- 1Skogforsk, Uppsala, Sweden
- 2Forest Research, Roslin, United Kingdom
- 3BIOGECO, INRAE, Univ. Bordeaux, Bordeaux, France
- 4Skogforsk, Svalöv, Sweden
- 5CNR - Institute of Biosciences and BioResources, Florence, Italy
Forests provide important ecosystem services and renewable materials. Yet, under a future climate, optimal conditions will likely shift outside the current range for some tree species. This will challenge the persistence of populations to rely on inherent plasticity and genetic diversity to acclimate or adapt to future uncertain conditions. An opportunity to study such processes is offered by Scots pine (Pinus sylvestris L.), a forest tree with a large distribution range including populations locally adapted to a wide variety of environments, which hinders a range-wide assessment of the species to climate change. Here we evaluate tree height growth uncertainty of Scots pine marginal populations in Spain and the Nordic countries linked to their genetic adaptation promoted by different climatic drivers. Our aims are to: (i) review the main climatic drivers of Scots pine adaptation across its range; (ii) undertake provenance-based modeling and prediction of tree height under current and future climate scenarios including four representative concentration pathways (RCPs) and five general circulation models (GCMs) at two extremes of its climatic niche; (iii) estimate uncertainty in population tree height linked to the main drivers of local adaptation that may change among RCPs and GCMs in the Nordic countries and Spain. Our models revealed that tree height adaptation is mostly driven by drought in Spain and by photoperiod in the Nordic countries, whereas the literature review also highlighted temperature as a climatic driver for the Nordic region. Model predictions for the Nordic countries showed an overall increase in tree height but with high uncertainty in magnitude depending on the RCPs and GCMs whereas predictions for Spain showed tree height to be maintained in the north and reduced in the south, but with similar magnitudes among RCPs and GCMs. Both models predicted tree height outside the data range used to develop the models (extrapolation). Predictions using higher emission RCPs resulted in larger extrapolated areas, constituting a further source of uncertainty. An expanded network of Scots pine field trials throughout Europe, facilitated by data collection and international research collaboration, would limit the need for uncertain predictions based on extrapolation.
Introduction
Climate change is reshaping species distributions at an unprecedented pace. Evidence of range contraction has been observed in European forests, for example, caused by the increase in drought-related tree mortality rates in climatically marginal populations (Archambeau et al., 2020; Changenet et al., 2021), as well as the maladaptation of climatically marginal populations to current climate conditions observed in North American and European forests (Pedlar and McKenney, 2017; Fréjaville et al., 2020). To survive under new climates, climatically marginal populations can persist in situ through genetic adaptation or phenotypic plasticity (Valladares et al., 2014; Aitken and Bemmels, 2016). However, these two evolutionary processes have different implications for long-lived species such as trees. Plasticity imparts a rapid response to new environments without changes in the genetic structure (Nicotra et al., 2010) and can help some marginal populations to survive under climate change (Gárate Escamilla et al., 2019), whereas genetic adaptation occurs over generations, implying that trees may not be able to adapt to rapid environmental changes but rather carry the burden of maladaptation (Pedlar and McKenney, 2017; Fréjaville et al., 2020). Generally, conifers present higher adaptation rates for tree growth than broadleaf species (Benito Garzón et al., 2019), and that broadleaves tend to express high plasticity (Sáenz-Romero et al., 2017; Gárate Escamilla et al., 2019). Whether this characteristic would confer an advantage to broadleaf species over conifers to survive under fast climate change is still unclear because phenotypic plasticity may delay adaptation processes in the long term (Chevin et al., 2010). Furthermore, the drivers triggering adaptation may change across species ranges as a consequence of different climatic pressures, at least in trees covering large distribution areas. This can present different odds for a population to survive under future climates (Atkins and Travis, 2010). Therefore, understanding the climatic drivers of adaptation in the leading and trailing edges of species ranges is key to assessing the vulnerability of marginal and peripheral forest populations to climate change.
Genetic field trials (also called provenance tests) are controlled experiments where seed from natural populations is planted across large geographical gradients. Although field trials were originally planted for breeding purposes, they have proved to be a useful resource to understand the likelihood of survival under climate change (Mátyás, 1994) providing accurate indicators of plasticity and local adaptation of fitness-related traits among provenances. As such, field trials have been extensively used to improve breeding programs (Gray et al., 2016), quantify phenotypic plasticity (Matesanz and Ramírez-Valiente, 2019; Vizcaíno-Palomar et al., 2020), perform species range predictions accounting for local adaptation and phenotypic plasticity (Benito Garzón et al., 2019), and design assisted migration programs (Isaac-Renton et al., 2014).
One of the species most widely planted in genetic field trials is Scots pine (Pinus sylvestris L.), an economically and ecologically important species with the largest distribution among tree species in Europe. Its distribution range covers a wide climatic niche (Benito Garzón and Vizcaíno-Palomar, 2021) from southernmost Spain to Scandinavia (Caudullo et al., 2017). In spite of the large network of field trials, modeling approaches covering the entire distribution range of the species are scarce (Reich and Oleksyn, 2008), probably owing to the different drivers triggering adaptation of the population across its range (Torssonen et al., 2015; Berlin et al., 2016; Vizcaíno-Palomar et al., 2019; Rubio-Cuadrado et al., 2020) but also because of a lack of access to relevant range-wide comprehensive data. As such, many regional modeling approaches targeting Scots pine adaptation have been developed over the years. Studies of marginal populations suggest that Scots pine has adapted to different environments over time. For instance, populations at the northernmost part of the species range are mostly adapted to photoperiod and temperature (Rehfeldt et al., 2002; Berlin et al., 2016), whereas those at the southernmost part of Scots pine range show adaptation to drought (Matías and Jump, 2014; Vizcaíno-Palomar et al., 2019). Yet, to what extent these drivers triggering population adaptation across the range can confer an advantage of survival and growth, to this long lived tree species under climate change, is still not clear because of the uncertainty of the future climate.
The IPCC 5th Assessment Report (IPCC, 2014) synthesized knowledge regarding current climate change science and classified four scenarios of greenhouse gas (GHG) emissions leading to increased radiative forcing, causing a warming of the atmosphere by 2100. These Representative Concentration Pathways (RCPs) include: RCP 2.6, RCP 4.5, RCP 6.0 and RCP 8.5. Many General Atmospheric-Ocean Circulation Models (GCMs) have been parameterised to link scenarios of population growth and socio-economic futures to the range of RCPs; these are termed Shared Socio-economic Pathways (SSPs). The link between SSPs and RCPs allows future global socio-economic scenarios to be assessed in terms of the spatial variation in changing temperatures. There is uncertainty about which SSP trajectory the global system will follow. The RCP 2.6 represents an increase in global temperatures below 2°C, whereas the intermediate RCP 4.5 is projected to cause temperatures to rise until 2040-50 after which reductions in GHG emissions forcing will occur. For RCP 6.0 emissions will peak in 2080 and then decline. For RCP 8.5 GHG emissions continue to rise throughout the 21st century leading to global warming of up to 4.8°C by 2080. However, some recent research suggests that the RCP 8.5 scenario is unlikely due to the recent technological development of renewable energy sources (see e.g., Hausfather and Peters, 2020). Additional uncertainty occurs among the GCMs as each differs slightly in model parameters. This is shown in the range of temperature increase in each of the RCP categories (IPCC, 2014).
Scots pine has a large natural distribution range from Finland and Sweden (hereafter Nordic countries) to Spain and it is locally adapted to a wide variety of environments. Uncertainty inherent in future climate projections hinders the assessment of the species and the vulnerability of the populations to global climate change. Our main goal is to assess this uncertainty in tree height growth linked to the genetic adaptation of Scots pine populations driven by different climatic drivers across its distribution range. Here we (i) review the main climatic drivers of adaptation of Scots pine range-wide; (ii) re-analyze tree height growth at the two extremes of the thermal gradient of its climatic niche: Spain and Nordic countries, where populations show adaptation to drought and cold conditions, respectively; and (iii) estimate uncertainty in population tree height growth spatial predictions linked to the main drivers of local adaptation that may change among future scenarios (RCP 2.6, RCP 4.5, RCP 6, and RCP 8.5) differently in the Nordic countries and Spain.
Materials and Methods
Tree Height Measurements and Field Trials
We used tree height data of Scots pine (Pinus sylvestris L.) compiled in the Nordic countries and Spain. For the Nordic experiments, tree height data were collected from 378 different field trials (Figure 1) established from 1951 to 1996 and measured at ages ranging from 8 to 35 years-old. Several of the trials were measured repeatedly but only one measurement per tree was used for further analysis. Among these field trials, a total of 276 seed-lots sampled from wild Scots pine provenances (populations) were distributed. This was not a complete reciprocal-transplant experiment because field trials were established according to slightly different objectives and using different materials. Since the Nordic tree height dataset had a heterogeneous planting design, data from single trees were not used for analysis. Instead, least-square means (LS-means) for each provenance/field trial combination were estimated in preparatory site-wise analyses. For these analyses GLM and MIXED procedures in the SAS STAT package were used (SAS Institute Inc., 2011; see Berlin et al., 2016 for details). LS-means were transformed by the natural logarithm prior to further analysis to assure normality and homogeneity of the data.
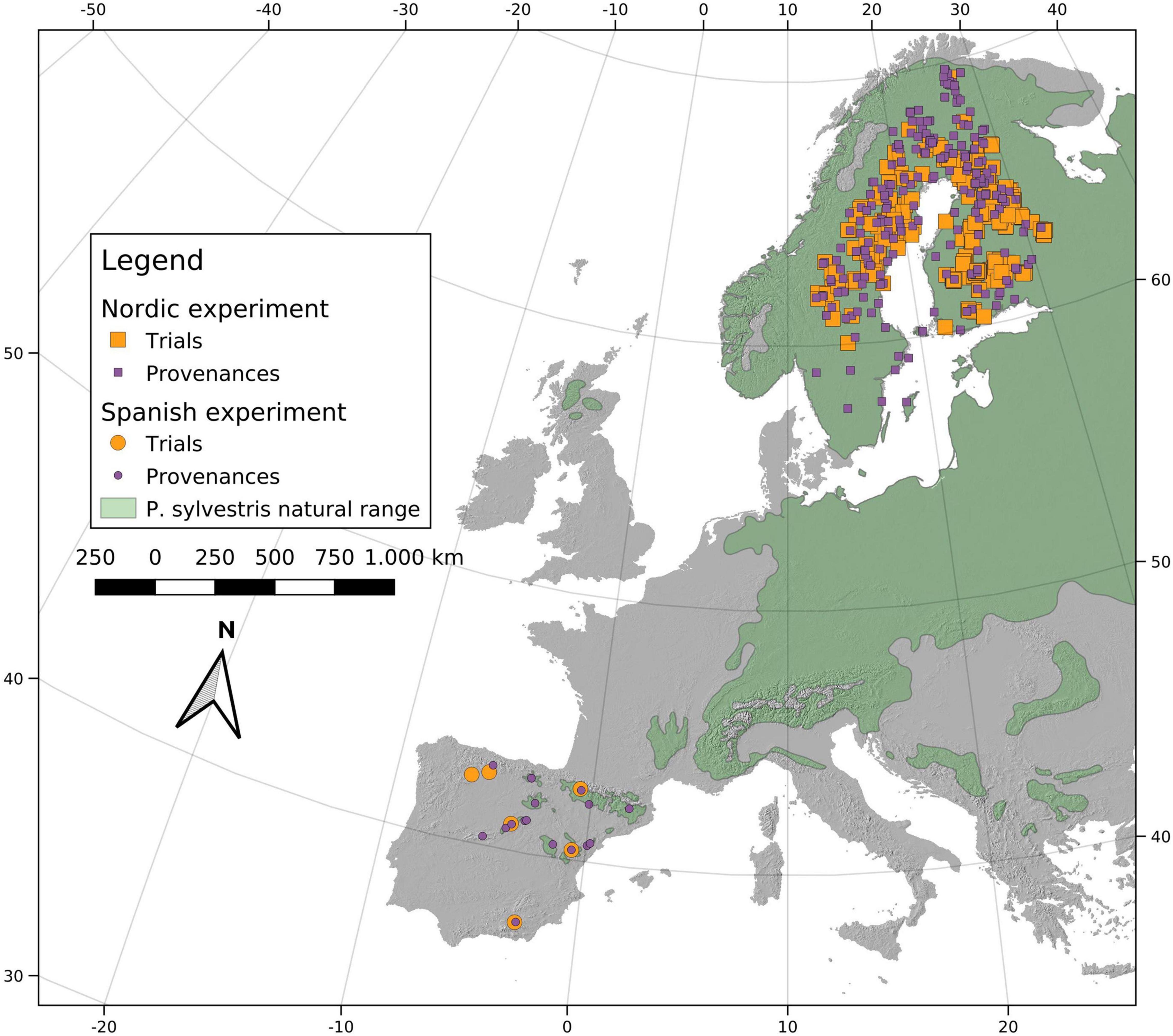
Figure 1. Field trials and provenances planted in Sweden, Finland and Spain. The background area colored in green corresponds to the Scots pine native range (Caudullo et al., 2017).
For the Spanish experiment, at the southern range of the species, tree height of individuals after 14 and 15 years of growth was measured at six field trials (Figure 1). Sixteen genetically distinct Spanish populations (Alía et al., 2001; Prus-Glowacki et al., 2003; Robledo-Arnuncio et al., 2005) covering the species distribution in Spain were planted in each of the six field trials. During the years 1988 and 1989, seeds were collected to form provenance (population) seed-lots (Agúndez et al., 1992; Alía et al., 2001). A seed-lot was created by mixing the clumped seeds collected in natural populations from at least 25 mother trees, with a 50 m separation distance between each individual to avoid interbreeding (González-Martínez et al., 2006). Between 1990 and 1991, 2-year-old plantlets originating from the seed-lots were planted in each field trial, following a randomized complete block design, with four blocks and a 16-tree square plot for each population, planted at a 2.5 m × 2.5 m spacing.
Climatic and Environmental Data
We combined several databases to characterize the climate at the origin of provenances and the climate at the field trials. This was achieved by downscaling the CRU-TS dataset (Harris et al., 2020) from ∼50 to ∼1 km (30-arc s) using WorldClim v1.4 (Hijmans et al., 2005) with the 1961–1990 climatic normal period as baseline. Our downscaling technique used the delta method (Ramirez-Villegas and Jarvis, 2010), a technique that accounts for topographic variation and improving the reliability and spatial resolution of coarser spatial datasets (Moreno and Hasenauer, 2016; Fréjaville and Benito Garzón, 2018). To characterize the climate of the future (period of years: 2056–2085 and called 2070s), we used five GCMs for each RCP generated from CMIP5 (see Supplementary Material 1 for further details).
For the Nordic experiment, the annual accumulated growing day-degree temperature sum above a 5°C threshold (GDD5) was used as a climate variable for field trials (Table 1) as had been determined by previous modeling efforts (Persson and Ståhl, 1993; Persson, 1994; Berlin et al., 2016). For this variable, we used an average across all annual GDD5 values from the field trial establishment to the time of phenotypic measurements. In the Nordic experiment, however, the time periods varied substantially per se. The year of establishment ranged from 1951 to 1996 and year of height measurement varied from 1963 to 2011. With respect to conditions at the origin location of Nordic provenances, latitude was used as a proxy for photoperiod in the model, and was constant over time. For the Spanish experiment, we used the summer heat moisture index (SHM), spring precipitation (SPR) and temperature differential (TD–as a measure of continentality); these variables were selected previously in Vizcaíno-Palomar et al. (2019) (Table 1). Climate variables for the provenances consisted of averages calculated from 1901 to 1950, whereas the climate variables for field trials were obtained by calculating averages from the time the trial was planted to the time phenotypic measurements were taken (as in the Nordic experiment). As the Spanish field trials were established and measured in concert, this implies a time-period from 1990 or 1991 to 2005.
Linear Mixed-Effect Models
We calibrated two independent models to assess how tree height (H) for different Scots pine populations respond to environmental variation. The Nordic model fitted field trial/provenance- wise means of tree height obtained from Nordic field trials and the Spanish model fitted individual tree height data measured in Spanish field trials. In both models, we used the environmental transfer distance, Δenv, calculated as the difference between the environment at the provenance origin and the environment of the field trial. This Δenv term allows us to assess variation in tree height due to shifts across environmental gradient. This variation occurs when Δenv < 0, meaning that the provenance is transferred to sites with a greater environmental value than its origin, or transferred to sites with smaller environmental values than its origin when Δenv>0. Δenv = 0 means that the provenance is growing under local environmental conditions. Environmental variables relevant for the study of climate change and prediction uncertainty are listed in Table 1. For both Nordic and Spanish models, fixed effects were tested using the maximum likelihood (ML), and random effects were tested using the restricted maximum-likelihood method (REML).
Nordic Model
A linear mixed-effects model was used to predict tree height as a function of the transfer distance in latitude (Δenv = ΔLAT) being a proxy primarily for photoperiod. In addition, the GDD5 was used to describe the climate of the field trial and was used in interaction terms with the latitudinal transfer distance. These variables were included as fixed effects and the field trial site as a random effect, to account for unexplained environmental variation. This model was established by a hypothesis-driven process (see Supplementary Material 2 for more details), building on the work of previous regional modeling (Persson and Ståhl, 1993; Persson, 1994; Eriksson, 2008; Berlin et al., 2016). Logarithm-transformed LS-means of tree height estimated for each provenance-field trial combination [ln(Hjk)] were fitted as follows:
where AGEk is the tree age for the kth field trial at measurement, ESTk is the establishment year of the kth field trial and GDD5_sk is the kth field trial averaged from the year of planting to the year of height measurement. In addition, ΔLATjk is the latitudinal transfer distance between the kth field trial and the jth provenance present at the kth field trial. Finally, β is the random field trial effect and εijk is the residual distribution of the jth provenance at the kth field trial following a Gaussian distribution.
Spanish Model
Also here we used a linear mixed-effects model to predict tree height as a function of the environmental transfer distance of the Summer Heat Moisture index (Δenv = ΔSHM), the SPRing precipitation climate at the field trial site (SPR_s) and the Temperature Differential (TD_s) following previous models of the species in Spain (Benito Garzón et al., 2011; Valladares et al., 2014; Vizcaíno-Palomar et al., 2019). In this model, climatic variables were included as fixed effects and specifically we modeled the linear, quadratic and linear interaction terms of ΔSHM and SPR_s, and the linear term for the covariate TD_s. In the random part of the model we included provenance for unaccounted environmental variation among populations (β). The final model was built based upon a hierarchical backward selection procedure from the most complex model and the procedure is further detailed in Supplementary Material 2. The final Spanish model for individual tree height, Hijk, was fitted as follows:
where Hijk is tree height of the ith individual of the jth provenance in the kth field trial and αs is the set of n parameters associated with the fixed effects of the model. Finally εijk is the residual distribution of the ith individual of the jth provenance at the kth field trial following a Gaussian distribution.
Drivers of Adaptation
We performed a literature compilation of the different drivers triggering Scots pine adaptation across its distribution range. The review was done by searches on relevant phrases such as adaptation, Scots pine, Pinus sylvestris and climate change in Google scholar and by inspecting their reference lists in turn.
Uncertainty Analysis of Tree Height Predictions
Our uncertainty analysis used spatial predictions of tree height in Nordic countries and Spain performed on a synthetic mean provenance for each region, reflecting an average among the provenances used to develop the models. The predictions were performed only within the species distribution range taken from Caudullo et al. (2017). For the Nordic region the mean provenance had a latitudinal origin at 64.87°N, whereas for Spain, the mean provenance had an SHM value of 90.55°C/m. In addition, for model predictions across the Nordic region, the tree age (AGE) was set at 15 years in order to correspond with the Spanish model. For the Nordic model predictions, the year of establishment (EST) was set at 1980 as height predictions, plotted across the range of EST-values, suggested that no appreciable silvicultural improvements had been achieved since that time. We predicted tree height for the Nordic region and Spain using the respective models. Predictions were performed for all four RCPs scenarios combined with the five GCM variants resulting in a total of 20 scenario-variant predictions. In addition, we also calculated mean predictions for each RCP across all five GCMs by using the respective climate ensemble mean of that RCP. All analyses were undertaken in R (R Core Team, 2020) with the raster (Hijmans, 2020) and tidyverse (Wickham et al., 2019) packages.
Assessing Spatial Agreement Between General Circulation Models
For each scenario-variant combination described above, we calculated the difference in tree height between a prediction made for the future climate period (2070s) and the reference height corresponding to the climate normal period (1961–1990). Such prediction calculations (future height–reference height) were performed for each 1 km × 1 km location and a record was made where predicted future height was greater than the reference height. We grouped these records by RCP, and for every 1 km × 1 km location summed the number of GCMs which “agreed” that future height was greater than the reference height. We then subtracted from the sum, the number of GCMs which “disagreed” that future height was greater than the reference height. This assessment resulted in a score which ranged from +5 (maximum agreement among GCMs that future height will exceed reference height) to −5 (maximum agreement among GCMs that future height will be lower than the reference height). Areas where three or two GCMs either agreed or disagreed (scores +1 and −1) thus highlight areas of greater prediction uncertainty.
Model Predictability Limits
We defined model predictability based on the ecophysiological limits of the species recorded from our trials to account for areas where predictions were generated by extrapolation outside the environmental ranges of our data used for model development. For Nordic predictions, these limits were recorded for locations where environmental data points exceeded: (a) GDD5 below 527 day°C or above 1,349 day°C which, according to our climate data, was the smallest and greatest GDD5 experienced in the Nordic field trials included in the model, and; (b) a latitude greater than 5 degrees above or below the latitude of the mean provenance because provenance transfers of that magnitude were the greatest undertaken within the data used to calibrate the models. For the Nordic mean provenance these latitudinal limits were thus set at < 59.87°N and > 69.87°N. Following the same principles we established the ecophysiological limits of the species from recorded locations for Spanish predictions where climatic conditions exceeded: (a) TD below 13.65°C or above 17.25°C; (b) SPR below 177.75 mm or above 269.97 mm and; (c) where the location SHM differed below −213°C/m or above +115°C/m that of the mean provenance SHM.
Consequently, in order to visualize the model predictability limits, we carried out the same type of assessment based on GCM-agreement on increased tree height in a future climate, and where agreement was determined by whether model predictability limits were exceeded or not. We chose to only show where limits were exceeded by three or more GCMs, as we considered this to be “more likely than not.”
Using Coefficient of Variation as an Uncertainty Metric
We used the Coefficient of Variation (CoV) as an indicator of variability between future predictions of tree height. This statistic presents a ratio of the standard deviation to the mean, with higher values indicating greater variation between predictions and therefore higher uncertainty in terms of the most likely outcome. CoV values were estimated for two different aspects of variation/uncertainty.
The first important aspect of prediction uncertainty is connected to the spatial variation of tree height predictions over the geographic study area for a specific climate scenario. In order to cover spatial prediction variation and uncertainty we calculated CoV for the ensemble mean predictions for each RCP across the studied regions (Nordic countries and Spain) and related the standard deviations to the average tree height prediction for the reference period. The nature of such geographic predictions means they have a very large number of data points, especially at higher resolutions, and this can affect statistical analysis. To reduce this spatial autocorrelation effect, we took 100 random samples of 1,000 data points from each set of predictions. We calculated the standard deviation of each RCP-prediction from the reference period mean height. CoV was then calculated as a percentage of the reference period mean height. This calculation was carried out for (a) a complete random sample, and (b) a random sample with data points removed where model predictability limits were exceeded.
The second quantitative measure of tree height prediction uncertainty is the variation in predictions at each location among different GCMs and RCPs. This uncertainty can also be quantified by estimating CoV under a future climate in relation to the predicted tree height during the reference period. We calculated CoV across GCMs within each RCP for each 1 × 1km location. For each location, we calculated an RCP mean prediction using all five GCMs, and the standard deviation of each individual GCM prediction from this overall RCP mean. CoV was calculated as follows, where σ is the RCP standard deviation and μ is the RCP mean:
Results
Drivers of Adaptation as Identified by the Literature and Models
A literature search for important climatic drivers of Scots pine growth across Europe indicated that drivers differed across the range; with temperature and photoperiod often found as the main drivers at high latitudes, while drought was found to dominate as a driver in central and southern Europe (Table 2).
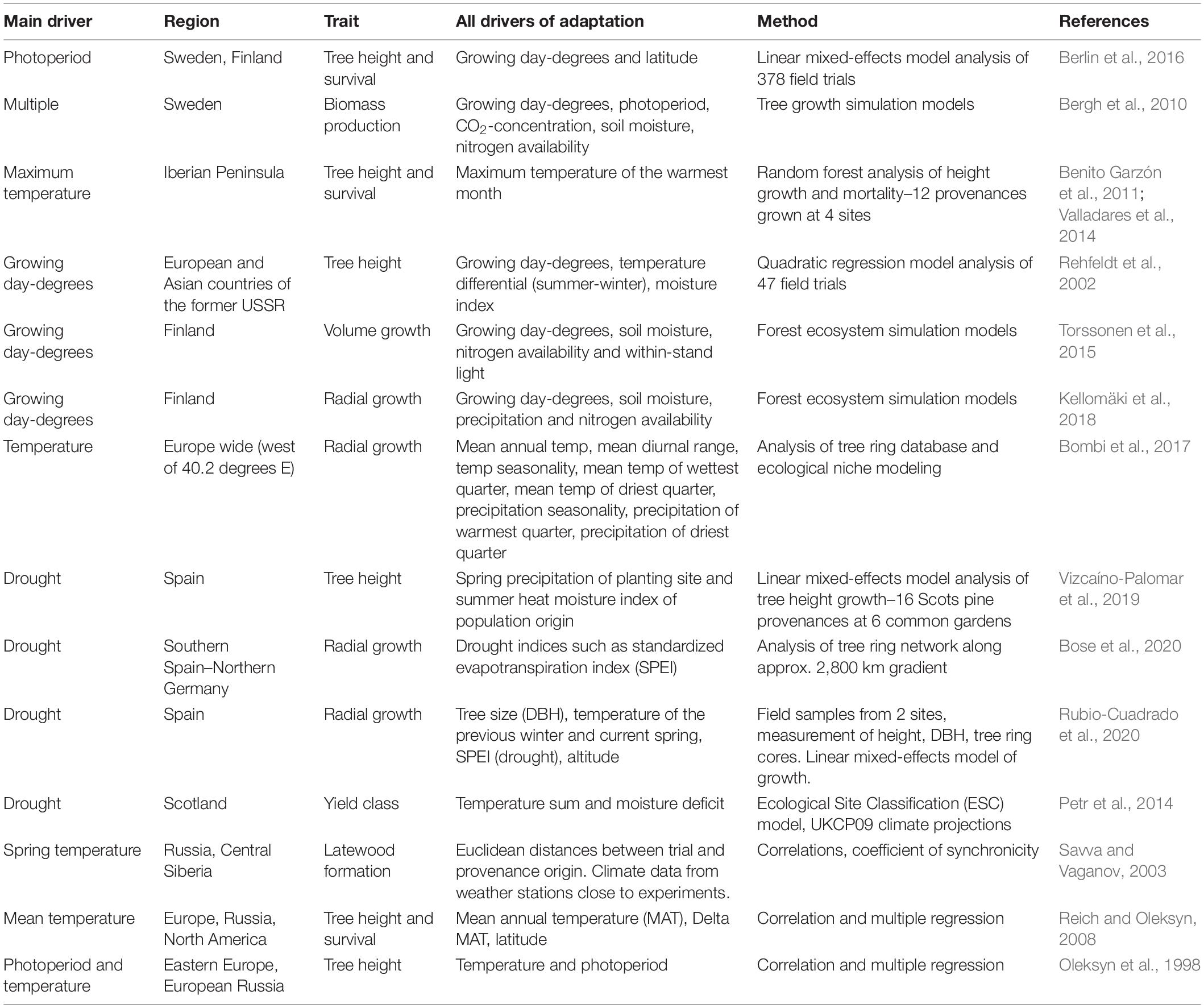
Table 2. Literature search for environmental adaptive drivers (All drivers of Adaptation and Main driver) of Scots pine phenotypic traits (Trait) and the geographic regions (Region) where these were found to be relevant.
Given the down-scaled CRU-TS dataset, GDD5 was confirmed to be a highly significant predictor (p < 0.001) for tree height at a given field trial in the Nordic region (Table 3A). Photoperiod, described as latitudinal transfer, was confirmed as a highly influential driver of adaptation. In the Spanish model, the final best model included SHM as the main driver of adaptation while TD and SPR were retained as significant site predictors of tree height (Table 3B). When accounting only for fixed effects, the height growth model in the Nordic region explained 77% of the total variance while the corresponding model in Spain explained 39% of the total variance.
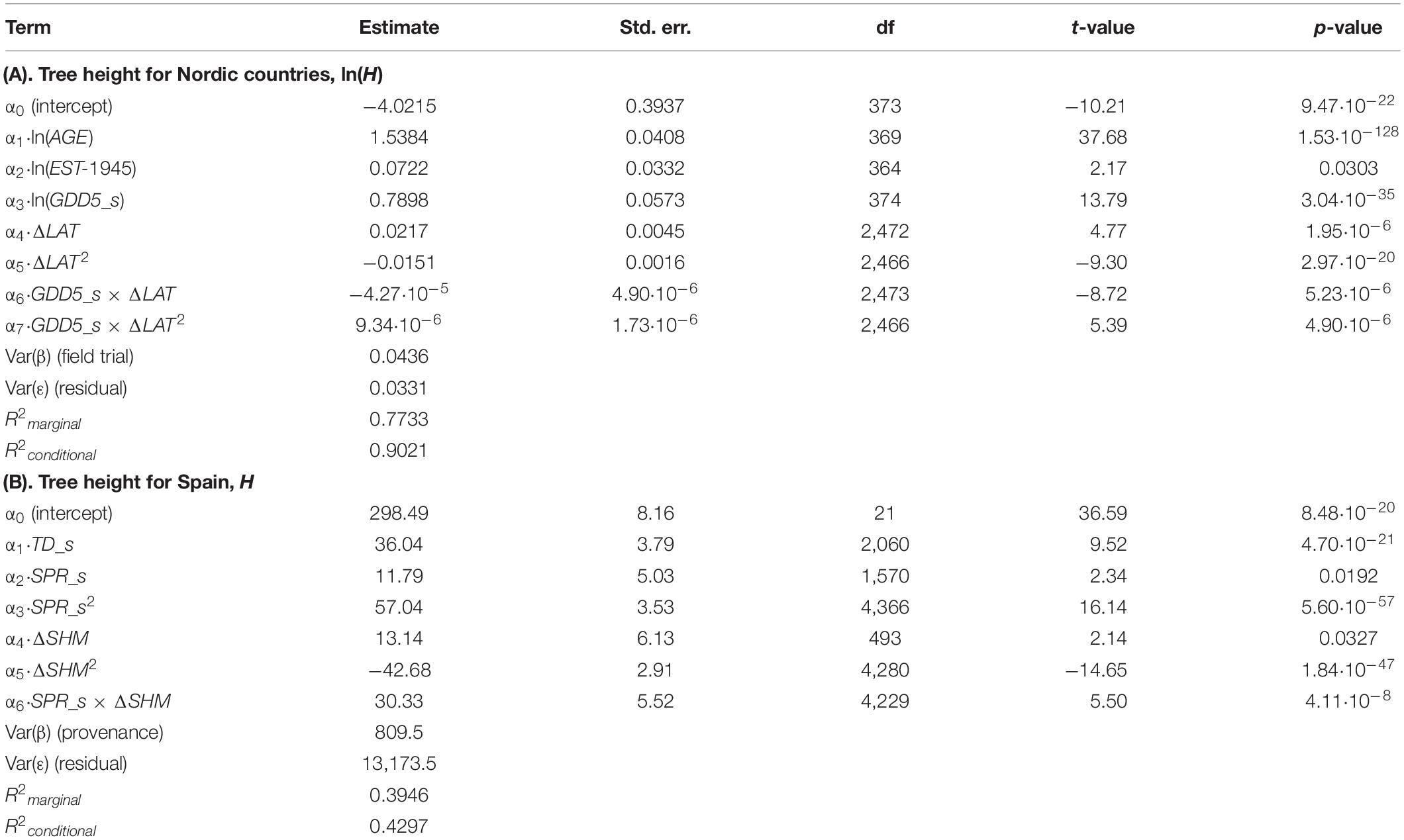
Table 3. Regression coefficients (estimate and standard errors), significance tests, variance component estimates [Var(x)] plus marginal and conditional coefficients of determination (R2) for both Nordic (A) and Spanish (B) mixed-effect models.
Tree Height Predictions and Transfer Distances
Using our linear mixed models, we predicted tree height over a range of environmental transfer distances, i.e., across a range of values of the Δenv for a sample of field trials in the Nordic experiment and for all six available planting sites in the Spanish experiment (Figure 2). In general, we found that tree height curves were either almost flat (Nordic experiment) or slightly concave (Spanish experiment). This implies that optimal tree height was predicted over a broad range of values of ΔLAT using the Nordic model, or over a narrower range of values of ΔSHM using the Spanish model. From a practical point of view, this means that there are provenances of nearby origins that all would perform reasonably well at a given planting site both in Nordic and Spanish regions.
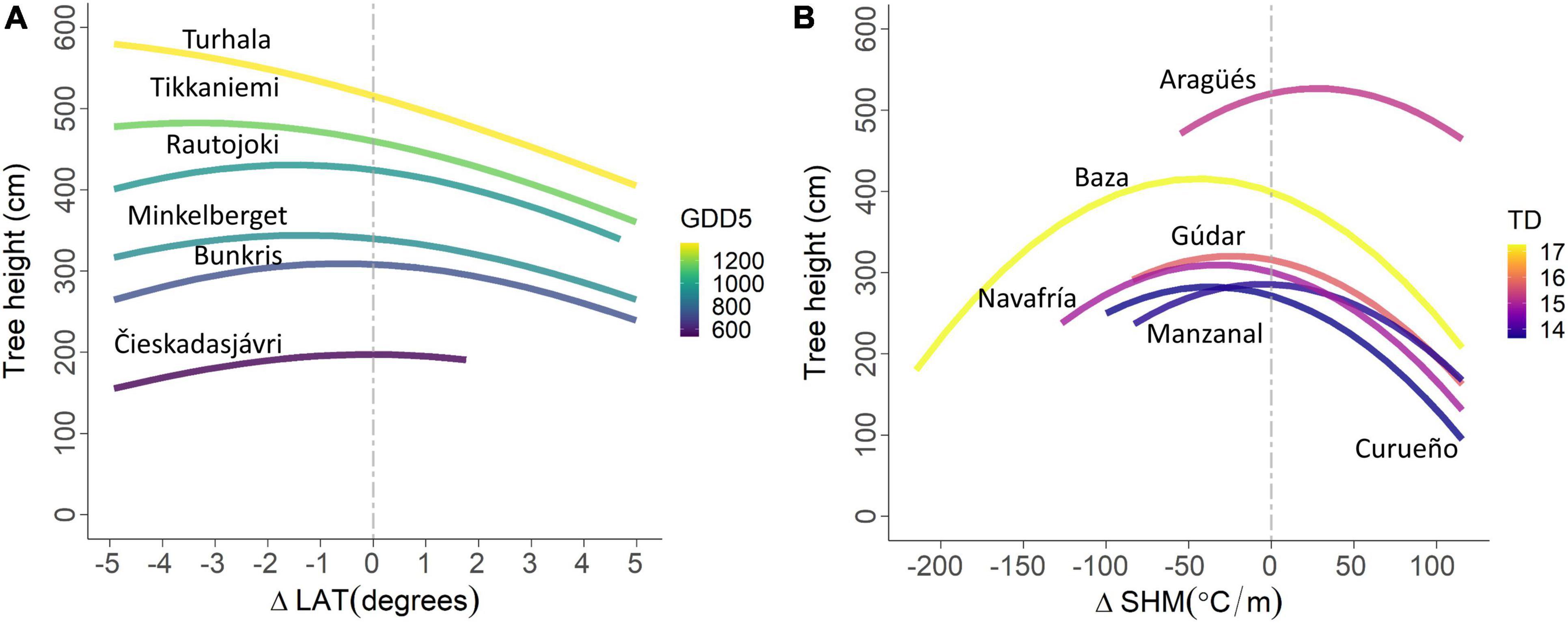
Figure 2. Scots pine tree height predictions as a function of the environmental transfer distances for a sample of six field trials in the Nordic region (A) and for all six field trials in Spain (B) under the average climate during the growth of the trees (see Table 4 for details). The plotting of biologically unreasonable transfer distances such as Nordic provenances originating north of Nordkapp (71.19°N) or Spanish provenances with negative SHM values were omitted.
For the Nordic experiment, we usually observed that transfers of more southern provenances (ΔLAT < 0) would result in higher predicted tree height in comparison to that of a local provenance, that is ΔLAT = 0 (Figure 2A). However, the temperature climate at the field trial location (GDD5, Table 4A) interacted with this trend suggesting that the optimal transfer lengths would be longer for the trials with a mild climate (high GDD5) whereas, at the other extreme, the local provenance would be the best adapted given the coldest climate (low GDD5). For the Spanish experiment, in five out the six planting sites, we observed that transfers of provenances from colder or wetter summers, ΔSHM < 0, than that of the local provenance, ΔSHM = 0, would result in a slight improvement in tree height that is (Figure 2B, except the uppermost curve). For the uppermost curve (Aragüés) however, the finding was the reverse and that transfer of provenances from hotter or drier summers, ΔSHM > 0, than that of the local provenance would perform better (Table 4B).
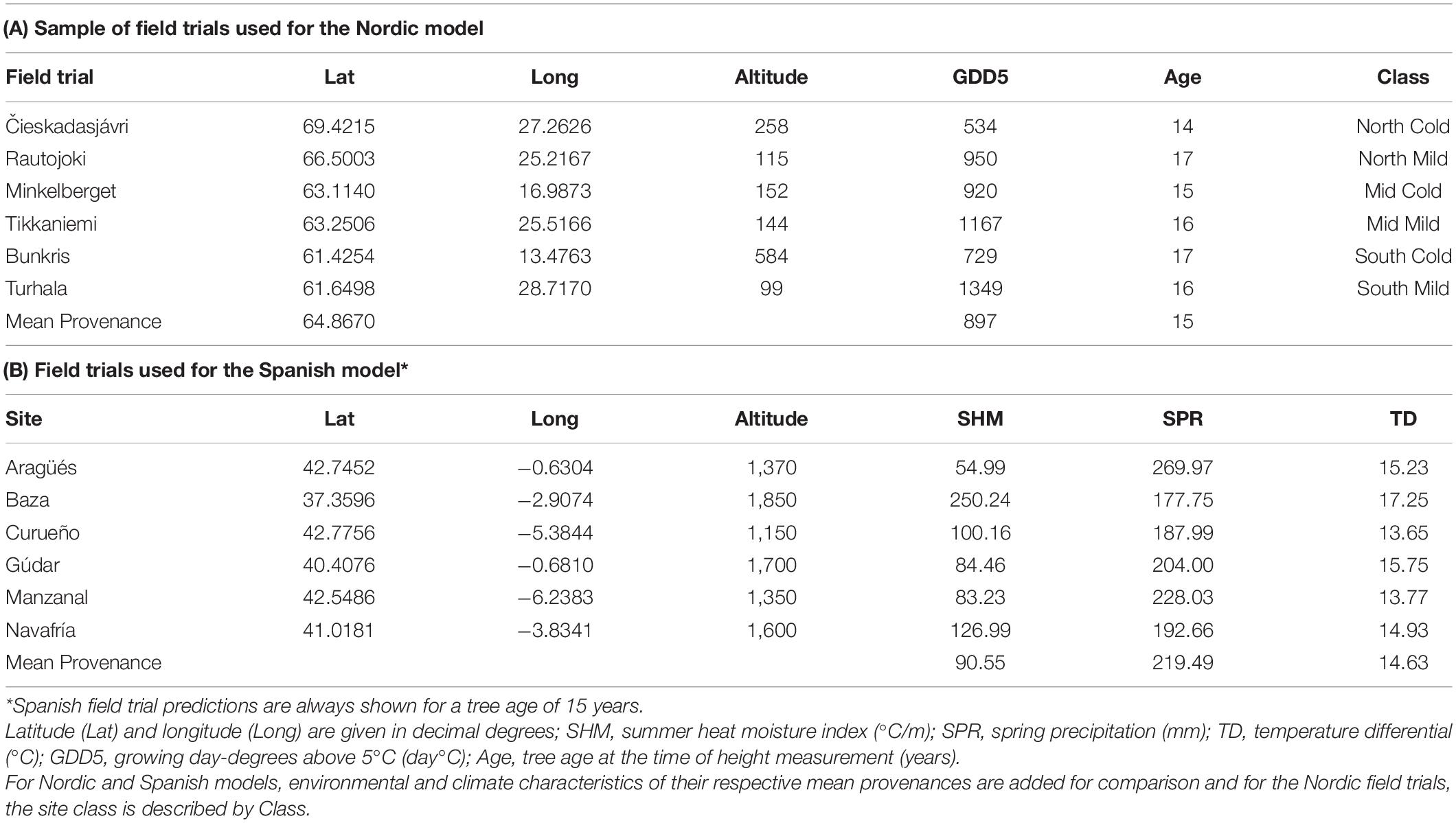
Table 4. Environmental, climatic and age characterization of six field trials representing a sample of the 378 field trials used in the Nordic model (A) and the six field trials used in the Spanish model (B).
Uncertainty in Predicted Increase/Decrease of Tree Height Under Alternative Representative Concentration Pathways
For the Nordic countries, model predictions for tree height at age 15 years showed an increase under various future climate scenarios (Figure 3). Predictions of increased height were consistently certain (predicted by all five GCMs) under all RCPs and at all locations. Predictions made using RCP ensemble means (Supplementary Figure 1) showed that the increase in height growth largely followed the greater increases in GDD5 shown for higher RCPs (up to 81%) in comparison to lower RCPs (39% at the lowest). The magnitudes of predicted increases were often substantial (by 300 cm or more). However, even if these results at first glance present a very clear view on future tree height in the Nordic region, it should be noted that for considerable areas predictions were also shown to be the result of extrapolation (Figure 3). Firstly, it was easy to identify the areas where latitudinal transfers of the mean provenance exceeded the coverage of the underlying model data (LAT < 59.87°N and LAT > 69.87°N). Secondly, increasing GDD5 (by 3–1,117 day°C, Supplementary Figure 2) also caused the areas within model thresholds to shift northwards, toward the interior of the Nordic landmass and toward the higher altitude Scandian mountain ranges (Figure 3). This trend was more pronounced for high emission RCPs (6.0 or 8.5) than for low emission RCPs (2.6 and 4.5).
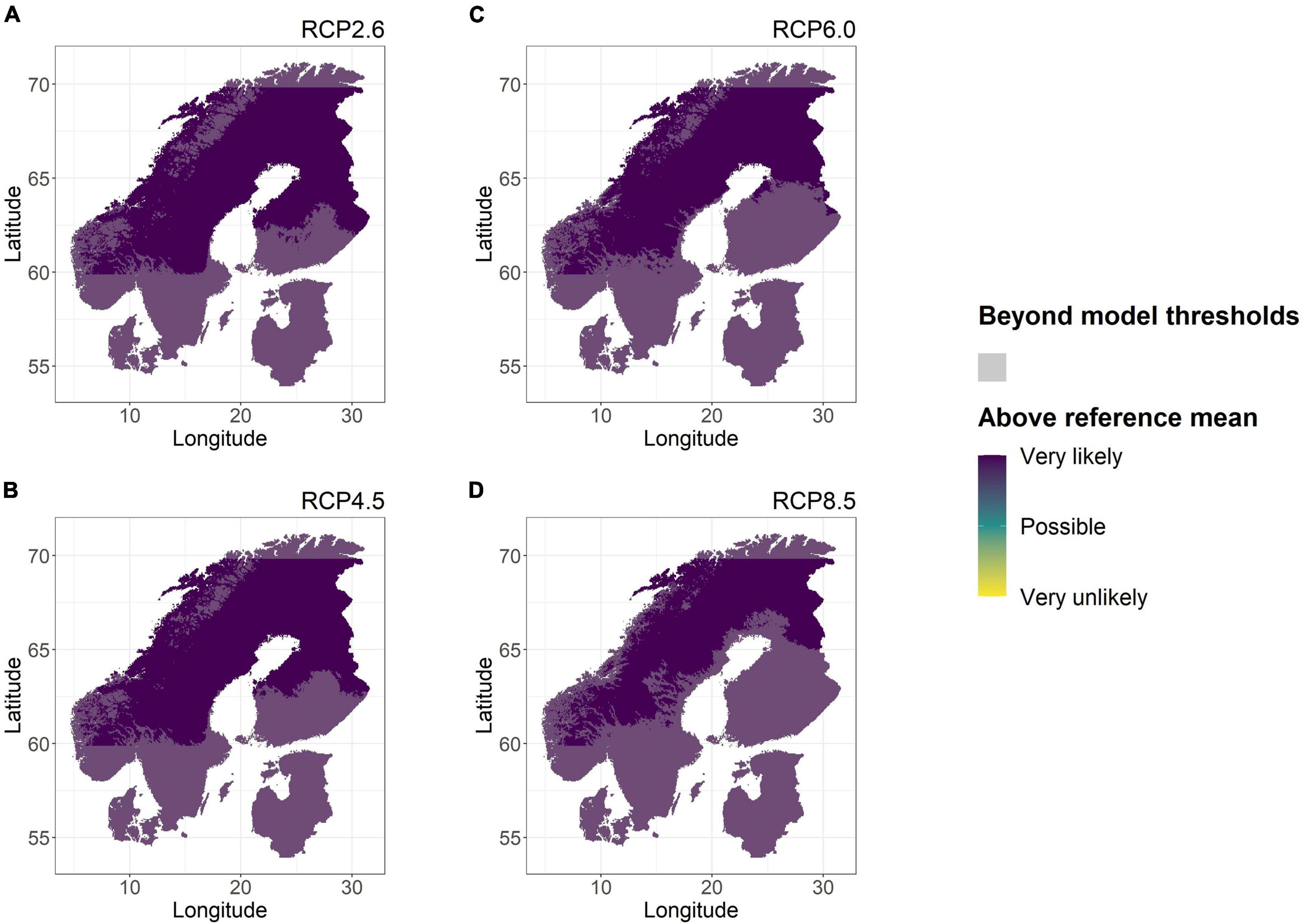
Figure 3. Likelihood of tree height increase for Scots pine in the Nordic region under four future climate scenarios (RCP) in comparison to that of the reference period (1961–1990). The probability was assessed as the agreement among five GCMs within each RCP on tree height increase based on model predictions for RCPs 2.6 (A), 4.5 (B), 6.0 (C), and 8.5 (D). All locations where environmental variables exceeded those of the model data range according to at least three GCMs have been dimmed in semi-transparent gray.
In contrast to the Nordic region, the model results for Scots pine in Spain showed more uncertainty in the likelihood of increased tree height in 2070 (Figure 4). In the southwestern and northeastern part of the Pyrenees, and in the western part of central Spain tree height was predicted to increase under future RCPs in comparison to the reference period. Simultaneously, the magnitudes of tree height difference between climate scenarios and the reference period (Supplementary Figure 3) were small as they usually ranged from a 100 cm height loss to a 100 cm gain in height and there were no clear trends by increasing RCP. RCP ensemble means averaged across Spain ranged only from a 1% decrease to a 0.2% increase. The only exceptions to this lack of trend were the predictions for the southern refugium area in Andalusia which showed a consistent decrease (<−100 cm) in tree height for high emission RCP scenarios. When taking into account model predictability thresholds, southern and central areas plus the central Pyrenees consistently fell outside of the model predictability range thereby increasing uncertainty in results from the model. The area within the model predictability range was predicted to decrease along with RCPs emission scenarios (Figure 4). Cross-examination with predictions of climate variables for Spain in 2070 indicated that increased future temperature differential (TD) was the most likely driving factor behind the shrinking areas of model predictability (Supplementary Figure 4) because the increase in TD was substantial (by 0.5 to 5.3°C) and became stronger with increasing RCP. For the Andalusian area, a considerable increase observed for SHM (by 11.5–309.7°C/m) may exacerbate the reliance on extrapolation (Supplementary Figure 5).
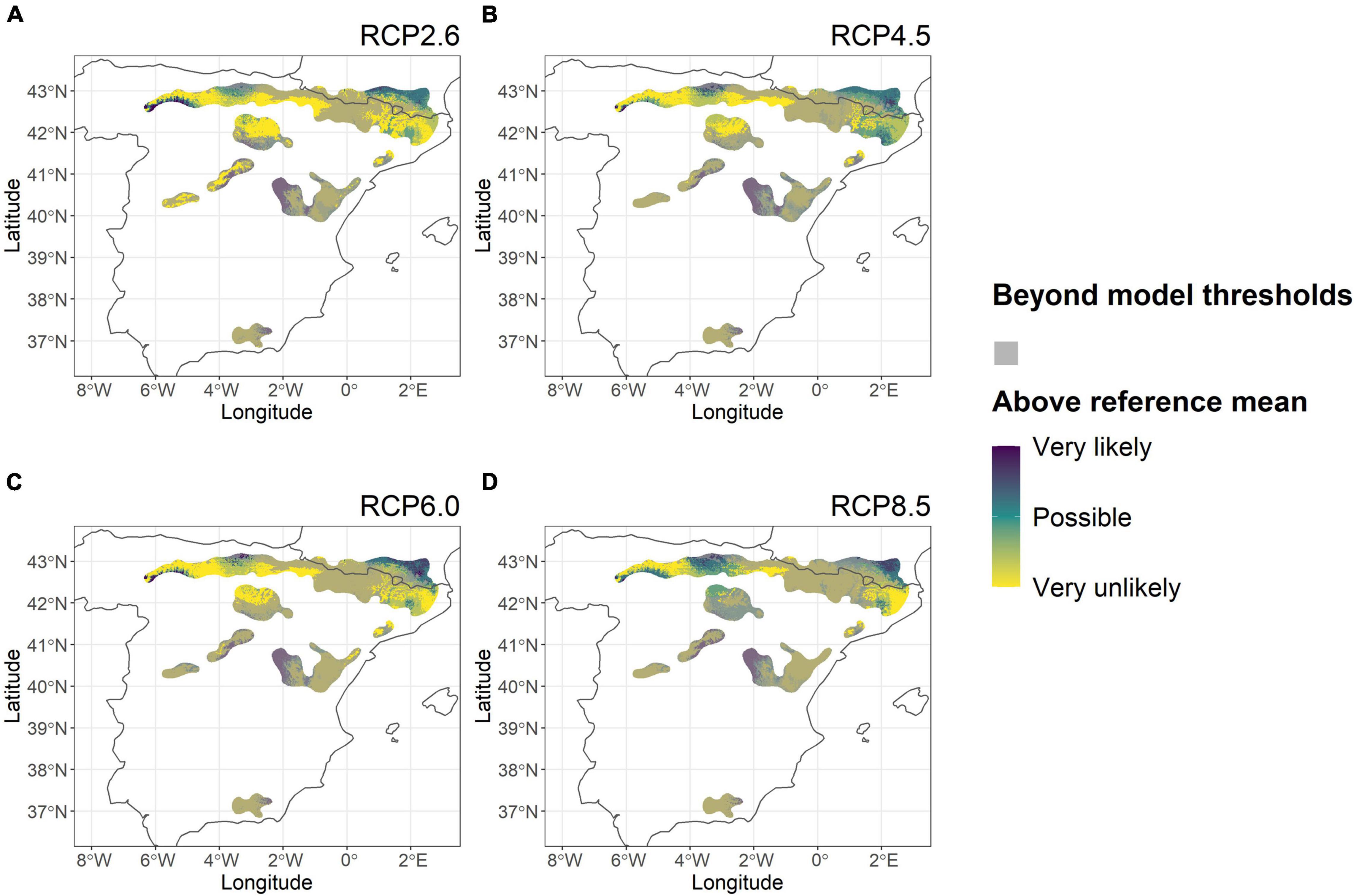
Figure 4. Likelihood of tree height increase for Scots pine within its distribution range in Spain under future climate scenarios in comparison to that of the reference period (1961–1990). The probability was assessed as the agreement among five GCMs within each RCPs on tree height increase based on model predictions for RCPs 2.6 (A), 4.5 (B), 6.0 (C), and 8.5 (D). All locations where environmental variables exceeded those of the model data range according to at least three GCMs have been dimmed in semi-transparent gray.
Spatial Variation in Tree Height Predictions for Each Representative Concentration Pathway
When considering all data, spatial CoV-estimates were in the range 12–18% in the Nordic area (Figure 5), with the range of CoV increasing under higher emission RCPs. In contrast, spatial CoV-estimates for Spain were lower (5–6%) and showed no discernable trend with respect to RCP. It was also notable that spatial CoV-estimates were considerably lower if the study areas were restricted to those within model predictability limits (4–5% for the Nordic region and < 2% for Spain). If only areas within model predictability limits were considered there was no longer any relationship between CoV-magnitude and RCP either in the Nordic or Spanish regions. This suggests that the considerable spatial CoV estimates using all data were associated with model extrapolation, in particular for the higher emission RCPs.
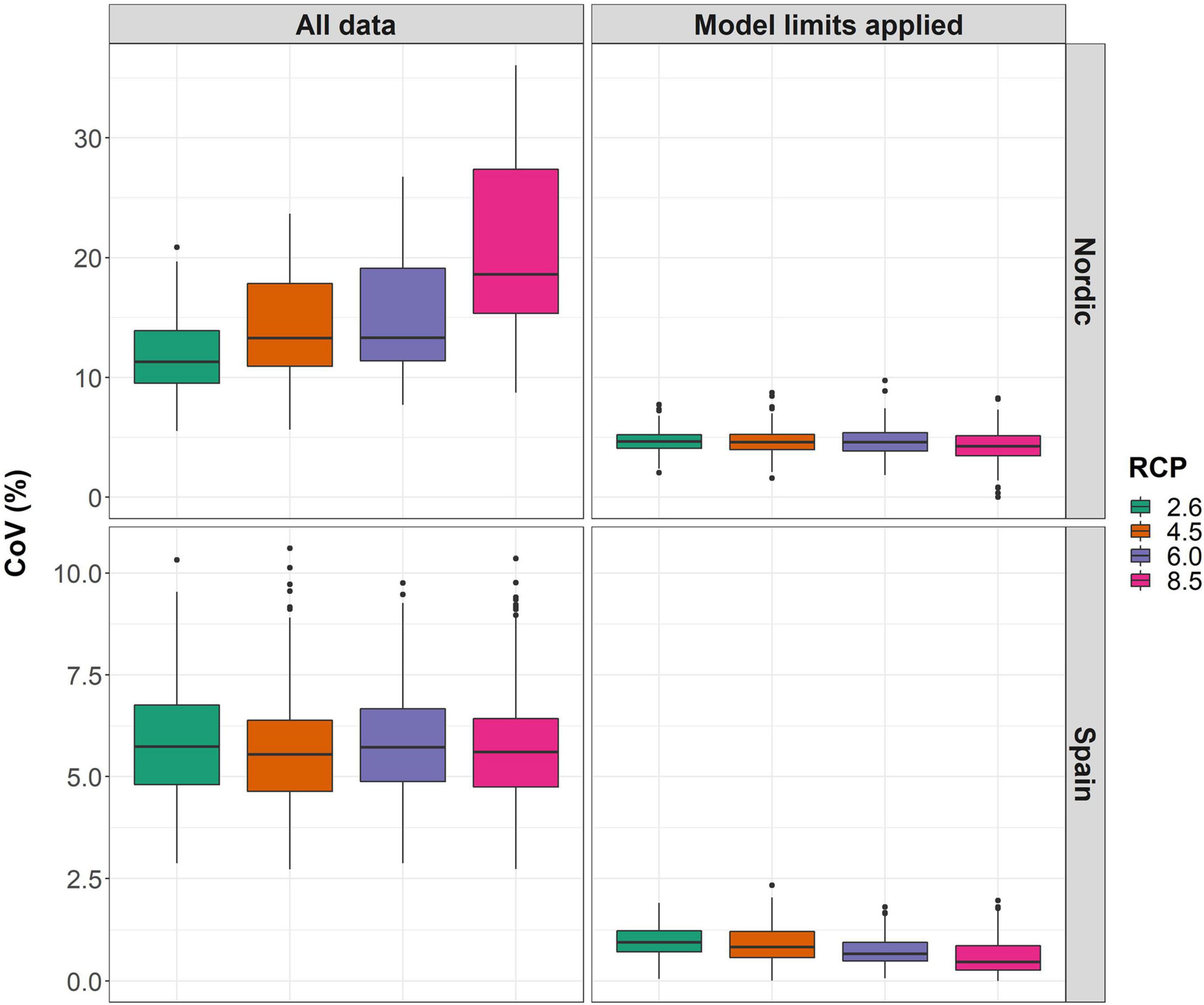
Figure 5. Coefficients of Variation (CoV) calculated for Scots pine tree height predictions across the Nordic and Spain study areas respectively where the standard deviation of ensemble mean height predictions per RCP are given as a percentage of the 1961–1990 average height. On the left half of the figure CoV-calculations using all height prediction data is shown whereas for CoV-calculations made only from areas within the respective data ranges used for model development on the right half.
Coefficient of Variation Estimates Among General Atmospheric-Ocean Circulation Models Over the Study Areas
With respect to CoV among GCM for each potential planting site in the Nordic region we observed a substantial variation (0–27%, Figure 6) even when only considering areas within model predictability limits. CoV-estimates were also systematically greater and more widespread for more extreme RCPs. Regarding geographical trends, CoV-estimates were notably high (1034%) in the southernmost (LAT < 57°N, Denmark and Lithuania) and northernmost (LAT > 71°N, Nordkapp) margins of the studied region (11–61%). However, these regions were also consistently outside the model predictability (too long transfer distance) thus relying on extrapolation. There were also other specific alpine areas along the Scandian mountain range where CoV estimates were large (5% to a max of 187%). For low RCPs these areas were still largely outside model predictability limits as GDD5-levels were too low (Figures 6A,B), but for higher RCPs considerable portions of largely alpine locations were increasingly included within the area suitable for the model. For the parts of the mountain ranges, the high CoV-estimates in tree height are not ascribed to extrapolation.
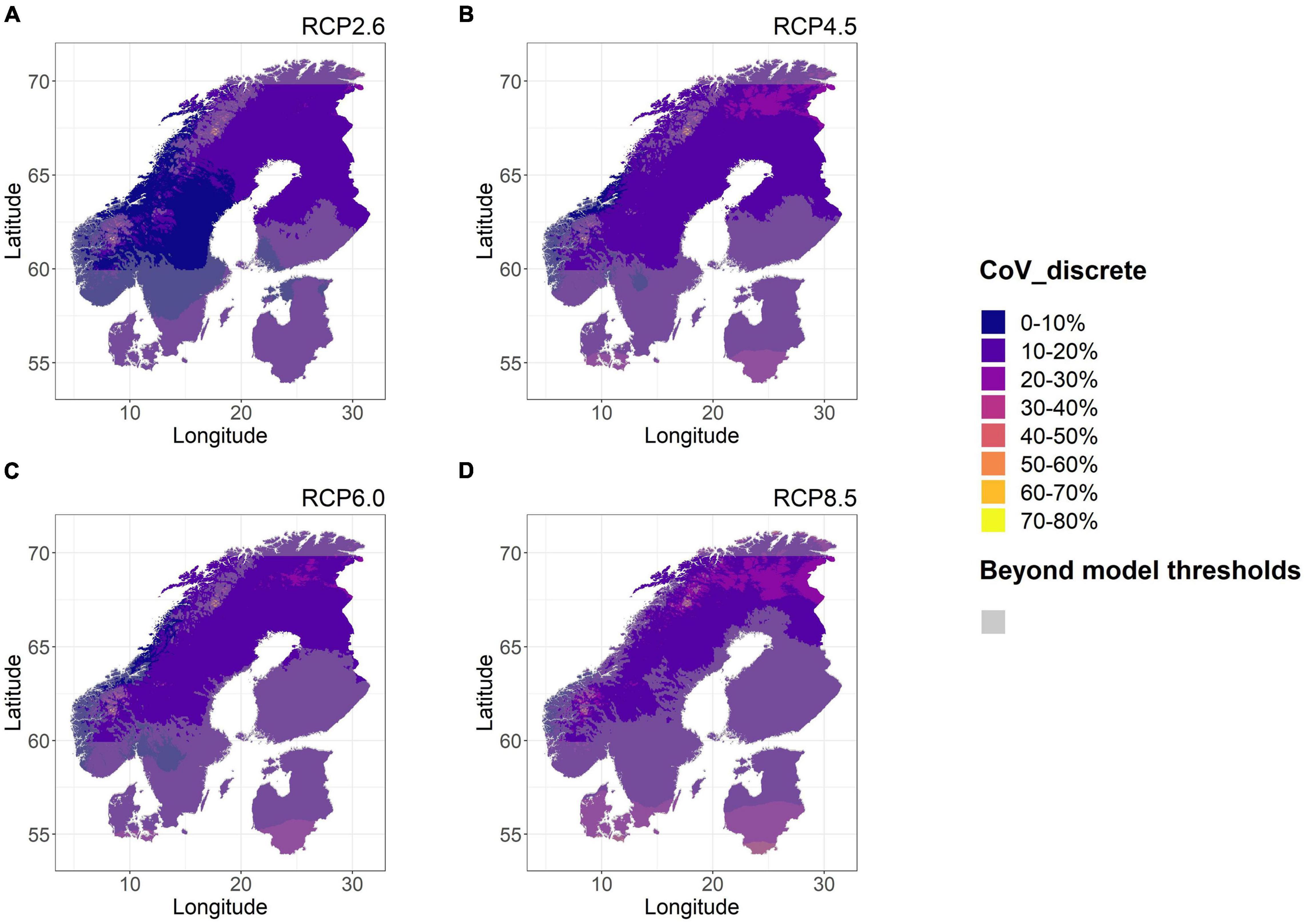
Figure 6. Coefficients of variation (CoV) for Scots pine tree height among GCMs, calculated for each 1 km × 1 km prediction and for each RCPs 2.6 (A), 4.5 (B), 6.0 (C), and 8.5 (D) in the Nordic region. All locations where environmental variables exceeded the model data range according to three GCMs or more have been dimmed in semi-transparent gray.
In contrast to the Nordic region, tree height CoV among GCMs for Scots pine in Spain, was consistently low (Figure 7) ranging only from (3–11%). Also tree height CoV did not depend on RCP or on whether the studied areas were within the model predictability thresholds (0–2%). This finding suggests that, in quantitative terms, the uncertainty of future tree height predictions in Spain is very limited.
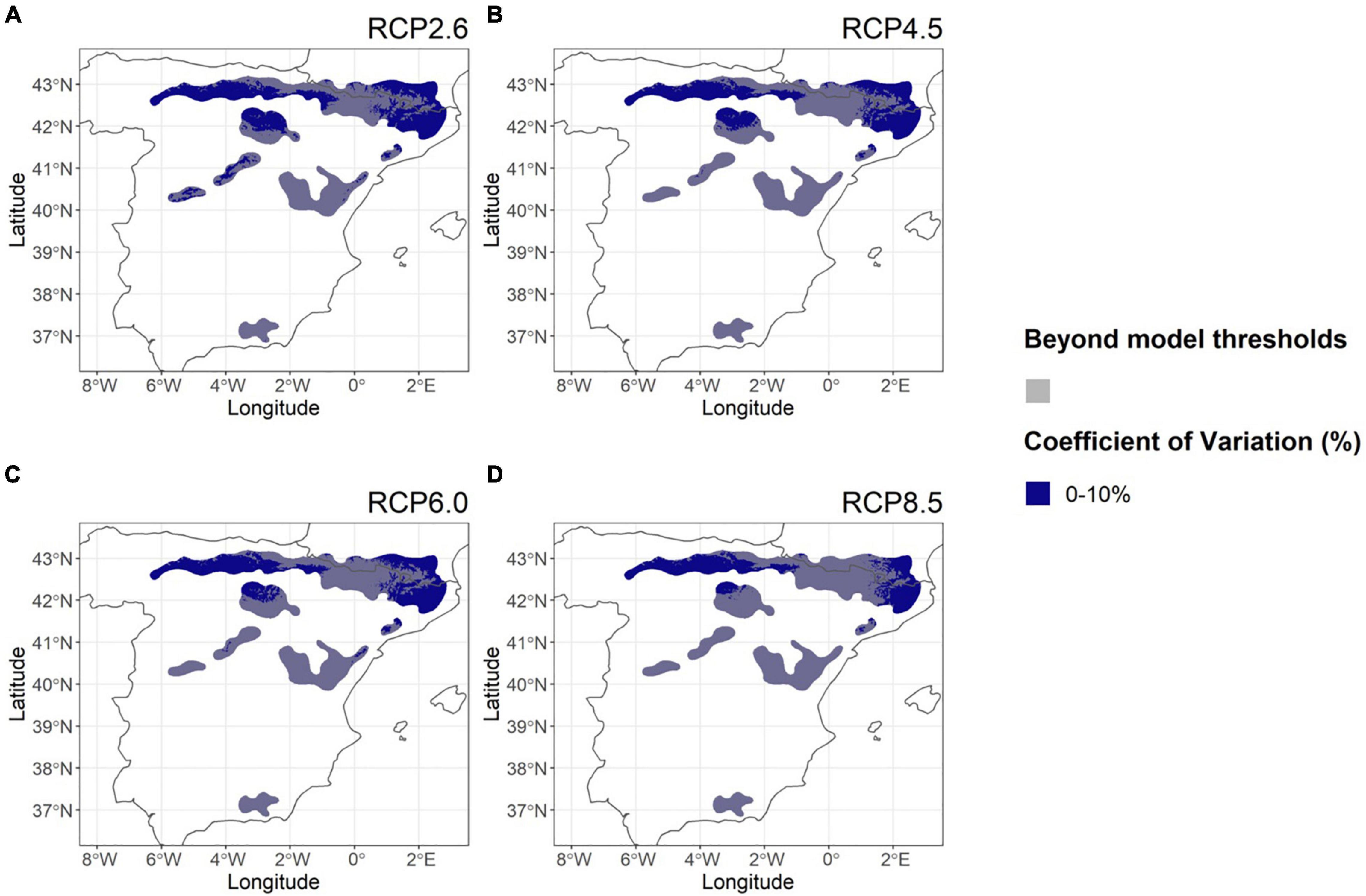
Figure 7. Coefficients of variation (CoV) for Scots pine tree height among available GCMs, calculated for each 1 km × 1 km prediction and for each RCPs 2.6 (A), 4.5 (B), 6.0 (C), and 8.5 (D) with Scots pine distribution areas in Spain. All locations where environmental/climate variables exceeded the model data range according to three GCMs have been dimmed in semi-transparent gray.
Discussion
The large variety of environments inhabited by Scots pine has promoted populations adapted to a multitude of range-wide environmental conditions. Identifying these conditions is essential to assess the vulnerability of different populations to future climates, which are themselves inherently uncertain. With a literature review and a modeling approach covering populations at the leading and trailing edge of Scots pine, we addressed the problem of how the uncertainty associated with future climate projections may differently affect populations’ vulnerability at the leading and trailing edges of the species range.
Drivers of Adaptation: From Literature Review to Modeling Approaches at the Leading and Trailing Margins
In general, our literature review showed that photoperiod constrains the tree height growth of local provenances in northern countries (Berlin et al., 2016), but also that northern and continental populations have adapted to a lower temperature sum to complete phenophases (Mátyás et al., 2004). Likewise, the Nordic model highlighted that photoperiod triggered local adaptation at the leading edge of the species, and that the GDD5 is an influential site-climatic predictor for tree height. This particular model is not new (Berlin et al., 2016), but we found it was robust for the Nordic area as all model terms previously indicated as highly significant remained highly significant, even though a very different climate dataset was used for this study (Supplementary Material 2). As in previous studies, we found that predictions of latitudinal transfer distance suggest that northward transferred material would achieve a greater height than local provenances, given increased GDD5 at the planting site (Rehfeldt et al., 2002; Reich and Oleksyn, 2008; Berlin et al., 2016). However, at the extreme leading margin where temperatures are low, the model suggested that provenances with very small transfer-distances would be best adapted with respect to tree height. It should, however, be cautioned that transfer distance curves for the Nordic model had a very slight curvature suggesting that adaptational patterns were not sharply expressed and that, in many situations, a wide set of provenances would be likely to perform well at any given planting site.
The literature search showed that in southern and central areas of Europe, the effect of drought on Scots pine growth is not latitudinally driven, but depends on soil fertility, site topography and tree growth in the pre-drought period (Bose et al., 2020). Earlier assessments of future climate change suggest that drought could also become an important driver of Scots pine growth in lowland areas in north-western Europe (Petr et al., 2014). Recent work suggests that Spanish plantations show a great capacity to adapt to local climate conditions (Vizcaíno-Palomar et al., 2019), and that provenances from wetter sites in particular are expected to demonstrate resilience to drought and show increased growth under warmer conditions (Rubio-Cuadrado et al., 2020). Maximum temperature of the warmest month can also be an important driver in southern Europe, with populations able to cope with a wider range of maximum temperatures expected to be more likely to survive under climate warming (Valladares et al., 2014). Scots pine populations at the trailing edge of the range can adapt to drought, as was confirmed by our Spanish model, adapted from Vizcaíno-Palomar et al. (2019) to be comparable to the Nordic one. The main change was that the climate of the site and the provenance were independent drivers in Vizcaíno-Palomar et al. (2019), whereas the model presented here combined these in a transfer distance. In our Spanish model the peaks of the SHM-transfer distance curves were generally close to the SHM index experienced at the planting site, suggesting close-to-local adaptation by SHM. This suggests a certain resilience to future increased SHM-values associated with drier site types, and agrees with the results of some previous studies (Vizcaíno-Palomar et al., 2019; Rubio-Cuadrado et al., 2020).
The notable discrepancies in marginal and conditional R2 between the Nordic and Spanish models are mostly due to the use of transfer distances that combine the climate of the provenance and the climate of field trial instead of using them separately in the model. As such, previous models based on the same data but calibrated with the climate of the field trial and the provenance independently (i.e., without using the transfer distance), showed higher statistical performance (Vizcaíno-Palomar et al., 2019) than results presented here. Thus, this difference in statistical performance is likely due to the use of the transfer distance, a useful variable for estimating the odds of a population to survive in different environmental conditions, but difficult to interpret in biological terms as it merges the climate of the field trial (that we can attribute to the effect of phenotypic plasticity) and the climate of the provenances (that we can relate to the genetic effect of the provenance).
Prediction Uncertainty With Respect to Different Climate Change Projections
The most fundamental issue linked to adaptation and resilience to climate change is related to the question whether tree height at a predetermined age would increase or decrease at the northern and southern margin regions of the Scots pine distribution and whether this is subject to considerable uncertainty dependent on the variation in climate change projections. To address this question, we used a classification method based on climate projection data from five GCMs (within an RCP), agreeing or disagreeing with the notion that tree height will increase under a future climate. Varying classifications among GCMs constitute a useful measure of prediction uncertainty, whereas agreement among classifications suggests a greater likelihood of tree height increase. To our knowledge, such an approach has not been used to assess prediction certainty/uncertainty for tree species in boreal or temperate regions, although a similar method was used to determine the impact of climate extremes on a broad European range of environmental situations (Lung et al., 2013), and on climate change impact on a tropical rubber tree species (Hevea brasiliensis, Golbon et al., 2018). In addition to addressing such a dichotomous question as presence/absence or height increase/decrease, it is also important to evaluate a quantitative degree of uncertainty in the magnitude of tree height increase/decrease. For this purpose, we opted to use the percentage coefficient of variation in order to quantify the prediction uncertainty among GCMs.
In the Nordic region, the results of this study suggest that Scots pine tree height will increase in the 2070s as a result of warming temperatures (higher GDD5) in comparison to the reference climate period. This prediction was stable across the entire Nordic region and did not vary with RCP or GCM, thus giving an impression of great certainty at first glance. This observation agrees with a series of ecological model simulation studies separately performed for Sweden (Bergh et al., 2010) and for Finland (Torssonen et al., 2015; Kellomäki et al., 2018), where the growth of Scots pine was expected to increase regardless of climate change scenario or timespan based on increasing GDD5, along with site factors such as soil moisture, nitrogen availability and within-stand light. When considering the magnitudes of the predicted tree height increases, however, we observed substantial variation (i.e., uncertainty) both spatially across the Nordic region and connected to GCMs and RCPs. Given a situation where all predictions were considered, the uncertainty in magnitude also appeared to be greater for high emission RCPs (e.g., 8.5) than for low emission RCPs (2.6). From a quantitative perspective our results suggest considerable variation and uncertainty for the Nordic region with respect to the magnitude of tree height increase among differing climate scenarios. In summary, our results indicate that Scots pine height growth in the Nordic region will benefit from climate change. However, considerable uncertainty was revealed in the extent and magnitude of that potential climate change benefit.
For Spain, our model predictions indicated greater uncertainty with respect to whether future Scots pine tree height would increase or decrease as a result of climate change, compared to the Nordic model. Some areas in northern Spain showed increases in future tree height, and other areas in central and southern Spain showed tree height decreases. However, the observed patterns did not vary much across emission scenarios (RCP) and in quantitative terms prediction variation, and thereby uncertainty, was small both with respect to spatial predictions and across different GCMs. This agreement of predictions suggests that even though there is some uncertainty whether Scots pine provenances will remain adapted to their Spanish range under a future climate, the quantitative consequences of climate change will be limited. It should be cautioned that soil physical and chemical properties might interact with climatic factors, providing the detailed site conditions that maintain a site suitable for Scots pine resilience into the future (Mathys et al., 2014; Redmond et al., 2015). However, our study did not take account of local variation in site soil conditions. The cautious predictions reported for Spain in our study contrasts considerably with an Europe-wide analysis of radial growth which suggested that climate suitability for Scots pine will decline in central Europe (Bombi et al., 2017). In particular, this study observed that the most extreme climate GCMs included were coherent with observed trends from dendrochronological data. However, the use of Ecological Niche Modeling (ENM) which relies on static species distribution data means that these stark findings do not account for potential local adaptation or plasticity that are both included in our models. Our results are nevertheless in agreement with another dendrochronological study that specifically covered the Spanish region and which also indicated some resilience of a central Spanish provenance to very dry conditions to which it was expected to be maladapted (Rubio-Cuadrado et al., 2020).
Prediction Uncertainties With Respect to Model Extrapolations
Apart from the uncertainty connected to the multitude of climate projection scenarios and their accuracy, the limitations of the applied linear mixed-effects models also have to be considered. A particular concern is that even predictions created by well developed and accurate models are subject to considerable error, and thereby uncertainty, if applied to climatic conditions outside the data range used for model development. Such problems of model limitation and extrapolation are relevant across several scientific fields that develop and utilize statistical models for prediction (see e.g., Miller et al., 2004; Stohlgren et al., 2011). Given the environmental conditions used for developing our adaptation transfer models, it is unlikely that extrapolation would be necessary because a wide variety of environmental conditions at field trial and provenance locations were studied. Nonetheless we observed that future climate change per se will create environmental conditions that necessitate extrapolated predictions provided that current height prediction models are used. We determined the extent of this issue and how its severity increased with increasing GHG emissions (i.e., RCP scenarios). For the Nordic region, we identified increasing GDD5 as the main reason for prediction extrapolation. For the areas where the majority of GCM predictions were made by extrapolation, there can be uncertainty about prediction quality even when GCM agreement is formally unanimous. For Spain, increasing temperature differentials, and to a limited degree increasing SHM, appeared to be the cause for extrapolated predictions. These increased temperature differentials may be linked to increased continentality as predicted for southern Europe (Szabó-Takács et al., 2015).
Given our findings it is reasonable to believe that the uncertainty and errors due to extrapolation in turn acts as a multiplier of the uncertainties connected to the variation in climate change scenarios previously discussed and may also result in systematic biases. In practical terms this presents a trade-off dilemma for foresters and tree ecologists because, on one hand, they need predictions of tree adaptation in order to assess the species resilience to climate change, while on the other hand, extrapolations which threaten model prediction accuracy and precision should always be avoided from a strictly conservative perspective. As a compromise, we present predictions that often are extrapolated, but we also explicitly highlight this type of uncertainty thereby making it possible to localize the issue. In the longer term, we instead emphasize increased provenance field testing throughout large environmental gradients including those outside the species range as a remedy. For the Nordic region, our results suggest that collection, collation, pre-analysis and modeling of additional data from field trials experiencing higher temperatures than what is currently included would result in improved models. For Nordic tree height models, it would also be possible to formally test climate variables that are currently employed in corresponding Spanish tree height models. For the Spanish model, our results also indicate that field trials located at sites experiencing greater temperature differentials would be useful in order to improve models and to reduce prediction uncertainty, although trees planted within very dry regions may not survive.
Other Uncertainties
For the purpose of this study, we used tree height as a proxy trait for tree fitness and using this trait we have illustrated climate associated prediction uncertainties. However, we are well aware that tree height growth only partially accounts for overall health, fitness and resilience of Scots pine. Seedling stage survival, phenology, water use efficiency, radial growth, fertility and resistance to pests and pathogens are additional and important aspects of fitness. Some of these traits are more or less important at the northern and southern margins, respectively. In the Nordic region, it is well known that early-life hardiness (i.e., seedling survival) is a crucial character in the face of a cold environment in general (Persson and Ståhl, 1993; Berlin et al., 2016), especially in the far North (LAT > 65°N) and at higher elevations (>400 m asl) where GDD5 historically is low (<800 day°C). In Spain, Scots pine survival analyzed from the same network of field trials and provenances was predicted to decrease in most parts of the species range, including the northern part of the range (Benito Garzón et al., 2011; Valladares et al., 2014), suggesting a likely trade-off between survival and growth in northern Spain that may lead to a less optimistic future than that shown here. This demographic compensation is a common phenomenon at the species margins (Doak and Morris, 2010; Benito Garzón et al., 2013; Peterson et al., 2018), where populations are expected to reach their tolerance limits and may present maladaptation (Fréjaville et al., 2020). If such additional measures of fitness are taken into account they may affect the assessment about the outlook of the species and the uncertainty of our predictions.
Another dimension of uncertainty is that the use of broad average-based and persistent climate variables based (e.g., temperature, precipitation, GDD5, SHM, etc.) overlooks the potential impact of extreme events and calamities that may occur sporadically but which may have a profound impact on Scots pine survival and health in general, and increases the uncertainty of predictions. For Scots pine, such extreme events could be storms, forest fires and attacks by various pests and pathogens (Lung et al., 2013; Allen et al., 2015; Seidl et al., 2017; Seidel et al., 2019). The prediction of stochastic extreme events is not as straightforward as the use of topographically downscaled climate data, based on averages of 30-year periods, which we used here. Extreme climatic events are more likely to be brief and could be extremely localized, thus requiring meteorological assessments made at an hour-by-hour resolution and which might have to target a different height above the ground than the classically used 2 m (e.g., Svystun et al., 2021). The impact of extreme events is also difficult to integrate into the classical adaptational model framework, but has been framed by Seidl et al. (2017).
Conclusion and Future Perspectives
In summary, the GCM-agreement results of our study suggests that Scots pine tree height will increase with great certainty in the Nordic region as an effect of climate change, while predictions of tree height in Spain under a future climate are more varied, and therefore uncertain with respect to increase or decrease. Coefficients of variation for tree height predictions in the Nordic region among climate scenarios were, however, considerably high, thereby suggesting uncertainty to the extent of predicted tree height increase and hence implying uncertainty in a quantitative sense. Conversely, coefficients of variation, spatial and among climate scenarios, for the Spanish region were consistently low thereby indicating limited prediction uncertainty. If taken at face value, prediction of future tree height in the Nordic region and in Spain appears to be subject to only low-to-moderate degrees of uncertainty if we only consider the variety of available climate change projections per se. For Scots pine in the Nordic region, climate change appears to have a predominantly positive influence and there were no obvious indications of threats against Scots pine adaptation and resilience. This was also the case in Spain except possibly for the southernmost Andalusian refugium. However, demographic compensation due to a likely decrease in population survival as a consequence of frost hardiness at the leading edge and drought at the trailing edge would reduce the odds of marginal populations to inhabit these regions in the near future.
Furthermore, our results showed that climate change will cause tree height model predictions to be increasingly based on extrapolation both in the Nordic and Spanish region. Our interpretation is that uncertainty due to extrapolation is the most important source of uncertainty with regards to future environmental conditions and this type of uncertainty is also more difficult to properly quantify. By using the method of GCM-agreement, we were nonetheless able to illustrate the geographical extent of uncertainty due to model extrapolation and thus assess the extent of the issue. However, in order to better account for model extrapolation uncertainty, we encourage more international collaboration to share datasets from larger networks of field trials throughout the Scots pine distribution range. Such model integration would then encompass data spanning a greater variety of environmental and climatological conditions thereby decreasing the need of extrapolated predictions. Nonetheless some extrapolation will still be unavoidable due to novel climates appearing.
Our attempt to review and model tree height with different adaptation drivers at the leading and trailing edge of Scots pine highlights the difficulty to apply range-wide models to a species with marked local adaptation to different conditions across its range. Furthermore, it suggests that new generation models accounting for Scots pine adaptation at large geographical scales under new climates would need to integrate fitness-related traits other than tree height, account for the risk and severity of extreme events and calamities induced by climate change and the odds of populations to adapt to them.
Data Availability Statement
The data analyzed in this study is subject to the following licenses/restrictions: Spanish field trial data is available upon request through www.genfored.es and Nordic field trial data is available upon request through www.skogforsk.se (aGVucmlrLmhhbGxpbmdiYWNrQHNrb2dmb3Jzay5zZQ==). Climate data is available through the Climate Downscaling Tool (ClimateDT) at ibbr.cnr.it/climate-dt. The raw global files of the downloaded GCMs are available at https://worldclim.org/data/v1.4/cmip5_30s.html.
Author Contributions
HH, MB, VB, MBG, NV-P, MM, ML, and DR conceived the study and its objectives. MM generated climate data for the reference period and for future climate scenarios. HH, NV-P, and MBG recalibrated and redeveloped the prediction models and generated the spatial tree height predictions. HH, VB, FT, NV-P, and MBG performed the downstream analyses on predictions and generated figures thereof. ML and VB performed the literature research on adaptive environmental drivers. HH and MBG organized and coordinated the manuscript writing and wrote the first draft. MB, MBG, and DR acted as quality checkers and contributed with the additional references. All authors read and commented on the manuscript.
Funding
This study was funded by the European Project H2020 “Adaptive breeding for productive, sustainable and resilient forests under climate change” (B4EST; grant agreement No 773383). In addition, a smaller portion (∼20%) which B4EST could not fund, was instead funded internally by the Forest Research Institute of Sweden (Skogforsk) for long-term strategic purposes for Swedish forest genetics and tree breeding (Project numbers 22211 and 22213).
Conflict of Interest
The authors declare that the research was conducted in the absence of any commercial or financial relationships that could be construed as a potential conflict of interest.
Publisher’s Note
All claims expressed in this article are solely those of the authors and do not necessarily represent those of their affiliated organizations, or those of the publisher, the editors and the reviewers. Any product that may be evaluated in this article, or claim that may be made by its manufacturer, is not guaranteed or endorsed by the publisher.
Acknowledgments
We would like to thank the Spanish Network of Genetic Trials (GENFORED) for access to the tree height data of Spanish field trials, which is publicly available upon request through www.genfored.es. We would also like to thank the Swedish University of Agricultural Sciences (SLU) and to Luonnonvarakeskus (LUKE) for the use of tree height data for Swedish and Finnish field trials respectively. Data is stored in DATAPLAN hosted by the Southern Tree Breeding Association (Australia) and is available upon request through contact with Skogforsk www.skogforsk.se. Finally we would also like to thank the assistance of Skogforsk colleagues Aron Davidsson and Sven Tegelmo for establishing the infrastructure for climate data grid management for the Nordic region.
Supplementary Material
The Supplementary Material for this article can be found online at: https://www.frontiersin.org/articles/10.3389/fevo.2021.724051/full#supplementary-material
References
Agúndez, D., Alía, R., and Diez, R. (1992). Variación de Pinus sylvestris en España: características de piñas y piñones. Investig. Agrar. Sist. Recur. For. 1, 151–162.
Aitken, S. N., and Bemmels, J. B. (2016). Time to get moving: assisted gene flow of forest trees. Evol. Appl. 9, 271–290. doi: 10.1111/eva.12293
Alía, R., Moro, J., Notivol, E., and Moro-Serrano, J. (2001). Genetic variability of Scots pine (Pinus sylvestris L.) provenances in Spain: growth traits and survival. Silva Fenn. 35, 27–38. doi: 10.14214/sf.601
Allen, C. D., Breshears, D. D., and McDowell, N. G. (2015). On underestimation of global vulnerability to tree mortality and forest die-off from hotter drought in the Anthropocene. Ecosphere 6, 1–55. doi: 10.1890/ES15-00203.1
Archambeau, J., Ruiz-Benito, P., Ratcliffe, S., Fréjaville, T., Changenet, A., Munoz Castaneda, J. M., et al. (2020). Similar patterns of background mortality across Europe are mostly driven by drought in European beech and a combination of drought and competition in Scots pine. Agric. For. Meteorol. 280:107772. doi: 10.1016/j.agrformet.2019.107772
Atkins, K. E., and Travis, J. M. J. (2010). Local adaptation and the evolution of species’ ranges under climate change. J. Theor. Biol. 266, 449–457. doi: 10.1016/j.jtbi.2010.07.014
Benito Garzón, M., Alía, R., Robson, T. M., and Zavala, M. A. (2011). Intra-specific variability and plasticity influence potential tree species distribution under climate change. Glob. Ecol. Biogeogr. 20, 766–778. doi: 10.1111/j.1466-8238.2010.00646.x
Benito Garzón, M., Robson, T. M., and Hampe, A. (2019). ΔTraitSDMs: species distribution models that account for local adaptation and phenotypic plasticity. New Phytol. 222, 1757–1765. doi: 10.1111/nph.15716
Benito Garzón, M., Ruiz-Benito, P., and Zavala, M. A. (2013). Interspecific differences in tree growth and mortality responses to environmental drivers determine potential species distributional limits in Iberian forests. Glob. Ecol. Biogeogr. 22, 1141–1151. doi: 10.1111/geb.12075
Benito Garzón, M., and Vizcaíno-Palomar, N. (2021). “Biogeographical patterns of local adaptation and plasticity of Mediterranean pines and their implication under climate change,” in MEDPINE - Pines and Their Mixed Forest Ecosystems in the Mediterranean Basin, eds G. Ne’eman and Y. Osem (Switzerland: Springer International Publishing).
Bergh, J., Nilsson, U., Kjartansson, B., and Karlsson, M. (2010). Impact of climate change on the productivity of silver birch, Norway spruce and Scots pine stands in Sweden and economic implications for timber production. Ecol. Bull. 53, 185–195.
Berlin, M., Persson, T., Jansson, G., Haapanen, M., Ruotsalainen, S., Bärring, L., et al. (2016). Scots pine transfer effect models for growth and survival in Sweden and Finland. Silva fenn. 50:1562. doi: 10.14214/sf.1562
Bombi, P., D’Andrea, E., Rezaie, N., Cammarano, M., and Matteucci, G. (2017). Which climate change path are we following? Bad news from Scots pine. PLoS One 12:e0189468. doi: 10.1317/journal.pone.0189468
Bose, A. K., Gessler, A., Bolte, A., Bottero, A., Buras, A., Cailleret, M., et al. (2020). Growth and resilience responses of Scots pine to extreme droughts across Europe depend on predrought growth conditions. Glob. Change Biol. 26, 4521–4537. doi: 10.1111/gcb.15153
Caudullo, G., Welk, E., and San-Miguel-Ayanz, J. (2017). Chorological maps for the main European woody species. Data Brief 12, 662–666. doi: 10.1016/j.dib.2017.05.007
Changenet, A., Ruiz-Benito, P., Ratcliffe, S., Fréjaville, T., Archambeau, J., Lehtonen, A., et al. (2021). Occurrence but not intensity of mortality rises towards the climatic trailing edge of tree species ranges in European forests. Glob. Ecol. Biogeogr. 30, 1356–1374. doi: 10.1111/geb.13301
Chevin, L.-M., Lande, R., and Mace, G. M. (2010). Adaptation, plasticity, and extinction in a changing environment: towards a predictive theory. PLoS Biol. 8:e1000357. doi: 10.1371/journal.pbio.1000357
Doak, D. F., and Morris, W. F. (2010). Demographic compensation and tipping points in climate-induced range shifts. Nature 467, 959–962. doi: 10.1038/nature09439
Eriksson, G. (2008). Pinus sylvestris : Recent Genetic Research. Uppsala: Department of Plant Biology and Forest Genetics.
Fréjaville, T., and Benito Garzón, M. (2018). The EuMedClim database: yearly climate data (1901–2014) of 1 km resolution grids for Europe and the Mediterranean basin. Front. Ecol. Evol. 6:31. doi: 10.3389/fevo.2018.00031
Fréjaville, T., Vizcaíno-Palomar, N., Fady, B., Kremer, A., and Benito Garzón, M. (2020). Range margin populations show high climate adaptation lags in European trees. Glob. Change Biol. 26, 484–495. doi: 10.1111/gcb.14881
Gárate Escamilla, H., Hampe, A., Vizcaíno-Palomar, N., Robson, T. M., and Benito Garzón, M. (2019). Range-wide variation in local adaptation and phenotypic plasticity of fitness-related traits in Fagus sylvatica and their implications under climate change. Glob. Ecol. Biogeogr. 28, 1336–1350. doi: 10.1111/geb.12936
Golbon, R., Cotter, M., and Sauerborn, J. (2018). Climate change impact assessment on the potential rubber cultivating area in the greater Mekong subregion. Environ. Res. Lett. 13:084002. doi: 10.1088/1748-9326/aad1d1
González-Martínez, S. C., Burczyk, J., Nathan, R., Nanos, N., Gil, L., and Alía, R. (2006). Effective gene dispersal and female reproductive success in Mediterranean maritime pine (Pinus pinaster Aiton). Mol. Ecol. 15, 4577–4588. doi: 10.1111/j.1365-294X.2006.03118.x
Gray, L. K., Rweyongeza, D., Hamann, A., John, S., and Thomas, B. R. (2016). Developing management strategies for tree improvement programs under climate change: Insights gained from long-term field trials with lodgepole pine. For. Ecol. Manage. 377, 128–138. doi: 10.1016/j.foreco.2016.06.041
Harris, I., Osborn, T. J., Jones, P., and Lister, D. (2020). Version 4 of the CRU TS monthly high-resolution gridded multivariate climate dataset. Sci. Data 7:109. doi: 10.1038/s41597-020-0453-3
Hausfather, Z., and Peters, G. P. (2020). Emissions - the ‘business as usual’ story is misleading. Nature 577, 618–620. doi: 10.1038/d41586-020-00177-3
Hijmans, R. J. (2020). raster: Geographic Data Analysis and Modeling. R Package Version 3.3-13. Avalable online at: https://CRAN.R-project.org/package=raster (accessed October 11, 2021).
Hijmans, R. J., Cameron, S. E., Parra, J. L., Jones, P. G., and Jarvis, A. (2005). Very high resolution interpolated climate surfaces for global land areas. Int. J. Clim. 25, 1965–1978. doi: 10.1002/joc.1276
IPCC (2014). “Climate change 2014: synthesis report,” in Contribution of Working Groups I, II and III to the Fifth Assessment Report of the Intergovernmental Panel on Climate Change, eds R. K. Pachauri and L. A. Meyer (Geneva: IPCC).
Isaac-Renton, M. G., Roberts, D. R., Hamann, A., and Spiecker, H. (2014). Douglas-fir plantations in Europe: a retrospective test of assisted migration to address climate change. Glob. Change Biol. 20, 2607–2617. doi: 10.1111/gcb.12604
Kellomäki, S., Strandman, H., Heinonen, T., Asikainen, A., Venäläinen, A., and Peltola, H. (2018). Temporal and spatial change in diameter growth of boreal Scots pine, Norway spruce, and Birch under recent-generation (CMIP5) global climate model projections for the 21st century. Forests 9:118. doi: 10.3390/f9030118
Lung, T., Dosio, A., Becker, W., Lavalle, C., and Bouwer, L. M. (2013). Assessing the influence of climate model uncertainty on EU-wide climate change impact indicators. Clim. Change 120, 211–227. doi: 10.1007/s10584-013-0825-1
Matesanz, S., and Ramírez-Valiente, J. A. (2019). A review and meta-analysis of intraspecific differences in phenotypic plasticity: implications to forecast plant responses to climate change. Glob. Ecol. Biogeogr. 28, 1682–1694. doi: 10.1111/geb.12972
Mathys, A., Coops, N. C., and Waring, R. H. (2014). Soil water availability effects on the distribution of 20 tree species in western North America. For. Ecol. Manage. 313, 144–152. doi: 10.1016/j.foreco.2013.11.005
Matías, L., and Jump, A. (2014). Impacts of predicted climate change on recruitment at the geographical limits of Scots pine. J. Exp. Bot. 65, 299–310. doi: 10.1093/jxb/ert376
Mátyás, C. (1994). Modeling climate-change effects with provenance test data. Tree Physiol. 14, 797–804.
Mátyás, C., Ackzell, L., and Samuel, C. J. A. (2004). EUFORGEN Technical Guidelines for Genetic Conservation and Use for Scots Pine (Pinus sylvestris). Rome: International Plant Genetic Resources Institute.
Miller, J. R., Turner, M. G., Smithwick, E. A. H., Dent, L., and Stanley, E. H. (2004). Spatial extrapolation: the science of predicting ecological patterns and processes. Bioscience 54, 310–320.
Moreno, A., and Hasenauer, H. (2016). Spatial downscaling of European climate data. Int. J. Climatol. 36, 1444–1458. doi: 10.1002/joc.4436
Nicotra, A. B., Atkin, O. K., Bonser, S. P., Davidson, A. M., Finnegan, E. J., Mathesius, U., et al. (2010). Plant phenotypic plasticity in a changing climate. Trends Plant Sci. 15, 684–692. doi: 10.1016/j.tplants.2010.09.008
Oleksyn, J., Tjoelker, M. G., and Reich, P. B. (1998). Adaptation to changing environment in Scots pine populations across a latitudinal gradient. Silva Fenn. 32, 129–140. doi: 10.14214/sf.691
Pedlar, J. H., and McKenney, D. W. (2017). Assessing the anticipated growth response of northern conifer populations to a warming climate. Sci. Rep. 7:43881. doi: 10.1038/srep43881
Persson, B. (1994). Effects of Climate and Provenance Transfer on Survival, Production and Stem Quality of Scots Pine (Pinus sylvestris L.) in Northern Sweden. Ph.D. thesis. Sweden: Swedish University of Agricultural Sciences.
Persson, B., and Ståhl, E. G. (1993). Effects of Provenance Transfers in an Experimental Series of Scots Pine (Pinus sylvestris L.) in Northern Sweden. Report 35. Sweden: Swedish University of Agricultural Sciences. In Swedish with English summary).
Peterson, M. L., Doak, D. F., and Morris, W. F. (2018). Both life-history plasticity and local adaptation will shape range-wide responses to climate warming in the tundra plant Silene acaulis. Glob. Change Biol. 24, 1614–1625. doi: 10.1111/gcb.13990
Petr, M., Boerboom, L. G. J., van der Veen, A., and Ray, D. (2014). A spatial and temporal drought risk assessment of three major tree species in Britain using probabilistic climate change projections. Clim. Change 124, 791–803. doi: 10.1007/s10584-014-1122-3
Prus-Glowacki, W., Stephan, B. R., Bujas, E., Alía, R., and Marciniak, A. (2003). Genetic differentiation of autochthonous populations of Pinus sylvestris (Pinaceae) from the Iberian Peninsula. Plant. Syst. Evol. 239, 55–66. doi: 10.1007/s00606-002-0256-3
R Core Team (2020). R: A Language and Environment for Statistical Computing. Vienna: R Foundation for Statistical Computing.
Ramirez-Villegas, J., and Jarvis, A. (2010). Downscaling Global Circulation Model Outputs: The Delta Method Decision and Policy Analysis Working Paper no. 1. Consultative Group on International Agricultural Research. Report, May 2010. Cali: International Center for Tropical Agriculture (CIAT).
Redmond, M. D., Cobb, N. S., Clifford, M. J., and Barger, N. N. (2015). Woodland recovery following drought-induced tree mortality across an environmental stress gradient. Glob. Change Biol. 21, 3685–3695. doi: 10.1111/gcb.12976
Rehfeldt, G. E., Tchebakova, N. M., Parfenova, Y. I., Wykoff, W. R., Kuzmina, N. A., and Milyutin, L. I. (2002). Intraspecific responses to climate in Pinus sylvestris. Glob. Change Biol. 8, 912–929. doi: 10.1046/j.1365-2486.2002.00516.x
Reich, P. B., and Oleksyn, J. (2008). Climate warming will reduce growth and survival of Scots pine except in the far north. Ecol. Lett. 11, 588–597. doi: 10.1111/j.1461-0248.2008.01172.x
Robledo-Arnuncio, J. J., Collada, C., Alía, R., and Gil, L. (2005). Genetic structure of montane isolates of Pinus sylvestris L. in a Mediterranean refugial area. J. Biogeogr. 32, 595–605. doi: 10.1111/j.1365-2699.2004.01196.x
Rubio-Cuadrado, Á, Camarero, J. J., Gómez, C., Cañellas, I., Aulló-Maestro, I., Gil, L., et al. (2020). Scots pine plantations growth adaptation to climate warming in locations at the southernmost distribution limit of the species. Dendrochronologia 63:125745. doi: 10.1016/j.dendro.2020.125745
Sáenz-Romero, C., Lamy, J.-B., Ducousso, A., Musch, B., Ehrenmann, F., Delzon, S., et al. (2017). Adaptive and plastic responses of Quercus petraea populations to climate across Europe. Glob. Change Biol. 23, 2831–2847. doi: 10.1111/gcb.13576
Savva, Y. V., and Vaganov, E. A. (2003). Scots Pine Adaptability to Climatic Change. XII World Forestry Congress, 2003, Conference Proceedings 0507-B4. Québec. Available online at: https://www.fao.org/3/XII/0507-B4.htm (accessed November 2, 2020).
Seidel, H., Matiu, M., and Menzel, A. (2019). Compensatory growth of Scots pine seedlings mitigates impacts of multiple droughts within and across years. Front. Plant Sci. 10:519. doi: 10.3389/fpls.2019.00519
Seidl, R., Thom, D., Kautz, M., Martin-Benito, D., Peltoniemi, M., Vacchiano, G., et al. (2017). Forest disturbances under climate change. Nat. Clim. Change 7, 395–402. doi: 10.1038/nclimate3303
Stohlgren, T. J., Jarnevich, C. S., Esaias, W. E., and Morisette, J. T. (2011). Bounding species distribution models. Curr. Zool. 57, 642–647. doi: 10.1093/czoolo/57.5.642
Svystun, T., Lundströmer, J., Berlin, M., Westin, J., and Jönsson, A. M. (2021). Model analysis of temperature impact on the Norway spruce provenance specific bud burst and risk of frost damage. For. Ecol. Manage 493:16. doi: 10.1016/j.foreco.2021.119252
Szabó-Takács, B., Farda, A., Zahradníček, P., and Štěpánek, P. (2015). Continentality in Europe according to various resolution regional climate models with A1B scenario in the 21st century. Idõjárás Q. J. Hung. Meteorol. Ser. 119, 515–535.
Torssonen, P., Strandman, H., Kellomäki, S., Kilpelainen, A., Jylha, K., Asikainen, A., et al. (2015). Do we need to adapt the choice of main boreal tree species in forest regeneration under the projected climate change? Forestry 88, 564–572. doi: 10.1093/forestry/cpv023
Valladares, F., Matesanz, S., Guilhaumon, F., Araújo, M. B., Balaguer, L., Benito Garzón, M., et al. (2014). The effects of phenotypic plasticity and local adaptation on forecasts of species range shifts under climate change. Ecol. Lett. 17, 1351–1364. doi: 10.1111/ele.12348
Vizcaíno-Palomar, N., Fady, B., Alia Miranda, R., Mutke, S., Raffin, A., and Benito Garzón, M. (2020). The legacy of climate variability over the last century on populations’ phenotypic variation in tree height. Sci. Tot. Environ. 749:141454. doi: 10.1016/j.scitotenv.2020.141454
Vizcaíno-Palomar, N., González-Muñoz, N., González-Martínez, S. C., Alia, R., and Benito Garzón, M. (2019). Most southern Scots pine populations are locally adapted to drought for tree height growth. Forests 10:555. doi: 10.3390/f10070555
Keywords: adaptation, tree height, mixed-effect models, climate change, Spain, Nordic countries of Europe, provenance, Pinus sylvestris (L.)
Citation: Hallingbäck HR, Burton V, Vizcaíno-Palomar N, Trotter F, Liziniewicz M, Marchi M, Berlin M, Ray D and Benito Garzón M (2021) Managing Uncertainty in Scots Pine Range-Wide Adaptation Under Climate Change. Front. Ecol. Evol. 9:724051. doi: 10.3389/fevo.2021.724051
Received: 11 June 2021; Accepted: 21 October 2021;
Published: 12 November 2021.
Edited by:
Bradley S. Case, Auckland University of Technology, New ZealandReviewed by:
Sergio Noce, Fondazione Centro Euro-Mediterraneo sui Cambiamenti Climatici (CMCC), ItalyMartina Pollastrini, University of Florence, Italy
Copyright © 2021 Hallingbäck, Burton, Vizcaíno-Palomar, Trotter, Liziniewicz, Marchi, Berlin, Ray and Benito Garzón. This is an open-access article distributed under the terms of the Creative Commons Attribution License (CC BY). The use, distribution or reproduction in other forums is permitted, provided the original author(s) and the copyright owner(s) are credited and that the original publication in this journal is cited, in accordance with accepted academic practice. No use, distribution or reproduction is permitted which does not comply with these terms.
*Correspondence: Henrik R. Hallingbäck, SGVucmlrLkhhbGxpbmdiYWNrQHNrb2dmb3Jzay5zZQ==; Marta Benito Garzón, bWFydGEuYmVuaXRvLWdhcnpvbkBpbnJhZS5mcg==