- Faculty of Science, Department of Mathematics, Freudenthal Institute for Science and Mathematics Education, Utrecht University, Utrecht, Netherlands
Systems thinking has become synonymous to developing coherent understanding of complex biological processes and phenomena from the molecular level to the level of ecosystems. The importance of systems and systems models in science education has been widely recognized, as illustrated by its definition as crosscutting concept by the Next Generation Science Standards (NGSS Lead States, 2013). However, there still seems no consensus on what systems thinking exactly implies or how it can be fostered by adequate learning and teaching strategies. This paper stresses the theoretical or abstract nature of systems thinking. Systems thinking is not just perceived here as “coherent understanding,” but as a learning strategy in which systems theoretical concepts are deliberately used to explain and predict natural phenomena. As such, we argue that systems thinking is not to be defined as a set of skills, that can be learned “one by one,” but instead asks for consideration of systems characteristics and the systems theories they are derived from. After a short elaboration of the conceptual nature of systems thinking, we portray the diversity of educational approaches to foster systems thinking that have been reported in the empirical literature. Our frame of analysis focuses on the extent to which attention has been given to the matching of natural phenomena to one of three systems theories, the integration of different systems thinking skills and the role of modeling. Subsequently, we discuss the epistemological nature of the systems concept and we present some conclusions on embedding systems thinking in the secondary biology curriculum.
Introduction
Modern science has made so many advances that the quantity of “basic” science to be taught in the classroom tends to increase every year. Therefore, current rapid developments in (life) sciences necessitate selecting a core set of essential and interconnected concepts and skills to serve as a basis for making sense of observable phenomena and for lifelong learning. This is underlined by among others the National Research Council (2011), the NGSS Lead States (2013), and the Dutch Board for the Innovation of Biology Education (Boersma et al., 2010).
A core characteristic of biological sciences is that they deal with multiple levels of organization, e.g., molecule, cell, organ, organism, population, on which phenomena and processes occur and can be explained. To understand complex biological processes, different levels of organization should be distinguished and the relations within and between these levels should be elaborated (Knippels, 2002; Verhoeff, 2003). The way of thinking to explain, understand and interpret complex and dynamic (biological) systems is called “systems thinking” (Evagorou et al., 2009). According to NGSS Lead States (2013, p.79) systems thinking can be seen as a crosscutting concept, namely “systems and system models,” that “helps students deepen their understanding of the disciplinary core ideas, and that helps students to develop a coherent and scientifically based view of the world.”
Systems thinking is an important skill in different research fields, e.g., sociology, psychology, technology, meteorology, earth sciences, and biology. In this paper we will focus on systems thinking in biology (education). Several studies have reported on fostering students' systems thinking related to different biological topics, such as the human body as a system (Ben-Zvi Assaraf and Orion, 2010; Tripto et al., 2016, 2017), ecosystems (Jordan et al., 2014), and the cell (Verhoeff, 2003; Verhoeff et al., 2008). The diversity of these studies underlines the relevance and applicability of systems thinking in biology education at different educational levels. However, there is no consensus about which systems thinking skills students should achieve. According to Boersma et al. (2011)—who compared several empirical studies on systems thinking in science education—the differences can be attributed to whether the underlying systems theories are ignored or referred to implicitly or explicitly. A remarkable finding in their study was that four out of five analyzed studies did not include the identification of the system to be learned and the distinction of its (system) boundaries. In our view, this is questionable, as thinking back and forth between the system as a whole and its components is a key aspect of acquiring a coherent understanding of biological phenomena. Another notable difference between studies on systems thinking is that they differ in the extent to which they include modeling as an explicit part of systems thinking. In contrast to the other studies in Boersma's analysis, Verhoeff et al. (2008) for example, includes thinking backward and forward between general systems models and concrete biological objects and processes as an explicit element of systems thinking. The importance of modeling is also underlined by NGSS Lead States (2013, p.80), who state that the crosscutting concept “systems and system models” is clearly related to the crosscutting practice “development and use of models.”
In this paper we emphasize the theoretical nature of the systems concept. Systems thinking is not just perceived here as “coherent understanding,” but as a cognitive skill in which systems theoretical concepts are deliberately used to explain and predict natural phenomena. As such, we argue that systems thinking is not to be defined as a subset of skills that can be learned “one by one.” Systems thinking asks for consideration of the systems characteristics of the three systems theories—such as the systems boundary, the vertical coherence between systems at different organizational levels or dynamicity—which has been referred to as “high-order systems thinking” (Levy and Wilensky, 2008; Eilam and Reisfeld, 2017).
There are two reasons for writing this paper. First, the available empirical studies are very diverse in their perspectives on systems thinking, which makes it difficult to find some general trends in promoting systems thinking in biology education. Second, a closer analysis of empirical studies shows that learning outcomes are sometimes disappointing, while other studies present promising results regarding students systems thinking abilities (Ben-Zvi Assaraf and Orion, 2005; Sommer, 2005; Evagorou et al., 2009). In an attempt to explain these different results, Boersma et al. (2011) questioned whether what was measured in all these studies should be considered as systems thinking. They claim that the different results of the empirical studies have to a large extent been caused by an imprecise definition of systems thinking and underestimation of its conceptual nature. The main aim of this paper is to provide a reasoned definition of systems thinking (perspectives), taking into account the different conceptual nature of the three systems theories. This will result in educational implications that may be useful for forthcoming empirical studies.
This paper addresses the following questions:
A. What is the conceptual nature of systems thinking?
B. What perspectives in systems thinking can be identified in empirical studies on systems thinking in biology education?
C. What perspective(s) in systems thinking can be recommended considering its conceptual nature, and what are the educational implications?
These questions are addressed by an analysis of empirical literature on systems thinking in biology education. First, we shortly elaborate the conceptual nature of systems thinking by presenting the key concepts from three systems theories. In section Systems Thinking in Biology Education, we analyze some empirical articles on systems thinking by reflecting on their perspectives on systems thinking. To portray the diversity of perspectives we carried out a more in-depth analysis of a small number of selected studies that used different approaches to implement systems thinking in biology education. Here, we present studies from four different research groups, i.e., Ben-Zvi Assaraf and Orion (2005, 2010), Hmelo et al. (2000; Hmelo-Silver et al., 2009), Verhoeff (2003); Verhoeff et al. (2008), and Eilam and Reisfeld (2017). Subsequently, we discuss the epistemological nature of the systems concept, including the educational implications. Finally, we present conclusions on embedding systems thinking in the secondary biology curriculum.
The Conceptual Nature of Systems Thinking
We believe that the use of systems theory is conditional for successful application of systems thinking in a diversity of contexts. Consequently, developing students' systems thinking skill(s) should include the development of a systems concept. We are dealing with three systems theories, the GST, cybernetics and dynamic systems theory, each focusing on different aspects of biological systems. Each systems theory uses its own concepts (see Table 1), and the most basic of these concepts could be considered as cognitive tools in systems thinking.
Systems thinking has its roots in the organismic perspective of biologists at the beginning of the twentieth century. In order to grasp the differences between fixed and static machines and the dynamic processes of life, Bertalanffy launched his conception of the organism as “open system” in his General Systems Theory (GST). After the publication of the revised edition of von Bertalanffy (1968) his theory became a source for inspiration in many disciplines (Gray and Rizzo, 1973). Systems were conceived as hierarchical and “Janus-faced” (Koestler, 1973): The face turned toward the higher levels is that of the dependent and functional part; the face turned downward, toward its own constituents, is that of the whole of remarkable self-sufficiency in interaction with its environment.
In the 1970s the focus shifted to communication patterns in closed networks. Within this so-called Cybernetic perspective, living systems were conceived as (self)-regulating patterns of organization by means of non-linear causality. The major achievements of Cybernetics originated in comparisons between organisms and machines and led to the concepts of feedback and self-regulation (Capra and Luigi Luisi, 2014). For a short while in the 1970s, Cybernetics was rather popular in ecological research since it seemed to provide an empirically testable theory. However, since cybernetics models are basically deterministic, there was not much correspondence between the values predicted by the model and the measured changes in open systems like in systems consisting of two populations (Westra, 2008).
The dynamic systems theory or nonlinear systems theory has been linked to the development of powerful computers in the 1970s, as they opened the possibility to (mathematically) model the enormous complexity of life. Even the simplest form of life, a bacterial cell, is a highly complex network involving thousands of chemical processes. Now, nonlinear mathematics could be used to describe self-organization as a dynamic process, marking “a shift of perspective from objects to relationships, from measuring to mapping and from quantity to quality” (Capra and Luigi Luisi, 2014). In current biological research, and other disciplines like meteorology and environmental science, modeling of processes in dynamic systems is frequently applied as a third way of research, besides empirical and theoretical research. The added value of systems modeling is the possibility to calculate the changes in open systems such as ecosystems and the biosphere. Systems modeling is grounded in dynamic systems theory and requires advanced mathematical methods.
The three described systems theories present a conceptual framework to understand biological phenomena. Systems thinking should not just be perceived as “coherent understanding,” but the descriptions above illustrate that theoretical concepts (see Table 1) are deliberately used to explain and predict natural phenomena. As such, we argue that systems thinking asks for consideration of systems characteristics and the systems theories they are derived from, such as the systems boundary or the vertical coherence between systems at different organizational levels that cannot in themselves be perceived by the senses. This raises the question to what extent attention has been given to matching natural phenomena to the three systems theories in empirical studies that promote systems thinking in biology education.
Systems Thinking in Biology Education
The increasing number of studies in systems thinking in primary and secondary biology education from a diversity of countries indicate that systems thinking is increasingly accepted as an educational objective. In the Netherlands, for example, it is included as a domain-specific skill in the examination programs for biology (Boersma et al., 2010). Consequently, the studies categorized in section Categorization of the Diversity of Studies in Systems Thinking also consider systems thinking as a learning objective. Before we present some typical examples of empirical studies on systems thinking, we put them in a slightly broader context.
Empirical Studies on Systems Thinking
A group of Israeli researchers (Ben-Zvi Assaraf and Orion, 2005, 2010; Tripto et al., 2017) report on multiple empirical studies that follow students' systems thinking skills over time. The authors envision systems thinking as holistic understanding of these systems (they focused on the hydro-water cycle system and the human body system) as complex and functioning wholes. They offer a list of systems thinking skills from an elementary level, i.e., the ability to identify the components of a system and processes within that system; the ability to identify relationships among the system's components up to higher order systems thinking skills such as the ability to understand the cyclic nature of systems and thinking temporally. Students' concept maps are used to illustrate and externalize students' mental models of the systems involved to assess to what degree, and what level, systems thinking is achieved. The authors conclude that although students made meaningful progress in their systems thinking skills, the systems were still perceived as unrelated pieces of knowledges (Tripto et al., 2017). For example, students did not use cellular level processes to explain phenomena on the level of the human body and most of the students did not make connections between the different body systems. The researchers stress the advantage of explicit scaffolding of systems thinking as a metacognitive strategy and explicit usage of “systems language” including terms like “interactions, patterns and dynamism, homeostasis, and hierarchy” (Tripto et al., 2016).
A group of American researchers (Hmelo et al., 2000; Liu and Hmelo-Silver, 2009; Hmelo-Silver et al., 2017) focused on helping Sixth grade children to acquire a deeper systemic understanding of the human respiratory system, including structural, behavioral, and functional relations. They used a function-centered conceptual representation emphasizing the function and behavior of the human respiratory system. The authors conclude that, although the students tended to map the respiratory system across different organizational levels, examining one complex system is not enough for improving learners' global mental models reflecting the dynamics of (all) biological systems (Liu and Hmelo-Silver, 2009). Recently, a refined conceptual representation was presented by Hmelo-Silver et al. (2017) reflecting the mechanistic reasoning of ecosystem learning, termed Components, Mechanisms, and Phenomenon (CMP). The representation was used as a framework to help students organize their ideas before they engaged in model development. The combination of a conceptual representation with the explicit practice of modeling allowed learners to externalize their thinking and collaboratively discuss their ideas. The results suggest that the approach helps students to deepen their understanding of systems and to extend their ecosystem learning beyond a particular context. This is due to the fact that the students are reasoning about system elements in a generic way. This process of abstraction allows students to relearn system ideas in novel contexts, because students can use the CMP frame as cognitive support.
A group of Dutch researchers (Verhoeff, 2003; Verhoeff et al., 2008, 2013; Westra, 2008) describe a similar process of abstraction. They present two modeling approaches to facilitate students' conceptual understanding of the topic at hand and understanding the way different representations are instrumental in acquiring this understanding (metacognition). In the first approach (Verhoeff et al., 2008), upper-secondary students were actively engaged in constructing and revising cellular models and comparing familiar representations of biological phenomena to more abstract system models, based on the GST. In a subsequent approach, students were involved in a sequence of computer modeling activities to clarify the dynamics of ecosystem behavior at the level of the organism, population and ecosystem (Westra, 2008). Based on the empirical results of the study, Westra concludes that coherent understanding of complex biological systems needed more explicit matching of system characteristics, i.e., systems boundary, vertical coherence, to empirical phenomena. They conclude that coherent understanding of complex systems requires explicit attention for navigation between the levels of organization and also for the stepwise change from concrete models to models of higher abstraction.
Recently, Eilam and Reisfeld (2017) designed a simulation-based curriculum in which 16 students (14–15 years old) navigated the levels of organization both downward and upward by means of two contrasting simulations. A System Dynamics-based simulation offered a macro view on the population as a whole entity and an Agents-based simulation provided a perspective on the population's single agents' behavior. The authors focused on cognitive aspects involved in the manipulation of each of the two simulation types, explicitly contrasting their simulation output. In line with Verhoeff et al. (2008) the authors conclude that shifting between complementary multiple representations of the macro- and micro-level scaffolds students' complex systems thinking, i.e., understanding the visualization of the macro-level as emerging from the micro-level interactions occurring among the system components. In addition, the dynamic and quantitative nature of the simulations and the graphs they produced, improved students' stochastic thinking and their ability to perceive biological phenomena as a series of complex events that occur simultaneously over time.
We started by the assumption that although systems thinking is an accepted educational objective in biology education, no single definition of systems thinking becomes apparent, nor consensus how it can be fostered by adequate learning and teaching strategies. It is remarkable that many studies (Verhoeff et al., 2008; Hmelo-Silver et al., 2017; Tripto et al., 2017) highlight the development of systems language, while (except for Verhoeff et al., 2008) no explicit reference is made to one of the systems theories from which the vocabulary has been derived from. Apart from the conceptual nature of systems thinking, there is a remarkable difference to the extent that modeling is conceived as a central tool and/or aspect of systems thinking. In the next section, we categorize and compare the core ideas of four typical educational approaches to systems thinking representing the empirical literature sketched above.
Categorization of the Diversity of Studies in Systems Thinking
Many lists of systems thinking skills have been presented in the educational literature (Booth Sweeney and Sterman, 2000). Here, we aim to provide an overview of different perspectives that have been reported in empirical studies on systems thinking. Our overview includes a more in-depth analysis of four of these studies, as they represent rather particular portrayals of systems thinking.
As a first step in categorizing perspectives on systems thinking we focused on the source and nature of the learning objectives that have to be attained by learners. Learning objectives could either be defined as a set of cognitive skills derived from systems theories as outlined in section The Conceptual Nature of Systems Thinking or as behavioral actions with no direct link to a systems theory:
1. Behavioral perspective. Systems thinking is defined by a mixed set of learning objectives. Some objectives refer to one or more systems characteristics (such as components, or interaction between systems components), while others do not and only indicate a behavioral component (Ben-Zvi Assaraf and Orion, 2005, 2010). Systems characteristics do frequently not originate from systems theory, and some characteristics of systems that are recognized in systems theory may be missing.
2. Cognitive skills perspective. Systems thinking is defined by a set of learning objectives with one or more systems characteristics generally derived from systems theory (Verhoeff, 2003; Sommer, 2005; Verhoeff et al., 2008; Evagorou et al., 2009) A set of “cognitive skills” makes it clear that the focus is on application of the systems concept in exploring and analyzing complex biological systems, not just on developing conceptual understanding of the systems concept (e.g., Eilam and Reisfeld, 2017; Hmelo-Silver et al., 2017), i.e., higher order systems thinking.
A second distinction between perspectives on systems thinking is whether systems thinking is defined as a set of sub skills that can be acquired independently and have their own value in understanding biological phenomena, or that systems thinking should only be seen as meaningful when it constitutes a coherent system of skills and concepts that emphasize the system as a whole:
3. Component perspective. In the component perspective the skills or conceptual components defining systems thinking are considered as parts that can be acquired (and scored) independently, while these parts together do define systems thinking (Ben-Zvi Assaraf and Orion, 2005; Sommer, 2005). The focus is not on how the components can be integrated into a systems concept or an integrated systems thinking skill.
4. Holistic perspective. In the holistic perspective the system as a whole is emphasized, and complex systems learning is aimed at understanding biological phenomena as emerging from the dynamic interactions between components across different levels of organization. This requires students to recognize these phenomena as “systems” (Verhoeff et al., 2008; Boersma et al., 2011; Eilam and Reisfeld, 2017; Hmelo-Silver et al., 2017).
Modeling is central to the crosscutting concept of systems and systems models, so a third way of categorizing studies is based on the representation of biological phenomena; either as qualitative or as quantitative models:
5. Qualitative modeling perspective. In a qualitative modeling perspective a systems model is developed as an abstract representation of a system, showing some systems characteristics (Verhoeff et al., 2008, 2013). The model is used in thinking forward and backward between systems and biological objects.
6. Quantitative modeling perspective. A quantitative modeling perspective focuses on the prediction of quantitative changes in biological systems (Westra, 2008; Eilam and Reisfeld, 2017; Hmelo-Silver et al., 2017). Computer tools for quantitative modeling are grounded in dynamic systems theory, although that is not evident for the students because the mathematics underlying the calculations is not accessible.
The perspectives of four typical empirical studies on systems thinking are identified and discussed below.
Ben-Zvi Assaraf and Orion (2005)
The study presents the results of students' advances in systems learning in junior high school when working on a module about the water cycle. The authors define systems thinking as a set of system-thinking skills in the context of the water cycle system without referring explicitly to a systems theory. The systems boundary is not indicated, so that it seems that implicitly the water cycle of the whole earth is considered. A figure showing a diagrammatic representation of the water cycle includes all components, lacks a systems boundary and does not consider the possibility that some parts may only be found in specific areas.
Some of the system-thinking skills enclose a cognitive component of systems theory (systems, components, but considering relationships instead of dynamical interaction). However, a skill referring to the ability to recognize a set of components as a whole is not included, although most skills include the systems concept. General characteristics of systems are not identified. Consequently, the conceptual components in the study are the components of the water cycle, and not the components of general systems. The lack of a holistic perspective and clear system boundaries might have hindered students' integration of the different components into a systems concept and their understanding of different systems levels. Since only few data are presented about learning and teaching processes, it is unclear whether components of the water cycle were indicated with terms that are linked to a certain systems theory.
Categorization
Systems thinking is defined as a set of skills, although most skills include a cognitive component (1.Cognitive skills perspective). Furthermore, students are invited to construct a water cycle from its constituting parts (3. Component perspective). Modeling is not explicitly addressed as part of systems thinking.
Verhoeff et al. (2008)
The study aims to develop coherent cell biological knowledge, and an initial model for systems thinking in upper secondary education by means of an emergent modeling strategy. Systems thinking is considered as a metacognitive skill consisting of a limited set of partial skills. The study is grounded in the GST. The first part of the educational strategy, focuses on the development of coherent understanding of the cell by means of a sequence of two- and three-dimensional system models with increasing abstraction. In the second part of the strategy a computer program is used to show how a general systems model can be developed by further abstraction of models of cells, embedded in organs and an organism. The qualitative model that is developed represents several levels of biological organization, interaction between components, a systems boundary, and the open nature of all included systems (Verhoeff et al., 2013). Finally, students apply this general hierarchical systems model to the topic of breast-feeding. Although the testing procedure was restricted, the intermediate learning outcomes including the last assignment about breast-feeding were in accordance with was expected. Consequently, it was concluded that the strategy is feasible for students and teachers and may result in the development of coherent cell biological knowledge when integrated in systems thinking.
Categorization
Systems thinking is defined as a set of cognitive skills, derived from the GST (2. Cognitive skills perspective). The study focuses on the cell and the system as a whole (4. Holistic perspective) and uses a qualitative model (5. Qualitative modeling perspective).
Hmelo-Silver et al. (2017)
Hmelo-Silver et al. (2017) report a quasi-experimental study on an 8-week classroom intervention to support students' reasoning about ecosystems in the seventh grade. Students were engaged in modeling and simulations with a conceptual representation termed Components, Mechanisms, and Phenomenon (CMP). The CMP conceptual representation intended to “support learners in framing systems thinking around a particular phenomenon or ecological pattern (P); encouraging learners to generate or recall plausible mechanisms (M) that may result in the (P); and explore the parts or components (C) that interact to result in (M and P).” The CMP conceptual framework reflects the mechanistic reasoning of ecosystem learning and is used as an indicator of systems thinking, i.e., “dynamic and multi-leveled thinking.” Dynamic thinking is further specified as the identification of components in relation to mechanisms and behavior. The Mechanisms here refer to the underlying ecosystem processes (decomposition) and serve as a mediator between Phenomena (dying fish in a pond) and Components (fish, plants, bacteria). Multi-level thinking is specified as the identification of relationships between macro and micro structures or processes. During the intervention students were asked to explain a Phenomenon, e.g., dead and dying fish in a pond. Their inquiry was guided by CMP-based hypermedia, and simulations were used to provide opportunities for students to engage with evidence and mechanisms that underlie the phenomenon at different scales. The results of the study show that students who engaged in the intervention deepened their understanding of ecosystem dynamics compared to students who engaged in traditional instruction. The authors suggest that the use of the combination of a conceptual representation and modeling practices helps students to deepen their understanding of systems and to extend their ecosystem learning beyond a particular context. This is due to the fact that the students are reasoning about system elements in a more generic way. This process of abstraction allows students to relearn system ideas in novel contexts, because students can use the CMP frame as cognitive support.
Categorization
Systems thinking is defined as a combination of conceptual knowledge and dynamic and multi-leveled thinking (2. Cognitive skills perspective). The study aims to promote holistic understanding of system elements, i.e., underlying Components and Mechanisms that explain a complex Phenomenon at a macro level, in a more generic way (4. Holistic perspective). The model based approach includes qualitative CMP conceptual representations (5. Qualitative modeling perspective). By means of computer based simulations students can gather evidence and raw data to refine their models' plausibility and parsimony to support/refute their ideas. (6. Quantitative modeling perspective).
Eilam and Reisfeld (2017)
The authors present an innovative curriculum unit for promoting complex systems thinking in which students manipulate and contrast two simulations of population growth, i.e., a system dynamics model presenting the macro level and an agent-based model presenting the micro level. The System Dynamics model expresses the temporal cause-and-effect relationships between variables without directly representing the involved agents. It enables learners' to explore the macro level of population dynamics and present a graph representing quantitative interactions of variables within a system, stock and flow. The Agent Based-simulation simulates qualitatively the interaction between the different agents (individuals) of the system (population) and between the agents and their environment. This enables a better understanding of the variability and causality of the system at the micro level. By means of computerized experiments and analysis of self-constructed graphs, students examined the system's mechanisms and behaviors, while considering both macro and micro levels. The authors mention that prior to these experiments students raised research questions regarding the manipulation of the system behavior: “How would the system behave if the initial population size increased?” illustrating the conceptual nature of “systems thinking.” After using both simulation perspectives, students were asked to compare between the output of the System Dynamics based and Agent based simulations and explain the differences. The joint use of the two perspectives offered by the simulations contributed to increasing students' ability to relate between the micro and macro levels of a complex system, as well as stochastic thinking and dynamics involved in achieving equilibrium, i.e., students' higher order systems thinking. In addition, the curriculum increased conceptual knowledge of the systems' basic components, the relations between these components, and of the process of population growth (i.e., stock, flow, interactions, equilibrium, etc.). The authors do suggest to include more varied and complex systems in students' inquiry, to enable them in using the simulations as cognitive tools in learning about complex systems.
Categorization
Systems thinking is defined as a combination of conceptual knowledge and thinking modes (2. Cognitive skills perspective). The study aims to promote holistic understanding of the behavior of a complex system requiring comprehension of many system aspects, like the components, their interactions and equilibrium processes (4. Holistic perspective). The model/based approach includes both qualitative agent-based animations representing population growth (5. Qualitative modeling perspective) and quantitative modeling, based on the Lotka-Volterra prey-predator differential equations (6. Quantitative modeling perspective).
The analysis of the perspectives on systems thinking of the four selected studies is summarized in Table 2. The table shows that in the study of Ben-Zvi Assaraf and Orion (2005) two of the six perspectives were recognized, and respectively three, four and four of the perspectives in the studies of Verhoeff et al. (2008), Hmelo-Silver et al. (2017) and Eilam and Reisfeld (2017).
The Epistemological Nature of the Systems Concept
The previous section shows that, except for the study of Ben-Zvi Assaraf and Orion (2005), there is remarkable similarity between the studies on three aspects: systems thinking is presented as understanding complex systems as a whole (1); it is regarded as a set of “higher order cognitive skills” (2), that are learned by the application of (multiple) representations or models (3). In these studies, all models represent an underlying systems concept, i.e., the hierarchical systems concept in the study of Verhoeff et al. (2008), the Component Mechanisms Phenomenon concept of Hmelo-Silver et al. (2017), and the Dynamical Systems concept in the study of Eilam and Reisfeld (2017). Notably, only Verhoeff et al. explicitly refer to an underlying systems theory, i.e., GST.
We found no empirical studies that focused on the system as a theoretical concept. However, Boersma (2016) proposed to reconsider the epistemological nature of the systems concept to emphasize that it is derived from systems theory and not from the process of abstracting (multiple) empirical phenomena. To clarify why it is sensible to consider the systems concept as a theoretical concept, we can consider a system with an ill-defined systems boundary, like a marine ecosystem such as the North Sea. In such ecosystems a boundary is concluded from observable organisms and their interactions, although its exact spatial position may be discussed. Nevertheless, we consider the North Sea to be an ecosystem. Seeing an ecosystem as a whole, also when it is not visible, implies that an ecosystem actually is a mental construct that allows us to recognize entities and to predict a number of empirical phenomena. We may predict, for example, that an ecosystem maintains its identity, that feedback mechanisms occur between populations and that it is self-organizing over time. The mental construct that we may consider an ecosystem as a whole may find its origin in a theory like systems theory. Systems theory is a mental construct which describes the characteristics of theoretical objects and not of empirical objects. Actually, systems thinking implies framing of empirical phenomena from a systems perspective. In other words, systems theoretical knowledge shows a coherence between phenomena which are not accessible to direct observation. This implies a distinction between theoretical and empirical concepts [Hempel (1966/1973) (Koningsveld, 1987; Van Aalsvoort, 2000)]. Empirical concepts are then defined as abstractions of empirical phenomena, while theoretical concepts are derived from an axiomatic theory like systems theory, or particle theory. It should be noted that in this line of reasoning the term theory has a more restricted meaning than in daily practice and unfortunately also in many scientific studies, where it is frequently used as another term for pattern, regularity or what has been indicated as “law of nature.”
Distinguishing theoretical from empirical concepts inevitably evokes the question how to define the relation between both. Figure 1 presents two lines of relations, i.e., a developmental line (starting from the top left box) and the application line (starting from the top right box). The developmental line focuses on the development of theoretical concepts like the system boundary. Theoretical concepts are not developed empirically, but can be seen as parts of webs of belief that together provide a theoretical perspective. A historical example of the development of a scientific theory is the invention of the structure of benzene by Kekulé (1829–1896) who derived his theory about the cyclic structure of benzene from two dreams (Rocke, 2010). This illustrates 1) that formation of a theory requires extensive prior knowledge of the domain the theory refers to, and 2) scientific theories are not just developed by abstraction of empirical phenomena, but require a source from outside, such as dreams, fantasies, metaphors, or a seemingly unrelated domain of knowledge.
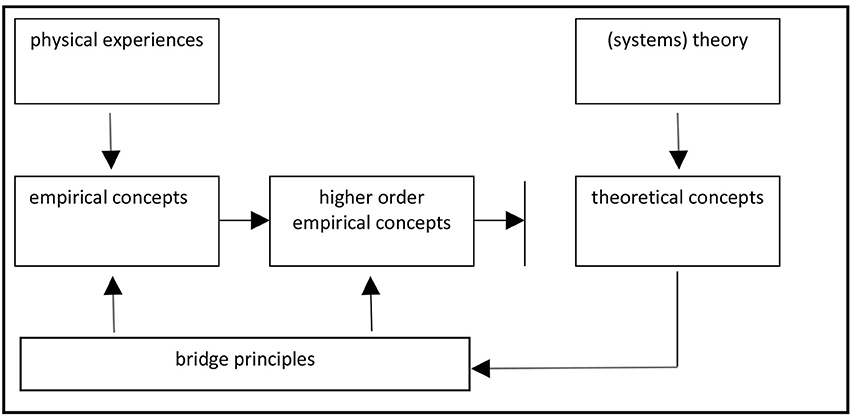
Figure 1. The relations between empirical concepts and theoretical concepts. The model illustrates a gap between theoretical conceptions and concrete events. This gap can only be crossed by means of bridge principles after Boersma (2016).
Application of a theory to explain empirical phenomena asks for a connection between concepts from two different languages, and this can be accomplished via bridging principles or rules of correspondence (Koningsveld, 1987; Van Aalsvoort, 2000). Bridging principles are meant to connect a theory and its observational basis. Without these principles a theory would be scientifically meaningless, since there is no way of testing it empirically. In the example of the North Sea it means that individual organisms or populations, concepts of our empirical language, are compared with systems components. The line of reasoning is then: “If populations are understood as components of an ecosystem…; followed by an empirically testable statement, such as:…; the size of a population is depending (to some extent) on the size of one or more other populations.”
A theory cannot provide explanations, since theory and empiricism use different languages that cannot be combined in chains of causal reasoning. A theory can suggest empirically testable predictions, and if affirmed the theory can contribute to causal chains in empiricism. Confirmation of predictions does not count as a proof of the theory, since there is no causal relation between the empirical and theoretical knowledge domains. Consequently, a scientific theory is not true or proven (or dismissed), but functional (or dysfunctional) as long as it generates empirically testable predictions. Of course empirical outcomes may differ from what was predicted, and if methodological mistakes are excluded, it may be concluded that the theory should be adapted or even rejected. The ultimate test is the design of experiments with the purpose to test or falsify the functionality of the scientific theory (Popper, 1963).
Educational Implications of the Theoretical Nature of Systems Thinking
Understanding complex living systems involves integration of the key theoretical concepts of the three systems theories summarized in section The Conceptual Nature of Systems Thinking. It refers to thinking about multiple interdependent levels, nonlinear causality and emergence (Jacobsen and Wilensky, 2006). Understanding such concepts is difficult as they cannot (simultaneously) be perceived by the senses and students often have difficulty in thinking beyond linear relationships, single causality and visible structures. A strategy to overcome these difficulties might be to start with one systems perspective, guided by conceptual representations or models (Verhoeff et al., 2013; Hmelo-Silver et al., 2017). Such representations can promote understanding of the systems interrelationships by highlighting key aspects of a certain system. For example, a model based on the GST highlights the hierarchal structure of living systems, while a Lotka-Volterra model highlights the dynamics of interspecific competition. Thinking back and forth between real biological phenomena and a model based on a specific systems theory might be a first step toward systems thinking. “Higher order systems thinking” could then be described at the metacognitive level, where students can navigate between models based on different systems theories and employ them to understand biological phenomena. Looking at our analysis in section The Conceptual Nature of Systems Thinking, it is striking that three studies feature systems modeling strategies that clearly have a theoretical basis Eilam and Reisfeld (2017), Hmelo-Silver et al. (2017), and Verhoeff et al. (2008). Each of these studies reports that additional attention should be given to the practice of modeling and how it fosters conceptual understanding of biological phenomena. Eilam and Reisfeld (2017, p. 57). for example stress that students may need “more time, higher number and diversity of manipulation experiences and examples (…) of varied complex systems.”
Our argument that systems concepts should be classified as theoretical concepts has several implications for determining an appropriate educational strategy. The most important implication is evidently that when students have to learn systems thinking, they have to learn to apply a systems theory (or model) as a coherent “holistic” “framework” in which the “key concepts” become “meaningful.” Consequently, our next question is what this reclassification of system concepts and models implies for the selection of systems perspectives and educational strategies that aim for higher order systems thinking.
Promising Perspectives on Systems Thinking
Considering the perspectives on systems thinking identified in section Categorization of the Diversity of Studies in Systems Thinking, the reclassification of the systems concept implies that the cognitive nature of systems thinking is emphasized and that a cognitive skill perspective is desirable. Furthermore, since key systems concepts are embedded in a specific systems theory, applying a holistic approach becomes unavoidable. Systems thinking should focus primarily on the development of the theoretical systems concept, and not just on understanding individual concepts or sub skills, such as identifying the components of a system. Thus, the crucial step in systems learning is the step from empirically observable phenomena to a systems theoretical conceptualization of such phenomena. The three studies in our analysis that feature qualitative modeling indicate that a qualitative modeling strategy in which complementary multiple representations are used may be helpful in the development of an initial systems concept. Verhoeff et al. (2008) have suggested to derive such a first systems concept from the GST as in biology systems are quite often identified as structural organizations, i.e., a “cell as a system” and a “population as a system.” Also Ben-Zvi Assaraf and Orion (2005), Eilam and Reisfeld (2017), and Hmelo-Silver et al. (2017) start with identification of the structural components involved in the phenomenon to be studied. Such “systems” are still quite abstract; it is not possible to make a photograph of a “cell as a system.” Consequently, the question arises how a “cell as a system” can be represented pictorially. The most appropriate representation of a system seems to be a hierarchical systems model, derived from the GST (Verhoeff et al., 2008, 2013). Therefore it is recommended to select a qualitative modeling approach, in the initial development of systems thinking. As the studies of Eilam and Reisfeld (2017) and Hmelo-Silver et al. (2017) illustrate, deeper understanding of the dynamic nature of systems could be furthered by a quantitative modeling approach. However, in our view, introducing students to the “theoretical” vocabulary of cybernetics and/or dynamical systems theory in which these quantitative models are embedded (Tripto et al., 2017) needs to be carefully guided by bridge principles.
The Distinction Between Empirical and Theoretical Concepts
In section The Epistemological Nature of the Systems Concept we concluded that theoretical and empirical concepts are connected by bridge principles. In other words, bridge principles propose a connection between two languages, i.e., an empirical language describing observations and a theoretical language using systems theoretical concepts. To avoid conceptual confusion it is necessary to distinguish these two languages and to allow students to grasp the benefits of explicit usage of “systems language” including terms like “interactions, patterns and dynamism, homeostasis, and hierarchy.” This raises the question how to distinguish the two languages in the biology classroom. In current biology textbooks, empirical and theoretical concepts seem to be hardly distinguished at all and the theoretical nature of some concepts is not recognized, so that almost only empirical language is used. Many textbooks, for example, use the concept “population,” but it is unclear whether it refers to an (observable) collection of organisms of the same species, living in a specific area, or to a population as a system. Although no empirical studies are available, it seems obvious that both biology teachers and authors of biology textbooks implicitly support the view that the systems concept is a further abstraction of empirical concepts. Consequently, systems models are considered implicitly as abstract representations of biological objects (Verhoeff et al., 2013; Hmelo-Silver et al., 2017) as well as representations of a specific systems theory. To overcome conceptual confusion, we propose to explicitly address the difference between theoretical models and empirical models as a bridge principle. Figure 2 indicates which models can be used to avoid confusion.
This is in line with suggestions by Hmelo-Silver et al. (2017), albeit that they do not stress the theoretical basis of systems thinking. They propose that explicit introduction of the language of their CMP-framework fosters students' reasoning about complex systems. They also underline the importance of activities that explicitly support systematic thinking about systems and models.
Conclusion and Discussion
In this paper we presented systems thinking as a cognitive skill in which systems theory is deliberately used to explain and predict natural phenomena that cannot per se be observed empirically. This means that in the development of students' systems thinking we should include bridging principles, which we have articulated as explicitly introducing students to the theoretical basis of systems models (and key concepts): If we approach the North Sea from a GST perspective, we can identify the systems borders and the systems components with their interactions within a framework of functional relationships.
Available empirical studies are very diverse in their perspectives on systems thinking. Nevertheless, a closer categorization of perspectives on systems thinking in four studies revealed some similar trends in promoting systems thinking in biology education: (1) Systems learning should focus on application of a systems concept in exploring and analyzing complex biological systems (cognitive skills perspective). (2) Emphasizing the need to focus on the conceptual core of systems theory also implies that attention should be given to the systems boundary. Students should learn to see a system as a whole (holistic perspective), and not only as a network of interacting components. (3) Using systems models may support students' conceptual development of the systems concept. Consequently, it was recommended to select the qualitative modeling perspective. Quantitative modeling is not recommended in developing an initial systems concept, because it requires substantial effort from both students and teachers and may result in less explicit attention to the development of the systems concept (i.e., bridge principle).
The conceptual core of systems thinking, was elaborated in two parts. First, we presented a set of key concepts from each of the three systems theories: the GST, Cybernetics and the Dynamical systems theory. We proposed to consider this set of systems concepts as the focus of students' conceptual development in systems thinking. And second, the epistemological nature of systems theory was considered. We argued that systems theories and their concepts are theoretical and cannot be developed or learned just by further abstraction of empirical phenomena. As a consequence, empirical and theoretical concepts should be explicitly distinguished in learning about systems.
In this paper we only discussed the initial step in the development of systems thinking. Since systems thinking is considered here as a metacognitive skill, students should also have the opportunity to practice it in a subsequent learning trajectory, as also strongly suggested by Eilam and Reisfeld (2017) and Hmelo-Silver et al. (2017). Since there are three different systems theories, each focusing on different characteristics, a trajectory aiming for development of a complete systems concept should include all three. However, a systems concept integrating all three systems theories might be too complex. A further complication of developing such a concept is that no model seems available that represents the characteristics of all three theories. Therefore, we recommend introducing the systems concept in three steps, coinciding with the three systems theories. An initial systems concept could be derived from the GST, since it considers biological systems as open systems. Following the course of history, Cybernetics and the Dynamical systems theory could follow. Another option may be to first focus on the development of some basic empirical components of systems that can be directly observed or link to students' prior empirical knowledge. For example, although students in secondary education will not have developed a theoretical concept of populations, they can recognize that the size of a group of organisms in predator–prey relationships will be influenced by groups of other species. That may result in the development of an initial concept of feedback at the empirical level (and not at the theoretical level). The question remains whether it is desirable to focus first on the development of a theoretical systems concept, or on the development of preparatory empirical concepts like community, population and feedback. Finally, to develop an understanding of all three systems theories that together form a complete systems concept, students should have the opportunity to practice each of them. They should explore all three theoretical system conceptualizations at different levels of biological organization, with varied complex phenomena and maybe also in other disciplines than biology, such as chemistry, developmental psychology, and environmental science (Eilam and Reisfeld, 2017)
A complete program of systems thinking in biology education, and as a crosscutting concept, in adjacent disciplines, cannot yet be realized with current understanding of how students can master “higher order” systems thinking. Suggestions from this paper and from the analyzed studies should be reconsidered, elaborated and tested in educational practice. Although it is not yet possible to sketch the outlines of one or more complete programs focusing on the full complexity of systems thinking, it is worthwhile to strive for, as systems thinking provides students with a useful cognitive toolbox for considering complexity in a variety of domains.
The development of such a program in systems thinking would require a lot of teaching time in biology education. At first glance, that may conflict with already overloaded current educational programs. At the moment, biology curricula have to comply with a number of conflicting demands, such as reducing curricular overload, teaching according to a developmental perspective, and elaborating coherence with other science subjects. It would seem that some choices will have to be made, and a restructuring of biology education according to systems thinking might well offer an attractive alternative.
Author Contributions
All authors listed have made a substantial, direct and intellectual contribution to the work, and approved it for publication.
Conflict of Interest Statement
The authors declare that the research was conducted in the absence of any commercial or financial relationships that could be construed as a potential conflict of interest.
References
Ben-Zvi Assaraf, O., and Orion, N. (2005). Development of system thinking skills in the context of earth system education. J. Res. Sci. Teach. 42, 518–560. doi: 10.1002/tea.20061
Ben-Zvi Assaraf, O., and Orion, N. (2010). Four case studies, six years later: developing system thinking skills in junior high school and sustaining them over time. J. Res. Sci. Teach. 47, 1253–1280. doi: 10.1002/tea.20383
Boersma, K. (2016). Bouwstenen Voor Begripsontwikkeling in Het Biologieonderwijs. [Building Blocks for Conceptual Development in Biology Education]. Utrecht: Nederlands Instituut voor Biologie.
Boersma, K. T., Kamp, M. J. A., Van den Oever, L., and Schalk, H. H. (2010). Naar Actueel, Relevant en Samenhangend Biologieonderwijs. Eindrapportage van de commissie Vernieuwing Biologie Onderwijs, met nieuwe examenprogramma's voor havo en vwo. [Towards actual, relevant and coherent biology education. Final report of the board for the innovation of biology education, with new examinations programs for general upper secondary and pre-university education]. Utrecht: CVBO.
Boersma, K. T., Waarlo, A. J., and Klaassen, K. (2011). Rethinking the introduction of systems thinking in biology education. J. Biol. Educ. 45, 190–197. doi: 10.1080/00219266.2011.627139
Booth Sweeney, L. B., and Sterman, J. D. (2000). Bathtub dynamics: initial results of a systems thinking inventory. Syst. Dyn. Rev. 16, 249–286. doi: 10.1002/sdr.198
Capra, F., and Luigi Luisi, P. (2014). The Systems View of Life: A Unifying Vision. Cambridge: Cambridge University Press.
Eilam, B., and Reisfeld, D. (2017). A curriculum unit for promoting complex system thinking: the case of combined system dynamics and agent based models for population growth. J. Adv. Educ. Res. 2, 39–60. doi: 10.22606/jaer.2017.22001
Evagorou, M., Korfiatis, K., Nicolaou, C., and Constantinou, C. (2009). An investigation of the potential of interactive simulations for developing system thinking skills in elementary school: a case study with fifth-graders and sixth-graders. Int. J. Sci. Educ. 31, 655–674. doi: 10.1080/09500690701749313
Gray, W., and Rizzo, N. (1973). Unity through Diversity. A Festschrift for Ludwig von Bertalanffy. New York; London; Paris: Gordon and Breach Science Publishers.
Hmelo, C. E., Holton, D. L., and Kolodner, J. L. (2000). Designing to learn about complex systems. J. Learn. Sci. 9, 247–298. doi: 10.1207/S15327809JLS0903_2
Hmelo-Silver, C. E., Jordan, R., Eberbach, C., and Sinha, S. (2017). Systems learning with a conceptual representation: a quasi-experimental study. Instruc. Sci. 45, 53–72. doi: 10.1007/s11251-016-9392-y
Hmelo-Silver, C. E., Lui, L., and Jordan, R. (2009). Visual representation of a multidimensional codingscheme for understanding technology-mediated learning about complex natural systems. Res. Pract. Technol. Enhan. Learn. Environ. 4, 253–280. doi: 10.1142/S1793206809000714
Jacobsen, M. J., and Wilensky, U. (2006). Complex systems in education: scientific and educational importance and implications for the learning sciences. J. Learn. Sci. 15, 11–34. doi: 10.1207/s15327809jls1501_4
Jordan, R. C., Brooks, W. R., Hmelo-Silver, C., Eberbach, C., and Sinha, S. (2014). Balancing broad ideas with context: an evaluation of student accuracy in describing ecosystem processes after a system-level intervention. J. Biol. Educ. 48, 57–62. doi: 10.1080/00219266.2013.821080
Knippels, M. C. P. J. (2002). Coping With the Abstract and Complex Nature of Genetics in Biology Education. Utrecht: CDß Wetenschappelijke reeks. Dissertation Utrecht University.
Koestler, A. (1973). “The tree and the candle,” in Unity through diversity. A Festschrift for Ludwig von Bertalanffy, eds W. Gray and N. D. Rizzo (New York, NY: Gordon and Breach Science Publishers), 287–314.
Koningsveld, H. (1987). Het Verschijnsel Wetenschap. Inleiding tot de wetenschapsfilosofie. [The phenomenon of science. Introduction to the philosophy of science]. Amsterdam; Meppel: Boom.
Levy, T. S., and Wilensky, U. (2008). Inventing a ‘mid-level’ to make ends meet: reasoning between the levels of complexity. Cogn. Instr. 26, 1–47. doi: 10.1080/07370000701798479
Liu, L., and Hmelo-Silver, C. E. (2009). Promoting complex systems learning through the use of conceptual representations in hypermedia. J. Res. Sci. Teach. 46, 1023–1040. doi: 10.1002/tea.20297
National Research Council (2011). A framework for K-12 science education: practices, crosscutting concepts, and core ideas. Washington, DC: The National Academies Press.
NGSS Lead States (2013). Next Generation Science Standards: For States, by States. Achieve, Inc. on Behalf of the Twenty-Six States and Partners That Collaborated on the NGSS, 1–103. doi: 10.17226/18930
Popper, C. (1963). Conjectures and Refutations: The Growth of Scientific Knowledge. London: Routledge and Kegan.
Rocke, A. J. (2010). Image and Reality. Kekulé, Kopp, and the Scientific Imagination. Chicago: Chicago Press.
Sommer, C. (2005). Untersuchung der Systemkompetenz von Grundschulern im Bereich Biologie. [Study on the Primary Students' Systems Competence of in Biology]. Dissertation, Christian-Albrechts-Universität Kiel/Germany.
Tripto, J., Ben-Zvi Assaraf, O., Snapir, Z., and Amit, M. (2016). A reflection interview – “What is a system” as a knowledge integration activity for high school students' understanding of complex systems in human biology. Int. J. Sci. Educ. 38,564–595. doi: 10.1080/09500693.2016.1150620
Tripto, J., Ben-Zvi Assaraf, O., Snapir, Z., and Amit, M. (2017). How is the body's systemic nature manifested amongst high school biology students? Instruc. Sci. 45, 73–98. doi: 10.1007/s11251-016-9390-0
Van Aalsvoort, J. G. M. (2000). Chemistry in Products. A Cultural-Historical Approach to Initial Chemical Education. Dissertation, Utrecht University.
Verhoeff, R. P. (2003). Towards Systems Thinking in Cell Biology Education. Utrecht: C.D.ß Wetenschappelijke reeks (dissertation).
Verhoeff, R. P., Boersma, K. T., and Waarlo, A., J. (2013). “Multiple representations in modeling strategies for the development of systems thinking in biology education,” in Multiple Representations in Biological Education. eds D. F. Treagust and Chi-Yan Tsui (Dordrecht; Heidelberg; New York, NY; London: Springer), 331–348.
Verhoeff, R. P., Waarlo, A. J., and Boersma, K. T. (2008). Systems modelling and the development coherent understanding of cell biology. Int. J. Sci. Educ. 30, 543–568. doi: 10.1080/09500690701237780
von Bertalanffy, L. (1968). General System Theory. Foundations, Development, Applications. New York, NY: Braziler.
Keywords: systems thinking, systems theory, modeling, biology education, qualitative analysis, coherent understanding, cognitive skill
Citation: Verhoeff RP, Knippels MCPJ, Gilissen MGR and Boersma KT (2018) The Theoretical Nature of Systems Thinking. Perspectives on Systems Thinking in Biology Education. Front. Educ. 3:40. doi: 10.3389/feduc.2018.00040
Received: 21 March 2018; Accepted: 17 May 2018;
Published: 05 June 2018.
Edited by:
Amy Ardell, Chapman University, United StatesReviewed by:
Suparna Sinha, Rutgers, The State University of New Jersey, United StatesRosemary Hipkins, New Zealand Council for Educational Research, New Zealand
Copyright © 2018 Verhoeff, Knippels, Gilissen and Boersma. This is an open-access article distributed under the terms of the Creative Commons Attribution License (CC BY). The use, distribution or reproduction in other forums is permitted, provided the original author(s) and the copyright owner are credited and that the original publication in this journal is cited, in accordance with accepted academic practice. No use, distribution or reproduction is permitted which does not comply with these terms.
*Correspondence: Roald P. Verhoeff, ci5wLnZlcmhvZWZmQHV1Lm5s