- 1The College of Geography and Environmental Science, Henan University, Kaifeng, China
- 2Key Laboratory of Geospatial Technology for Middle and Lower Yellow River Regions, Ministry of Education, Henan University, Kaifeng, China
- 3Key Research Institute of Yellow River Civilization and Sustainable Development, Collaborative Innovation Center on Yellow River Civilization Jointly Built by Henan Province and Ministry of Education, Henan University, Kaifeng, China
- 4Center for Computational Geography, The College of Geography and Environmental Science, Henan University, Kaifeng, China
- 5College of Urban and Environmental Sciences, Northwest University, Xi’an, China
- 6Shaanxi Key Laboratory of Earth Surface System and Environmental Carrying Capacity, Northwest University, Xi’an, China
- 7Department of Geography, Hong Kong Baptist University, Kowloon, Hong Kong SAR, China
While urbanization puts lots of pressure on green areas, the transition of green-to-grey surfaces under land use land cover change is directly related to increased land surface temperature–compromising livability and comfort in cities due to the heat island effect. In this context, we evaluate historical and future associations between land use land cover changes and land surface temperature in Dera Ghazi Khan–one of the top cities in Pakistan–using multi-temporal Landsat data over two decades (2002–2022). After assessing current land use changes and future predictions, their impact on land surface temperature and urban heat island effect is measured using machine learning via Multi-Layer Perceptron-Markov Chain, Artificial Neural Network and Cellular Automata. Significant changes in land use land cover were observed in the last two decades. The built-up area expanded greatly (874 ha) while agriculture land (−687 ha) and barren land (−253 ha) show decreasing trend. The water bodies were found the lowest changes (57 ha) and vegetation cover got the largest proportion in all the years. This green-grey conversion in the last two decades (8.7%) and prospect along the main corridors show the gravity of unplanned urban growth at the cost of vegetation and agricultural land (−6.8%). The land surface temperature and urban heat island effect shows a strong positive correlation between urbanization and vegetation removal. The simulation results presented in this study confirm that by 2032, the city will face a 5° C high mean temperature based on historical patterns, which could potentially lead to more challenges associated with urban heat island if no appropriate measures are taken. It is expected that due to land cover changes by 2032, ~60% of urban and peri-urban areas will experience very hot to hot temperatures (> 31.5°C). Our results provide baseline information to urban managers and planners to understand the increasing trends of land surface temperature in response to land cover changes. The study is important for urban resource management, sustainable development policies, and actions to mitigate the heat island effect. It will further asset the broader audience to understand the impact of land use land cover changes on the land surface temperature and urban heat island effect in the light of historic pattern and machine learning approach.
1. Introduction
Land use (LU)/land cover (LC) refers to the classification of landscape according to the natural elements and anthropogenic activities. Collectively, the terms are often used as LULC due to their interrelationship (Hussain et al., 2020; Sadiq Khan et al., 2020; Das et al., 2021). In recent decades, urban sprawl caused a tremendous amount of landscape changes that led to land surface temperature (LST) variation from local to global scales. In the 1950s, the urban area contained only 3% of the world’s population, which boosted to 51% in 2007, and will reach 70% by 2050 (UN, 2017). The massive population influx in urban areas causes the exploitation of resources and economic development (Castelli, 2018), which results in huge impacts on local LULC changes (Thériault et al., 2020; Aktaş and Dönmez, 2021), LST variation (Kafy et al., 2020; Das et al., 2021; Kafy et al., 2021a), seasonal variability (Bera et al., 2022) and regional to global climate change (Zhou et al., 2019; Lustgarten, 2020). This situation often leads to pollution and unequal distribution of resources and services, creating significant challenges for urban developers and policymakers (Lakshmisha et al., 2019; Gan et al., 2020).
Cities are considered the locomotive of development. Development practices across the world have accelerated urbanization (Wang et al., 2020; Korkmaz and Meşhur, 2021). Unplanned urbanization often results in degraded ecology, biodiversity, and landscapes along with several surface changes (Hassan, 2017; Heikkinen et al., 2019). This situation represents negative consequences (both short and long-term) of economic developments in cities. The short-term consequences that urban dwellers face include degraded natural systems, poor sanitation, waste management, and poor air/water quality (Kadhim et al., 2016; Musse et al., 2018; Luo et al., 2019). The increase in LST in cities is one of the long-term repercussions of changes in LULC (Peng et al., 2018; Akinyemi et al., 2019; Kafy et al., 2021a). These LULC transitions from marshes, vegetation, and agricultural lands into the impervious surface are particularly associated with increased LST into urban roots. According to recent studies (Cai et al., 2018; Peng et al., 2018; Kafy et al., 2021a,b), the average LST in cities is typically 2–4°C higher than in rural areas, which is mostly contributed by the removal of green cover and installation of gray infrastructure. In addition, the increase in the impervious surface makes societies more vulnerable to flooding due to the higher flow velocity of the water (Rehman et al., 2022).
The high LST concentration is primarily influenced by both horizontal and vertical expansion (Crum and Jenerette, 2017), the space between buildings, building material (Faroughi et al., 2020; Sadiq Khan et al., 2020; Song et al., 2020), landscape composition, and topographic parameters (Peng et al., 2017; Bera et al., 2022) among other factors. Also, the geographical location and seasonal variations play important roles in increasing LST, resulting in the creation of Urban Heat Islands (UHIs) (Khan I. et al., 2019; Guo et al., 2020; Bera et al., 2022; Tariq et al., 2022a;Mehmood et al., 2023). These geographical locations/places near the equator receive more radiation and thus, are more susceptible to the formation of UHIs, which is directly linked to high energy consumption, air pollution, and risks to human health (Shahmohamadi et al., 2011; Heaviside et al., 2017). For better living conditions, public health, and community well-being, systematic adoption of measures to mitigate the consequences of UHI is desirable.
To measure variations in LULC and LST in cities, geo-information technologies such as Geographic Information Systems (GIS) and remote sensing (RS) are flourishing (Ahmed et al., 2013; Alqurashi et al., 2016; Hassan, 2017; Shen et al., 2017; Tariq et al., 2021). GIS and RS applications have gained much attention in studies related to ecosystem change, biodiversity, and changes in climatic conditions (Mengistu and Salami, 2007; Maurya et al., 2021). Monitoring LST through direct site visits to detect changes in LULC is time-consuming, tedious, labor-intensive, and error prone. Furthermore, the coupling of GIS and RS allows for the practical evaluation, monitoring, and simulation of LULC and LST variations (Samie et al., 2017; Kafy et al., 2020, 2021c). Spatial–temporal modeling of LULC and LST dynamics is also necessary to address the difficulties related to land cover change and temperature rise, thanks to developments in statistical methods using remote sensing data (Wang et al., 2019). Several studies have employed thermal infrared sensors with varying spatial resolutions to study LST properties in diverse urban areas (by LULC categories), which reflects the applicability and utilization of remotely sensed data (Zhou et al., 2019; Tariq et al., 2022b). Another example of RS data is the derivative products of Landsat imageries such as the Normalized Difference Vegetation Index (NDVI) and Normalized Difference Built-up Index (NDBI). These spatial indices are used to identify the spatial–temporal LULC trend and LST variation. Based on the historical trends, climate data along with anthropogenic activities information can be used to seek the prediction of LULC changes and LST variations.
Although some previous studies have focused on the historical changes and future LULC scenarios in Pakistan and worldwide (Bokaie et al., 2016; Heaviside et al., 2017; Ullah et al., 2019a; Amir Siddique et al., 2020; Imran and Mehmood, 2020; Kafy et al., 2020; Sadiq Khan et al., 2020; Tariq and Shu, 2020; Arshad et al., 2022; Bera et al., 2022; Tariq and Mumtaz, 2022; Waleed and Sajjad, 2022; Mehmood et al., 2023; Zafar et al., 2023), research on LULC and LST modeling, particularly under historical and future scenarios, is rare to find. This situation of absence of information on LULC transitions, their association with LST, and future dynamics of both hinder informed planning of urban regions in Pakistan in the face of environmental changes—representing a potential domain of research that requires the attention of researchers. Similarly, the site-specific spatial–temporal insights related to LULC transitions and LST variations are a prerequisite to planning effective measures for livable and comfortable urban designs. It is crucial to examine how urban surface temperature is affected by different spatial patterns of LULC. For urban planners, understanding which kinds of LULC changes exacerbate or mitigate impacts on urban surface temperature can contribute significantly to UHI mitigation strategy. In this context, the present study is focused on Dera Ghazi Khan (hereafter D. G. Khan) city in Pakistan, to explore historical changes and predict LULC scenarios along with their influence on urban LST. This study uses multi-source earth observation data to investigate the temporal and spatial trends of LULC and LST changes in the study area over the past two decades (2002–2022). Further this research enlighten the scientific community and urban managers to design better mitigation strategies to cope the growing trend of UHI especially at city scale. The main contributions of this work are to provide a reliable analysis on LST patterns and the UHI effect along with predicting LULC and LST change and investigating relationships between them, if any. Therefore, the objectives of this work are: (1) to examine the LULC changes and their transitions from a spatial–temporal lens; (2) model LST and its association with spatial indices as NDVI and NDBI; (3) simulate future changes in LULC and LST for 2032; and (4) explore the impact of different LULC changes on LST and formation of UHI effect.
1.1. Study area
The study area is situated in the southwestern district in Punjab province at the foothill of the Koh-e-Suleiman mountain range (Suleiman Range) (Munir and Iqbal, 2016). The city has gone through a population increase of ~110% between 1998 and 2017 (FBS, 2017). To accommodate this population, the city has also gone through an immense urbanization process, resulting in several challenges for city dwellers (Garcia et al., 2019). Geographically, the D. G. Khan district is the third largest district in the Punjab province in terms of area (13,740 km2). The city area lies between 30° to 30° 05/ 28// N Latitude and 73° 35/ 33// to 73° 41/ 41// E Longitude, which covers ~100 km2 (10,000 hectors) (Supplementary Figure S1, a separate file containing additional information). In terms of demography, D. G. Khan district is the most populous in the Southern Punjab, with ~3 million people and the city has 0.4 million urban dwellers as per the recent census in 2017 (FBS, 2017). The climate of the study area defines the arid zone with average annual precipitation of about 150 mm mostly in the summer monsoon season July–August. The average temperature range from cold-mild winter and hot summer shows a huge difference from 13 to 50°C (Munir and Iqbal, 2016). The landscape is characterized by a flat city center and gradually increasing topography towards the west.
The study area received a large population from the surrounding less developed areas in the past two decades mainly due to better quality of life, health care, education, and security reasons. Also, an influx of migrants from rural areas after the destructive flood event in 2010 brought significant changes in the existing LULC pattern (Hashmi et al., 2012). The study area was selected due to its geo-strategic location as it it situated almost in the center of the country and provide access to three provinces (i.e., Punjab, Sindh, and Baluchistan). Due to its significant international economic importance, the city is now a part of the China-Pakistan Economic Corridor (CPEC) under the One Road One Built project. The basic aim is to identify the current LULC transitions along with LST variation to measure the UHI effect. The prospect based on the historical trend analysis at the city scale will allow an understanding of the current situation to implement the right decision and policies for sustainable resource management and urban development.
2. Methodology
2.1. Data Collection and Pre-processing
For this research, the study period is considered as the years 2002, 2012, and 2022. In these two decades, three Multi-spectral Landsat Satellite data (for the years mentioned) are downloaded from the United States Geological Survey’s (USGS) Earth Explorer archives to evaluate LULC changes and their association with LST. The Landsat 5 Thematic Mapper (TM) data are used for 2002 and 2012, and the Landsat 8 Operational Land Imager (OLI) data are used for 2022. All the data are retrieved for March to prevent any influences of seasonal variations. Cloud coverage is set to a minimum scale of <10% for all Landsat images. However, it is notable that the cloud cover is nearly 0 % for all the used images of the study area. Additional details (metadata) regarding the images were retrieved from the USGS repository. Detailed information about the images is provided in Table 1.
2.2. Classification of LULC and change detection
The Image Classification tool in ArcGIS 10.5 is used to classify the LULC map due to its straightforward processing approach and simple-to-use nature with higher accuracy in terms of LULC classification. Following other research in this field, we use the supervised classification based on the maximum likelihood technique–one of the most applied techniques for LULC classification. Under the supervised classification, the images are classified using spectral signatures that are provided through training samples. Landsat-5 TM imaging bands 1–5 and band-7 are utilized to classify different land use classes. It is noted that band 6 is rejected since it represents a thermal band. In the case of Landsat-8 OLI imagery, bands 1–7 are employed. To create the LULC maps, all bands are initially stacked in ArcGIS 10.5 software using the Image Analyst Tool. To attain the accurate LULC classification, Landsat surface reflectance-derived spectral indices, such as the Normalized Difference Vegetation Index (NDVI) and Normalized Difference Built-up Index (NDBI) were utilized (Tariq et al., 2020; Andrade et al., 2021; Das et al., 2021). Later, the Training Sample Manager tool is employed to detect the pixels’ signatures. The training samples for the supervised image classification are collected randomly across the entire study area and 150 samples (30 samples per LULC type) were collected for each year. A total number of five LULC types were identified and detail of each LULC type (see Supplementary Table S1) and their associated classes are explained in section 3.1.
2.2.1. Accuracy assessment of classified maps
The confusion matrix, also known as the error matrix, is used to measure the accuracy of the classified maps. The pixel’s actual and expected identity information is explained in this matrix as suggested by (Tilahun and Teferie, 2015; Rwanga and Ndambuki, 2017; Rehman et al., 2021). For this purpose, a total of 210 sample locations are selected using the stratified sampling tool in ArcGIS for equal representation of all LULC types. Later, these sample locations act as ground truths to verify the classified maps. These samples are collected using the Google Earth platform coupled with actual ground conditions of LULC via the Global Positioning System (GPS) for the recent year (2022). The 2002 ground truth data were also acquired from the Land Cover Atlas of Pakistan-The Punjab Province series which is prepared by the collaboration of The Space & Upper Atmosphere Research Commission (SUPARCO) and the Food and Agricultural Organization (FAO) due to lower resolution image data of Google Earth before 2005. These samples are cross-examined with the classified maps to estimate the accuracy. The producer’s accuracy (PA) is a referenced-based approach in which the accuracy is calculated by analyzing the forecasts provided for a class and expressed as a percentage. Besides PA, there is another type of map-based accuracy measure known as user accuracy (UA). This measure is calculated by analyzing a class’s referenced data and expressed as a percentage (Platt and Rapoza, 2008; Shafi et al., 2023). Aside from the approaches mentioned above, another commonly adopted accuracy coefficient is the Kappa coefficient. Due to its wide use in LULC classification, we use the Kappa coefficient in our study to estimate the accuracy of classified maps. The Kappa value varies from 0 to 1, with 0 denoting low agreement and representing 1 nearly perfect agreement between ground truths and the classes obtained through the classification of images (Foody et al., 1992).
2.3. Retrieval of land surface temperature
The LST is calculated by employing the thermal bands of radiometrically and geometrically corrected earth observation data from Landsat satellite during 2002–2022 for the periods 2002, 2012, and 2022. Thermal data are retrieved from the Landsat sensors and stored as Digital Numbers (DN). Later, these DNs are transformed into LST using a four-step procedure described by (Artis and Carnahan, 1982).
Step 1 involves the conversion of DNs values to radiance utilizing the following Equation (1):
where.
L represents spectral radiance for both Landsat 5 TM and 8 OLI images;
LMIN for Landsat 5 TM is 1.238 and 0.10033 for both thermal bands (Bands 10 and 11) of Landsat 8 OLI image.
LMAX represents the Landsat 5 TM as 15.30 and 22.00180 for both thermal bands (Bands 10 and 11) of the Landsat 8 OLI image.
Step 2 follows the conversion of the radiance above to satellite brightness temperature (BT) at sensors into degree Celsius as Equation 2:
Here, K1 and K2 reflect calibration constants given as 607.76 and 1260.56 accordingly for Landsat 5 TM. For Landsat 8, OLI images of K1 and K2 (both bands 10 and 11) are given as 774.89, 1321.08, and 480.88, 1201.14, respectively. The information on these constants is available from the USGS through the metadata files of the satellite images.
Step 3 involves the final step to calculate the LST by utilizing the following three Equations 3:
in which, LST reflects land surface temperature; TB represents satellite brightness temperature; represents the wavelength of emitted radiance with a peak discharge of 11.5 μm; can be calculated by applying the following Equation (4), and offers the ground surface emissivity that can be calculated from proportion vegetation (PV) using Equation 5.
where.
h is Planck’s constant (6.626∗10–34 J s), σ is Boltzmann constant (1.380649 ∗10–23 J/K), and c = velocity of light (2.998∗108 m/s).
Further,
PV represents vegetation proportion calculated from the normalized difference vegetation index (NDVI) for all the respective years (2002, 2012, and 2022). Finally, the surface emissivity ( is calculated using Equation 6.
The LST drives from Landsat 8, bands 10, and 11 are then averaged to obtain the cumulative LST composite image using the Cell Statistics Spatial Analyst tool extension in ArcGIS 10.5 software.
2.3.1. Land surface temperature classification in relation to LULC changes
To determine the relationship between LST and land cover changes over the past 20 years, if any, the LST is categorized into six different zones to better understand the temporal and spatial differences. The selection of LST threshold values to visualize clusters/zones, past literature, expert opinion, and study area characteristics were considered. We categorize the LST zones as very cold (<21.1°C), cold (21.1-<23.1°C), chilly (23.1-<25.1°C), cool (25.1–27.1°C), warmish (27.1–29.1°C), hot (29.1-<31.1°C), and very hot (> 31.1°C) (Utomo and Kurniawan, 2016). After the classification, each LST zone is overlaid on LULC change maps, and LST variations are calculated using the “Tabulate Area” tool in ArcGIS 10.5.
2.3.2. Land surface temperature in relation to spatial indices
Indices such as the Normalized Difference Vegetation Index (NDVI) and the Normalized Difference Built-up Index (NDBI) have been linked to LST (Guha et al., 2019). The NDVI characterizes vegetation phenology as the difference between near-infrared and red reflectance (Wessels et al., 2011; Mas and Soares de Araújo, 2021). The following procedure (Equation 7) is used to extract the NDVI value (Townshend and Justice, 1986).
where NIR represents the Near-infrared band and R is the Red band of the satellite image. Landsat TM bands 4 and 3 are utilized to derive NDVI, while Landsat OLI bands 5 and 4 are used. The NDVI scale runs between −1 to +1, with a negative value of NDVI indicating water whereas positive NDVI indicates vegetation.
Except for NDVI, LST is significantly influenced by impervious surfaces. Hence, we also consider this parameter and represent it using the well-known NDBI. The following equation (Equation 8) is used to calculate NDBI, as given by (Zha et al., 2003).
where,
SWIR represents the Short-wave Infrared band and NIR is the Near-infrared band of satellite images.
Utilizing the NDBI, we can determine the built-up areas using remotely sensed data. For Landsat TM and Landsat OLI, bands 5 and 6 are utilized for SWIR, respectively. Similarly, bands 4 and 5 are utilized from Landsat TM and Landsat OLI images for NIR, respectively. Similar to NDVI, the NDBI values lie between −1 to +1, with values closer to −1 reflecting lesser built-up and values closer to +1 showing a high density of built-up areas.
The association between LST and spatial indices is initially determined using single and multiple linear regression analysis (Tran et al., 2017; Das et al., 2021). For each point data type, values for NDVI and NDBI are retrieved from each pixel in the study area. The linear regression model is fed with these data points as input. This model depicts the correlation/association between LST and LULC, if any, in broader terms.
2.4. Simulating LULC projections for the year 2032
Given the fact that LULC is spatially dynamic, their simulation necessitates the use of spatial techniques for guaranteeing long-term sustainability and addressing current and future development needs. By combining GIS and RS methodologies, precise spatial models such as the Multi-layer Perceptron-Markov Chain (MLP-MC) have been developed to properly simulate future LULC (Hamad et al., 2018; Kafy et al., 2021b). Based on the MLP-MC, the MOLUSCE tool in QGIS and the cellular automata (CA) model are used to forecast future LULC changes. The CA model, for example, accounts for both aspects (i.e., static and dynamic) of LULC changes, which are essential for detailed insights related to urban planning and design (Ullah et al., 2019a; Kafy et al., 2021c). Another reason to utilize this model is its well-document accuracy to project future land cover patterns (Xu et al., 2016) based on a set of the function of five-parts transition rules on the status of cells to simulate and predict the future LULC changes (Wang et al., 2020).
The projection is made using two sorts of data: dependent variables like the previous LULC changes estimated (transitional matrix) from Landsat images of 2012, and 2022 and independent variables including elevation, slope, distance from activity centers, distance from waterways, and proximity to road networks. The distances to activity centers, waterways, and roads are estimated using the Euclidian distance function in ArcMap software. Furthermore, the ALOS-PALSAR DEM (12.5 × 12.5 meters spatial resolution) is employed in ArcMap software to estimate the elevation and slope of the study area. The up-scaling procedure with mean aggregation rules (resampling function in ArcGIS) was used to homogenize the spatial resolution of DEM and Landsat images. The transition potential matrix is created using the aforementioned variables. A random sampling approach is used to collect the samples of LULC. The maximum iteration (1000) and neighborhood pixel (9 cells) are selected to automatically train the prediction model (Mansour et al., 2020). After modeling the transition potential matrix with the logistic regression, the CA model creates a future LULC map (for 2032 in our case). Validation of the model using current datasets is required to show that it is trustworthy in projecting LULC change for a specific forecasted year (Ruben et al., 2020; dos Santos et al., 2021). Therefore, before the projection for 2032 is made, the CA model is tested for accuracy via simulating LULC for 2022 and then compared to the study area’s estimated LULC (based on Landsat data) for the same year. The QGIS-MULUSCE validation module is used to calculate the overall kappa coefficients and percent-correctness of 2022 classified and projected LULC maps. Once a reasonable accuracy is determined, the model is finally used for the 2032 LULC prediction.
2.5. Simulating LST projection for years 2032
Under the global warming situation, increased LST in metropolitan areas is a matter of serious concern for urban planners and decision-makers as it would impact energy demands, compromise livability in cities, and has potential health-related challenges (Li et al., 2017). With the advancement in artificial intelligence and machine learning-based modeling approaches, it has now become possible to spatially simulate climate-related indices such as LST with higher confidence. For instance, a Multi-layer Feed-Forward Back-Propagation Artificial Neural Network approach in TerrSet software (Maduako et al., 2016) is used to model, simulate, and project future LST patterns (Maduako et al., 2016; Ullah et al., 2019a). The Multi-Layer Perceptron (MLP) neural network decides network parameters and how they should be updated to automatically model the networks (Dey et al., 2021). This MLP algorithm is based on the concept of error-corrective learning. When a network gets a pattern, the MLP analyzes it and a potentially less accurate random output is generated. Furthermore, it computes a self-computed error function by subtracting the random output from the intended (goal) output. Using the “Leveraging back-propagation” algorithm, correction weights are estimated between the output and hidden layers as well as between hidden and input layers (Kafy et al., 2021c). This iterative approach is repeated until a reasonable and acceptable error is achieved between the network and the desired output.
In this study, LST simulation is based on the LST data from 2002 to 2022. Using QGIS software, the study area is subdivided into 500 × 500 meters spatial grids (fishnet) to obtain the sample points. The said grid size is set with the minimum spacing range in mind where features of one point can significantly influence LST. The LST and LULC data from previous steps are utilized for the training of a Neural Network in Terrset in order to predict LST (Vinayak et al., 2021). Additionally, we input the information on the latitudes and longitude of the defined samples to improve the model efficiency considering the notion; the more the input parameters, the better the network model’s efficiency. The process of LST prediction includes network building ➔ network training ➔ network performance evaluation ➔ and prediction. The confidence of the network is determined through the Mean Square Error (MSE) and the correlation coefficient (R).
The regression analysis offers information on how well the target data set describes the variance in the output results. The closer the value of R is to 1, the perfect correlation between the output and target data is expected. The Graphic User Interface (GUI) is created to test the performance indication before implementing the network. After the network’s performance metrics are reasonable, they are retained for prediction. During the evaluation, the values of R and MSE are reported as 0.8 and 0.5, respectively. Thus, LST for 2032 is simulated using the call-back function. The number of hidden layers is determined by numerous experiments depending on MSE and R values. These hidden layers are significant because they influence the outcomes by allowing the network to display non-linear behavior. Three hidden layers were chosen for the current study (Mallick et al., 2021). We use 0.1 as the starting learning rate and control it using the decay rate. To update the learning rate, the decay rate of 0.9 is used. If the error function between the current and previous iterations increases, the learning rate is upgraded by division. Similarly, it is multiplied when the error function decreases to recede (Kazemzadeh-Zow et al., 2017; Talukdar et al., 2020; Alqadhi et al., 2021).
3. Results
3.1. Spatial–temporal heterogeneities in LULC
Two precise patterns are observed in terms of LULC classification (Figure 1A) and the statistical results are detailed in Table 2. As per the analysis, a constant increase in urban areas and water bodies is observed at the cost of vegetation and bare lands. For instance, ~37% of the land in 2002 was urban, which increased to 40% in 2012, and 45.7% in 2022 (Figure 1B). It is noted that the consistent observed increase in water bodies from 2002 to 2022 (0.7–1.3%) is due to the construction of a new project called the “Kachhi Canal.” This project is a part of a government initiative for Baluchistan province to provide water for drinking and agriculture purposes (Yasin and Nabi, 2014; Khan, 2018). The study area is a significant part of this project. The visual interpretation of this project can be observed in Figure 1A (2022 image), where a new canal suddenly appeared parallel to the old, constructed Ghazi Canal. A gradual downfall is observed in vegetation areas as 58.6% of vegetation land cover in 2002 decreased to 56.5% in 2012 and 51.9% in 2022. The amount of bare land was reduced to 1.2% in 2022, almost half of the amount in 2002 (3.7%).
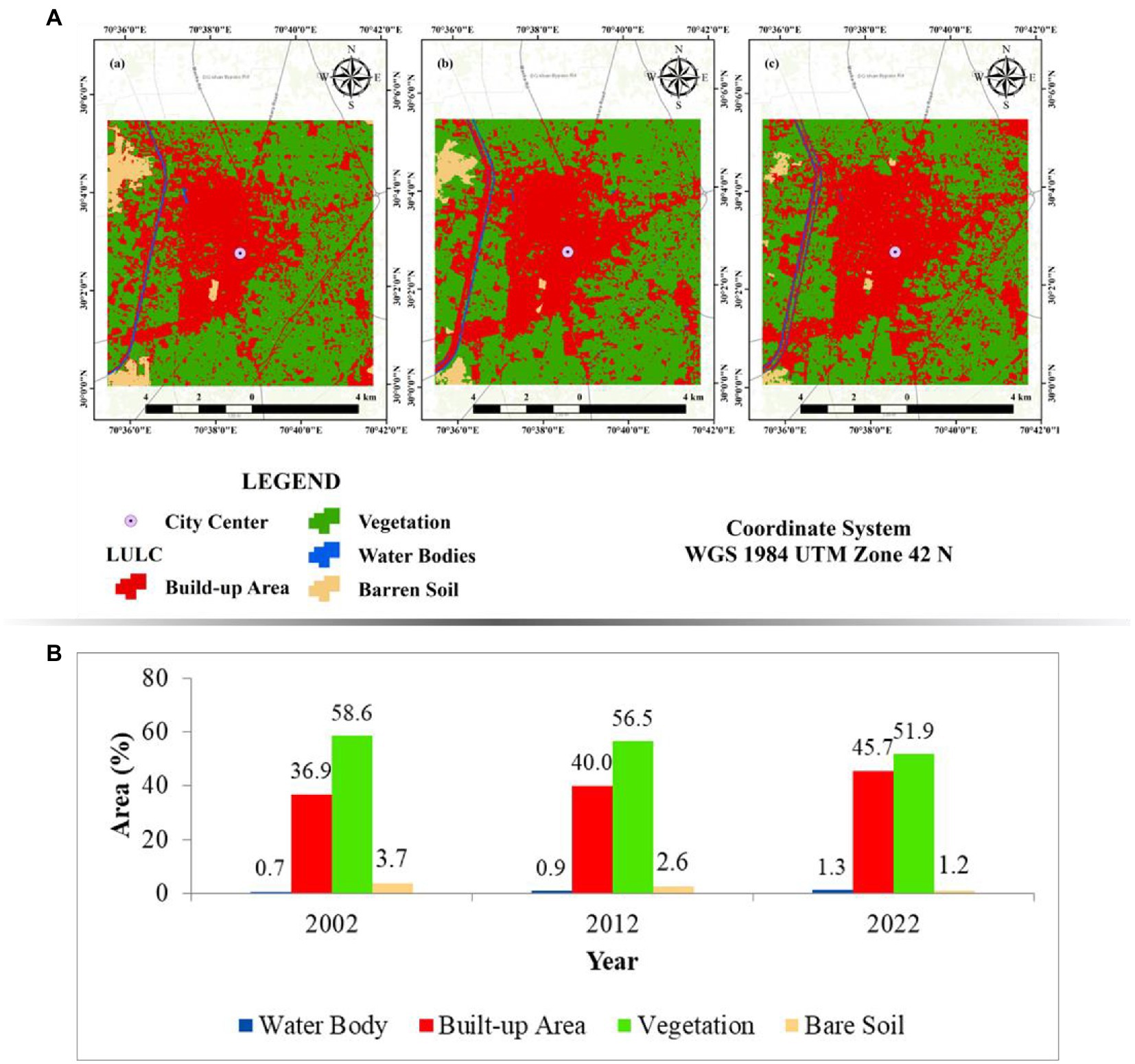
Figure 1. (A) Land use land cover (LULC) maps of Dera Ghazi Khan City from 2002 (left), 2012 (center), and 2022 (right). (B) Area percentage of different LULC types from 2002, 2012 and 2022.
Moving forward, Supplementary Figure S2 shows the graphical representation of the year-to-year land cover transition in relation to positive and negative changes. Two clear patterns are a constant increase in the urban areas as in 2002–2012; almost 3% of the area was transformed into urban areas. Also, 2.1% of vegetation land decreased and transitioned to urban areas and other land use. In the next decade (2012–2022), 5.7% of the land cover transformed into urban areas with a decrease of 4.7% in vegetation land and a 1.1% decrease in bare land. Analyzing the two-decade scenario, 8.7% of the total land was converted to urban areas resulting in a vast increase in built-up areas and a 6.8% decrease in vegetation cover along with a 2.5% decrease in bare soil. As for the water body, a small amount of increase is observed across the overall study period. For 2002–2012, a 0.2% increase in water bodies can be seen, which accelerated to a 0.4% increase in the 2012–2022 period. Overall, a 0.6% increase is estimated in water bodies during 2002-2022–see Table 2 for detailed statistics.
One of the important aspects of LULC classification is its reliability and accuracy. Detailed accuracy assessment is performed by picking 210 ground truth points (GTP) for all years. Multiple sources were used to seek the accuracy assessment and their detail is given in section 2.2.1. Table 3 shows the detailed assessment scenario of the LULC classification. The estimated Kappa Coefficient is higher than 0.90 for each year. Similarly, the Overall accuracy is also higher than 90% for all the years, whereas it almost reached perfection in 2022. However, the producer’s accuracy is low for Bare Soil for 2002 (56.25%). A possible justification for this low producer accuracy is the limited exposure area for sample collection but in the recent decade, it shows satisfactory results as 81.82% (2012) and 81.30% (2022), accordingly. All other accuracy level is more than 90%. The most corrected classification was recorded for water bodies for almost all the periods, which reached 100% for the producer’s accuracy scenario in 2022. This situation shows the reliability of the LULC classification results from this study.
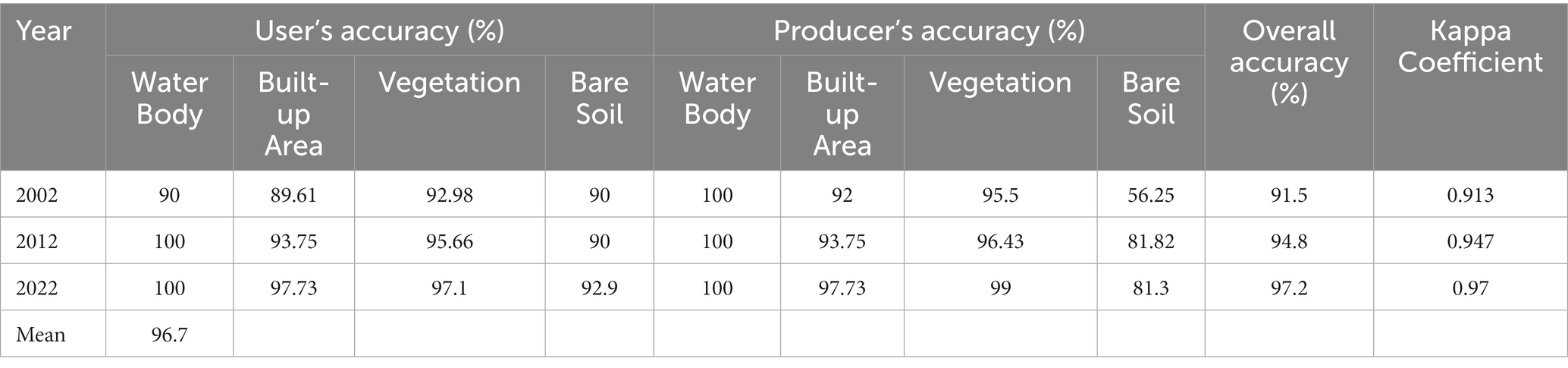
Table 3. Detail of all LULC classification accuracy assessments from 2002, 2012, and 2022 Landsat images.
3.2. Spatial–temporal heterogeneities in LST (2002–2022)
As the urban areas increased, so did the study area’s temperature in the last two decades (Figure 2A). In 2002, a meager amount of hot areas is observed in the study area. Major areas are covered in very cold to chilly class (<21.1-<25.1). Around 33% of the area is covered in a very cold region. Nearly 2% of the area lies in the hot region. However, this situation changed in 2012 as the maximum temperature in the study area raised to 33.096 ° C from 30.896 ° C—approximately 3° C higher than in the year 2002. Similarly, the mean temperature increased by approximately 2° C, but the standard deviation remained the same (Table 4). About 40% of the area falls into the chilly region, and around 20% falls into the cold region. However, in 2012, the very cold regions consisted of only 3% of areas, approximately decreasing from 33% in 2002. Some areas in the 2012 scenario also fall into a very hot region. In 2022, the maximum temperature increased at the same rate. The highest temperature recorded was 36.661°C in 2022, having the same increasing trend as 2002–2012. Despite this, the mean temperature increased at an alarming rate. Almost a 7° C increase is observed in the 2012–2022 LST scenarios. This situation shows no area falling into cool, chilly, cold, and very cold regions. Most areas in 2022 are covered with “very hot” and “hot” regions (85% approximately). Nearly 11% of the area is covered with warmish regions. These results show an overall increase in the mean, minimum, and maximum LST in the study area—which should be a matter of serious concern for the relevant authorities. The rate of average annual LST change for the study area is noted as 0.3° C.
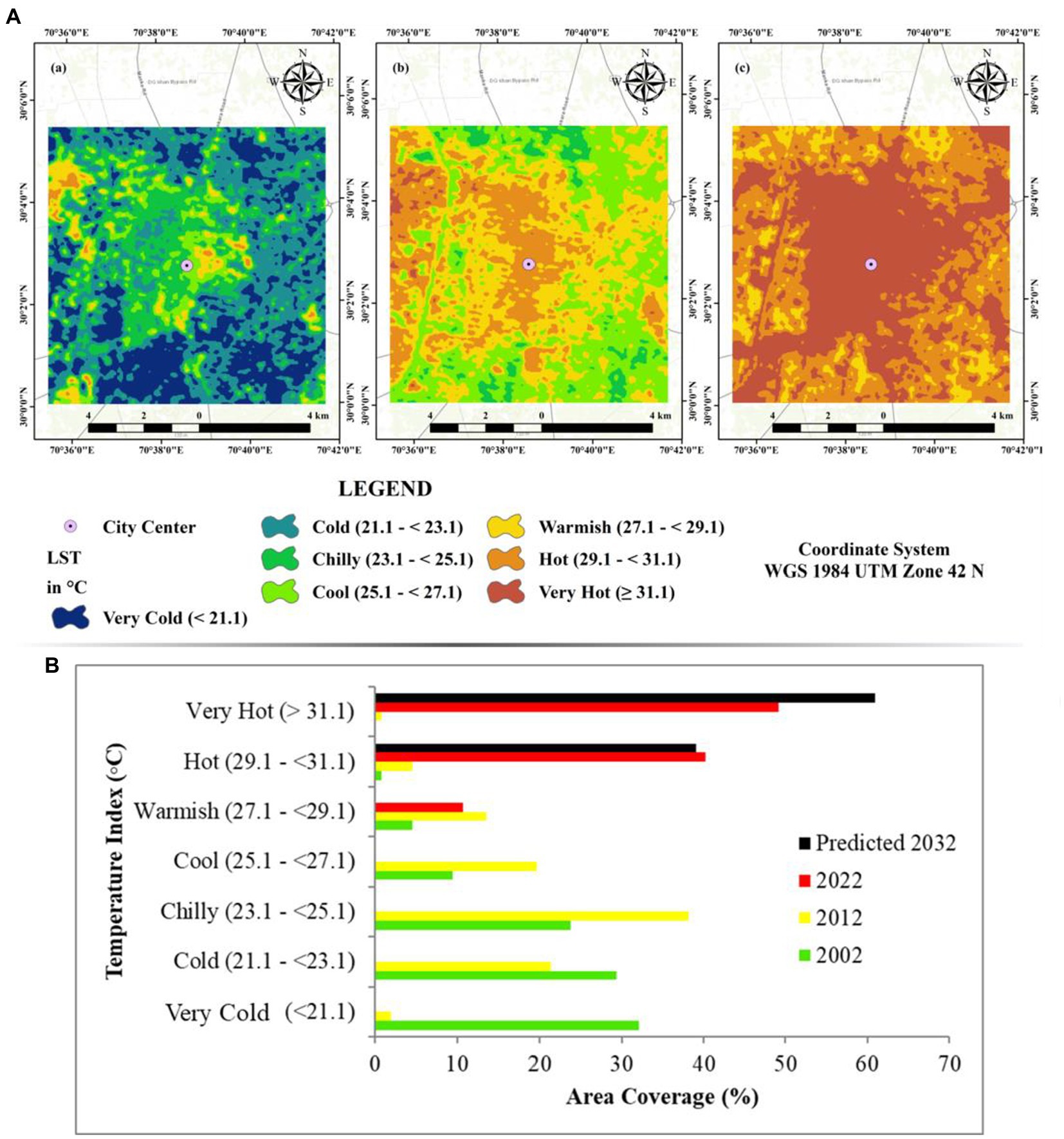
Figure 2. (A) Spatial Distribution of LST Change in Dera Ghazi City from 2002, 2012, and 2022. (B) Spatial distribution of LST index with percent area coverage in D. G. Khan City from 2002 to 2032.
The prediction of LST for 2032 shows that an additional 5° C increase in maximum and a 4° C raise in the mean temperature is expected. Under these circumstances, the study area is expected to consist of very hot and hot regions mostly. By 2032, nearly 60% of areas in the study area are expected to be in the “very hot” class along with 40% of areas in hot regions (Figure 2B).
3.2.1. LULC-wise LST discrepancies
As expected, the lowest temperature in comparison with other land use classes is recorded for water bodies over 2002–2022 and in the predicted scenario for 2032. Where 23°C is the mean temperature in 2002 for water bodies, the value remained almost the same in 2012. In 2022, however, the value jumped to 33°C (Figure 3). The predicted scenario also recorded nearly a similar situation for water bodies in 2032. For the built-up areas, the mean temperature gradually increased throughout the study period. For instance, in 2002, the mean temperature is estimated as 23°C, which increased to ~25°C in 2012. However, in the next decade, the mean temperature increased to more than 30° C, which eventually became nearly 38°C in the predicted scenario of 2032. This situation represents that the temperature for the built-up areas is the highest as compared with other land use types–which is expected due to an increase in impervious surface. On the other hand, the mean temperature for vegetation cover is observed at ~22° C in 2002, which gradually increased to 25°C in 2012, and nearly 30°C in 2022. For the simulated scenario, the mean temperature is predicted as 35°C in 2032 (Figure 3). Lastly, bare soil shows the highest mean temperature in almost all the years, even in a predicted scenario. In both 2002 and 2012, the mean temperature of bare soil land cover is approximately 26° C. In 2022, the temperature reached 33°C, and it is predicted to reach 35°C in 2032. Overall, there has been an increase in the mean temperature for almost all of the LULC types during the study period, and this increasing trend is more likely to remain the same in the future without proper measures.
3.3. Exploring connections among LST, NDBI and NDVI
NDBI is used to identify the human settlements in land cover with few other essential features like roads, dams, canals, etc. (Kafy et al., 2019). A value of NDBI closer to +1 indicates land use covered with man-made features like buildings, roads, etc. Conversely, the values relative to −1 represent natural features like a forest, water body, vegetation, etc. Based on our assessment, an increasing NDBI index is as the value approached gradually +1 in the urban areas (Figure 4A). As for 2002, the maximum value for NDBI was closest to 0.35, which increased to 0.38 in 2012 and reached 0.43 in 2022. The increased value of NDBI in the central region of the study area represents a major urban expansion during the study period.
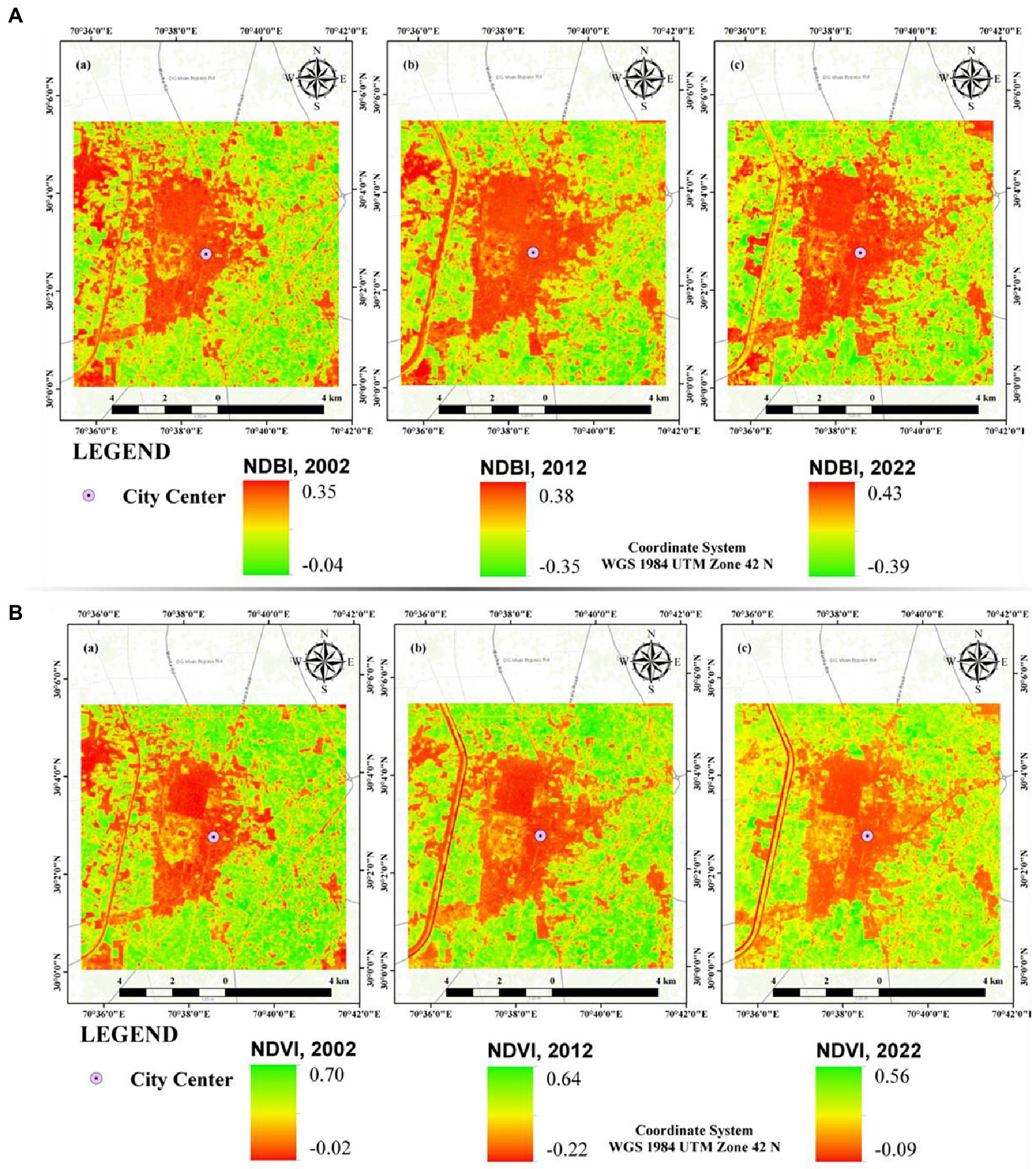
Figure 4. (A) Spatial pattern of NDBI in Dera Ghazi Khan City. (B) Spatial pattern of NDVI in Dera Ghazi Khan City. It is noted that the left, center, and right figures in both panels represent 2002, 2012, and 2022, respectively.
As for the NDVI, a completely mirrored scenario can be seen. For instance, the variation of NDVI values can be analyzed based on the radiation absorbed by the red spectral area chlorophyll and reflectance near the infrared spectral area of NDVI the more vegetation there will be. However, Figure 4B shows a different scenario as the value is decreasing towards −1. A gradual increase of urban areas at a cost of vegetation removal can be seen in a gradual decrease of NDVI values, as the maximum value for 2012 was reduced to 0.64 from 0.70 for 2002. In the recent decade (2022), the NDVI value decreased to 0.56. A similar pattern is observed for the minimum value too. In the 2002–2012 period, the minimum value of NDVI decreased by about 0.20 percent.
The relation between LST and NDVI for the study area during the last decade shows a disproportionate relation. In 2002, the highest concentration of NDVI values was between the 25 to 30°C range, with a low value of −0.2 to 0.2 (Supplementary Figure S3). In 2012, a different scenario was seen. The value was not concentrated in a specific range but equally distributed in all LST ranges, having the highest value of 0.6 in the 21–25°C LST range. In 2022, higher values of NDVI (0.6–0.3) were seen in the low value of the LST range (27–29°C). The overall scenario shows that the lower the value of NDVI, the higher the value of LST in the study area. The goodness-of-fit for all the linear relationships is well observed by higher values of R2 (Supplementary Figure S3).
The relation between LST and NDBI shows the corresponding scenario of LST and NDBI. An evaluation of the linear relationship between NDBI and LST shows a positive association between both (Supplementary Figure S3). This implied that the LST is expected to rise in the study area with an increase in NDBI. Similar to NDVI, there is high confidence in this association as reflected by higher goodness of fit values for all the periods (0.83, 0.85, and 0.89 for 2002, 2012, and 2022, respectively). In 2002, the NDBI pointed to having a higher value of 0.1 to 0.4 concentrated in the LST region of 25–30°C. The same scenario can be seen in the next decade as the higher valued NDBI points (0.2–0.4) were in the LST range of 27–33°C. In 2022, the scenario remained the same, but the value changed considerably (Supplementary Figure S3).
3.4. Drivers of LULC and LST changes
Five driving forces are selected to predict the LST and LULC changes for the year 2032. As for the elevation (Figure 5A), the highest peak was seen in the western part of the study area with a maximum of 94 m. The elevation scenario was gradually decreasing value in the eastern region. A maximum of 15° slope was seen in the study area (Figure 5B). Buffer distances for waterways and road distances are essential to simulate the urban areas in the study area. As for the city center, the urban expansion region was determined by anthropogenic activity centers (Figure 5C). Panels d and e (Figure 5) show the buffered distance covered by the waterways and road distance, respectively, where a low value derives that this area will remain unchanged for at least 2032.
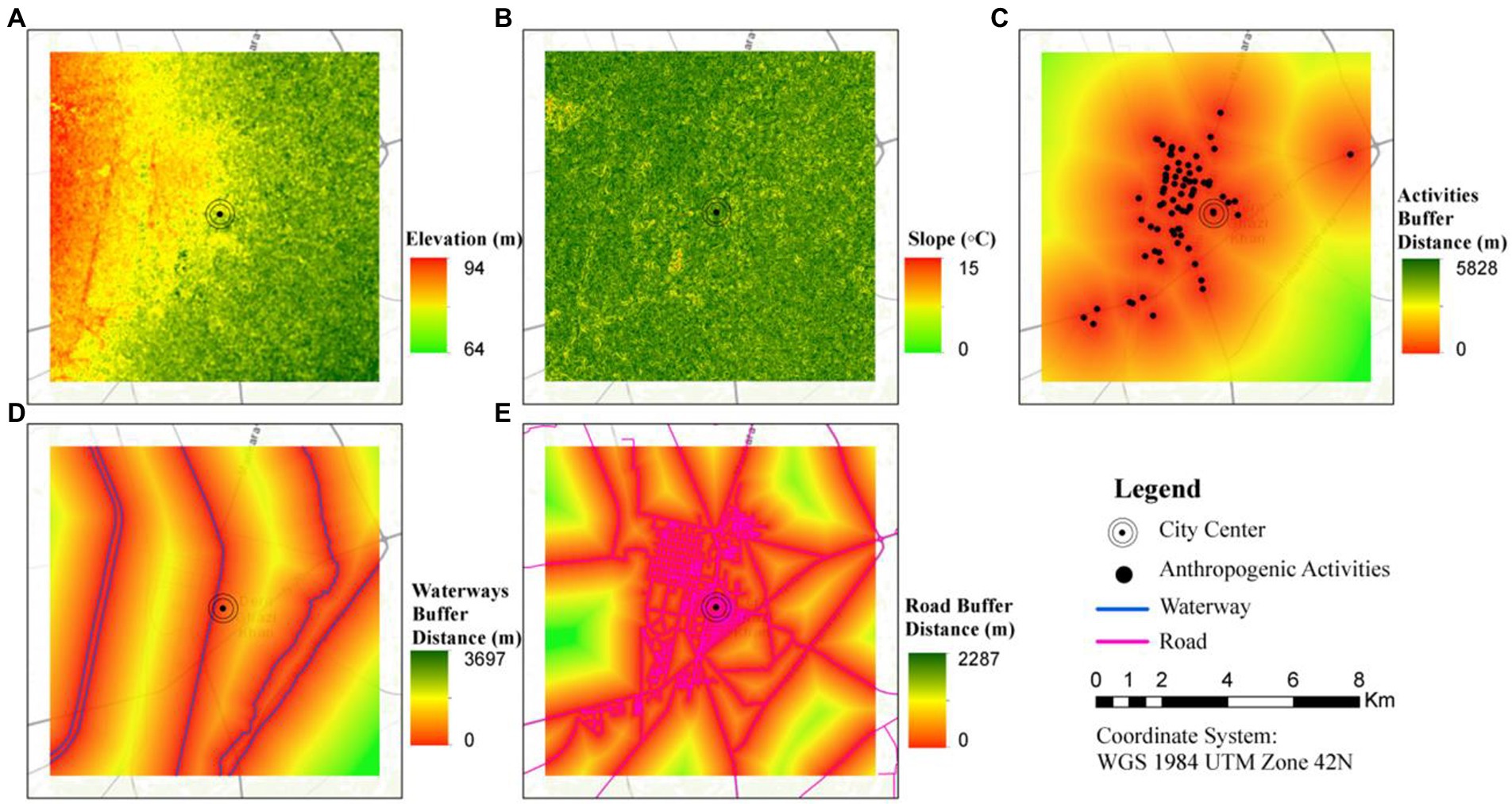
Figure 5. All drivers for predicting the LULC and LST change for the year 2032. (A) elevation, (B) slope, (C) anthropogenic activities buffer, (D) waterways buffer, and (E) road buffer.
The predicted LULC and LST are illustrated in Figures 6A,B, respectively. According to the simulation results, the study area will go throw a tremendous amount of changes in the built-up area at the cost of green areas. Similarly, the LST predicted a high amount of temperature in the city center and its peripheries. The uncontrolled urbanization and increasing trends of LST in the study area should be a potential concern for urban managers, city planners, and urban dwellers. The areas where the predicted LST is comparatively high (i.e., very hot zones represented by black shades in Figure 6B) should be prioritized for measures to mitigate the heat island effect.
4. Discussion
Sustainability in the long-term context requires informed planning and management in the face of environmental changes through space and time. In an anthropogenic activities-based climate change-induced warming world, rapid urbanization-led impervious surface in cities leads to land resource degradation and scorching heat, which creates challenging conditions in terms of health issues and energy use among many others 1. Evaluating drivers of LULC changes, its association with LST, and their simulation for future decision-making is a progressive approach to tackling related climate change impacts. The present study not only effectively explore the historical patterns in LULC and LST, but further goes ahead one step to simulate future expected LULC patterns and LST situation using machine learning, artificial intelligence, and spatial modeling techniques (e.g., Figures 1, 2, 4, 6). The observed changes indicated a constantly increasing trend of built-up area and a consistently decreasing trend of green areas at a pace of 8.7 and 6.8%, accordingly, from 2002 to 2022, which is in line with existing studies in different parts of the world (e.g., Alqurashi et al., 2016; Das et al., 2021; Waleed and Sajjad, 2022). Such findings have particular implications to plan and strategize action plans for sustainable resource use particularly the land resource in cities. While the findings are useful in the elaborated context, similar studies are recommended in other regions beyond the study area in Pakistan to have a broader understanding and provincial and national scale policy on LULC (i.e., no-net-loss of green cover). In terms of LULC classification using the supervised- maximum likelihood classification, the accuracy assessment results (Kappa’s Coefficient) found almost perfect agreement as >90% as compared with the Bera et al. (2022) (87%), Das et al. (2021) (88%), Kafy et al. (2021c) (96%) and Hussain et al. (2019) (> 80%).
Notably, the urban fabric in the city area increased by 874 ha with high intensity towards the main corridors in the northeast to the southwest direction (Figure 1A). The haphazard urban expansion and messy development in the study area’s center increased LST and spotted the urban heat island effect (Figure 2A). Due to the development planning-related negligence, the city has only one older park and fewer green spaces constructed in recent years, which provided fewer opportunities to lower LST. On the other hand, several large-scale commercial activities and services are distributed in the urban center adding more impervious surfaces to the urban regions of the city. In the future, the introduction of modern integrated gray-green structural development along with initiatives to preserve vegetation cover could potentially compensate for the lack of green areas in the study area leading to lower LST. Except for the central dense urban regions, the peri-urban areas comprised the newly developed housing societies and industrial areas where green spaces are unevenly distributed. The removal of trees and agricultural land, no insertion of new green spaces, and construction are among the critical issues that lead to the rising temperature and, ultimately, the heat island effect (Figure 2). The future scenario depicts the worst scenario if proper planning and mitigation measures are not followed promptly. Hence, evaluations as such are of high importance to identify priority intervention areas for immediate or gradual actions in the context of climate change adaptations in the study area and beyond.
The historical city of D. G. Khan was the first of its kind gridded planned city in 1911 (Garcia et al., 2019) in the British colonial period where each grid (Block) is interlinked with roads and a community gathering space in the block center with proper green space. The Tehsil Municipal Authority (TMA) autonomously governed the city planning and developmental activities and was responsible for a better quality of life. After the independence on 14th August 1947, district D. G. khan and its historical city were neglected, and unplanned developmental activities were started due to improper resource allocation and the absence of any effective urban land use policy in Pakistan (Arshad et al., 2022).
In 1970, a flux of labor migration toward the middle east countries (e.g., the United Arab Emirates and Saudi Arabia) (Azhar, 2008), and flood events in 1992, 1998, 2010, 2014 and 2022 (Ahmed et al., 2014; Munir and Iqbal, 2016; Garcia et al., 2018) acted as trigger points that initiated urban migration from rural areas. Such migration, especially from the de-excluded areas, led the high land price and renters, unplanned construction activities, congested road development, improper green space management, and removal of agriculture and scrubland. Similar studies also show the same results in other parts of the country. Arshad et al. (2022), for example, conducted the temporal changes of LULC in association with the surface urban heat island (SUHI) effect. Their study argued that the high demand for housing schemes to a flux of migration from neighboring rural areas in the recent two decades had put lots of pressure on Lahore city (the 2nd largest city in Pakistan). This disorganized urban growth leads to the removal of green spaces and causes 3–4°C higher temperatures and the SUHI effect in the central urban district (CBD) and slum areas. Similar results were found in the capital city of Pakistan (Islamabad) (Waseem and Khayyam, 2019; Aslam et al., 2021). Waseem and Khayyam (2019) discovered the 3–9°C higher LST (0.52°C per year) at the cost of 51% vegetation removal. This green-gray conversion increasing LST and UHI effect also affects the northern mountainous region of Pakistan. Where Ullah et al. (2019b) found that the previous three decades were the most critical that put a lot of pressure on Pakistan’s mountainous cities and LULC changes had a stronger relationship to raising the LST in the lower Himalayan region, which further shows our findings’ consistency with the literature. In the same year, Ullah et al., (2019a,b) discovered that the flux of migrants from neighboring villages due to insecurity and to seek better living standards causes deforestation and consequently, the removal of urban forest (4.42%) and agriculture land areas (2.74%) led to the rising LST (greater than 27°C) and UHI effect will increased to 42 and 60% in the upcoming years (2032 and 2047). This situation further stresses the need for informed planning of land resources along with preserving the vegetation through no-net-loss policies via empirical references such as those presented in this study.
A clear trend of decreasing NDVI and increasing NDBI (Figure 4) in the study area further confirms the consequences of lack of management, ill-informed construction, and unplanned resource allocation that affect the LST and UHI hotspots. D. G. Khan city is the center point of Pakistan that connects all the adjacent provinces. Being divisional capital, it is the hub of commercial and economic activities. Economic growth results in the LULC change and causes socio-environmental problems. The simulation results presented in this study confirm that by 2032, the city will face a 5° C high mean temperature based on the historical patterns, which could potentially lead to more challenges associated with UHI (Figures 4, 6 and Table 4) if no appropriate measures are taken. These findings are similar to (Tariq et al., 2023).
Recently, the government developed several land-use policies and rehabilitation programs at the federal and provincial levels. Still, lack of political interest and insufficient urban governance are the main barriers to implementing these initiatives. In recent years, the government has launched a Billion Trees Tsunami program all over Pakistan to tackle green cover degradation in the country, which has important implications for urban climatic conditions (Sajjad, 2020). For instance, this program was initially introduced in Khyber Pakhtunkhwa province and recent research shows positive outcomes regarding land surface temperature reduction (Mumtaz et al., 2020), air surface temperature reduction (Mahmood, 2020), better ecosystem quality (Khan N. et al., 2019), and air pollution control measures (Kharl and Xie, 2017). Taking such programs to the city level particularly to zones of higher LST, as identified in this study, could be a useful approach to mitigate the UHI effect in cities.
Our results have shown that green spaces are insufficient to overcome the large urban footprint. Hence, the green cover should be introduced and preserved on large patches to overcome the impact of impervious surfaces. The city and divisional government should introduce strict policies to implement green space initiatives at a larger scale to overcome the LST effect. The predicted results of 2032 also indicated the tremendous increase of 4–5° C LST and UHI, which represents an alarming situation for urban dwellers and should be a matter of the highest concern for relevant authorities. Estoque et al. (2017) and Arshad et al. (2022) investigated that the temperature of green spaces is ~3° C lower than urban fabric temperature and strongly suggested that the urban green space is one of the most optimal solutions to overcome the LST and mitigate the UHI effect. Hence, we recommend prioritization of the areas with higher LST, particularly in the 2032 prediction map, to initiate efforts for green spaces in the context of mitigating the impacts, especially the health issues, which the country has witnessed recently due to heat wave.
5. Conclusions and recommendations
This study leverages multi-temporal remote sensing data to track historical and predict future patterns of land use/cover changes, LST, and the association between them in a mid-country city that is a part of the China-Pakistan Economic Corridor (CPEC) under the One Road One Built project. The overarching goal of the study is to determine how changes in land use classes affected LST. The methods used in this study are quite effective in accomplishing the research objectives. The study area is divided into four LULC categories, such as water bodies, built-up land, bare land, and vegetation. Due to political and socio-economic considerations, the land cover classification revealed that built-up areas and water bodies increased by 8.7 and 0.6%, respectively, over the study period (2002–2022). In contrast, bare land and vegetation dropped by 2.5 and 6.8%, respectively.
Furthermore, the study determines a negative association of vegetation area (NDVI) and water bodies with LST. The LULC significantly influences the LST and is extremely sensitive to vegetation and soil moisture; vegetation is the most important element in this connection. Although there is a positive association between NDBI and LST, higher LST is reported in areas with less vegetated (barren land) and vice versa. We conclude that without proper measures, a tremendous increase in the impervious surface is expected at the cost of green areas, which could potentially compromise the livability and comfortability of cities in the wake of global warming. This situation would very well lead to the UHI effect influencing millions of people in terms of increased energy demands and health-related challenges.
In order to cope with this increasing LST situation, green urban design and infrastructure planning and development must be prioritized. Similarly, enhancing water features like lakes, canals, waterfalls, and public fountains along with significantly increasing green areas like artificial parks, green walls, gardens, and linear plantings, particularly woody plants could positively drive the UHI mitigation efforts. In addition, to achieve the intended ecological development in terms of environmental resource planning and management, LULC alteration activities should be minimized, and environmental education should be reawakened. The findings of this study have important implications for urban landscape planning, particularly when it comes to landscape connectivity between green and impervious surfaces and their impact on LST. All of these would result in science-based information having important implications for urban planning and land resource management along with providing opportunities to design appropriate action plans to mitigate the UHI effect.
6. Limitations and future works
The present study provides important and useful information for the study area to design proper adaptation and mitigation policies and action plans. Such actions at local levels would progressively contribute to regional sustainability from a bottom-up perspective. From a short-to-medium-term impact perspective, predicting future changes in LULC and how it will influence the LST under environmental changes provides key references for planning and management of the land resource in a more sustainable way along with providing opportunities for adaptation and mitigating climate change through local actions. Future urban studies could concentrate on the topic of rapid urbanization’s impact on public health and infrastructure along with the influence of predicted LST on health-related issues in urban societies—left for upcoming studies. Future research should address the following limitations. Higher-resolution images for LULC categorization may improve LULC composition-LST correlation explanation and urban planner usability. Urban morphology is needed to better understand the nonlinear association between LULC and LST in urban areas. For more conclusive LST prediction findings, other nonlinear regression approaches may need to be investigated. This study used temperature indices to analyze LST; multi-season analysis will provide more information. Although LULC is a primary influence in LST variation, other characteristics affecting LST, most likely topography and elevation, are equally essential. In commercial/industrial locations, surface morphology also effects surface temperature retrieval. More precise spatial distribution information regarding urban land shape is required for more accurate characterization of patterns and changes of the urban heat island effect.
Landsat data can detect LULC and LST effects, but projecting future changes is challenging. MC, CA, and ANN models are most often employed to predict LULC change. We used MC model based on the LULC conversion is known but spatial dependency and dissemination are unavailable. Future studies will focus on the CA and ANN models. CA is employed in utility and resource research since its greatest advantage depends on the initial configuration of variables like distance to road and rail network, slope, and elevation. To forecast LST, ANN can be used in conjunction with associated LULC indices like as NDVI, NDBI, NDBSI, and NDWI to successfully estimate future changes. ANN technique is an LST predictor because it employs LULC indices to anticipate future scenarios and reflects past patterns. As a result, ANN is often recognized as the best tool for forecasting the effects of LST.
Data availability statement
The original contributions presented in the study are included in the article/Supplementary material, further inquiries can be directed to the corresponding author.
Author contributions
MM and AR acquired data. MM, AR, and ZZ performed data analysis. MM, AR, ZZ, and MS wrote the first draft of the manuscript. QY and ZS supervised and did the final proofreading of the article. All authors substantially contributed to the study’s conception and design, feedback and input during the write-up, analysis, data validation, and proofreading of the present research work.
Funding
This research was funded by the National Natural Science Foundation of China (42171295), and the Major Project of Collaborative Innovation Center on Yellow River Civilization jointly built by Henan Province and the Ministry of Education (2020 M19).
Conflict of interest
The authors declare that the research was conducted in the absence of any commercial or financial relationships that could be construed as a potential conflict of interest.
Publisher’s note
All claims expressed in this article are solely those of the authors and do not necessarily represent those of their affiliated organizations, or those of the publisher, the editors and the reviewers. Any product that may be evaluated in this article, or claim that may be made by its manufacturer, is not guaranteed or endorsed by the publisher.
Supplementary material
The Supplementary material for this article can be found online at: https://www.frontiersin.org/articles/10.3389/fevo.2023.1115074/full#supplementary-material
Abbreviations
LULC, Land Use Land Cover, LST, Land Surface Temperature; TM/OLI, Thematic Mapper/Operational Land Imager; UHI, Urban Heat Island; UN, United Nations; GIS, Geographic Information System; RS, Remote Sensing; NDVI, Normalized Difference Vegetation Index; NDBI, Normalized Difference Built-up Index; DG Khan, Dera Ghazi Khan; FBS, Federal bureau of Statistics; CPEC, China Pakistan Economic Corridor; SUPARCO, Space & Upper Atmosphere Research Commission; FAO, Food and Agricultural Organization; MLP-MC, Multi-layer Perceptron-Markov Chain; CA, Cellular Automata; ANN, Artificial Neural Network.
Footnotes
1. ^https://www.worldweatherattribution.org/climate-change-made-devastating-early-heat-in-india-and-pakistan-30-times-more-likely/
References
Ahmed, B., Kamruzzaman, M., Zhu, X., Rahman, M., and Choi, K. (2013). Simulating land cover changes and their impacts on land surface temperature in Dhaka, Bangladesh. Remote Sens. 5, 5969–5998. doi: 10.3390/rs5115969
Ahmed, B., Wei, S., Fu, Y. G., Shabbir, M., and Nabi, G. (2014). Effects of floods policy in Pakistan and management issues: (case of district Dera-ghazi Khan). Int. J. Adv. Res. 2, 967–974.
Akinyemi, F. O., Ikanyeng, M., and Muro, J. (2019). Land cover change effects on land surface temperature trends in an African urbanizing dryland region. City Environ. Interact. 4:100029. doi: 10.1016/j.cacint.2020.100029
Aktaş, N. K., and Dönmez, N. Y. (2021). “An urban paradox: urban resilience or human needs” in Design of Cities and Buildings: Sustainability and Resilience in the Built Environment. ed. M. E. Samad (London: IntechOpen), 173.
Alqadhi, S., Mallick, J., Balha, A., Bindajam, A., Singh, C. K., and Hoa, P. V. (2021). Spatial and decadal prediction of land use/land cover using multi-layer perceptron-neural network (Mlp-Nn) algorithm for a semi-arid region of Asir, Saudi Arabia. Earth Sci. Inf. 14, 1547–1562. doi: 10.1007/s12145-021-00633-2
Alqurashi, A., Kumar, L., and Sinha, P. (2016). Urban land cover change modelling using time-series satellite images: a case study of urban growth in five cities of Saudi Arabia. Remote Sens. 8:838. doi: 10.3390/rs8100838
Amir Siddique, M., Dongyun, L., Li, P., Rasool, U., Ullah Khan, T., Javaid Aini Farooqi, T., et al. (2020). Assessment and simulation of land use and land cover change impacts on the land surface temperature of Chaoyang District in Beijing, China. PeerJ 8:e9115. doi: 10.7717/peerj.9115
Andrade, J., Cunha, J., Silva, J., Rufino, I., and Galvão, C. (2021). Evaluating single and multi-date landsat classifications of land-cover in a seasonally dry tropical forest. Remote Sens. Appl. 2021:100515. doi: 10.1016/j.rsase.2021.100515
Arshad, S., Ahmad, S. R., Abbas, S., Asharf, A., Siddiqui, N. A., and Islam, Z. (2022). Quantifying the contribution of diminishing green spaces and urban sprawl to urban heat island effect in a rapidly urbanizing metropolitan city of Pakistan. Land Use Policy 113:105874. doi: 10.1016/j.landusepol.2021.105874
Artis, D. A., and Carnahan, W. H. (1982). Survey of emissivity variability in thermography of urban areas. Remote Sens. Environ. 12, 313–329. doi: 10.1016/0034-4257(82)90043-8
Aslam, A., Rana, I. A., and Bhatti, S. S. (2021). The spatiotemporal dynamics of urbanisation and local climate: a case study of Islamabad, Pakistan. Environ. Impact Assess. Rev. 91:106666. doi: 10.1016/j.eiar.2021.106666
Azhar, I. A. K. (2008). Overseas Migration and its Socio-economic Impacts on the Families Left Behind in Pakistan: A Case Study in the Province. Punjab, Pakistan, Kassel University Press GmbH.
Bera, D., Das Chatterjee, N., Mumtaz, F., Dinda, S., Ghosh, S., Zhao, N., et al. (2022). Integrated influencing mechanism of potential drivers on seasonal variability of Lst in Kolkata municipal corporation, India. Land 11:1461. doi: 10.3390/land11091461
Bokaie, M., Zarkesh, M. K., Arasteh, P. D., and Hosseini, A. (2016). Assessment of urban Heat Island based on the relationship between land surface temperature and land use/ land cover in Tehran. Sustain. Cities Soc. 23, 94–104. doi: 10.1016/j.scs.2016.03.009
Cai, M., Ren, C., Xu, Y., Lau, K. K.-L., and Wang, R. (2018). Investigating the relationship between local climate zone and land surface temperature using an improved Wudapt methodology–a case study of Yangtze River Delta, China. Urban Clim. 24, 485–502. doi: 10.1016/j.uclim.2017.05.010
Castelli, F. (2018). Drivers of migration: why do people move? J. Travel Med. 25:tay040. doi: 10.1093/jtm/tay040
Crum, S. M., and Jenerette, G. D. (2017). Microclimate variation among urban land covers: the importance of vertical and horizontal structure in air and land surface temperature relationships. J. Appl. Meteorol. Climatol. 56, 2531–2543. doi: 10.1175/JAMC-D-17-0054.1
Das, N., Mondal, P., Sutradhar, S., and Ghosh, R. (2021). Assessment of variation of land use/land cover and its impact on land surface temperature of Asansol subdivision. Egypt. J. Remote Sens. Space Sci. 24, 131–149. doi: 10.1016/j.ejrs.2020.05.001
Dey, N. N., Al Rakib, A., Kafy, A.-A., and Raikwar, V. (2021). Geospatial modelling of changes in land use/land cover dynamics using multi-layer perception Markov chain model in Rajshahi City, Bangladesh. Environ. Chall. 4:100148. doi: 10.1016/j.envc.2021.100148
Dos Santos, J. Y. G., Montenegro, S. M. G. L., Da Silva, R. M., Santos, C. A. G., Quinn, N. W., Dantas, A. P. X., et al. (2021). Modeling the impacts of future Lulc and climate change on runoff and sediment yield in a strategic basin in the Caatinga/Atlantic forest ecotone of Brazil. Catena 203:105308. doi: 10.1016/j.catena.2021.105308
Estoque, R. C., Murayama, Y., and Myint, S. W. (2017). Effects of landscape composition and pattern on land surface temperature: an urban heat island study in the megacities of Southeast Asia. Sci. Total Environ. 577, 349–359. doi: 10.1016/j.scitotenv.2016.10.195
Faroughi, M., Karimimoshaver, M., Aram, F., Solgi, E., Mosavi, A., Nabipour, N., et al. (2020). Computational modeling of land surface temperature using remote sensing data to investigate the spatial arrangement of buildings and energy consumption relationship. Eng. Appl. Comput. Fluid Mech. 14, 254–270. doi: 10.1080/19942060.2019.1707711
FBS. (2017). Federal Bureau of Statistics: Provisional Summary Results of 6th Population and Housing Census. Islamabad: Pakistan Bureau Of Statistics, Ministry of Statistics, Islamabad, Islamic Republic of Pakistan.
Foody, G. M., Campbell, N., Trodd, N., and Wood, T. (1992). Derivation and applications of probabilistic measures of class membership from the maximum-likelihood classification. Photogramm. Eng. Remote. Sens. 58, 1335–1341.
Gan, T., Liang, W., Yang, H., and Liao, X. (2020). The effect of economic development on haze pollution (Pm2. 5) based on a spatial perspective: urbanization as a mediating variable. J. Clean. Prod. 266:121880. doi: 10.1016/j.jclepro.2020.121880
Garcia, A., Orengo, H. A., Conesa, F. C., Green, A. S., and Petrie, C. A. (2018). Remote sensing and historical morphodynamics of alluvial plains. The 1909 Indus flood and the city of Dera Gazhi Khan (province of Punjab, Pakistan). Geosciences 9:21. doi: 10.3390/geosciences9010021
Garcia, A., Orengo, H., Conesa, F., Green, A., and Petrie, C. (2019). Remote sensing and historical Morphodynamics of Alluvial Plains. The 1909 Indus flood and the City of Dera Ghazi Khan (province of Punjab, Pakistan). Geosciences 9:21. doi: 10.3390/geosciences9010021
Guha, S., Govil, H., and Diwan, P. (2019). Analytical study of seasonal variability in land surface temperature with normalized difference vegetation index, normalized difference water index, normalized difference built-up index, and normalized multiband drought index. J. Appl. Remote. Sens. 13:024518. doi: 10.1117/1.JRS.13.024518
Guo, A., Yang, J., Xiao, X., Xia, J., Jin, C., and Li, X. (2020). Influences of urban spatial form on urban heat island effects at the community level in China. Sustain. Cities Soc. 53:101972. doi: 10.1016/j.scs.2019.101972
Hamad, R., Balzter, H., and Kolo, K. (2018). Predicting land use/land cover changes using a ca-Markov model under two different scenarios. Sustainability 10:3421. doi: 10.3390/su10103421
Hashmi, H. N., Siddiqui, Q. T. M., Ghumman, A. R., and Kamal, M. A. (2012). A critical analysis of 2010 floods in Pakistan. Afr. J. Agric. Res. 7, 1054–1067. doi: 10.5897/AJARX11.036
Hassan, M. M. (2017). Monitoring land use/land cover change, urban growth dynamics and landscape pattern analysis in five fastest urbanized cities in Bangladesh. Remote Sens. Appl. 7, 69–83. doi: 10.1016/j.rsase.2017.07.001
Heaviside, C., Macintyre, H., and Vardoulakis, S. (2017). The urban heat island: implications for health in a changing environment. Curr. Environ. Health Rep. 4, 296–305. doi: 10.1007/s40572-017-0150-3
Heikkinen, A., Mäkelä, H., Kujala, J., Nieminen, J., Jokinen, A., and Rekola, H. (2019). Urban Ecosystem Services and Stakeholders: Towards a Sustainable Capability Approach. Strongly Sustainable Societies. Taylor & Francis.
Hussain, S., Mubeen, M., Ahmad, A., Akram, W., Hammad, H. M., Ali, M., et al. (2020). Using Gis tools to detect the land use/land cover changes during forty years in Lodhran District of Pakistan. Environ. Sci. Pollut. Res. Int. 27, 39676–39692. doi: 10.1007/s11356-019-06072-3
Hussain, S., Mubeen, M., Akram, W., Ahmad, A., Habib-Ur-Rahman, M., Ghaffar, A., et al. (2019). Study of land cover/land use changes using Rs and Gis: a case study of Multan district, Pakistan. Environ. Monit. Assess. 192:2. doi: 10.1007/s10661-019-7959-1
Imran, M., and Mehmood, A. (2020). Analysis and mapping of present and future drivers of local urban climate using remote sensing: a case of Lahore, Pakistan. Arab. J. Geosci. 13, 1–14. doi: 10.1007/s12517-020-5214-2
Kadhim, N., Mourshed, M., and Bray, M. (2016). Advances in remote sensing applications for urban sustainability. EuroMediterr. J. Environ. Integr. 1, 1–22. doi: 10.1007/s41207-016-0007-4
Kafy, A.-A., Al Rakib, A., Akter, K. S., Rahaman, Z. A., Faisal, A.-A., Mallik, S., et al. (2021a). Monitoring the effects of vegetation cover losses on land surface temperature dynamics using geospatial approach in Rajshahi City, Bangladesh. Environ. Chall. 4:100187. doi: 10.1016/j.envc.2021.100187
Kafy, A.-A., Faisal, A.-A., Sikdar, M. S., Hasan, M. M., and Ahmmed, R. (2019). Using Geographic Information System and Remote Sensing Techniques in Environmental Management: A Case Study in Cumilla City Corporation. 1st International Conference on Urban & Regional Planning. Dhaka, Bangladesh: Bangladesh Institute of Planners (Bip).
Kafy, A. A., Rahman, M. S., Faisal, A.-A., Hasan, M. M., and Islam, M. (2020). Modelling future land use land cover changes and their impacts on land surface temperatures in Rajshahi, Bangladesh. Remote Sens. Appl. 18:100314. doi: 10.1016/j.rsase.2020.100314
Kafy, A.-A., Rahman, M. S., Islam, M., Al Rakib, A., Islam, M. A., Khan, M. H. H., et al. (2021b). Prediction of seasonal urban thermal field variance index using machine learning algorithms in Cumilla, Bangladesh. Sustain. Cities Soc. 64:102542. doi: 10.1016/j.scs.2020.102542
Kafy, A.-A., Shuvo, R. M., Naim, M. N. H., Sikdar, M. S., Chowdhury, R. R., Islam, M. A., et al. (2021c). Remote sensing approach to simulate the land use/land cover and seasonal land surface temperature change using machine learning algorithms in a fastest-growing megacity of Bangladesh. Remote Sens. Appl. 21:100463. doi: 10.1016/j.rsase.2020.100463
Kazemzadeh-Zow, A., Zanganeh Shahraki, S., Salvati, L., and Samani, N. N. (2017). A spatial zoning approach to calibrate and validate urban growth models. Int. J. Geogr. Inf. Sci. 31, 763–782. doi: 10.1080/13658816.2016.1236927
Khan, I. (2018). ‘Cpec will be Reviewed for Balochistan’Promises. Karachi: Fortnightly Engineering Review.
Khan, I., Javed, T., Khan, A., Lei, H., Muhammad, I., Ali, I., et al. (2019). Impact assessment of land use change on surface temperature and agricultural productivity in Peshawar-Pakistan. Environ. Sci. Pollut. Res. 26, 33076–33085. doi: 10.1007/s11356-019-06448-5
Khan, N., Shah, S. J., Rauf, T., Zada, M., Yukun, C., and Harbi, J. (2019). Socioeconomic impacts of the billion trees afforestation program in Khyber Pakhtunkhwa Province (kpk). Pakistan. Forests 10:703. doi: 10.3390/f10080703
Kharl, S., and Xie, X. (2017). Green growth initative will lead toward sustainable development of natural resources in Pakistan: an Investgation of “billion tree tsunami afforestation project”. Sci Int 29, 841–843.
Korkmaz, C., and Meşhur, H. F. A. (2021). Neo-liberal urbanism and sustainability in Turkey: commodification of nature in gated community marketing. J. Housing Built Environ. 3, 1–34. doi: 10.1007/s10901-020-09800-1
Lakshmisha, A., Agarwal, P., and Nikam, M. (2019). Assessing the Double Injustice of Climate Change and Urbanization on Water Security in Peri-urban Areas: Creating Citizen-Centric Scenarios. Water Insecur. Sanit. Asia 1:301.
Li, W., Cao, Q., Lang, K., and Wu, J. (2017). Linking potential heat source and sink to urban heat island: heterogeneous effects of landscape pattern on land surface temperature. Sci. Total Environ. 586, 457–465. doi: 10.1016/j.scitotenv.2017.01.191
Luo, P., Kang, S., Zhou, M., Lyu, J., Aisyah, S., Binaya, M., et al. (2019). Water quality trend assessment in Jakarta: a rapidly growing Asian megacity. PLoS One 14:e0219009. doi: 10.1371/journal.pone.0219009
Maduako, I., Yun, Z., and Patrick, B. (2016). Simulation and prediction of land surface temperature (Lst) dynamics within Ikom City in Nigeria using artificial neural network (Ann). J. Remote Sens. Gis 5, 1–7. doi: 10.4172/2469-4134.1000158
Mahmood, F. (2020). Politics for environment: youth perception on campaign for billion tree tsunami to combat climate change situation in Pakistan. J. Med. Stud. 35, 41–61.
Mallick, J., Singh, V. P., Almesfer, M. K., Talukdar, S., Alsubhi, M., Ahmed, M., et al. (2021). Spatio-temporal analysis and simulation of land cover changes and their impacts on land surface temperature in urban agglomeration of Bisha watershed, Saudi Arabia. Geocarto Int. 37, 1–27. doi: 10.1080/10106049.2021.1980616
Mansour, S., Al-Belushi, M., and Al-Awadhi, T. (2020). Monitoring land use and land cover changes in the mountainous cities of Oman using Gis and ca-Markov modelling techniques. Land Use Policy 91:104414. doi: 10.1016/j.landusepol.2019.104414
Mas, J.-F., and Soares De Araújo, F. (2021). Assessing Landsat images availability and its effects on Phenological metrics. Forests 12:574. doi: 10.3390/f12050574
Maurya, P., Das, A. K., and Kumari, R. (2021). Managing the blue carbon ecosystem: a remote sensing and Gis approach. Adv. Remote Sens. Nat. Resour. Monit., 247–268. doi: 10.1002/9781119616016.ch13
Mehmood, M. S., Zafar, Z., Sajjad, M., Hussain, S., Zhai, S., and Qin, Y. (2023). Time series analyses and forecasting of surface urban Heat Island intensity using Arima model in Punjab, Pakistan. Land 12:142. doi: 10.3390/land12010142
Mengistu, D. A., and Salami, A. T. (2007). Application of remote sensing and Gis inland use/land cover mapping and change detection in a part of South Western Nigeria. Afr. J. Environ. Sci. Technol. 1, 99–109.
Mumtaz, F., Tao, Y., De Leeuw, G., Zhao, L., Fan, C., Elnashar, A., et al. (2020). Modeling spatio-temporal land transformation and its associated impacts on land surface temperature (Lst). Remote Sens. 12:2987. doi: 10.3390/rs12182987
Munir, B. A., and Iqbal, J. (2016). Flash flood water management practices in Dera Ghazi Khan City (Pakistan): a remote sensing and Gis prospective. Nat. Hazards 81, 1303–1321. doi: 10.1007/s11069-015-2136-5
Musse, M. A., Barona, D. A., and Rodriguez, L. M. S. (2018). Urban environmental quality assessment using remote sensing and census data. Int. J. Appl. Earth Obs. Geoinf. 71, 95–108. doi: 10.1016/j.jag.2018.05.010
Peng, J., Jia, J., Liu, Y., Li, H., and Wu, J. (2018). Seasonal contrast of the dominant factors for spatial distribution of land surface temperature in urban areas. Remote Sens. Environ. 215, 255–267. doi: 10.1016/j.rse.2018.06.010
Peng, W., Zhou, J., Wen, L., Xue, S., and Dong, L. (2017). Land surface temperature and its impact factors in Western Sichuan plateau, China. Geocarto Int. 32, 919–934. doi: 10.1080/10106049.2016.1188167
Platt, R. V., and Rapoza, L. (2008). An evaluation of an object-oriented paradigm for land use/land cover classification. Prof. Geogr. 60, 87–100. doi: 10.1080/00330120701724152
Rehman, A., Song, J., Haq, F., Ahamad, M. I., Sajid, M., and Zahid, Z. (2021). Geo-physical hazards microzonation and suitable site selection through multicriteria analysis using geographical information system. Appl. Geogr. 135:102550. doi: 10.1016/j.apgeog.2021.102550
Rehman, A., Song, J., Haq, F., Mahmood, S., Ahamad, M. I., Basharat, M., et al. (2022). Multi-Hazard susceptibility assessment using the analytical hierarchy process and frequency ratio techniques in the Northwest Himalayas, Pakistan. Remote Sens. 14:554. doi: 10.3390/rs14030554
Ruben, G. B., Zhang, K., Dong, Z., and Xia, J. (2020). Analysis and projection of land-use/land-cover dynamics through scenario-based simulations using the ca-Markov model: a case study in guanting reservoir basin, China. Sustainability 12:3747. doi: 10.3390/su12093747
Rwanga, S. S., and Ndambuki, J. M. (2017). Accuracy assessment of land use/land cover classification using remote sensing and Gis. Int. J. Geosci. 8:611. doi: 10.4236/ijg.2017.84033
Sadiq Khan, M., Ullah, S., Sun, T., Rehman, A. U. R., and Chen, L. (2020). Land-use/land-cover changes and its contribution to urban Heat Island: a case study of Islamabad, Pakistan. Sustainability 12:3861. doi: 10.3390/su12093861
Sajjad, M. (2020). Impacts, Drivers, and Future Adaptation Opportunities for a Warming Pakistan: Learnings from an In- dustrialized City. Handbook of Climate Change Manage- ment: Research, Leadership, Transformation, 1–22.
Samie, A., Deng, X., Jia, S., and Chen, D. (2017). Scenario-based simulation on dynamics of land-use-land-cover change in Punjab Province, Pakistan. Sustainability 9:1285. doi: 10.3390/su9081285
Shafi, A., Chen, S., Waleed, M., and Sajjad, M. (2023). Leveraging machine learning and remote sensing to monitor long-term spatial-temporal wetland changes: towards a national Ramsar inventory in Pakistan. Appl. Geogr. 151:102868. doi: 10.1016/j.apgeog.2022.102868
Shahmohamadi, P., Che-Ani, A., Etessam, I., Maulud, K., and Tawil, N. (2011). Healthy environment: the need to mitigate urban heat island effects on human health. Proc. Eng. 20, 61–70. doi: 10.1016/j.proeng.2011.11.139
Shen, X., Liu, B., and Lu, X. (2017). Effects of land use/land cover on diurnal temperature range in the temperate grassland region of China. Sci. Total Environ. 575, 1211–1218. doi: 10.1016/j.scitotenv.2016.09.187
Song, J., Chen, W., Zhang, J., Huang, K., Hou, B., and Prishchepov, A. V. (2020). Effects of building density on land surface temperature in China: spatial patterns and determinants. Landsc. Urban Plan. 198:103794. doi: 10.1016/j.landurbplan.2020.103794
Talukdar, S., Singha, P., Mahato, S., Pal, S., Liou, Y.-A., and Rahman, A. (2020). Land-use land-cover classification by machine learning classifiers for satellite observations—a review. Remote Sens. 12:1135. doi: 10.3390/rs12071135
Tariq, A., and Mumtaz, F. (2022). Modeling spatio-temporal assessment of land use land cover of Lahore and its impact on land surface temperature using multi-spectral remote sensing data. Environ. Sci. Pollut. Res. 30, 23908–23924. doi: 10.1007/s11356-022-23928-3
Tariq, A., Mumtaz, F., Majeed, M., and Zeng, X. (2023). Spatio-temporal assessment of land use land cover based on trajectories and cellular automata Markov modelling and its impact on land surface temperature of Lahore district Pakistan. Environ. Monit. Assess. 195:114. doi: 10.1007/s10661-022-10738-w
Tariq, A., Mumtaz, F., Zeng, X., Baloch, M. Y. J., and Moazzam, M. F. U. (2022b). Spatio-temporal variation of seasonal heat islands mapping of Pakistan during 2000–2019, using day-time and night-time land surface temperatures Modis and meteorological stations data. Remote Sens. Appl. 27:100779. doi: 10.1016/j.rsase.2022.100779
Tariq, A., Riaz, I., Ahmad, Z., Yang, B., Amin, M., Kausar, R., et al. (2020). Land surface temperature relation with normalized satellite indices for the estimation of spatio-temporal trends in temperature among various land use land cover classes of an arid Potohar region using Landsat data. Environ. Earth Sci. 79, 1–15. doi: 10.1007/s12665-019-8766-2
Tariq, A., and Shu, H. (2020). Ca-Markov chain analysis of seasonal land surface temperature and land use land cover change using optical multi-temporal satellite data of Faisalabad, Pakistan. Remote Sens. 12:3402. doi: 10.3390/rs12203402
Tariq, A., Shu, H., Siddiqui, S., Imran, M., and Farhan, M. (2021). Monitoring land use and land cover changes using geospatial techniques, a case study of Fateh Jang, Attock, Pakistan. Geogr. Environ. Sustain. 14, 41–52. doi: 10.24057/2071-9388-2020-117
Tariq, A., Yan, J., and Mumtaz, F. (2022a). Land change modeler and ca-Markov chain analysis for land use land cover change using satellite data of Peshawar, Pakistan. Phys. Chem. Earth Parts A/B/C 128:103286. doi: 10.1016/j.pce.2022.103286
Thériault, M., Le Berre, I., Dubé, J., Maulpoix, A., and Vandersmissen, M.-H. (2020). The effects of land use planning on housing spread: a case study in the region of Brest, France. Land Use Policy 92:104428. doi: 10.1016/j.landusepol.2019.104428
Tilahun, A., and Teferie, B. (2015). Accuracy assessment of land use land cover classification using Google earth. Am. J. Environ. Prot. 4, 193–198. doi: 10.11648/j.ajep.20150404.14
Townshend, J. R., and Justice, C. (1986). Analysis of the dynamics of African vegetation using the normalized difference vegetation index. Int. J. Remote Sens. 7, 1435–1445. doi: 10.1080/01431168608948946
Tran, D. X., Pla, F., Latorre-Carmona, P., Myint, S. W., Caetano, M., and Kieu, H. V. (2017). Characterizing the relationship between land use land cover change and land surface temperature. ISPRS J. Photogramm. Remote Sens. 124, 119–132. doi: 10.1016/j.isprsjprs.2017.01.001
Ullah, S., Ahmad, K., Sajjad, R. U., Abbasi, A. M., Nazeer, A., and Tahir, A. A. (2019a). Analysis and simulation of land cover changes and their impacts on land surface temperature in a lower Himalayan region. J. Environ. Manag. 245, 348–357. doi: 10.1016/j.jenvman.2019.05.063
Ullah, S., Tahir, A. A., Akbar, T. A., Hassan, Q. K., Dewan, A., Khan, A. J., et al. (2019b). Remote sensing-based quantification of the relationships between land use land cover changes and surface temperature over the lower Himalayan region. Sustainability 11:5492. doi: 10.3390/su11195492
UN. (2017). World Population Prospects: The 2015 Revision: Key Findings and Advance Tables. Available at: https://esa.un.org/unpd/wpp/publications/Files/Wpp2017_KeyFindings.pdf.Un.
Utomo, D. H., and Kurniawan, B. R. (2016). Spatio temporal analysis trend of land use and land cover change against temperature based on remote sensing data in Malang City. Procedia Soc. Behav. Sci. 227, 232–238. doi: 10.1016/j.sbspro.2016.06.066
Vinayak, B., Lee, H. S., and Gedem, S. (2021). Prediction of land use and land cover changes in Mumbai City, India, using remote sensing data and a multilayer perceptron neural network-based markov chain model. Sustainability 13:471. doi: 10.3390/su13020471
Waleed, M., and Sajjad, M. (2022). Leveraging cloud-based computing and spatial modeling approaches for land surface temperature disparities in response to land cover change: evidence from Pakistan. Remote Sens. Appl. 25:100665. doi: 10.1016/j.rsase.2021.100665
Wang, R., Cai, M., Ren, C., Bechtel, B., Xu, Y., and Ng, E. (2019). Detecting multi-temporal land cover change and land surface temperature in Pearl River Delta by adopting local climate zone. Urban Clim. 28:100455. doi: 10.1016/j.uclim.2019.100455
Wang, Z., Xu, X., Wang, H., and Meng, S. (2020). Does land reserve system improve quality of urbanization? Evidence from China. Habitat Int. 106:102291. doi: 10.1016/j.habitatint.2020.102291
Waseem, S., and Khayyam, U. (2019). Loss of vegetative cover and increased land surface temperature: a case study of Islamabad, Pakistan. J. Clean. Prod. 234, 972–983. doi: 10.1016/j.jclepro.2019.06.228
Wessels, K., Steenkamp, K., Von Maltitz, G., and Archibald, S. (2011). Remotely sensed vegetation phenology for describing and predicting the biomes of South Africa. Appl. Veg. Sci. 14, 49–66. doi: 10.1111/j.1654-109X.2010.01100.x
Xu, X., Du, Z., and Zhang, H. (2016). Integrating the system dynamic and cellular automata models to predict land use and land cover change. Int. J. Appl. Earth Obs. Geoinf. 52, 568–579. doi: 10.1016/j.jag.2016.07.022
Yasin, Z., and Nabi, G. (2014). Alternative management plan for flash floods/flows of Mithawan Hill torrents in Pakistan. Int. J. Sci. Eng. Res. 5:95518
Zafar, Z., Mehmood, M. S., Shiyan, Z., Zubair, M., Sajjad, M., and Yaochen, Q. (2023). Fostering deep learning approaches to evaluate the impact of urbanization on vegetation and future prospects. Ecol. Indic. 146:109788. doi: 10.1016/j.ecolind.2022.109788
Zha, Y., Gao, J., and Ni, S. (2003). Use of normalized difference built-up index in automatically mapping urban areas from tm imagery. Int. J. Remote Sens. 24, 583–594. doi: 10.1080/01431160304987
Keywords: land use land cover, land surface temperature, urban heat island, artificial neural network, Markov chain
Citation: Mehmood MS, Rehman A, Sajjad M, Song J, Zafar Z, Shiyan Z and Yaochen Q (2023) Evaluating land use/cover change associations with urban surface temperature via machine learning and spatial modeling: Past trends and future simulations in Dera Ghazi Khan, Pakistan. Front. Ecol. Evol. 11:1115074. doi: 10.3389/fevo.2023.1115074
Edited by:
Alban Kuriqi, University of Lisbon, PortugalCopyright © 2023 Mehmood, Rehman, Sajjad, Song, Zafar, Shiyan and Yaochen. This is an open-access article distributed under the terms of the Creative Commons Attribution License (CC BY). The use, distribution or reproduction in other forums is permitted, provided the original author(s) and the copyright owner(s) are credited and that the original publication in this journal is cited, in accordance with accepted academic practice. No use, distribution or reproduction is permitted which does not comply with these terms.
*Correspondence: Qin Yaochen, cWlueWNAaGVudS5lZHUuY24=
†These authors have contributed equally to this work