- 1Department of Psychology and Neuroscience, University of North Carolina at Chapel Hill, Chapel Hill, NC, United States
- 2Department of Science and Mathematics, Clarke University, Dubuque, IA, United States
- 3Department of Chemistry and Life Science, United States Military Academy, West Point, NY, United States
- 4Department of Natural and Applied Sciences, University of Dubuque, Dubuque, IA, United States
- 5Department of Biology, Emory University, Atlanta, GA, United States
- 6Department of Biological Sciences, Fordham University, Bronx, NY, United States
- 7Department of Biological Sciences and Biotechnology Program, North Carolina State University, Bronx, NY, United States
As educators and researchers, we often enjoy enlivening classroom discussions by including examples of cutting-edge high-throughput (HT) technologies that propelled scientific discovery and created repositories of new information. We also call for the use of evidence-based teaching practices to engage students in ways that promote equity and learning. The complex datasets produced by HT approaches can open the doors to discovery of novel genes, drugs, and regulatory networks, so students need experience with the effective design, implementation, and analysis of HT research. Nevertheless, we miss opportunities to contextualize, define, and explain the potential and limitations of HT methods. One evidence-based approach is to engage students in realistic HT case studies. HT cases immerse students with messy data, asking them to critically consider data analysis, experimental design, ethical implications, and HT technologies.The NSF HITS (High-throughput Discovery Science and Inquiry-based Case Studies for Today’s Students) Research Coordination Network in Undergraduate Biology Education seeks to improve student quantitative skills and participation in HT discovery. Researchers and instructors in the network learn about case pedagogy, HT technologies, publicly available datasets, and computational tools. Leveraging this training and interdisciplinary teamwork, HITS participants then create and implement HT cases. Our initial case collection has been used in >15 different courses at a variety of institutions engaging >600 students in HT discovery. We share here our rationale for engaging students in HT science, our HT cases, and network model to encourage other life science educators to join us and further develop and integrate HT complex datasets into curricula.
Introduction—A Call to Integrate High-Throughput Discovery Science Into Curricula
High-throughput (HT) approaches have become critical for contemporary scientific discovery. HT research often leverages the power of automation and miniaturization to make large-scale experimentation feasible and promote discovery. Advances in sequencing (McCombie et al., 2019), drug discovery (da Silva Rocha et al., 2019; Farha and Brown, 2019; Vamathevan et al., 2019; Zhu, 2020), imaging (Pegoraro and Misteli, 2017), proteomics (Tyers and Mann, 2003), machine learning and genome editing are transforming the way scientists perform research. HT approaches allow researchers to conduct multiple chemical, genetic, and phenotypic tests to identify active molecules, conditions, or genes with desired or useful features (Appleton et al., 2017). HT omics have enabled simultaneous examinations of hundreds or thousands of genes, proteins, and metabolites (Judes et al., 2016). Large NIH (Collins, 2010) and NSF initiatives (e.g., BREAD PHENO) also take advantage of HT methodologies (ENCODE, 2004; Regev et al., 2017; Hutter and Zenklusen, 2018; Koroshetz et al., 2018; McDonald et al., 2018). This fast-paced development and diversity of HT approaches necessitates effective training of scientists and engineers (Miller, 2014; Stephens et al., 2015; Batut et al., 2018). To better prepare students for this new HT research world, an understanding of HT approaches and dataset wrangling must be broadly integrated into curricula to empower all students to analyze and discover.
Variations in HT terminology may refer to a discipline (e.g., biology, genetics, bioinformatics), a technique (e.g., screening, sequencing), or a volume of data, but it is the data science and analysis skills that are more essential than ever for STEM students. For students to analyze and appreciate results, a general understanding of how data is validated and processed is required. Yet, undergraduate biology programs have been slow to adopt practices and curricula that enhance such quantitative skills (Feser et al., 2013; Hoffman et al., 2016). Educators have generated a multitude of reports and educational initiatives to fill this void (National Research Council, 2003; Bialek and Botstein, 2004; National Research Council, 2009). Despite the calls to action, there remains a relative dearth of educational resources that engage students with complex, HT datasets aimed at enhancing their quantitative skills (Aikens and Dolan, 2014). In part, this may reflect a lack of sufficient faculty training and a need for professional development and teaching support opportunities (AAAS, 2011). Since the design, execution, and analysis of HT data require fundamental quantitative skills, collectively this provides a ripe opportunity for faculty and students alike to expand these skills. Indeed, these skills align with the first two core competencies outlined by Vision and Change and the BioSkills Guide for revolutionizing undergraduate biology education (AAAS, 2011; Clemmons et al., 2020). Successful examples of undergraduate data mining and contribution to research (i.e., Genomics Education Partnership (Lopatto et al., 2014) and Genome Solver programs (Rosenwald et al., 2012) are becoming more common, and this integration of authentic research has a multitude of strongly supported student benefits (Lopatto et al., 2008; Thiry et al., 2011; Jordan et al., 2014; Shaffer et al., 2014).
High-Throughput Discovery Science and Inquiry-Based Case Studies for Today’s Students Network
We created the NSF-funded Research Coordination Network (RCN) known asHigh-throughput Discovery Science & Inquiry-based Case Studies for Today’s Students (HITS), to develop innovative curriculum materials using a case-based approach. RCNs have had a profound scholarly impact (Porter et al., 2012) and continue to generate and disseminate resources that improve research and education. Other RCNs have focused on the development of case studies (NeuroCaseNet, Molecular case Net (Goodsell et al., 2021), OCELOTS), bioinformatics (NIBLSE) or next generation sequencing (GCAT-SEEK). Additional educational initiatives (BEDROCK, ESTEEM, NUMB3R5 COUNT!, EDDIE) aim to increase student quantitative skills in biology but are not focused on case studies or high-throughput discovery science. HITS specifically addresses a unique need for case studies that provide exposure to and practice with manipulating and analyzing high-throughput datasets and understanding HT experimental approaches.
Case-based learning has been adopted and adapted into undergraduate and graduate education (Blosser, 1988; Gallagher et al., 1995; Krynock and Robb, 1996) and has become recognized as a high impact practice that supports Vision and Change core competencies (Lombardi, 2007; Freeman et al., 2014). According to the Vision and Change 2010 report (AAAS, 2011), all students need to develop the ability to apply the process of science, use quantitative reasoning skills, use models and simulations, understand the interdisciplinary nature of scientific investigations, and be able to communicate science well and in collaboration with other disciplines. Students also need to place their science knowledge in a societal context and learn to apply scientific concepts to real world problems. Case-based instructional methods, which include the case study method, problem-based learning, and investigative case-based learning, use realistic narratives to engage students in solving problems, building analytical skills, and working cooperatively in a self-directed way (Herreid, 1994; Torp and Sage, 2002; Duch et al., 2011). The complex nature of case studies leads students to assess problems from a myriad of perspectives and those that emphasize the human dimension of an issue or controversy powerfully demonstrate the relevance of a given topic to students and generate engagement (Yadav et al., 2007). Approaches to writing and adapting case studies have been previously well documented (Herreid, 1997; Herreid, 2018; Prud’homme-Generaux et al., 2018; https://sciencecases.lib.buffalo.edu/teaching/publications/).
Datasets produced by HT approaches contain untold stories about the biological world around us. Providing “scaffolded” cases for students to understand HT methodology, dive into data, and uncover trends promotes quantitative skills, hypothesis development, and the excitement for discovery. The HITS research and educational network provides structure and support for the development of HT case studies that promote student learning of core HT concepts and competencies. The resulting inquiry-based cases engage students in authentic research and better train future scientists for jobs in modern biology. Recently, our network shared insights on how to adapt HT cases for the classroom (Bixler et al., 2021). The HITS network also includes established non-profit and industry collaborations with researchers, institutes, and companies that produce datasets amenable for exemplary HT case studies. For example, The Allen Institute has worked with HITS to create case studies that use resources on the Allen Cell Explorer and Allen Brain Map websites to engage students in analysis of thousands of microscopy images of engineered cells and real electrophysiology data from human neurons. These datasets and equipment alone encompass several research areas and HT approaches, including sequencing, imaging, and screening. As HITS expands, additional collaborations and projects provide more datasets and resources to explore ways to connect students and courses at different institutions. By partnering researchers, educators, and case study experts to create, implement, and share HT case-based tools, our HITS network improves curricula across the nation and better equips life science students with essential science process skills and complex dataset literacy.
How High-Throughput Discovery Science and Inquiry-Based Case Studies for Today’s Students Cases Are Unique
HT case studies characteristically integrate large quantitative datasets with an engaging biological narrative to directly showcase the real-world relevance of course concepts. The unique features that make HT cases stand out include the combined focus on HT experimental approaches and contemporary techniques, the use of quantitative data analysis skills and computational tools, and showcasing the interdisciplinary nature of science. To ensure our cases capture the essence of HT discovery science, we crafted a set of learning objectives to ground our HT case study development (Supplementary Table S1). Our HT cases also often use publicly-available large, complex datasets to facilitate reproducibility studies (OSC, 2015) as well as authentic data mining (Hand, 2007). In addition to introducing students to databases/repositories and HT experimental design, a second focus of HT cases is to immerse students in the development of analytical skills (e.g., filter, analyze, and interpret data; synthesize data and draw appropriate inferences) with large, complex datasets.
Williams et al. (2019) identified that faculty perceive barriers to integrating computational and bioinformatics competencies involving HT “big data” into curricula. HITS helps lower these barriers by offering validated case studies that can facilitate the execution of competency-based learning outcomes (e.g., analytical skills). HT cases also address the barriers of limited instructor training and lack of HT technology on many campuses. The use of publicly available datasets, instructor collaboration with experts, detailed implementation notes, and the modular and adaptable nature of HITS cases make them easy to adopt or alter to meet specific curricular learning goals. HT cases are also well suited for use in a variety of modalities including interactive face-to-face classrooms, collaborative distance learning, and the laboratory environment (Bixler et al., 2021). HT cases in the laboratory setting can also provide learning resources for HT computational biology and bioinformatics-centric experiments. Over recent decades, computational biology has shifted from being a set of tools used to support biological studies to its own discipline that often drives research programs, with physical experiments and field research playing an equal or supporting role (Yanai and Chmielnicki, 2017; Tapprich et al., 2021). This interdisciplinary (e.g., life science, computer science, mathematics, statistics) nature of HT cases promotes multidisciplinary knowledge within the classroom, providing students with practice synthesizing ideas in the context of the collaborative nature of science. Here we share several examples of successful implementation of HT cases in a broad range of institutions and courses (Table 1) as well as our model for HT case development.
The High-Throughput Discovery Science and Inquiry-Based Case Studies for Today’s Students Network Model for High-Throughput Case Study Development
The goals of the HITS network (Figure 1; go.ncsu.edu/hits) are to…
• Organize interdisciplinary annual workshops, create a virtual community, and publish educational resources to foster HT research and its integration into the classroom.
• Create novel working groups of researchers and educators to design, assess, and disseminate much needed quantitative biology case studies based on HT discovery research.
• Build a diverse consortium of institutions committed to implementing these quantitative educational tools in biology courses and curricula across the country and world.
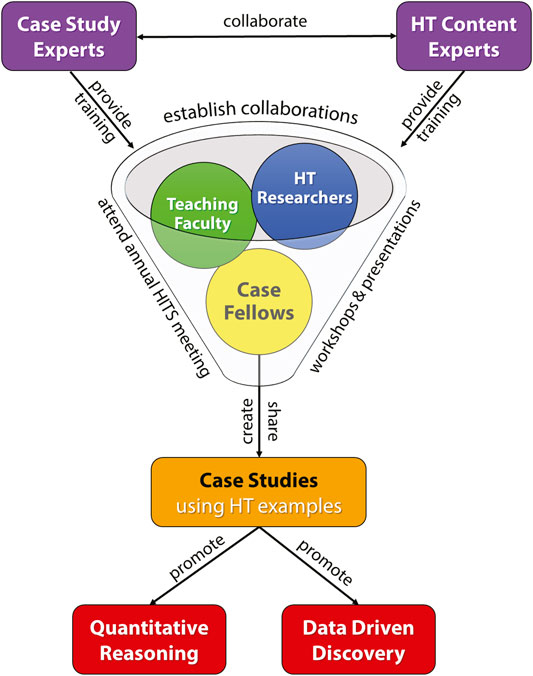
FIGURE 1. The HITS Network Model to integrate HT discovery into curricula. Case Fellows collaborate with educators and HT Researchers at the annual HITS meeting and within a virtual support community (funnel). They receive training from case Study Experts and HT Content Experts in order to gain the skills and knowledge to develop and disseminate novel HT teaching resources that promote data driven discovery and quantitative reasoning.
HITS case Fellows: As seen in Figure 1 and Supplementary Figure S1, the HITS network provides networking, research, and training opportunities for a diverse group of undergraduates, graduate students, postdoctoral fellows, researchers, and educators. Participants from a myriad of institutions and backgrounds interact at meetings, bringing creative energy and varied expertise to make HT discovery science, bioinformatics tools, case study design, and large, complex datasets accessible. Key among our participants are the HITS case Fellows. Given the barriers to integrating HT data into curricula (e.g., time, bioinformatics training, case study familiarity), HITS provides structured time, accountability, training, professional development, and financial support to case fellows who commit to creating and implementing HT case studies. We support at least 10 new HITS case Fellows each year by providing their HT case study training (i.e., 2 years of stipends and the opportunity to participate in two annual HITS meetings free of cost). In turn HITS fellows collaborate in teams to build modular and adaptable HT case studies for a variety of classroom environments (Table 1). Our case Fellows also commit to validating the newly created HT cases through cycles of assessment and revision. Once validated, dissemination of the HT cases for broader use in the educational community is a key goal. To recruit HITS case Fellows, each year we advertise widely primarily through the QUBES platform (Donovan et al., 2015) and our collective listservs and professional networks. We leverage attendance and participation at various national meetings (e.g., NIBLSE conference 2019; PANPBL 2019; NCCSTS 2018, BIOME 2018 and 2020). Now in our fourth year we’ve expanded to an international network that includes >80 members, and to date, we’ve supported >60 HITS participants from 34 institutions at our annual conference (Supplementary Figure S1).
HITS Annual Conference: The HITS conference occurs annually and has taken place both in person (2018 and 2019) and virtually due to the COVID-19 pandemic (2020 and 2021). The workshop’s three-day schedule has evolved over time but generally includes: 1) exposure to HT Discovery Science from research experts on day one, 2) interactive workshop sessions with case study experts and brainstorming of case ideas to facilitate team formation on day two, and 3) protected time for interdisciplinary teams to collaborate on cases on day three (Sample Schedule Supplementary Material 3). Key to the success of these workshop sessions is the active collaboration of HT researchers and case study development experts. HT scientists provide short talks and opportunities for case Fellows to learn about or work with large, complex HT datasets. To complement the HT training, pedagogical case study experts provide evidence-based best practices for case design and have participants work through exemplary case-based learning resources and tools.
Case Fellows participate in our HITS summer workshop twice, which allows them to first become familiar with HT research and case study development, and later gain confidence in their abilities to generate, implement, assess, and disseminate HT case studies. Given the conference format and their 2-year involvement, all fellows contribute to multiple cases. Indeed, interdisciplinary collaboration is key in the development of these HT case studies. Often, research experts familiar with the datasets or even responsible for generating them collaborate with classroom teachers to select and trim appropriate datasets as well as design case studies that can be implemented in a variety of courses. At the conference, case Fellows generate a list of HT topics which later narrows as teams are formed. Within each team tasks are distributed to lessen each instructor’s burden, ensuring the HT case is integrated into each classroom. Teams then have the opportunity to present at the conference and receive feedback from HT experts, other fellows, and case design experts. This piloting with both students and HITS participants alike enables rounds of revision to refine and validate the newly created HT case for assessment and broader dissemination, which includes student materials, detailed teaching notes, and in some cases cloud-based computing resources through QUBES.
The diverse perspectives among case Fellows ensures the development of cases that can be adapted to either introductory or advanced courses in a wide variety of topics (e.g., Neuroscience, Anatomy and Physiology, Genetics, Ecology, Biotechnology), broadening the impact of our network. During our conference there is also opportunity to discuss and address barriers to implementation (e.g., teaching load, class size, heterogeneity of student background and preparation). Modular design to enable adaptability for VERY different classrooms and virtual case Fellow support throughout the 2 years beyond the conference are key. This continued virtual collaboration to facilitate development, implementation, assessment, and dissemination is supported by QUBES (Donovan et al., 2015) and modeled by other successful networks (Ryder et al., 2020). Our HITS website allows us to track case study team progress and to feature examples of newly created HT case studies. Access to collaborative teams and the virtual HITS support network is essential to the success of each case Fellow and HITS participant. Indeed, the three-year evaluation of the program prepared by the NC State Office of Faculty Development found that 94% of prior year respondents published one or more cases since becoming a HITS case Fellow and 13% created ≥3.
HITS Steering Committee: HITS also relies on a senior leadership team composed of the PI, Co-PI and steering committee that analyzes report data to identify improvement ideas, suggests methods for network expansion, and promotes inclusivity. This team includes diverse faculty from all career levels and types of academic institutions (public and private, R1, primarily undergraduate, and minority serving institutions). The diversity of perspectives has enabled us to identify and adjust to major roadblocks in the development of the HITS network. Our steering committee has also shared their own HT research, led case study workshop sessions and interactive QUBES tutorials. Steering committee members work with individual teams of case fellows throughout each year to assist in continued virtual case study development, assessment and dissemination. The extensive networks of our steering committee team has also enabled us to coordinate with existing resources (ScienceCaseNet, QUBES, NIBLSE, BioQuest) to disseminate products and recruit interested faculty. The steering committee’s exemplary, strong, and diverse leadership is essential to meeting our call to integrate HT discovery science into life science education.
Discussion
Case studies based on authentic HT data present an untapped opportunity to engage students in the process of discovery while also teaching them fundamental quantitative reasoning skills. HITS has capitalized on the synergy between diverse HT researchers and educators to create such cases through interdisciplinary collaboration and professional development training opportunities at all levels. The accomplishments of HITS case Fellows are showcased in several publications as either open educational resources or as free resources with access to supplemental documents for a nominal fee depending on the outlet (Table 1). These cooperative teams leverage individual expertise to develop ambitious, often modular, cases for use across the nation. Our cases have immersed hundreds of students in cutting-edge HT-experimental approaches, which are transforming how biology research is conducted, all while ensuring students develop essential data literacy and quantitative skills. Such curricular reform establishes a shared community of ideas with themes that cross multi-disciplinary thresholds and incorporate our established shareholders.
Our network has developed a long-term sustainability plan to continue to generate high-impact, HT case studies. A growing awareness of HITS and our goals and sense of community are necessary to have a lasting impact (AAAS, 2011). In particular, this requires a clear identification of stakeholders, in our case, 1) HITS case Fellows (e.g., seasoned faculty, mid-career, new trainees) 2) researchers (e.g., science, technology, bioinformatics), 3) educators (undergraduate and graduate education) 4) curriculum specialists (educators, information technologists) and 5) partner organizations (e.g., HHMI, AAAS, SABER). Synergistic approaches with these stakeholders will widen the HITS aperture to support our organizational goals. Here are five key steps in our strategic plan to further integrate HT discovery science and bioinformatics skill development into life science curricula across the nation and internationally:
1. Recruit and develop enduring teams who commit to leveraging their interdisciplinary expertise in STEM-related fields and bioinformatics to integrate HT science into undergraduate and graduate curricula.
2. Identify and address barriers to implementing HT approaches in the classroom (e.g., faculty/student reluctance, technology, accessibility, language/terminology, perceptions).
3. Continue to create, validate, and share curriculum products that train other faculty and students in the acquisition, analysis, and discovery of the HT data sciences.
4. Encourage the diversity, equity, and inclusion of students and trainees in HT discovery science through the development of cutting-edge curricula that promote STEM retention and leadership of the next generation.
5. Expand the HITS network through broad dissemination of our goals and products as well as through strategic partnerships with key life science and bioinformatics educational communities.
Our network’s educational resources can be implemented in a variety of classrooms. Our HITS framework can also serve as a model for related fields with similar training bottlenecks to help create resources that address key gaps in current biology curricula. We also welcome collaboration to support other ongoing related initiatives (e.g., NIBLSE, NEUBIAS, NUMB3R5 COUNT!, EDDIE) and will continue to share products through publications, presentations, workshops, and open educational resources through QUBES. This will encourage adoption, adaptation, assessment, and creation of additional cases that use HT approaches and data to transform life science curricula and adequately equip our next generation of scientists with key quantitative and data science skills.
Permission to Reuse and Copyright
We created our figures and tables and are able to publish under the creative Commons CC-BY licence.
Data Availability Statement
Publicly available datasets were analyzed in this study. This data can be found here: go.ncsu.edu/hits.
Author Contributions
All authors collaborated to create the manuscript. CG and SR lead the HITS network and led the development and publication of this manuscript. AB, ME, MG-D, AK, PM, KO’T, and US are all HITS participants, and all contributed significantly to the writing and development of the manuscript including figure and table creation.
Funding
NSF HITS RCN network (NSF award 1730317).
Conflict of Interest
The authors declare that the research was conducted in the absence of any commercial or financial relationships that could be construed as a potential conflict of interest.
Publisher’s Note
All claims expressed in this article are solely those of the authors and do not necessarily represent those of their affiliated organizations, or those of the publisher, the editors and the reviewers. Any product that may be evaluated in this article, or claim that may be made by its manufacturer, is not guaranteed or endorsed by the publisher.
Acknowledgments
We appreciate the patience, energy, and wonderful ideas students provided. We the authors are members of the High-throughput Discovery Science and Inquiry-based case Studies for Today’s Students (HITS) RCN network (NSF award 1730317). Our goal is to raise awareness of the use of high-throughput approaches and datasets using case study pedagogies.
Supplementary Material
The Supplementary Material for this article can be found online at: https://www.frontiersin.org/articles/10.3389/feduc.2021.711512/full#supplementary-material
Supplementary Figure 1 | Distribution of HITS network across North America. The HITS network includes 77 participants from 35 institutions from 2018 to 2020. Each circle represents a unique academic, non-profit, or government institution. Size and color represent the number of participants from each institution. Represented institutions in alphabetical order: Adams State University, Alamance Community College, Allen Institute for Science, Barton College, Campbell University, Carroll University, Central Piedmont Community College, Clarke University, Colorado State University, Davenport University, Elon University, Emory University, Fordham University, Hartwick College, Hastings College, Heidelberg University, High Point University, Meredith College, Morehouse College, National Institute of Environmental Health Sciences (NIEHS), New School, North Carolina Agricultural and Technical State University, North Carolina State University, Northwestern Connecticut Community College, Saint Augustine University, Salem College, Telus World of Science, Trinity Washington University, United States Military Academy West Point, University of Dubuque, University of New Haven, University of North Carolina at Chapel Hill, University of North Carolina at Pembroke, University of Pittsburgh, and University of Richmond.
References
Aikens, M. L., and Dolan, E. L. (2014). Teaching Quantitative Biology: Goals, Assessments, and Resources. MBoC 25, 3478–3481. doi:10.1091/mbc.E14-06-1045
American Association for the Advancement of Science (2011). Vision and Change: A Call to Action. Washington, DC: Final report.
Appleton, E., Densmore, D., Madsen, C., and Roehner, N. (2017). Needs and Opportunities in Bio-Design Automation: Four Areas for Focus. Curr. Opin. Chem. Biol. 40, 111–118. doi:10.1016/j.cbpa.2017.08.005
Batut, B., Hiltemann, S., Bagnacani, A., Baker, D., Bhardwaj, V., Blank, C., et al. (2018). Community-driven Data Analysis Training for Biology. Cel Syst. 6, 752–758. doi:10.1016/j.cels.2018.05.012
Bialek, W., and Botstein, D. (2004). Introductory Science and Mathematics Education for 21st-Century Biologists. Science 303, 788–790. doi:10.1126/science.1095480
Bixler, A., Eslinger, M., Kleinschmit, A. J., Gaudier-Diaz, M. M., Sankar, U., Marsteller, P., et al. (2021). Three Steps to Adapt Case Studies for Synchronous and Asynchronous Online Learning†. J. Microbiol. Biol. Educ. 22, 22. doi:10.1128/jmbe.v22i1.2337
Blosser, P. E. (1988). Teaching Problem Solving--Secondary School Science ERIC/SMEAC Science Education Digest No. 2. Available at: https://eric.ed.gov/?id=ED309049 (Accessed May 14, 2021).
Clemmons, A. W., Timbrook, J., Herron, J. C., and Crowe, A. J. (2020). BioSkills Guide: Development and National Validation of a Tool for Interpreting theVision and ChangeCore Competencies. Life. Sci. Edu. 19, ar53. doi:10.1187/cbe.19-11-0259
Collins, F. S. (2010). Opportunities for Research and NIH. Science 327, 36–37. doi:10.1126/science.1185055
da Silva Rocha, S. F. L., Olanda, C. G., Fokoue, H. H., and Sant'Anna, C. M. R. (2019). Virtual Screening Techniques in Drug Discovery: Review and Recent Applications. Cur. Top. Med. Chem. 19, 1751–1767. doi:10.2174/1568026619666190816101948
Donovan, S., Eaton, C. D., Gower, S. T., Jenkins, K. P., LaMar, M. D., Poli, D., et al. (2015). QUBES: A Community Focused on Supporting Teaching and Learning in Quantitative Biology. Lett. Biomathematics 2, 46–55. doi:10.1080/23737867.2015.1049969
Duch, B., Groh, S., and Allen, D. (2011). The Power of Problem-Based Learning. Available: http://site.ebrary.com/id/11170657 (Accessed May 14, 2021) Dulles: Stylus Publishing. doi:10.2307/j.ctvt7x6h8
ENCODE (2004). The ENCODE (ENCyclopedia of DNA Elements) Project. Science 306, 636–640. doi:10.1126/science.1105136
Farha, M. A., and Brown, E. D. (2019). Drug Repurposing for Antimicrobial Discovery. Nat. Microbiol. 4, 565–577. doi:10.1038/s41564-019-0357-1
Feser, J., Vasaly, H., and Herrera, J. (2013). On the Edge of Mathematics and Biology Integration: Improving Quantitative Skills in Undergraduate Biology Education. Life. Sci. Edu. 12, 124–128. doi:10.1187/cbe.13-03-0057
Freeman, S., Eddy, S. L., McDonough, M., Smith, M. K., Okoroafor, N., Jordt, H., et al. (2014). Active Learning Increases Student Performance in Science, Engineering, and Mathematics. Proc. Natl. Acad. Sci. 111, 8410–8415. doi:10.1073/pnas.1319030111
Gallagher, S. A., Sher, B. T., Stepien, W. J., and Workman, D. (1995). Implementing Problem-Based Learning in Science Classrooms. Sch. Sci. Math. 95, 136–146. doi:10.1111/j.1949-8594.1995.tb15748.x
Goodsell, D. S., Dutta, S., Voigt, M., and Zardecki, C. (2021). Molecular Storytelling for Online Structural Biology Outreach and Education. Struct. Dyn. 8, 020401. doi:10.1063/4.0000077
Hand, D. J. (2007). Principles of Data Mining. Drug Safe. 30 (7), 621–622. doi:10.2165/00002018-200730070-00010
Herreid, C. F. (1994). Case Studies in Science--A Novel Method of Science Education. J. Coll. Sci. Teach. 23, 221–229. doi:10.2505/4/jcst18_048_02_34
Herreid, C. F. (2018). Exercises in Style: Is There a Best Way to Write a Case Study? J. Coll. Sci. Teach. 48 (2), 34–38. doi:10.2505/4/jcst18_048_02_34
Hoffman, K., Leupen, S., Dowell, K., Kephart, K., and Leips, J. (2016). Development and Assessment of Modules to Integrate Quantitative Skills in Introductory Biology Courses. Life. Sci. Edu. 15, ar14. doi:10.1187/cbe.15-09-0186
Hutter, C., and Zenklusen, J. C. (2018). The Cancer Genome Atlas: Creating Lasting Value beyond its Data. Cell 173, 283–285. doi:10.1016/j.cell.2018.03.042
Jordan, T. C., Burnett, S. H., Carson, S., Caruso, S. M., Clase, K., DeJong, R. J., et al. (2014). A Broadly Implementable Research Course in Phage Discovery and Genomics for First-Year Undergraduate Students. mBio 5, e01051. doi:10.1128/mBio.01051-13
Judes, G., Rifaï, K., Daures, M., Dubois, L., Bignon, Y.-J., Penault-Llorca, F., et al. (2016). High-throughput "Omics» Technologies: New Tools for the Study of Triple-Negative Breast Cancer. Cancer Lett. 382, 77–85. doi:10.1016/j.canlet.2016.03.001
Koroshetz, W., Gordon, J., Adams, A., Beckel-Mitchener, A., Churchill, J., Farber, G., et al. (2018). The State of the NIH BRAIN Initiative. J. Neurosci. 38, 6427–6438. doi:10.1523/JNEUROSCI.3174-17.2018
Krynock, K. B., and Robb, L. (1996). Is Problem-Based Learning a Problem for Your Curriculum? Ill. Sch. Res. Dev. 33, 21–24.
Lombardi, M. M. (2007). Authentic Learning for the 21st century: An Overview. Educause Learning Initiative 1, 1–12.
Lopatto, D., Alvarez, C., Barnard, D., Chandrasekaran, C., and Chung, H.-M. (2008). Undergraduate Research. Genomics Education Partnership. Science 322, 1684–12685. doi:10.1126/science.1165351
Lopatto, D., Hauser, C., Jones, C. J., Paetkau, D., Chandrasekaran, V., Dunbar, D., et al. (2014). A Central Support System Can Facilitate Implementation and Sustainability of a Classroom-Based Undergraduate Research Experience (CURE) in Genomics. Life. Sci. Eduse 13, 711–723. doi:10.1187/cbe.13-10-0200
McCombie, W. R., McPherson, J. D., and Mardis, E. R. (2019). Next-Generation Sequencing Technologies. Cold Spring Harb. Perspect. Med. 9, a036798. doi:10.1101/cshperspect.a036798
McDonald, D., Hyde, E., Debelius, J. W., Morton, J. T., Gonzalez, A., Ackermann, G., et al. (2018). American Gut: An Open Platform for Citizen Science Microbiome Research, mSystems 3, e000031. doi:10.1128/mSystems.00031-18
Miller, S. (2014). Collaborative Approaches Needed to Close the Big Data Skills gap. J. Organisation. Design. 3 (1), 26–30. doi:10.7146/jod.9823
National Research Council (2009). A New Biology for the 21st Century. Washington DC: National Academies Press. doi:10.17226/12764
National Research Council (2003). BIO2010: Transforming undergraduate education for future research biologists. Washington DC: National Academies Press.
Open Science Collaboration (2015). Estimating the Reproducibility of Psychological Science. Science 349, aac4716. doi:10.1126/science.aac4716
Pegoraro, G., and Misteli, T. (2017). High-throughput Imaging for the Discovery of Cellular Mechanisms of Disease. Trends Genet. 33, 604–615. doi:10.1016/j.tig.2017.06.005
Porter, A. L., Garner, J., and Crowl, T. (2012). Research Coordination Networks: Evidence of the Relationship between Funded Interdisciplinary Networking and Scholarly Impact. Bioscience 62 (3), 282–288. doi:10.1525/bio.2012.62.3.9
Prud’homme-Generaux, A., Schiller, N. A., Wild, J. H., and Herreid, C. F. (2018). Guidelines for Producing Videos to Accompany Flipped Cases. J. Coll. Sci. Teach. 46 (5), 40–48. doi:10.2505/4/jcst17_046_05_40
Regev, A., Teichmann, S. A., Lander, E. S., Amit, I., Benoist, C., Birney, E., et al. (2017). The Human Cell Atlas. eLife 6, e270401. doi:10.7554/eLife.27041
Rosenwald, A. G., Russell, J. S., and Arora, G. (2012). The Genome Solver Website: A Virtual Space Fostering High Impact Practices for Undergraduate Biology. J. Microbiol. Biol. Educ. 13, 188–190. doi:10.1128/jmbe.v13i2.444
Ryder, E. F., Morgan, W. R., Sierk, M., Donovan, S. S., Robertson, S. D., Orndorf, H. C., et al. (2020). Incubators: Building Community Networks and Developing Open Educational Resources to Integrate Bioinformatics into Life Science Education. Biochem. Mol. Biol. Educ. 48, 381–390. doi:10.1002/bmb.21387
Shaffer, C. D., Alvarez, C. J., Bednarski, A. E., Dunbar, D., Goodman, A. L., Reinke, C., et al. (2014). A Course-Based Research Experience: How Benefits Change with Increased Investment in Instructional Time. Life. Sci. Edu. 13, 111–130. doi:10.1187/cbe-13-08-0152
Stephens, Z. D., Lee, S. Y., Faghri, F., Campbell, R. H., Zhai, C., Efron, M. J., et al. (2015). Big Data: Astronomical or Genomical? PLOS Biol. 13, e1002195. doi:10.1371/journal.pbio.1002195
Tapprich, W. E., Reichart, L., Simon, D. M., Duncan, G., McClung, W., Grandgenett, N., et al. (2021). An Instructional Definition and Assessment Rubric for Bioinformatics Instruction. Biochem. Mol. Biol. Educ. 49, 38–45. doi:10.1002/bmb.21361
Thiry, H., Laursen, S. L., and Hunter, A.-B. (2011). What Experiences Help Students Become Scientists?: A Comparative Study of Research and Other Sources of Personal and Professional Gains for STEM Undergraduates. J. Higher Edu. 82, 357–388. doi:10.1080/00221546.2011.1177720910.1353/jhe.2011.0023
Torp, L., and Sage, S. (2002). Problems as Possibilities: Problem-Based Learning for K-16 Education. 2nd ed. Alexandria, Va: Association for Supervision and Curriculum Development.
Tyers, M., and Mann, M. (2003). From Genomics to Proteomics. Nature 422, 193–197. doi:10.1038/nature01510
Vamathevan, J., Clark, D., Czodrowski, P., Dunham, I., Ferran, E., Lee, G., et al. (2019). Applications of Machine Learning in Drug Discovery and Development. Nat. Rev. Drug Discov. 18, 463–477. doi:10.1038/s41573-019-0024-5
Williams, J. J., Drew, J. C., Galindo-Gonzalez, S., Robic, S., Dinsdale, E., Morgan, W. R., et al. (2019). Barriers to Integration of Bioinformatics into Undergraduate Life Sciences Education: A National Study of US Life Sciences Faculty Uncover Significant Barriers to Integrating Bioinformatics into Undergraduate Instruction. PLOS ONE 14, e0224288. doi:10.1371/journal.pone.0224288
Yadav, A., Lundeberg, M., DeSchryver, M., Dirkin, K., Schiller, N. A., Maier, K., et al. (2007). Teaching Science with Case Studies: A National Survey of Faculty Perceptions of the Benefits and Challenges of Using Cases. J. Coll. Sci. Teach. 37, 34–38.
Yanai, I., and Chmielnicki, E. (2017). Computational Biologists: Moving to the Driver's Seat. Genome Biol. 18, 223. doi:10.1186/s13059-017-1357-1
Keywords: high-throughput, collaborative network, data analyses, quantitative skills, pedagogical case studies
Citation: Robertson SD, Bixler A, Eslinger MR, Gaudier-Diaz MM, Kleinschmit AJ, Marsteller P, O’Toole KK, Sankar U and Goller CC (2021) HITS: Harnessing a Collaborative Training Network to Create Case Studies that Integrate High-Throughput, Complex Datasets into Curricula. Front. Educ. 6:711512. doi: 10.3389/feduc.2021.711512
Received: 18 May 2021; Accepted: 20 July 2021;
Published: 09 August 2021.
Edited by:
Hugo Verli, Federal University of Rio Grande do Sul, BrazilReviewed by:
Jean-Karim Hériché, European Molecular Biology Laboratory Heidelberg, GermanyMartin Leonard Jones, Francis Crick Institute, United Kingdom
Copyright © 2021 Robertson, Bixler, Eslinger, Gaudier-Diaz, Kleinschmit, Marsteller, O’Toole, Sankar and Goller. This is an open-access article distributed under the terms of the Creative Commons Attribution License (CC BY). The use, distribution or reproduction in other forums is permitted, provided the original author(s) and the copyright owner(s) are credited and that the original publication in this journal is cited, in accordance with accepted academic practice. No use, distribution or reproduction is permitted which does not comply with these terms.
*Correspondence: Carlos C. Goller, Y2Nnb2xsZXJAbmNzdS5lZHU=