- 1School of Education, University of Strathclyde, Glasgow, United Kingdom
- 2Faculty of Management Science, Ubon Ratchathani University, Ubon Ratchathani, Thailand
University dropout is a major policy concern around the world because of its consequences for the individual, institutions and society. In this study, we offer new evidence by examining the cumulative effect of academic vulnerability and family support on trajectory of dropout among cohorts of undergraduate students in Thailand. Data were drawn from administrative records of two cohorts of students (n = 1,613), and consisted of information on which semester individual's dropped out of university. Using discrete time survival analysis, we first modeled the trajectory of dropout without predictors followed by a conditional model which examined the effects of predictors on the trajectory of dropout. Cumulative effects of predictors were then examined by plotting the probabilities of their combined effects on dropout in each semester. Our findings show that while the beginning of the second year was a critical period of dropout with almost 20% of students leaving by this time, as much as 10% of students drop out between the second and final year. Students with the lowest entry grades were about 2.17 times more likely to dropout while those who were farther away from family support were 1.32 times more likely to drop out across each semester. The cumulative effect of low entry grades and living away from family support resulted in a 30% probability of dropping out in the second year. The dropout rate among this category of students by the final year was 60% compared to only 14% for students with high entry grades and who live close to their families. Among other things, we recommend that interventions to reduce dropout should encompass both helping students to access family support and develop personal connections at university to compensate for absence of family support, as well as academically focused support for student who do not have a strong entry qualification.
Introduction
Dropping out of university without having gained a degree have significant consequences for individuals, institutions and society (Voelkle and Sander, 2008; O'Neill et al., 2011; Hällsten, 2017; Sarra et al., 2018). Apart from wasted time, private cost and potential psychological trauma for the individual (Ortiz and Dehon, 2013; Faas et al., 2018), those who dropout suffer a scarring effect in the form of greater marginalization and negative labor market outcomes. In a study of mid-life marginalization of Swedish males, Hällsten (2017) found that dropouts spent about 3 percentage points more of their first 8 years in a state of low earnings compared to those who never entered university. Dropout rates may also cast an institution in a negative light and affect its future recruitment drive. This is because institutional dropout rates can be construed by potential students as a sign of deficient teaching and support available at the institution (Voelkle and Sander, 2008). From a societal point of view, dropout is argued to be a waste of tax resources due to the individual blocking a university place which could have been taken by another student (Voelkle and Sander, 2008; Ortiz and Dehon, 2013). Although students who dropout may re-enroll into another institution or field of study (Voelkle and Sander, 2008; Hovdhaugen, 2009; Sittichai, 2012; Ortiz and Dehon, 2013), ensuring retention has become a major policy concern for governments and institutions around the world due to the aforementioned negative consequences of dropout.
Several studies have investigated the nature and predictors of university dropout among students in a bid to inform appropriate interventions. The conclusion from these studies is that the determinants of dropout are many and complex with little consensus on which combination of factors is important (Tinto, 1975; Stratton et al., 2008; Melguizo et al., 2011; Ortiz and Dehon, 2013; Heublein, 2014; Bernardo et al., 2016; Contini et al., 2018; Mabel and Britton, 2018). For instance, Tinto's (1975) seminal student integration model proposed a complex array of risk antecedents encompassing students' pre-entry attributes, goals and commitments, as well as academic and social experiences at university.
However, our knowledge of the determinants of dropout is limited in several ways. First, despite the prevalence of dropout in universities around the world, majority of the literature has emanated from the US and Europe (Voelkle and Sander, 2008; Ortiz and Dehon, 2013; Stewart et al., 2015), with comparatively very few studies undertaken outside high income countries. Second, due to the absence of longitudinal data, very few studies have examined risk antecedent of university dropout across time. Most studies have tended to focus on whether students dropped out or not without taking the trajectory of dropout into account (e.g., Melguizo et al., 2011). Third, the majority of studies examining the determinants of dropout tend to focus on dropout intentions rather than actual dropout (e.g., Alkan, 2014; Bonaldo and Pereira, 2016). Fourth, existing studies argue that student dropout consists of interplay among several factors (e.g., Paterson, 2017; Contini et al., 2018; Sarra et al., 2018). However, a review of research on dropout indicates that most studies mainly tell us about the predictive power of each risk antecedent rather than their cumulative effect on dropout over time. The importance of examining cumulative risk has been well-documented in research on child development (e.g., Atzaba-Poria et al., 2004; Mackenzie et al., 2011; Solomon et al., 2016). According to this cumulative risk model, the synergistic effect of a variety of risk factors is more important in determining adverse outcome than the aggregated effect of a single risk factor (e.g., Evans et al., 2013). Thus, a student who experiences multiple risk factors should be at a greater risk of dropout than a student who experiences only one of these factors. Drawing on the cumulative risk hypothesis, this study addresses the current gap in the literature by examining how the cumulative effect of two key determinants found in the literature, that is, academic vulnerability and family support, influences the trajectory of dropout in Thailand. We argue that, understanding how cumulative risk influences dropout can help identify which groups are most at risk and to develop interventions targeted at retaining members of the at-risk group.
The Role of Academic Vulnerability and Family Support in Dropout
Students' intellectual capability to cope with the academic demands of university study is one of the most significant risk antecedents consistently identified across the literature (Voelkle and Sander, 2008; O'Neill et al., 2011; Alarcon and Edwards, 2013; Stewart et al., 2015; Contini et al., 2018; Sarra et al., 2018). On the whole, students who enter university with lower grades are at greater risk of dropping out. According to Voelkle and Sander (2008), entry grades affect dropout through an influence on university grades. Students with low entry grades perform poorly in their university exams leading them to either dropout voluntarily or being involuntarily withdrawn by the institution for not meeting the academic grades required for continuing with their studies. Academic vulnerability is also directly related to psychological factors such as academic self-efficacy and academic self-control, an important predictor of persistence at university (Respondek et al., 2017). More recently, there has been greater focus on academic vulnerability in light of policies by governments around the world to achieve equity and social justice in access to higher education (UNESCO, 2015). To widen participation, students from disadvantaged and under-represented groups who are judged as having the potential to succeed at university are usually admitted with relatively lower grades than those advertised by universities (e.g., Sosu et al., 2018). While evidence shows that such students achieve similar and sometimes significantly better academic classifications at the end of their degree, they are usually at a greater risk of dropping out due to being academically more vulnerable among other factors (Hoare and Johnston, 2011). However, the effect of academic vulnerability is not deterministic. Voelkle and Sander (2008) in examining group differences in student dropout found that while pre-entry grades predicted dropout for one group, it was not associated with dropout for a second at-risk group, suggesting that pre-entry grades may interact with other factors to influence levels of dropout.
Contextual factors such as the support mechanisms that enable individuals to experience lower levels of emotional distress and become fully integrated into the institution constitute another important determinant of university dropout. Previous studies have suggested that institutional and individual psychological factors (e.g., Bernardo et al., 2016; Suhlmann et al., 2018) can enable students to cope emotionally and become integrated into the institution. A key factor for optimum integration especially in the first year of university is the support students receive from their families. Theoretical and empirical findings suggest that social support offered by close relations is crucial and a universal need for all people. Strong, supportive relationships from families enables individuals to thrive and achieve optimal psychological well-being (Ryan et al., 2005; Brannan et al., 2013; Romero et al., 2015). This is because families and close relations offer a secure base for people and serve as a source of confidence especially in the time of stress (Torres and Solberg, 2001; Uchida et al., 2008). Research on university students has found that family support is strongly associated with experiences of lower emotional distress (Hamdan-Mansour and Dawani, 2008; Brannan et al., 2013), sense of self-esteem (Uchida et al., 2008) and academic self-efficacy (Torres and Solberg, 2001). In a cross cultural study of university students, Brannan et al. (2013) found that social support from family was significantly associated with several components of subjective well-being, including satisfaction with life, positive mood and negative mood, in all the countries examined.
Family support may be particularly salient for students who are embarking on a university degree because it represents a time of significant change and adjustment. First year students have to adjust to a new environment, develop new friendships, and engage in new and challenging modes of independent learning. There is also a greater risk of homesickness and loneliness as this is when most students are separated for the first time from their families. Being homesick or lonely is related to support received from family members and the less family support one has the greater their feelings of loneliness (Nicpon et al., 2006). Availability of family support may also compensate for a lack of social integration at university, one of the important predictors of dropout. In other words, where students are unable to form strong friendships and bonds, closeness to family can compensate for issues of loneliness. However, if family support is not readily available, students might not have compensatory mechanisms available to deal with social isolation and loneliness at university, thereby leading to a higher likelihood of dropping out. Students who feel homesick may take steps to withdraw while those with greater family support are more likely to persist (Mackie, 2001; Walker and Satterwhite, 2002; Nicpon et al., 2006). Mackie's (2001) qualitative study of students who remained and students who dropped out from university suggested that, although very similar problems of academic and social integration were experienced by both categories, the main difference between those who stayed and those who quit involved feeling of homesickness.
On the whole, very few studies (e.g., Bennett, 2003; Nicpon et al., 2006) have investigated the effect of both academic vulnerability and family support on dropout in a single study. This is despite the fact that the effect of academic vulnerability may be dependent on contextual factors such availability of family support to cope with both loneliness and difficulties of academic work during the time at university. Those studies that have examined both factors are limited in several ways. First, they are based on surveys about students' academic persistence rather than actual dropout over time. Second, these studies employ cross sectional rather than longitudinal data which do not tell us about how these risk antecedents influence the nature of dropout over time. A key reason is the general absence of longitudinal data and the difficulties of analyzing such datasets (Voelkle and Sander, 2008).
Context and the Current Study
The current study examined dropout rates among university students in Thailand. Despite the prevalence of dropout in universities around the world, very few studies have examined dropout outside high income countries. Within the Thai context we came across only one published quantitative study (Sittichai et al., 2009) which examined actual rates of dropout among university students. Another purported study on dropout (Iam-On and Boongoen, 2017) was mainly focused on demonstrating the methodological approaches of educational data mining rather than substantive findings on the trajectory and predictors of dropout. In their study, Sittichai et al. found that 20.3% of students enrolling at the Prince Songkla University from 1999 to 2006 dropped out. Unfortunately, like most other studies into university drop out, this study was based on cross-sectional data and no information was provided on the trajectory or critical period of dropout. Previous studies suggest that the highest probability of dropping out from university occurs during the first 2 years of enrolment (Voelkle and Sander, 2008; Alarcon and Edwards, 2013; Ortiz and Dehon, 2013). The first aim of this study is therefore to examine the trajectory and critical period of dropout among Thai students using longitudinal data. An advantage of our data in comparison to previous international longitudinal studies is that it covers dropout rates across the two main semesters in each academic year, and over the 4 years of study rather than just annually.
The second aim of the study is to examine how the cumulative effect of proximity to family support and academic vulnerability influence trajectory of dropout. Sittichai (2012) using a qualitative approach explored why students in the Prince Songkla University in Thailand dropout. A key finding was that, students who came from outside the province where the university was located were disproportionately represented among those dropping out. While several reasons including security concerns in the province were suggested by participants, Sittichai speculated that “studying away from home might be a factor causing dropping out” (2012, p. 286) possibly due to lower levels of emotional support available from their family. In fact, most participants in the study reported visiting home either weekly or once a month. The relative importance of family support for the well-being of students in Asia compared with Europe has been documented (e.g., Uchida et al., 2008; Brannan et al., 2013). It can be hypothesized from the foregone discussion that family support ensures optimum social integration and coping which is necessary for students to thrive academically and by extension enable them to persist with their studies. Thus, academically vulnerable students who are not close to family support will be at a greater risk of dropping out than those who are only either academically vulnerable or far away from family support. As far as we are aware no studies on dropout internationally, or in the Thai context have explored how the cumulative effect from these two factors influences the risk of dropping out. To summarize, the current study was guided by the following research questions:
1. What is the trajectory and critical period of dropout among university students in Thailand?
2. How do cumulative effects of pre-entry grades and proximity to family support influence dropout over time?
In what follows, we review the methodological approach used and present our findings. We then discuss these findings in light of the existing literature on dropout. We conclude by making recommendations on possible support mechanisms that can be provided to help reduce the risk of dropout especially among academically vulnerable students who are farther away from their families.
Methodology
Participants and Ethics
Data for this study was drawn from administrative records of students enrolled in a university located in the North-East of Thailand. Administrative data sets are usually collected by institutions for administrative purposes, but can with careful consideration be used to explore complex research questions where data availability is sparse. Such data can provide a solution to the problem of availability of longitudinal data. Ethical approval for the use of the anonymized administrative data for research purposes was obtained from the University Management Committee, who were the data owners/custodians. Since only anonymized data was obtained from the institution and the research was in line with the institution's aim to improve the student experience, direct consent was not obtained from students. This approach of not obtaining individual consent is in line with existing ethical protocols relating to the use of administrative data (Regidor, 2004; Stiles and Boothroyd, 2015). Further, direct consent from students was not possible in our case due to the focus of this research, that is, dropout. This is because institutions usually have limited to no direct contact with students who have dropped out. Ethical protocols relating to data security, disclosure and appropriate use were followed throughout the research process.
For the current study, data were drawn from two cohorts of students (2009–2013 and 2010–2014) enrolled on a Faculty of Management Science programmes of Ubon Ratchathani University, Thailand. Available information covered eight semesters (4 year programme) for both cohorts. Following data cleaning, 1,016 records were retained for the 2009 cohort and 597 records for the 2010 cohort. We selected only students who met the full criteria of enrolling on the programme. Thus, students who were admitted but did not take up their admission were eliminated from the records. Only anonymised version of the administrative data were obtained following approval from the university management board.
Measures
Several items and variables were recoded, dichotomised or re-categorized in order to compute new scales, clarify variables of interest to ensure results are meaningful and easy to interpret. The approaches used were informed by discrete time survival analysis procedures, the analytical approach used in this study.
Dropout
Dropout was measured using a variable called “Student Status” which indicated whether a student was still studying or dropped out in that semester. Information on dropout was available for each of the 8 semesters (two semesters per year), enabling us to determine the time of dropout. A coding system which assigned students to one of two categories (dropout-1; continuing-0) was used for coding outcomes in the first semester. For subsequent semesters, students who drop out in previous semesters were assigned a missing value of −999 as they have already experienced the event and were therefore no longer “at risk” of dropping out (Muthén and Masyn, 2005). Further details on the data preparation for analysis are presented in the analytic procedure section below.
Academic Vulnerability
This was measured using high school grade point average (GPA) scores of students. Based on previous evidence discussed above, we consider students with lower entry grades to be academically vulnerable. Entry grades ranged from 0 to 4 with higher scores indicating better attainment. The average entry GPA across the 2 cohorts was 2.89 to 2.80 (Table 1). Entry grades were recoded as polytomous variables with the following cut-offs: Low (0–2.49), Average (2.5–2.99), and High (3–4) in line with classifications employed by the institution. Analyses were also undertaken with the full scale to ensure that levels and directions of effects were not different.
Proximity to Family Support
This was measured using responses on students' place of abode prior to enrolling at the university. Participants were classified as Close to Family Support where they indicated their place of residence to have been from the province where the university was located (Close = 1) and Far from Family Support where they indicated they came from a province other than where the institution was located (Far = 0). It is important to stress that, this variable only represents where students said they originated from (where they resided with parents or guardians prior to coming to the university) rather than where they were currently residing as students. Across the two cohorts, 41% of the students were close to family support as per our classification and 59% were far from their families (Table 1).
Gender
Evidence suggests that female students have lower failure rates during the first year at university and a lower hazard of dropping out of university (Ortiz and Dehon, 2013), although opposite findings have also been reported (Belloc et al., 2010; Stewart et al., 2015). There is also some suggestion that gender might be related to the level of social support received (e.g., Nicpon et al., 2006). As a result, we controlled for the effect of gender in the analysis. Gender was measured as a dichotomous variable with Males coded [0] and Females coded [1]. Majority of students (83%) on the programme were female (Table 1).
Analytic Procedure
A discrete time survival analysis (DTSA) within the latent variable framework was utilized in analyzing the data (Muthén and Masyn, 2005). It is appropriate when modeling survival rates with longitudinal categorical data such as student dropout from university. The procedure enables researchers to estimate if an event has occurred (e.g., students dropped out or not) and when an event has occurred (e.g., semester in which they dropped out), rather than a simple focus on if the event has occurred (i.e., dropout vs. not dropping out). Thus, DTSA enables us to examine the trajectory of dropout over the course of a university degree. Additionally, DTSA makes it possible to include covariates in order to examine how these predict the trajectory of dropout (Willett and Singer, 1991; Muthén and Masyn, 2005; Ortiz and Dehon, 2013).
We followed several steps in the analytic process (see e.g., Muthén and Masyn, 2005). First, the data for each individual were converted into a set of binary event history indicators (0—individual is at risk of dropping out but does not dropout; 1—individual has dropped out). A missing value (−999) is recorded for all subsequent periods for any individual who experienced the event (i.e., dropped out). An event history was created for each individual covering the 8 main semesters within the 4 year programme. For example, Participant A (Table 2), has the event history of an individual who did not dropout by the 8th semester. Participant B is an individual who experienced dropout in semester 8, while Participant C is an individual who experienced dropout in semester 7.
Second, we estimated an unconditional survival model. This model assesses the hazard probability of dropout over time without including predictors. The hazard probability is defined as the probability of dropping out in discrete time period, given that dropout was not experienced before that time period (Muthén and Masyn, 2005). Thus, for a given semester, the hazard probability is the number of students who experienced dropout at that time period divided by the total number of students who are at risk in that time period. Students are classified as being at risk if they have not experienced the event in an earlier semester. For example, for the 2009 cohort (Table 3), the number of students defined as being at risk in the first semester is all students who enrolled in the programme (n = 1,016). The number who experienced the event, that is dropped out was 32. Thus, the estimated hazard probability is 0.03. However, for the second semester, only those who have not experienced the event in semester 1 (n = 984) are defined as at risk. With 59 students dropping out the hazard probability is 0.05 signifying an increase in the risk of dropping out in the second semester. Conversely, a survival probability which is the probability of not dropping out beyond a specified time period is estimated. For each time period, the probability of not experiencing an event is simply one minus the hazard probability. The probability of surviving beyond a specific time period is therefore the product of the time specific survival probabilities (Muthén and Masyn, 2005; Masyn, 2014). For example, the survival probability beyond the second semester will be defined as (1–0.03*1–0.05) giving us a survival probability of 0.92.
Third, we estimated a conditional survival model which includes predictors of the hazard probabilities, that is, trajectory of dropout. The predictors are related to the hazard probability using logistic regression to estimate their effect on the log hazard odds of dropout. Figure 1 is a diagrammatic representation of the conditional model within the structural equation modeling framework where the effects of predictors on dropout do not vary across time.
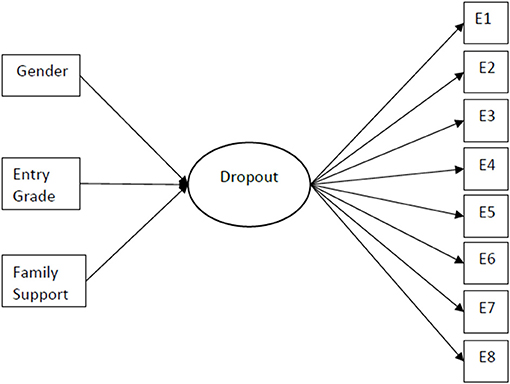
Figure 1. A conditional model of dropout with time-invariant predictors. The 8 binary time-specific indicators correspond to the hazard probabilities of dropout across semesters.
Model Evaluation
Different aspects of the hazard model were evaluated using the Bayesian Information Criteria (BIC) and the Log-likelihood ratio test statistics which assesses the difference in fit between any two nested models (Muthén and Masyn, 2005; Masyn, 2014; Maslowsky et al., 2015). Specifically, we compared unconditional and conditional models to evaluate whether the inclusion of predictors resulted in significant improvement in the model. Additionally, we assessed the proportionality assumption by comparing a model where the effects of the predictors are constrained to be equal across time to one where the effects of the predictors are allowed to vary across time. A smaller BIC is indicative of a better model fit. The likelihood ratio test statistic (LRTS) is computed as:
LRTS = −2[(log-likelihood for Model 0)–(log-likelihood for Model 1)]
The LRTS is approximately distributed as chi-square. The degrees of freedom (df) for determining the significance of LRTS is obtained by subtracting the number of free parameters in Model 0 from the number of free parameters in Model 1 (npar1 – npar0). If the p-value is significant, then it suggests that the alternative model fits the data statistically significantly better than the more restrictive null model (Masyn, 2014). The predictors included in our analysis were entry grade, proximity to family support, and gender. All DTSA models were analyzed using Mplus 8 with maximum likelihood estimation.
Results
Unconditional Survival Model
Results of the unconditional hazard model are identical to that indicated in Table 3 (Log-likelihood = −1687.787; BIC = 34134.662, number of parameters = 8). A graphic representation of the hazard and corresponding survival probabilities for the individual (2009 and 2010 cohorts) and combined cohorts indicates similar trends in the trajectory of dropout (Figure 2). The hazard probability of dropping out initially increases from an average of 3–5% in semesters one and two in the first year of university, peaking at semester 3 (i.e., beginning of second year), with an average hazard of 13% for those still at risk, before decreasing over time. From the second semester of third year of university to the end of the programme in year 4, the hazard probability for those at risk decreases to 1%. The survival probabilities indicate an increase in the number of students dropping out from university with almost 20% leaving by the beginning of the second year and close to 30% by the 8th semester.
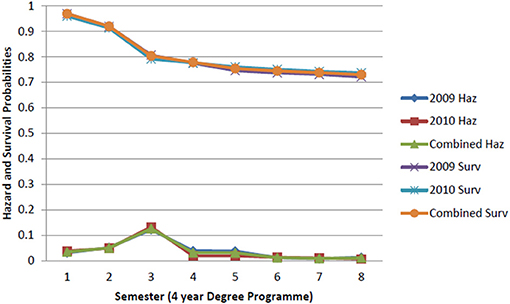
Figure 2. Unconditional hazard and survival probability plots for the 2009, 2010, and combined cohorts.
Since similar trends in dropout were observed for the two cohorts, all subsequent analyses were undertaken using the combined data sets. This approach enabled us to examine and draw conclusions about trends across cohorts.
Conditional Model
In the conditional model, we estimated the effect of academic vulnerability, proximity to familial support, and gender on trajectory of dropout. We estimated a proportional hazard model which assumes the effect of the predictors to be equal across all time points. The model fit indicates an improvement over the previous model (Log-likelihood = −1552.329; BIC = 3185.658, number of parameters = 11) and results from the Log-likelihood test indicates that this model was a better fit than the unconditional model df = 3, X2 = 270.916, p < 0.001.
The findings (Table 4) indicate that students' entry grades have a significant negative effect on the hazard of dropout, with a 46% (hazard odd ratio = 0.46) decrease in the average hazard odds of dropout for each unit increase in entry grades. Stated differently, students with the lowest entry grade have approximately 1/0.46 = 2.17 times higher hazard odds for dropping out of university. Additionally, students who were close to family support were significantly less likely to dropout, with closeness to family support associated with a 76% decrease in the average hazard odds of dropout compared to students whose families were farther away. In other words, students who were farther away from family support have ~1.32 times higher hazard odds for dropping out university. While the analysis suggest that female students were less likely to dropout, this gender effect was not statistically significant. A follow-up analysis indicates that differences in male and female dropout were due to differences in entry grade, with females having higher entry qualification t(1, 564) = −10.72, p < 0.001.
The finding from this model is underpinned by a proportionality assumption which suggests that the effect of the predictors on dropout is the same across time. To evaluate this assumption, we compared the fit of the model which constraints the covariate effects to be equal across time (Log-likelihood = −1552.329; BIC = 3185.577, number of parameters = 11) to a model which allows the effect of the predictors to vary across time (Log-likelihood = −1539.154; BIC = 3313.708, number of parameters = 32). Results from the log-likelihood test indicates that the model which allows the effect of predictors on dropout to vary across time was not significantly better than the model with the proportionality assumption df = 21, X2 = 26.011, p > 0.05. The assumption that the effect of academic vulnerability and distance from family on dropout is the same across time was therefore supported.
In order to estimate the cumulative effect of academic vulnerability and distance from familial support on dropout, we computed hazard and survival probabilities for each time point for six different sub-groups of students based on combinations of levels of entry qualifications (low, average, high) and proximity to family support (close vs. far) using an equation analogous to a traditional logistic regression (Muthén and Masyn, 2005; Masyn, 2014). Results (Figure 3) indicate that the subpopulation with the highest risk of dropout across the 8 semesters was students with low entry qualifications who are farther away from family support. The hazard probability of dropping out for this group was 30% during the second year of university. The second group with a high risk of dropout was those with low entry grades but whose families are close by. The hazard probability of dropout for this group during the critical second year was 24%. Thus, students who experienced both risk factors were consistently at a greater risk of dropping out in comparison to peers who were only academically vulnerable. Additionally, across all entry grade groups, students who are closer to family support had a lower risk of dropping out.
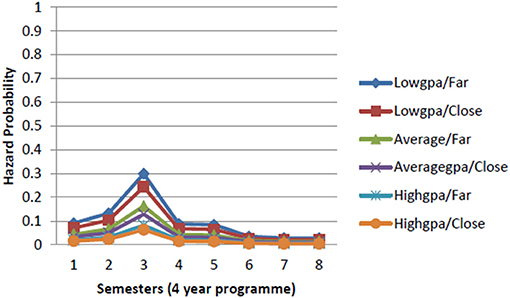
Figure 3. Hazard probability plots for the cumulative effect of academic vulnerability and proximity to family support on dropout over time.
The corresponding survival probabilities (Figure 4) indicate that only about 40% of students with low entry qualifications who are farther away from family support remained at university by the end of the 8th semester with about 60% dropping out by the final semester. This represent a significant level of vulnerability when compared to an overall dropout rate of <14% for students with high entry grades and who are close to family support.
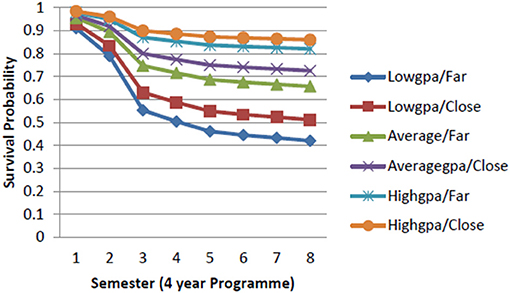
Figure 4. Survival probability plots for the cumulative effect of academic vulnerability and proximity to family support on dropout over time.
Discussion
The present study examined the cumulative effect of academic vulnerability and family support on the trajectory of dropout among undergraduate students in Thailand. We found the probability of dropping out increases sharply from the first semester of study to its peak in the third semester (second year of study), and decreases over the remaining period of study. Consistent with previous research on the critical period of dropout (Voelkle and Sander, 2008; Alarcon and Edwards, 2013; Ortiz and Dehon, 2013), dropout was highest within the first 2 years in our sample with about 20% of students leaving the programme. It is therefore a critical period to ensure retention. Our findings however indicate that an additional 10% of students leave after the critical period and that student dropout occurs in every semester of the 4 year programme. This latter finding is rarely emphasized in existing studies (Mabel and Britton, 2018) despite the likelihood that dropping out much later in the course of a degree might be emotionally more traumatizing for students than quitting during the first 2 years of study due to a higher level of investment. Our findings were slightly different from (Mabel and Britton, 2018) study which also examined dropout in later years of study. Unlike their study, we did not find a spike in dropout among students who were closer to completion. These differences may be due to differences in our samples. While our sample consisted entirely of students enrolled on a 4 year degree, Mabel and Britton (2018) sample included students enrolled on 2 years college courses.
The question of why students drop out from university was illuminated by our findings on the cumulative effect of academic vulnerability and proximity to family support. As demonstrated by other studies (Voelkle and Sander, 2008; O'Neill et al., 2011; Alarcon and Edwards, 2013; Stewart et al., 2015; Contini et al., 2018; Sarra et al., 2018) we found that academic vulnerability significantly increased the risk of dropping out. Students with lower entry grades were more likely to dropout in comparison to their peers with average to high entry grades. This is because those with lower entry grades are more likely to perform poorly in university exams leading them to either dropout voluntarily or be involuntarily withdrawn by the university for not meeting the minimum grade requirements (Voelkle and Sander, 2008). Additionally, distance from family support was a significant risk antecedent of dropout as hypothesized in our study. Students who originated from the province within which the institution was located, a proxy measure for closeness to family support, demonstrated greater persistence than those who were farther away from family support, even if they had similar entry grades. As suggested by previous studies, family support is strongly associated with experiences of lower emotional distress (Hamdan-Mansour and Dawani, 2008; Brannan et al., 2013), sense of self-esteem (Uchida et al., 2008), feeling of lonliness and academic self-efficacy (Torres and Solberg, 2001). These factors are crucial in helping university students to cope and adjust to their new environment especially during the first year of study (Mackie, 2001; Walker and Satterwhite, 2002; Nicpon et al., 2006).
The cumulative effect of these two factors was revealing. The most academically vulnerable students who were farther away from family support were at the greatest risk of dropping out from university. The dropout rate for this particular group of students within the critical first 2 years of study was about 45% with almost 60% of students belonging to this group dropping out by the end of their study. Consistent with the cumulative risk model (Evans et al., 2013), those who experienced both risk factors, were at a greater risk of dropping out in comparison to peers who experienced only one of the risk factors. While academic vulnerability was the main driver of dropping out, within each entry qualification group, students who are close to family support tended to be at a lower risk than their peers whose families are farther away. It can be argued from the findings that distance from family support may lead to emotional difficulties that can exacerbate the effect of academic vulnerability in influencing dropout. Alternatively, it is plausible that being academically vulnerable leads to greater levels of stress (Respondek et al., 2017) and the absence of family support to help cope increases the risk of dropout.
A possible reason why academically vulnerable students who are close to their families fare better than those whose families are farther away is that those with families close by may be able to easily turn to their families by visiting home for moral support when they are experiencing academic difficulties. Additionally, these students only have to contend with one challenge at the beginning of university, which is, overcoming academic difficulties while their peers contend with both emotional and academic difficulties due to absence of immediate support. It is also possible that our measures of proximity to family support simply reflects other contextual factors such as familiarity with the environment (province where the institution is located) and culture making it easier for them to easily integrate in university life and persist. A key theoretical implication of our finding is the need to adopt a cumulative risk perspective to get a deeper understanding of the risk antecedents as well as protective factors relating to university dropout.
Limitations
Our findings are limited by the fact that we were only able to explore the cumulative effects of two of the main predictors of dropout. Determinants of dropout are numerous and complex (Stratton et al., 2008; Ortiz and Dehon, 2013; Contini et al., 2018; Sarra et al., 2018) and other important factors such as students' socioeconomic positions or parental education were not explored due to these not being available in our administrative data. It is possible that some of these effects are captured through academic vulnerability possibly inflating the effect of entry grades on dropout. Secondly, our measure of proximity to family support was based on a proxy variable derived from where students indicated as their place of domicile prior to entering university. This variable does not capture any changes in a family's place of residence during the student's time at university. Thirdly, our sample is limited to one faculty in a Thai university. While this provides a useful first insight into cumulative effects of risk factors on the trajectory dropout, caution is needed in drawing generalizations from these findings. Future research using national datasets will help provide findings that are more generalizable. Finally, our data does not account for students who directly re-enroll at a different institution which might lead to an overestimation of actual dropout rates in our sample.
Educational and Policy Implications
Despite the above limitations, several policy and educational implications can be drawn from our study. Firstly, our findings suggest that policies and resources should be targeted at supporting students during the first 2 years of enrolment which is the critical period of dropout. However, considering a substantial proportion of students drop out after this critical period, ongoing support should be in place to help students who are at risk of dropping out.
Our findings on the cumulative effect of proximity to family support and academic vulnerability suggests that interventions to reduce dropout should encompass both attention to helping students to access social and family support, as well as academically focused support for students who might not have the strong academic background at entry to university. All around the world students travel away from their families to begin university studies and it is a period of significant change and adjustment where students have to cope with new environments, loneliness, development of new friendships and engagement with independent learning. Helping these students to access family support and strengthening the social support available to compensate for the absence of family support should help increase retention and decrease dropout during this critical period. In doing so, institutions may want to look at decentralizing support closer to students. These may be support provided at a level of small tutor groups, in halls of residence, and through buddy systems that use mentors who share similar backgrounds to enable students make friends and establish a sense of belonging. Universities should also consider the potential use of technology to enable students easily access family support. Institutions may for instance want to establish state of the art “family communication hubs” where students can go and make video or voice calls to their families at no cost. Such hubs may be particularly useful for students who may not have the means or access to smartphones to be able to communicate with families via voice or audio-visual media platforms. Universities can also consider developing programmes to help parents on how they can provide support for their children at the beginning of university study (Nicpon et al., 2006). With respect to academic vulnerability, support could be provided through organization of pre-university summer schools, or making available extra tuition and mentoring sessions provided by second to final year students from similar backgrounds or tutors. An alternative is for institutions and specific university courses to identify the basic skills required by all students and providing general catch up sessions for all students as part of their initial lectures and tutorial sessions. Finally, we agree with the argument put forward by Mabel and Britton (2018) that universities need to do more to target students who are closer to graduating but remain at risk of dropout as a cost-effective strategy for increasing retention. As demonstrated by the present study and (Mabel and Britton, 2018) findings, these are students who are generally academically vulnerable.
To conclude, our study extends current theoretical insights on the determinants of university dropout by examining the cumulative effect of academic vulnerability and proximity to family support across time. As far as we are aware it is the first paper to apply longitudinal approach in examining cumulative effects on university student dropout outside of a US or European context. Recommendations from this study will contribute to efforts by universities and policy makers in increasing retention among students.
Author Contributions
ES and PP contributed to the conceptualization of the study and undertook data analysis and contributed to the writing of the manuscript. ES undertook literature review.
Conflict of Interest Statement
The authors declare that the research was conducted in the absence of any commercial or financial relationships that could be construed as a potential conflict of interest.
Acknowledgments
We are grateful for the British Council Researcher Links Award which enabled the researchers to collaborate and work on this project.
References
Alarcon, G. M., and Edwards, J. M. (2013). Ability and motivation: assessing individual factors that contribute to university retention. J. Educ. Psychol. 105, 129–137. doi: 10.1037/a0028496
Alkan, N. (2014). Humor, loneliness, and acceptance: predictors of university drop-out intentions. Proc. Soc. Behav. Sci. 152, 1079–1086. doi: 10.1016/j.sbspro.2014.09.278
Atzaba-Poria, M., Pike, A., and Deater-Deckard, K. (2004). Do risk factors for problem behaviour act in a cumulative manner? An examination of ethnic minority and majority children through an ecological perspective. J. Child Psychol. Psychiatry 45, 707–718. doi: 10.1111/j.1469-7610.2004.00265.x
Belloc, F., Maruotti, A., and Petrella, L. (2010). University drop-out: an Italian experience. High. Educ. 60, 127–138. doi: 10.1007/s10734-009-9290-1
Bennett, R. (2003). Determinants of undergraduate student drop out rates in a university business studies department. J. Further High. Educ. 27, 123–141. doi: 10.1080/030987703200065154
Bernardo, A., Esteban, M., Fernández, E., Cervero, A., Tuero, E., and Solano, P. (2016). Comparison of personal, social, and academic variables related to university drop-out and persistence. Front. Psychol. 7:1610. doi: 10.3389/fpsyg.2016.01610
Bonaldo, L., and Pereira, L. N. (2016). Dropout: demographic profile of Brazilian university students. Proc. Soc. Behav. Sci. 228, 138–143. doi: 10.1016/j.sbspro.2016.07.020
Brannan, D., Biswas-Diener, R., Mohr, C. D., Mortazavi, S., Stein, N., and Brannan, D. (2013). Friends and family: a cross-cultural investigation of social support and subjective well-being among college students. J. Posit. Psychol. 8, 65–75. doi: 10.1080/17439760.2012.743573
Contini, D., Cugnata, F., and Scagni, A. (2018). Social selection in higher education. Enrolment, dropout and timely degree attainment in Italy. High. Educ. 75, 785–808. doi: 10.1007/s10734-017-0170-9
Evans, G. W., Li, D., and Whipple, S. S. (2013). Cumulative risk and child development. Psychol. Bull. 139, 1342–1396. doi: 10.1037/a0031808
Faas, C., Benson, M. J., Kaestle, E. C., and Savla, J. (2018). Socioeconomic success and mental health profiles of young adults who drop out of college. J. Youth Stud. 21, 669–686. doi: 10.1080/13676261.2017.1406598
Hällsten, M. (2017). Is education a risky investment? The scarring effect of university dropout in Sweden. Eur. Sociol. Rev. 33, 169–181. doi: 10.1093/esr/jcw053
Hamdan-Mansour, A. M., and Dawani, H. A. (2008). Social support and stress among university students in Jordan. Int. J. Ment. Health Addict. 6, 442–450. doi: 10.1007/s11469-007-9112-6
Heublein, U. (2014). Student drop-out from German higher education institutions. Eur. J. Educ. 49, 497–513. doi: 10.1111/ejed.12097
Hoare, A., and Johnston, R. (2011). Widening participation through admissions policy – a British case study of school and university performance. Stud. High. Educ. 36, 21–41. doi: 10.1080/03075070903414297
Hovdhaugen, E. (2009). Studies in higher education transfer and dropout : different forms of student departure in Norway. Stud. High. Educ. 34, 1–17. doi: 10.1080/03075070802457009
Iam-On, N., and Boongoen, T. (2017). Improved student dropout prediction in Thai University using ensemble of mixed-type data clustering. Int. J. Mach. Learn. Cyber. 8, 497–510. doi: 10.1007/s13042-015-0341-x
Mabel, Z., and Britton, T. A. (2018). Leaving late: understanding the extent and predictors of college late departure. Soc. Sci. Res. 69, 34–51. doi: 10.1016/j.ssresearch.2017.10.001
Mackenzie, M. J., Kotch, J. B., Lee, L.-C., Augsberger, A., and Hutto, N. (2011). A cumulative ecological–transactional risk model of child maltreatment and behavioral outcomes: reconceptualizing early maltreatment report as risk factor. Child. Youth Serv. Rev. 33, 2392–2398. doi: 10.1016/j.childyouth.2011.08.030
Mackie, S. E. (2001). Jumping the hurdles: undergraduate student withdrawal behaviour. Innov. Educ. Teach. Int. 38, 265–276. doi: 10.1080/14703290110056371
Maslowsky, J., Jager, J., and Hemken, D. (2015). Estimating and interpreting latent variable interactions: a tutorial for applying the latent moderated structural equations method. Int. J. Behav. Dev. 39, 87–96. doi: 10.1177/0165025414552301
Masyn, K. E. (2014). “Discrete-time survival analysis in prevention science,” in Defining Prevention Science, Advances in Prevention Science, eds Z. Sloboda, and H. Petras (New York, NY: Springer Science), 513–535.
Melguizo, T., Torres, F. S., and Jaime, H. (2011). The association between financial aid availability and the college dropout rates in Colombia. High. Educ. 62, 231–247. doi: 10.1007/s10734-010-9385-8
Muthén, B., and Masyn, K. (2005). Discrete-time survival mixture analysis. J. Educ. Behav. 30, 27–58. doi: 10.3102/10769986030001027
Nicpon, M. F., Huser, L., Blanks, E. H., Sollenberger, S., Befort, C., and Kurpius, S. E. R. (2006). The relationship of loneliness and social support with college freshmen's academic performance and persistence. J. Coll. Stud. Ret. 8, 345–358. doi: 10.2190/A465-356M-7652-783R
O'Neill, L. D., Wallstedt, B., Eika, B., and Hartvigsen, J. (2011). Factors associated with dropout in medical education: a literature review. Med. Educ. 45, 440–454. doi: 10.1111/j.1365-2923.2010.03898.x
Ortiz, E. A., and Dehon, C. (2013). Roads to success in the Belgian French community's higher education system: predictors of dropout Bruxelles. Res. High. Educ. 54, 693–723. doi: 10.1007/s11162-013-9290-y
Paterson, N. D. (2017). Predictors of first year retention rates at the university of the west Indies, Jamaica. Int. J. Educ. Dev. 55, 63–68. doi: 10.1016/j.ijedudev.2017.06.001
Regidor, E. (2004). The use of personal data from medical records and biological materials: ethical perspectives and the basis for legal restrictions in health research. Soc. Sci. Med. 59, 1975–1984. doi: 10.1016/j.socscimed.2004.02.032
Respondek, L., Seufert, T., Stupnisky, R., and Nett, U. E. (2017). Perceived academic control and academic emotions predict undergraduate university student success: examining effects on dropout intention and achievement. Front. Psychol. 8:243. doi: 10.3389/fpsyg.2017.00243
Romero, D. H., Riggs, S. A., and Ruggero, C. (2015). Coping, family social support, and psychological symptoms among student veterans. J. Couns. Psychol. 62, 242–252. doi: 10.1037/cou0000061
Ryan, R. M., Guardia, G. L. A., Solky-butzel, J., Chirkov, V., and Kim, Y. (2005). On the interpersonal regulation of emotions : emotional reliance across gender, relationships, and cultures. Pers. Relatsh. 12, 145–163. doi: 10.1111/j.1350-4126.2005.00106.x
Sarra, A., Fontanella, L., and Di Zio, S. (2018). Identifying students at risk of academic failure within the educational data mining framework. Soc. Ind. Res. 1–20. doi: 10.1007/s11205-018-1901-8
Sittichai, R. (2012). Why are there dropouts among university students? Experiences in a Thai university. Int. J. Educ. Dev. 32, 283–289. doi: 10.1016/j.ijedudev.2011.04.010
Sittichai, R., Tongkumchum, P., and McNeil, N. (2009). Discontinuation among university students in southern Thailand. Int. Educ. Stud. 2, 17–21. doi: 10.5539/ies.v2n2p17
Solomon, D., Åsberg, K., Peer, S., and Prince, G. (2016). Cumulative risk hypothesis: predicting and preventing child maltreatment recidivism. Child Abuse Neglect. 58, 80–90. doi: 10.1016/j.chiabu.2016.06.012
Sosu, E. M., Smith, L., Santoro, N., and McKendry, S. (2018). Addressing socioeconomic inequality in access to university education: an analysis of synergies and tensions in Scottish policy. Palgrave Commun. 4, 1–8. doi: 10.1057/s41599-018-0206-5
Stewart, S., Lim, D. H., and Kim, J. H. (2015). Factors influencing college persistence for first-time students. J. Dev. Educ. 38, 12–20.
Stiles, P. G., and Boothroyd, R. A. (2015). “Ethical use of administrative data for research purposes,” in Actionable Intelligence, eds J. Fantuzzo, and D. P. Culhane (New York, NY: Palgrave Macmillan), 125–155.
Stratton, L. S., O'Toole, D. M., and Wetzel, J. N. (2008). A multinomial logit model of college stopout and dropout behavior. Econ. Educ. Rev. 27, 319–331. doi: 10.1016/j.econedurev.2007.04.003
Suhlmann, M., Sassenberg, K., Nagengast, B., and Trautwein, U. (2018). Belonging mediates effects of student-university fit on well-being, motivation, and dropout intention. Soc. Psychol. 49, 16–28. doi: 10.1027/1864-9335/a000325
Tinto, V. (1975). Dropout from higher education: a theoretical synthesis of recent research. Rev. Educ. Res. 45, 89–125. doi: 10.3102/00346543045001089
Torres, J. B., and Solberg, V. S. (2001). Role of self-efficacy, stress, social integration, and family support in Latino college student persistence and health. J. Vocat. Behav. 59, 53–63. doi: 10.1006/jvbe.2000.1785
Uchida, Y., Kitaya, S., Mesquita, B., Reyes, J. A., and Morling, B. (2008). Is perceived emotional support beneficial? Well-being and health in independent and interdependent cultures. Pers Soc Psychol Bull. 34, 741–754. doi: 10.1177/0146167208315157
UNESCO (2015). Incheon Declaration: Education 2030: Towards Inclusive and Equitable Quality Education and Lifelong Learning for all. World Education Forum 2015. (Incheon: Republic of Korea). Available online at: http://uis.unesco.org/sites/default/files/documents/education-2030-incheon-framework-for-action-implementation-of-sdg4-2016-en_2.pdf
Voelkle, M. C., and Sander, N. (2008). A structural equation approach to discrete-time survival analysis. J. Individ. Dif. 29, 134–147. doi: 10.1027/1614-0001.29.3.134
Walker, K. L., and Satterwhite, T. (2002). Academic performance among African-American and Caucasian students: is the family still important? Coll. Stud. J. 36, 113–128.
Keywords: University dropout, academic vulnerability, family support, cumulative risk hypothesis, discrete time survival analysis, longitudinal administrative data, Thai University
Citation: Sosu EM and Pheunpha P (2019) Trajectory of University Dropout: Investigating the Cumulative Effect of Academic Vulnerability and Proximity to Family Support. Front. Educ. 4:6. doi: 10.3389/feduc.2019.00006
Received: 19 June 2018; Accepted: 22 January 2019;
Published: 12 February 2019.
Edited by:
Lia M. Daniels, University of Alberta, CanadaReviewed by:
Christian Wandeler, California State University, Fresno, United StatesAna Bernardo, Universidad de Oviedo Mieres, Spain
Copyright © 2019 Sosu and Pheunpha. This is an open-access article distributed under the terms of the Creative Commons Attribution License (CC BY). The use, distribution or reproduction in other forums is permitted, provided the original author(s) and the copyright owner(s) are credited and that the original publication in this journal is cited, in accordance with accepted academic practice. No use, distribution or reproduction is permitted which does not comply with these terms.
*Correspondence: Edward M. Sosu, ZWR3YXJkLnNvc3VAc3RyYXRoLmFjLnVr