- 1Department of Geography and Spatial Information Techniques, Ningbo University, Ningbo, China
- 2Ningbo Universities Collaborative Innovation Center for Land and Marine Spatial Utilization and Governance Research at Ningbo University, Ningbo, China
- 3College of Life Sciences, Zaozhuang University, Zaozhuang, China
- 4Geography Department, Humboldt-Universität zu Berlin, Berlin, Germany
- 5Center for Biodiversity Dynamics in a Changing World (BIOCHANGE) and Section for Ecoinformatics and Biodiversity, Department of Biology, Aarhus University, Aarhus, Denmark
The metacommunity concept has been widely used to explain the biodiversity patterns at various scales. It considers the influences of both local (e.g., environmental filtering and biotic interactions) and regional processes (e.g., dispersal limitation) in shaping community structures. Compared to environmental filtering and spatial processes, the influence of biotic interactions on biodiversity patterns in streams has received limited attention. We investigated the relative importance of three ecological processes, namely environmental filtering (including local environmental and geo-climatic factors), spatial processes and biotic interactions (represented by interactions of macroinvertebrates and diatom), in shaping different traits of macroinvertebrate communities in subtropical streams, Eastern China. We applied variance partitioning to uncover the pure and shared effects of different ecological processes in explaining community variation. The results showed that environmental filtering, spatial processes, and biotic interactions jointly determined taxonomic and trait compositions of stream macroinvertebrates. Spatial processes showed a stronger influence in shaping stream macroinvertebrate communities than environmental filtering. The contribution of biotic interactions to explain variables was, albeit significant, rather small, which was likely a result of insufficient representation (by diatom traits) of trophic interactions associated with macroinvertebrates. Moreover, the impact of three ecological processes on macroinvertebrate communities depends on different traits, especially in terms of environmental filtering and spatial processes. For example, spatial processes and environmental filtering have the strongest effect on strong dispersal ability groups; spatial processes have a greater effect on scrapers than other functional feeding groups. Overall, our results showed that the integration of metacommunity theory and functional traits provides a valuable framework for understanding the drivers of community structuring in streams, which will facilitate the development of effective bioassessment and management strategies.
1 Introduction
Understanding the variation in biodiversity patterns and underlying mechanisms is a central topic of community ecology and biogeography (Gaston, 2000; Chase, 2003). Previous research has showed the importance of niche-based processes (e.g., through environmental filtering and biotic interaction) and dispersal-related processes in structuring communities at different scales (Mykra et al., 2007). These two types of processes are not independent of each other, and often work in concert to shape community structure (Logue et al., 2011; Leibold et al., 2017; Guzman et al., 2019).
The metacommunity theory considers both local and spatial processes and provides an excellent framework for understanding the diversity and abundance of species at various scales (Heino, 2013). Many theories have been proposed to explain the metacommunity dynamics, which could be grouped into four paradigms, including species sorting, patch dynamics, mass effects, and neutral models (Leibold et al., 2004; Declerck et al., 2011; Zhou et al., 2020). These four paradigms differ in their emphasis on local processes, regional processes, disturbances and the equivalence of species traits. Species sorting (i.e., “deterministic process”) focuses more on local environmental contexts and presumes that good dispersers have the ability to reach all suitable niches (Cottenie et al., 2003) while mass effects (i.e., “stochastic processes”) place more emphasis on the influence of dispersal on local communities (Mouquet et al., 2003). According to patch dynamics paradigm, environmental filtering does not play an important role in shaping local communities whereas regional dynamics are the consequence of extinction and colonization (Cadotte, 2007). Neutral models assume no differences among species in terms of their traits and model community compositions by species losses, gains and probabilistic colonization (Hubbell and Borda-De-Agua, 2004). Of these four paradigms, species sorting and mass effects are considered as the two main paradigms for simultaneous community construction (Vilmi et al., 2017). However, other paradigms may also have an impact on community constructing processes and these processes may not be mutually exclusive. It is therefore essential to consider the impact of multiple ecological processes on community assembly simultaneously (Winegardner et al., 2012). The life history of macroinvertebrates includes dispersal behaviors that can span multiple spatial scales, making them ideal organisms for studying the mechanisms of metacommunity assembly in stream networks (Brown et al., 2011; Sarremejane et al., 2020).
Macroinvertebrates are widespread and support important ecological functions in freshwater ecosystems (Schmera et al., 2017). They have been widely used in water quality assessment and environmental monitoring due to several reasons, including their short lifespan and rapid responses to environmental change (Armitage et al., 1983). Macroinvertebrate communities are often directly influenced by physiochemical variables in the aquatic environment (Li et al., 2020; Jiang et al., 2021a; Wu et al., 2022a). In addition, climate changes often lead to alterations in environmental conditions that indirectly affect macroinvertebrate communities. For example, changes in temperature affect the survival of aquatic organisms and alter their distribution ranges (Li et al., 2012). Likewise, flow dynamic in stream are strongly affected by precipitation, in turn, posing impacts on macroinvertebrates (Perez Rocha et al., 2018; Hsieh et al., 2022). In addition, macroinvertebrates link primary producers and vertebrate predators in aquatic food web. They feed on diatom, coarse detritus or fine-grained organic matter, and fall prey to species at higher trophic levels, such as fish (Covich et al., 1999; Statzner, 2012). However, only a few studies have included biotic interactions in their analysis when investigating the processes determining community structures (Garcia-Giron et al., 2020; Kurthen et al., 2020). Mountainous streams provide an ideal context for studying the influence of multiple ecological processes on biotic communities. Such areas typically have environmental heterogeneity, complex topographic structure, wide spatial extent, and high levels of biodiversity (Tockner et al., 2010; Wu et al., 2021).
Compared to taxonomic composition, trait composition can directly reflect the influence of environment on species (ecological, behavioral and morphological characteristics). This can help researchers understand the underlying factors of community assembly (Gianuca et al., 2017; Rideout et al., 2022). Traits have received increasing attention in ecological and biogeographical research to study the association between the environment and biodiversity (Meynard et al., 2011; Heino and Tolonen, 2017; Wang et al., 2023). Macroinvertebrates are an excellent model for trait-based research because they vary in morphology, feeding and behavior habits, dispersal ability, and life history strategies (Cummins et al., 2005). Indeed, these traits are essential in determining the success of their survival, dispersal, colonization of new habitats (Jiang et al., 2021b).
To fill the aforementioned knowledge gap, we aim to disentangle the effects of environmental filtering (i.e., local environment and geo-climate), spatial processes, and biotic interactions (i.e., between macroinvertebrates and diatom) on the taxonomic and trait compositions of stream macroinvertebrates. Furthermore, we analyzed the relative importance of ecological processes affecting macroinvertebrate communities with different traits, which is particularly important for biodiversity conservation and management (Doledec and Statzner, 2010). We hypothesize that (H1) environmental filters, spatial processes, and biotic interactions jointly shape the structure of macroinvertebrate communities. Since taxa that share similar ecological niches are often grouped into the same trait categories, replacement of taxa is not necessarily associated with turnover of traits (Tapolczai et al., 2016; Wu et al., 2022a). In addition, dispersal limitation is likely to influence taxonomic compositions of macroinvertebrates among study sites given the large spatial extent of our study area. We therefore hypothesize that (H2) environmental filtering has a greater effect on trait composition, while taxonomic composition is more influenced by spatial processes. As biological and ecological traits are strongly linked to their interactions with the environment, we therefore assume that (H3) the relative importance of local and regional processes in structuring communities varies between groups with different traits. Two specific hypotheses (H3a and H3b) regarding dispersal ability and functional feeding habit are as follows:
a. for dispersal ability groups (DAG), we expect that taxa with stronger dispersal ability are more influences by environmental conditions than weak dispersals. Since macroinvertebrates with strong dispersal ability often can overcome land barrier and move to new suitable habitats, their community similarity can reflect the environmental gradients between habitats (Heino, 2013).
b. for functional feeding groups (FFG), we hypothesized that the influence of biotic interactions on the scraper would be stronger than on the other groups when interactions between benthic diatom and macroinvertebrates were considered because scrapers mainly consume the biofilm on substrates (Heino, 2005; Ramirez and Gutierrez-Fonseca, 2014).
2 Materials and methods
2.1 Study area description
Our study area is the catchment of the Thousand Islands Lake (TIL), which is located in the subtropical monsoon climate zone of Eastern China (Figure 1). The area has a catchment area of approximately 10,080 km2, with an average annual precipitation of 1430 mm. Within the catchment, the sampling sites have an elevation gradient of over 500 m. Studies across such large environmental gradients are extremely helpful in revealing the influence of the environment on community assembly mechanisms (Thrush et al., 2006).
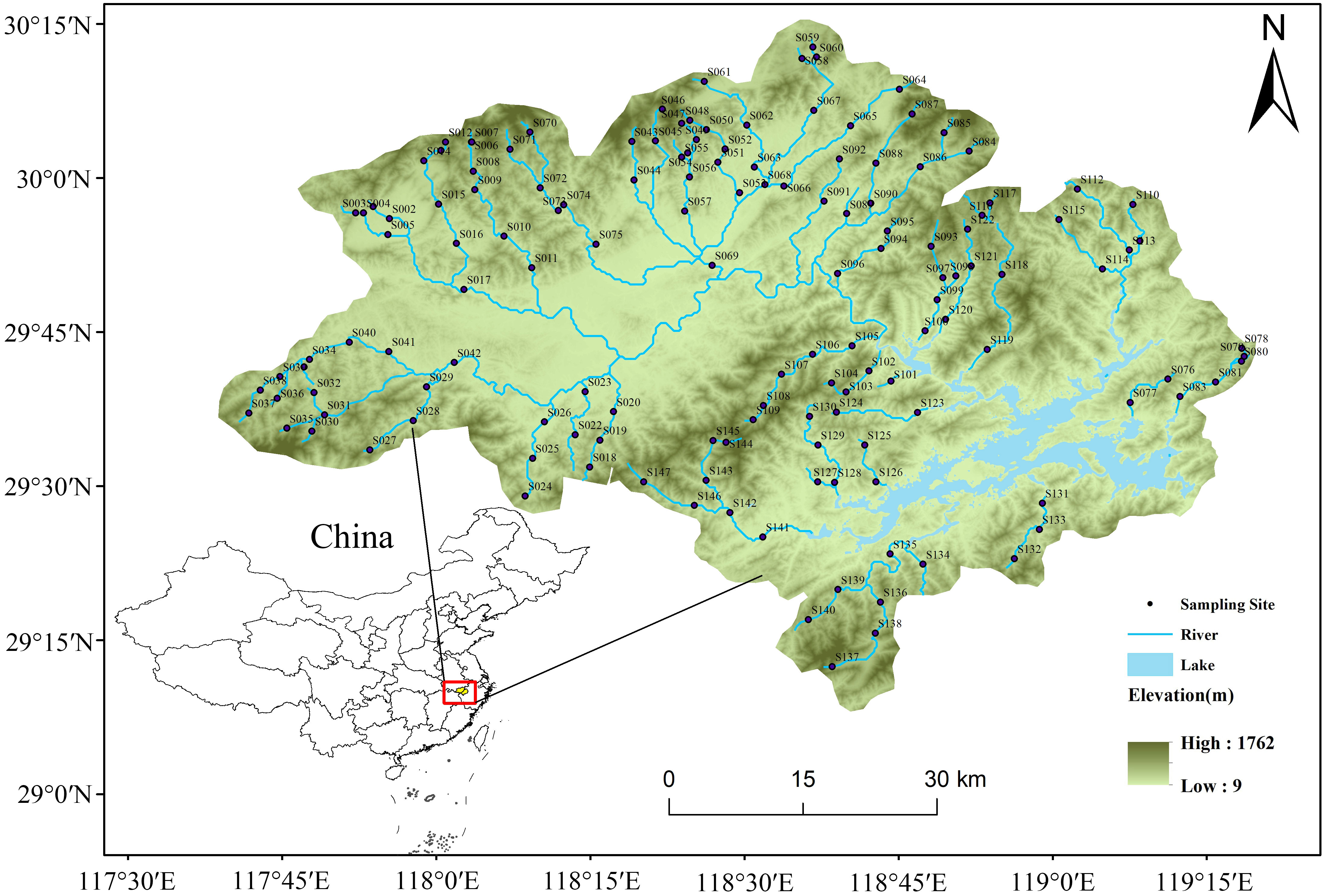
Figure 1 Distribution map of the 147 sampling sites in the Thousand Islands Lake (TIL) catchment, China.
2.2 Sampling and identification of macroinvertebrates
We investigated 147 sites in the TIL catchment in April and May 2021. Sampling sites cover as evenly as possible the wadable section of the river from the headwaters to large rivers. Meanwhile, sampling sites were selected to avoid stream sections directly below the confluence or with severe impacts from anthropogenic disturbances (e.g., wastewater outlets, bridges and villages).
At each sampling sites, macroinvertebrates were collected with a Surber net (30 × 30 cm2, 500 μm mesh) from habitats with different substrate compositions (e.g., cobble, rock, fine sediment) and flow conditions (e.g., water depth and current). The collection was repeated three times at each sampling site and the collected samples were stored in a portable holding tank and then sorted and then preserved in 70% alcohol. Macroinvertebrates were identified in the laboratory to the lowest possible taxonomic level (mainly to genus) using both books (Morse et al., 1994; Holzenthal, 2009; Merritt et al., 2017) and online identification resources (https://www.macroinvertebrates.org/).
2.3 Explanatory variables
2.3.1 Local environment (Local)
Local hydro-morphological parameters were measured in situ, including river width (Width), water depth (Depth), and flow velocity (Velocity, measured with Global Water flow meter). Habitat characteristics, including percentages of different hydrological conditions and substrates, were assessed following Qualitative Habitat Evaluation Index (QHEI), which is a multiscale qualitative habitat evaluation index published by the US EPA in 1989 as a measure of the physical integrity of streams (Taft and Koncelik, 2006). In addition, pH, conductivity, and water temperature were measured with a water quality handheld meter (YSI Professional Plus). Water samples were collected at each site and stored at −4°C before further analysis in the laboratory. The concentrations of total phosphorus (TP), total nitrogen (TN), chemical oxygen demand (CODMn), ammonia nitrogen (NH3-N), nitrate nitrogen (NO3-N), soluble reactive phosphorus (PO4-P) and different metals (e.g., Cu, Cr, and Mn) were measured in the laboratory according to the Water and Waste Water Monitoring and Analysis Method (The State Environmental Protection Administration The Water and Wastewater Monitoring Analysis Method Editorial Board, 2002).
2.3.2 Geo-climatic (Geo)
We grouped climate (e.g., annual mean temperature and annual precipitation), land use and topographic data into a group as Geo-climatic variables. Land-use data were obtained from Yang and Huang (2021), which provided data on land cover in China with a spatial resolution of 30 m. Based on the characteristics of the study area, we regrouped the land-use types into six categories, namely forest, cropland, shrubs, grassland, water and impervious. Topographic variables, including elevation, slope, and aspect, were extracted from Amatulli et al. (2018). Slope indicates the steepness of the stream along a longitudinal gradient and aspect captures information on the north–south and east–west orientation of each sampling site (Amatulli et al., 2018). In total, 19 bioclimatic variables (Bio1 to Bio19) were obtained from the WorldClim 2 (Fick and Hijmans, 2017) database and consist mainly of data derived from monthly temperature and precipitation values.
2.3.3 Spatial
We used asymmetric eigenvector maps (AEMs) to represent our spatial factors, which was specifically designed to model directional patterns (Blanchet et al., 2008b). This spatial analysis generates spatial variables along directional flow and has proven to be an effective way of modelling dispersal processes in stream communities (Dong et al., 2016; Wan et al., 2018). AEMs with smaller eigenvalues indicate broad-scale spatial patterns whereas larger eigenvalues indicate fine-scale patterns. First, the grid2nb function with the R package spdep was used to calculate the neighbors list (Bivand, 2022). Next, A site-by-edge binary matrix was constructed based on coordinates of the sampling sites and the directional links among sites using the aem.build.binary function in R package adespatial (Blanchet et al., 2011). Finally, the aem function in the same package was used to create eigenvectors. Due to the large spatial extent of our study area, we finally obtained 134 AEMs (i.e., AEM1-AEM134).
2.3.4 Biotic interactions (Bio)
Biotic interactions in this study were represented primarily by macroinvertebrates and diatom interactions and included mainly chlorophyll a and the percentage content of diatom traits at each sampling site. Many macroinvertebrates feed on organic matter and diatom (Schmera et al., 2017). Recent study showed that diatom are a high quality food for stream macroinvertebrates and influence the growth and reproduction of macroinvertebrates (Guo et al., 2016).The influence of benthic diatom on scrapers might be profound as they mainly feed on biofilm attached to the substrate (Heino, 2005). Diatom were classified into different groups according to cell size, guild and life form (Wang et al., 2022), see Appendix 1 for details.
2.4 Data analysis
In our study, metacommunity deconstruction, selection of explanatory variables (Local, Geo and Spatial only, while Bio data were enforced into all models) and variation partitioning analysis were major processes (Figure 2).
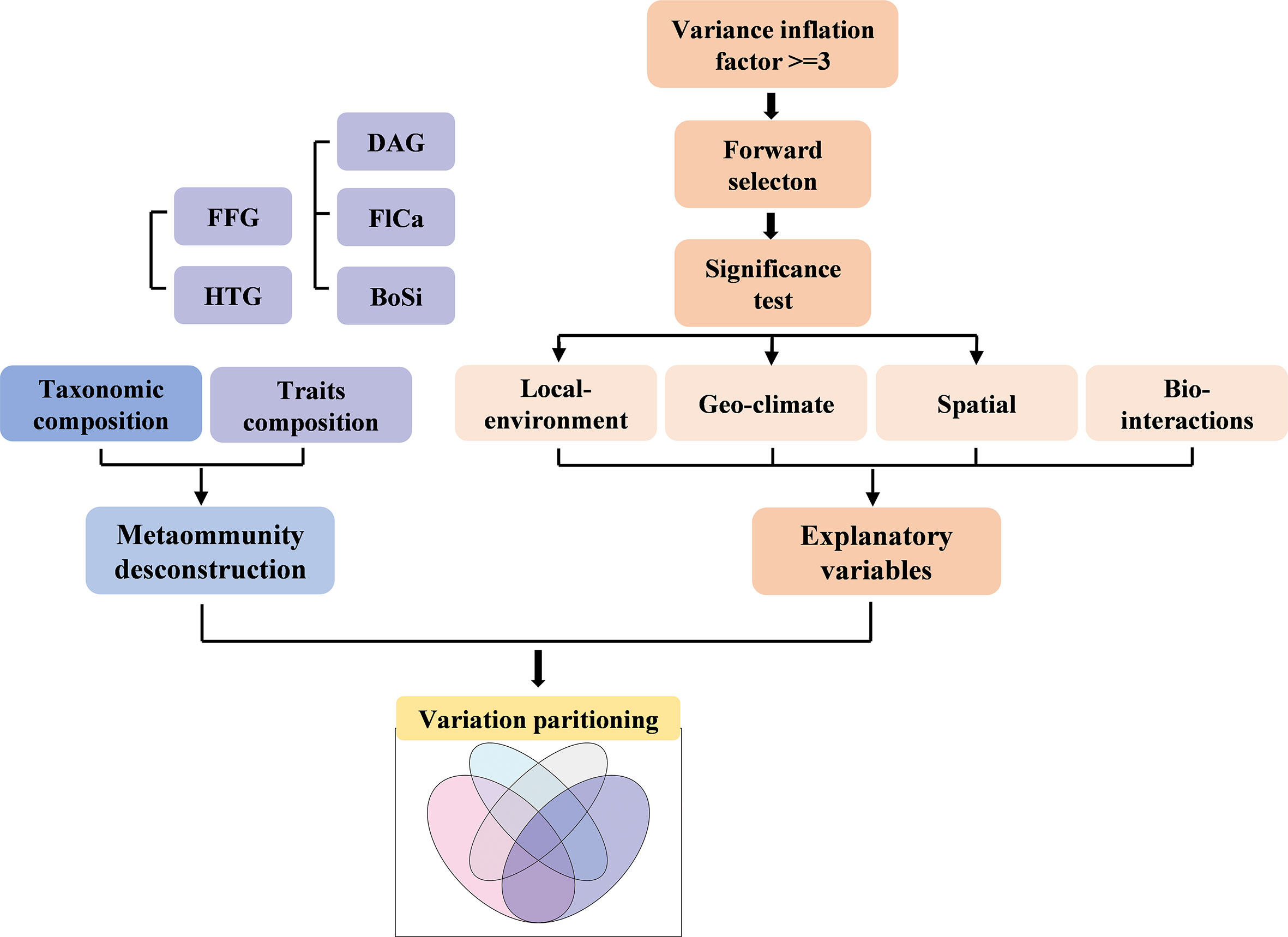
Figure 2 Diagram of the main analytical methods used in this study. FFG, functional feeding groups; HTG, Habit trait groups; DAG, Dispersal ability groups; FlCa, Flight capacity groups; BoSi, Body size groups.
In total, five trait groups were collected for each species (Table 1, Appendix 2). Two groups of traits (i.e., DAG and FFG) were deconstructed and included in the next step of the analysis.
All data processing was performed in R (Version 4.2.1, R Core Team, 2020). First, we prepared explanatory factors datasets including local environmental (Local), geo-climatic (Geo), spatial (Spatial) and biotic interactions (Bio) factors (Table 2, Appendix 3). In the next step, the abundance matrix was subjected to a Hellinger transformation. Prior to the analysis, we removed variables with significant multicollinearity (with variance inflation factor ≥ 3; vifstep function in the R package usdm) for all explanatory factors (Naimi et al., 2014). The forward.sel function in the R package adespatial was used for forward selection (Blanchet et al., 2008a), which contains two stopping criteria: significance level and adjusted coefficient of determination (adjusted R2). Subsequently, we obtained a final group of Local, Geo and Spatial factors and tested the significance of each component using the anova function in the R package vegan (Oksanen et al., 2019). Details of forward selection were described in Appendices 3 and 4. Because we wanted to know the effects of biotic interactions on macroinvertebrates communities, biotic interactions factors were forced into all of the variation partitioning models without the above procedures. Finally, the varpart function in the R package vegan was used in the variance partitioning analysis to quantify the pure and shared effects of the four types of explanatory factors on the communities of macroinvertebrates.
3 Results
3.1 Explanatory variables and macroinvertebrate metacommunity
Abiotic and biotic variables showed a large variation across the sampling sites (Table 2). For example, conductivity (Cond) ranged from 14.23 to 363.00 µs/cm (mean: 167.07 µs/cm) while the concentrations of nitrate nitrogen (NO3-N) ranged from 0.21 to 3.40 mg/L (mean: 1.02 mg/L).
In total, 199 taxa were observed, belonging to 4 phyla, 9 classes, 22 orders and 89 families. Aquatic insects accounted for 75% of the total richness, with Ephemeroptera (36 taxa) and Diptera (31 taxa) being the most abundant taxa. In terms of traits composition, the dominant functional feeding group and habit trait group were predator (64 taxa) and clinger (88 taxa).
3.2 Main drivers of taxonomic and traits metacommunity
The results of forward selection procedures were in Appendix 4 and 5. Results of the variance partitioning showed that local environmental, geo-climatic, spatial and biotic interactions all had considerable impacts on taxonomic and trait compositions of macroinvertebrates (Figure 3), supporting our first hypothesis (H1). The total explained variation by the four explanatory factors for taxonomic composition and trait compositions was 15% and 28%, respectively. The pure effect of environmental filtering (i.e., local environmental and geo-climatic) was smaller than that of spatial processes for both taxonomic and trait compositions, which rejected part of our second hypothesis (H2). In addition, biotic interactions contributed more to the explained variation in taxonomic composition than local environmental and geo-climatic variables.
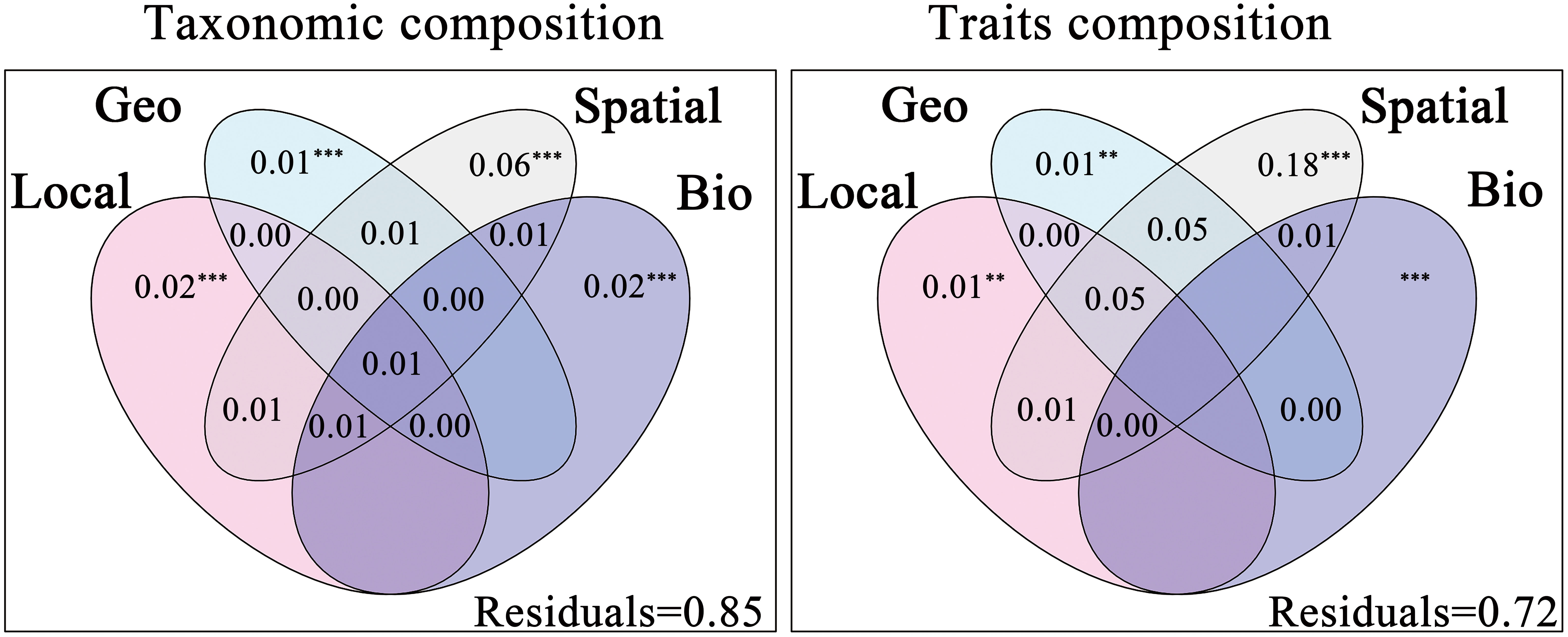
Figure 3 Results of variation partitioning based Venn diagrams, showing taxonomic composition and trait compositions explained by pure and shared effects of local environment (Local), geo-climate (Geo), spatial (Spatial) and biotic interactions (Bio). Local, Geo and Spatial variables were passed through forward selection procedure, while Bio variables were forced into all models. All FIGUREs represented the percentage of total variance derived for each fraction based on adjusted R2 and negative values were not shown. Significance was indicated as *** p < 0.001, ** p < 0.01.
3.3 Relative importance of environmental filtering and spatial processes to different traits metacommunity
For the dispersal ability groups, the total explained variance ranged from 9% to 61%. The highest pure effect of environmental filtering (local environmental and geo-climatic) was for DAG04 (2% and 1%), followed by DAG03 (1% and 1%), and the lowest was for DAG02 (only geo-climatic 1%) and DAG01 (only geo-climatic 1%), which supported our third hypothesis (H3a). Meanwhile, the highest pure effect explained by spatial was for DAG04 (52%), followed by DAG01 (15%) and DAG02 (11%) and the lowest was for DAG03 (4%) (Figure 4).
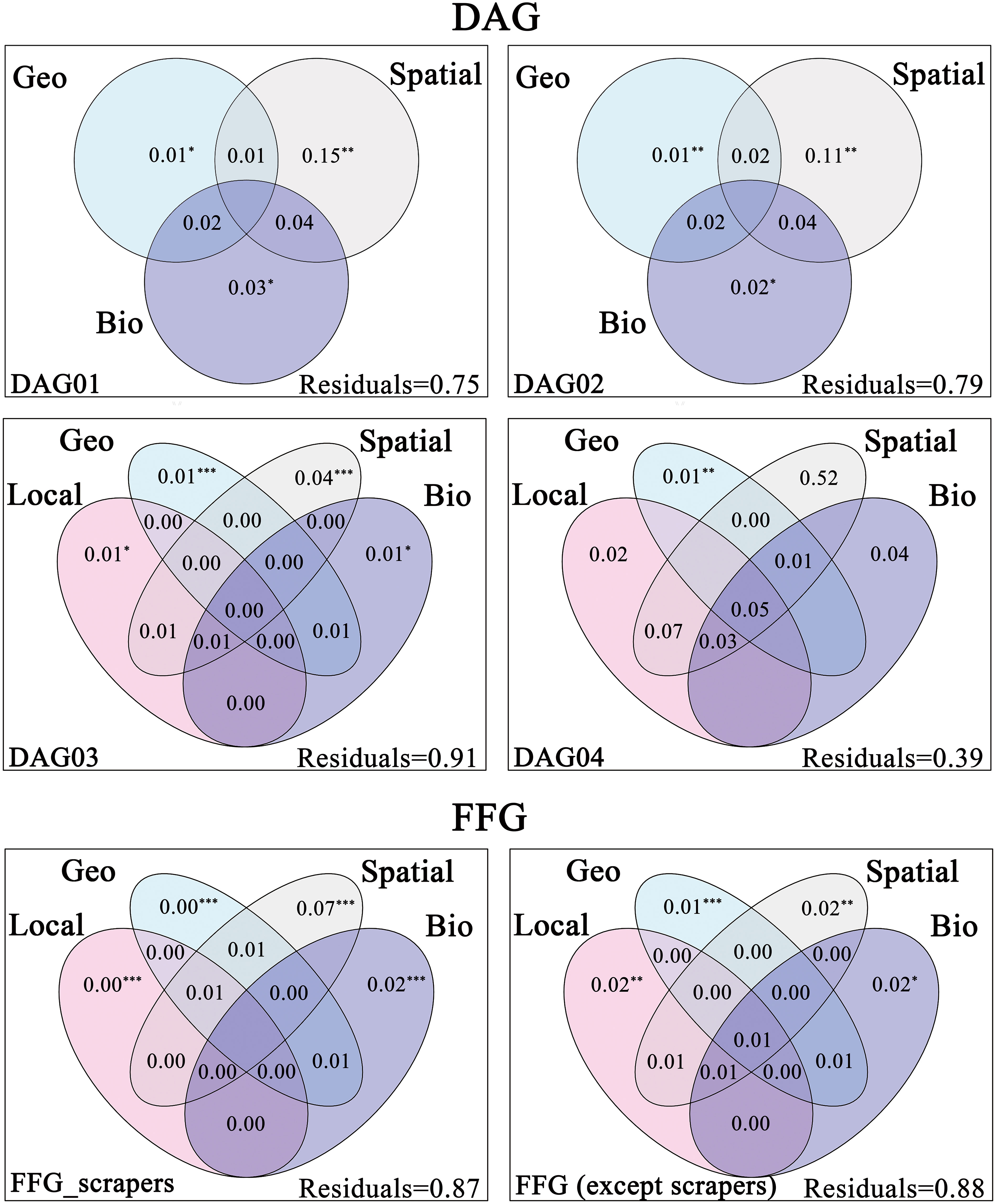
Figure 4 Results of variation partitioning based Venn diagrams, showing different traits metacommunity (i.e., DAG and FFG) explained by unique and joint effects of local environment (Local), geo-climate (Geo), spatial (Spatial) and biotic interactions (Bio). Local, Geo and Spatial variables were passed through forward selection procedure, while Bio variables were forced into all models. All FIGUREs represented the percentage of total variance derived for each fraction based on adjusted R2 and negative values were not shown. Significance was indicated as *** p < 0.001, ** p < 0.01, * p < 0.05.
For the functional feeding groups, the pure effect explained by biotic interactions was 2% for scrapers and non-scrapers, which did not prove our third hypothesis (H3b). In addition, the pure effect of spatial on scrapers (7%) was much higher than other functional feeding groups (2%) (Figure 4).
4 Discussion
4.1 Main drivers of macroinvertebrate metacommunity
Our results showed that local (i.e., environmental filtering and biotic interactions) and regional (i.e., dispersal limitation) processes jointly shaped the metacommunity structure of stream macroinvertebrates in the Thousand Islands Lake Catchment, which supports our first hypothesis (H1). This stands in line with observations in previous studies that biodiversity patterns in stream ecosystems are influenced by a multitude of ecological processes at local and regional scales (Poff, 1997; Heino et al., 2007; Chiu et al., 2020). We have investigated the potential role of biotic interactions in community assembly of stream macroinvertebrates, which has received limited attention in previous research (Barbee, 2005; Schneck et al., 2013). Despite of this, the proportion of explained variation in our study remained quite low (15–28%). Although low explained variation was also observed in previous studies that investigated the factors shaping metacommunity of stream macroinvertebrates (Cai et al., 2017; Perez Rocha et al., 2018; Branco et al., 2020), such phenomena indicates that some underlying drivers of macroinvertebrates metacommunity structure may have been overlooked. Indeed, many environmental variables (e.g., detailed information on hydromorphology of sampling sites), environmental regimes (e.g., temporal regimes of flow and nutrient concentrations), spatial processes (e.g., different dispersal pathways between sampling sites) and stochastic events (e.g., random local extinctions), albeit challenging to measure, have not been included in the analyses but might have substantial influence on metacommunity structure of stream organisms (Heino et al., 2015; Wu et al., 2022b; Wu et al., 2023).
The effects of environmental filterings on taxonomic and trait compositions were largely consistent, while the spatial factors had a greater effect on both taxonomic and trait compositions than the environmental variables, which partially rejects our second hypothesis (H2). The unexpected large influence of spatial processes is consistent with the findings of recent studies (Mugnai et al., 2022; Wu et al., 2022a). We speculate that this may be related to the following reasons. First, previous studies had generally concluded that spatial processes had a limited impact on small organisms, with environmental filtering playing a dominant role (Leibold, 1998; Cottenie et al., 2003). However, over the last two decades an increasing number of studies demonstrated that spatial processes also have a significant impact on small organisms (Liu et al., 2021), and this was similarly the case in streams (Wan et al., 2015; Wu et al., 2022a). Second, the heterogeneous mountain landscape provided a variety of unique ecological niche opportunities and microhabitats for macrobenthos, as well as relatively large dispersal barriers for species with poor dispersal abilities (Li et al., 2021). As a result, these factors might contribute to the strong influence of spatial factors on taxonomic and trait compositions. Surprisingly, although biotic interactions did not affect trait composition, they explained a non-negligible percentage (2%) of the taxonomic composition. Macroinvertebrates play an important role in stream ecosystems, some of the macroinvertebrates diet also includes coarse or fine particles and zooplankton, and macroinvertebrates may also be food for some fish (Guo et al., 2016; Schmera et al., 2017). Therefore, if future studies consider quantifying biotic interactions across multiple trophic levels, the explanatory rate of this process may be further improved.
4.2 Metacommunity and traits of stream macroinvertebrates
These three ecological processes (i.e., environmental filtering, spatial processes and biotic interactions) have varying effects on different macroinvertebrate traits groups (supporting H2), as found in our findings and other related studies (Souffreau et al., 2015; Wan et al., 2015; Liu et al., 2016). Trait-based deconstruction approach can improve our understanding of the mechanisms of community assembly in different traits groups. This demonstrates the importance of using traits in bioassessment. For example, certain species that are little affected by spatial processes (mainly mass effects) and are closely linked to their environment may be excellent candidates for biological evaluation (Leboucher et al., 2020; Bried and Vilmi, 2022). However, only part of the priori expectations has been verified (H3).
We expected that the importance of environmental filtering was positively associated with the dispersal ability of macroinvertebrates. For taxa with strong dispersal ability, it is assumed that they could overcome the barriers within the catchment and find suitable habitats. Therefore, dissimilarity of their compositions could be used to track environmental gradients within the catchment (Heino, 2013). The predicted importance of environmental filtering for different groups of dispersal ability was broadly in line with our expectations. At the same time, the effect of the spatial processes gradually decreased from DAG01 to DAG03, which was in line with our expectations. However, the effect of the spatial factors on DAG04 was surprisingly high. This might be due to the strong mass effects on DAG04 (i.e., strong aerial dispersers) in this particular basin.
Mass effects allow species to persist by dispersing to unfavorable environments (Mouquet and Loreau, 2003). Species in DAG04 have a strong dispersal capacity, which may lead to such species being subject to mass effects or even severing their links with their environment (Heino et al., 2017; Corte et al., 2018; Wu et al., 2022a).
For FFG, we hypothesized that the effect of biotic interactions on the scraper would be stronger than on the other groups. However, we did not detect an obvious difference between the two groups. The use of diatom traits alone to indicate complex biotic interactions associated with macroinvertebrates is likely insufficient. Moreover, we only considered diatom traits while scrapers also feed on other components of periphyton (i.e., fungi, microbes, bacteria, plant detritus, and animals) on substrates (Stelzer and Lamberti, 2002; Heino, 2005). A more comprehensive representation of the effect of biotic interactions on scrapers would be possible if the above factors were considered in the future studies. We also observed that the spatial processes had a greater effect on the scrapers than other functional feeding groups. In the Thousand Islands Lake Catchment, many scrapers are aquatic obligate species (e.g., Gastropoda) and have poor dispersal ability. Dispersal limitation is likely to play an important role in shaping their spatial distributions (Firmiano et al., 2021).
5 Conclusion
In summary, our study contributes to metacommunity research in stream ecosystems, which provide a promising framework for biodiversity conservation and management in stream ecosystems (Cid et al., 2022). Our results highlighted the influence of biotic interactions, which is often overlooked in previous research, on community assembly of stream macroinvertebrates. Although the observed effect of biotic interactions is small, it is likely due to insufficient representation of trophic interactions associated with macroinvertebrates. Future studies need to comprehensively investigate the roles of biotic interactions across multiple trophic levels in shaping community structures in stream ecosystems. We found that environmental filtering, spatial processes and biotic interactions jointly shaped community assembly of stream macroinvertebrates, and their relative importance to groups with different traits varied. Hence, trait-based approaches with spatial processes and connectivity of stream ecosystems taken into consideration, can strengthen our understanding of community structuring in stream ecosystems and facilitate the development of effective conservation and management strategies.
Data availability statement
The original contributions presented in the study are included in the article/Supplementary Material. Further inquiries can be directed to the corresponding author.
Author contributions
NW and GL conceived the ideas. GL, NW, and ZL performed the data analyses. GL, NW, and FH led the writing. GL and WJ identified the macroinvertebrates. WJ, YXW, YCW, and XQ performed the sampling collection and processing. All authors contributed to the article and approved the submitted version.
Funding
This study was funded by the National Natural Science Foundation of China (No. 52279068).
Acknowledgments
We thank colleagues of Anhui Normal University for their field supports.
Conflict of interest
The authors declare that the research was conducted in the absence of any commercial or financial relationships that could be construed as a potential conflict of interest.
Publisher’s note
All claims expressed in this article are solely those of the authors and do not necessarily represent those of their affiliated organizations, or those of the publisher, the editors and the reviewers. Any product that may be evaluated in this article, or claim that may be made by its manufacturer, is not guaranteed or endorsed by the publisher.
Supplementary material
The Supplementary Material for this article can be found online at: https://www.frontiersin.org/articles/10.3389/fevo.2023.1196296/full#supplementary-material
References
Amatulli G., Domisch S., Tuanmu M.-N., Parmentier B., Ranipeta A., Malczyk J., et al. (2018). A suite of global, cross-scale topographic variables for environmental and biodiversity modeling. Sci. Data 5 (1), 180040. doi: 10.1038/sdata.2018.40
Armitage P. D., Moss D., Wright J. F., Furse M. T. (1983). The performance of a new biological water quality score system based on macroinvertebrates over a wide range of unpolluted running-water sites. Water Res. 17 (3), 333–347. doi: 10.1016/0043-1354(83)90188-4
Barbee N. C. (2005). Grazing insects reduce algal biomass in a neotropical stream. Hydrobiologia 532, 153–165. doi: 10.1007/s10750-004-9527-z
Bilton D. T., Freeland J. R., Okamura B. (2001). Dispersal in freshwater invertebrates. Annu. Rev. Ecol. System. 32, 159–181. doi: 10.1146/annurev.ecolsys.32.081501.114016
Bivand R. (2022). R packages for analyzing spatial data: a comparative case study with areal data. Geogr. Anal. 54 (3), 488–518. doi: 10.1111/gean.12319
Blanchet F. G., Legendre P., Borcard D. (2008a). Forward selection of explanatory variables. Ecology 89 (9), 2623–2632. doi: 10.1890/07-0986.1
Blanchet F. G., Legendre P., Borcard D. (2008b). Modelling directional spatial processes in ecological data. Ecol. Model. 215 (4), 325–336. doi: 10.1016/j.ecolmodel.2008.04.001
Blanchet F. G., Legendre P., Maranger R., Monti D., Pepin P. (2011). Modelling the effect of directional spatial ecological processes at different scales. Oecologia 166 (2), 357–368. doi: 10.1007/s00442-010-1867-y
Branco C. C. Z., Bispo P. C., Peres C. K., Tonetto A. F., Krupek R. A., Barfield M., et al. (2020). Partitioning multiple facets of beta diversity in a tropical stream macroalgal metacommunity. J. Biogeogr. 47 (8), 1765–1780. doi: 10.1111/jbi.13879
Bried J. T., Vilmi A. (2022). Improved detection of mass effect species assembly for applied metacommunity thinking. J. Appl. Ecol. 59 (4), 921–926. doi: 10.1111/1365-2664.14115
Brown B. L., Swan C. M., Auerbach D. A., Grant E. H. C., Hitt N. P., Maloney K. O., et al. (2011). Metacommunity theory as a multispecies, multiscale framework for studying the influence of river network structure on riverine communities and ecosystems. J. North Am. Benthol. Soc. 30 (1), 310–327. doi: 10.1899/10-129.1
Cadotte M. W. (2007). Competition-colonization trade-offs and disturbance effects at multiple scales. Ecology 88 (4), 823–829. doi: 10.1890/06-1117
Cai Y., Xu H., Vilmi A., Tolonen K. T., Tang X., Qin B., et al. (2017). Relative roles of spatial processes, natural factors and anthropogenic stressors in structuring a lake macroinvertebrate metacommunity. Sci. Total Environ. 601, 1702–1711. doi: 10.1016/j.scitotenv.2017.05.264
Chase J. M. (2003). Community assembly: when should history matter? Oecologia 136 (4), 489–498. doi: 10.1007/s00442-003-1311-7
Chiu M.-C., Ao S., He F., Resh V. H., Cai Q. (2020). Elevation shapes biodiversity patterns through metacommunity-structuring processes. Sci. Total Environ. 743, 140548. doi: 10.1016/j.scitotenv.2020.140548
Cid N., Eros T., Heino J., Singer G., Jahnig S. C., Canedo-Arguelles M., et al. (2022). From meta-system theory to the sustainable management of rivers in the anthropocene. Front. Ecol. Environ. 20 (1), 49–57. doi: 10.1002/fee.2417
Corte G. N., Goncalves-Souza T., Checon H. H., Siegle E., Coleman R. A., Amaral A. C. Z. (2018). When time affects space: dispersal ability and extreme weather events determine metacommunity organization in marine sediments. Mar. Environ. Res. 136, 139–152. doi: 10.1016/j.marenvres.2018.02.009
Cottenie K., Michels E., Nuytten N., De Meester L. (2003). Zooplankton metacommunity structure: regional vs. local processes in highly interconnected ponds. Ecology 84 (4), 991–1000. doi: 10.1890/0012-9658(2003)084[0991:Zmsrvl]2.0.Co;2
Covich A. P., Palmer M. A., Crowl T. A. (1999). The role of benthic invertebrate species in freshwater ecosystems: zoobenthic species influence energy flows and nutrient cycling. BioScience 49 (2), 119–127. doi: 10.2307/1313537
Cummins K. W., Merritt R. W., Andrade P. C. N. (2005). The use of invertebrate functional groups to characterize ecosystem attributes in selected streams and rivers in south Brazil. Stud. Neotropic. Fauna Environ. 40 (1), 69–89. doi: 10.1080/01650520400025720
Declerck S. A. J., Coronel J. S., Legendre P., Brendonck L. (2011). Scale dependency of processes structuring metacommunities of cladocerans in temporary pools of high-Andes wetlands. Ecography 34 (2), 296–305. doi: 10.1111/j.1600-0587.2010.06462.x
Doledec S., Statzner B. (2010). Responses of freshwater biota to human disturbances: contribution of J-NABS to developments in ecological integrity assessments. J. North Am. Benthol. Soc. 29 (1), 286–311. doi: 10.1899/08-090.1
Dong X., Li B., He F., Gu Y., Sun M., Zhang H., et al. (2016). Flow directionality, mountain barriers and functional traits determine diatom metacommunity structuring of high mountain streams. Sci. Rep. 6, 24711. doi: 10.1038/srep24711
Fick S. E., Hijmans R. J. (2017). WorldClim 2: new 1-km spatial resolution climate surfaces for global land areas. Int. J. Climatol. 37 (12), 4302–4315. doi: 10.1002/joc.5086
Firmiano K. R., Canedo-Arguelles M., Gutierrez-Canovas C., Macedo D. R., Linares M. S., Bonada N., et al. (2021). Land use and local environment affect macroinvertebrate metacommunity organization in neotropical stream networks. J. Biogeogr. 48 (3), 479–491. doi: 10.1111/jbi.14020
Garcia-Giron J., Heino J., Garcia-Criado F., Fernandez-Alaez C., Alahuhta J. (2020). Biotic interactions hold the key to understanding metacommunity organisation. Ecography 43 (8), 1180–1190. doi: 10.1111/ecog.05032
Gaston K. J. (2000). Global patterns in biodiversity. Nature 405 (6783), 220–227. doi: 10.1038/35012228
Gianuca A. T., Declerck S. A. J., Cadotte M. W., Souffreau C., De Bie T., De Meester L. (2017). Integrating trait and phylogenetic distances to assess scale-dependent community assembly processes. Ecography 40 (6), 742–752. doi: 10.1111/ecog.02263
Guo F., Kainz M. J., Sheldon F., Bunn S. E. (2016). The importance of high-quality algal food sources in stream food webs – current status and future perspectives. Freshw. Biol. 61 (6), 815–831. doi: 10.1111/fwb.12755
Guzman L. M., Germain R. M., Forbes C., Straus S., O’Connor M. I., Gravel D., et al. (2019). Towards a multi-trophic extension of metacommunity ecology. Ecol. Lett. 22 (1), 19–33. doi: 10.1111/ele.13162
Heino J. (2005). Functional biodiversity of macroinvertebrate assemblages along major ecological gradients of boreal headwater streams. Freshw. Biol. 50 (9), 1578–1587. doi: 10.1111/j.1365-2427.2005.01418.x
Heino J. (2013). Does dispersal ability affect the relative importance of environmental control and spatial structuring of littoral macroinvertebrate communities? Oecologia 171 (4), 971–980. doi: 10.1007/s00442-012-2451-4
Heino J., Alahuhta J., Ala-Hulkko T., Antikainen H., Bini L. M., Bonada N., et al. (2017). Integrating dispersal proxies in ecological and environmental research in the freshwater realm. Environ. Rev. 25 (3), 334–349. doi: 10.1139/er-2016-0110
Heino J., Melo A. S., Siqueira T., Soininen J., Valanko S., Bini L. M. (2015). Metacommunity organisation, spatial extent and dispersal in aquatic systems: patterns, processes and prospects. Freshw. Biol. 60 (5), 845–869. doi: 10.1111/fwb.12533
Heino J., Mykra H., Kotanen J., Muotka T. (2007). Ecological filters and variability in stream macroinvertebrate communities: do taxonomic and functional structure follow the same path? Ecography 30 (2), 217–230. doi: 10.1111/j.2007.0906-7590.04894.x
Heino J., Tolonen K. T. (2017). Ecological drivers of multiple facets of beta diversity in a lentic macroinvertebrate metacommunity. Limnol. Oceanogr. 62 (6), 2431–2444. doi: 10.1002/lno.10577
Holzenthal R. W. (2009). Trichoptera (Caddisflies), in Encyclopedia of Inland Waters. (Oxford: Academic Press) 456–467.
Hsieh T.-T., Chiu M.-C., Resh V. H., Kuo M.-H. (2022). Biological traits can mediate species-specific, quasi-extinction risks of macroinvertebrates in streams experiencing frequent extreme floods. Sci. Total Environ. 806, 150313. doi: 10.1016/j.scitotenv.2021.150313
Hubbell S. P., Borda-De-Agua L. (2004). The unified neutral theory of biodiversity and biogeography: reply. Ecology 85 (11), 3175–3178. doi: 10.1890/04-0808
Jiang X., Pan B., Jiang W., Hou Y., Yang H., Zhu P., et al. (2021a). The role of environmental conditions, climatic factors and spatial processes in driving multiple facets of stream macroinvertebrate beta diversity in a climatically heterogeneous mountain region. Ecol. Indic. 124, 107407. doi: 10.1016/j.ecolind.2021.107407
Jiang X., Xu X., Tao M., Li Z., Zhang S., Li H. (2021b). The effects of dispersal ability on metacommunity structure of macroinvertebrates in subtropical Chinese high-mountain streams: seasonal shifts in relative contribution of local environment and spatial processes. Environ. Sci. pollut. Res. 28 (29), 38573–38583. doi: 10.1007/s11356-021-13281-2
Kurthen A. L., He F., Dong X., Maasri A., Wu N., Cai Q., et al. (2020). Metacommunity structures of macroinvertebrates and diatoms in high mountain streams, Yunnan, China. Front. Ecol. Evol. 8 (10). doi: 10.3389/fevo.2020.571887
Leboucher T., Tison-Rosebery J., Budnick W., Jamoneau A., Vyverman W., Soininen J., et al. (2020). A metacommunity approach for detecting species influenced by mass effect. J. Appl. Ecol. 57 (10), 2031–2040. doi: 10.1111/1365-2664.13701
Leibold M. A. (1998). Similarity and local co-existence of species in regional biotas. Evolution. Ecol. 12 (1), 95–110. doi: 10.1023/A:1006511124428
Leibold M. A., Chase J. M., Ernest S. K. M. (2017). Community assembly and the functioning of ecosystems: how metacommunity processes alter ecosystems attributes. Ecology 98 (4), 909–919. doi: 10.1002/ecy.1697
Leibold M. A., Holyoak M., Mouquet N., Amarasekare P., Chase J. M., Hoopes M. F., et al. (2004). The metacommunity concept: a framework for multi-scale community ecology. Ecol. Lett. 7 (7), 601–613. doi: 10.1111/j.1461-0248.2004.00608.x
Li F., Cai Q., Jiang W., Qu X. (2012). The response of benthic macroinvertebrate communities to climate change: evidence from subtropical mountain streams in central China. Int. Rev. Hydrobiol. 97 (3), 200–214. doi: 10.1002/iroh.201111489
Li Z., Heino J., Liu Z., Meng X., Chen X., Ge Y., et al. (2021). The drivers of multiple dimensions of stream macroinvertebrate beta diversity across a large montane landscape. Limnol. Oceanogr. 66 (1), 226–236. doi: 10.1002/lno.11599
Li Z., Liu Z., Heino J., Jiang X., Wang J., Tang T., et al. (2020). Discriminating the effects of local stressors from climatic factors and dispersal processes on multiple biodiversity dimensions of macroinvertebrate communities across subtropical drainage basins. Sci. Total Environ. 711, 134750. doi: 10.1016/j.scitotenv.2019.134750
Liu S., Xie G., Wang L., Cottenie K., Liu D., Wang B. (2016). Different roles of environmental variables and spatial factors in structuring stream benthic diatom and macroinvertebrate in Yangtze river delta, China. Ecol. Indic. 61, 602–611. doi: 10.1016/j.ecolind.2015.10.011
Liu Z., Zhou T., Cui Y., Li Z., Wang W., Chen Y., et al. (2021). Environmental filtering and spatial processes equally contributed to macroinvertebrate metacommunity dynamics in the highly urbanized river networks in Shenzhen, south China. Ecol. Processes 10 (1), 23. doi: 10.1186/s13717-021-00297-2
Logue J. B., Mouquet N., Peter H., Hillebrand H., Metacommunity Working G. (2011). Empirical approaches to metacommunities: a review and comparison with theory. Trends Ecol. Evol. 26 (9), 482–491. doi: 10.1016/j.tree.2011.04.009
Merritt R. W., Cummins K. W., Berg M. B. (2017). Chapter 20 – Trophic Relationships of macroinvertebrates, in Methods in Stream Ecology, Volume 1. (Boston, MA, USA: Academic Press) 413–433.
Merritt R., Cummins K., Berg M. B., Adler P. H., Allen M., Batzer D. P., et al. (2010). An introduction to the aquatic insects of North America. Rev. Mexicana Biodiversidad 81 (2), 593–595. doi: 10.2307/1467288
Meynard C. N., Devictor V., Mouillot D., Thuiller W., Jiguet F., Mouquet N. (2011). Beyond taxonomic diversity patterns: how do α, β and γ components of bird functional and phylogenetic diversity respond to environmental gradients across France? Global Ecol. Biogeogr. 20 (6), 893–903. doi: 10.1111/j.1466-8238.2010.00647.x
Morse J. C., Lianfang Y., Lixin T. (1994). Aquatic insects of China useful for monitoring water quality (Nanjing, People’s Republic of China: Hohai University Press).
Mouquet N., Loreau M. (2003). Community patterns in source-sink metacommunities. Am. Nat. 162 (5), 544–557. doi: 10.1086/378857
Mouquet N., Munguia P., Kneitel J. M., Miller T. E. (2003). Community assembly time and the relationship between local and regional species richness. Oikos 103 (3), 618–626. doi: 10.1034/j.1600-0706.2003.12772.x
Mugnai M., Trindade D. P. F., Thierry M., Kaushik K., Hrček J., Götzenberger L. (2022). Environment and space drive the community assembly of Atlantic European grasslands: insights from multiple facets. J. Biogeogr. 49 (4), 699–711. doi: 10.1111/jbi.14331
Mykra H., Heino J., Muotka T. (2007). Scale-related patterns in the spatial and environmental components of stream macroinvertebrate assemblage variation. Global Ecol. Biogeogr. 16 (2), 149–159. doi: 10.1111/j.1466-8238.2006.00272.x
Naimi B., Hamm N. A. S., Groen T. A., Skidmore A. K., Toxopeus A. G. (2014). Where is positional uncertainty a problem for species distribution modelling? Ecography 37 (2), 191–203. doi: 10.1111/j.1600-0587.2013.00205.x
Oksanen J., Blanchet F. G., Friendly M., Kindt R., Wagner H. H. (2019). Vegan community ecology package version 2.5-6.
Perez Rocha M., Bini L. M., Domisch S., Tolonen K. T., Jyrkänkallio-Mikkola J., Soininen J., et al. (2018). Local environment and space drive multiple facets of stream macroinvertebrate beta diversity. J. Biogeogr. 45 (12), 2744–2754. doi: 10.1111/jbi.13457
Poff N. L. (1997). Landscape filters and species traits: towards mechanistic understanding and prediction in stream ecology. J. North Am. Benthol. Soc. 16 (2), 391–409. doi: 10.2307/1468026
Poff N. L., Olden J. D., Vieira N. K. M., Finn D. S., Simmons M. P., Kondratieff B. C. (2006). Functional trait niches of North American lotic insects: traits-based ecological applications in light of phylogenetic relationships. J. North Am. Benthol. Soc. 25 (4), 730–755. doi: 10.1899/0887-3593(2006)025[0730:Ftnona]2.0.Co;2
Ramirez A., Gutierrez-Fonseca P. E. (2014). Functional feeding groups of aquatic insect families in Latin America: a critical analysis and review of existing literature. Rev. Biol. Trop. 62, 155–167. doi: 10.15517/rbt.v62i0.15785
R Core Team (2020). R: a language and environment for statistical computing (Vienna, Austria: R Foundation for Statistical Computing).
Rideout N. K., Compson Z. G., Monk W. A., Bruce M. R., Hajibabaei M., Porter T. M., et al. (2022). Environmental filtering of macroinvertebrate traits influences ecosystem functioning in a large river floodplain. Funct. Ecol. 36 (11), 2791–2805. doi: 10.1111/1365-2435.14168
Saito V. S., Soininen J., Fonseca-Gessner A. A., Siqueira T. (2015). Dispersal traits drive the phylogenetic distance decay of similarity in neotropical stream metacommunities. J. Biogeogr. 42 (11), 2101–2111. doi: 10.1111/jbi.12577
Sarremejane R., Cid N., Stubbington R., Datry T., Alp M., Canedo-Arguelles M., et al. (2020). DISPERSE, a trait database to assess the dispersal potential of European aquatic macroinvertebrates. Sci. Data 7 (1), 386. doi: 10.1038/s41597-020-00732-7
Schmera D., Heino J., Podani J., Eros T., Doledec S. (2017). Functional diversity: a review of methodology and current knowledge in freshwater macroinvertebrate research. Hydrobiologia 787 (1), 27–44. doi: 10.1007/s10750-016-2974-5
Schneck F., Schwarzbold A., Melo A. S. (2013). Substrate roughness, fish grazers, and mesohabitat type interact to determine algal biomass and sediment accrual in a high-altitude subtropical stream. Hydrobiologia 711 (1), 165–173. doi: 10.1007/s10750-013-1477-x
Souffreau C., van der Gucht K., van Gremberghe I., Kosten S., Lacerot G., Lobao L. M., et al. (2015). Environmental rather than spatial factors structure bacterioplankton communities in shallow lakes along a > 6000km latitudinal gradient in South America. Environ. Microbiol. 17 (7), 2336–2351. doi: 10.1111/1462-2920.12692
Statzner B. (2012). Geomorphological implications of engineering bed sediments by lotic animals. Geomorphology 157-158, 49–65. doi: 10.1016/j.geomorph.2011.03.022
Stelzer R. S., Lamberti G. A. (2002). Ecological stoichiometry in running waters: periphyton chemical composition and snail growth. Ecology 83 (4), 1039–1051. doi: 10.1890/0012-9658(2002)083[1039:ESIRWP]2.0.CO;2
Taft B., Koncelik J. P. (2006). Methods for assessing habitat in flowing waters: using the qualitative habitat evaluation index (QHEI).
Tapolczai K., Bouchez A., Stenger-Kovacs C., Padisak J., Rimet F. (2016). Trait-based ecological classifications for benthic algae: review and perspectives. Hydrobiologia 776 (1), 1–17. doi: 10.1007/s10750-016-2736-4
The State Environmental Protection Administration The Water and Wastewater Monitoring Analysis Method Editorial Board (2002). Water and wastewater monitoring analysis method (Beijing: China Environmental Science Press), 38–47.
Thrush S., Dayton P., Cattaneo-Vietti R., Chiantore M., Cummings V., Andrew N., et al. (2006). Broad-scale factors influencing the biodiversity of coastal benthic communities of the Ross Sea. Deep-Sea Res. Part Ii-Topical Stud. Oceanogr. 53 (8-10), 959–971. doi: 10.1016/j.dsr2.2006.02.006
Tockner K., Lorang M. S., Stanford J. A. (2010). River flood plains are model ecosystems to test general hydrogeomorphic and ecological concepts. River Res. Appl. 26 (1), 76–86. doi: 10.1002/rra.1328
Van De Meutter F., De Meester L., Stoks R. (2007). Metacommunity structure of pond macro invertebrates: effects of dispersal mode and generation time. Ecology 88 (7), 1687–1695. doi: 10.1890/06-0333.1
Vilmi A., Tolonen K. T., Karjalainen S. M., Heino J. (2017). Metacommunity structuring in a highly-connected aquatic system: effects of dispersal, abiotic environment and grazing pressure on microalgal guilds. Hydrobiologia 790 (1), 125–140. doi: 10.1007/s10750-016-3024-z
Wan Y., Xu L., Hu J., Xu C., Wan A., An S., et al. (2015). The role of environmental and spatial processes in structuring stream macroinvertebrates communities in a Large river basin. Clean-Soil Air Water 43 (12), 1633–1639. doi: 10.1002/clen.201300861
Wan Y., Yang J. Q., Zou D. W., Li J. J., Qiao Y. J., An S. Q., et al. (2018). Effects of multiple dams on the metacommunity structure of stream macroinvertebrates. Mar. Freshw. Res. 69 (5), 721–729. doi: 10.1071/mf17159
Wang Y., Wu N., Liu G., Mu H., Gao C., Wang Y., et al. (2023). Incorporating functional metrics into the development of a diatom-based index of biotic integrity (D-IBI) in Thousand Islands Lake (TIL) catchment, China. Ecol. Indic. 153, 110405. doi: 10.1016/j.ecolind.2023.110405
Wang Y., Wu N., Tang T., Wang Y., Cai Q. (2022). Small run-of-river hydropower dams and associated water regulation filter benthic diatom traits and affect functional diversity. Sci. Total Environ. 813, 152566. doi: 10.1016/j.scitotenv.2021.152566
Winegardner A. K., Jones B. K., Ng I. S. Y., Siqueira T., Cottenie K. (2012). The terminology of metacommunity ecology. Trends Ecol. Evol. 27 (5), 253–254. doi: 10.1016/j.tree.2012.01.007
Wu N., Guo K., Zou Y., He F., Riis T. (2023). SER: an r package to characterize environmental regimes. Ecol. Evol. 13 (3), e9882. doi: 10.1002/ece3.9882
Wu N., Liu G., Zhang M., Wang Y., Peng W., Qu X. (2022a). Spatial factors outperform local environmental and geo-climatic variables in structuring multiple facets of stream macroinvertebrates’ β-diversity. Animals 12 (19), 2648. doi: 10.3390/ani12192648
Wu N., Wang Y., Wang Y., Sun X., Faber C., Fohrer N. (2022b). Environment regimes play an important role in structuring trait- and taxonomy-based temporal beta diversity of riverine diatoms. J. Ecol. 110 (6), 1442–1454. doi: 10.1111/1365-2745.13859
Wu N., Zhou S., Zhang M., Peng W., Guo K., Qu X., et al. (2021). Spatial and local environmental factors outweigh geo-climatic gradients in structuring taxonomically and trait-based β-diversity of benthic algae. J. Biogeogr. 48 (8), 1842–1857. doi: 10.1111/jbi.14108
Yang J., Huang X. (2021). The 30 m annual land cover dataset and its dynamics in China from 1990 to 2019. Earth System Sci. Data 13 (8), 3907–3925. doi: 10.5194/essd-13-3907-2021
Zhou S., Wu N., Zhang M., Peng W., He F., Guo K., et al. (2020). Local environmental, geo-climatic and spatial factors interact to drive community distributions and diversity patterns of stream benthic algae, macroinvertebrates and fishes in a large basin, northeast China. Ecol. Indic. 117, 106673. doi: 10.1016/j.ecolind.2020.106673
Keywords: biotic interactions, diversity, environmental filtering, metacommunity, spatial processes, trait
Citation: Liu G, Lin Z, Qi X, Wang Y, Wang Y, Jiang W, He F and Wu N (2023) Environmental filtering, spatial processes and biotic interactions jointly shape different traits communities of stream macroinvertebrates. Front. Ecol. Evol. 11:1196296. doi: 10.3389/fevo.2023.1196296
Received: 29 March 2023; Accepted: 06 June 2023;
Published: 19 June 2023.
Edited by:
Ming-Chih Chiu, Chinese Academy of Sciences (CAS), ChinaReviewed by:
Tan Lu, Chinese Academy of Sciences (CAS), ChinaBin Li, Shandong Normal University, China
Copyright © 2023 Liu, Lin, Qi, Wang, Wang, Jiang, He and Wu. This is an open-access article distributed under the terms of the Creative Commons Attribution License (CC BY). The use, distribution or reproduction in other forums is permitted, provided the original author(s) and the copyright owner(s) are credited and that the original publication in this journal is cited, in accordance with accepted academic practice. No use, distribution or reproduction is permitted which does not comply with these terms.
*Correspondence: Naicheng Wu, bmFpY2hlbmd3dTg4QGdtYWlsLmNvbQ==