- 1Joint Research Centre, European Commission, Ispra, Italy
- 2Department of Biodiversity and Molecular Ecology, Research and Innovation Centre, Fondazione Edmund Mach, San Michele all’Adige, Italy
The current and future consequences of anthropogenic impacts such as climate change and habitat loss on ecosystems will be better understood and therefore addressed if diverse ecological data from multiple environmental contexts are more effectively shared. Re-use requires that data are readily available to the scientific scrutiny of the research community. A number of repositories to store shared data have emerged in different ecological domains and developments are underway to define common data and metadata standards. Nevertheless, the goal is far from being achieved and many challenges still need to be addressed. The definition of best practices for data sharing and re-use can benefit from the experience accumulated by pilot collaborative projects. The Euromammals bottom-up initiative has pioneered collaborative science in spatial animal ecology since 2007. It involves more than 150 institutes to address scientific, management and conservation questions regarding terrestrial mammal species in Europe using data stored in a shared database. In this manuscript we present some key lessons that we have learnt from the process of making shared data and knowledge accessible to researchers and we stress the importance of data management for data quality assurance. We suggest putting in place a pro-active data review before data are made available in shared repositories via robust technical support and users’ training in data management and standards. We recommend pursuing the definition of common data collection protocols, data and metadata standards, and shared vocabularies with direct involvement of the community to boost their implementation. We stress the importance of knowledge sharing, in addition to data sharing. We show the crucial relevance of collaborative networking with pro-active involvement of data providers in all stages of the scientific process. Our main message is that for data-sharing collaborative efforts to obtain substantial and durable scientific returns, the goals should not only consist in the creation of e-infrastructures and software tools but primarily in the establishment of a network and community trust. This requires moderate investment, but over long-term horizons.
Introduction
The knowledge of intimate details of animal lives has immensely grown in the last decades, alongside technologies and techniques to study them (Kays et al., 2015; Harcourt et al., 2019). If ecology is evolution in space and time, ecological processes are characterised by spatial and temporal dimensions that only recently could be taken into account (Cagnacci et al., 2010). Bio-logging units mounted on individual animals have become increasingly sophisticated, long-lasting and able to record near-to-continuous information on animal behaviour and physiology. In parallel, remote sensing sensors on- (e.g., camera traps) or off-ground (e.g., drones, satellites) allow the extraction of environmental variables associated with animal behaviour (Ossi et al., 2019). One ultimate ambition is the reconciliation of individual-based empirical knowledge and modelling, with a population- (or ecosystem-) level state at a certain point in time (or Lagrangian vs Eulerian approach; Smouse et al., 2010). This step can only be made if data from individuals across multiple populations and diverse ecosystems are analysed, and such information is leveraged to parametrise mechanistic models (Morales et al., 2010).
The ability to scale up from individual-based knowledge to ecosystem complexity becomes particularly relevant to forecast current and future scenarios of climate change and habitat loss (Jeltsch et al., 2013). Further, the dramatic emergence of the field of “crisis ecology” (Leigh, 2005) and the compelling need to deeply understand the inter-relationship between human threats and ecosystem resilience, emphasises the importance of joining and analysing data from different sources in a timely, robust and effective way (“data sharing readiness”). The importance of data sharing and reuse is well recognised in the ecological community (Michener, 2015) and in science in general (see e.g., Gewin, 2016), and it is becoming more and more urgent. Animal ecology data that have been, or are being collected, might become of paramount importance to address questions that were unforeseen when data collection projects were initiated. This is, for example, the case for the COVID-19 bio-logging initiative, which is using data collected during the Anthropause (Rutz et al., 2020) to disentangle animal behavioural responses to human presence and disturbance, while data collection during COVID-19 pandemic are ongoing. The concept of “data sharing readiness” refers to reliable and consistently curated data that are stored in accessible repositories for use by the scientific community. In more technical terms this corresponds to standardised data sources shared in repositories that are recognised and accessible to the scientific communities. This is especially relevant in animal ecology where the acquisition of large scale individual-based information currently relies on collaborative networks and data sharing initiatives due to the cost of field projects and bio-logging equipment.
While possible standards to translate movement ecology data and metadata into relevant conservation and management practices (Hays et al., 2019) are being discussed in the literature (Campbell et al., 2016; Sequeira et al., 2021), at this stage they are not yet completely defined, shared with the community and adopted. In particular, existing data are not exposed in a standard, machine-readable format, limiting the establishment of a network of automatically communicating repositories. Nevertheless, examples of community-based data repositories that facilitate data gathering, harmonisation and sharing can support this process. In this manuscript we aim to provide practical directions to a more effective data management and sharing in movement ecology, presented as lessons learnt from the Euromammals collaborative network for terrestrial animal ecology.
Materials and Methods
The Euromammals Initiative
The Euromammals bottom-up initiative has pioneered collaborative science in spatial animal ecology. Euromammals began in 2007 as a data sharing platform to facilitate collaborative work for the European roe deer working group (Andersen et al., 1998; Cagnacci and Urbano, 2008). Since then, the Euromammals collaborative network has expanded to include research communities working on several European terrestrial mammals. The network includes 7 species or groups of species and more than 150 institutes from 34 countries in June 2021 (see Figure 1). The number of partners, once the project was established and consolidated, has been growing quickly in parallel with the trust from the scientific communities studying the target species. The goal of the platform is to harmonise (i.e., normalise in format, structure, information content and terminology), data sets shared by different partners, to make them analysis-ready to address ecological questions at broader spatio-temporal scales.
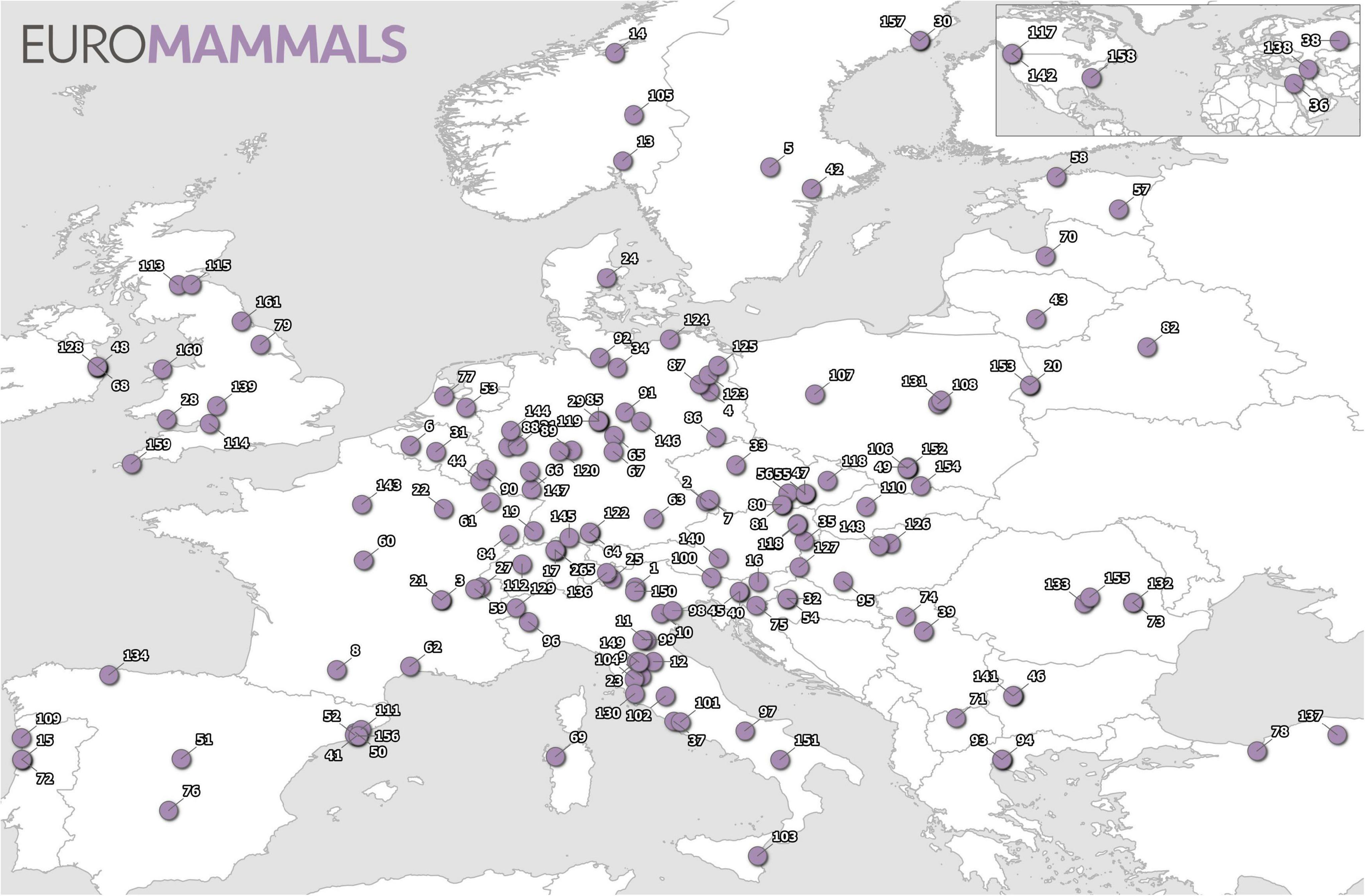
Figure 1. Distribution of the Euromammals network partners as of June 2021. The name of partners is reported in the Supplementary Table 1.
For almost 15 years, Euromammals has tackled data management problems for collaborative science while considering the requirements for information and data sharing emerging from species-based communities with a large number of partners. Indeed, the direct and active involvement of the larger scientific community makes knowledge gaps more visible and encourages researchers to fill them (Costello et al., 2018). Euromammals relies on a shared repository that stores species-specific databases organised and managed to optimise support to the scientific networks. The initial development of the system was an extension of the platform created for one of the first partner institutes (Cagnacci and Urbano, 2008) and contributed to setting clearly defined principles in wildlife tracking data management (Urbano et al., 2010). Currently, seven databases have been developed: roe deer (Capreolus capreolus)1, red deer (Cervus elaphus)2, wild boar (Sus scrofa)3, wildcat (Felis silvestris)4, lynx (Lynx lynx)5, ibex (Capra ibex)6, and small mammals.7 The core information collected is telemetry data (GPS, VHF, and accelerometers) and, depending on the species, information about individuals, mortality, captures, contacts, hunting regimes, and road kills. A simplified model of the core components of the eurodeer database is illustrated in Figure 2. Additionally, the longitudinal database EuroCaM, with image analysis outputs from camera traps, has also been developed.
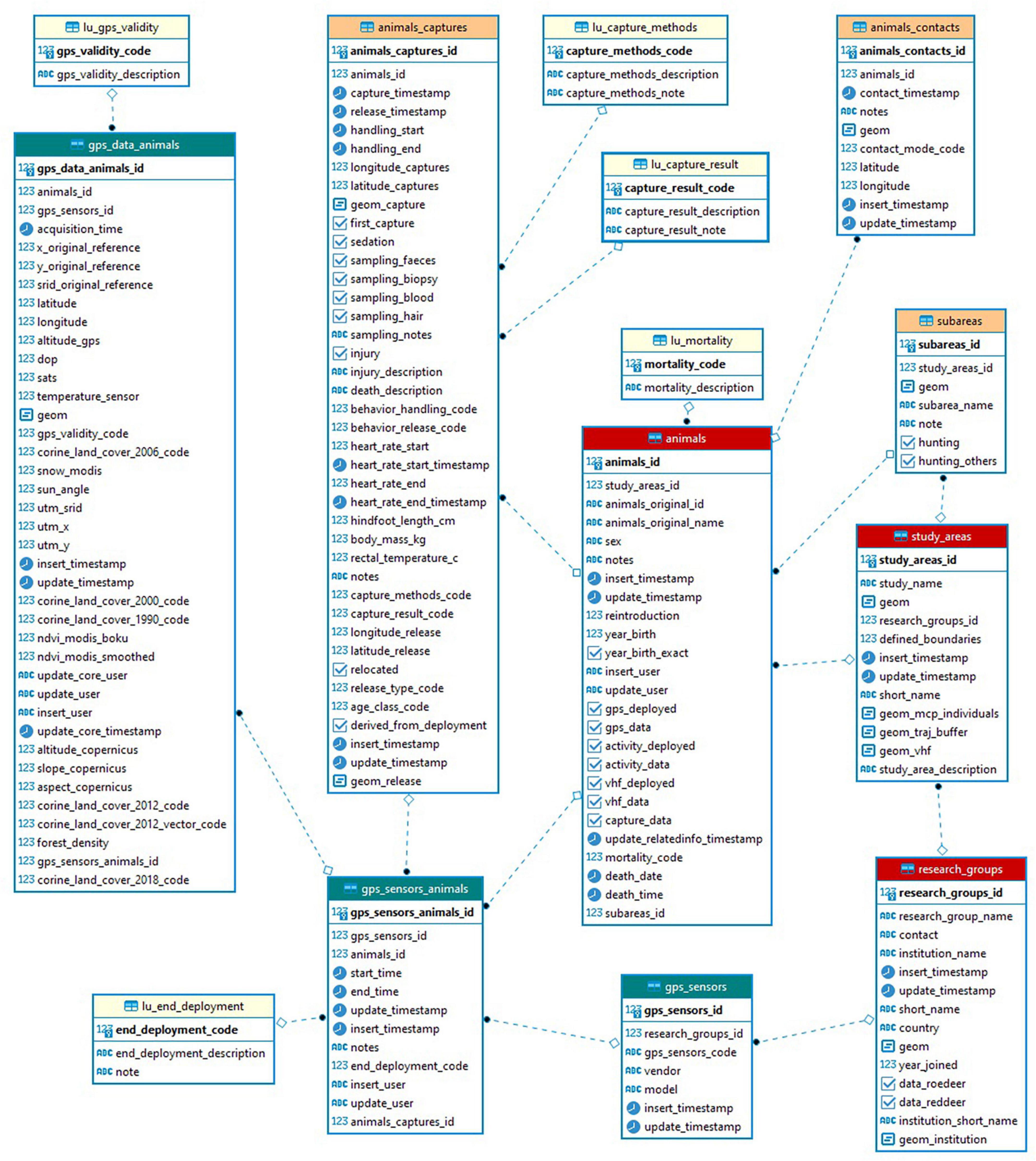
Figure 2. Euromammals simplified database core data model for roe deer GPS data. The main elements are the research groups, the study areas, the animals, the sensors, the deployments of the sensors on the animals, the animals captures, the animal generic contacts (e.g., direct sightseeing, carcass recovery), and the locations generated by the sensors associated to an animal and to a set of environmental variables based on European wide data sets.
Euromammals databases are not meant to be the principal repository of the data provided by partners. The Euromammals databases store harmonised and quality checked copies of the original data sets, integrated in a single, centralised repository where partners can jointly analyse the combination of all available data from different areas and environmental gradients. Each database has a data curator with a background both in biology and data management who collates data from partners. Data quality is reviewed through formal and partially automated controls of consistency and completeness, but also through expert-based checks specific to the biology of each species. Errors, outlier and suspicious information are relayed back to data owners and fixed in an iterative and interactive process that often lasts several weeks. Data curators are also available to provide all necessary support to project partners, for example helping to query the databases for specific analysis. At the end of the process, all data is integrated under a common database data model with the same semantic meaning, references, and units across the different data sets. Data is then associated with a set of environmental covariates (i.e., land cover/use, altitude, slope, aspect, forest density, NDVI, snow cover) that are available homogeneously for all of Europe, most of them obtained by the EU Copernicus project (European Union, 2021). A database manager develops the databases, defines the data processing protocols, ensures that the same level of quality is reached in all databases and manage the data access policy based on the database permission tools.
The databases are built on an open software platform (PostgreSQL + PostGIS) and are accessible to all partners. The database server and IT support is provided by one of the founding partners, the Edmund Mach Foundation, Trento, Italy. The documentation about the databases is published on Github.8 Technical support is also provided when data is extracted for specific studies, as described under the Terms of Use signed by all partners (in essence, research institutes or organisations that own the data must be asked permission for their use for each new study).
To date (June 2021) the databases store movement data of more than 4,500 individuals and 15,000,000 GPS locations distributed across 120 study areas from southern Italy to north of Sweden, and from Portugal to eastern Poland, extending across a wide gradient of environmental, topographic and climatic contexts.
The Euromammals collaborative network aims to produce scientific outcomes based on data and knowledge sharing regarding animal populations across environmental gradients. Indeed, partners cooperate on the entire scientific process, from analysis to publishing, so that data sharing is the mean for the collaborative scientific work, rather than the final goal. This peculiarity also shapes the way data is made available, i.e., through the network that is open to any interested researcher with data, knowledge or interest in the scientific goals, striving toward the FAIR Principles for scientific data and metadata (Findable, Accessible, Interoperable, and Reusable; Wilkinson et al., 2016). Once harmonised and quality checked in the Euromammals community-specific databases, data owners can easily upload their data onto larger open data repositories, also assuring a controlled data quality for downstream data users. Further, Euromammals provides its partners with a data storage infrastructure that is coherent with the TRUST guiding principles to promote digital repository trustworthiness (Lin et al., 2020): Transparency, Responsibility, User focus, Sustainability and Technology. The idea behind these principles is that repositories must earn the trust of the communities they intend to serve and demonstrate that they are reliable and capable of appropriately managing the data they hold. In the Euromammals repositories this goal is reached by reversing the perspective: the repository is not distinct from the community it serves, but it is a tool created and managed for, and by, the community itself, creating a deeper sense of belonging.
Results
The Euromammals network has been actively exploiting the shared databases to produce collaborative science. Some of the scientific outputs are listed in Table 1. The bottom-up development of the Euromammals databases made us encounter challenges and seize opportunities in the different phases of the collaborative process: from data use policy to data gathering, from the creation of mutual community trust to data harmonisation, from financial sustainability to data analysis and finalising scientific outputs. In the next sections, we focus on the technical challenges and main lessons learnt from the data management process over the years.
Quality Assurance/Quality Control Process
The data collation could not simply consist of gathering data from network members and depositing them in a common repository. The data sets provided were affected by errors, inconsistencies or incomplete information that, although rare (2–3% ca of the whole data points), would impact the reliability of some analysis. Indeed, some research questions target rare movements (e.g., rut excursion, Debeffe et al., 2014) or behaviours happening in short intervals of time (e.g., migratory movements between seasonal ranges, Cagnacci et al., 2011), for which even rare data errors can undermine robust analysis and lead to misleading conclusions. Errors did not depend on negligence, rather on the lack of proper tools, expertise and resources for optimised data management. Further, data were often screened and quality checked for the specific goals they were collected for, but inconsistencies could emerge when other analyses were attempted. A sketch of the typical process of the data quality control in Euromammals is illustrated in the Figure 3.
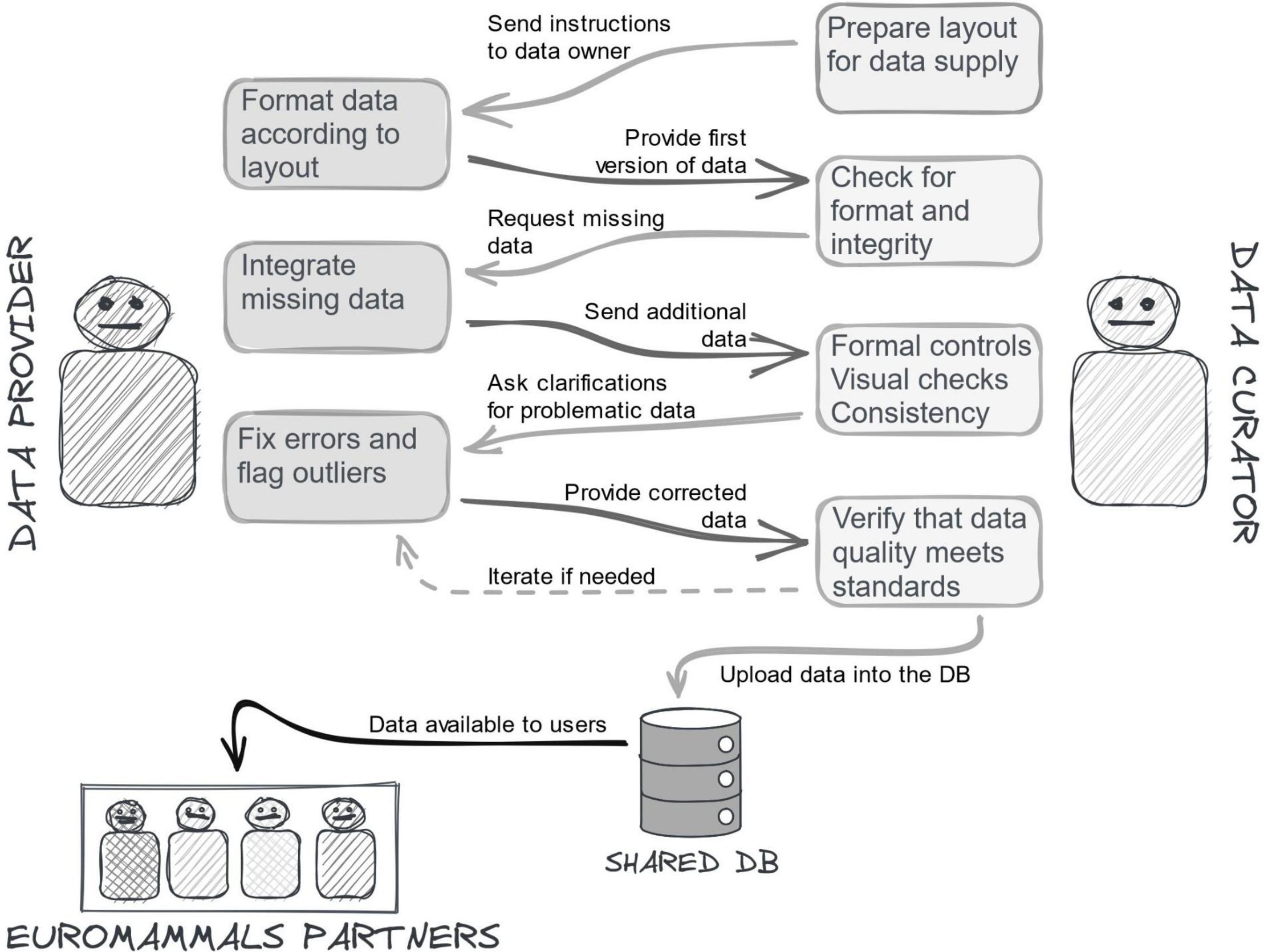
Figure 3. Entering data into Euromammals databases is an interactive and iterative process with multiple steps between the partners who provide the data and the data curators who check for their completeness, correctness and consistency. The controls are not only formal, but also substantial. For this reason, data curators are always biologists acquainted with the scientific studies. The process can be time consuming, but it is the only way to ensure the best possible quality of stored data.
Examples below provide further clarity on the types of emerging issues we encountered.
Example 1
GPS sensors can miss the location acquisition and such instances are normally recorded in the sensor records as missing coordinates. This information is important to estimate the effect of imprecise and habitat-biased locations in ecological analyses (Frair et al., 2010) and to evaluate the efficiency of telemetry devices (Hofman et al., 2019). When data providers omitted these records from their data sets because it was not relevant for their study, we had to request the full list of records to be included instead.
Example 2
An imprecise definition of the time of deployment of the GPS sensor on animals can be identified through suspicious patterns of the trajectory (for example including the road from the research centre to the animal capture site). These anomalies can be easily spotted with ad hoc visual inspection of data but can be potentially hard to identify with automated procedures.
Example 3
A common problem is the time zone of the time of deployment of the GPS sensor on animals. The time of deployment is often provided in local time, including light-saving time shifts, with no explicit reference to the time zone. Instead, the acquisition time of the GPS device is normally set to UTC by default, so this can result in a mismatched identification of the first animal relocations. Similarly, in some cases, data providers excluded the first part of the animal trajectory (for example, data were provided from the second week after release). These inconsistencies can be particularly problematic for studies focussing on post-capture-and-release behaviour. We always verify these potential issues with data owners during the quality assurance process.
Example 4
Many problems were identified by cross-checking the consistency between different types of information, for example animal capture history and the sensor deployment interval: sensors often resulted in having been deployed before the capture, or relocations were associated with an animal after its death.
Example 5
Erroneous GPS locations can be identified applying movement models parametrised with species-specific values. In particular, maximum speed constraints can automatically identify impossible spikes in the trajectories. In some cases, though, a further visual inspection pointed at movements that were unlikely although not impossible so that we discussed with data providers whether they were to be flagged as wrong, or acceptable instead. Similarly, relocations in bodies of water are unlikely, but not impossible, and a final decision on their reliability has to be taken together with data providers who have specific knowledge of the context.
To face these and many other similar problems, we developed a protocol for quality checking based on the following principles:
1. data are requested from data providers in raw format generated by sensors;
2. quality checks are run both using automated procedures and expert-based assessments by trained data curators: this is time consuming, but key for assuring a high-quality level;
3. data providers are engaged in the quality checks in an iterative process: once identified, errors, inconsistencies and missing information can be fixed only by those who collected the data;
4. data are requested with a certain level of redundancy to allow cross-checks;
5. data considered erroneous or suspicious are never removed from the data set, instead are flagged with codes: when data are queried from the database, the code selected indicates the uncertainty embedded in the data.
The application of these principles have a cost in terms of personnel time (especially Point 2), but the process guarantees more robust and reliable scientific outputs, and improved quality of the original data sets, so benefitting the data providers.
It is important to stress that whatever the data screening procedures, not all errors can be detected, especially for internally consistent data sets without clear anomalies.
Common Understand of Objects and Vocabulary
In bio-logging studies, where the sensor is “animal-borne” (attached to animals), the abstraction of the empirical context into basic formal objects is pretty simple (Figure 4): a sensor is deployed on an animal that records (and often transmits) data related to the position, the movement, the physiology or the external environment of the animal (Urbano et al., 2010).
Nevertheless, we found that it was a challenge to formalise this model [i.e., defining the various objects, their attributes, relationships and the constraints governing the integrity of the elements in the model (Chamanara and König-Ries, 2014)] so that all partners had the same understanding and thus provided comparable data. This is clearly illustrated by the example described in Box 1.
Box 1. A deployment tale.
When the first Euromammals database was created and data collection from the network partners started, we initially asked data recorded by the sensor during the “monitoring period” of the animal by a “collar” (or “tag”), assuming that it was a clear, robust and unambiguous concept. Soon after, we realised that “monitoring” was not a correct term as we got some data sets with locations excluded from the data set but showing movement that seemed to be that of the animal. We investigated if this was an error of a wrong setting of the monitoring period, and the partner group replied that it was not: the collar was still on the animal but they stopped to monitor it. As we wanted all the available and valid locations of the animal, we had to better specify the request. At the same time, we saw that the concept of collar (tag) was wrong. The collar was just the physical support for multiple sensors which are attached to it (typically, GPS, VHF and accelerometer sensors for Euromammals). Even if the tag is the same, the period of activity of each sensor can be different (for example, one can stop working while the others still provide valid data), so the start and end period had to refer to the sensor, not to the tag. The tag-deployment approach is used by other systems (Kranstauber et al., 2011) and these different models can potentially lead to inconsistencies if data and deployment information from multiple repositories are joined, calling for a community-based formalisation of the terms that describe the objects of study. We reformulated our definition of deployment as the “period when the sensor is attached to the animal.” Then, we noticed that many deployment intervals were much longer than the period covered by data. When we investigated, we discovered that, according to the definitions, many data providers used as end date the date of the collar drop-off or recovery, even if the battery discharged long before or the sensor stopped working because of malfunctioning. To accommodate this situation, we adapted the definition as “the period when the sensor is attached to the animal and records information.” This seemed to be a solid definition, but then we detected animals that remained for a long time in the same position at the end of the monitoring. We discovered that this was because data providers, following the definition that we gave, set the end of the deployment at the time when the collar, still working, was removed from the animal even if the animal died days before. We modified the definition as “the period when the sensor is attached to the animal alive and records information.” We thought this was a robust definition, until we received a data set of GPS data from animals that were captured and collared in a country then moved and released in another country, including all the locations taken during the relocation. This is our current definition of deployment: “the period when the sensor is attached to the animal alive and free ranging and records information.”
Even a baseline definition of the bio-logging workflow, such as the “sensor deployment,” or the reasons for the deployment to end (drop-off, animal’s death, etc.) had to undergo multiple revisions: this provides an idea of the complexity of the process that has to accommodate situations that are hard to be a priori identified given the variety of environmental conditions, projects’ goals, and technological options.
The study area is an additional object, the definition of which is considered intuitive, but is instead very hard to formalise beyond users’ subjective indications. The subject of most bio-logging projects are individual animals. Therefore we formulated a sampled animal-based (hence dynamic) definition, i.e., the union of all the minimum convex polygons of the locations of each animal, plus a buffer of 1 km.
These issues might seem obvious and easily addressed applying general principles of Ecoinformatics for consistent and robust data management (Michener and Jones, 2012; Recknagel and Michener, 2017), but they only emerged with the need of formalisation to reuse and share the data in the collaborative context of Euromammals. The bio-logging community is already working on the definition of a community-controlled vocabulary and set of data and metadata standards (Campbell et al., 2016; Sequeira et al., 2021), trying to extend existing resources [e.g., Darwin Core (Wieczorek et al., 2012); Ecological Metadata Language (Jones et al., 2019)]. The use by the community of such standards for data and formats based on an agreed semantic description of the wildlife monitoring objects and events (Chamanara and König-Ries, 2014) is key for effective combination of data from different sources.
Extending Bio-Logging Data With Data Sets on Animals and Their Context
Euromammals started as a shared repository of telemetry data (i.e., VHF relocations, and GPS and accelerometer sensors) associated with some individual traits of the tagged animals (e.g., age at capture, sex), details about the sensors, and the study area. These data sets were then integrated with environmental covariates derived from remote sensing (see Section “Integration of Remote Sensing Products”). Soon, this information was revealed as insufficient to use movement data for process-based (rather than patter only based) analysis. Additional information on the individual and its context, including human activities affecting the animals, was essential to answer the biological questions that the network was addressing. Data providers were requested to also share information on animal mortality, captures, hunting regimes, and study area characteristics (not derivable from remote sensing), among others. Such information, initially added to the database as metadata of the bio-logging data, finally contributed to stand-alone data sets, spurring research questions and analysis (e.g., capture-mark-recapture analysis in case of capture and mortality data). The integration of bio-logging and ancillary data created a more complex but richer information base that enabled and improved the formulation of scientific questions, the interpretation of the results of the analysis and opened up new frontiers. Instead of seeing animals as moving objects, the database offered scenarios of individuals with their life-history interacting with the environment under defined pressures.
In addition to environmental and individual information, the integration of data recorded by different devices for animal monitoring can open up new research perspectives. Particularly, in the context of Euromammals, camera trapping data became so relevant that a dedicated cross-network shared database was established.
We acknowledge that the additional information that can be integrated with bio-logging data largely depends on the species-specific traits and type of research and cannot be generalised. While the species-specific networks of Euromammals were an ideal context to develop such integration, repositories with a more general taxonomic target would not be suitable for ad hoc database expansion like the ones described.
From Data to Outputs: Pro-active Involvement of Data Providers in the Whole Scientific Cycle
The richness and complexity of the database reflects the knowledge of the researchers who collected the data. This is fundamental both for the formulation of research questions and for a well-informed interpretation of results. In Euromammals we have verified how the involvement of members in the full process, from data collation, to quality-check, and pro-active involvement in the formulation and development of research goals and analysis has assured the quality and reliability of the outputs. Often, the knowledge of the local system and context, difficult to formalise in data and metadata (but see Section “Extending Bio-logging Data With Data Sets on Animals and Their Context”), has fundamentally helped to identify artifacts in the analysis outputs, or contributed indications on relevant explanatory variables.
Our experience suggests that the involvement of those who collected the data in large, longitudinal projects is important not only to valorise the contribution of data collection and stimulate data sharing, but above all to improve the quality and robustness of analyses and their interpretation.
Training in Data Management for All Practitioners Providing Data
The involvement of scientists in data management has evolved through time. Whereas before a certain disconnect was common (Lynch, 2008), nowadays, the trend of ecological information toward “Big data” makes data management a necessary skill for spatial ecologists, as it has already happened with statistics and GIS (Urbano et al., 2010). The quantity, frequency of acquisition and complexity of data generated by bio-logging projects require a software architecture that cannot be based anymore on spreadsheets or flat files. More advanced data management systems based on structured databases must be handled by ecologists for the data to be fully exploited and not wasted or, worse, misused (Urbano and Cagnacci, 2014).
Within Euromammals, the knowledge of basic principles of data management by those who collect and share data was important to increase the data quality and optimise their use once stored in the database. To use the Euromammals database, at least basic knowledge of the language for interacting with relational databases (SQL) is needed. Users can either query the database directly, or ask for data curators’ support to query and export data according to the analysis requirements, usually in a simple and easy to manage format (comma separated values, CSV files). Although some basic training is needed, users have appreciated the database potential for large data sets processing and integration with analysis tools (e.g., R, R Core Team, 2021). In this aspect, Euromammals is a privileged laboratory because smaller groups initially without specific data management skills have benefitted from support and training by data management experts within the network. One of the key activities of the network has been the promotion of periodic summer schools in data management (in 2012, 2015, and 2018) open to all but especially targetting Euromammals members.
Another very important aspect of creating and consolidating data management skills among the repository users is to convey the concept that what drives the form in which data is organised in a repository is not necessarily the form in which the data will then be viewed and analysed, i.e., separating the logic of using data from the logic of organising it. This is a key element to organise the data in the right format without inconsistencies across multiple data sets, whereas when stored locally data are often adjusted to meet specific needs, rather than letting this be done by operations within a relational database. This approach has a positive impact also on the standardisation of the data when recorded in the field, as how they will be stored and used is accounted for in the collection protocols (Petters et al., 2019).
The more researchers who collect and process data are familiar with data management, the higher the quality of the data shared will be. In general, every data collection should a priori be based on a good data management plan (Michener, 2015) that ensures an optimised data workflow (Hackett et al., 2019). In this sense, large training initiatives, such as Data Carpentry9 and CODATA10 are also important opportunities.
Data First, Then and Only Then the Interfaces
Within Euromammals, the priority has always been to collect and harmonise the data and provide them ready for analysis by the researchers of the network. For this reason, all available resources have been used to improve data quality and gather and process more data. It has not been in the goals of Euromammals to create Graphical User Interfaces (GUIs) for data upload and query, rather we relied on expert knowledge and existing tools for these tasks (see Section “Training in Data Management for All Practitioners Providing Data”), and invested in interoperability. This approach has always paid off and ensured that the project could last more than 15 years independently from software obsolescence.
Our experience is that the most critical step is not the e-infrastructure to host, visualise and disseminate data, but the creation of connections and trust inside the scientific community. This can only be achieved in the medium and long term and once established can easily adjust to more technical requirements with limited and focussed investments. We think that this perspective should be considered carefully by agencies that offer funding opportunities that are often supporting development of data sharing software platforms rather than network establishment, data management training and data quality check and gathering.
Harmonisation of Data Collection Protocols
In the absence of shared protocols, data sharing is limited by the risk of pooling incoherent sources. Given the nature of the Euromammals networks, in which the majority of researchers working on a given species actively participate, it was possible to harmonise not only the collation of data, but also the data collection protocols, i.e., in the field. For example, most movement analysis rely on regular trajectories (Calenge et al., 2009), so that when data sets from different sources are used, trajectories have to be subsetted, or regularised. In Euromammals, members have agreed upon a minimum species-specific frequency for GPS location acquisition, so that trajectories can be easily collated before analysis.
Integration of Remote Sensing Products
Remote sensing is a key data source to contextualise animal movement and behaviour in species’ habitat. Since the start of the project and with the support of the University of Natural Resources and Life Sciences, BOKU, Austria (Vuolo et al., 2012; Atzberger et al., 2013), the Euromammals spatial databases integrated many remote sensing products with pan-european coverage, updating the annotation of animal movement data with more recent products as they became available, and progressively dismissing the older ones, such as the Copernicus products (European Union, 2021). Corine Land Cover, Digital Elevation Model, Forest Density; the NASA MODIS-derived NDVI (Didan, 2015), and snow cover (Hall et al., 2006); CGIAR-DEM/SRTM1 (Jarvis et al., 2008) and ASTER-DEM (Hirano et al., 2003), among the others. The opportunities (and challenges) in the use of remote sensing for animal movement studies are quickly evolving with the availability of new sensors in both domains. In particular, the European Sentinel-1 and Sentinel-2 satellites can potentially match the spatial and temporal scale of animal movement studies for a large set of species (terrestrial, marine and avian), with high resolution time series. Nowadays, the increasing amount and spatio-temporal resolution of available remote sensing data is making the annotation of movement data by downloading the products and carrying out a local spatio-temporal join outdated and impractical (Dodge et al., 2013). Euromammals is now moving from an internal acquisition and processing approach to the connection with cloud-based infrastructures like Google Earth Engine (Gorelick et al., 2017) and the emerging European Copernicus Data and Information Access Services (DIAS).
The sensitive point in remote sensing data is its appropriate use. Users without a specific remote sensing background tend to rely on remote sensing data in their animal ecology research without a proper assessment of the characteristics and limits of such products. Using the nominal spatial and temporal resolution as the only criteria to select the best remote sensing product, assuming they provide an error-free and homogeneous picture of the earth’s surface are common pitfalls when annotating animal movement data. As no clear rule can be set a priori to identify the best remote sensing products and their limits, results must be correctly interpreted case by case. The recommendation is to inform users of the characteristics of the products and possibly involve remote sensing experts in the study.
Data Gathering and Engagement of Contributors
A bottom-up, collaborative initiative like Euromammals can rely on the open, participatory attitude of members. However, as members mainly offer in-kind contribution of their time, certain phases in the integration of different data sets inevitably took a substantial amount of time, especially the phase of data preparation, for data checking and documenting, or the gathering of ancillary, non-sensor based standardised information (e.g., population level characteristics). The technical assistance by a database manager and data curators has proved essential to assure the effectiveness of these steps.
Further to technical help, researchers are motivated to provide data and prioritise the initiative’s activities if they feel well-informed and proactively involved, thus developing trust and a sense of belonging to the network that overcomes the perplexities raised by some researchers about possible misuse of shared data (Mills et al., 2015, 2016). In Euromammals, writing joint papers proposed by the community itself has been the signature trait of the initiative, and has fuelled the engine of the network (see Table 1). For this to be realistic, a scientific coordinator recognised as an integral part of the community kept the overview of the scientific goals and engaged the participants to achieve them.
Long Term Sustainability
Euromammals is a bottom-up initiative also from a financial point of view: there is no grant that financially has supported its creation and maintenance. After an initial investment of about 100KEuro from one of the founding members stemming from institutional activities, the project has supported the technical needs (i.e., database development, maintenance and management, and partly data curatorship) through sponsorship from a private company, and ad hoc contributions from the partners. This constitutes an approximate annual investment of 25k Euro. To this, a large amount of in-kind time from several members of the network engaging in the management tasks (often Ph.D. students and postdocs) and from the IT support of the institution hosting the database has to be added and proved essential for the sustainability of the project.
For more than a decade, this model has worked and ensured the sustainability of Euromammals even though no stable funding has been provided. However, this system has pros and cons. On the one hand, it has ensured that the initiative was not limited by the temporal horizon of a grant (that rarely goes beyond 3–5 years) and by the requests of funding agencies that are necessarily constrained to specific objectives, so that the researchers could be creative in proposing research topics and member institutions could seek for immediate responses to ad hoc issues. On the other hand, this system has reduced the speed of Euromammals development and finalisation of initiatives, because of limited dedicated resources. Additional funding would be important to add new features, increase the frequency of meetings, add more data sets per year into the databases, work more closely to the implementation of standards, but not necessarily to maintain the core structure, which is a guarantee of long term sustainability. Essentially, Euromammals always had more data and initiatives proposed than those that could actually be processed.
In our experience, the significant and durable scientific return of a collaborative project is not only due to the e-infrastructure and software development, which can be achieved with substantial financial support in the short term, but rather to the establishment of trust in the network which in turn is the expression of a scientific community based on community trust. This requires moderate investment distributed over time, with a long-term perspective.
Discussion
The value and impact of scientific data increase when all researchers within a community are able to share knowledge and interact with one another (Michener and Jones, 2012; Chamanara and König-Ries, 2014), and data sharing initiatives are invaluable opportunities to this end. In this manuscript, we report the main lessons that we have learnt in the 15 years of the Euromammals project for collaborative science in animal spatial ecology. In particular, re-using data to scale up from single study areas to a broader range of habitat conditions allows to address more generalisable scientific goals and support decision making (Applegate, 2015). Conversely, it requires that data are harmonised, managed and stored beyond the scope they are collected for. These are the premises that since 2007 pushed an increasing number of European institutes and organisations studying or managing terrestrial mammals to join Euromammals. The species-specific Euromammals scientific networks have created, populated and managed shared databases that are a fertile laboratory to identify challenges and opportunities in collaborative science that we have summarised here. The establishment of a collaborative network was achieved through the involvement of those who collected the data in all stages, from data quality checks, to financial support and production of scientific outputs. We had to adapt our approach to data management multiple times to take into account issues and opportunities that arose as data types and network requirements evolved over time, so that a diffused, basic knowledge of data management was of great aid to the success of the initiative. These good practices and critical points can help address solutions that are currently being developed by larger standardisation and data sharing initiatives (Sequeira et al., 2021).
According to our experience, quality of shared data is an issue that is often overlooked, assuming that formal and automated controls are sufficient to provide reliable information. Once published in a repository, though, issues may remain hidden to final users. We recommend putting in place more active, controlled and scrupulous review before data are made available in shared repositories. In our case, an intermediate step of data sharing closer to the specific scientific community, before sharing the data into generalist repositories represented an incredible added value to obtain better science-based information. In Euromammals, an insufficiently formalised definition of monitoring events or procedures was observed as a common reason for data inconsistency or incompleteness, even when recorded from bio-logging sources. In particular, to be fully effective, we realised that standardisation should start from data collection protocols. Ancillary, complementary data of individual life- and capture-history, cause of death, sensor retrieval and malfunctioning, as well as on population-level general descriptors (presence of competing species, hunting regime, intensity of tourism, presence of artificial feeding) also strongly contribute to a well-informed and robust use of bio-logging data sets. Such information is mostly species-specific, and difficult to generalise, but it can be refined as new questions or observations emerge from the users. Remote-sensing data are of great aid in obtaining standardised descriptors of the environmental context of the studied species, but should also be used coherently to their spatio-temporal resolution and potential sources of bias.
From a technical point of view, there is an urgent need for movement ecology to move forward in defining common data collection protocols, data and metadata standards, and shared vocabularies. The community should be involved, including smaller players, the so-called long tail of scientific research (Petters et al., 2019), so as to increase the consensus and engagement in their use of standards. Often, standards remain a topic for experts, limiting their impact and adoption and slowing down the acceptance of data sharing principles by part of the scientific community.
A direct involvement of those collecting the data in the analysis phase fosters the willingness to share data and improves the scientific outcomes. In general, the establishment of data sharing networks and repositories requires a long process to build trust in the communities. Conversely, the primary goal by funding entities remains the creation of e-infrastructures and software tools for data sharing and discovering. However, a moderate, but distributed investment over time is essential to engage the community and ensure sustainability after the e-infrastructure has been created.
No scientific community “is an island entire of itself but is a part of the main” (Donne, 1624): in the future Euromammals plans to connect with broader international initiatives that aim at setting cross-discipline guidelines, standards and tools for an integrated approach to data and knowledge sharing. In this regard, networks of networks like the European Open Science Cloud (EOSC)11 are a key element to maximise the impact of community based initiatives in science.
Whatever the model for managing the data sharing process, it is clear that there is no alternative to data sharing in the medium and long term in ecology and conservation biology to address the emerging global challenges.
Data Availability Statement
The original contributions presented in the study are included in the article/Supplementary Material, further inquiries can be directed to the corresponding author/s.
Author Contributions
All authors equally contributed to the article and approved the submitted version.
Conflict of Interest
The authors declare that the research was conducted in the absence of any commercial or financial relationships that could be construed as a potential conflict of interest.
Publisher’s Note
All claims expressed in this article are solely those of the authors and do not necessarily represent those of their affiliated organizations, or those of the publisher, the editors and the reviewers. Any product that may be evaluated in this article, or claim that may be made by its manufacturer, is not guaranteed or endorsed by the publisher.
Acknowledgments
Euromammals would not exist without the dedication, support, trust of each and every one among the hundreds of members. The authors are grateful to the scientific coordinators, to the data curators, to the IT technicians, to the sponsor and all members for making a little funded, bottom-up, and challenging idea into a large, productive and, above all, enjoyable and trustable scientific family. The authors are also grateful to Sarah Davidson and Meredith VanAcker who critically commented on earlier drafts of the manuscript.
Supplementary Material
The Supplementary Material for this article can be found online at: https://www.frontiersin.org/articles/10.3389/fevo.2021.727023/full#supplementary-material
Footnotes
- ^ https://eurodeer.org
- ^ https://eureddeer.org
- ^ https://euroboar.org
- ^ https://eurowildcat.org
- ^ https://eurolynx.org
- ^ https://euroibex.org
- ^ https://eurosmallmammals.org
- ^ https://github.com/feurbano/eurodeer_db
- ^ https://datacarpentry.org/
- ^ https://codata.org/
- ^ https://eosc-portal.eu/
References
Andersen, R., Duncan, P., and Linne, J. D. C. (1998). The European Roe Deer: The Biology Of Success. Oslo: Scandinavian university press.
Applegate, R. D. (2015). The importance of data management in wildlife conservation. Wildl. Soc. Bull. 39, 449–450. doi: 10.1002/wsb.570
Atzberger, C., Klisch, A., Mattiuzzi, M., and Vuolo, F. (2013). Phenological metrics derived over the European continent from NDVI data and MODIS time series. Remote Sens. 6, 257–284. doi: 10.3390/rs6010257
Bastianelli, M. L., Premier, J., Herrmann, M., Anile, S., Monterroso, P., Kuemmerle, T., et al. (2021). Survival and cause-specific mortality of European wildcat (Felis silvestris) across Europe. Biol. Conserv. 261:109239. doi: 10.1016/j.biocon.2021.109239
Bonnot, N., Couriot, O., Berger, A., Cagnacci, F., Ciuti, S., De Groeve, J., et al. (2020). Fear of the dark? Contrasting impacts of humans versus lynx on diel activity of roe deer across Europe. J. Anim. Ecol. 89, 132–145. doi: 10.1111/1365-2656.13161
Bright Ross, J. G., Peters, W., Ossi, F., Moorcroft, P. R., Cordano, E., Eccel, E., et al. (2021). Climate change and anthropogenic food manipulation interact in shifting the distribution of a large herbivore at its altitudinal range limit. Sci. Rep. 11:7600. doi: 10.1038/s41598-021-86720-2
Cagnacci, F., and Urbano, F. (2008). Managing wildlife: a spatial information system for GPS collars data. Environ. Model. Softw. 23, 957–959. doi: 10.1016/j.envsoft.2008.01.003
Cagnacci, F., Boitani, L., Powell, R. A., and Boyce, M. S. (2010). Challenges and opportunities of using GPS-based location data in animal ecology. Philos. Trans. R. Soc. B 365:2155. doi: 10.1098/rstb.2010.0098
Cagnacci, F., Focardi, S., Ghisla, A., van Moorter, B., Merril, E., Gurarie, E., et al. (2016). How many routes lead to migration? Comparison of methods to assess and characterise migratory movements. J. Anim. Ecol. 85, 54–68. doi: 10.1111/1365-2656.12449
Cagnacci, F., Focardi, S., Hewison, A. J. M., Morellet, N., Heurich, M., Stache, A., et al. (2011). Partial migration in roe deer: migratory and resident tactics are end points of a behavioural gradient determined by ecological factors. OIKOS 120, 1790–1802. doi: 10.1111/j.1600-0706.2011.19441.x
Calenge, C., Dray, S., and Royer-Carenzi, M. (2009). The concept of animals’ trajectories from a data analysis perspective. Ecol. Inform. 4, 34–41. doi: 10.1016/j.ecoinf.2008.10.002
Campbell, H. A., Urbano, F., Davidson, S., Dettki, H., and Cagnacci, F. (2016). A plea for standards in reporting data collected by animal-borne electronic devices. Anim. Biotelemetry 4:1(2016). doi: 10.1186/s40317-015-0096-x
Chamanara, J., and König-Ries, B. (2014). A conceptual model for data management in the field of ecology. Ecol. Inform. 24, 261–272. doi: 10.1016/j.ecoinf.2013.12.003
Costello, M. J., Horton, T., and Kroh, A. (2018). Sustainable biodiversity databasing: international. collaborative, dynamic, centralised. Trends Ecol. Evol. 33, 803–805. doi: 10.1016/j.tree.2018.08.006
Couriot, O., Hewison, A. J. M., Said, S., Cagnacci, F., Chamaillé-Jammes, S., Linnel, J. D. C., et al. (2018). Truly sedentary? The multi-range tactic as a response to resource heterogeneity and unpredictability in a large herbivore. Oecologia 187, 47–60. doi: 10.1007/s00442-018-4131-5
De Groeve, J., Cagnacci, F., Ranc, N., Bonnot, N. C., Gehr, B., Heurich, M., et al. (2019). Individual Movement-Sequence Analysis Method (IM-SAM): characterizing spatio-temporal patterns of animal habitat use across landscapes. Int. J. Geogr. Inf. Sci. 34, 1530–1551. doi: 10.1080/13658816.2019.1594822
Debeffe, L., Focardi, S., Bonenfant, C., Hewison, A. J. M., Morellet, N., Vanpé, C., et al. (2014). A one night stand? Reproductive excursions of female roe deer as a breeding dispersal tactic. Oecologia 176, 431–443. doi: 10.1007/s00442-014-3021-8
Didan, K. (2015). MOD13Q1 MODIS/Terra Vegetation Indices 16-Day L3 Global 250m SIN Grid V006. NASA EOSDIS Land Processes DAAC. doi: 10.5067/MODIS/MOD13Q1.006
Dodge, S., Bohrer, G., Weinzierl, R., Davidson, S., Kays, R., Douglas, D., et al. (2013). The environmental-data automated track annotation (Env-DATA) system: linking animal tracks with environmental data. Mov. Ecol. 1:3. doi: 10.1186/2051-3933-1-3
European Union (2021). Copernicus Land Monitoring Service. Denmark: European Environment Agency (EEA).
Frair, J. L., Fieberg, J., Hebblewhite, M., Cagnacci, F., DeCesare, N. J., and Pedrotti, L. (2010). Resolving issues of imprecise and habitat-biased locations in ecological analyses using GPS telemetry data. Philos. Trans. R. Soc. B Biol. Sci. 365, 2187–2200. doi: 10.1098/rstb.2010.0084
Gehr, B., Bonnot, N., Heurich, M., Cagnacci, F., Ciuti, S., Hewison, M., et al. (2020). Stay home, stay safe - site familiarity reduces predation risk in a large herbivore in two contrasting study sites. J. Anim. Ecol. 89, 1329–1339. doi: 10.1111/1365-2656.13202
Gewin, V. (2016). Data sharing: an open mind on open data. Nature 529, 117–119. doi: 10.1038/nj7584-117a
Gorelick, N., Hancher, M., Dixon, M., Ilyushchenko, S., Thau, D., and Moore, R. (2017). Google earth engine: planetary-scale geospatial analysis for everyone. Remote Sens. Environ. 202, 18–27. doi: 10.1016/j.rse.2017.06.031
Hackett, R. A., Belitz, M. W., Gilbert, E. E., and Monfils, A. K. (2019). A data management workflow of biodiversity data from the field to data users. Appl. Plant Sci. 7:e11310. doi: 10.1002/aps3.11310
Hall, D. K., Riggs, G. A., and Salomonson, V. V. (2006). MODIS/Terra Snow Cover 5-Min L2 Swath 500m. Boulder, CO: NASA National Snow and Ice Data Center Distributed Active Archive Center, doi: 10.5067/ACYTYZB9BEOS
Harcourt, R., Sequeira, A. M. M., Zhang, X., Roquet, F., Komatsu, K., Heupel, M., et al. (2019). Animal-borne telemetry: an integral component of the ocean observing toolkit. Front. Mar. Sci. 6:326. doi: 10.3389/fmars.2019.00326
Hays, G. C., Bailey, H., Bograd, S. J., Bowen, W. D., Campagna, C., Carmichael, R. H., et al. (2019). Translating marine animal tracking data into conservation policy and management. Trends Ecol. Evol. 34, 459–473. doi: 10.1016/j.tree.2019.01.009
Hewison, A. J. M., Gaillard, J. M., Morellet, N., Cagnacci, F., Debeffe, L., Cargnelutti, B., et al. (2021). Sex differences in condition dependence of natal dispersal in a large herbivore: dispersal propensity and distance are decoupled. Proc. Biol. Sci. 288:20202947. doi: 10.1098/rspb.2020.2947
Hirano, A., Welch, R., and Lang, H. (2003). Mapping from ASTER stereo image data:DEM validation and accuracy assessment. ISPRS J. Photogramm. Remote. Sens. 57, 356–370. doi: 10.1016/S0924-2716(02)00164-8
Hofman, M. P. G., Hayward, M. W., Heim, M., Marchand, P., Rolandsen, C. M., Mattisson, J., et al. (2019). Right on track? Performance of satellite telemetry in terrestrial wildlife research. PLoS One 14:e0216223. doi: 10.1371/journal.pone.0216223
Jarvis, A., Reuter, H. I, Nelson, A., and Guevara, E. (2008). Hole-filled seamless SRTM data V4, InternationalCentre for Tropical Agriculture (CIAT). Cali: International Center for Tropical Agriculture.
Jeltsch, F., Bonte, D., Pe′er, G., Reineking, B., Leimgruber, P., Balkenhol, N., et al. (2013). Integrating movement ecology with biodiversity research-exploring new avenues to address spatiotemporal biodiversity dynamics. Mov. Ecol. 1:6. doi: 10.1186/2051-3933-1-6
Jones, M. B., O′Brien, M., Mecum, B., Boettiger, C., Schildhauer, M., Maier, M., et al. (2019). Ecological Metadata Language version 2.2.0. KNB Data Repository. doi: 10.5063/F11834T2
Kays, R., Crofoot, M. C., Jetz, W., and Wikelski, M. (2015). Terrestrial animal tracking as an eye on life and planet. Science 348:aaa2478. doi: 10.1126/science.aaa2478
Kranstauber, B., Cameron, A., Weinzerl, R., Fountain, T., Tilak, S., Wikelski, M., et al. (2011). The Movebank data model for animal tracking. Environ. Model. Softw. 26, 834–835. doi: 10.1016/j.envsoft.2010.12.005
Leigh, P. (2005). The ecological crisis, the human condition, and community-based restoration as an instrument for its cure. Ethics Sci. Environ. Polit. 5, 3–15. doi: 10.3354/esep005003
Lin, D., Crabtree, J., Dillo, I., Downs, R. R., Edmunds, R., Giaretta, D., et al. (2020). The TRUST Principles for digital repositories. Sci. Data 7:144. doi: 10.1038/s41597-020-0486-7
Michener, W. K. (2015). Ten simple rules for creating a good data management plan. PLoS Comput. Biol. 11:e1004525. doi: 10.1371/journal.pcbi.1004525
Michener, W. K., and Jones, M. B. (2012). Ecoinformatics: supporting ecology as a data-intensive science. Trends Ecol. Evol. 27, 85–93. doi: 10.1016/j.tree.2011.11.016
Mills, J. A., Teplitsky, C., Arroyo, B., Charmantier, A., Becker, P. H., Birkhead, T. R., et al. (2015). Archiving primary data: solutions for long-term studies. Trends Ecol. Evol. 30, 581–589. doi: 10.1016/j.tree.2015.07.006
Mills, J. A., Teplitsky, C., Arroyo, B., Charmantier, A., Becker, P. H., Birkhead, T. R., et al. (2016). Solutions for archiving data in long-term studies: a reply to whitlock et al. Trends Ecol. Evol. 31, 85–87. doi: 10.1016/j.tree.2015.12.004
Morales, J. M., Moorcroft, P. R., Matthiopoulos, J., Frair, J. L., Kie, J. G., Powell, R. A., et al. (2010). Building the bridge between animal movement and population dynamics. Philos. Trans. R. Soc. B 365, 2289–2301. doi: 10.1098/rstb.2010.0082
Morellet, N., Bonenfant, C., Börger, L., Ossi, F., Cagnacci, F., Heurich, M., et al. (2013). Seasonality, weather, and climate affect home range size in roe deer across a wide latitudinal gradient within Europe. J. Anim. Ecol. 82, 1326–1339. doi: 10.1111/1365-2656.12105
Ossi, F., Gaillard, J. M., Hebblewhite, M., Morellet, N., Ranc, N., Sandfort, R., et al. (2016). Plastic response by a small cervid to ad-libitum supplemental feeding in winter across a wide environmental gradient. Ecosphere 8:e01629. doi: 10.1002/ecs2.1629
Ossi, F., Urbano, F., and Cagnacci, F. (2019). “Biologging and remote-sensing of behavior,” in Encyclopedia of Animal Behavior, ed. J. Chun Choe (Cambridge, MA: Elsevier, Academic Press), doi: 10.1016/B978-0-12-809633-8.90089-X
Peters, W., Hebblewhite, M., Mysterud, A., Eacker, D., Hewison, M., Focardi, S., et al. (2019). Large herbivores migration plasticity along wide environmental gradients in Europe: life-history traits modulate forage effect. Oikos 128, 416–429. doi: 10.1111/oik.05588
Peters, W., Hebblewhite, M., Mysterud, A., Spitz, D., Focardi, S., Urbano, F., et al. (2017). Migration in geographic and ecological space by a large herbivore. Ecol. Monogr. 87, 297–320. doi: 10.1002/ecm.1250
Petters, J. L., Brooks, G. C., Smith, J. A., and Haas, C. A. (2019). The impact of targeted data management training for field research projects-A case study. Data Sci. J. 18:43. doi: 10.5334/dsj-2019-043
R Core Team (2021). R: A language and environment for statistical computing. R Foundation for Statistical Computing. Vienna. URL: https://www.R-project.org/ (accessed June 1, 2021).
Recknagel, F., and Michener, W. K. (2017). Ecological Informatics: Data Management And Knowledge Discovery: Third Edition New York, NY: Springer, doi: 10.1007/978-3-319-59928-1
Rutz, C., Loretto, M. C., Bates, A. E., Davidson, S., Duarte, C. M., Jetz, W., et al. (2020). COVID-19 lockdown allows researchers to quantify the effects of human activity on wildlife. Nat. Ecol. Evol. 4, 1156–1159. doi: 10.1038/s41559-020-1237-z
Sequeira, A. M. M., O′Toole, M., Keates, T. R., McDonnell, L. H., Braun, C. D., Hoenner, X., et al. (2021). A standardization framework for bio-logging data to advance ecological research and conservation. Methods Ecol. Evol. 12, 996–1007. doi: 10.1111/2041-210X.13593
Small, C., Pozzi, F., and Elvidge, C. D. (2005). Spatial analysis of global urban extent from DMSP-OLS nighttime lights. Remote Sens. Environ. 96, 277–291. doi: 10.1016/j.rse.2005.02.002
Smouse, P. E., Focardi, S., Moorcroft, P. R., Kie, J. G., Forester, J. D., and Morales, J. M. (2010). Stochastic modelling of animal movement. Philos. Trans. R. Soc. B 365, 2201–2211. doi: 10.1098/rstb.2010.0078
Urbano, F., and Cagnacci, F. (2014). Spatial Database for GPS Wildlife Tracking Data. Cham: Springer International Publishing, doi: 10.1007/978-3-319-03743-1
Urbano, F., Cagnacci, F., Calenge, C., Dettki, H., Cameron, A., and Neteler, M. (2010). Wildlife tracking data management: a new vision. Philos. Trans. R. Soc. B Biol. Sci. 365, 2177–2185. doi: 10.1098/rstb.2010.0081
Venter, O., Sanderson, E., Magrach, A., Allan, J. R., Beher, J., Jones, K. R., et al. (2016). Global terrestrial human footprint maps for 1993 and 2009. Sci. Data 3:160067. doi: 10.1038/sdata.2016.67
Vogt, P., Riitters, K. H., Estreguil, C., Kozak, J., Wade, T. G., and Wickham, J. D. (2007). Mapping spatial patterns with morphological image processing. Landsc. Ecol. 22, 171–177. doi: 10.1007/s10980-006-9013-2
Vuolo, F., Mattiuzzi, M., Klisch, A., and Atzberger, C. (2012). “Data service platform for MODIS vegetation indices time series processing at BOKU Vienna: Current status and future perspectives,” in Proceedings of the SPIE 8538, Earth Resources and Environmental Remote Sensing/GIS Applications III, 85380A, October 25, 2012, Edinburgh, United Kingdom. doi: 10.1117/12.974857
Wieczorek, J., Bloom, D., Guralnick, R., Blum, S., Döring, M., Giovanni, R., et al. (2012). Darwin core: an evolving community-developed biodiversity data standard. PLoS One 7:e29715. doi: 10.1371/journal.pone.0029715
Keywords: database, Data curation, wildlife monitoring, terrestrial ecology, European mammals, data sharing, bio-logging, Ecoinformatics
Citation: Urbano F and Cagnacci F (2021) Data Management and Sharing for Collaborative Science: Lessons Learnt From the Euromammals Initiative. Front. Ecol. Evol. 9:727023. doi: 10.3389/fevo.2021.727023
Received: 17 June 2021; Accepted: 10 August 2021;
Published: 10 September 2021.
Edited by:
Max A. Alekseyev, George Washington University, United StatesReviewed by:
Serge Morand, Centre National de la Recherche Scientifique (CNRS), FranceEmma Louise Burns, Australian National University, Australia
Copyright © 2021 Urbano and Cagnacci. This is an open-access article distributed under the terms of the Creative Commons Attribution License (CC BY). The use, distribution or reproduction in other forums is permitted, provided the original author(s) and the copyright owner(s) are credited and that the original publication in this journal is cited, in accordance with accepted academic practice. No use, distribution or reproduction is permitted which does not comply with these terms.
*Correspondence: Ferdinando Urbano, ZmVyZGluYW5kby51cmJhbm9AZWMuZXVyb3BhLmV1
†See Supplementary Table 1 for the complete list of Euromammals partners