- International Centre of Insect Physiology and Ecology (icipe), Nairobi, Kenya
The mango mealybug Rastrococcus invadens (Williams) (Homoptera: Pseudococcidae) is a destructive and important insect pest of fruit trees in Africa and Asia, especially the mango. Females and nymphs feed on plant leaves and fruits and produce honeydew that causes sooty mold, leading to yield reduction. Although it is an important pest, the distribution of R. invadens under different climate change scenarios has not been established. In this study, we predicted the suitable habitat for R. invadens occurrence under current and future [two Shared Socioeconomic Pathways (SSPs) scenarios: (SSP2-4.5 and SSP5-8.5) for the years 2050s and 2070s], using environmental variables and four ecological niche models viz., maxent, random forest, boosted regression trees, and support vector machines. The performance and accuracy of these models were evaluated using the area under the curve (AUC), the true skill statistic (TSS), correlation (COR), and deviance. All models had high accuracy (AUC ≥ 0.96, TSS ≥ 0.88, COR ≥ 0.74 and deviance ≤ 0.3) in predicting the potential distribution of R. invadens. Among the four models, the random forest algorithm had the highest performance (AUC = 0.99, TSS = 0.95, COR = 0.91 and deviance = 0.14) in predicting the potential distribution of R. invadens, followed by maxent (AUC = 0.97, TSS = 0.90, COR = 0.81 and deviance = 0.22). However, the maxent model was the best among the four algorithms in predicting the ecological niche of R. invadens. The precipitation of the wettest month (bio13) was the most crucial environmental variable that contributed to the predictions of the four models. The results revealed that most areas in East, Central, and West Africa were projected with high suitability for R. invadens to occur under current climatic conditions. Similarly, Bangladesh, Laos, Myanmar, India, Thailand, Vietnam and Cambodia in Asia, as well as Brazil, and Venezuela in South America showed high suitability for R. invadens establishment. However, under future climatic conditions (the years 2050s and 2070s), the suitable areas for R. invadens will increase regardless of the SSPs scenario (SSP2-4.5 and SSP5-8.5) indicating an expansion of the geographical range for this pest. This expansion is projected to be higher for the years 2070s than the 2050s. Similarly, the invasion risk of R. invadens is predicted to be higher under SSP2-4.5 scenario compared to SSP5-8.5 scenario, regardless of the year of the projection. Our results serve as an early warning tool that could serve as a guide to prevent further spread and invasion of this pest to new areas as well as help in developing an effective management strategy against R. invadens.
1 Introduction
The invasion by alien insect pests is among the most serious menace to biodiversity and agricultural production worldwide. Climate change is considered to be one of the factors that facilitate the movement of invasive pests to new areas (Early et al., 2016; Tabor and Koch, 2021). Due to the temperature rise, the geographical distribution of many insect pests in the future is expected to substantially differ from the current scenario (Azrag et al., 2018; Azrag et al., 2022; Istifanus et al., 2023). This change is anticipated to raise the risk of biological invasions by alien species (Early et al., 2016; Tabor and Koch, 2021), resulting in huge economic losses, especially in the agricultural sector. Hence, predicting the habitat suitability of invasive insect species has become more relevant than ever to serve as an early warning system to prevent the invasion of pests to new areas.
The fruit trees mealybug Rastrococcus invadens (Williams) (Hemiptera: Pseudococcidae), is an important invasive insect pest that causes severe damage to several fruit trees, including mango, Mangifera indica L (Anacardiaceae) (Agounké et al., 1988). It is a polyphagous pest attacking over forty-five plant species in twenty-two families including economically important cultivated crops like mango and Citrus, Citrus spp. (Rutaceae) (Agounké et al., 1988). R. invadens is native to Southern Asia (Bokonon-Ganta and Neuenschwander, 1995), but currently, it is distributed in over 27 countries in Asia, Africa, and French Guiana in Southern America (CABI, 2022). Out of its native range, R. invadens invaded West Africa in the 1980s, posing a serious threat to mango production in the region (Narasimham and Chacko, 1988; Agricola et al., 1989). It was reported in Togo, Benin, Ghana, Nigeria, Burkina Faso, Côte d’Ivoire, Senegal, and Sierra Leone (Narasimham and Chacko, 1988). However, due to the classical biological control programme that was undertaken against this pest in West Africa (Agricola et al., 1989; Neuenschwander et al., 1994; Mohamed et al., 2022), the population abundance of R. invadens and mango losses caused by this pest has substantially reduced. Despite the impressive impact of the imported parasitoids against the pest, R. invadens continued to spread further to Central Africa region where it was reported on mango in the Democratic Republic of Congo, Republic of Congo (Matokot et al., 1992) and Gabon (Boussienguet and Herren, 1992). Recently, R. invadens was detected in East Africa specifically in Rwanda (IPPC, 2019) and Burundi (IPPC, 2022). This indicates that the pest is expanding its geographical range toward new areas and climate change might increase the infestation risk and severity of R. invadens in the future. Yet, no studies have been conducted to predict its potential range expansion under varying climate change scenarios. Control and mitigation strategies based on the ecological traits of R. invadens are also urgently needed to minimize the risk.
Infestation of R. invadens causes a serious threat to small-scale farmers, especially in regions where fruits such as mango and citrus are the most available crops for income generation and livelihood improvement (Pitan et al., 2002). Females and nymphs feed on leaves and fruit leading to severe damage (Pitan et al., 2002; Nébié et al., 2016). Feeding damage can be categorised into two types: - firstly, sap-sucking can lead to shrivelling and dryness of the affected inflorescences, leaves, and twigs (Fall et al., 2017b), with severe infestation and feeding affecting fruit set and leading to dropping of fruit, hence yield reduction. Secondly, R. invadens produces honeydew that causes the growth of sooty mould on the branches, leaves and fruits. This affects the photosynthetic activity of the plant resulting in yield reduction (Lanjar et al., 2015; Fall et al., 2017b). In addition, the growth of sooty mould on fruits reduces the potential export to foreign markets, hence a lower profit margin (Pitan et al., 2002).
The environmental variables such as temperature, precipitation and humidity are the primary factors that influence the survival, geographical distribution, seasonal variation and population dynamics of R. invadens (Nébié et al., 2016; Fall et al., 2017b). As temperature increases, the geographical distribution of several insect species is anticipated to shift from the current scenario as a result of global warming (Bale et al., 2002; Azrag et al., 2018) and R. invadens is no exception and could expand its geographical distribution and invade new areas in the future as already reported in East Africa. Species distribution models like maxent, GLMs, and random forest are powerful tools that have been previously utilised to predict the habitat of several insect species (Merow et al., 2014; Norberg et al., 2019; Valavi et al., 2022). These models use the correlation method to correlate the presence of the species to the climatic conditions where the species is found to calculate the probability of occurrence. They use statistical and machine learning algorithms to predict the suitable habitat for the species to establish and survive (Merow et al., 2014). Therefore, ecological niche models enable researchers to evaluate the consequence of varying climates on distribution and spread of the species, to guide decision-making. However, each modelling tool has a certain level of uncertainty, and this results in different outputs for the same dataset when different models are used for the prediction (Norberg et al., 2019; Valavi et al., 2022). To overcome the issue of uncertainty, joining single models in an ensemble has been introduced to maximize the prediction accuracy (Capinha et al., 2011). The advantage of an ensembled model does not only improve the accuracy and performance, but also reduces the overfitting and underfitting by balancing the trade-off between bias and variance, using different subsets and features of the data.
Many studies have shown that climate change will directly impact the geographical distribution, abundance, and outbreak of many insect species (Azrag et al., 2018; Aidoo et al., 2022a; Aidoo et al., 2022b). This might increase the extent of crop losses, leading to food security issues, especially in developing countries. The recent invasion of R. invadens in East Africa (IPPC, 2019; IPPC, 2022), and French Guiana in Southern America (Germain et al., 2015), suggests that the pest might expand its geographical range toward new areas due to temperature rise, causing a serious impact on mango production. This highlights the urgent need of predicting the potential distribution and suitable habitat for this pest to guide for monitoring and surveillance of future invasion pathways to new areas. However, the effects of climate change on the distribution of R. invadens have never been predicted at the local, regional or global scale. In addition, the environmental predictors that drive the geographical distribution of R. invadens are still unknown. In this regard, our study aimed to i) predict the potential distribution (habitat suitability) of R. invadens under different climate change scenarios using environmental variables and four ecological niche models, ii) project the output of the four algorithms in a single layer using ensemble projection method to maximize the prediction accuracy of R. invadens occurrence.
2 Methodology
2.1 Occurrence datasets
The occurrence records of R. invadens were gathered from the Global Biodiversity Information Facility (GBIF https://www.gbif.org/) (GBIF, 2022) and published literature (Bokonon-Ganta and Neuenschwander, 1995; Bokonon-ganta et al., 2002; Pitan et al., 2002; Hala et al., 2004; Pitan, 2008; Nébié et al., 2016; Fall et al., 2017a; Nebie et al., 2018; Fall et al., 2021). These articles contained R. invadens occurrence records and they were obtained by a comprehensive literature search in Science Direct, Web of Science Google Scholar, and PubMed. Although R. invadens is present in Ghana, Burkina Faso, Burundi, Rwanda, and Uganda as well as some countries in South-East Asia (CABI, 2022), our search did not result in accurate georeferenced points that could be used for modelling (Figure 1). In other words, the exact geographical locations where the pest has been found are unknown in these countries. As a result, these countries were excluded from the analysis. Overall, 204 records were obtained from GBIF and literature searches. These records were filtered using Excel and spThin R package to remove the duplicated records as well as the records within 5 km2 to reduce the spatial autocorrelation (Boria et al., 2014). This filtering reduced the number of occurrence records to 166 which were utilized in predicting the suitable habitat of R. invadens (Figure 1).
2.2 Environmental variables
A total of 19 environmental predictors were gathered from Worldclim database and then used to model the habitat suitability of R. invadens (Table 1). These environmental variables are generated from temperature and precipitation, and they have been intensively utilized to predict the habitats suitability of many insect species (Biber-Freudenberger et al., 2016; Tepa-yotto et al., 2021; Aidoo et al., 2022b; Azrag et al., 2022). In this study, we used interpolated historical data for the years 1970-2000 to project the suitable habitat for R. invadens establishment in the current scenario (Hijmans et al., 2005). For future scenarios (2050s and 2070s), we used environmental variables simulated by the Hadley Centre Global Environmental Model version 2-Earth System (HADGEM2-ES) of two Shared Socioeconomic Pathways scenarios, SSP2-4.5 and SSP5-8.5 (previously known as Representative Concentration Pathways scenarios, RCP4.5 and RCP8.5) (Collins et al., 2011). We used the SSP2-4.5 climate change scenario because it is considered to be the moderate greenhouse gas emissions scenario that stabilises the emissions by utilizing various technologies and mitigation measures (Fujino et al., 2006). The radiative force of SSP2-4.5 is 4.5 Watts for each square meter (W/m2), while the emission of carbon dioxide (CO2) for this scenario is 538 parts per million (ppm) (Riahi et al., 2011). In addition, we used the SSP5-8.5 climate change scenario because it is an extreme emission scenario with an increase in greenhouse gas emissions and temperature over time. This scenario has a maximum CO2 emission of 936 ppm and a radiative force of 8.5 W/m2 (Riahi et al., 2011).
2.3 Variable selection for the modelling
Two selection criteria viz., variance inflation factor (VIF) and Pearson correlation were used to select uncorrelated predictors for modelling the suitable habitat of R. invadens. This is because the correlated predictor variables affect the model performance and sometimes lead to overfitting. The VIF assess whether the variance is inflated due to multicollinearity that exists between the predictors. Variables that have VIF higher than 10 indicate that these variables have multicollinearity thus they were removed when the model was fitted. In contrast, Pearson correlation is also one of the methods used to evaluate the multicollinearity amongst the predictor variables based on the correlation coefficient |r|. The variables that have |r|≥ 0.7 indicate high correlation (Figure 2) and therefore they were eliminated from the model fitting. Both selection methods produced 9 independent variables (Figure 2; Table 1), which were utilized in modelling the habitat suitability of R. invadens.
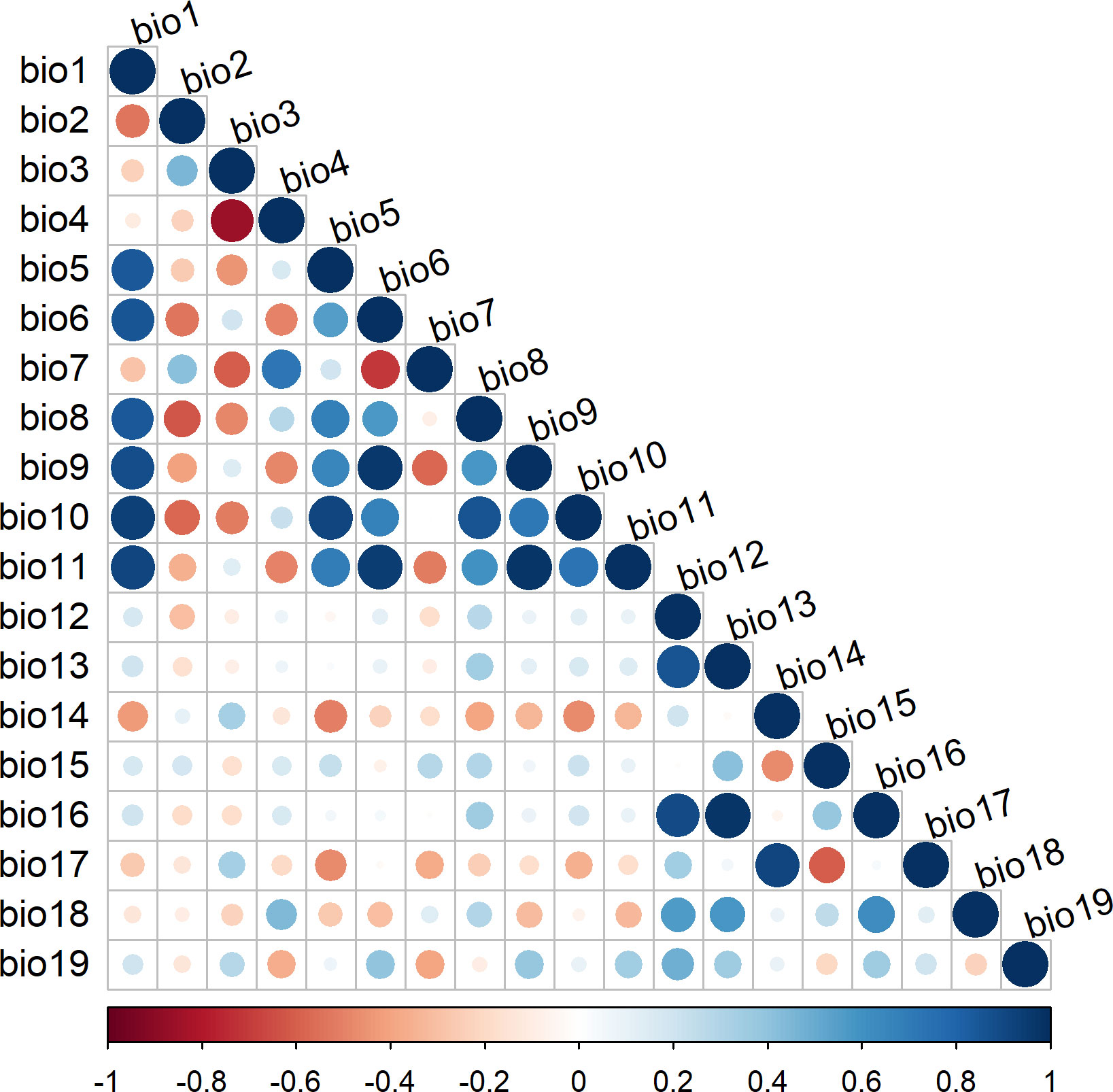
Figure 2 Collinearity matrix for environmental predictors utilized to model the habitat suitability of R. invadens. Darker shaded red and blue circles reflect high multicollinearity between the predictors, while light shades show low multicollinearity between the predictor variables.
2.4 Modelling framework
We used “sdm” package in R software (Naimi and Araújo, 2016) to predict the habitat suitability of R. invadens. This package employs object-oriented, repeatable, and extendable techniques to integrate several ecological niche models on a single platform (Naimi and Araújo, 2016). There are 18 ecological niche and machine learning algorithms embedded in “sdm” package that can be used to predict the habitat suitability of the species. This package provides a standardized and unified structure for handling species distribution data and modelling techniques (e.g. a unified interface is used to fit different models offered by different packages). Nevertheless, model parameterization can influence the prediction output of the species’ occurrence. The parameterization usually varies form one algorithm to another, and they are chosen depending on the species attributes such as thermal limits, biological interpretation of the niche and addressing issues related to small sample size (Merow et al., 2013). Although these settings improve the prediction accuracy, they sometimes lead to overfitting in predicting the suitable habitat for the species’ occurrence (Radosavljevic and Anderson, 2014). Therefore, we used the default modelling parameters for each method as defined in the “sdm” modelling platform (Naimi and Araújo, 2016). We used the standard default settings for all models to enable us to compare the output of the four models and avoid bias and overfitting of parameterization settings. Because we used presence-only data, we first employed the “sdmData” function in “sdm” package to randomly generate 1000 pseudo-absence records for R. invadens throughout the study area for each model run (maximum entropy (maxent) random forest (RF), boosted regression trees (BRT), and support vector machines (SVM). These background data were used against 166 presence-only records to predict the habitat suitability of R. invadens. Then, we used “sdm” and “predict” functions, respectively to calibrate the model and predict the suitable areas for R. invadens establishment. We employed four machine learning algorithms for the predictions viz., maxent RF, BRT, and SVM. A summary of these models’ packages used by “sdm” and their execution syntax are given in Table 2. Maxent is a well-known tool that has been intensively utilized for projecting the habitat of many species worldwide (Phillips and Dudík, 2008; Merow et al., 2013). It correlates the species occurrence to the predictor variables to determine the probabilities of the species establishment (Phillips and Dudík, 2008; Elith et al., 2011). FR is a machine learning algorithm which has been intensively employed in species distribution modelling and land use/land cover mapping (Liaw and Wiener, 2002; Pal, 2005; Shabani et al., 2016). The prediction of RF is based on the highest votes of the class that generate using decision trees in a forest (Pal, 2005). The BRT is one of the most popular models for predicting the distribution and habitat suitability of the species (Elith et al., 2008; Yu et al., 2020). It combines both decision tree algorithms and boosting methods to predict species occurrence (Elith et al., 2008; Yu et al., 2020). The SVM algorithm predicts the divergence of class categories using hyperplane (Karatzoglou et al., 2004; Vapnik, 2006). The selection of these algorithms was based on the fact that they are widely used in complex modelling processes and provide predictions with high accuracy (Elith et al., 2008; Merow et al., 2013; Shabani et al., 2016). In this modelling framework, we processed results for each model independently using one run. However, due to the fact that different models have some level of uncertainty and provide different outcomes for the same dataset, we combined the results of the four models using an “ensemble” function that is embedded in the “sdm” package to maximize the prediction accuracy using the weighted average of each pixel.
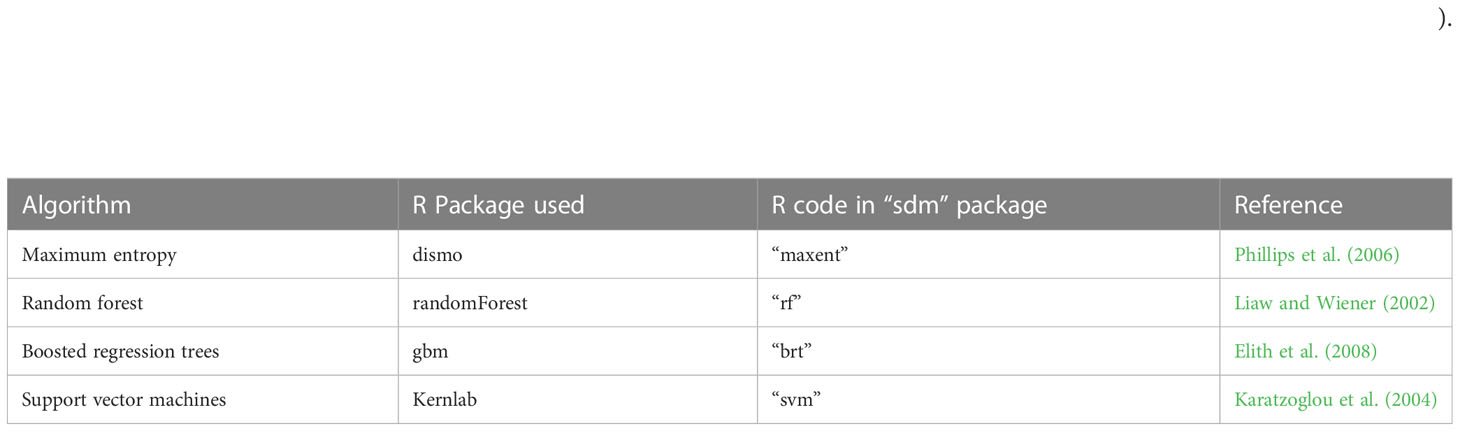
Table 2 Models, their packages and R codes utilized by “sdm” to project the habitat suitability R. invadens.
2.5 Models’ validation and accuracy assessment
We employed 10-fold cross-validation (CV) technique to test and validate the models and assess their prediction accuracy. The CV procedure provides more robust results because each data point in the dataset is tested only once, and thereafter these points are utilized to train the dataset k-1 times. Therefore, CV reduces the selection bias and prevents model overfitting, hence providing an accurate prediction. In this study, we used four metrics namely the area under the curve (AUC), true skill statistic (TSS), correlation (COR), and explained deviance between the calibrated and evaluated values to assess the performance and accuracy of each model (Allouche et al., 2006). Overall, AUC ranges from 0 to 1, with value 1 proving the maximum accuracy and performance of the algorithm in predicting the habitat suitability of the species. In contrast, TSS range between −1 and +1, with the value +1 demonstrating the highest agreement among the predicted and observed values of the species establishment. Generally, models with AUC and TSS of ≥ 0.7 demonstrate high predictive power and performance and therefore accurately predict the potential distribution and habitat suitability of the species (Tsoar et al., 2007). The correlation (COR) is a discrimination metric that measures the relationship between the model’s predictions for the presence data (test data) versus the absence data (background data) (Elith and Graham, 2009). It has values between 0 and 1, with higher values (close to 1) indicating huge differences in predicting the presence and absence records, hence large differences in the habitat suitability. The deviance expresses the magnitude of the deviations of the fitted values from the observations. It measures whether the model reliably predicted the occurrence records (Elith and Graham, 2009). The small values of deviance indicate that there is a low unexplained variance in the dataset, hence good model performance.
3 Results
3.1 Variables selection and model accuracy
Though 19 environmental predictors were gathered for modelling, only 9 were appropriate for predicting the potential distribution of R. invadens (Table 1). The eligible environmental variables selected based on VIF, and correlation metrics were bio2, bio3, bio8, bio9, bio13, bio14, bio15, bio18 and bio19 (Table 1; Figure 2). These environmental predictors had VIF lower than 10 and correlation coefficient |r|< 0.7 (Figure 2; Table 1), indicating their suitability in the modelling of the habitat suitability of R. invadens. The receiver operating curves (ROC) for 10-fold replication (light blue lines) in each fitted model showed that the outcome of the replication was relatively similar in their predictions (Figure 3). The four algorithms accurately projected the habitat suitability of R. invadens with high test AUC ranging from 0.96 to 0.99, TSS of 0.88 to 0.95, COR ≥ 0.81 and deviance between 0.14 and 0.3 (Table 3). Among the four models, the random forest algorithm had the highest performance (AUC = 0.99, TSS = 0.95, COR =0.91 and deviance = 0.14) in predicting the occurrence of R. invadens, followed by maxent (AUC = 0.97, TSS = 0.90, COR = 0.81 and deviance = 0.22) (Table 3).
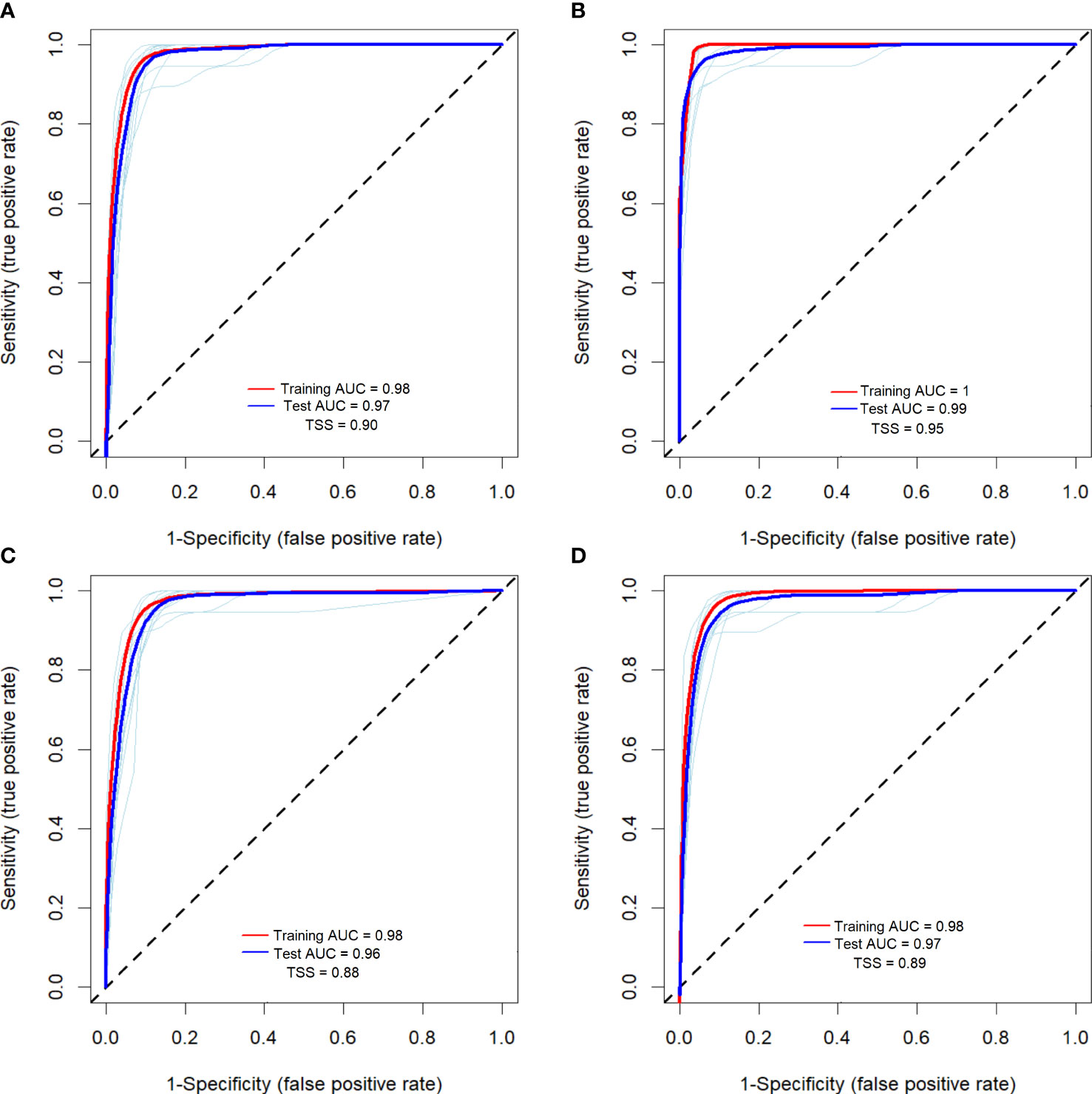
Figure 3 The area under the curve (AUC) of the receiver operating characteristic (ROC), and the true skill statistic (TSS) of the four machine learning algorithms used to project the suitable habitat of R. invadens, with (A) maxent; (B) RF; (C) BRT; and (D) SVM. The red line describes the average AUC of the training dataset, the dark blue line is the average AUC for the testing dataset, and the light blue lines in each model are representing the 10-fold replication of the training dataset.
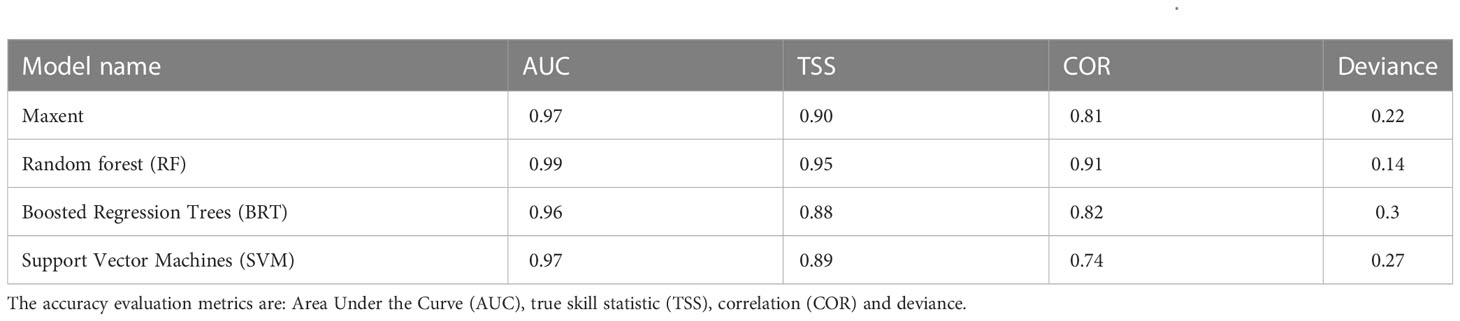
Table 3 The accuracy assessment of the four ecological niche models used to predict the habitat suitably of R. invadens.
3.2 Variable importance
The significance of the 9 environmental predictors in predicting the habitat suitability of R. invadens for each model is illustrated in Figure 4. Amongst the 9 predictors, precipitation of the wettest month (bio13) was the most relevant variable that contributed to the performance of the four algorithms (Figure 4). This environmental variable had the highest influence in projecting the habitat of R. invadens with a contribution of 29.1%, 30.3%, 43.2% and 62.6% for RF, SVM, maxent and BRT, respectively. The environmental variables bio9, bio13, bio14, and bio15 were the most important in modelling the habitat of R. invadens using maxent (Figure 4A). Similarly, the RF algorithm selected bio3, bio13, bio14, and bio15 as the most environmental predictors that contributed to predicting the habitat suitability of R. invadens (Figure 4B). The bio13, bio14, bio15 and bio19 were selected by BRT as the most crucial environmental variables in predicting the occurrence of R. invadens (Figure 4C). For SVM, all environmental variables relatively contributed to the model performance except bio14 and bio19 which had the lowest contribution amongst the 9 predictors that were eligible for modelling (Figure 4D).
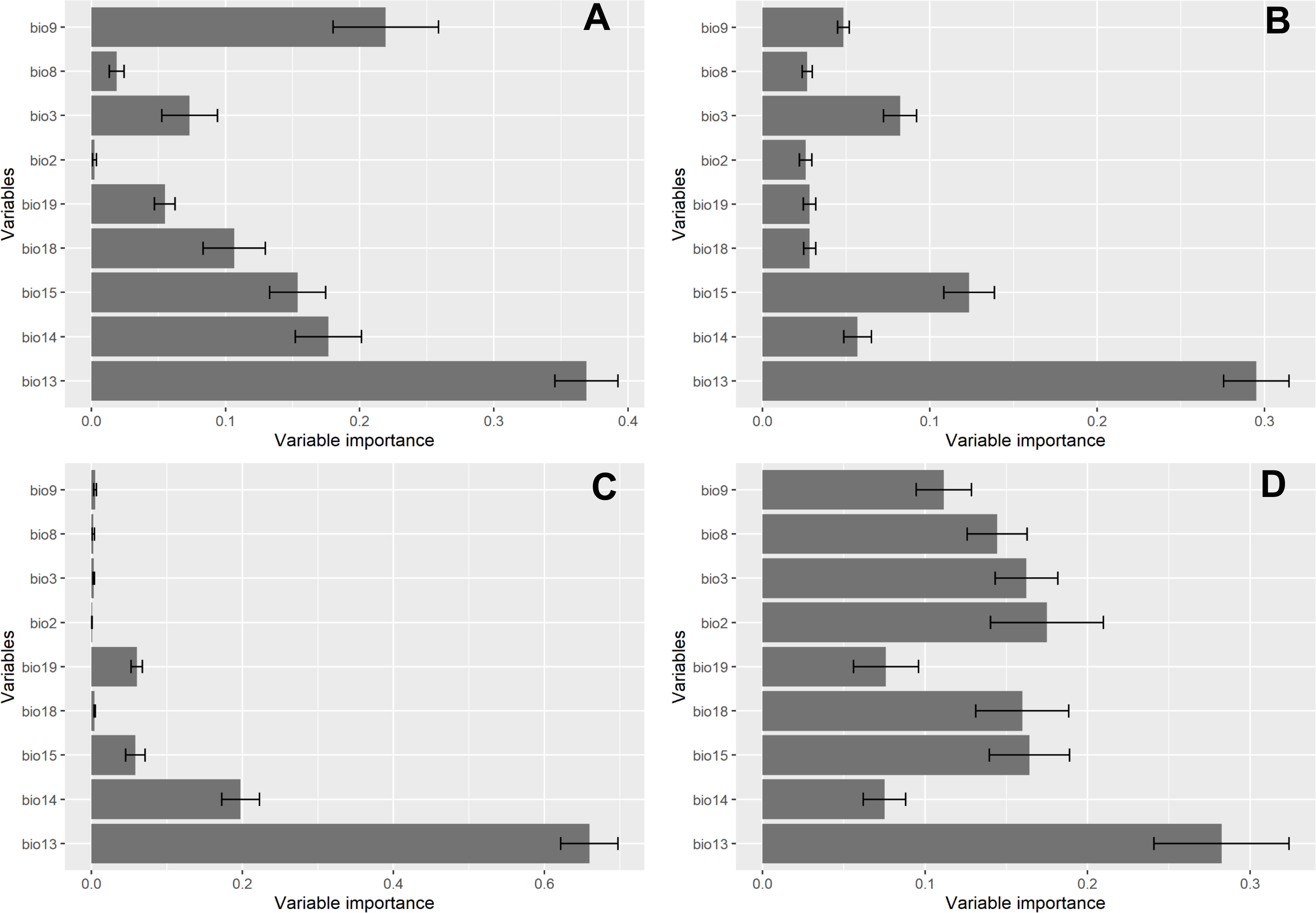
Figure 4 The contribution of the selected environmental predictors for modelling the potential distribution of R.invadens, with (A) maxent; (B) RF; (C) BRT, and (D) SVM. The bars indicate 95% confidence interval.
3.3 Predicting the habitat suitability of R. invadens under current climatic conditions
Under the current climatic scenario, the predicted habitat suitability of R. invadens varied among the four models (Figure 5). Countries in East, Central and West African regions were projected by the maxent algorithm with high suitability for R. invadens occurrence under current climatic conditions (Figure 5A). Similarly, maxent predicted high suitability areas for R. invadens to thrive in Thailand, India, Vietnam, Bangladesh, Laos, Myanmar, and Cambodia (Figure 5A). These areas were also projected by the RF algorithm to be appropriate for R. invadens establishment (Figure 5B). However, the predicted suitable areas by RF were lower than those predicted by maxent. In contrast, the most predicted areas by maxent across the world were projected by BRT to be either low or medium suitable under current climatic conditions (Figure 5C). The prediction based on the SVM algorithm projected high suitability for R. invadens occurrence in Ethiopia, South Sudan, Cameroon, Nigeria, Côte d’Ivoire, Ghana, Togo, Benin, India, Vietnam, Thailand, Bangladesh, Myanmar, Laos, Cambodia and some parts of Brazil (Figure 5D). Overall, the maxent algorithm gave the best prediction for the ecological niche of R. invadens compared to the other three algorithms.
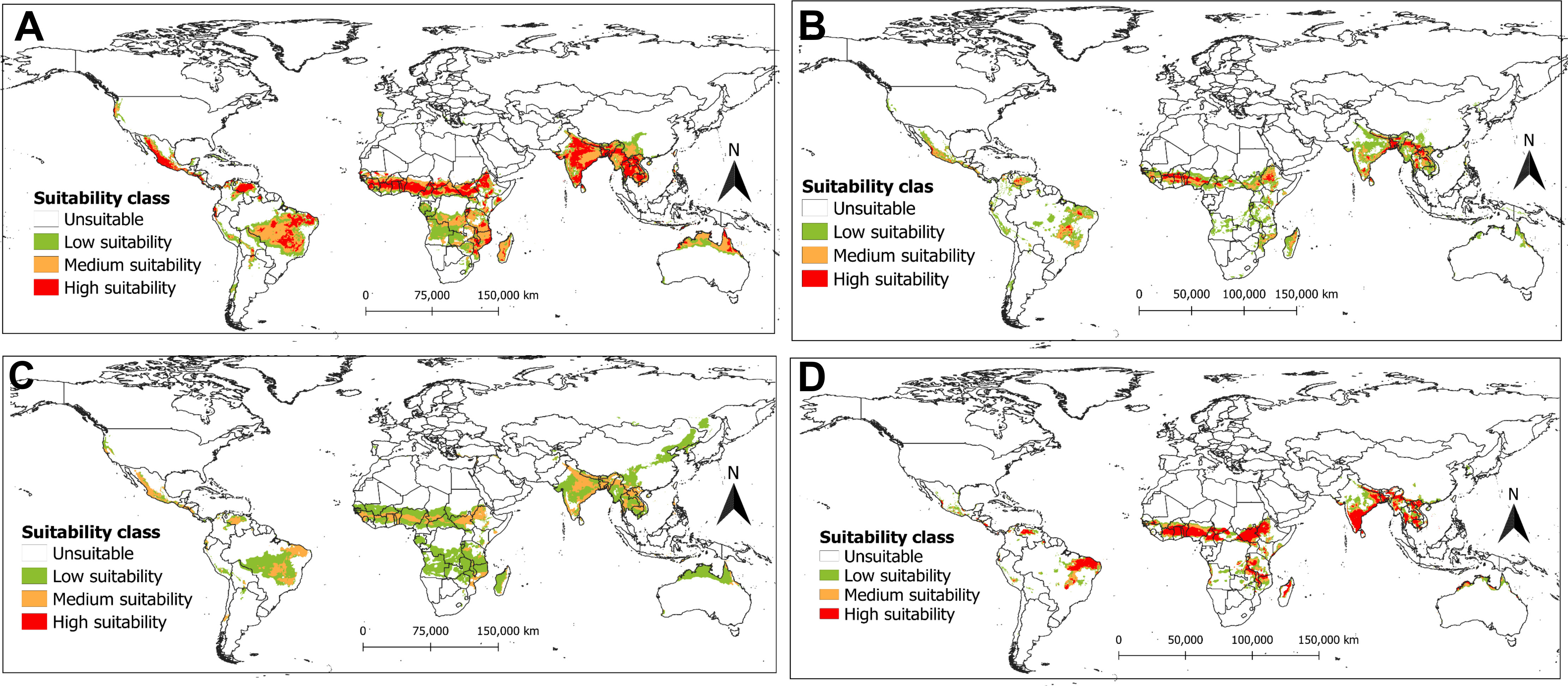
Figure 5 The potential distribution (habitat suitability) of R. invadens under the current climate scenario projected using four machine learning algorithms (A) Maxent; (B) RF; (C) BRT; and (D) SVM.
3.4 Predicting the habitat suitability of R. invadens under future climatic scenarios
The future projection for both SSP2-4.5 and SSP5-8.5 scenarios for the years 2050s revealed that the suitable habitat of R. invadens establishment would increase in the future with a slightly higher risk projected for the SSP2.4.5 scenario (Figure 6), compared to SSP5-8.5 scenarios (Figure 7). The highly suitable areas predicted by maxent were in East, Central, West and some regions in Southern Africa, with an increase in the risk under both SSP2-4.5 and SSP5-8.5 scenarios (Figures 6A, 7A). The same is true for India, Laos, Bangladesh, Vietnam, Myanmar, Thailand, Cambodia and Brazil, Venezuela and Mexico (Figures 6A, 7A). The RF algorithm predicts range expansion in suitable habitats by 2050s, with most areas projected in Ethiopia, South Sudan, Cameroon, Nigeria, Togo, Ghana, Côte d’Ivoire, and Benin (Figures 6B, 7B). Although BRT projected some low suitable areas in China which were not predicted by other models, the predicted climate suitability for R. invadens occurrence was classified as low or medium under both SSP2-4.5 and SSP5-8.5 scenarios (Figures 6C, 7C). However, most areas predicted by SVM for the years 2050s fall in the category of highly suitable which agreed to some extent with maxent prediction, regardless of climatic scenario (Figures 6D, 7D). Though these models produced different results, their future prediction for the year 2070s under both SSP2-4.5 and SSP5-8.5 showed that the invasion risk by R. invadens would highly increase as its suitable habitat for the establishment will increase under future climatic scenarios (Figures 8A-D, 9A-D). The models’ projection showed the range expansion of R. invadens in sub-Saharan Africa, China and Australia by 2070s (Figures 8A-D, 9A-D). However, the risk of R. invadens in 2070s is projected to be slightly higher under the SSP2-4.5 scenario compared to the SSP5-8.5 scenario (Figures 8A-D, 9A-D).
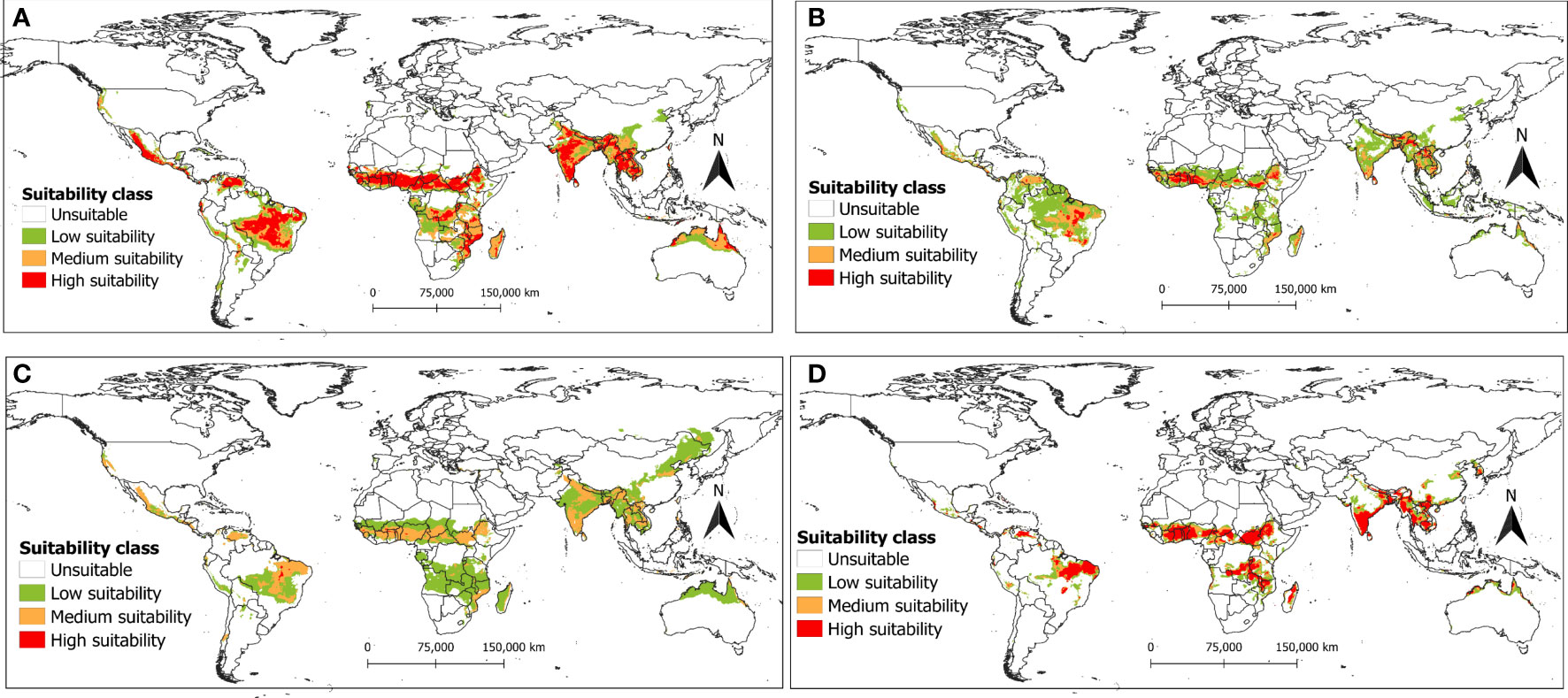
Figure 6 The potential distribution (habitat suitability) of R. invadens under the future clinate change SSP2-4.5 scenario for the year 2050s predicted using four machine learning algorithms with (A) maxent; (B) RF; (C) BRT; and (D) SVM.
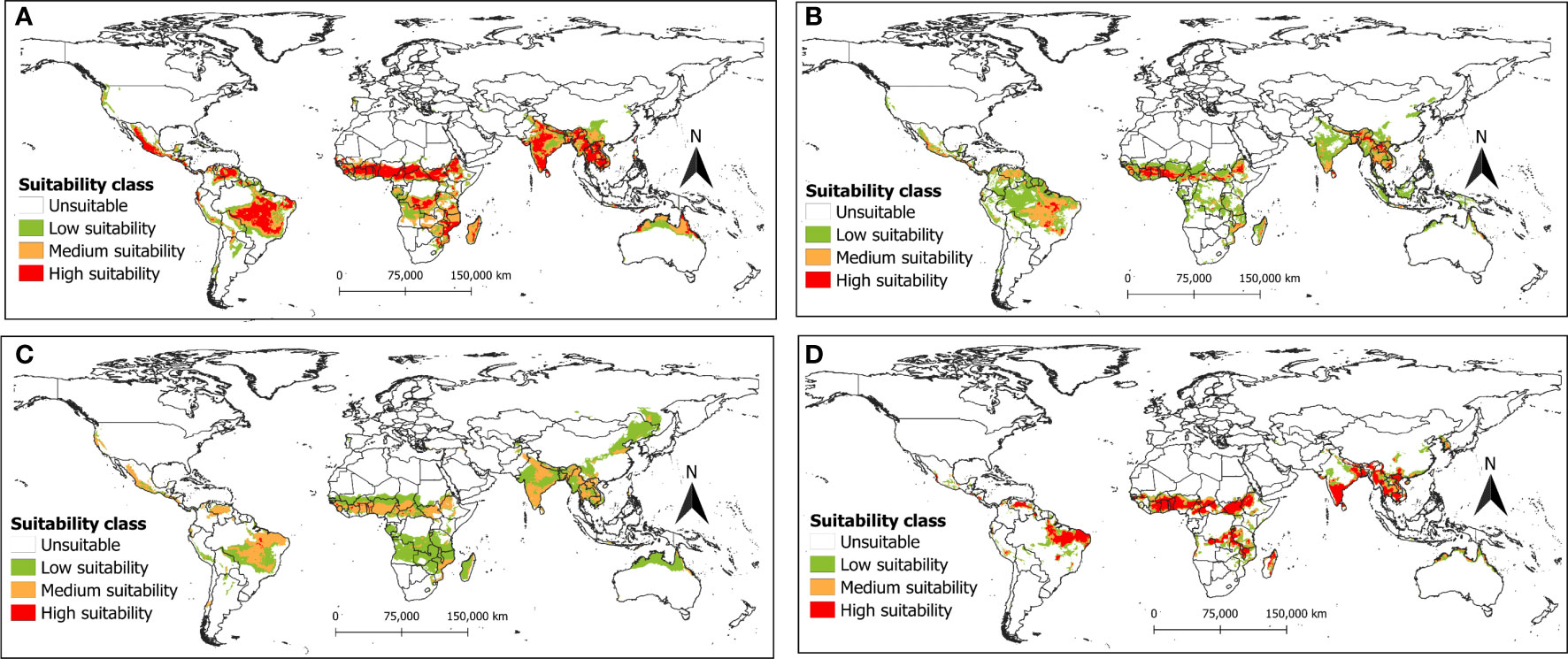
Figure 7 The potential distribution (habitat suitability) of R. invadens under the future climate change scenario SSP5-8.5 for the year 2050s predicted using four machine learning algorithms with (A) maxent; (B) RF; (C) BRT; and (D) SVM.
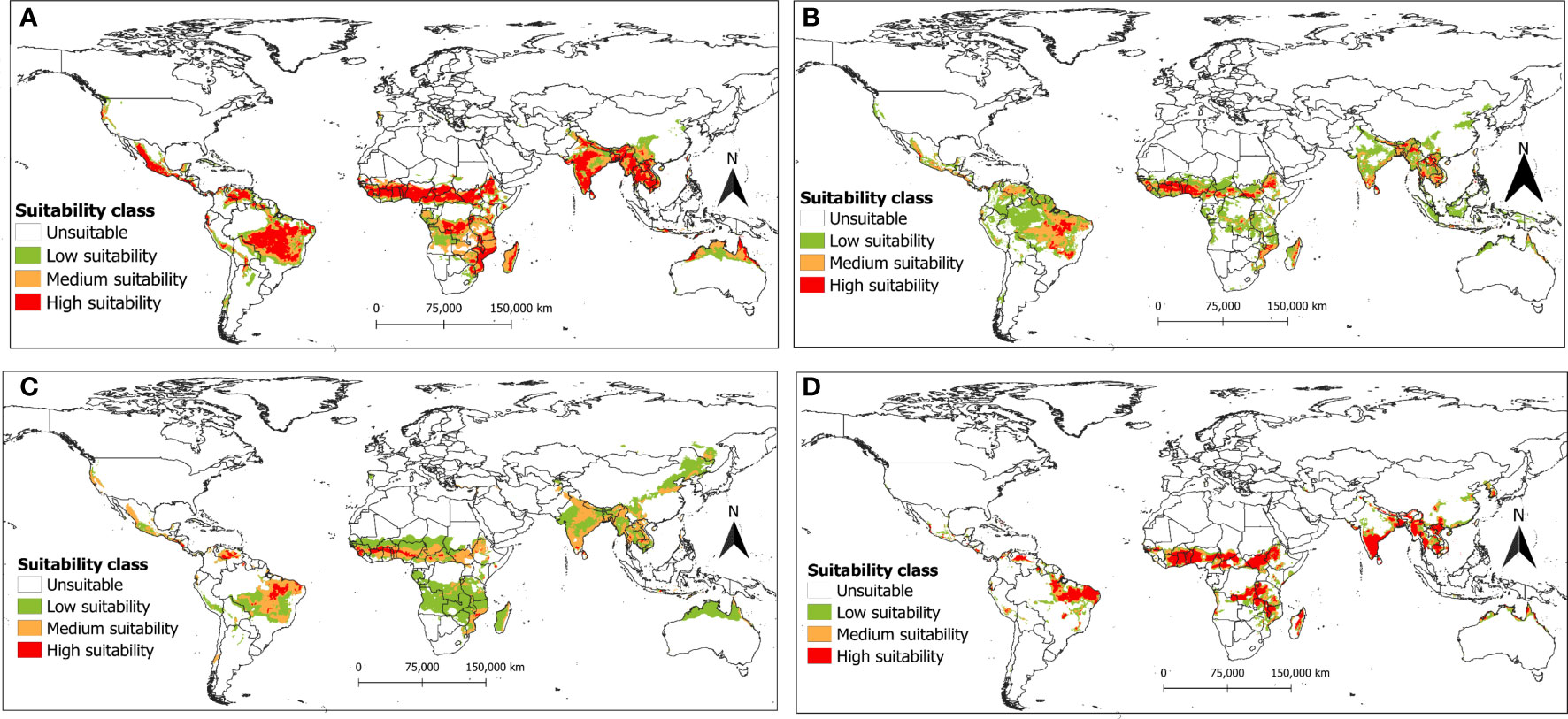
Figure 8 The potential distribution (habitat suitability) of R. invadens under future climate change scenario SSP2-4.5 for the year 2070s is predicted using four machine learning algorithms with (A) maxent; (B) RF; (C) BRT; and (D) SVM.
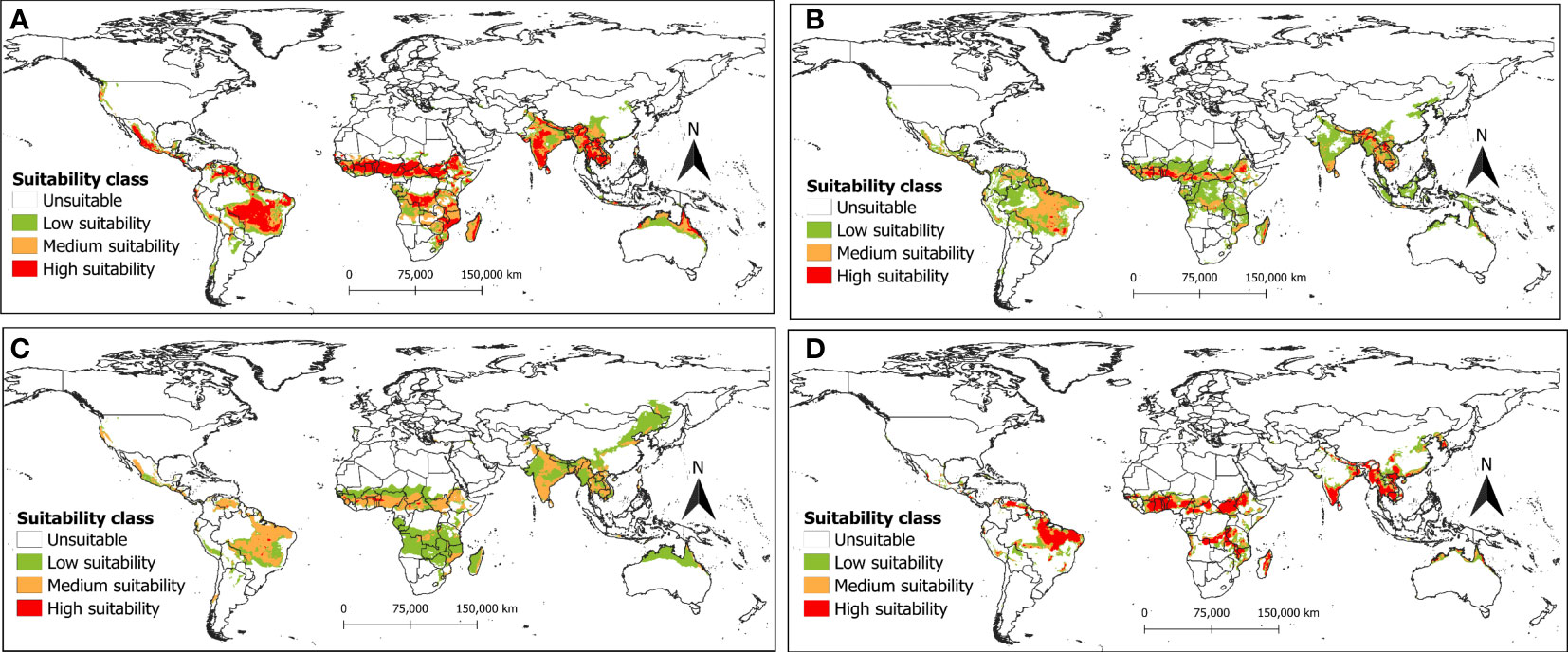
Figure 9 The potential distribution (habitat suitability) of R. invadens under future climate change scenario SSP5-8.5 for the year 2070s is predicted using four machine learning algorithms with (A) maxent; (B) RF; (C) BRT; and (D) SVM.
3.5 Ensembling the prediction of R. invadens occurrence under different scenarios
The ensemble prediction combined the results of the four models using a weighted average of each pixel to project the suitable areas for R. invadens occurrence under current and future climate change scenarios (SSP2-4.5 and SSP5-8.5) (Figures 10A-E). Overall, the ensemble model predicted the suitable habitat for R. invadens occurrence with high performance and accuracy (AUC = 0.96, TSS = 0.85, COR = 0.84 and deviance = 0.22). Under current climatic conditions, most areas in Africa are predicted to be either low or medium suitable for R. invadens establishment except Ethiopia, Nigeria, Benin, Côte d’Ivoire, Togo, and Ghana, where the risk is projected to be higher (Figure 10A). However, under the future scenarios (SSP2-4.5 and SSP5-8.5), an expansion in highly suitable areas is expected, especially in India, Myanmar, Bangladesh, Thailand, Laos, Vietnam, Cambodia and Brazil, Venezuela, and Mexico (Figures 10B-E). Similarly, in Africa, the risk is expected to be higher in Ethiopia, South Sudan, Cameroon, Nigeria, Mozambique, Côte d’Ivoire, Benin, Ghana, Togo, Madagascar, Central Africa Republic, Rwanda, and the Democratic Republic of the Congo (Figures 10B-E). Overall, the suitable areas in the future are predicted to be higher for the year 2070s (Figures 10D, E), compared to 2050s (Figures 10B, C), indicating that the changing climate may increase the invasion risk by R. invadens. The projection also showed that the risk of R. invadens would slightly be higher under SSP2-4.5 scenario compared to SSP5-8.5 scenario, regardless of the year of projection (Figures 10B-E)
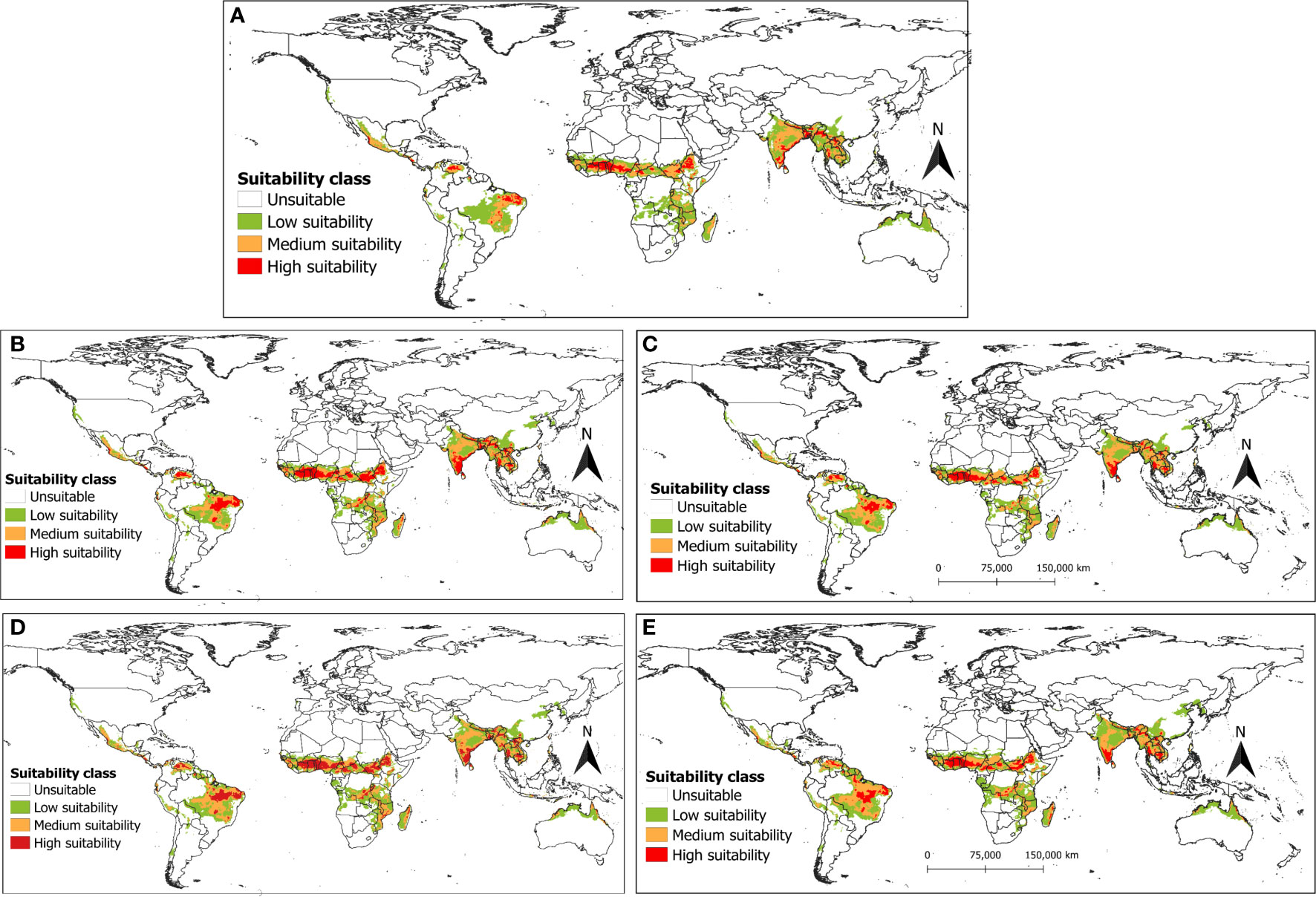
Figure 10 The potential distribution (habitat suitability) of R. invadens projected using the ensemble of the four machine learning algorithms (Maxent, RF, BRT and SVM) with (A) ensemble projection under the current climatic scenario; (B) ensemble projection for the future scenario SSP2-4.5 for the year 2050s; (C) ensemble projection for the future SSP5-8.5 scenario for the year 2050s; (D) ensemble projection for the future SSP2-4.5 scenario for the year 2070s and (E) ensemble projection for the future SSP5-8.5 scenario for theyear2070s.
4 Discussion
Rastrococcus invadens is an invasive pest which causes massive economic losses to various fruit trees in Africa and Asia. Recently, this pest has expanded its geographical range to new areas in East Africa (IPPC, 2019; IPPC, 2022) and French Guiana in Southern America (Germain et al., 2015). This indicates that R. invadens may continue to spread further in the future causing huge damage to fruit crops. Although it is an important pest in the horticultural industry, the potential distribution under current and future climate change scenarios is largely unknown to prevent the invasion of this R. invadens to new areas. Therefore, in the present study, we projected the suitable habitat of R. invadens worldwide using four machine learning algorithms and their ensemble (maxent, RF, BRT and SVM) under two climate change scenarios (SSP2-4.5 and SSP5-8.5). These models were developed by utilizing the same occurrence dataset of R. invadens using the method developed by Naimi and Araújo, 2016 and Araújo et al., 2019. Our results revealed a high prediction accuracy by the four algorithms with AUC ranging from 0.96 to 0.99, TSS ranging between 0.88 and 0.95, COR ≥ 0.74 and deviance ≤ 0.3. These findings indicate that the four algorithms projected the habitat of R. invadens occurrence with high predictive power and accuracy (Elith et al., 2010).
The predicted habitat suitability results of the four algorithms were relatively different though the same dataset was used for modelling. This could be attributed to the fact that different algorithms employ different methodologies for predicting the habitat suitability of the species, and therefore, different outputs are expected when various models are fitted to the same dataset (Naimi and Araújo, 2016). Similar results were found in early studies that employed different algorithms to project the potential distribution of arthropods using the same dataset (i.e., Hao et al., 2019; Guan et al., 2020; Azrag et al., 2022). Nonetheless, our results showed that the maxent algorithm was the best among the four models in projecting the suitable habitat of R. invadens confirming its outstanding performance in predicting the potential distribution of many species (Norberg et al., 2019; Valavi et al., 2022). However, the results of Maxent, RF and SVM showed a huge overlap, especially in West Africa and Asia, where R. invadens is known to be well-established. We joined the results of the four models together using an ensemble function to maximize the prediction accuracy of R. invadens. Joining single models in an ensemble has been recommended for habitat suitability modelling rather than using an individual model (Capinha et al., 2011; Naimi and Araújo, 2016). This is due to the fact that the reliability of the individual model has some uncertainty and therefore this issue could be resolved by utilizing several algorithms and their ensemble to maximise the projection accuracy by averaging the weight of each pixel in each model (Capinha et al., 2011; Naimi and Araújo, 2016).
Under the current climatic conditions, models’ predictions were consistent with the current known distribution of R. invadens in Togo, Côte d’Ivoire, Benin, Nigeria, Ghana, Burkina Faso, Sierra Leone, and Senegal (Narasimham and Chacko, 1988), where our models projected high suitable habitat for R. invadens establishment. In Asia, Bangladesh, India, Laos, Myanmar, Cambodia, Thailand, and Vietnam were projected by maxent, RF and SVM to have highly suitable areas for R. invaden to thrive. Furthermore, the individual models, as well as the ensemble projection, showed medium to high suitable areas for R. invadens in Brazil, Venezuela, Mexico, Ethiopia, South Sudan, Central Africa Republic, Kenya, Tanzania, Rwanda, Burundi Malawi, Zambia, Mozambique, and Madagascar. Since R. invadens has recently been reported in Rwanda and Burundi (IPPC, 2019; IPPC, 2022), it is posing a serious risk of invasion to the neighbouring countries especially Tanzania and Uganda as well as the whole East African region. The same is true for Southern America where the pest currently exists in French Guiana (Germain et al., 2015), posing a serious threat to Suriname and Brazil. The future prediction showed that the invasion risk by R. invadens would increase especially in sub-Saharan Africa and South America, regardless of the model used for the prediction. Models’ projections showed a range expansion of the pest in West and East African countries where the climate will be more appropriate than the current scenario, especially under the SSP2-4.5 scenario. Similarly, an increase in climate suitability is projected in South America which raises the alert of invasion risk of this pest in South America. This indicates that there is an urgent need for strong phytosanitary management and quarantine restrictions to minimize the invasion risk of R. invadens in countries where the pest has not yet to invade.
In the current study, the precipitation of the wettest month (bio13) and the precipitation of the driest month (bio14) were the most crucial bioclimatic factor that explained the occurrence of R. invadens using the four ecological niche models. Indeed, some studies from West Africa have shown that the amount of rainfall significantly affects the population abundance of R. invadens (Hala et al., 2011; Fall et al., 2017a). Rainfall could positively impact the population abundance of R. invadens at the beginning of the rainfall due to the vegetative growth, particularly the new leaves that have high nutrients for the pest to develop (Hala et al., 2011). However, the frequent and heavy rainfall washes R. invadens from the plant leaves and this results in a decline in the population abundance (Hala et al., 2011; Fall et al., 2017b). Apart from the precipitation, temperature variables, especially isothermality (bio3) and the mean temperature of the driest quarter (bio9) also relatively contributed to the occurrence of R. invadens. In fact, previous studies demonstrated that temperature is the most crucial environmental variable that affects insect developmental time, survivorship and reproduction (Ahmed et al., 2016; Azrag et al., 2018). To this end, our results clearly indicate that the change in temperature and the amount of precipitation are vital in evaluating the habitat suitability and establishment of R. invadens.
The change in climate especially temperature increase and change in precipitation has accelerated the spread of invasive species (Aidoo et al., 2022c), and R. invadens is no exception as the current study shows an increase in suitable areas under future climatic conditions. Indeed, the change in environmental variables significantly impacts the geographical distribution, reproductive success, abundance, establishment, and survival of insect species (Ahmed et al., 2016; Azrag et al., 2018). Therefore, climate change might have a considerable impact on the distribution and spread of R. invadens toward new areas and this might lead to huge crop losses. In this regard, there is an urgent need to develop effective phytosanitary measures and efficient control strategies to prevent future spread and curb the current invasion. Identifying the areas that are at high risk of R. invadens invasion is critical for surveillance, monitoring and developing management strategies. In this regard, our prediction will be useful for national plant protection and regulatory organizations, especially in uninvaded countries to develop pest risk analysis areas to prevent the invasion of R. invadens and safeguard future spread.
Even though we employed four machine learning algorithms and their ensembled weighted averages to project the potential distribution and habitat suitability of R. invadens, our study has certain limitations. In this study, we only utilized environmental variables as predictors to project the suitable habitat for R. invadens establishment. There are some important biotic factors like natural enemies such as parasitoids and predators and host plants that determine the potential distribution and establishment of the pest, which were not considered in this study. In addition, were did not incorporate topographic variables like elevation, aspect and slope in our model. These factors might influence the dispersal capacity of insects. Nevertheless, predicting the potential distribution and suitable habitat of the pest using environmental variables provides relevant information on the ecological niche of the insect species, hence helping in decision-making and developing appropriate management measures against the pest (Biber-Freudenberger et al., 2016; Tepa-yotto et al., 2021; Azrag et al., 2022). Another limitation of our study is that the georeferenced points were obtained only from West Africa and Asia where the pest is currently known to occur. There are some unconfirmed reports of R. invadens in some countries in South-East Asia, where our models showed unsuitable areas of this pest. Adding more georeferenced records from the newly invaded areas in the future could certainly improve the prediction. Nonetheless, our results provide an important overview and raise an alert on the invasion risk of R. invadens.
In conclusion, this is the first study that predicted the habitat suitability of R. invadens occurrence worldwide. We used four machine learning algorithms (maxent, RF, BRT and SVM) to predict the potential distribution of R. invadens. The evaluation metrics including AUC, TSS, COR and deviance were excellent for the four models, suggesting that these models well predicted the habitat suitability of R. invadens. However, the maxent algorithm was the best among the four models in projecting the suitable habitat of R. invadens confirming its outstanding performance in predicting the potential distribution of many species. Due to the fact that ecological niche models have some levels of uncertainty, we combined the results of the four models in ensembled layers to maximize the prediction accuracy. Our findings could serve as an early warning tool and a guide that could inform policy formulation and also guide to prevent further spread and invasion of this pest to new areas. The results also could help in developing an effective management strategy against R. invadens adapted to specific agroecological zones.
Data availability statement
The original contributions presented in the study are included in the article/supplementary material. Further inquiries can be directed to the corresponding author.
Author contributions
AA: conceptualization, data curation, formal analysis, investigation methodology, software, validation, visualization, writing - original draft, writing - review & editing. SM: conceptualization, funding acquisition, investigation, project administration, supervision, validation, writing - review & editing. SN: conceptualization, investigation, supervision, validation, writing - review & editing. SE: conceptualization, funding acquisition, investigation, project administration, supervision, validation, writing - review & editing. All authors contributed to the article and approved the submitted version.
Funding
This research received financial support from the Norwegian Agency for Development Cooperation, the section for research, innovation and higher education grant number RAF‐3058 KEN‐18/0005, the International Development Research Centre (IDRC-Canada) and the Australian Centre for International Agricultural Research (ACIAR). The authors gratefully acknowledge the financial support granted to icipe core funding by the following organizations and agencies: UK’s Foreign, Commonwealth and Development Office (FCDO); the Swedish International Development Cooperation Agency (Sida); the Swiss Agency for Development and Cooperation (SDC); the Federal Democratic Republic of Ethiopia; and the Government of the Republic of Kenya. The views ex‐ pressed herein do not necessarily reflect the official opinion of the donors.
Conflict of interest
The authors declare that the research was conducted in the absence of any commercial or financial relationships that could be construed as a potential conflict of interest.
Publisher’s note
All claims expressed in this article are solely those of the authors and do not necessarily represent those of their affiliated organizations, or those of the publisher, the editors and the reviewers. Any product that may be evaluated in this article, or claim that may be made by its manufacturer, is not guaranteed or endorsed by the publisher.
References
Agounké D., Agricola U., Bokonon-Ganta H. A. (1988). Rastrococcus invadens Williams (Hemiptera: Pseudococcidae), a serious exotic pest of fruit trees and other plants in West Africa. Bull. Entomol. Res. 78, 695–702. doi: 10.1017/S0007485300015558
Agricola U., Agounké D., Fischer H. U., Moore D. (1989). The control of Rastrococcus invadens Williams ( Hemiptera: Pseudo- coccidae ) in Togo by the introduction of Gyranusoidea tebygi Noyes ( Hymenoptera: Encyrtidae ). Bull. Entomol. Res. 79, 671–678. doi: 10.1017/S0007485300018824
Ahmed A. G., Murungi L. K., Babin R. (2016). Developmental biology and demographic parameters of antestia bug Antestiopsis thunbergii (Hemiptera: Pentatomidae ), on Coffea arabica ( Rubiaceae ) at different constant temperatures. Int. J. Trop. Insect Sci. 36, 119–127. doi: 10.1017/S1742758416000072
Aidoo O. F., Ding F., Ma T., Jiang D., Wang D., Hao M., et al. (2022a). Determining the potential distribution of Oryctes monoceros and Oryctes rhinoceros by combining machine-learning with high-dimensional multidisciplinary environmental variables. Sci. Rep. 12, 1–14. doi: 10.1038/s41598-022-21367-1
Aidoo O. F., Hao M., Ding F., Wang D., Jiang D., Ma T., et al. (2022b). The impact of climate change on potential invasion risk of Oryctes monoceros Worldwide. Front. Ecol. Evol. 10. doi: 10.3389/fevo.2022.895906
Aidoo O. F., Souza P. G. C., da Silva R. S., Picanço M. C., Kyerematen R., et al. (2022c). Climate-induced range shifts of invasive species (Diaphorina citri Kuwayama). Pest Manage. Sci. 78, 2534–2549. doi: 10.1002/ps.6886
Allouche O., Tsoar A., Kadmon R. (2006). Assessing the accuracy of species distribution models: Prevalence, kappa and the true skill statistic (TSS). J. Appl. Ecol. 43, 1223–1232. doi: 10.1111/j.1365-2664.2006.01214.x
Araújo M. B., Anderson R. P., Barbosa A. M., Beale C. M., Dormann C. F., Early R., et al. (2019). Standards for distribution models in biodiversity assessments. Sci. Adv. 5, 1–12. doi: 10.1126/sciadv.aat4858
Azrag A. G. A., Mohamed S. A., Ndlela S., Ekesi S. (2022). Predicting the habitat suitability of the invasive white mango scale, Aulacaspis tubercularis; Newstead 1906 (Hemiptera: Diaspididae) using bioclimatic variables. Pest Manage. Sci. 78, 4114–4126. doi: 10.1002/ps.7030
Azrag A. G. A., Pirk C. W. W., Yusuf A. A., Pinard F., Niassy S., Mosomtai G., et al. (2018). Prediction of insect pest distribution as influenced by elevation: Combining field observations and temperature-dependent development models for the coffee stink bug, Antestiopsis thunbergii ( Gmelin ). PloS One 13, e0199569. doi: 10.1371/journal.pone.0199569
Bale J. S., Masters G. J., Hodkinson I. D., Awmack C., Bezemer T. M., Brown V. K., et al. (2002). Herbivory in global climate change research: Direct effects of rising temperature on insect herbivores. Glob. Change Biol. 8, 1–16. doi: 10.1046/j.1365-2486.2002.00451.x
Biber-Freudenberger L., Ziemacki J., Tonnang H. E. Z., Borgemeister C. (2016). Future risks of pest species under changing climatic conditions. PloS One 11, e0153237. doi: 10.1371/journal.pone.0153237
Bokonon-ganta A. H., De Groote H., Neuenschwander P. (2002). Socio-economic impact of biological control of mango mealybug in Benin. Agric. Ecosyst. Environ. 93, 367–378. doi: 10.1016/S0167-8809(01)00337-1
Bokonon-Ganta A. H., Neuenschwander P. (1995). Impact of the biological control agent Gyranusoidea tebygi Noyes (Hymenoptera: Encyrtidae) on the mango mealybug, Rastrococcus invadens Williams (Homoptera: Pseudococcidae), in Benin. Biocontrol Sci. Technol. 5, 95–108. doi: 10.1080/09583159550040042
Boria R. A., Olson L. E., Goodman S. M., Anderson R. P. (2014). Spatial filtering to reduce sampling bias can improve the performance of ecological niche models. Ecol. Modell. 275, 73–77. doi: 10.1016/j.ecolmodel.2013.12.012
Boussienguet J., Herren H. (1992). Introduction et dynamique de dispersion de la cochenille du manguier, Rastrococcus invadens Williams (Hom., Pseudococcidae) au Gabon. Mémoirs. la. Société. R. Belge. en Entomol. 35, 363–367.
CABI (2022). “Rastrococcus invadens,” in Invasive species compendium (Wallingford, UK: International). Available at: https://www.cabi.org/iscCAB.
Capinha C., Leung B., Anastácio P. (2011). Predicting worldwide invasiveness for four major problematic decapods: An evaluation of using different calibration sets. Ecograp. (Cop.). 34, 448–459. doi: 10.1111/j.1600-0587.2010.06369.x
Collins W. J., Bellouin N., Doutriaux-Boucher M., Gedney N., Halloran P., Hinton T., et al. (2011). Development and evaluation of an Earth-System model - HadGEM2. Geosci. Model. Dev. 4, 1051–1075. doi: 10.5194/gmd-4-1051-2011
Early R., Bradley B. A., Dukes J. S., Lawler J. J., Olden J. D., Blumenthal D. M., et al. (2016). Global threats from invasive alien species in the twenty-first century and national response capacities. Nat. Commun. 7, 1–9. doi: 10.1038/ncomms12485
Elith J., Graham C. H. (2009). Do they? How do they? Why do they differ? On finding reasons for differing performances of species distribution models. Ecography 32, 66–77. doi: 10.1111/j.1600-0587.2008.05505.x
Elith J., Kearney M., Phillips S. (2010). The art of modelling range-shifting species. Methods Ecol. Evol. 1, 330–342. doi: 10.1111/j.2041-210x.2010.00036.x
Elith J., Leathwick J. R., Hastie T. (2008). A working guide to boosted regression trees. J. Anim. Ecol. 77, 802–813. doi: 10.1111/j.1365-2656.2008.01390.x
Elith J., Phillips S. J., Hastie T., Dudík M., Chee Y. E., Yates C. J. (2011). A statistical explanation of MaxEnt for ecologists. Divers. Distrib. 17, 43–57. doi: 10.1111/j.1472-4642.2010.00725.x
Fall A., Sarr A. G. R. J., Seye F., Toure M., Ndione R. D. (2021). Morphometric characterization of Rastrococcus invadens on four varieties of mango trees ( Pout, Thies ). World J. Adv. Res. Rev. 9, 116–124. doi: 10.30574/wjarr.2021.9.3.0072
Fall A., Toure M., Seye F., Ndiaye M. (2017a). Inventaire des plantes hôtes comestibles et évaluation du degré d’infestation par Rastrococcus invadens (Willams 1986) (Homoptera, Pseudococcidae) au Sénégal. Afrique Sci. 13, 344–353.
Fall A., Toure M., Seye F., Ndione R. D., Badiane T. S., Ndiaye M. (2017b). Evaluation damage caused by Rastrococcus invadens (Willams. 1986) (Homoptera. Pseudococcidae) on mango in Casamance (Senegal). J. Biotechnol. Res. 3, 114–119.
Fujino J., Nair R., Kainuma M., Masuif T., Matsuok Y. (2006). Multi-gas mitigation analysis on stabilization scenarios using aim global model. Energy J. 27, 343–353. doi: 10.5547/ISSN0195-6574-EJ-VolSI2006-NoSI3-17
Germain J., Laplace D., Devarieux A., Boavida C. (2015). First records of the mealybug Rastrococcus invadens Williams (Hemiptera: Pseudococcidae) in French Guiana and the Americas. Zootaxa 3905, 447–450. doi: 10.11646/zootaxa.3905.3.11
Guan B.c., Guo H.j., Chen S., Li D.m., Liu X., Gong X., et al. (2020). Shifting ranges of eleven invasive alien plants in China in the face of climate change. Ecol. Inform. 55, 101024. doi: 10.1016/j.ecoinf.2019.101024
Hala N., Bajougué D., Achille N. A., Félix C., Alphonse N. Y., Mamadou D. (2011). Population dynamics of the mango mealybug, Rastrococcus invadens Williams (Homoptera: Pseudococcidae) in northern Côte d’Ivoire. J. Anim. Plant Sci. 12, 1481–1492.
Hala N., Kehe K., Allou M. (2004). Incidence de la cochenille farineuse du manguier Rastrococcus invadens Williams 1986 (Homoptera; Pseudococcidae) En Côte d’ivoire. Agron. Africaine. 16, 29–36. doi: 10.4314/aga.v16i3.1651
Hao T., Elith J., Guillera-Arroita G., Lahoz-Monfort J. J. (2019). A review of evidence about use and performance of species distribution modelling ensembles like BIOMOD. Divers. Distrib. 25, 839–852. doi: 10.1111/ddi.12892
Hijmans R. J., Cameron S. E., Parra J. L., Jones P. G., Jarvis A. (2005). Very high resolution interpolated climate surfaces for global land areas. Int. J. Climatol. 25, 1965–1978. doi: 10.1002/joc.1276
IPPC (2019) Official Pest Reports – Rwanda (RWA-02/1 of 2019-10-28) Mango mealybug: Rastrococcus invadens Williams. Available at: https://www.ippc.int/en/countries/Rwanda/pestreports/2019/10/mango-mealybug-rastrococcus-invadens-williams/.
IPPC (2022) Official Pest Reports – Burundi (BDI-01/1 of 2022-01-16) Cochenille farineuse du manguier. Available at: https://www.ippc.int/fr/countries/Burundi/pestreports/2022/01/cochenille-farineuse-du-manguier/.
Istifanus A. P., Azrag G. A., Pirk C. W. W., Yusuf A. A. (2023). Predicting the habitat suitability and distribution of two species of mound-building termites in Nigeria using bioclimatic and vegetation variables. Diversity 15, 157. doi: 10.3390/d15020157
Karatzoglou A., Hornik K., Smola A., Zeileis A. (2004). kernlab - An S4 package for kernel methods in R. J. Stat. Software 11, 1–20. doi: 10.18637/jss.v011.i09
Lanjar A. G., Rustamani M. A., Solangi A. W. (2015). Effect of botanical extract against mango mealy bug Drosicha mangiferae (green). Sci. Int. 27, 343–346.
Matokot L., Reyd G., Malonga P., Le Ro B. (1992). Dynamique des populations de Rastrococcus invadens [Hom.: Pseudococcidae] au Congo; Influence de l’introduction accidentelle du parasitoïde asiatique Gyranusoïdea tebygi [Hym.: Encyrtidae]. Entomophaga 37, 123–140. doi: 10.1007/BF02372981
Merow C., Smith M. J., Edwards T. C., Guisan A., Mcmahon S. M., Normand S., et al. (2014). What do we gain from simplicity versus complexity in species distribution models? Ecograp. (Cop.). 37, 1267–1281. doi: 10.1111/ecog.00845
Merow C., Smith M. J., Silander J. A. (2013). A practical guide to MaxEnt for modeling species’ distributions: What it does, and why inputs and settings matter. Ecograp. (Cop.). 36, 1058–1069. doi: 10.1111/j.1600-0587.2013.07872.x
Mohamed S. A., Dubois T., Azrag A. G., Ndlela S., Neuenschwander P. (2022). Classical biological of key horticultural pests in Africa: successes, challenges, and opportunities. Curr. Opin. Insect Sci. 53, 100945. doi: 10.1016/j.cois.2022.100945
Naimi B., Araújo M. B. (2016). Sdm: A reproducible and extensible R platform for species distribution modelling. Ecograp. (Cop.). 39, 368–375. doi: 10.1111/ecog.01881
Narasimham A. U., Chacko M. J. (1988). Rastrococcus spp . (Hemiptera: Pseudococcidae) and their natural enemies in India as potential biocontrol agents for R. invadens Williams. Bull. Entomol. Res. 78, 703–708. doi: 10.1017/S000748530001556X
Nebie K., Nacro S., Dabire R. A., Otoidobiga L. C. (2018). Compared effects of Metarhizium anisopliae ICIPE 69 and Chlorpyriphos- ethyl on the mango mealybug, Rastrococcus invadens Williams (Homoptera: Pseudococcidae) and its parasitoids in western Burkina Faso. Int. J. Biol. Chem. Sci. 12, 2738–2752. doi: 10.4314/ijbcs.v12i6.21
Nébié K., Nacro S., Otoidobiga L. C., Dakouo D., Somda I. (2016). Population Dynamics of the mango mealybug Rastrococcus invadens Williams (Homoptera: Pseudococcidea) in Western Burkina Faso. Am. J. Exp. Agric. 11, 1–11. doi: 10.9734/AJEA/2016/24819
Neuenschwander P., Boavida C., Bokonon-ganta A., Gado A., Herren H. R. (1994). Establishment and Spread of Gyranusoidea tebygi Noyes and Anagyrus mangicola Noyes (Hymenoptera: Encyrtidae), Two Biological Control Agents Released against the Mango Mealybug Rastrococcus invadens Williams (Homoptera: Pseudococcidae) in Africa. Biocontrol Sci. Technol. 4, 61–69. doi: 10.1007/bf00343337
Norberg A., Abrego N., Blanchet F. G., Adler F. R., Anderson B. J., Anttila J., et al. (2019). A comprehensive evaluation of predictive performance of 33 species distribution models at species and community levels. Ecol. Monogr. 89, 1–24. doi: 10.1002/ecm.1370
Pal M. (2005). Random forest classifier for remote sensing classification. Int. J. Remote Sens. 26, 217–222. doi: 10.1080/01431160412331269698
Phillips S. J., R. P. Anderson., R. E. Schapire. (2006). Maximum entropy modeling of species geographic distributions. Ecological Modelling. 190, 231–259. doi: 10.1016/j.ecolmodel.2005.03.026
Phillips S. J., Dudík M. (2008). Modeling of species distributions with Maxent: new extensions and a comprehensive evaluation. Ecograp. (Cop.). 31, 161–175. doi: 10.1111/j.0906-7590.2008.5203.x
Pitan O. O. R. (2008). Variations in the population of the mango mealybug Rastrococcus invadens (Homoptera: Pseudococcidae), and its parasitism , in relation to smoke pollution. Int. J. Trop. Insect Sci. 28, 119–125. doi: 10.1017/S174275840806757X
Pitan O. O. R., Mwansat G., Akinyemi S. O. S., Adebayo O. S., Akinlosotu T. A. (2002). Effect of mango mealybug and sooty mould attack on mango and the impact of the released Gyranusoidea tebygi Noyes on yield. Fruits 57, 105–113. doi: 10.1051/fruits
Radosavljevic A., Anderson R. P. (2014). Making better Maxent models of species distributions: Complexity, overfitting and evaluation. J. Biogeogr. 41, 629–643. doi: 10.1111/jbi.12227
Riahi K., Rao S., Krey V., Cho C., Chirkov V., Fischer G., et al. (2011). RCP 8.5-A scenario of comparatively high greenhouse gas emissions. Clim. Change 109, 33–57. doi: 10.1007/s10584-011-0149-y
Shabani F., Kumar L., Ahmadi M. (2016). A comparison of absolute performance of different correlative and mechanistic species distribution models in an independent area. Ecol. Evol. 6, 5973–5986. doi: 10.1002/ece3.2332
Tabor J. A., Koch J. B. (2021). Ensemble models predict invasive bee habitat suitability will expand under future climate scenarios in hawai’i. Insects 12, 443. doi: 10.3390/insects12050443
Tepa-yotto G. T., Tonnang H. E. Z., Goergen G., Subramanian S., Kimathi E., Abdel-rahman E. M., et al. (2021). Global habitat suitability of Spodoptera frugiperda (JE Smith) (Lepidoptera, Noctuidae): key parasitoids considered for its biological control. Insects 12, 273. doi: 10.3390/insects12040273
Tsoar A., Allouche O., Steinitz O., Rotem D., Kadmon R. (2007). A comparative evaluation of presence- only methods for modelling species distribution. Divers. Distrib. 13, 397–405. doi: 10.1111/j.1472-4642.2007.00346.x
Valavi R., Guillera-Arroita G., Lahoz-Monfor J. J., Elith J. (2022). Predictive performance of presence-only species distribution models: a benchmark study with reproducible code. Ecol. Monogr. 92, e01486. doi: 10.1002/ecm.1486
Vapnik V. (2006). Estimation of dependences based on empirical data (New York: Springer Science & Business Media).
Keywords: mango, mealybugs, machine learning algorithms, species distribution, invasive species
Citation: Azrag AGA, Mohamed SA, Ndlela S and Ekesi S (2023) Invasion risk by fruit trees mealybug Rastrococcus invadens (Williams) (Homoptera: Pseudococcidae) under climate warming. Front. Ecol. Evol. 11:1182370. doi: 10.3389/fevo.2023.1182370
Received: 08 March 2023; Accepted: 19 July 2023;
Published: 08 August 2023.
Edited by:
Paulo A. V. Borges, University of the Azores, PortugalReviewed by:
Owusu Fordjour Aidoo, University of Environment and Sustainable Development, GhanaJiufeng Wei, Shanxi Agricultural University, China
Copyright © 2023 Azrag, Mohamed, Ndlela and Ekesi. This is an open-access article distributed under the terms of the Creative Commons Attribution License (CC BY). The use, distribution or reproduction in other forums is permitted, provided the original author(s) and the copyright owner(s) are credited and that the original publication in this journal is cited, in accordance with accepted academic practice. No use, distribution or reproduction is permitted which does not comply with these terms.
*Correspondence: Abdelmutalab G. A. Azrag, YWdhenJhZ0B5YWhvby5jb20=; YWdlc21hbGxhQGljaXBlLm9yZw==
‡ORCID: Abdelmutalab G. A. Azrag, orcid.org/0000-0002-2192-3803
Samira A. Mohamed, orcid.org/0000-0001-6836-7528
Shepard Ndlela, orcid.org/0000-0003-0015-9946
Sunday Ekesi, orcid.org/0000-0001-9787-1360