- Department of Animal Ecology and Conservation Biology, Wildlife Institute of India, Dehradun, India
The wolves in the Hindukush–Himalayan region belong to one of the most basal lineages of Canis lupus, yet little is known about their ecology and behavior. In this study, we determine wolf movement and analyze diet patterns in contrast with studies from within its distribution range. We determine conflict perception and identify hotspots using ecological, social, and remotely sensed information. Wolf diet (n = 283 scats) constituted mostly of domestic prey (79%), while wild prey constituted 17.8% of the wolf diet. Interview-based questionnaire surveys revealed that 55% of the respondents claimed to have seen wolves. Over 98% of the respondents claimed wolves as a possible threat to various livestock in the study area. Marginal response curves through the MaxEnt model showed that wolf hotspots were positive in response to their density of location, landuse-landcover, village population, village density, and depredation. Comparisons between scat- and questionnaire-based depredation data showed that the perceived levels of depredation by wolves differed significantly from the actual proportion of livestock prey species consumed by wolves (χ2 = 99.64, p-value < 0.0001). Wolf conflict hotspots showed a very high conflict zone area of 36 km2, high conflict zone of 62 km2, medium conflict zone of 196 km2, and low conflict zone of 3,636 km2. Future conflict mitigation strategies may focus on such areas primarily to reduce livestock losses and enhance conservation outcomes. Negative perceptions toward wolves can be managed through a holistic conservation action plan in concert with the existing snow leopard conservation program alongside local traditions that do not hinder livelihood security.
Introduction
In the Indian sub-continent, wolves are represented by two geographically isolated broadly allopatric populations (Habib, 2007). One population of wolves extends from the upper Hindukush–Himalayan region of India across the two northernmost states of Himachal Pradesh and Ladakh (Fox and Chundawat, 1995). This wolf population is well adapted to the cold environment and is known as the Himalayan wolf, Canis lupus chanco (Shrotriya et al., 2012; Joshi et al., 2020; Lyngdoh et al., 2020). Found in the arid and hypoxic high-altitude ecosystems of the Himalayas and the Tibetan Plateau, extending into China, Manchuria, and Mongolia these “woolly” wolves descended from a unique ancient lineage (Zhang et al., 2014; Werhahn et al., 2020). Most studies agree though that the Himalayan wolf is the basal taxon within the phylogeny of the wolf and wolf-dog clades (Werhahn et al., 2017, 2020; Joshi et al., 2020). In terms of their ecological role, population, and behavior, Himalayan wolves are probably one of the least known wolf subspecies (Fox and Chundawat, 1995).
Although wolves originally lived almost throughout North America and Eurasia, negative attitudes against wolves throughout the world allowed them to be viewed as a threat to personal safety and an impediment to progress and civilization (Kellert et al., 1996). Wolf depredation of stock convinced government-aided “legal” extirpations across many areas in Central Asia, Europe, United States, and Canada (Proulx and Rodtka, 2015). Subsequently, wolves became absent in many areas, for example, in 1966, the wolf was declared functionally extinct in the Scandinavian Peninsula (Wabakken et al., 2001). Wolves were almost systematically exterminated until 1940 in the United States of America by government-backed programs since the 19th century (Hesse, 2020). Nearly, all mortalities in Scandinavia, Italy, Germany, and England were reported from poaching or vehicle strikes (Mech, 2017). Despite long-standing persecution, it is noteworthy that in recent times, with improved legal and management scenarios, many large carnivores including wolves have been documented to be recolonizing parts of their former ranges in Europe (Chapron, 2005; Nowak et al., 2011).
In the Asian context as well, a major cause of Human-Wolf conflict has been livestock depredation (Bagchi and Mishra, 2006; Shrotriya et al., 2012; Ghoshal et al., 2018; Lyngdoh et al., 2020). The unavailability of wild prey and the decline of natural habitats have driven wolves to subsist mainly on livestock and life outside protected areas which makes them prone to conflict, social dislike, and retaliation (Jhala, 1991; Jethva and Jhala, 2004; Lyngdoh et al., 2020). Wolf conflict may exist despite wild prey availability (Suryawanshi et al., 2017). In India, however, attitudes toward wolves have been less destructive (Bagchi and Mishra, 2006; Namgail, 2007). Today, despite complex human-wolf associations, in many Indian landscapes, they continue to survive despite disturbance and other human-induced factors such as land-use changes, habitat loss, and urbanization (Habib and Kumar, 2007). However, no matter how great the tolerance, persecution remains as one of the biggest obstacles to wolf recovery (Jhala, 1991; Newsome et al., 2016), including the Himalayan wolf (Bagchi and Mishra, 2006; Suryawanshi et al., 2013) whose genetic singularity and small population make its more vulnerable to extinction (Blandford, 1988; Aggarwal et al., 2003; Shrotriya et al., 2012; Joshi et al., 2020). Apart from such threats, feral dogs also negatively impact wolf conservation efforts (Lescureux and Linnell, 2014; Hennelly et al., 2015). Although such threats are well known, their ecological attributes and the environmental characteristics of the areas where they occur are limited (Ugarte et al., 2019). Thus, studies on the actual damage caused by wildlife species that can tease apart the actual damage and perceived damage are crucial for conflict mitigation. They may offer a nuanced approach by taking into account the behavior and ecology of the species concerned (Anand and Radhakrishna, 2017).
The current study, therefore, aims to understand the ecological attributes of human-wolf conflict through the use of telemetry, scatological, and distribution modeling techniques (Mech and Boitani, 2003; Monterroso et al., 2011; Bassi et al., 2015). The human-wolf conflict-co-existence scenario in the Spiti subdivision (India) may be considered representative of the situation in the Himalayas. We (a) investigate dietary choices of Himalayan wolves; (b) delineate wolf conflict hotspots in the scope of its movement, diet, and public perception; and (c) describe prevailing perceptions toward large carnivores in the socio-cultural context of the study area and compare it to other areas in the Trans-Himalayan landscapes. We determine whether prey consumption information alone through scats can serve as reliable surrogates for modeling wolf conflict hotspots. It is expected that wolves will be in conflict within human use areas that most closely match their own ecological needs in terms of food and space. We therefore examine if and what such attributes are by modeling human-wolf conflict.
Study area and methods
The Spiti catchment area (Figure 1) which is divided by the Spiti River is a cold semi-arid region of Himachal Pradesh. The Greater Himalayas are to the south of the valley while to the north of it is Ladakh and east lies Tibet. The study area includes two protected areas of Kibber Wildlife Sanctuary (WLS) (32°5 to 32°30 N and 78°1 to 78°32E) and Pin Valley National Park (NP) in the southwest corner of the Spiti Catchment area. It is a mountainous cold desert, where altitudes range between 3,600 and 6,700 m above mean sea level. About 3,800 km2 of the area is being managed as Upper Spiti Landscape under the National Scheme of Project Snow Leopard. Temperatures range between −23 and 3°C in winter and between 1 and 28°C in summer. The vegetation of this area has been broadly classified as dry alpine steppe (Champion and Seth, 1968).
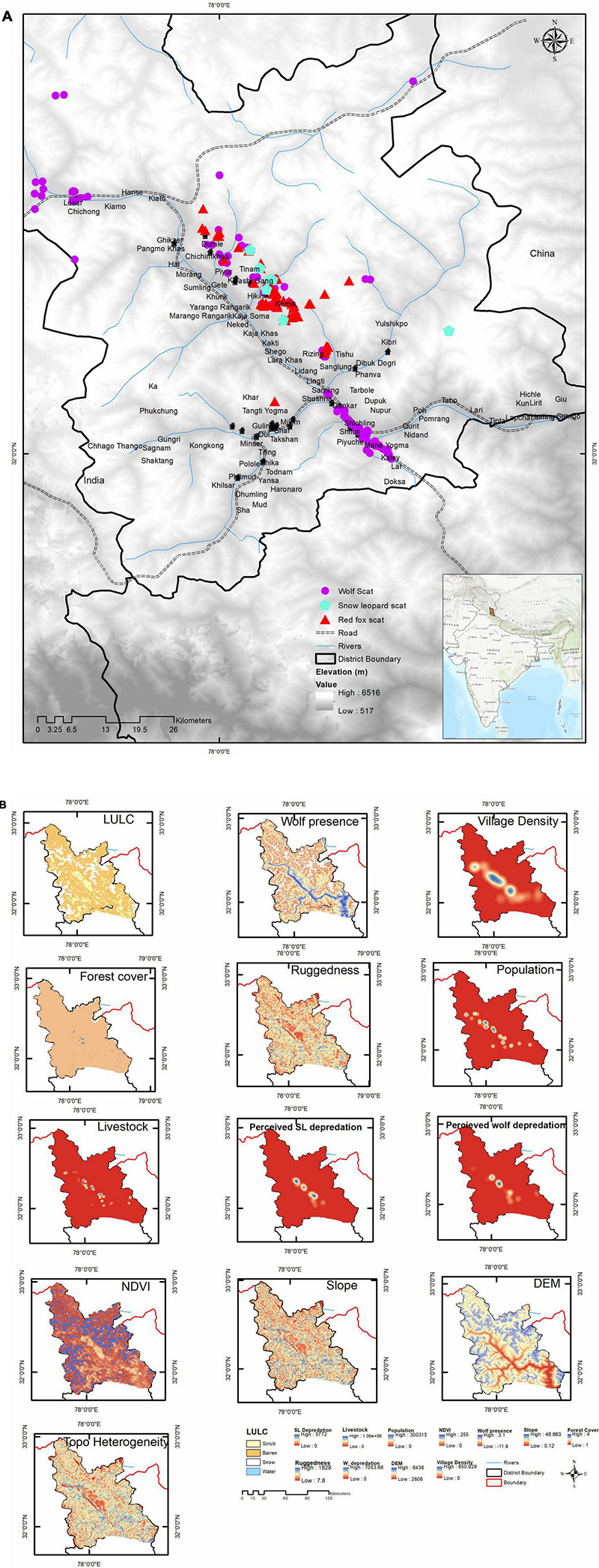
Figure 1. (A) Study area depicting Spiti Valley with locations of scats and villages. (B) Layers used in modeling wolf conflict hotspot in MaxEnt. A total of 11 covariate layers were used. Perceived snow leopard depredation and topographic heterogeneity were dropped however included in this composite figure. Higher values are indicated by blue.
The large mammalian fauna of the area includes bharal (Pseudois nayaur), relatively few ibex (Capra sibirica), and their predators, namely the snow leopard (Panthera uncia) and the wolf. Other fauna includes the red fox (Vulpes vulpes), Altai weasel (Mustela altaica), stone marten (Martes foina), and the Himalayan mouse hare (Ochotona roylei). Nearly 45 species of birds were identified, including several species typical of this alpine habitat, such as chukar (Alectoris chukar) and Tibetan snowcock (Tetraogallus tibetanus) (Mishra, 1997; Lyngdoh et al., 2014b,2020).
The local agro-pastoral community also known as Spitian Bhot or Bodh, largely Buddhist, is concentrated in village clusters largely along the main Spiti River (Bhasin et al., 1983). There are approximately 60 villages throughout the length and breadth of the valley with a population of 12,445 with a density of 2 person km–2 (Government of Himachal Pradesh, 2021). Most agriculture-related activities are restricted to the short growing season (from May to September). The main crops cultivated are barley (Hordeum vulgare) and green peas (Pisum sativum). Livestock includes goats and sheep, cattle, “dzomo” (a female hybrid of cattle and yak), and yaks. An indigenous breed of donkeys, a potential prey for large carnivores, Equus asinus, well adapted to their agroclimatic environment and ecology are also used as beasts of burden (Behl et al., 2017), while horses (Equus ferus caballus), apart from being used for transportation and religious ceremonies, are mainly raised for trade (Chauhan et al., 2004). Migratory and resident herders are present throughout the region. The study area (∼4,000 km2, Sharma et al., 2015) was conducted within the hospitable zone up to 5,200 m that includes villages surrounding Pin valley NP and Kibber WLS within the Himalayan wolf’s altitudinal niche (Suryawanshi et al., 2013; Joshi et al., 2020).
Data collection
Village surveys
To determine livestock depredation and large carnivore presence, a semi-structured questionnaire (Figure 1A; Lyngdoh et al., 2014a) was conducted in 2015–2016 across 35 villages. The unit of sampling was considered to be a household. The questionnaire surveys were distributed systematically to represent most of the target villages in the study area within the altitudinal range of 3,000–4,300 m. A total of 200 households (adults older than 18 years) were surveyed, with a random selection of three to four households in each village. We conducted the questionnaire surveys in compliance with national ethical guidelines of conduct (IFSW, 2015). Verbal consent from the respondents and confidentiality of the individual responses have been maintained. The sighting of a wolf (shanku) was confirmed by the respondents through photographs of different species of canids. Subsequent details of their presence, sighting, and depredation including possible scavenging were recorded through this standard questionnaire (see also Supplementary Material 1). The questionnaire noted claims by respondents into various categories such as presence (yes/no) and visual confirmation through sighting or signs or depredation or scavenging (yes/no). Depredation events were noted in response to questions relating to the number of livestock lost due to a carnivore. Any ambiguity in the confirmation was not recorded or noted as unknown. The questions were also focused on household characteristics such as demography, income, grazing distance, livestock holding, and carnivore presence or depredation by other carnivores (see also Supplementary Material 1).
Scat collection and DNA verification of select scats
Sign surveys were conducted throughout the landscape with approximately ∼1,000 km tracked and 300 person-days of effort through post-winter and pre-winter surveys. Trails, riverbanks, hilltops, village periphery, and grazing pastures were searched on foot for signs of a large predator as well as other meso-predators. We collected scats (n = 283) of wolves and other predators over a period of 3 years (2014–2017). All scats collected were labeled with location and time references in Universal Transverse Mercator (WGS 84 / UTM zone 44N). Wolf scats were collected through field observations including traversing areas of regular movement trajectories of collared wolves in the landscape. Domestic dog scats could also be easily confused for wolf scats; therefore, we collected scats from areas that were devoid of active dog presence. For example, areas that were too close to villages (i.e., 1–2 km radius) or human settlements would have a heavy dog presence as also confirmed through site visits during interviews. Furthermore, to confirm wolf scats and observer error, DNA extraction from only sampled scats (n = 118) was done by using a QIAamp stool DNA kit (QIAGEN, Germany) in a dedicated room to avoid contamination with some minor modifications (Joshi et al., 2020). We used only a random proportion of the total number of scats for DNA verification from the suspected 118 fecal samples that were collected. However, we used all suspected wolf scats (n = 283) for the verification of dietary habits with reasonable confidence through field validation, excluding scats that were very near to villages and below the size class of red fox scats (n = 127). We did not sequence fox scats that were easily distinguishable due to their small size and quantity; moreover, the diet of foxes is well known from the same area in a distinct study (Reshamwala et al., 2018). We targeted the 148 bp region of the mitochondrial Cytochrome b gene for identifying species using carnivore-specific primers (Farrell et al., 2000), and the scat samples (n = 118) were PCR amplified. All PCR reactions were performed using an Applied Biosystems thermal cycler (ABI, 2720) in a reaction volume of 10 μl containing 1 × PCR buffer, 2.5 mM of MgCl2, 200 μM of each d-NTP, 1.25 μg of BSA, 4 pM of each primer, 0.25 U of Taq DNA polymerase (Genie), and 1 μl of genomic DNA. The PCR cycling conditions were as follows: initial denaturation at 95°C for 3 min, followed by 32 cycles of denaturation at 94°C for 30 s., primer annealing at 55°C for 50 s. min, and primer extension at 72°C for 40 s. with a final extension at 72°C for 10 min. We tested diet consumption patterns between scat identified through genetic and non-genetic conformation using a t-test to check for any drastic differences in interpretation of diet. Amplified PCR products were cleaned up using the Exo-SAP treatment to remove residual oligonucleotides and dNTPs prior to DNA sequencing. Forward and reverse primers of the Cytochrome b gene were used for setting up the cycle sequencing reaction. Unbound ddNTPs were removed by using the alcoholic precipitation method and subjected for sequencing to ABI 3130 Genetic Analyzer (Applied Biosystems). Sequence qualities were determined using the Sequence Analysis v 5.2 software (Applied Biosystems) and validated by Sequencer v 4.7 software.1 All good quality sequences were compared with NCBI/GenBank2 database using the BLAST tool, and species were confirmed with most homologous sequences (100%) available from the NCBI database. Multiple sequence alignment (MSA) was performed using CLUSTAL was implemented in the BioEdit v 7.0.9.0 software (Hall, 1999).
Data on wolf food habits, availability, and statistical analysis
Scat samples were washed and examined under the microscope for medullary patterns to identify different prey species based on standard methods (Mukherjee et al., 2004; Lyngdoh et al., 2014b). Relative frequencies of occurrence (Lyngdoh et al., 2014b) of species were calculated. Biomass consumed was calculated using consumed mean prey mass (kg) per wolf to excrete one collectible scat as a function of mean prey body mass (x kg) provided per feeding experiment by Wachter et al. (2012) correction factor 1 (CF1), y = 1.798 (1-exp (−0.008x). A conventional correction factor CF2, y = 0.439 + 0.008x was also used (Weaver, 1993). Information on wolf diet was also compared with data from Lyngdoh et al. (2020), using descriptive statistics to compare the relative frequency of occurrence between common prey consumed with that of the rest of the highland wolves of Asia. We used this information to determine the dietary similarity between the wolves from the Himalayas and their conspecifics in the Asian region. Jacob’s preference index was used to determine prey selectivity using available data from published sources (Lyngdoh et al., 2020; Anon, 2021; Sharma et al., 2021).
Perceived depredation by wolf or snow leopard was derived from interview surveys in which respondent claims were recorded. Similarly, the proportion of livestock in diet (whether depredated or scavenged) was identified through scat analysis. Data comparison on livestock population, diet, and perceived depredation by large carnivores as well as other carnivores was done using the Pearson’s correlation co-efficient and the χ2 test. We also performed correlations to determine the relationship between perceived depredation and livestock population. Wolf depredation claims were tested against dietary consumption patterns for domestic prey to see if they emulated a similar proportion of diet frequencies using the Chi-squared test. Livestock data provided through the Divisional Forest Officer, Spiti Wildlife Division, were used to account for the availability of domestic prey.
Wolf tracking
To understand wolf movements in the study area, we used telemetry data from three individual wolves of independent packs. The study area was extensively searched for wolf dens, signs, and scat to determine probable areas of wolf activity through personal observations and local information over extensive local surveys and ground sign surveys by SL. Local field-level permissions were obtained from the Chief Wildlife Warden, Govt. of Himachal Pradesh (Letter No. WL/Research Study/WLM/4671 and Office order even number/87 dated 07/04/2010). Before initiating the trapping procedures, the entire area was pre-monitored for the presence of wolves. On confirmation, camera traps were laid in the area to ascertain possible movement patterns and probable movement paths. Based on results, 15 to 20 padded leg-hold traps (Duke 3 coil spring rubber jaw trap, 3OS–RJ, Duke Company, West Point, MS, United States) were set concealed in a circular manner along the trails where wolves frequented; around a live bait or partially consumed kill (whenever available). The trap sites were also laced with coyote and wolf gland lure as well as food lure (Hawbaker & Sons, wolf gland lure 100 and Hawbaker & Sons, coyote food lure no. 10 Stanley Hawbaker and Sons, Fort Loudon, PA, United States), in order to increase the possibility of wolf encountering the trap. Once set, traps were kept undisturbed and were manually checked every hour from a distance of 500 m with help of binoculars (Nikon Monarch 5). The core capture team, which included an experienced biologist, a wildlife health professional, and two assistants, camped at a distance of 1 to 1.5 km from the trap site, to minimize the response time, thereby minimizing the possibility of trap-related injuries. Wolves and bycatches, i.e., red foxes were restrained using a snare pole (Midwest Tongs, Inc., 48–72 inch) and a nylon net. Non-target individuals, i.e., red foxes were released immediately. Captured wolves were sedated in the hindquarters with Cyclohexane (Ketamine) and a tranquilizer (Xylazine) by a dart gun (i.e., one individual wolf) or with the help of manual restraint using the aforementioned tools (i.e., two individual wolves, Kreeger et al., 1988; Mech and Boitani, 2003; Boitani and Powell, 2012). Considering logistic constraints at high altitudes, the usage of minimal equipment such as leg hold traps, snare pole, requisite drugs and nylon nets was used to successfully capture–restrain wolves and red foxes. Dosages were administered with 5 mg/kg (Ketamine) and 1 mg/kg (Xylazine) for the estimated weight of wolves (30–35 kg). Animals were revived with Yohimbine (0.12 mg/kg) after collaring. Animals were fastened with Vectronics GPS Vertex collars weighing approximately 470 g (∼ one-tenth kg of estimated wolf weight, Globalstar Satellite System/Iridium based satellite collars, VECTRONIC Aerospace GmbH Berlin Germany) once trapped, principally to locate their movements. The collars were programmed to provide regular GPS fixes at every 5-h intervals to generate information on a cyclic basis. A total of 904, 742, and 955 GPS fixes were used for three wolves, Dhankar (male), Kunzum (female), and Leica (female) respectively. Wolves were monitored individually for a period between July 2015 and June 2017. All calculations were done in (R Core Team, 2013) using the Adehabitat package (Calenge, 2006) for the calculation of Minimum Convex Polygon (MCP) and kernel utilization distribution (KUD–50 and 95% isopleths).
Conflict modeling
To determine the most probable areas of Human–wolf conflict, we used wolf scat locations in a presence-only modeling Maximum Entropy (MaxEnt) algorithm. MaxEnt finds the probability distribution of maximum entropy, which is the most spread out or closest to uniform with limited information given species locations (Phillips et al., 2004, 2006; Hijmans and Elith, 2011). MaxEnt has the potential to map the spatial suitability of species with fewer locations and has performed well as compared to other available presence-only models (Hernandez et al., 2006; Pape and Gaubert, 2007; Pearson et al., 2007; Wisz et al., 2008). Models were run with the exclusion of areas that has no chance of wolf, i.e., beyond 5,500 a.m.s.l and model selection was carried out to select the best SDMs (Warren and Seifert, 2011; Yackulic et al., 2013, the statistical approach detailed later). We then masked out areas below 5,200 a.m.s.l to calculate wolf conflict zones as areas above 5200 were likely to be highly inhospitable for most large mammals. Wolf scat locations of only 214 from 283 samples collected were used as a surrogate for conflict locations to account for livestock consumption and predation locations. The remaining scat locations were not used as they did not account for domestic prey as items consumed after scatological analysis. We used scats to model conflict as scat locations can be reliable indicators of predator territory and intensity of use. Although scat deposition may not occur in the exact spot where predation/scavenging may have taken place, one can assume that predators such as wolves are likely to defecate within their territory/path where they often predate or scavenge. Given that domestic prey can only be located in an area close to human settlements, any wolf scat containing livestock would mean predation/scavenging would have been sourced from a village or its grazing pasture within that wolf’s territory. We took adequate precautions not to collect scats that were extremely close (2–3 km approximately) or within villages as these were known to be frequented by domestic dogs. However, dog numbers in these areas of Spiti are known to be low when compared with the sub-district center (Home et al., 2018). Also, domestic dog scats can have multiple non-prey items such as garbage, and human-generated waste which can be identified on the ground with some field ecological knowledge. Wolf presence records were obtained from data of three wolf packs that were GPS collared from a period of 2015–2017. Regular GPS fixes were obtained, and presence points of individuals were thus used from 2,601 locational data that were recorded.
A total of 12 covariate layers were used to model wolf conflict zones using MaxEnt (Figure 1 and Supplementary Table 1a). We generated compatible layers from Digital Elevation Model (30 m), forest cover map (FSI, 2015), topographic heterogeneity, ruggedness index (Riley et al., 1999), NDVI, and slope. Layers for livestock and depredation were rasterized from questionnaires using a kernel density function. For example, depredation claims for wolf (wolf depredation) and snow leopards (snow leopard depredation) from questionnaire surveys were used for preparing respective maps. The livestock density surface layer was also generated through village surveys and kernel maps were reproduced. Kernel density calculates the density per unit area from point or polygon features by using a kernel function described by Silverman (1986) to fit a smoothly tapered surface to each point or polygon (Silverman, 1986). The algorithm determines the bandwidth from a search radius from the degree to which features are concentrated or dispersed around the geometric mean center. Point data were used as input features, and attributes to be kernelled were incorporated into the “population field” using the following formula where SD is the standard distance, Dm is the median of distance, and n is the sum of population field values such as “livestock numbers.”
As we had limited knowledge of wolf movement from a few individuals, we modeled wolf presence surface layer through GPS relocations. This was generated through a GLM surface model using a resource selection function (RSF). Terrain features such as elevation, slope, aspect, Terrain roughness index, Terrain percentage index and flow direction were generated. These were used in a model selection, and a wolf presence layer was generated based on the best model according to AIC in the R software using packages glmm, raster, plyr, and magrittr. The best RSF was then used as a covariate to account for wolf presence in the study area of interest (Morris et al., 2016; see Figure 1B and Supplementary Material 1c).
Output cell sizes were generated to 100 m by rescaling in ArcMap with the same extents and pixel size. Subsequently, kernel density analysis was performed in a geo-spatial environment using ArcMap RELEASE 10.7.1 (Environmental Systems Research Institute). The generated layers were then used as response variables in ASCII format for further analysis in MaxEnt. The model selection process was performed after excluding highly correlated layers that had >80% correlation for MaxEnt modeling (Figure 1B and see also the Supplementary Material). Except for terrain heterogeneity and slope; wolf and snow leopard depredation, all layers showed <80% Pearson correlation.
Statistical analysis, model validation, and selection
The predictive performance of the model was estimated using the area under the curve (AUC) derived from relative operating characteristic (ROC) plots. To select the best model parameters, we compared different models with a combination of the “feature class” and “regularization multiplier.” MaxEnt provides different types of restrictions (“feature class”) in the modeling stage such as lineal (L), quadratic (Q), product (P), threshold (T), and hinge (H). We used all the possible combinations of these features. The used regularization (beta) multiplier values; 0.25, 0.5, 0.75, 1, 2, 3, 4, and 5 were based on the study by Morales et al. (2017). Models with lower multipliers tend to overfit data; however, higher multipliers may lead to a loss in capturing species relations to various environmental components (Radosavljevic and Anderson, 2014). By combining feature classes and regularization multipliers, we assessed a total of 408 models for each case study, plus the default auto-feature with 5000 background points as the study area had limited spatial extent. The best MaxEnt model parameters were used for predicting wolf predation hotspots generated through all wolf scat samples (n = 232).
To evaluate whether competing models were significantly different from each other, we used ENMTools (Warren et al., 2010). We compared all the generated models by utilizing the corrected Akaike information criterion (AICc) available in the software ENMTools version 1.4.4 (Warren et al., 2010). The information score of the model indicates that the value has been calculated from the AIC corrected for bias due to limited samples. The smaller the AIC value, the better the model fit. Results of simple model selection implemented by ENMTools were carefully interpreted for each parameter value for the calculation of AICc (Warren and Seifert, 2011). We selected the best model and ran 10 replicates with cross-validation to test for model consistency and used the best model to finally generate a conflict map in Arc Gis 10.6. The final cumulative output was used to depict conflict values from 0 to 100. We selected cumulative values of 75–100 as high, 50–75 as medium, 25–50 as low, and 0–25 as no data or conflict zones (Beane et al., 2013). We calculated the area and proportion of such conflict zones subsequently. Similarly, we also used the best model from the above with available locations of wolf scats from all prey items to generate wolf-livestock depredation hotspots (n = 232). We also modeled wolf conflict by considering only habitat covariates and wolf presence data (excluding wolf depredation covariate) using the best model features in MaxEnt to check for any differences in model output with respect to conflict hotspot mapping. This was done to also examine if conflict hotspots modeled through environmental variables alone can serve as reliable surrogates for determining human-wolf conflict.
Results
Wolf diet
Out of 118 scats subjected to DNA tests, 105 scat samples were sequenced successfully on amplification. Out of 105 amplified scats, 101 scats belonged to Canis lupus with 99% match in the Genbank BLAST database. It was found that 11% of the scats did not produce any results. We also found no differences in prey consumption proportions reported by genetically confirmed scat and otherwise, i.e., n = 283 (t-test, P = 0.4, df = 20). We, therefore, used other suspected wolf scats for further analysis in combination with DNA verified scats considering an error rate of 4%. Wolf diet constituted mostly of livestock (79%) while wild prey constituted 17%. The remaining prey items which amounted to 3% were either unidentifiable or vegetative matter. Wolf diet composition accounted for 44.45% (± 7.25, numbers include SE) of cattle or yak as a prey item, 14.63% of sheep/goat. Bharal constituted 4.01% (± 2.08) and Ibex constituted 3.05% (± 0.4) of the diet of wolves in the study area (Figure 2). Jacob’s index showed wolves preferred domestic prey, i.e., cattle, equid, sheep, and goat (Figure 2A). Additional details of a diet of the red foxes and snow leopards have been included in Supplementary Material 1 for information.
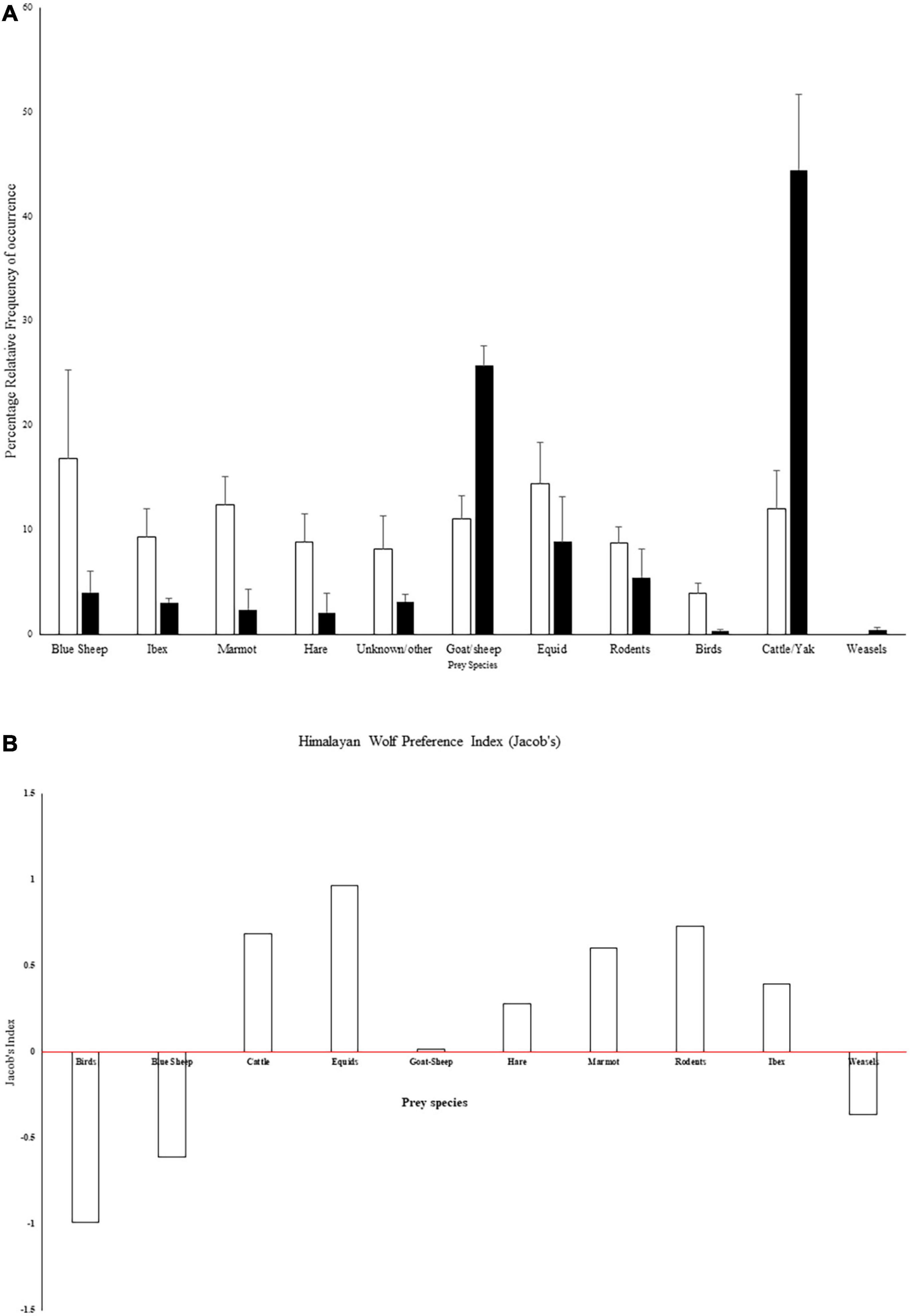
Figure 2. (A) Percentage relative frequency of occurrence of prey items in wolf scats from the Spiti region (Filled bars) in comparison with wolves from 21 studies across Asia (Lyngdoh et al., 2020) across years (2015–2017, n = 283). Filled/White bars indicate percentage and whiskers indicate ± 1 SE. (B) Jacob’s index for wolf prey preference. Except for Birds, blue sheep and weasels wolves consumed other prey species more than their availability. Preference may change according to availability.
Perceived human-wolf conflict
We found that 92.2% of the respondents were primarily agro-pastoralists, while 7.7% of the respondents have engaged in other forms of occupation such as skilled or semi-skilled labor. Formal education levels of respondents were 17.5%–primary level, 40.7%–middle level, 16.5%–higher secondary level, and 15.5% had no formal education. About 5% of the respondents were engaged in more than one occupation. Local interviews revealed that 147 livestock kills were claimed by respondents to be due to wolves and 262 kills due to snow leopards, totaling 409. These account for ∼ 2% of livestock loss due to wolves and ∼3% to snow leopards, assuming these are annual losses. Although we found that only 55% of the respondents asserted to have seen wolves, 98% perceived them as a possible threat to various livestock (Figures 2, 3). Similarly, 97% of the respondents claimed snow leopards also were a threat to livestock. Claims by 97% of the respondents were that feral dogs as well posed a threat to livestock apart from wolves and snow leopards. Villages that reported higher livestock numbers also reported higher livestock depredation (Pearson’s = 0.47, r2 = 0.22). Mean livestock depredation kills reported were 5.25 ± 0.90 per year (S.E) and 7.70 ± 1.66 per year for wolf and snow leopard, respectively. Claims of wolf depredation showed higher correlation with livestock population in villages than snow leopard depredation (Pearson’s = 0.67 vs. 0.56, r2 = 0.46, 0.35). The proportion of livestock depredated by wolves (through questionnaires) vs. the proportion of livestock in the diet through scats was 33 vs. 50% for sheep/goat, 28 vs. 10%, for horse/donkey, and 21 vs. 56% for cattle species. We found that perceived depredation (claims of livestock consumption pattern calculated only within domestic prey class) was significantly different from the actual proportion of diet pattern exhibited by wolves (χ2 = 68.04, p-value < 0.0001, df = 2). Perceived depredation was higher for equids and lower for domestic bovids.
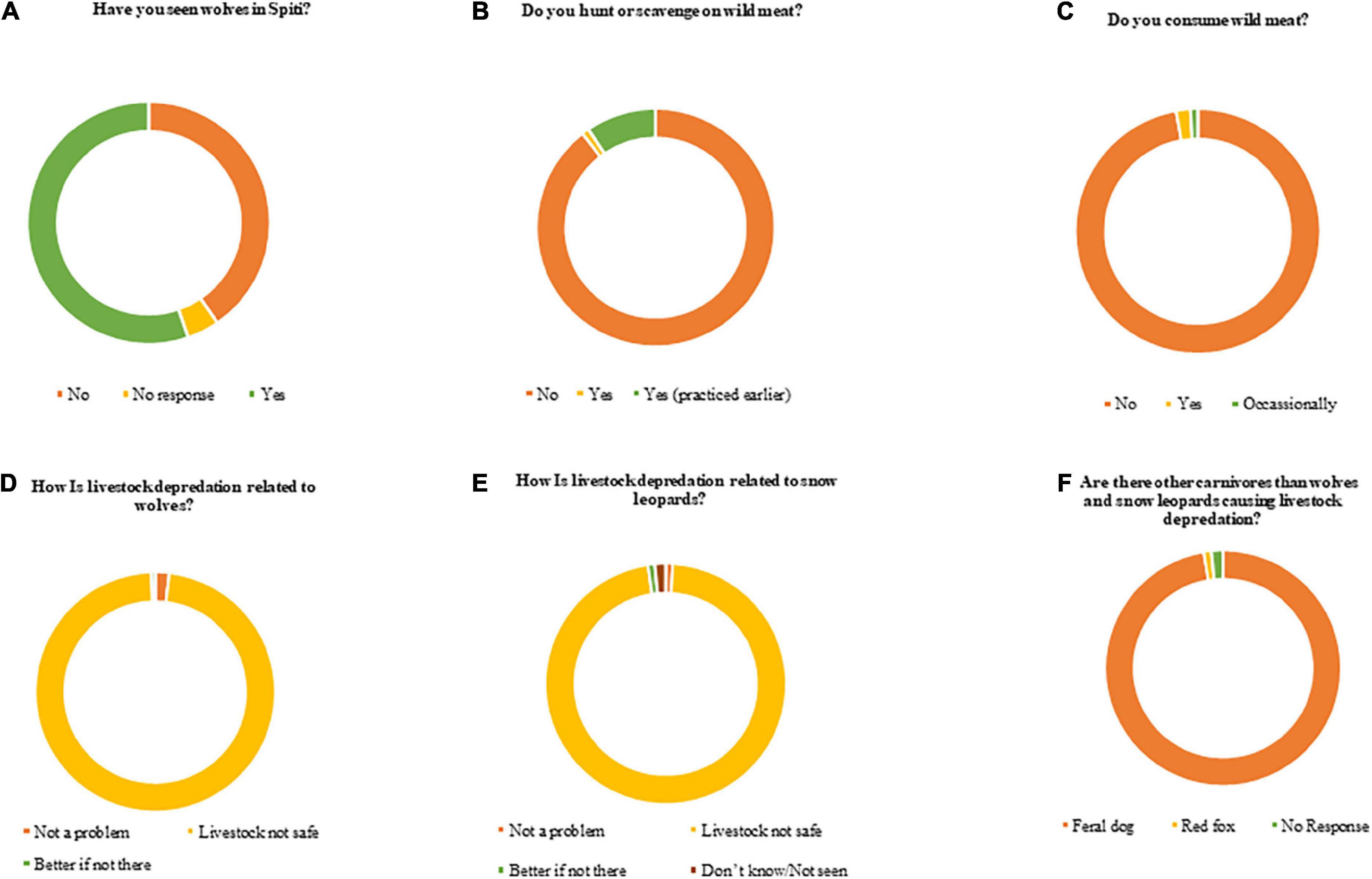
Figure 3. Attitudes and response of households with respect to questionnaire surveys on wolf conflict in 35 villages (n = 200).
Wolf conflict hotspots with and without perceived depredation
The 100% MCP home ranges of wolves ranged from 936 to 2,773 km2 while the 95% Kernel Utilization Distribution (KUD) areas ranged from 179 to 918 km2. It was observed that the 50% MCP home ranges of wolves were 338 ± 122 km2. The 50% Kernel Utilization Distribution (KUD) home ranges were 86 ± 81 km2. The 95% home ranges were 1,044 ± 56 km2 and 449 ± 407 km2 for MCP and KUD, respectively.
Across 418 MaxEnt models that excluded perceived wolf depredation as a covariate as well, we found the threshold model was the best model (Log Likelihood = −1529, AICc = 3113, Figure 4 and Supplementary Figures 1, 2). The mean AUC was 0.92. The average test AUC for the replicate runs was 0.91, and the standard deviation is 0.03. The average training AUC was 0.95. The average model output regularized training gain was 1.811 while the test gain was 1.65. The average algorithm convergence reported was 228 iterations with an average of 5071 background points and entropy of 6.71. Conflict hotspots generated were mostly explained by perceived wolf depredation locations from questionnaires (40%), wolf presence (21%), and village density (23%) which were the top three variables contributions. However, by permutation, village density (42%), perceived depredation (18%), wolf presence (17%), and NDVI (8%) were most important. The environmental variable with the highest gain when used in isolation was perceived wolf depredation, wolf presence, village density, and elevation which had the most useful information by themselves, respectively. Similarly, a decrease in the gain was most when the perceived wolf depredation was omitted, therefore generating the most information that was present in the other variables. Marginal response curves depicted the model to have positive responses to wolf presence, perceived wolf depredation, village population, village density, and wolf depredation. Marginal curves were negative to slope, ruggedness, and elevation.
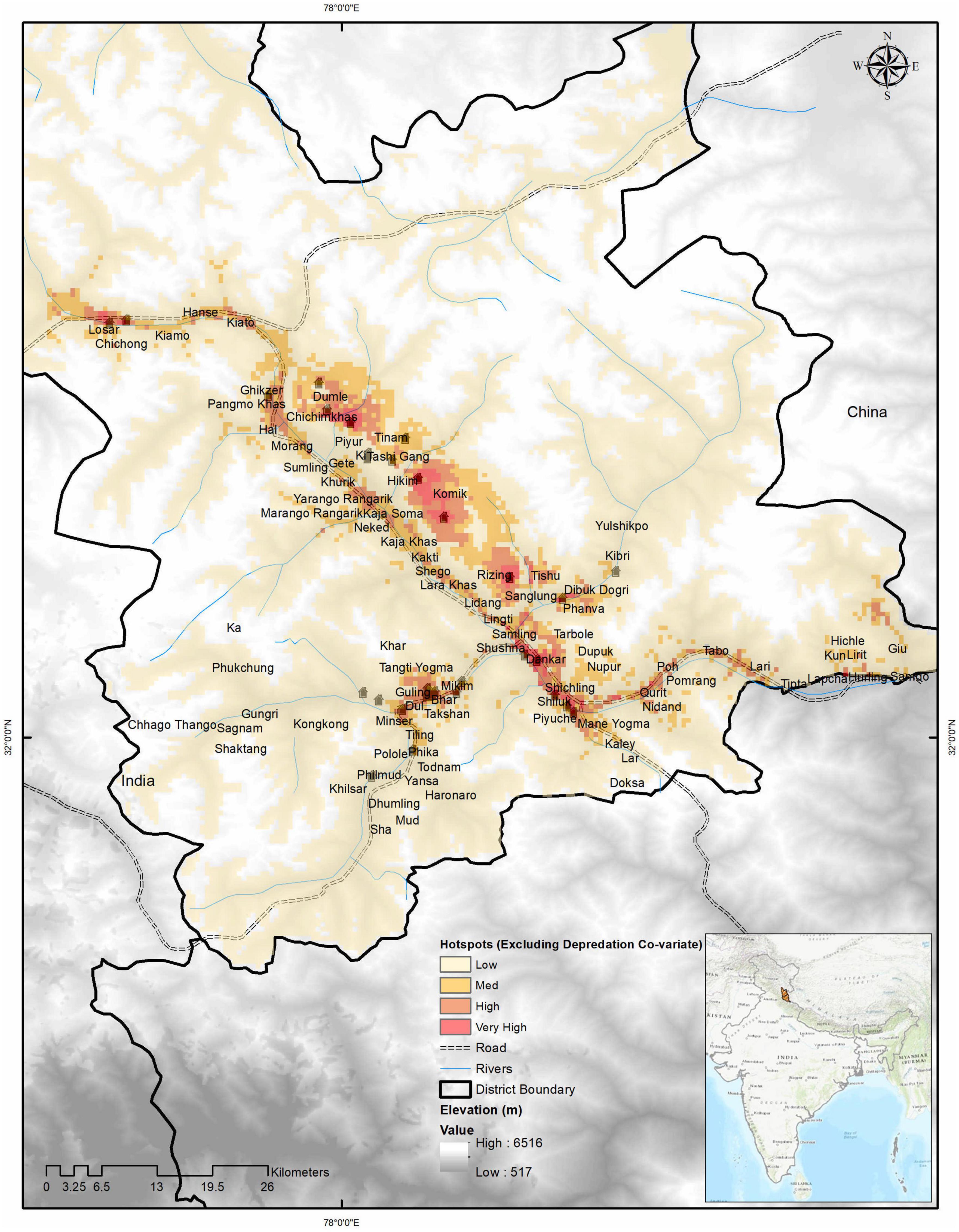
Figure 4. Wolf predation hotspots when all prey data is included from scats of all prey combined. The very high predation zone is 34 km2, the high predation zone is 89 km2, the medium predation zone is 239 km2, and the low predation zone was 3,569 km2 of the habitable study area.
When we excluded the depredation covariate (perceived wolf depredation), the mean AUC was 0.92. The environmental variable with the highest gain when used in isolation was wolf presence, which therefore appears to have the most useful information by itself. However, the environmental variable that decreases the gain the most when omitted was village density, which therefore appears to have the most information that was not present in the other variables. It was found that village density (39%), wolf presence (23%), slope (11%), and elevation (7%) were the most important variables by contribution. In terms of permutation, village density (37%), slope (28%), elevation (19%), and ruggedness (11%) were important, respectively.
We found area denoted as a very high conflict zone was 36 km2 (∼ 1%), a high conflict zone was 62 km2 (∼ 2%), a medium conflict zone was 196 km2 (∼ 5%), and a low conflict zone was 3,696 km2 (92%) of the hospitable study area (Figure 5). Model outputs of conflict hotspots by excluding depredation covariate generated some larger conflict areas in terms of high (140 km2) and medium (270 km2) conflict zones, but predicted lesser areas in the low conflict zone (3,480 km2) and very high conflict zone (35 km2). Images of wolf predation hotspots generated for all scat locations used also show 34, 89 and 239, and 3,569 km2 of the area as (wild prey included) hotspots in very high, high, medium, and low categories, respectively (Figure 4, see also Supplementary Material 2).
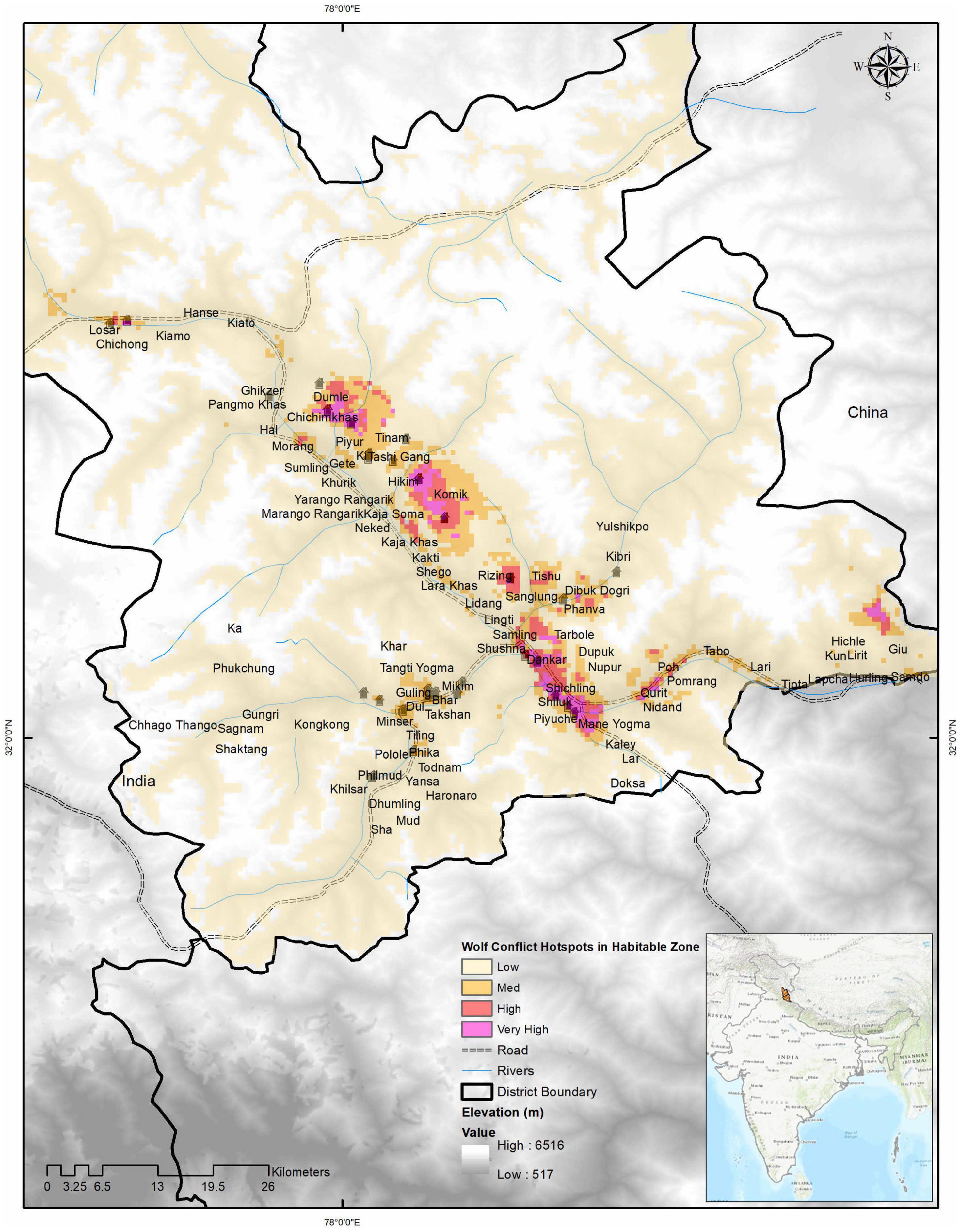
Figure 5. Wolf conflict hotspots modeled showing darker pink regions with higher conflicting potential. The very high conflict zone is 36 km2, the high conflict zone is 62 km2, the low conflict zone is 196 km2, and the low conflict zone below the threshold was 3,686 km2 of the habitable study area.
Discussion
Wolf diet and livestock
We found that wolves of Spiti primarily killed large domestic prey (also see Lyngdoh et al., 2020). Similar studies from the adjoining landscape of the regions reflect comparable patterns (Bagchi and Mishra, 2006; Namgail, 2007). A review of the dietary habits of gray wolves (Newsome et al., 2016; Lyngdoh et al., 2020) showed wolves from India preferred mostly medium-sized wild ungulates and domestic prey. The results of the review (Newsome et al., 2016) present mostly dietary habits of peninsular wolves of India, the current study thus adds to the existing information. Similarly, Zhang et al. (2009) found that in the Dalai Lake Natural Reserve, Mongolia; livestock formed much of the wolf’s diet probably due to the overabundance of livestock. These patterns of prey consumption are more common across Central Asia, where Mongolian wolves co-occur in agro-pastoral landscapes (Lyngdoh et al., 2020). As preference data may be highly dependent on availability, it is suggested that seasonal and larger coverage may provide better conclusions on dietary habits especially for conflict areas.
Wolf conflict model and landscape characteristics
The simplicity and ease of use of MaxEnt have prompted many researchers to use the software (Warren and Seifert, 2011). We avoided default settings and approached the modeling process to arrive at an optimal regularization parameter (Warren et al., 2010). Our model predicted that wolves use much of the valley areas within the study landscape along with plateau-like hill tops in the Spiti area. This is supported by the choice of lower areas close to 3,500 m, decreasing ruggedness and slope but increasing human and livestock populated areas. In other areas, one may find similar patterns depending on the size of the area available based on such preferred landscape and resource characteristics. The variables that were permutationally important were wolf presence, perceived wolf depredation, slope, elevation, and village density, respectively.
On the exclusion of depredation as a layer, environmental variables such as village density, wolf presence, slope, elevation, and ruggedness were the only important predictors of wolf conflict. Hotspots generated from only these variables may be useful in reducing any inherent biases that could have come from perceived conflict data. They may even be cost-effective if one were to use only habitat covariates.
However, livestock losses modeled (with all 11 variables) in terms of conflict were explained well by perceived depredation. These may be interpreted with caution as perceived depredation by snow leopard was also highly correlated (and hence dropped as a covariate). Wolf conflict hotspots modeled through depredation data could serve as reliable data in cases where the animal (wolf) locational data is not available. Scat presences were also explained well with village density which is not unexpected as wolves consumed mostly livestock.
Wolf conflict hotspots were located in areas that had low topographic heterogeneity and, decreasing ruggedness, which were probably historically colonized by humans as well. Being pursuit predators, wolves utilized areas that were undulating and gentler on their slopes. Whether this is driven by competing species or niche exclusion in terms of space and prey needs to be examined in detail. Wolves selected areas with optimal livestock densities and villages that maintained their agro-pastoralist characteristics. Predominantly selected areas of wolf depredation were villages occupied by the agro-pastoralist community situated on gentler terrain or topography. This means it was unlikely to find wolf depredation with increasing urbanization; however, as in the case of Indian wolves (Jhala, 1991; Chapron et al., 2008, 2014), fragmented landscapes that are interspersed with a mosaic of semi-urban to natural settings may allow co-existence despite conflicts. This does not imply that decreasing livestock would reduce the chances of wolf presence. Studies have shown that wolves may choose to predate or switch to wild prey if available in good numbers (Jȩdrzejewski et al., 2012). The proportion of livestock population to that of wild prey in the area is 10 times. By even naïve estimates, wild prey seems to be the preferred diet of wolves (Newsome et al., 2016; Mengüllüoğlu et al., 2019; Lyngdoh et al., 2020). From field observations, it was clear that in such a landscape of limited and short periods of plant growth, livestock herds became concentrated in productive pastures as seen with responses to NDVI. This may have led to prey switching among wolves due to the sheer likelihood of greater contact with domestic stock through past times, and resulted in behavioral manifestations over wolf generations. The implications with respect to future climate changes which predict greater energetic costs due to reduction in niche overlap between natural prey-predators in this landscape may exasperate existing levels of conflict with wolves (Aryal et al., 2016).
Himalayan wolves operated at altitudes of 3,300 m and above in areas with gentle slopes, which was expected. This may also be due to competition with sympatric species such as snow leopard which prefer more rugged and cliff-like terrain (Chetri et al., 2017; Watts et al., 2019). Snow leopards significantly preferred cliff-dwelling wild ungulates, whereas wolves preferred typically plain-dwellers and hence evolved within such niches (Chetri et al., 2017; Joshi et al., 2020). A better sampling strategy where both wolves and snow leopards are tracked through GPS telemetry could reveal finer differences in habitat use by individual species.
Perceptions toward large predators
Attitudes toward large carnivores are shaped not just by religion or culture but also by gender, education, and awareness of wildlife law (Bhatia et al., 2017). Semi-structured questionnaire surveys revealed there is perceived negativity and conflict between agro-pastoralists and wolves, which were claimed to be the main causes of livestock mortality. The northern part of the study area (Kibber WLS and adjacent areas) reported more conflict in terms of wolf predation while the southwestern part (Near Pin Valley NP area) reported relatively greater conflict arising due to snow leopards. In the recent past, the retaliatory killing of wolves was reported to occur (Mishra, 1997), but this practice has slowly been discontinued largely due to conservation awareness, enforcement, and religio-social sentiments of the predominant Buddhist communities of the region (Sharma et al., 2015; Ghoshal et al., 2018). In comparison, qualitative surveys across Ladakhi villages in neighboring union territory north of the study area report that people generally exhibited a significantly negative attitude toward wolves than snow leopards (Bhatia et al., 2017). In Hemis, Northern India, 64% of the respondents were tolerant toward snow leopards, while over three-quarters of respondents (78%) perceived wolves negatively (Maheshwari and Sathyakumar, 2019). Similarly, in the Nepalese Himalayas, the herding communities were much more antagonistic to wolves rather than snow leopards even though the latter caused much more economic losses (Kusi et al., 2020). We did not investigate the causes of perceived negativity toward wolves, but we suspect that such biases against wolves can be attributed to a lack of knowledge about species behavior rather than its relative presence (Bangs et al., 2005; Bisi et al., 2010; Suryawanshi et al., 2013; Duriez et al., 2019).
Our results showed that perceived levels of livestock depredation did not reflect actual levels of livestock consumption by wolves in concurrence with earlier studies (Suryawanshi et al., 2013, 2017). In terms of the type of prey and quantity of livestock consumed as claimed by respondents did not match the proportions observed from scat analysis. However, better insights can be delivered from more intensive sampling by taking into account season and village clusters. Respondents were likely to under or over report the number of livestock lost due to depredation. Scat analysis may only provide data on the proportion of livestock consumed; however, the extent of depredation is difficult to determine from -questionnaire surveys or scat analysis alone. We also found that livestock constituted major prey in the diet of wolves from Spiti unlike studies from similar landscapes in Asia (Lyngdoh et al., 2020). However, wolf depredation is lower than expected given its exposure to domestic livestock. From questionnaire surveys, depredation accounted for ∼ 2–3% of the total livestock for wolves and snow leopards. Given livestock densities are 10 to 14 times higher than wild prey densities in the area there may be other factors that contribute to livestock mortality and need to be investigated (Sharma et al., 2020). Similar findings have been reported from the Northern Rocky Mountains where wolves accounted for less than 0.04% of the total losses or 0.01% of all predator-caused mortalities (Bangs et al., 2005). The reason why perceived conflict is taken as a real conflict for wolves in our study area is probably due to local attitudes that may have over a long time festered negativity and persecution rather than co-existence. In Nepal, Kusi et al. (2020) also point out that local customs and beliefs toward snow leopards rather than wolves in sacred Buddhist literature, coupled with a longstanding conservation narrative of the former may be responsible for a discriminatory attitude against both these large predators.
An important caveat in using such information is that incomplete knowledge of the landscape and its use by animals may lead to erroneous conclusions by relying solely on model outputs. In the case of the current study, we have attempted to link and explain nuances in the model outputs pertaining to proximate drivers of conflict by considering the study area and local information from the pastoralist community. Traditional communities are a reliable source as they have been living with wolves and are rarely oblivious of their presence in proximity (Jhala, 1991).
Living with conflict or co-existence
Human-wolf conflict is global; however, their management strategies have been specific, regional, complex, and also controversial. The wolf is a top predator that has the capability to drive trophic cascades across large landscapes (Ripple and Beschta, 2007, 2012; Beschta and Ripple, 2009); this may be true even for its range in the Himalayas and merits further investigation. Its conservation is thus linked with securing not only the landscape but the people and the region as a whole. In Spiti, a stronghold for Himalayan wolves, cultural ethos, and wildlife have been the primary reasons for tolerance to large predators (Ghoshal et al., 2018). Therefore, non-lethal methods that can enhance livestock security, promote wild prey densities, eradicate feral dogs and foster tolerance must be advocated strongly in the short and medium terms (Jhala, 1991; Lozano et al., 2019).
Conservation agendas recognize wolves as a least concern species globally which does not give on the ground the attention of a priority species (Boitani et al., 2018). Therefore, complex cultural legacies, legal controls, global priorities that overlook local nuances, and scientific or conservation bias may have facilitated perceptions against wolves in the Himalayas as well. Since both species, i.e., the snow leopard and wolf occur in the same landscapes, we suggest that future efforts toward the former’s conservation must accommodate in tandem conservation of the latter as well. As these landscapes are extensive and often beyond the normal protected area paradigm, working with communities will play a crucial role in protecting the species.
Cultures that raise livestock have strongly disliked wolves (Bangs et al., 2005). The section of the Himalayan community which relies on pastoralism is sizeable (Roy et al., 2013; Gentle and Thwaites, 2016). Despite this huge dependency, pastoralism can contribute positively to biodiversity conservation (Sharma et al., 2003). Therefore individuals knowledgeable about herding and pasture management, trained in conservation and ecology, able to design management experiments, and develop novel but tradition-based holistic management practices are essential in the long term (Molnár et al., 2016). Raising awareness and development of professional capacities through regularized curricula and applicability of such methods for especially rangeland management must be piloted through a policy framework and field trials. This approach may also aid in informing the stakeholders toward timely interventions in order to ensure the survival of the last remaining populations of an ecologically unique and evolutionarily significant Himalayan wolf, its prey and habitat.
Data Availability Statement
The original contributions presented in this study are included in the article/Supplementary Material, further inquiries can be directed to the corresponding authors.
Ethics statement
The studies involving human participants were reviewed and conducted as per the National Code of Ethics. The patients/participants provided their informed consent to participate in this study. The animal study was reviewed and approved by the Chief Wildlife Warden, Himachal Pradesh and MoEFCC, Govt. of India under the WPA 1972.
Author contributions
SL designed the study, gathered the data, analyzed the data, wrote the manuscript, and approved the manuscript. BH conceptualized the work, revised the manuscript, reviewed the manuscript, and approved the manuscript. Both authors contributed to the article and approved the submitted version.
Conflict of Interest
The authors declare that the research was conducted in the absence of any commercial or financial relationships that could be construed as a potential conflict of interest.
Publisher’s Note
All claims expressed in this article are solely those of the authors and do not necessarily represent those of their affiliated organizations, or those of the publisher, the editors and the reviewers. Any product that may be evaluated in this article, or claim that may be made by its manufacturer, is not guaranteed or endorsed by the publisher.
Acknowledgments
We thank the encouragement given by the Director and Dean of WII with this study. We thank specially the CWLW, Himachal Forest Department, Spiti Wildlife Division, Rajesh Sharma, Rajeev Sharma, Devender Chauhan, and Staff for their support. We thank Parag Nigam and S. Krishna for their immense help in the immobilization of animals. We thank the help in the field by various volunteers and personnel. We thank Gompo, Namtak, Yarphel, Namgial, Dorje, Tenzing, Keysang, Tashi, Rajender, and Nishi, Chering, Thukten, and their Chammos for their field support. The residents of Mane, Kibber, Langcha, Suluk, Chichong, and Kholaksar. We thank the staff at Chandertal. We also thank to express our immense gratitude to the people of Spiti for their care and concern during our field times for this study. Julley!
Supplementary Material
The Supplementary Material for this article can be found online at: https://www.frontiersin.org/articles/10.3389/fevo.2022.739181/full#supplementary-material
Footnotes
References
Aggarwal, R. K., Ramadevi, J., and Singh, L. (2003). Ancient origin and evolution of the Indian wolf: evidence from mitochondrial DNA typing of wolves from Trans-Himalayan region and Pennisular India. Genome Biol. 4:6.
Anand, S., and Radhakrishna, S. (2017). Investigating trends in human-wildlife conflict: is conflict escalation real or imagined? J. Asia Pac. Biodivers. 10, 154–161. doi: 10.1016/j.japb.2017.02.003
Anon (2021). Status of Snow Leopard and Prey in Himachal Pradesh, 2021. Shimla: Wildlife Wing – Himachal Pradesh Forest Department, Government of Himachal Pradesh.
Aryal, A., Shrestha, U. B., Ji, W., Ale, S. B., Shrestha, S., Ingty, T., et al. (2016). Predicting the distributions of predator (snow leopard) and prey (blue sheep) under climate change in the Himalaya. Ecol. Evol. 6, 4065–4075. doi: 10.1002/ece3.2196
Bagchi, S., and Mishra, C. (2006). Living with large carnivores: predation on livestock by the snow leopard (Uncia uncia). J. Zool. 268, 217–224. doi: 10.1111/j.1469-7998.2005.00030.x
Bangs, E. E., Fontaine, J. A., Jimenez, M. D., Meier, T. J., Bradley, E. H., Niemeyer, C. C., et al. (2005). Managing wolf-human conflict in the northwestern United States. Conserv. Biol. Ser. Camb. 9:340.
Bassi, E., Willis, S. G., Passilongo, D., Mattioli, L., and Apollonio, M. (2015). Predicting the spatial distribution of wolf (Canis lupus) breeding areas in a mountainous region of central Italy. PLoS One 10:e0124698. doi: 10.1371/journal.pone.0124698
Beane, N. R., Rentch, J. S., and Schuler, T. M. (2013). ). Using Maximum Entropy Modeling to Identify and Prioritize Red Spruce Forest Habitat in West Virginia. Newtown Square, PA: U.S. Department of Agriculture, Forest Service, Northern Research Station, doi: 10.2737/NRS-RP-23
Behl, R., Sadana, D. K., Behl, J., Banerjee, P., Joshi, J., Vijh, R. K., et al. (2017). Characterization and microsatellite analysis for genetic diversity and bottlenecks of Spiti donkey. Indian J. Anim. Sci. 87, 1221–1225.
Beschta, R., and Ripple, W. (2009). Large predators and trophic cascades in terrestrial ecosystems of the western United States. Biol. Conserv. 142, 2401–2414.
Bhasin, M. K., Singh, I. P., Walter, H., and Bhardwaj, V. (1983). Genetic study of five population groups of Lahaul-Spiti and Kulu districts. Himachal Pradesh. Z. Für Morphol. Anthropol. 74, 13–38.
Bhatia, S., Redpath, S. M., Suryawanshi, K., and Mishra, C. (2017). The relationship between religion and attitudes toward large carnivores in Northern India? Hum. Dimens. Wildl. 22, 30–42. doi: 10.1080/10871209.2016.1220034
Bisi, J., Liukkonen, T., Mykrä, S., Pohja-Mykrä, M., and Kurki, S. (2010). The good bad wolf—wolf evaluation reveals the roots of the Finnish wolf conflict. Eur. J. Wildl. Res. 56, 771–779. doi: 10.1007/s10344-010-0374-0
Blandford, W. T. (1988). The Fauna of British India, including Cylon and Burma. Mammalia. London: Taylor and Francis.
Boitani, L., Phillips, M., and Jhala, Y. V. (2018). Canis lupus. The IUCN Red List of Threatened Species 2018: e.T3746A119623865. Available online at: https://dx.doi.org/10.2305/IUCN.UK.2018-2.RLTS.T3746A119623865.en (accessed May 20, 2020).
Boitani, L., and Powell, R. A. (2012). Carnivore Ecology and Conservation: A Handbook of Techniques. Oxford: Oxford University Press.
Calenge, C. (2006). The package “adehabitat” for the R software: a tool for the analysis of space and habitat use by animals. Ecol. Model. 197, 516–519.
Champion, S. H., and Seth, S. K. (1968). A Revised Survey of the Forest Types of India. New Delhi: Manager of Publications.
Chapron, G. (2005). Re-wilding: other projects help carnivores stay wild. Nature 437:318. doi: 10.1038/437318b
Chapron, G., Andren, H., and Liberg, O. (2008). Conserving top predators in ecosystems. Science 320:47.
Chapron, G., Kaczensky, P., Linnell, J. D. C., von Arx, M., Huber, D., Andrén, H., et al. (2014). Recovery of large carnivores in Europe’s modern human-dominated landscapes. Science 346, 1517–1519. doi: 10.1126/science.1257553
Chauhan, M., Gupta, A. K., and Dhillon, S. (2004). Genetic characterization of Indian Spiti horses. J. Genet. 83, 291–295. doi: 10.1007/BF02717899
Chetri, M., Odden, M., and Wegge, P. (2017). Snow Leopard and Himalayan Wolf: Food Habits and Prey Selection in the Central Himalayas, Nepal. PLoS One 12:e0170549. doi: 10.1371/journal.pone.0170549
Duriez, O., Descaves, S., Gallais, R., Neouze, R., Fluhr, J., and Decante, F. (2019). Vultures attacking livestock: a problem of vulture behavioural change or farmers’ perception? Bird Conserv. Int. 29, 437–453. doi: 10.1017/S0959270918000345
Farrell, L. E., Roman, J., and Sunquist, M. E. (2000). Dietary separation of sympatric carnivores identified by molecular analysis of scats. Mol. Ecol. 9, 1583–1590. doi: 10.1046/j.1365-294x.2000.01037.x
Fox, J. L., and Chundawat, R. S. (1995). Wolves in the trans Himalayan region of India: the continued survival of a low-density population. Ecol. Conserv. Wolves Chang. World 35, 95–103.
Forest Survey of India [FSI] (2015). India State of Forest Report. Dehradun: Forest Survey of India, Ministry of Environment, Forests & Climate Change (MoEF&CC).
Gentle, P., and Thwaites, R. (2016). Transhumant pastoralism in the context of socioeconomic and climate change in the mountains of Nepal. Mt. Res. Dev. 36, 173–182. doi: 10.1659/MRD-JOURNAL-D-15-00011.1
Ghoshal, A., Sonam, K., Namgail, S., Suryawanshi, K. R., Mishra, C., and Fiechter, M. (2018). Local community neutralizes traditional wolf traps and builds a Stupa. Oryx 52, 614–615. doi: 10.1017/S0030605318000959
Government of Himachal Pradesh (2021). District of Lahaul and Spiti, Government of Himachal Pradesh, India. Available online at: https://hplahaulspiti.nic.in/population/ (accessed June 18, 2021).
Habib, B. (2007). Ecology of Indian Wolf (Canis lupus pallipes Sykes, 1831), and Modeling its Potential Habitat in the Great Indian Bustard Sanctuary, Maharashtra, India. Ph.D. Thesis 274. Aligarh: Aligarh Muslim University.
Habib, B., and Kumar, S. (2007). Den shifting by wolves in semi-wild landscapes in the Deccan Plateau, Maharashtra, India. J. Zool. 272, 259–265.
Hall, T. A. (1999) BioEdit: a user-friendly biological sequence alignment editor and analysis program for windows 95/98/NT. Nucleic Acids Symp. Ser. 41, 95–98.
Hennelly, L., Habib, B., and Lyngdoh, S. (2015). Himalayan wolf and feral dog displaying mating behaviour in Spiti Valley, India, and potential conservation threats from sympatric feral dogs. Canid Biol. Conserv. 18, 27–30.
Hernandez, P. A., Graham, C. H., Master, L. L., and Albert, D. L. (2006). The effect of sample size and species characteristics on performance of different species distribution modeling methods. Ecography 29, 773–785. doi: 10.1093/bioinformatics/btaa255
Hesse, J. (2020). Gray Wolves, Once Nearly Extinct, Could be Coming Back to Colorado. London: The Guardian.
Home, C., Bhatnagar, Y., and Vanak, A. T. (2018). Canine conundrum: domestic dogs as an invasive species and their impacts on wildlife in India. Anim. Conserv. 21, 275–282. doi: 10.1111/ACV.12389
Jȩdrzejewski, W., Niedziałkowska, M., Hayward, M. W., Goszczyński, J., Jȩdrzejewska, B., Borowik, T., et al. (2012). Prey choice and diet of wolves related to ungulate communities and wolf subpopulations in Poland. J. Mammal 93, 1480–1492. doi: 10.1644/10-MAMM-A-132.1
Jethva, B. D., and Jhala, Y. V. (2004). Foraging ecology, economics and conservation of Indian wolves in the Bhal region of Gujarat, Western India. Biol. Conserv. 116, 351–357.
Jhala, Y. V. (1991). The status and conservation of the wolf in Gujarat and Rajasthan, India. Conserv. Biol. 5:9.
Joshi, B., Lyngdoh, S., Singh, S. K., Sharma, R., Kumar, V., Tiwari, V. P., et al. (2020). Revisiting the woolly wolf (Canis lupus chanco) phylogeny in Himalaya: addressing taxonomy, spatial extent and distribution of an ancient lineage in Asia. PLoS One 15:e0231621. doi: 10.1371/journal.pone.0231621
Kellert, S. R., Black, M., Rush, C. R., and Bath, A. J. (1996). Human culture and large carnivore conservation in North America. Conserv. Biol. 10, 977–990.
Kreeger, T. J., Seal, U. S., Callahan, M., and Beckel, M. (1988). Use of xylazine sedation with yohimbine antagonism in captive gray wolves. J. Wildl. Dis. 24, 689–690. doi: 10.7589/0090-3558-24.4.689
Kusi, N., Sillero-Zubiri, C., Macdonald, D. W., Johnson, P. J., and Werhahn, G. (2020). Perspectives of traditional Himalayan communities on fostering coexistence with Himalayan wolf and snow leopard. Conserv. Sci. Pract. 2:e165. doi: 10.1111/csp2.165
Lescureux, N., and Linnell, J. D. C. (2014). Warring brothers: The complex interactions between wolves (Canis lupus) and dogs (Canis familiaris) in a conservation context. Biol. Conserv. 171, 232–245. doi: 10.1016/j.biocon.2014.01.032
Lozano, J., Olszańska, A., Morales-Reyes, Z., Castro, A. A., Malo, A. F., Moleón, M., et al. (2019). Human-carnivore relations: a systematic review. Biol. Conserv. 237, 480–492. doi: 10.1016/j.biocon.2019.07.002
Lyngdoh, S., Shrotriyaa, S., Goyal, S. P., Clements, H., Hayward, M. W., and Habib, B. (2014b). Prey preferences of the snow leopard (Panthera uncia): regional diet specificity holds global significance for conservation. PLoS One 9:e88349. doi: 10.1371/journal.pone.0088349
Lyngdoh, S., Gopi, G. V., Selvan, K. M., and Habib, B. (2014a). Effect of interactions among ethnic communities, livestock and wild dogs (Cuon alpinus) in Arunachal Pradesh. India. Eur. J. Wildl. Res. 60, 771–780.
Lyngdoh, S. B., Habib, B., and Shrotriya, S. (2020). Dietary spectrum in Himalayan wolves: comparative analysis of prey choice in conspecifics across high-elevation rangelands of Asia. J. Zool. 310, 24–33. doi: 10.1111/jzo.12724
Maheshwari, A., and Sathyakumar, S. (2019). Snow leopard stewardship in mitigating human–wildlife conflict in Hemis National Park. Ladakh, India. Hum. Dimens. Wildl. 24, 395–399. doi: 10.1080/10871209.2019.1610815
Mech, D., and Boitani, L. (2003). Wolves: Behavior, Ecology, and Conservation. Chicago, IL: Chicago University Press.
Mech, L. D. (2017). Where can wolves live and how can we live with them? Biol. Conserv. 210, 310–317. doi: 10.1016/j.biocon.2017.04.029
Mengüllüoğlu, D., İlaslan, E., Emir, H., and Berger, A. (2019). Diet and wild ungulate preferences of wolves in northwestern Anatolia during winter. PeerJ 7:e7446. doi: 10.7717/peerj.7446
Mishra, C. (1997). Livestock depredation by large carnivores in the Indian trans-Himalaya? Environ. Conserv. 24, 338–343.
Molnár, Z., Kis, J., Vadász, C., Papp, L., Sándor, I., Béres, S., et al. (2016). Common and conflicting objectives and practices of herders and conservation managers: the need for a conservation herder. Ecosyst. Health Sustain. 2:e01215. doi: 10.1002/ehs2.1215
Monterroso, P., Alves, P. C., and Ferreras, P. (2011). Evaluation of attractants for non-invasive studies of Iberian carnivore communities. Wildl. Res. 38, 446–454.
Morales, N. S., Fernández, I. C., and Baca-González, V. (2017). MaxEnt’s parameter configuration and small samples: are we paying attention to recommendations? A systematic review. PeerJ 5:e3093. doi: 10.7717/peerj.3093
Morris, L. R., Proffitt, K. M., and Blackburn, J. K. (2016). Mapping resource selection functions in wildlife studies: Concerns and recommendations. Appl. Geogr. 76, 173–183. doi: 10.1016/j.apgeog.2016.09.025
Mukherjee, S., Goyal, S. P., Johnsingh, A. J. T., and Pitman, M. R. P. L. (2004). The importance of rodents in the diet of jungle cat (Felis chaus), caracal (Caracal caracal) and golden jackal (Canis aureus) in Sariska Tiger reserve, Rajasthan, India. J. Zool. 262, 405–411.
Namgail, T. (2007). Carnivore-caused livestock mortality in Trans-Himalaya. Environ. Manag. 39, 490–496. doi: 10.1007/s00267-005-0178-2
Newsome, T. M., Boitani, L., Chapron, G., Ciucci, P., Dickman, C. R., Dellinger, J. A., et al. (2016). Food habits of the world’s grey wolves. Mammal Rev. 46, 255–269. doi: 10.1111/mam.12067
Nowak, S., Myslajek, R. W., Klosinska, A., and Gabrys, G. (2011). Diet and prey selection of wolves (Canis lupus) recolonising Western and Central Poland. Mamm. Biol. 76, 709–715. doi: 10.1016/j.mambio.2011.06.007
Pape, M., and Gaubert, P. (2007). Modelling ecological niches from low numbers of occurrences: assessment of the conservation status of poorly known viverrids (Mammalia, Carnivora) across two continents. Divers. Distrib. 13, 890–902.
Pearson, R. G., Raxworthy, C. J., Nakamura, M., and Townsend Peterson, A. (2007). Predicting species distributions from small numbers of occurrence records: a test case using cryptic geckos in Madagascar. J. Biogeogr. 34, 102–117. doi: 10.1111/j.1365-2699.2006.01594.x
Phillips, S. J., Anderson, R. R. P., and Schapire, R. R. E. (2006). Maximum entropy modeling of species geographic distributions. Ecol. Model. 190, 231–259. doi: 10.1016/j.ecolmodel.2005.03.026
Phillips, S. J., Dudik, M., and Robert, E. S. (2004). “A maximum entropy approach to species distribution modeling,” in Proceedings of the 21st International Conference on Machine Learning, New York, NY, 83.
Proulx, G., and Rodtka, D. (2015). Predator bounties in Western Canada cause animal suffering and compromise wildlife conservation efforts. Anim. Open Access J. 5, 1034–1046. doi: 10.3390/ani5040397
Radosavljevic, A., and Anderson, R. P. (2014). Making better MAXENT models of species distributions: complexity, overfitting and evaluation. J. Biogeogr. 41, 629–643. doi: 10.1111/jbi.12227
Reshamwala, H. S., Shrotriya, S., Bora, B., Lyngdoh, S., Dirzo, R., and Habib, B. (2018). Anthropogenic food subsidies change the pattern of red fox diet and occurrence across Trans-Himalayas, India. J. Arid Environ. 150, 15–20.
Riley, S. J., DeGloria, S. D., and Elliot, R. (1999). Index that quantifies topographic heterogeneity. Intermt. J. Sci. 5, 23–27.
Ripple, W. J., and Beschta, R. L. (2007). Restoring Yellowstone’s aspen with wolves. Biol. Conserv. 138, 514–519.
Ripple, W. J., and Beschta, R. L. (2012). Trophic cascades in Yellowstone: the first 15years after wolf reintroduction. Biol. Conserv. 145, 205–213. doi: 10.1016/j.biocon.2011.11.005
Roy, A. K. Indian Grassland and Fodder Research Institute Jhansi, U. P., and Singh, J. P. (2013). Grasslands in India: Problems and perspectives for sustaining livestock and rural livelihoods. Trop. Grassl. Forrajes Trop. 1:240. doi: 10.17138/TGFT(1)240-243
Sharma, R. K., Bhatnagar, Y. V., and Mishra, C. (2015). Does livestock benefit or harm snow leopards? Biol. Conserv. 190, 8–13. doi: 10.1016/j.biocon.2015.04.026
Sharma, R. K., Sharma, K., Borchers, D., Bhatnagar, Y. V., Suryawanshi, K. R., and Mishra, C. (2021). Spatial variation in population-density of snow leopards in a multiple use landscape in Spiti Valley, Trans-Himalaya. PLoS One 16:e0250900. doi: 10.1371/journal.pone.0250900
Sharma, R. K., Sharma, K., Borchers, D., Bhatnagar, Y. V., Suryawanshi, K. S., and Mishra, C. (2020). Spatial variation in population-density, movement and detectability of snow leopards in a multiple use landscape in Spiti Valley, Trans-Himalaya. bioRxiv [Preprint]. doi: 10.1101/2020.09.09.289181
Sharma, V. P., Köhler-Rollefson, I., and Morton, J. (2003). Pastoralism in India: A Scoping Study, 63. London: DFID.
Shrotriya, S., Lyngdoh, S., and Habib, B. (2012). Wolves in Trans-Himalayas : 165 years of taxonomic confusion. Curr. Sci. 103, 885–887.
Silverman, B. W. (1986). Density Estimation for Statistics and Data Analysis. Boca Raton, FL: CRC Press.
Suryawanshi, K. R., Bhatnagar, Y. V., Redpath, S., and Mishra, C. (2013). People, predators and perceptions: patterns of livestock depredation by snow leopards and wolves. J. Appl. Ecol. 50, 550–560. doi: 10.1111/1365-2664.12061
Suryawanshi, K. R., Redpath, S. M., Bhatnagar, Y. V., Ramakrishnan, U., Chaturvedi, V., Smout, S. C., et al. (2017). Impact of wild prey availability on livestock predation by snow leopards. R. Soc. Open Sci. 4:170026. doi: 10.1098/rsos.170026
Ugarte, C. S., Moreira-Arce, D., and Simonetti, J. A. (2019). Ecological Attributes of Carnivore-Livestock Conflict. Front. Ecol. Evol. 7:433. doi: 10.3389/fevo.2019.00433
Wabakken, P., Sand, H., Liberg, O., and Bjarvall, A. (2001). The recovery, distribution, and population dynamics of wolves on the Scandinavian peninsula, 1978-1998. Can. J. Zool. 79, 710–725.
Wachter, B., Blanc, A. S., Melzheimer, J., Höner, O. P., Jago, M., and Hofer, H. (2012). An advanced method to assess the diet of free-ranging large carnivores based on scats. PLoS One 7:e38066. doi: 10.1371/journal.pone.0038066
Warren, D. L., Glor, R. E., and Turelli, M. (2010). ENMTools: a toolbox for comparative studies of environmental niche models. Ecography. 33, 607–611. doi: 10.1111/j.1600-0587.2009.06142.x
Warren, D. L., and Seifert, S. N. (2011). Ecological niche modeling in Maxent: the importance of model complexity and the performance of model selection criteria. Ecol. Appl. 21, 335–342. doi: 10.1890/10-1171.1
Watts, S. M., McCarthy, T. M., and Namgail, T. (2019). Modelling potential habitat for snow leopards (Panthera uncia) in Ladakh, India. PLoS One 14:e0211509. doi: 10.1371/journal.pone.0211509
Weaver, J. L. (1993). Refining the equation for interpreting prey occurrence in gray wolf scats. J. Wildl. Manag. 57, 534–538.
Werhahn, G., Liu, Y., Meng, Y., Cheng, C., Lu, Z., Atzeni, L., et al. (2020). Himalayan wolf distribution and admixture based on multiple genetic markers. J. Biogeogr. 47, 1272–1285. doi: 10.1111/jbi.13824
Werhahn, G., Senn, H., Kaden, J., Joshi, J., Bhattarai, S., Kusi, N., et al. (2017). Phylogenetic evidence for the ancient Himalayan wolf: towards a clarification of its taxonomic status based on genetic sampling from western Nepal. R. Soc. Open Sci. 4:170186. doi: 10.1098/rsos.170186
Wisz, M. S., Hijmans, R. J., Li, J., Peterson, A. T., Graham, C. H., Guisan, A., et al. (2008). Effects of sample size on the performance of species distribution models. Divers. Distrib. 14, 763–773.
Yackulic, C. B., Chandler, R., Zipkin, E. F., Royle, J. A., Nichols, J. D., Grant, E. H. C., et al. (2013). Presence-only modelling using MAXENT: when can we trust the inferences? Methods Ecol. Evol. 4, 236–243. doi: 10.1111/2041-210x.12004
Zhang, H. H., Liu, X. P., Dou, H. S., Zhang, C. D., and Ren, Y. (2009). Food composition and food niche overlap of three kinds of canidae. Acta Ecol. Sin. 29, 347–350. doi: 10.1016/j.chnaes.2009.09.015
Keywords: Canis lupus chanco, MaxEnt (maximum entropy), scat analysis, livestock depredation, cold desert, Buddhist, Trans-Himalaya, telemetry
Citation: Lyngdoh S and Habib B (2022) Understanding conflict and co-existence among Spiti Bhot community and large carnivores in high Himalaya: The case of Himalayan wolves. Front. Ecol. Evol. 10:739181. doi: 10.3389/fevo.2022.739181
Received: 10 July 2021; Accepted: 24 June 2022;
Published: 22 July 2022.
Edited by:
Yash Veer Bhatnagar, Nature Conservation Foundation, IndiaReviewed by:
Carlos Alberto Lopez Gonzalez, Universidad Autónoma de Querétaro, MexicoMatt W. Hayward, University of Newcastle, Australia
Copyright © 2022 Lyngdoh and Habib. This is an open-access article distributed under the terms of the Creative Commons Attribution License (CC BY). The use, distribution or reproduction in other forums is permitted, provided the original author(s) and the copyright owner(s) are credited and that the original publication in this journal is cited, in accordance with accepted academic practice. No use, distribution or reproduction is permitted which does not comply with these terms.
*Correspondence: Salvador Lyngdoh, c2FsdmFkb3JAd2lpLmdvdi5pbg==; Bilal Habib, YmhAd2lpLmdvdi5pbg==