feature classes paired with regularization multipliers (Table 1). Final models were generated using the parameter set (feature classes and regularization multiplier) described above, but built with the full set of data (training + test) to generate predictive maps (Figures 2, 3) and distributions (Figures 4, 5).
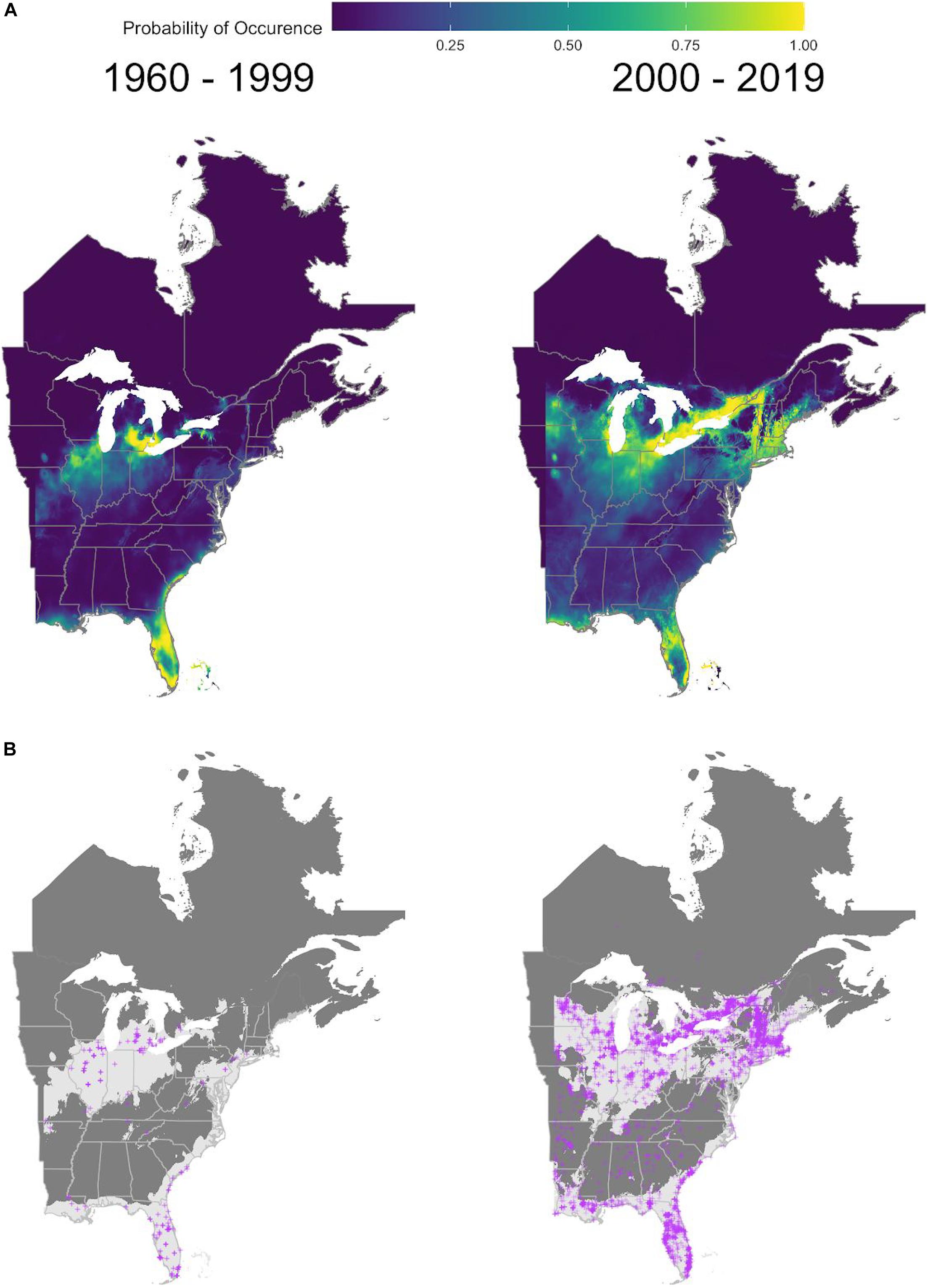
Figure 2. MaxEnt model predictions for the giant swallowtail butterfly, P. cresphontes for t1 (1959-1999) and t2 (2000-2018). (A) cloglog transformed output from full MaxEnt models for two time periods. Lighter yellow areas denote higher probabilities of occurrence. (B) Threshold maps of presence absence for the two time periods. Light areas represent predicted occurrence and dark gray represents predicted absence. Purple crosses represent actual occurrence data for each time period.
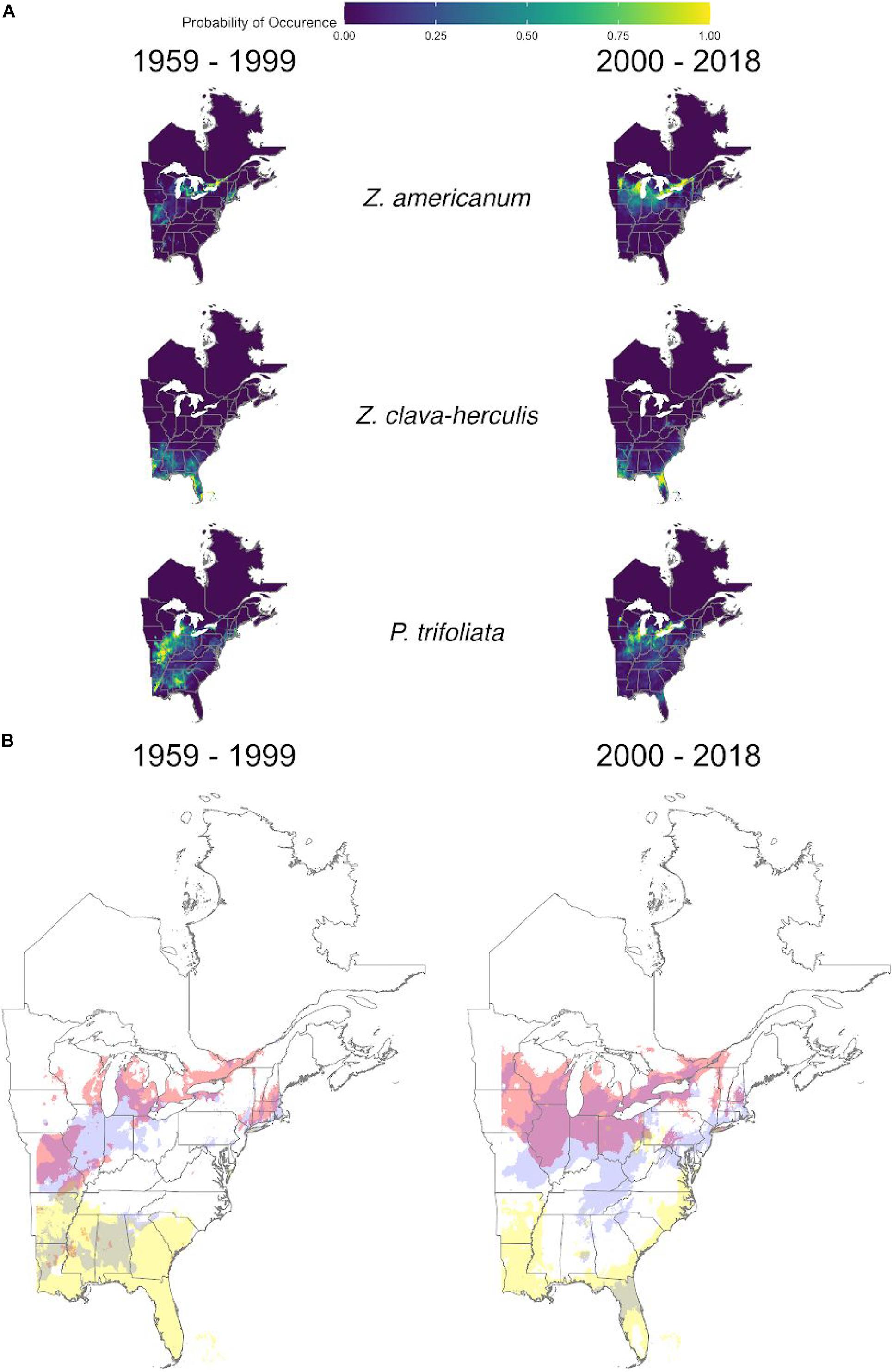
Figure 3. MaxEnt model predictions for predominant giant swallowtail butterfly host plants (Z. americanum, Z. clava-herculis and P. trifoliata) for T1 (1959-1999) and T2 (2000-2018). (A) cloglog transformed output from full MaxEnt models for each host plant across two time periods. Lighter yellow areas denote higher probabilities of occurrence. (B) Threshold maps of presence absence for the two time periods. Different colors (red, blue, and yellow) represent areas of predicted occurrence for each host plant and white represents predicted absence. Mixed colors indicate areas of overlap.
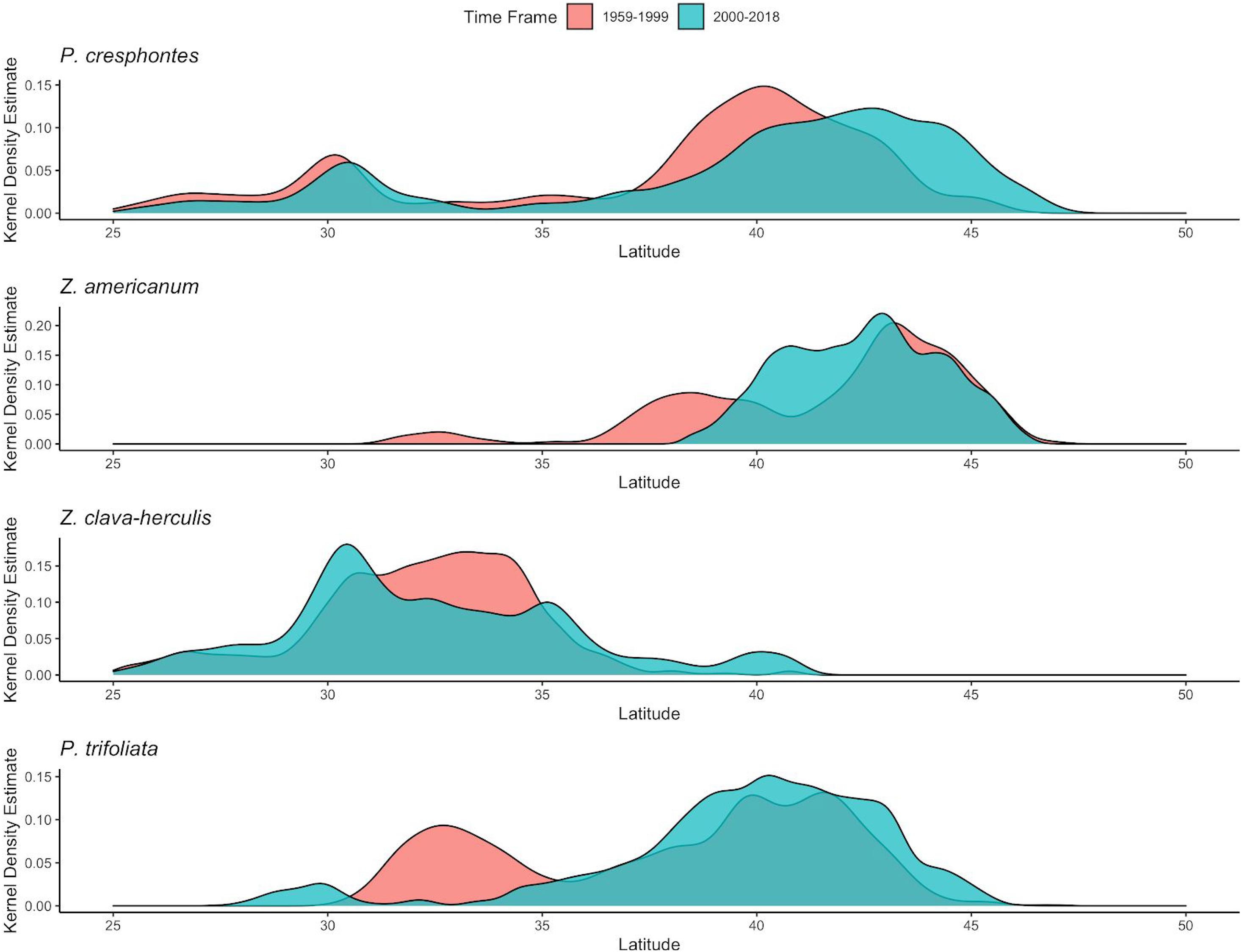
Figure 4. Kernel density estimates for modeled predicted presence of giant swallowtail butterfly P. cresphontes and host plants (Z. americanum, Z. clava-herculis and P. trifoliata) for T1 (1959-1999) and T2 (2000-2018). Red plots are from T1 (1959-1999) and blue plots are from T2 (2000-2018).
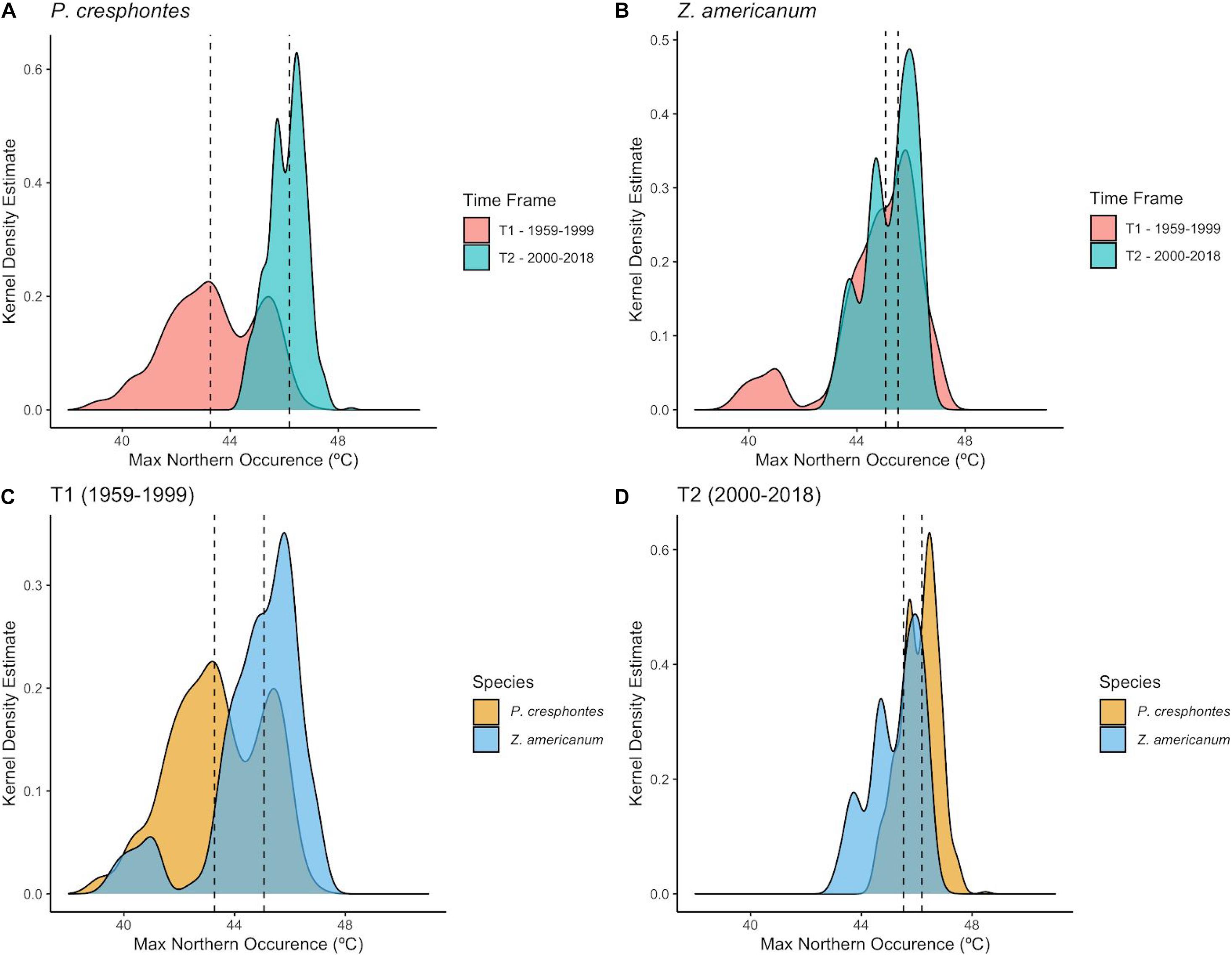
Figure 5. Predicted maximum northern occurence for P. cresphontes and host plants for T1 (1959-1999) and T2 (2000-2018). Dashed vertical lines represent the median value for each group. (A) P. cresphontes northern range limit between the two time periods. (B) Z. americanum northern range limit between the two time periods. (C) Northern range limit comparison for T1 (1959-1999) for P. cresphontes and Z. americanum. (D) Northern range limit comparison for T2 (2000-2018) for P. cresphontes and Z. americanum.
Papilio cresphontes Has Expanded Northward Due to Recent Climate Warming
Predictive maps generated from MaxEnt models clearly show a change in the distribution of P. cresphontes between T1 and T2, with a northward expansion since 2000 (Figures 2A,B). Kernel density estimate plots generated from threshold occurence predictions mirror this result (Figure 4), and highlight that different parts of P. cresphontes’ range match host plant use. Z. americanum closely matches P. cresphontes in the north, while the middle and southern part of the range is defined by the presence of Z. clava-herculis and P. trifoliata.
Host Plant Range Shifts
Overall, host plants (Z. americanum, Z. clava-herculis and P. trifoliata) demonstrated more complex changes in distribution between T1 and T2 compared to P. cresphontes (Figures 3A,B). Historically, the species were split latitudinally (with significant overlap) with Z. americanum occupying the northern part of the study area, P. trifoliata the middle, and Z. clava-herculis in the far south (Figure 3B). However, this pattern changes subtly in T2, with a range expansion of Z. americanum northward, but also westward to the boundary of our study area. Distribution changes in other host plants were more complex, with complicated range changes for P. trifoliata in the middle latitudes of the study area, and small range contraction of Z. clava-herculis to the south.
Northern Range Limits for P. cresphontes Have Shifted Northward and Closely Match Z. americanum
The northern range limit of P. cresphontes was significantly higher in T2 compared to T1 (t = −38.181, df = 560, p < 0.001; Figure 5A) where the median northern-most occurence for T2 (median = 46.1875 ± 0.675°) was 2.917° (∼324 km) higher in latitude than T1 (median = 43.2708 ± 1.692°). Z. americanum also demonstrated a significant (but small) northern range shift between T1 and T2 (t = −6.5717, df = 5510, p < 0.001; Figure 5B) where the median northern-most occurence for T2 (median = 45.5208 ± 0.914°) was 0.458° (∼51 km) higher in latitude than T1 (median = 45.0625. ± 1.667°). We also tested whether the northern range limits of P. cresphontes and Z. americanum differed from each other during each time period. In each time period, there was a significant difference between the northern range limits of P. cresphontes and Z. americanum (T1: t = −17.485, df = 550, p < 0.001; T2: t = 16.771, df = 551, p < 0.001). The difference between median butterfly and host plant northern range limits shrank from 1.75° (∼194 km) in T1 (with Z. americanum having a higher northern range limit) to 0.77° (∼85.47 km) in T2 (with P. cresphontes having a slightly higher median northern range limit; Figures 5B,C).
Climatic Variation in the Study Area Between T1 and T2
Overall, T2 had a higher mean annual temperature (9.45 ± 6.20° C) than T1 (8.67 ± 6.27°C) (t = −45.274, df = 534850, p < 0.001). Bioclim variables 10 and 11 [mean temperature of warmest quarter (breeding season) and mean temperature of the coldest quarter (pupal overwintering season)] had the biggest impacts on predicting P. cresphontes distribution, while variables 9 (mean temperature of driest quarter), 10 (mean temperature of warmest quarter) and 3 (isothermality) had the biggest impacts across both time periods for Z. americanum. Other host plants had multiple bioclim variables across time periods that impact distribution models (Figure 6). Variables that commonly had high permutation importance scores showed significant differences between T1 and T2 on average across our study area, with an overall trend of warmer patterns from 2000 to 2015 (T2) compared to 1959-1999 (T1) (Table 2).
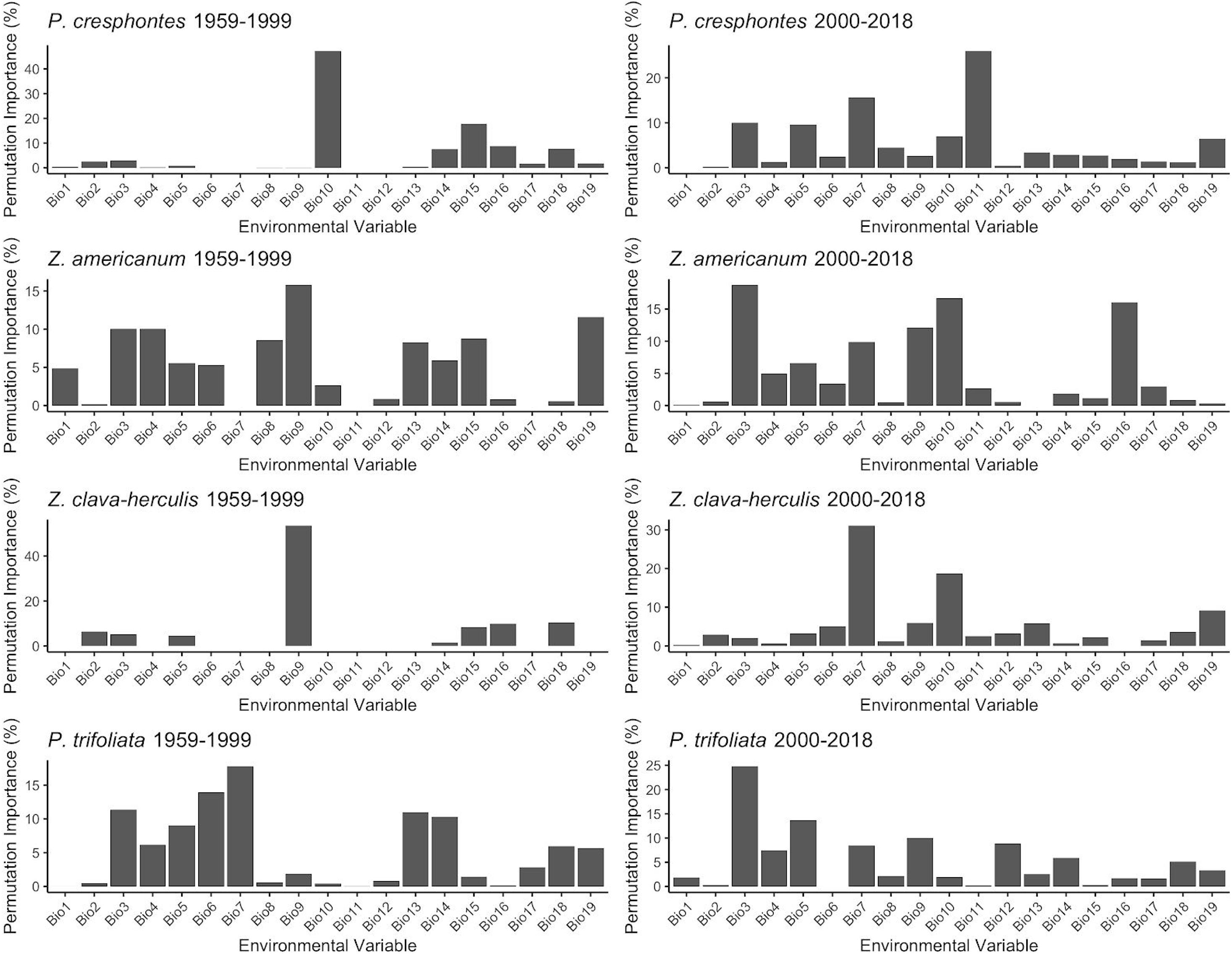
Figure 6. Percent contribution of each of the 19 bioclim variables to final models for each species and time period. Percentages are computed from MaxEnt model training – as predictive gains increase, environmental factors contributing to feature generation are calculated and summarized in the final model. Common major contributors across many models include BIO9 (mean temperature of the driest quarter), BIO10 (mean temperature of the warmest quarter) and BIO11 (mean temperature of the coldest quarter).

Table 2. Bioclimatic shifts in Bioclim variables between T1 (1959-1999) and T2 (2000-2015) that impact butterfly and host plant distributions.
Discussion
The determinants of species distributions have long been debated not just because they are essential in ecology and evolutionary biology, but also because where organisms are and where they will be is central to successful conservation and restoration practices in light of rapid climate change (Buckley et al., 2013; Gallagher et al., 2013; Robillard et al., 2015). Our study details a recent and rapid northward range expansion by P. cresphontes between 2000 and 2018 (Figure 1). We also model the distributions of the butterfly’s naturally occurring larval host plants, which, when combined with analysis of P. cresphontes range, result in different conclusions for the future distribution of this butterfly than if we had relied on abiotic variables alone (Figures 2, 3). Recent climatic shifts, particularly warmer, wetter temperatures during breeding season and warmer temperatures during pupal overwintering season, have allowed P. cresphontes to rapidly expand northward to now match or even surpass the slower moving northward range expansion of the northernmost host plant, Z. americanum, with further northward expansion of P. cresphontes now limited by host plant range, not climate (Figure 4). Our results highlight the importance of including biotic interactions (and interactions between herbivorous insects and host plants in particular) in examinations of range shifts and their speed, an idea often highlighted, (Urban et al., 2016) but infrequently implemented (Lemoine, 2015; Dilts et al., 2019; Svancara et al., 2019).
Poleward range shifts in herbivorous insects, particularly butterflies, have been documented for a number of species (Parmesan et al., 1999; Warren et al., 2001; Pöyry et al., 2009; Breed et al., 2012). Additionally, northward expansions of other butterfly species have been shown to have dramatic impacts on community composition through linked biotic interactions (Audusseau et al., 2017), which could be happening in this system as well, but would require further examination to determine. While studies demonstrating range shifts in multiple taxa provide valuable insights into the magnitude and direction of shifts for different taxa, gaps in knowledge remain (Pöyry et al., 2009). Namely, (1) how has warming acceleration affected recent range shifts during the last 10-15 years in poleward latitudes, and (2) how do abiotic and biotic factors interact to shape range shifts? Our study addresses both of these questions and provides a scalable, data acquisition and analytic pipeline by focusing on a single herbivore and multiple host plant species. We show an usually rapid northward range shift in this insect herbivore, P. cresphontes, over the last 18 years (predicted most northward occurrences differ by 2.917° of latitude (∼ 324 km) between T1 and T2, or a northward expansion of 180 km/decade) that is more than 27 times faster than the average of northward movement of global meta-analyses for plants, lichens, birds, mammals, insects, reptiles and amphibians, fish and marine organisms (Parmesan and Yohe, 2003) and over nine times faster than all butterfly species in Britain (Hickling et al., 2006). These observations are associated with warmer, wetter climate conditions during active flight times and overwintering. Our findings largely follow (Pöyry et al., 2009), who postulate that mobile species utilizing woody host plants like P. crespontes should exhibit large and fast range shifts northward, and that habitat availability and dispersal capacity largely determine success. We have laid the groundwork for one way to gather large amounts of data and analyze it as scale for future work across all butterfly and host plant species.
Interestingly, the northward incursion of P. cresphontes in northeastern North America is not a new phenomenon. Accounts detail movement into the region 145 years ago that lasted several decades (Scudder, 1889). In 1875, P. cresphontes were found in southern New England and by 1882 there are documented records just south of Montreal, Quebec. By the 1930s, the species had apparently retracted southward and were considered “extremely rare” in Massachusetts (Farquhar, 1934) and did not push northward into the region again until the last 8 years. Multiple long-term climate reconstructions (paired with historic instrument data) for the 145-year incursion period indicate a strong warming trend compared to the previous century (Marlon et al., 2016). However, this warming trend continues through the 1930s, so it is unclear which factors may have resulted in a retraction, though hydroclimatic reconstructions indicate an increase in drought in the northeastern United States over this time period, which likely had strong impacts on host-plant/nectar-plant distributions and quality through the range of P. cresphontes (Marlon et al., 2016), not to mention direct impacts on insect survival.
Our work also highlights the importance of including biotic interactions when predicting and projecting range shifts. Papillio cresphontes’ current northern range now closely matches the northernmost host plant (Z. americanum) (Figures 3, 5D) and this butterfly species is now limited by the ability of Z. americanum to expand its range northward. Because of the differences in life-history strategies, dispersal capabilities, reproductive outputs and environmental tolerances between insect and host plant, the northern expansion of P. cresphontes appears to now be largely curbed as the host plant is much more sessile and has much longer generation times. Though sightings of the winged adult stage of P. cresphontes will likely continue to be seen further north than the naturally occurring host plant range (Figure 5D), without a suitable host plant, further northward expansion seems unlikely but may be facilitated by recently documented P. cresphontes occurrences in horticultural settings. Papilio cresphontes lay eggs and larvae feed successfully on two non-native garden plants, garden rue (Ruta graveolens) and gas plant (Dictamnus albus). Common hoptree (P. trifoliata), is increasingly planted as an ornamental in the Northeast yet is a native species from central and southeastern North America. Although these exotics are not distributed uniformly across the region, dispersing P. cresphontes have an uncanny ability to find host plants in complex environments, perhaps further enabling them to expand their range in urban and suburban areas as abiotic conditions allow.
Data from community science sources continue to grow as platforms become more popular, and can provide tremendous boons to researchers across disciplines (Bonney et al., 2009, 2014; Dickinson et al., 2010), including those interested in creating species distribution models (Kéry et al., 2010; Yu et al., 2010). There has been debate about the quality and veracity of community science data, but recent work has demonstrated that citizen science initiatives can reliably produce research quality data though it often has similar biases to professionally-gathered data (Kosmala et al., 2016). Here, we use community science data sources supplemented by data from museum collections to generate species distribution models using the well-established MaxEnt modeling framework (Phillips and Dudík, 2008; Elith et al., 2011; Phillips et al., 2017), and advocate for continued development and use of community science data and its pairing with museum collection data in developing species distribution models in ecology and conservation.
Though we focused mostly on the distributional changes of P. cresphontes, there were also surprisingly large range shifts in host plant species (Figure 3). In contrast to the straightforward northward expansion of P. cresphontes, the distributional changes in host plants were more complex and nuanced. Z. americanum and P. trifoliata have both shifted northward between the two time periods in slightly different patterns (Figures 3, 4). While P. trifoliata appears to have shifted mostly northward (primarily gone from a large southern zone in T1), Z. americanum has undergone a northward and westward shift, and occupies areas that overlap with the range of P. trifoliata (Figure 3). The potential effects of this overlap on P. cresphontes (i.e., population dynamics, apparent competition, selection for oviposition behavior) are to our knowledge currently unknown, and warrants further examination in light of P. cresphontes westward expansion and previous work demonstrating significant within-population variation in oviposition behavior in Papilio (Thompson, 1988). Interestingly, mean temperature and annual temperature range (Bioclim variables 1 and 7) had the strongest impact in predicting the distribution of Z. americanum in T2, highlighting the impact that temperature may have in shaping and limiting current distribution. In contrast, the range of Z. clava-herculis appears to have contracted slightly in the southern United States. Compared to pre-2,000 distributions, available host plants to P. cresphontes are more widely distributed with greater overlap, but with notable gaps throughout portions of the southern United States. These complex distributional changes are likely driving part of the overall range shift northward for P. cresphontes (Figure 1B) and could also be potential drivers of speciation, and the evolution of specialization or host plant switching now and in the future (Descombes et al., 2016).
Conclusion
Multiple biotic interactions have evolved between insects and other species to create a wide variety of ecosystem services including herbivory and pollination (Losey and Vaughan, 2006). Anthropogenic climate change and habitat loss are creating a growing urgency for quantifying range size, understanding range boundaries, and assessing range shifts across insect species in order to preserve the integrity of future ecosystem function. Our work outlines the power of using increasingly abundant citizen science data, as well as the importance of including biotic interactions alongside environmental factors when developing analytical pipelines for biodiversity benchmarking studies. Future work should also incorporate climate change estimates into modeling efforts to project future distributions for both herbivores and host plants across many more butterfly and plant species. Incorporating both abiotic and biotic interactions in biodiversity benchmarking will provide a deeper, more nuanced understanding of temporal and spatial overlap among species, guiding conservation and management practices in a rapidly changing climate.
Statement of Data Archiving
Data and R scripts for all analyses are archived on Zenodo (https://doi.org/10.5281/zenodo.4476735).
Data Availability Statement
The datasets presented in this study can be found in online repositories. The names of the repository/repositories and accession number(s) can be found below: Zenodo doi: 10.5281/zenodo.4476735 (also in mansucript under Statement of Data Archiving).
Author Contributions
ML, KM, KP, DB, and JK conducted the project conception. JW carried out analyses (data acquisition, model building, statistical analyses, and visualization) with initial explorations of data and analyses from NC and support from RH. JW led manuscript preparation, with initial pieces in place from NC and DB. All authors supported in editing, commenting and adding material to the manuscript.
Funding
RH was supported in part by the National Science Foundation under grant no. 1910118.
Conflict of Interest
The authors declare that the research was conducted in the absence of any commercial or financial relationships that could be construed as a potential conflict of interest.
Acknowledgments
We would like to thank our reviewers and editors and Jeff Oliver for helpful comments and suggestions on the manuscript and analyses. Specifically, we would like to thank the Global Biodiversity Information Facility, the Maine Butterfly Atlas, the Maritime Canada Butterfly Atlas, the Massachusetts Butterfly Club, Butterflies and Moths of North America and eButterfly for the use of their data. We would also like to thank Scott Laurie and Ken-ichi Ueda at iNaturalist for their web platform, and all those relentless volunteers in various citizen science programs, past and present, who contributed these data for the greater good. This manuscript has been released as a pre-print at bioRxiv, (Wilson et al., 2019).
Supplementary Material
The Supplementary Material for this article can be found online at: https://www.frontiersin.org/articles/10.3389/fevo.2021.579230/full#supplementary-material
Supplementary Figure 1 | Hyperparameter tuning and model evaluation of P. cresphontes distribution during the T1 period.
Supplementary Figure 2 | Hyperparameter tuning and model evaluation of P. cresphontes distribution during the T2 period.
Supplementary Figure 3 | Hyperparameter tuning and model evaluation of Z. americanum distribution during the T1 period.
Supplementary Figure 4 | Hyperparameter tuning and model evaluation of Z. americanum distribution during the T2 period.
Supplementary Figure 5 | Hyperparameter tuning and model evaluation of Z. clava-herculis distribution during the T1 period.
Supplementary Figure 6 | Hyperparameter tuning and model evaluation of Z. clava-herculis distribution during the T2 period.
Supplementary Figure 7 | Hyperparameter tuning and model evaluation of P. trifoliata distribution during the T1 period.
Supplementary Figure 8 | Hyperparameter tuning and model evaluation of P. trifoliata distribution during the T2 period.
Supplementary Table 1 | Bioclim variable definitions.
Footnotes
- ^ www.inaturalist.org
- ^ www.gbif.org
- ^ https://mbs.umf.maine.edu
- ^ http://accdc.com/mba/index-mba.html
- ^ www.butterfliesandmoths.org
- ^ www.e-butterfly.org
References
Abatzoglou, J. T., Dobrowski, S. Z., Parks, S. A., and Hegewisch, K. C. (2018). TerraClimate, a high-resolution global dataset of monthly climate and climatic water balance from 1958-2015. Sci. Data 5:170191. doi: 10.1038/sdata.2017.191
Altieri, M. A., Nicholls, C. I., Henao, A., and Lana, M. A. (2015). Agroecology and the design of climate change-resilient farming systems. Agron. Sustain. Dev. 35, 869–890. doi: 10.1007/s13593-015-0285-2
Audusseau, H., Le Vaillant, M., Janz, N., Nylin, S., Karlsson, B., and Schmucki, R. (2017). Species range expansion constrains the ecological niches of resident butterflies. J. Biogeogr. 44, 28–38. doi: 10.1111/jbi.12787
Bader, M. Y., van Geloof, I., and Rietkerk, M. (2007). High solar radiation hinders tree regeneration above the alpine treeline in northern Ecuador. Plant Ecol. 191, 33–45. doi: 10.1007/s11258-006-9212-6
Bale, J. S., Masters, G. J., Hodkinson, I. D., Awmack, C., Martijn Bezemer, T., Brown, V. K., et al. (2002). Herbivory in global climate change research: direct effects of rising temperature on insect herbivores. Glob. Change Biol. 8, 1–16. doi: 10.1046/j.1365-2486.2002.00451.x
Battisti, A., Stastny, M., Netherer, S., Robinet, C., Schopf, A., Roques, A., et al. (2005). Expansion of geographic range in the pine processionary moth caused by increased winter temperatures. Ecol. Appl. 15, 2084–2096. doi: 10.1890/04-1903
Blois, J. L., Zarnetske, P. L., Fitzpatrick, M. C., and Finnegan, S. (2013). Climate change and the past, present, and future of biotic interactions. Science 341, 499–504. doi: 10.1126/science.1237184
Bonney, R., Cooper, C. B., Dickinson, J., Kelling, S., Phillips, T., Rosenberg, K. V., et al. (2009). Citizen Science: a developing tool for expanding science knowledge and scientific literacy. BioScience 59, 977–984. doi: 10.1525/bio.2009.59.11.9
Bonney, R., Shirk, J. L., Phillips, T. B., Wiggins, A., Ballard, H. L., Miller-Rushing, A. J., et al. (2014). Citizen science. Next steps for citizen science. Science 343, 1436–1437. doi: 10.1126/science.1251554
Breed, G. A., Stichter, S., and Crone, E. E. (2012). Climate-driven changes in northeastern US butterfly communities. Nat. Clim. Change 3, 142–145. doi: 10.1038/nclimate1663
Brown, C. J., O’Connor, M. I., Poloczanska, E. S., Schoeman, D. S., Buckley, L. B., Burrows, M. T., et al. (2016). Ecological and methodological drivers of species’ distribution and phenology responses to climate change. Glob. Change Biol. 22, 1548–1560. doi: 10.1111/gcb.13184
Buckley, R., Morrison, C., Guy Castley, J., de Vasconcellos Pegas, F., and Mossaz, A. (2013). Conservation science and practice. Biol. Conserv. 162:133. doi: 10.1016/j.biocon.2013.03.020
Bueno de Mesquita, C. P., King, A. J., Schmidt, S. K., Farrer, E. C., and Suding, K. N. (2016). Incorporating biotic factors in species distribution modeling: are interactions with soil microbes important? Ecography 39, 970–980. doi: 10.1111/ecog.01797
Cannon, R. J. C. (1998). The implications of predicted climate change for insect pests in the UK, with emphasis on non-indigenous species. Glob. Change Biol. 4, 785–796. doi: 10.1046/j.1365-2486.1998.00190.x
Castex, V., Beniston, M., Calanca, P., Fleury, D., and Moreau, J. (2018). Pest management under climate change: the importance of understanding tritrophic relations. Sci. Tot. Environ. 616, 397–407. doi: 10.1016/j.scitotenv.2017.11.027
Chalcoff, V. R., Aizen, M. A., and Ezcurra, C. (2012). Erosion of a pollination mutualism along an environmental gradient in a south Andean treelet, Embothrium coccineum (Proteaceae). Oikos 121, 471–480. doi: 10.1111/j.1600-0706.2011.19663.x
Chamberlain, S., Ram, K., and Hart, T. (2016). spocc: Interface to Species Occurrence Data Sources. R Package Version 0. 5. 0.
Connell, J. H. (1961). The influence of interspecific competition and other factors on the distribution of the barnacle Chthamalus stellatus. Ecology 42, 710–723. doi: 10.2307/1933500
Dempster, J. P., and Pollard, E. (1981). Fluctuations in resource availability and insect populations. Oecologia 50, 412–416. doi: 10.1007/BF00344984
Descombes, P., Pradervand, J.-N., Golay, J., Guisan, A., and Pellissier, L. (2016). Simulated shifts in trophic niche breadth modulate range loss of alpine butterflies under climate change. Ecography 39, 796–804. doi: 10.1111/ecog.01557
Deutsch, C. A., Tewksbury, J. J., Huey, R. B., Sheldon, K. S., Ghalambor, C. K., Haak, D. C., et al. (2008). Impacts of climate warming on terrestrial ectotherms across latitude. Proc. Natl. Acad. Sci. U.S.A. 105, 6668–6672. doi: 10.1073/pnas.0709472105
Dickinson, J. L., Zuckerberg, B., and Bonter, D. N. (2010). Citizen Science as an ecological research tool: challenges and benefits. Annu. Rev. Ecol. Evol. Syst. 41, 149–172. doi: 10.1146/annurev-ecolsys-102209-144636
Dilts, T. E., Steele, M. O., Engler, J. D., Pelton, E. M., Jepsen, S. J., McKnight, S. J., et al. (2019). Host plants and climate structure habitat associations of the western monarch butterfly. Front. Ecol. Evol. 7:188. doi: 10.3389/fevo.2019.00188
Elith, J., and Leathwick, J. R. (2009). Species distribution models: ecological explanation and prediction across space and time. Annu. Rev. Ecol. Evol. Syst. 40, 677–697. doi: 10.1146/annurev.ecolsys.110308.120159
Elith, J., Phillips, S. J., Hastie, T., Dudík, M., Chee, Y. E., and Yates, C. J. (2011). A statistical explanation of MaxEnt for ecologists. Divers. Distrib. 17, 43–57. doi: 10.1111/j.1472-4642.2010.00725.x
Ettinger, A., and HilleRisLambers, J. (2017). Competition and facilitation may lead to asymmetric range shift dynamics with climate change. Glob. Change Biol. 23, 3921–3933. doi: 10.1111/gcb.13649
Farquhar, D. W. (1934). The Lepidoptera of New England. Ph.D. dissertation, Harvard University, Cambridge, MA.
Feng, X., Qiao, H., and Enquist, B. J. (2020). Doubling demands in programming skills call for ecoinformatics education. Front. Ecol. Environ. 18, 123–124. doi: 10.1002/fee.2179
Fick, S. E., and Hijmans, R. J. (2017). WorldClim 2: new 1-km spatial resolution climate surfaces for global land areas. Int. J. Climatol. 37, 4302–4315. doi: 10.1002/joc.5086
Finkbeiner, S. D., Reed, R. D., Dirig, R., and Losey, J. E. (2011). The role of environmental factors in the northeastern range expansion of Papilio cresphontes Cramer (Papilionidae). J. Lepidopterists Soc. 65, 119–125. doi: 10.18473/lepi.v65i2.a4
Freeman, R. S., Brody, A. K., and Neefus, C. D. (2003). Flowering phenology and compensation for herbivory in Ipomopsis aggregata. Oecologia 136, 394–401. doi: 10.1007/s00442-003-1276-6
Gallagher, R. V., Hughes, L., and Leishman, M. R. (2013). Species loss and gain in communities under future climate change: consequences for functional diversity. Ecography 36, 531–540. doi: 10.1111/j.1600-0587.2012.07514.x
Harrington, R., Fleming, R. A., and Woiwod, I. P. (2001). Climate change impacts on insect management and conservation in temperate regions: can they be predicted? Agric. For. Entomol. 3, 233–240. doi: 10.1046/j.1461-9555.2001.00120.x
Hickling, R., Roy, D. B., Hill, J. K., Fox, R., and Thomas, C. D. (2006). The distributions of a wide range of taxonomic groups are expanding polewards. Glob. Change Biol. 12, 450–455. doi: 10.1111/j.1365-2486.2006.01116.x
Hijmans, R. J., Phillips, S., Leathwick, J., Elith, J., and Hijmans, M. R. J. (2017). Package “dismo.”. Circles 9, 1–68.
HilleRisLambers, J., Harsch, M. A., Ettinger, A. K., Ford, K. R., and Theobald, E. J. (2013). How will biotic interactions influence climate change-induced range shifts? Ann. N.Y. Acad. Sci. 1297, 112–125.
Huey, R. B., Deutsch, C. A., Tewksbury, J. J., Vitt, L. J., Hertz, P. E., Álvarez Pérez, H. J., et al. (2009). Why tropical forest lizards are vulnerable to climate warming. Proc. R. Soc. B Biol. Sci. 276, 1939–1948. doi: 10.1098/rspb.2008.1957
Kerr, J. T., Pindar, A., Galpern, P., Packer, L., Potts, S. G., Roberts, S. M., et al. (2015). Climate change impacts on bumblebees converge across continents. Science 349, 177–180. doi: 10.1126/science.aaa7031
Kéry, M., Gardner, B., and Monnerat, C. (2010). Predicting species distributions from checklist data using site-occupancy models. J. Biogeogr. 37, 1851–1862. doi: 10.1111/j.1365-2699.2010.02345.x
Kingsolver, J. G., Woods, H. A., Buckley, L. B., Potter, K. A., MacLean, H. J., and Higgins, J. K. (2011). Complex life cycles and the responses of insects to climate change. Integr. Compar. Biol. 51, 719–732. doi: 10.1093/icb/icr015
Kosmala, M., Wiggins, A., Swanson, A., and Simmons, B. (2016). Assessing data quality in citizen science. Front. Ecol. Environ. 14, 551–560. doi: 10.1002/fee.1436
Lany, N. K., Zarnetske, P. L., Schliep, E. M., Schaeffer, R. N., Orians, C. M., Orwig, D. A., et al. (2018). Asymmetric biotic interactions and abiotic niche differences revealed by a dynamic joint species distribution model. Ecology 99, 1018–1023. doi: 10.1002/ecy.2190
Lemoine, N. P. (2015). Climate change may alter breeding ground distributions of eastern migratory monarchs (Danaus plexippus) via range expansion of Asclepias host plants. PLoS One 10:e0118614. doi: 10.1371/journal.pone.0118614
Leroux, S. J., Larrivée, M., Boucher-Lalonde, V., Hurford, A., Zuloaga, J., Kerr, J. T., et al. (2013). Mechanistic models for the spatial spread of species under climate change. Ecol. Appl. 23, 815–828. doi: 10.1890/12-1407.1
Liu, C., Berry, P. M., Dawson, T. P., and Pearson, R. G. (2005). Selecting thresholds of occurrence in the prediction of species distributions. Ecography 28, 385–393. doi: 10.1111/j.0906-7590.2005.03957.x
Lobo, J. M., Jiménez-Valverde, A., and Real, R. (2008). AUC: a misleading measure of the performance of predictive distribution models. Glob. Ecol. Biogeogr. 17, 145–151. doi: 10.1111/j.1466-8238.2007.00358.x
Losey, J. E., and Vaughan, M. (2006). The economic value of ecological services provided by insects. BioScience 56:311.
Louthan, A. M., Doak, D. F., and Angert, A. L. (2015). Where and when do species interactions set range limits? Trends Ecol. Evol. 30, 780–792. doi: 10.1016/j.tree.2015.09.011
Marlon, J. R., Pederson, N., Nolan, C., Goring, S., Shuman, B., Booth, R., et al. (2016). Climatic history of the northeastern United States during the past 3000 years. Clim. Past 13, 1355–1378. doi: 10.5194/cp-13-1355-2017
Moeller, D. A., Geber, M. A., Eckhart, V. M., and Tiffin, P. (2012). Reduced pollinator service and elevated pollen limitation at the geographic range limit of an annual plant. Ecology 93, 1036–1048. doi: 10.1890/11-1462.1
Morales, N. S., Fernández, I. C., and Baca-González, V. (2017). MaxEnt’s parameter configuration and small samples: are we paying attention to recommendations? A systematic review. PeerJ 5:e3093. doi: 10.7717/peerj.3093
Muscarella, R., Galante, P. J., Soley-Guardia, M., Boria, R. A., Kass, J. M., Uriarte, M., et al. (2014). ENMeval: an R package for conducting spatially independent evaluations and estimating optimal model complexity for MaxEnt ecological niche models. Methods Ecol. Evol. 5, 1198–1205. doi: 10.1111/2041-210x.12261
Palacio, F. X., and Girini, J. M. (2018). Biotic interactions in species distribution models enhance model performance and shed light on natural history of rare birds: a case study using the straight-billed reedhaunter Limnoctites rectirostris. J. Avian Biol. 49:e01743. doi: 10.1111/jav.01743
Parmesan, C., Ryrholm, N., Stefanescu, C., Hill, J. K., Thomas, C. D., Descimon, H., et al. (1999). Poleward shifts in geographical ranges of butterfly species associated with regional warming. Nature 399, 579–583. doi: 10.1038/21181
Parmesan, C., and Yohe, G. (2003). A globally coherent fingerprint of climate change impacts across natural systems. Nature 421, 37–42. doi: 10.1038/nature01286
Pearson, D. L., and Knisley, C. B. (1985). Evidence for food as a limiting resource in the life cycle of tiger beetles (Coleoptera: Cicindelidae). Oikos 45, 161–168. doi: 10.2307/3565701
Phillips, S. J., Anderson, R. P., Dudík, M., Schapire, R. E., and Blair, M. E. (2017). Opening the black box: an open-source release of MaxEnt. Ecography 40, 887–893. doi: 10.1111/ecog.03049
Phillips, S. J., Anderson, R. P., and Schapire, R. E. (2006). Maximum entropy modeling of species geographic distributions. Ecol. Model. 190, 231–259. doi: 10.1016/j.ecolmodel.2005.03.026
Phillips, S. J., and Dudík, M. (2008). Modeling of species distributions with MaxEnt: new extensions and a comprehensive evaluation. Ecography 31, 161–175. doi: 10.1111/j.0906-7590.2007.5203.x
Poloczanska, E. S., Brown, C. J., Sydeman, W. J., Kiessling, W., Schoeman, D. S., Moore, P. J., et al. (2013). Global imprint of climate change on marine life. Nat. Clim. Change 3, 919–925. doi: 10.1038/nclimate1958
Porter, J. H., Parry, M. L., and Carter, T. R. (1991). The potential effects of climatic change on agricultural insect pests. Agric. For. Meteorol. 57, 221–240. doi: 10.1016/0168-1923(91)90088-8
Pöyry, J., Luoto, M., Heikkinen, R. K., Kuussaari, M., and Saarinen, K. (2009). Species traits explain recent range shifts of Finnish butterflies. Glob. Change Biol. 15, 732–743. doi: 10.1111/j.1365-2486.2008.01789.x
Prudic, K. L., McFarland, K. P., Oliver, J. C., Hutchinson, R. A., Long, E. C., Kerr, J. T., et al. (2017). eButterfly: leveraging massive online citizen science for butterfly conservation. Insects 8:53. doi: 10.3390/insects8020053
Radosavljevic, A., and Anderson, R. P. (2014). Making better MaxEnt models of species distributions: complexity, overfitting and evaluation. J. Biogeogr. 41, 629–643. doi: 10.1111/jbi.12227
Rahel, F. J., and Olden, J. D. (2008). Assessing the effects of climate change on aquatic invasive species. Conserv. Biol. 22, 521–533. doi: 10.1111/j.1523-1739.2008.00950.x
Robillard, C. M., Coristine, L. E., Soares, R. N., and Kerr, J. T. (2015). Facilitating climate-change-induced range shifts across continental land-use barriers. Conserv. Biol. 29, 1586–1595. doi: 10.1111/cobi.12556
Robinet, C., and Roques, A. (2010). Direct impacts of recent climate warming on insect populations. Integr. Zool. 5, 132–142. doi: 10.1111/j.1749-4877.2010.00196.x
Robinson, A., Inouye, D. W., Ogilvie, J. E., and Mooney, E. H. (2017). Multitrophic interactions mediate the effects of climate change on herbivore abundance. Oecologia 185, 181–190. doi: 10.1007/s00442-017-3934-0
Roth, T., Plattner, M., and Amrhein, V. (2014). Plants, birds and butterflies: short-term responses of species communities to climate warming vary by taxon and with altitude. PLoS One 9:e82490. doi: 10.1371/journal.pone.0082490
Sánchez-Bayo, F., and Wyckhuys, K. A. G. (2019). Worldwide decline of the entomofauna: a review of its drivers. Biol. Conserv. 232, 8–27. doi: 10.1016/j.biocon.2019.01.020
Scudder, S. H. (1889). The butterflies of the eastern United States and Canada with special reference to New England. Cambridge, MA: Creative Media Partners, LLC. 767–1774.
Sexton, J. P., McIntyre, P. J., Angert, A. L., and Rice, K. J. (2009). Evolution and ecology of species range limits. Annu. Rev. Ecol. Evol. Syst. 40, 415–436. doi: 10.1146/annurev.ecolsys.110308.120317
Speed, J. D. M., Austrheim, G., Hester, A. J., and Mysterud, A. (2010). Experimental evidence for herbivore limitation of the treeline. Ecology 91, 3414–3420. doi: 10.1890/09-2300.1
Stanton-Geddes, J., Tiffin, P., and Shaw, R. G. (2012). Role of climate and competitors in limiting fitness across range edges of an annual plant. Ecology 93, 1604–1613. doi: 10.1890/11-1701.1
Stueve, K. M., Isaacs, R. E., Tyrrell, L. E., and Densmore, R. V. (2011). Spatial variability of biotic and abiotic tree establishment constraints across a treeline ecotone in the Alaska Range. Ecology 92, 496–506. doi: 10.1890/09-1725.1
Sullivan, B. L., Wood, C. L., Iliff, M. J., Bonney, R. E., Fink, D., and Kelling, S. (2009). eBird: a citizen-based bird observation network in the biological sciences. Biol. Conserv. 142, 2282–2292. doi: 10.1016/j.biocon.2009.05.006
Svancara, L. K., Abatzoglou, J. T., and Waterbury, B. (2019). Modeling current and future potential distributions of milkweeds and the monarch butterfly in Idaho. Front. Ecol. Evol. 7:168. doi: 10.3389/fevo.2019.00168
Thompson, J. (1988). Variation in preference and specificity in monophagous and oligophagous swallowtail butterflies. Evolution 42, 118–218. doi: 10.1111/j.1558-5646.1988.tb04112.x
Urban, M. C., Bocedi, G., Hendry, A. P., Mihoub, J.-B., Pe’er, G., Singer, A., et al. (2016). Improving the forecast for biodiversity under climate change. Science 353:aad8466. doi: 10.1126/science.aad8466
Urban, M. C., Phillips, B. L., Skelly, D. K., and Shine, R. (2007). The cane toad’s (Chaunus [Bufo] marinus) increasing ability to invade Australia is revealed by a dynamically updated range model. Proc. R. Soc. B Biol. Sci. 274, 1413–1419. doi: 10.1098/rspb.2007.0114
Valavi, R., Elith, J., Lahoz-Monfort, J. J., and Guillera-Arroita, G. (2019). block CV: an r package for generating spatially or environmentally separated folds for k-fold cross-validation of species distribution models. Methods Ecol. Evol. 10, 225–232. doi: 10.1111/2041-210x.13107
Warren, M. S., Hill, J. K., Thomas, J. A., Asher, J., Fox, R., Huntley, B., et al. (2001). Rapid responses of British butterflies to opposing forces of climate and habitat change. Nature 414, 65–69. doi: 10.1038/35102054
Wilson, J. K., Casajus, N., Hutchinson, R. A., McFarland, K. P., Kerr, J. T., Berteaux, D., et al. (2019). Climate change and local host availability drive the northern range boundary in the rapid northward expansion of the eastern giant swallowtail butterfly. bioRxiv [Preprint]. doi: 10.1101/868125
Wisz, M. S., Hijmans, R. J., Li, J., Peterson, A. T., Graham, C. H., Guisan, A., et al. (2008). Effects of sample size on the performance of species distribution models. Divers. Distrib. 14, 763–773. doi: 10.1111/j.1472-4642.2008.00482.x
Wisz, M. S., Pottier, J., Kissling, W. D., Pellissier, L., Lenoir, J., Damgaard, C. F., et al. (2013). The role of biotic interactions in shaping distributions and realised assemblages of species: implications for species distribution modelling. Biol. Rev. Camb. Philos. Soc. 88, 15–30. doi: 10.1111/j.1469-185X.2012.00235.x
Ylioja, T., Roininen, H., Ayres, M. P., Rousi, M., and Price, P. W. (1999). Host-driven population dynamics in an herbivorous insect. Proc. Natl. Acad. Sci. U.S.A. 96, 10735–10740. doi: 10.1073/pnas.96.19.10735
Yu, J., Wong, W.-K., and Hutchinson, R. A. (2010). “Modeling experts and novices in citizen science data for species distribution modeling,” in Proceedings of the 2010 IEEE International Conference on Data Mining, Sydney.
Keywords: biotic interactions, benchmarking biodiversity, citizen science, species distribution models, climate change
Citation: Wilson JK, Casajus N, Hutchinson RA, McFarland KP, Kerr JT, Berteaux D, Larrivée M and Prudic KL (2021) Climate Change and Local Host Availability Drive the Northern Range Boundary in the Rapid Expansion of a Specialist Insect Herbivore, Papilio cresphontes. Front. Ecol. Evol. 9:579230. doi: 10.3389/fevo.2021.579230
Received: 02 July 2020; Accepted: 03 February 2021;
Published: 02 March 2021.
Edited by:
Umesh Srinivasan, Centre for Ecological Sciences, Division of Biological Sciences, Indian Institute of Science (IISc), IndiaReviewed by:
Jamie M. Kass, Okinawa Institute of Science and Technology Graduate University, JapanJochen Krauss, Julius Maximilian University of Würzburg, Germany
Copyright © 2021 Wilson, Casajus, Hutchinson, McFarland, Kerr, Berteaux, Larrivée and Prudic. This is an open-access article distributed under the terms of the Creative Commons Attribution License (CC BY). The use, distribution or reproduction in other forums is permitted, provided the original author(s) and the copyright owner(s) are credited and that the original publication in this journal is cited, in accordance with accepted academic practice. No use, distribution or reproduction is permitted which does not comply with these terms.
*Correspondence: J. Keaton Wilson, a2VhdG9ud2lsc29uQGFyaXpvbmEuZWR1; Kathleen L. Prudic, a2xwcnVkaWNAYXJpem9uYS5lZHU=