- 1Medicine School, César Vallejo University, Lima, Perú
- 2Faculty of Psychology, Federico Villarreal National University, Lima, Perú
- 3Faculty of Medicine, Federico Villarreal National University, Lima, Perú
- 4Faculty of Education Sciences, San Cristóbal de Huamanga National University, Ayacucho, Perú
Educational researchers have become interested in the study of teaching and feedback processes as important factors for learning and realizing achievements in the teaching–learning context at the undergraduate and postgraduate levels. The main objective of this research was to assess the effect of five variables of the teacher’s didactic performance (two from teaching and three from feedback) on students’ variable of evaluation and application, mediated by their performances in participation, pertinent practice, and improvement. Participants were 309 Peruvian masters and doctoral students of an in-person postgraduate course in educational sciences who, due to the COVID-19 pandemic, were attending classes and submitting assignments and exercises online. The students were asked to fill two online questionnaires in Google Forms format regarding didactic performance; the first questionnaire comprehended five dimensions of teacher performance: explicitness of criteria, illustration, supervision of learning activities, feedback, and evaluation, whereas the second one encompassed four dimensions of student performance: illustration–participation, pertinent practice (adjustment to supervision of practices), feedback–improvement, and evaluation–application. When tested, two structural regression models showed (with good goodness-of-fit values) that the evaluation–application student performance factor was significantly and similarly predicted by the illustration–participation and feedback–improvement student performance variables, and, to a lesser extent, yet significantly, by the pertinent practice student performance. Moreover, teacher performances had a significant effect with high regression coefficients on the three student performance variables included as mediators, both when the five teacher performance variables were included as predictor variables, and when arranged into two second-order factors (teaching and feedback).
1 Introduction
Educational research has focused on studying the teaching and feedback processes seen from different standpoints, with a major emphasis on the in-person modality. Numerous methods have been described in the past to assess teaching from the students’ outlook (Borges et al., 2016; Gitomer, 2018; Joyce et al., 2018; Aidoo and Shengquan, 2021), as well as to evaluate the feedback provided by teachers in terms of their own students’ performance (Jellicoe and Forsythe, 2019; Krijgsman et al., 2019; Winstone et al., 2019; Wisniewski et al., 2020; Krijgsman et al., 2021). The correlation between the teacher’s and the students’ performances during didactic interactions has also been an object of study (Peralta and Roselli, 2015; Velarde and Bazán, 2019; Kozuh and Maksimovic, 2020).
Some measurements having been developed and validated regarding the students’ self-assessment of their knowledge, skills, and competencies according to the academic programs they attended (Pop and Khampirat, 2019; Sarkar et al., 2021). But, little has been studied about the self-perception of students in terms of their own performances as part of their didactic interaction.
The COVID-19 pandemic, a disease caused by the SARS-CoV-2 virus, and the subsequent shutdown of in-person education programs around the world brought virtual teaching–learning to the forefront in order to preserve the educational processes at all levels. Hence, the conventional in-person teaching and learning activities in the university context were transformed into virtual processes. Also, the teachers of these academic programs were required to adapt their planning, teaching strategies and competencies, teaching tools and materials, and their ways of supervising, assessing, and providing feedback on the learning process and performance of their students (George, 2020; Loose and Ryan 2020; Thomas, 2020; Sumer et al., 2021).
Some of the teachers’ actions focused on adapting didactic strategies and traditional lessons into online activities (Howe and Watson, 2021), as well as on innovation of educational goals and processes, and management changes (Nugroho et al., 2021). These new ways of teaching and providing students with feedback in a virtual environment also meant having to adapt the programs and teaching strategies for the in-person undergraduate and postgraduate programs and testing them experimentally to apply them in the online learning environment (Abrahamsson and Dávila López, 2021; Rehman and Fatima, 2021).
Despite the growing interest in the study of how students perceive learning conditions in virtual environments for academic programs that used to be essentially in-person before the pandemic, there is a need to develop more studies on the teacher–student interaction processes during virtual classes. According to Prince et al. (2020), there are no critical differences between the research-proven best teaching practices for in-person sessions and those for virtual classes; however, online classes in the context of the pandemic come with greater technical challenges and interpersonal innovations for the professors. “Online classes particularly benefit from explicit instructional objectives, detailed grading rubrics, frequent formative assessments that clarify what good performance is, and above all, clear expectations for active student engagement and strategies to achieve it” (Prince et al., 2020, p. 18).
Based on the perceptions and self-assessments from educational sciences postgraduate students, this study examines the effect of the didactic performance and teacher assessment categories (teaching and feedback) on student performance variables (learning–participation, pertinent–practice, improvement–feedback, and application of the acquired knowledge) as self-assessed by the students.
1.1 Teaching and Feedback in the Students’ Learning
One fundamental aspect to understanding learning processes—especially in school-based learning—is the term “teaching.” In the assessments of non-cognitive variables of the Programme for International Student Assessment (PISA) applied by the Organization for Economic Cooperation and Development (OECD), teaching has been envisaged as a two-part concept: one part focusing on the behaviors of professors during the teaching practice, and the other related to the quality of teaching or instruction (Organization for Economic Cooperation and Development, OECD, 2013; Organization for Economic Cooperation and Development. OECD, 2017). According to the PISA tests, three general dimensions have been identified in the case of teaching practice behaviors: 1. teacher-led instruction: teacher behaviors for class structuring, defining objectives, monitoring the progress of the students, and modeling the skills to be developed by the latter; 2. formative assessment: behaviors of progressive feedback to their students, providing information about their weaknesses and strengths, fostering reflection on their expectations, goals, and progress throughout the learning process; 3. student orientation: behaviors of the teacher focused on the traits and needs of each student to advance through the contents and guarantee the acquisition of knowledge (Bazán-Ramírez et al., 2021b).
A recent systematic review research effort reported by Aidoo and Shengquan (2021) draws a distinction between the terms “teaching” and “teaching quality.” Nevertheless, in order to identify teaching, some of the authors referenced by Aiddo and Shengauan observe actions related to both teacher’s behavior during class (teaching), and instruction capacity circumstances (teaching quality) (Klieme et al., 2009; Bell et al., 2012; Hafen et al., 2014; Grosse et al., 2017; Fischer et al., 2019). When referring to teaching quality, characteristics such as emotional support, instructional support, classroom organization, type of cognitive demand, and class organization and management are described, among others (Gitomer et al., 2014; Joyce et al., 2018; Liu and Cohen, 2021). On the contrary, when teaching is described as forms of teacher behavior in teaching situations, it refers to the actions deployed by the teacher during the didactic interaction to promote learning in their students (Kaplan and Owings, 2001), and has to do with the teacher’s practices—for example, behavioral tendencies—in instruction and assessment during the learning processes (Bell et al., 2012; Organization for Economic Cooperation and Development, OECD, 2013; Serbati et al., 2020; Liu and Cohen, 2021).
As pointed out by Prince et al. (2020), synchronous and asynchronous didactic interactions involve teaching didactic performances (criteria explicitness, activities, and expectations regarding the students’ active participation and subsequent reflection), the implementation of various synchronous and asynchronous activities of comprehensive formative assessment, and drawing on results to perform continuous improvements in the implementation of learning activities. It can be considered that the professor’s teaching actions (e.g., in doing so, explaining class achievement criteria, exemplifying or presenting contents, or structuring various student–learning content interaction levels) are closely related to teaching feedback, supervision, feedback itself, and the application of acquired knowledge (evaluation).
The teacher, as part of the educational environment, creates the circumstances and conditions to foster the development of complex skills and behaviors, experiences and opportunities to leverage learning, and the academic development of their students (Kantor, 1975). As Dees et al. (2007) pointed out, teaching is a complex encounter of the human experience, characterized by a teacher–student dialog, in which the role of the teacher is essential to generate a favorable environment for learning. That learning environment also includes the behaviors of the teacher and the student body, as well as evaluation activities. Teaching and feedback can be seen as two multi-element concepts embedded in the same educational process between the teacher’s performances and the students’ performances with respect to the teaching and learning topics and contents in educational and psychological events as integrated fields (Hayes and Fryling, 2018).
On the other hand, several studies relying on student self-reports for in-person or virtual sessions have been conducted recently on the predictability correlation or implications between the feedback received and the academic performance of students, as well as related factors, for example, the content of the acquired knowledge, the explicitness of learning objectives, the student’s satisfaction, the illustration of skills, the psychological traits and expectations from students, and feedback implementation processes (Forsythe and Jellicoe, 2018; Jellicoe and Forsythe, 2019; Krijgsman et al., 2019; Winstone et al., 2019; Wisniewski et al., 2020; Gopal et al., 2021; Krijgsman et al., 2021; Wang et al., 2021).
The work of Forsythe and Jellicoe (2018) allowed for definition of some types and characteristics of a feedback process in higher education contexts and had some relevant constructs validated: the positive or negative value of the feedback message; the perceived legitimacy of the feedback message; the credibility of the feedback source and trust in the bearer; and challenge interventions to guide the student to balance their progress positively and work on their deficiencies and weaknesses. The authors conclude that the self-regulation of student behaviors and predicting them predict, in turn, feedback engagement and behavioral changes.
A meta-analysis study by Wisniewski et al. (2020) on 435 research articles revealed an approximate average effect of feedback on the students’ academic achievements (d = 0.48). However, their results showed that such impact is significantly influenced by the content of the information conveyed, that is, the different types and that feedback has a greater impact on outcomes related to cognitive and motor skills and a lesser impact on motivational and behavioral results. One aspect to highlight in the conclusion of Wisniewski et al. (2020) is that “feedback must be recognized as a complex and differentiated construct that includes many different forms with, at times, quite different effects on student learning” (p. 13). And, indeed, these different types of feedback and their contents can also be related to the different moments and performance criteria throughout the didactic interaction processes, which involve performances from both the teacher and the student.
The importance of the relationship between the teaching and feedback processes can be found in the articles reported by Krijgsman et al. (2019), Krijgsman et al. (2021), who used different methods to study the effect of the explicitness of learning and achievement objectives, and feedback processes on student satisfaction and academic achievement. One first research effort based on six different measurements over time revealed that students experienced more competence, autonomy, and relatedness satisfaction during class when sensing increased process feedback or explicitness of learning objectives, but no significant correlation was found between process feedback and explicitness of objectives (Krijgsman et al., 2019).
For a second quasi-experimental study with students of sport pedagogy and education, Krijgsman et al. (2021) used a 2 × 2 factorial design (absence vs. presence of explicitness of objectives and absence vs. presence of process feedback) to learn the effect of these two variables on the satisfaction of students regarding their need for competence, autonomy, and relatedness. The authors concluded that under certain conditions, the didactic processes (classes) can be perceived by the students as highly satisfactory, even when the teacher has not clarified what the learning objectives and achievement criteria are and/or has not provided feedback on the process.
The perception of feedback processes, acceptance, engagement, and participation in feedback processes, motivation, and awareness to engage in feedback to improve learning have been studied as well (Jellicoe and Forsythe, 2019; Winstone et al., 2019). Following the prior validation of measurement constructs of five characteristics associated with feedback, Jellicoe and Forsythe (2019) confirmed the structural and progressive effect between the students’ acceptance of feedback, awareness of feedback, motivated intentions related to feedback, behavioral changes, and the execution of actions in response to feedback using structural equation modeling on two groups of psychology students.
Embracing the outlook of students receiving appropriate feedback and the importance of building a feedback culture in psychology students (feedback literacy), Winstone et al. (2019) conducted a three-stage study with three different methods, concluding with a feedback workshop. They gathered information on the perceived usefulness of Developing Engagement with a Feedback Toolkit (DEFT) to support the development of the students’ feedback literacy skills and found that students perceived DEFT favorably and that such resources can improve students’ overall feedback literacy.
Studies on the relationship between in-class modeling and the presentation of content during class have also been reported, with feedback processes provided by the teachers. Blumenfeld et al. (2020) conducted a study with medical students and found that teaching basic surgical skills with online didactic videos and deliberate and supervised practice sessions, while providing feedback, led to 100% of students who did not have such skills prior to the intervention actually mastering the corresponding technique, according to a follow-up assessment.
Through a structural equation modeling study conducted during the COVID-19 pandemic with business administration and hotel administration students from India, Gopal et al. (2021) found that the instructor’s quality, course design, prompt feedback, and the students’ expectations had a positive and significant impact on student satisfaction. Likewise, higher student satisfaction had a positive impact on student performance. Similarly, Wang et al. (2021) carried out a study with Chinese university students and found that teacher’s innovation during class was perceived as a factor significantly influencing academic achievements or results and satisfaction in virtual classes during the pandemic. However, academic achievements and learning satisfaction were negatively correlated with the instructor’s performance. It is worth mentioning that the students’ academic self-efficacy was a significant mediator in terms of the effect of instructional support (including feedback actions) and teacher innovation on their perceived learning outcomes and learning satisfaction.
1.2 Current Study
In this work, we sought to answer the question: what is the influence of the variables teaching and feedback as factors of didactic performance of the teacher on the performance of the student body in the performance variable evaluation and application by the student body, mediated by the student body variables: participation, relevant practice, and improvement? Derived from this research, the objective of this study is to show the effect of the teacher’s didactic performance variables (teaching and feedback) perceived by educational sciences postgraduate students attending virtual classes during the pandemic on their self-assessment of their performance in evaluation and application, mediated by their performance in participation, relevant practice, and improvement.
In order to respond to the problem presented, an explanatory theoretical model was proposed, as shown in Figure 1. The model postulates that student performance in evaluation–application, referred to the exercise of self-evaluation and transfer of knowledge and competences achieved to the solution of new problems with effectiveness and variability (Ribes, 1990; Carpio and Irigoyen, 2005), is indirectly regulated by the teaching and feedback factors that correspond to teacher performance, and directly affected by three latent factors that correspond to student performance (illustration–participation, pertinent practice, and feedback–improvement).
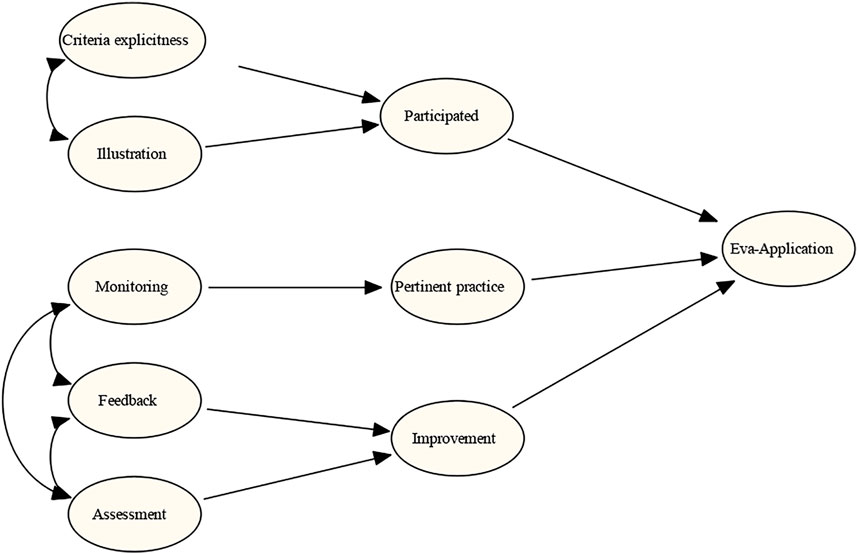
FIGURE 1. Theoretical model to explain didactic performances of the teacher and the student. Note. Teaching performance variables: criteria explicitness and illustration. Performance variables of teacher feedback: monitoring (practice supervision), feedback, and assessment. Student performance variables: illustration–participated, pertinent practice, feedback–improvement, and evaluation–application.
In the first aspect, the model posed two factors of teacher didactic performance: teaching and feedback. The teaching factor included two latent variables of teacher performance: explicitness and illustration, and the feedback factor included three latent variables of teacher performance: supervision of learning activities, feedback, and evaluation. These five criteria of teacher performance stem from the didactic performance model in the context of the teaching of psychology and related disciplines (Carpio, et al., 1998; Irigoyen et al., 2011; Silva, et al., 2014; Velarde and Bazán, 2019; Bazán-Ramírez et al., 2021b).
Also, this model included four latent student performance variables as correspondence with the didactic performance variables of the teacher: St evaluation–application, St illustration–participation (which is functionally related to the teaching factor of teacher performance), St practice, and St feedback–improvement (which are functionally related to the feedback factor of teacher performance). These student performance categories were derived from the didactic performance model disciplines (Carpio et al., 1998; Irigoyen et al., 2011; Silva et al., 2014) and previously validated (Morales et al., 2017; Velarde and Bazán, 2019; Bazán and Velarde, 2021).
Each of these teacher performance factors are briefly defined hereunder:
Criteria explicitness: This didactic performance implies having the teachers point out to their students at the beginning of a term, class, or practice what goal they are expected to reach and what performance criteria and parameters they must fulfill to achieve the expected objective.
Illustration: The teachers present the models and ways of working, as well as the strategies to identify problems and search for remedial tactics under a substantive theory conceptually and methodologically guiding the actions of the competency that is intended to be shaped in the students.
These two performances are related to teaching itself; teachers may establish certain conditions and procedures for teaching–learning depending on the achievements that they want their students to realize, to which end they establish criteria and requirements for compliance while using examples and the didactic discourse in order to shape the behavior or behaviors that they want their students to develop and apply.
Supervision of practice: The teacher brings the student into contact with conditions similar to those that they will face in their professional and/or scientific career and guides the actions of their students through monitoring and support devices and channels (oversees the actions of their students).
Feedback: It implies the assessment of both the learning process and the student’s subsequent performance according to their achievements and the established criteria. The teacher provides the student with an opportunity to ponder on how they have been carrying out the activities, and together they look for new ways to reach the expected objectives and achievements.
Evaluation: It involves activities and conditions made available to the teacher to evaluate the functional adjustment of the student’s performance according to the expected achievement and the previously explained criteria. These criteria also include the assessment of the application of the acquired knowledge when facing new problems and/or the use of earlier learning in new situations, different from those experienced during the learning process.
These three types of teacher performance are related to feedback as a general category within the learning process. According to Silva et al. (2014), the criteria under which the students’ performance is monitored and given feedback come from the original didactic plan and its learning objectives, without which the students’ performances could not be monitored or given feedback. While it is true that evaluations have been traditionally seen as summative mechanisms for assessing student learnings, they also lead to opportunities to reflect on student performance and to make modifications and adjustments to the learning objectives and the conditions of subsequent didactic interactions.
Moreover, in this first case related to the validation of measurements, the four types or categories of the students’ didactic performance underwent the same procedure. Four didactic performance categories of the educational sciences postgraduate students were addressed in this research: 1. Illustration–participation, 2. pertinent practice (adjustment to supervision of practices), 3. feedback–improvement, and 4. evaluation–application.
The following is a brief description of each of these four types of student performance according to the teacher’s performance.
Illustration–Participation: In terms of this didactic performance area, Bazán and Velarde (2021) pointed out that the student acts according to the criteria indicated by the teacher based on the didactic requirements and according to the appropriate linguistic mode. Morales et al. (2017) stated that this area involves both participation in class according to the criteria set forth by the teacher during class and the student’s utilization of strategies and resources to facilitate learning and actually learn action patterns specified in the syllabus or during class.
Pertinent practice: This area implies that the student’s performance adapts to the achievement criteria specified to realize the expected achievement(s) when facing practical situations similar to the exercise of competencies according to the pursued academic level or grade (under the supervision and guidance of the teacher). In this regard, Morales et al. (2017) pointed out that this practice “… is embedded within the achievement criterion in accordance with what, how, when, and where such practice can be supervised at all times as a form of permanent evaluation or in isolation as a test situation to demonstrate an already acquired way of behaving” (p. 31).
Feedback–Improvement: The student is brought into contact with their own performance and makes changes to their behavior according to the criteria and observations made by the teacher or by themselves (Velarde and Bazán, 2019; Bazán and Velarde, 2021). Likewise, according to Morales et al. (2017), the process of assessing the development of skills and knowledge to realize the expected achievements also implies the self-supervision (or monitoring) of the student’s own performance in accordance with the said expected achievements or learnings.
Evaluation–Application: The students perform activities, solve problems, and implement procedures according to the expected achievement, fulfilling effectiveness, and variability criteria (Velarde and Bazán, 2019; Bazán and Velarde, 2021). A noteworthy characteristic is that students apply the skills and knowledge that they have developed when facing new demands and new ways of encountering problems. In addition, this performance area relates to self-assessment as a comparison established by the student between their actual performance and their ideal performance, and the adaptation of knowledge to other situations (Morales et al., 2017).
The first type of didactic performance of the students (illustration–participation) relates to the students’ own learning process performances during a didactic interaction. These activities involve addressing and adjusting to the cognitive demands posed by the tasks and criteria set forth by the teacher. The other three student performances (pertinent practice, feedback–improvement, and evaluation–application) have to do with the students adjusting to formative assessment activities, supervision, feedback, and evaluation.
2 Methodology
2.1 Participants
Participants in this research were masters and doctoral students enrolled in the Postgraduate Program of the Faculty of Educational Sciences of a national university from the Peruvian mid-southern highlands. Originally intended as in-person curricula, the postgraduate program has been offered exclusively online since June 2020 as a result of the SARS-CoV-2 virus pandemic by means of video conferences using an institutional account of this university on Google Classroom and Google Meet. The student population is composed of active teachers in the elementary education system (private and public) and/or professors from different universities. Almost all students come from the state (region) where the university in which this study was conducted is located.
In this postgraduate program, the master’s degree curriculum is taught in four-month cycles (one course per month), whereas the doctorate level is scheduled in six-month cycles (with six-week courses). A modular system of four courses taught sequentially is in place for both academic levels; when a given course has been completed and the corresponding final grade reports have been submitted, the next course begins, and so forth. In the case of Peru, the master’s and doctoral programs do not differ substantially in terms of student training, as is the case in other countries, where the doctorate is essentially a research program oriented to the generation of basic knowledge.
This study was conducted at the end of the first course from both levels. Following the completion of the first course of the academic cycle 2020–2021 (between mid-July and early August 2020), all postgraduate students (approximately 500 masters and doctoral students) were invited via institutional mail to participate in this study, being informed of its voluntary nature and free of any conditions or consequences for students who did not wish to participate, and of the benefits that the study might bring to the academic program. A total of 309 students who agreed to participate in this research received textual information and a consent form to be filled electronically; all 309 students sent back their informed consent form filled accordingly.
The final sample of 309 students consisted of 198 males and 111 females; 222 (72%) were pursuing a master’s degree, and 87 (28%) a PhD. Ages ranged between 23 and 71 years old, with a mean age of 35. Table 1 shows the number and percentage of students from each cycle and level.
2.2 Design
This research had a cross-sectional design using short student self-reporting perception and self-perception scales following a quantitative and predictive approach of relationships between factors and variables included through structural equation modeling (SEM).
2.3 Measurements
2.3.1 Teacher Performance Questionnaire
A self-reporting questionnaire comprising five scales with four items each was applied in order to gather information on how students perceive their teacher’s performance. Two subscales or dimensions were used to evaluate the teacher’s performance in terms of teaching: criteria explicitness and illustration, whereas three subscales were used to evaluate feedback: supervision of practice, feedback, and evaluation, each of them scored using a Likert scale: 0 = never, 1 = sometimes, 2 = almost always, and 3 = always. Except for the subscale to evaluate supervision of practice, four subscales corresponding to four teaching performance criteria from the self-reporting questionnaire validated by Bazán-Ramírez et al. (2021b) were adapted by a panel of education postgraduate teachers to be applied in the Peruvian context.
A total of fifteen questions were adapted; in some cases, the adaptation consisted of modifying several sentences without disregarding the original sense of the question but making it specific to the current context. A subscale with four questions based on the same evaluation format as the other four performance areas was drafted to evaluate the supervision of practice performance area, for example: “In terms of the practical activities of the course, the teacher oversaw our activities and corrected our performance.” Table 2 shows the questions or statements from each of the five subscales (criteria) of the teacher’s didactic performance. For obtaining the Spanish version of these questions, see the Supplementary Material S1 section.
For this research, a confirmatory factor analysis was used to identify the internal structure of the teacher performance questionnaire, obtaining results with very good global fitness values, almost similar for the multidimensional model [χ2 (160) = 279.864, CFI = 0.988, TLI = 0.986, RMSEA = 0.049 (0.040, 0.059), SRMR = 0.048] and for the second-order factor model [χ2 (164) = 295.020, CFI = 0.987, TLI = 0.985, RMSEA = 051 (0.042, 0.060), SRMR = 0.051], in which the first-order factors criteria explicitness and illustration were contained by the second-order factor (teaching category), whereas the other first-order factors (supervision of practice and learning activities, feedback, and evaluation) were a part of the second-order factor feedback category. Standardized factorial loads for the multidimensional model ranged between 0.62 and 0.77, with covariances ranging from 0.73 to 0.90; in terms of the other model, the second-order factors showed a covariance of 0.95, whereas the correlations between second- and first-order factors varied between 0.89 and 0.93; in addition, factorial loads ranged between 0.62 and 0.77. In summary, satisfactory validity evidence was obtained based on the internal structure of the construct for the questionnaire scores, both for the five–oblique factor multidimensional model and for the second-order multifactor model.
Although these data showed that we have an instrument with good convergent and divergent construct validity, the questionnaire underwent invariance analysis according to sex, age, and postgraduate level of the students. Results in Table 3 reveal that the teacher’s performance questionnaire is invariant in terms of sex, age, and pursued degree (master’s or PhD). Implemented gradual restrictions did not impair the fitness of the models examined for the metric, scalar, and strict invariance levels as the differences found in the suggested fitness indices (∆CFI < −0.01 and ΔRMSEA <0.015) are within the permissible threshold (Cheung and Rensvold, 2002; Chen, 2007; Dimitrov, 2010). Therefore, according to the data found, it should be noted that students from all sociodemographic groups understood the meaning of the teacher’s performance latent construct equally, with unbiased indicators that are measured with the same precision in each group.
Given the ordinal nature of the items, reliability was estimated using McDonald’s Omega, with coefficients varying between 0.79 and 0.93 for the factors, whereas an Omega value of 0.96 and an H value of 0.96 were obtained for the general construct. These values indicate high reliability for the instrument scores.
2.3.2 Student Didactic Performance Scales
Four categories or criteria (illustration–participation, pertinent practice, feedback–improvement, and evaluation–application) of the students’ didactic performance were assessed using four short subscales, one for each performance. In each area, the short scale consisted of four sentences (items) whose responses were rated on a scale of 0 = never, 1 = sometimes, 2 = almost always, and 3 = always.
To evaluate the students’ self-assessment of their performance, in accordance with the didactic performance model assumed in this work, eleven questions were adapted from the questionnaire validated by Bazán and Velarde (2021) and grouped into three scales: illustration–participation, feedback–improvement, and evaluation–application. The adaptation consisted of a panel of teachers from the educational sciences postgraduate program adjusting several questions for better understanding by educational sciences students from Peru, adding a new question in the illustration–participation area: “I would solve a complex problem relevant to the education field, as close as possible to how an expert with a postgraduate degree would do it.”
Four additional questions were devised for the scale that would evaluate the pertinent practice student performance criterion, which corresponds to the supervision of practice (and learning activities) teaching performance criterion; an example of this is the sentence “During the practices, I carried out my activities under the guidance and supervision of the teacher.” Table 4 shows the questions for evaluating the four student performance criteria. Also, a Spanish version of these questions is available in the Supplementary Material S1 section.
A confirmatory factor analysis was conducted to prove the factorial structure of the scales of the students’ didactic performance, yielding very good fitness values for the multidimensional model: χ2 (84) = 204.747, CFI = 0.964, TLI = 0.955, RMSEA = 0.069 (0.057, 0.081), SRMR = 0.075. Standardized factorial loads ranged between 0.56 and 0.75, except for the item “My final evaluation has shown that I have acquired the expected knowledge and competencies according to the objectives set forth at the beginning of the term and which can be found in the syllabus” with a load of 0.30, and which was kept within the dimension to avoid impacting the factorial structure; covariances ranged between 0.66 and 0.84. The global fitness analysis reveals satisfactory validity evidence based on the internal structure of the construct. The internal consistency coefficients, estimated using McDonald’s Omega, range between 0.70 and 0.73, with omega and H values of 0.92 for the general latent variable.
Having identified the factorial structure of the scales of the students’ didactic performance, the measurement invariance according to sex, age, and pursued degree (master’s or PhD) was analyzed progressively. As can be seen in Table 5, fitness indices CFI and RMSEA reveal a very good fitness value for configurational variance. In terms of metric variance, fitness indices are among the expected thresholds, confirming the invariance (ΔCFI > −0.01 and ΔRMSEA <0.015), thus indicating that the latent construct has the same meaning across all groups. Regarding scalar invariance, the differences based on ΔCFI and ΔRMSEA with respect to the metric invariance remain within the recommended values, suggesting that students can obtain equivalent scores regardless of demographic features such as sex, age, and pursued degree. Last, because the suggested indices ∆CFI and ΔRMSEA are within the recommended cutoff threshold, strict invariance was deemed satisfactory as well; this means that the instrument items are measured with the same precision in each group.
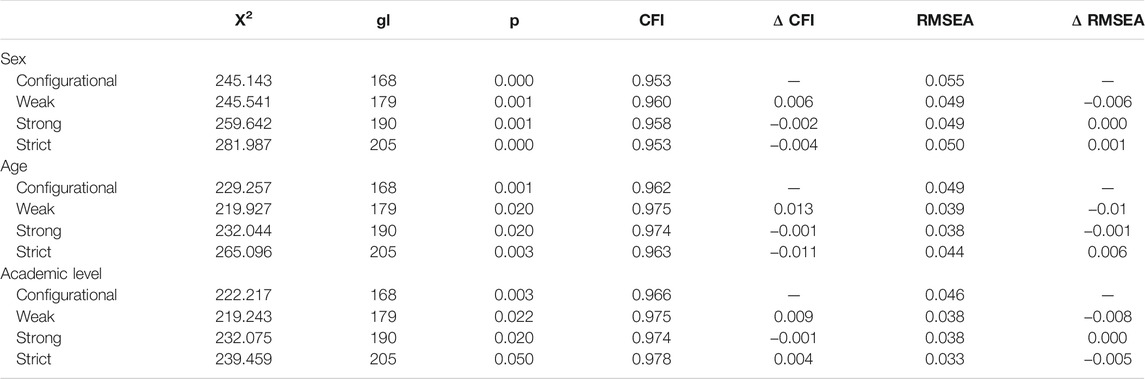
TABLE 5. Invariance according to sex, age, and postgraduate level of the scales of the students’ didactic performance.
2.4 Procedure and Data Analysis
The two measurement instruments were inspected psychometrically during the first stage. Validity evidence based on the internal structure and measurement invariances was examined through confirmatory factor analysis and using R software version 4.0.2 (R Development Core Team, 2020) respectively; because of the ordinal nature of the items that configured the multidimensional and second-order models, the WLSMV estimator (diagonally weighted least squares with mean and variance corrected) and polychoric correlation matrices (Brown, 2015; Li, 2016) were used. In addition to χ2 (chi-square), traditional indices such as comparative fit index (CFI), Tucker Lewis Index (TLI), root mean square error of approximation (RMSEA), and standardized root mean square residual (SRMR) were considered for the assessment of global fitness values of the models; a CFI and a TLI ≥0.90 or ≥0.95 mean either adequate or good fitness, respectively (Hu and Bentler, 1999; Keith, 2019), for RMSEA, indices ≤0.08 or ≤0.05 mean adequate or good fitness, respectively (Hu and Bentler, 1999; Keith, 2019); similarly, SRMR values ≤0.08 or ≤0.06 indicate adequate and good fitness, respectively (Hu and Bentler, 1999; Keith, 2019).
During the second stage, based on the theoretical model presented in Figure 1, two structural regression models were tested through structural equation modeling, using the robust maximum likelihood estimation method (MLM) and the semTools 0.5-3 and lavaan 0.6-7 packages in R software environment version 4.0.2 (Yuan and Bentler, 2000; Finney and DiStefano, 2008; Satorra and Bentler, 2010). In the first model, the five latent variables of the teacher’s didactic performance were directly included as predictor variables, covarying in the foreground the two variables of the teaching factor (criteria explicitness, illustration) and, on the other hand, the three feedback variables (supervision of practice, feedback, and evaluation). In the second model, the two second-order factors, teaching, feedback, and their respective categories as first-order variables, were included as predictors. The cutoff values suggested by Keith (2019) and Schumacker and Lomax (2016) were considered for fitness validation of the models; in this sense, the examined goodness-of-fit indices were Satorra–Bentler scaled chi-square (S-B χ2), CFI, TLI, RMSEA, and SRMR.
3 Results
The proposed hypothetical model is supported by empirical evidence, given that both the global goodness-of-fit indices of the model and the estimated parameters for the established relationships are satisfactory (Figure 2) having obtained goodness-of-fit indicators S-B χ2 (539) = 859.032, p = 0.000; CFI = 0.931; TLI = 0.923; IFI = 0.931; RMSEA = 0.044 (0.039, 0.049); SRMR = 0.065; that is, results reveal that the SEM model has an adequate parsimony measurement (S-B χ2/gl = 1.59), good fit incremental fitness (CFI and TLI); by showing good fitness values, absolute fitness indices such as RMSEA and SRMR also reveal a minimum error presence when replicating the empirical model.
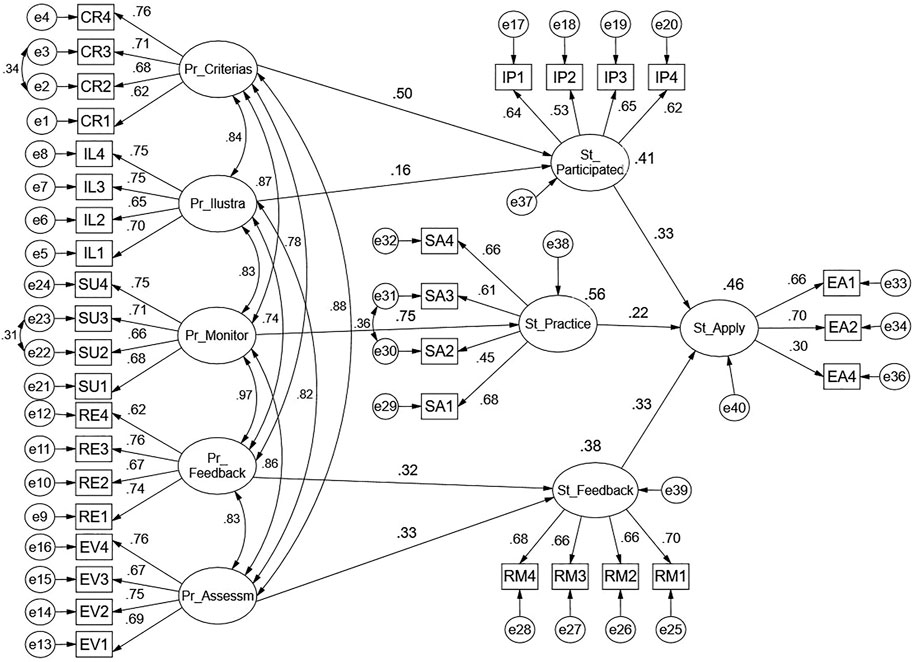
FIGURE 2. Structural regression model on the effects of the teaching performance criteria on the didactic performances of postgraduate students. Note. Teaching performance variables: Pr_Criterias = criteria explicitness, Pr_Ilustra = illustration, Pr_Monitor = supervision–monitoring, Pr_Feedback = feedback, and Pr_Assessm = evaluation. Student performance variables: St_Participated = illustration–participation, St_Practice = pertinent practice, St_Feedback = feedback–improvement, and St_Apply = evaluation–application.
The model shows that criteria explicitness (Pr_Criterias) and illustration (Pr_Ilustra) on the teacher’s side have positive effects on student participation (St_Participated) in order to realize learning achievements: the combined impact of both factors of teaching performance on student participation is 41%; the role played by the teacher in the supervision of practices has a direct impact of 56% on the pertinent practice of the student; the last two factors of teaching performance (feedback and evaluation) as generated conditions have a 38% direct impact on self-generated improvement by the student; finally, it is worth noting that the five teaching performance criteria have indirect effects on the application of the acquired knowledge and competencies by the students to solve new tasks or problems; this last criterion of student performance is partially explained (46%) by the direct effects of the other three student performance criteria.
For the second SEM model proposed (Figure 3), the teacher’s performance criteria are configured as a second-order latent construct where E (teaching category) and R (feedback category) are related to the factors of the students’ performance. Global indices reveal that the model has good fitness values: S-B χ2 (545) = 859.311, p = 0.000; CFI = 0.932; TLI = 0.926; IFI = 0.933; RMSEA = 0.043 (0.038, 0.048); SRMR = 0.062.
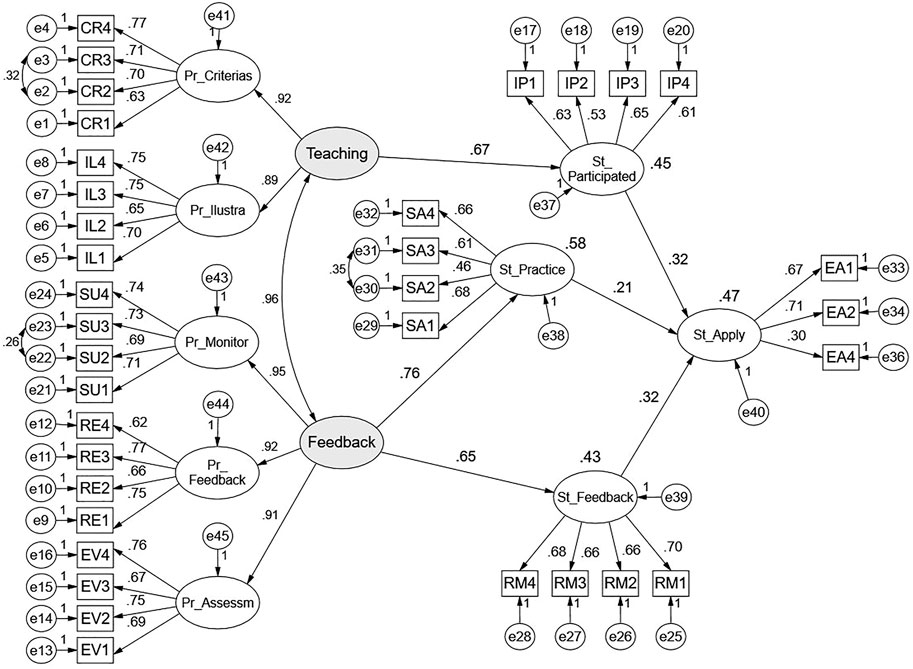
FIGURE 3. Structural regression model of Teaching and Feedback perceived on the students’ didactic performances. Note. Teaching performance variables: teaching, feedback, Pr_Criterias = criteria explicitness, Pr_Ilustra = illustration, Pr_Monitor = supervision–monitoring, Pr_Feedback = feedback, Pr_Assessm = evaluation, St_Participated = illustration–participation. Student performance variables: St_Practice = pertinent practice, St_Feedback = feedback–improvement, and St_Apply = evaluation–application.
As per the estimated parameters, the latent factor evaluation–application (EA), understood as the students’ capacity to apply the acquired knowledge in new and demanding situations, is partially explained (47%) by the latent categories of teacher performance (E and R) with a direct mediation from the students’ didactic performance criteria, such as student participation (IP), supervision of practices (SA), and feedback–Improvement (RM). In particular, it can be seen that the R category (feedback) from the teacher’s performance has positive direct effects on SA and RM of the students’ performance and that both factors predict the application and generalization of the acquired knowledge.
In order to identify whether there are significant differences in the perception of teaching performance and the teaching and feedback factors according to the level of studies, a complementary analysis of differences in means was performed. Table 6 shows that according to the results of Student’s t-test, there are no significant differences (p > 0.05) or practical differences (d < 0.20) between master’s and doctoral students when comparing the perception of teaching performance and the teaching and feedback factors.

TABLE 6. Comparison of perception of teacher performance and teaching and feedback factors according to postgraduate level.
On the other hand, Table 7 shows the results of Student’s t-test according to the postgraduate level (master’s and doctorate), in the four student performance variables: evaluation–application, illustration–participation, relevant practice, and feedback–improvement. The data in Table 7 show that there are no statistically significant differences between master’s and doctoral students in the four student didactic performance variables. Likewise, the effect size estimated with Cohen’s d reaffirms the absence of significant differences between master’s and doctoral students.
4 Discussion
The main objective of this study was to assess the effect of five variables from the teacher’s didactic performance (two from teaching and three from feedback) on self-assessed evaluation and application, mediated by the students’ participation, pertinent practice, and improvement performances by testing a structural regression model. For this purpose, self-reports from postgraduate students were used, understanding that this postgraduate program was intended for in-person sessions and that as a result of the COVID-19 pandemic, students started attending classes and submitting homework and assignments exclusively online.
As per the results shown in Figure 2, the resulting model that included the five teacher performance constructs as predictors yielded good goodness-of-fit indicators; however, it showed moderate divergent construct validity due to high covariation indices among teaching and feedback performances. This remained a methodological task to be solved. However, a greater effect of the supervision of practice teacher performance could be seen on the student’s pertinent practice variable (coefficient = 0.75). In addition, significant predictions were reported for the two teaching performances on illustration–participation student performance, although the explicitness of criteria had a greater predictive weight on student participation. Likewise, significant predictions were reported for the feedback and evaluation teacher performances on the feedback–improvement student performance. These data provide empirical evidence of the direct effect that both teaching and feedback have on the students’ didactic performance in virtual classes and practices.
The variable to predict was the evaluation–application student performance. The data from the structural regression model showed that both the Illustration–participation and the feedback–improvement student performances had a similar effect on evaluation–application. Likewise, the supervision of the pertinent practice significantly predicted evaluation–application, although to a lesser extent than the other two variables. This means that both the students’ learning performance (participation) linked to the teacher’s teaching variables and the students’ improvement performance linked to the teacher’s feedback variables equally influence the students’ application practice in self-assessed student evaluations.
These structural correlations had better configuration and goodness of fit than the obtained model when the five teacher performances were arranged as second-order factors, two performance constructs (criteria explicitness and illustration) and their four indicators, factorially belonging to teaching, and three performance constructs (supervision of practice, feedback, and evaluation), factorially belonging to feedback. Thus, the regression coefficients were higher from these two second-order factors (teaching and feedback) on the three student performance variables that functioned as mediating variables; however, the predictive values of these mediating variables on evaluation–application did not vary significantly for the first model (referred to in Figure 2). As per the parsimony principle, we can affirm that this second model was most adequate to explain the self-assessed evaluation–application student performance, mediated by the participation, pertinent practice, and feedback–improvement performances.
It is interesting noting that in the models obtained, teaching performances positively influence not only the students’ perception of their performance of participation and adjustment to the teaching and achievement criteria promoted by their teachers but also, albeit indirectly, the students’ performance of application and adjustment to the evaluations. The results of this study empirically support the assumption that teacher performance in the didactic interaction influences student learning (Kaplan and Owings, 2001), both in synchronous and asynchronous didactic interactions (Prince et al., 2020). The positive effect of teaching variables on achievement variables as perceived by the students has been reported by other researchers, namely, the positive and significant effect of interactive instruction (Liu and Cohen, 2021), and instructional support on the students’ performance (Wang et al., 2021).
On the other hand, feedback (supervision of practice, feedback itself, and evaluation) has a direct influence on the students’ performance in adjusting to feedback and improving their performance, a variable that, in turn, positively influences the students’ application and adjustment in self-assessed evaluations. In terms of what Jellicoe and Forsythe (2019) have outlined as crucial steps, the relationship between the teacher’s feedback actions and the student’s incorporation of such feedback into their behavioral repertoire implies the student accepting the feedback provided by their teacher and having trust that said feedback will demand plausible challenges and changes in their behavior and learning.
Another important aspect of this study is seeing the simultaneous effect of teaching and feedback on three different mediator variables that refer to student performances and their indirect effects on the students’ application and evaluation adjustment performance. This study found high covariation between these two factors, which demonstrates their interdependence as important variables in terms of teaching (also called instruction). Gopal et al. (2021) have found that variables related to teacher instructional quality were better predictors of student satisfaction during online classes, while teacher feedback as a predictor had a lesser effect than the instructional variable.
According to this study, and dismissing the teaching variables, the teacher performance addressing achievement criteria explicitness and learning objectives as a performance variable poses a greater impact on the students’ participation and adjustment to illustration performances. However, the impact of explicitness of achievement criteria on student adjustment to instruction (e.g., illustration) and their participation in lectures and derived tasks can be influenced by the feedback provided by the teacher. For example, Krijgsman et al. (2019) found that when students perceive very high feedback levels, the additional benefits of objective explicitness are reduced, and vice versa.
The results of this study also showed higher regression weight between teacher feedback and student performance in relevant practice and improvement. Likewise, high predictive weight was found for the teacher’s explicitness of criteria on student participation. However, the teacher’s didactic performance called illustration appears highly correlated with the teacher’s performance in monitoring, explicitness of achievement criteria, and feedback. This highlights the importance of the instructional process itself, a finding that is consistent with the contributions of Krijgsman et al. (2021); in the sense that learning objectives and criteria explicitness to the students, as well as feedback, by themselves will not have a direct effect on the self-assessment of learning, on achievement satisfaction or on indicators of academic achievement. Hence, the importance of treating teaching and feedback as multidimensional variables is shown, but closely related to the instructional process and formative assessment.
The implications of the findings of this study are several for what a teacher can do in a classroom. 1. The teacher’s monitoring and supervision of his or her students’ practice and actions is a fundamental variable in teaching and learning in the context of higher education. An aspect to highlight in this study is having considered the supervision of practice by the teacher not as a dimension of the teaching factor but as a dimension of the feedback factor, understood that this performance is more focused on the process of how the teacher monitors the progress of their students, and closely related to formative assessment and feedback. The data from the confirmatory factor analysis and invariance analysis on the teaching performance questionnaire have confirmed the monitoring variable (supervision of practice) together with the variables feedback and evaluation, constituted a second-order factor called feedback, which significantly influenced student performance in relevant practice and improvement. Similarly, these confirmed dimensions match other traits from the teacher’s feedback behavior reported by other authors, for example, the variables quality of feedback and using assessments in instruction (Liu and Cohen, 2021), and Supervision of student learning (Loose and Ryan, 2020).
In both structural regression models, feedback variables had a greater impact on student didactic performance than teaching variables. Thus, our results confirmed the importance that teachers should consider feedback as a multidimensional construct that has a significant effect on student performance variables in teaching and learning situations, particularly on relevant practice and adjustment to feedback for improvement in the learning process. In this study, three dimensions of feedback were included, supervision or monitoring of practice, learning feedback, and evaluation of what has been learned, but it is possible that teachers also use other ways to provide feedback to their students’ learning, for example, through online follow-up and monitoring applications, or by encouraging self-evaluation and self-feedback. Another aspect that teachers in the classroom should take into account is that feedback, as a multidimensional factor, can have direct and indirect influence on student performance in evaluations and the application of what they have learned.
2. Another aspect of importance for the teacher’s work in teaching and learning situations has to do with performance in the explicitness of the achievement criteria for student learning. This variable had a greater predictive weight in student performance in the participation variable and, to a lesser extent, but significantly, the teacher’s performance in illustration (presentation of the content to be learned). Likewise, in this study, the achievement in criteria explicitness and illustration form one performance factor (teaching), according to the perception of their postgraduate students. The two teaching behaviors correspond to what other researchers have reported as characteristics of the teacher’s teaching behavior, for example, creating conditions to provide students with the opportunity to learn explicit criteria according to their own curricular needs (Kaplan and Owings, 2001).
Although it is true that our results indicate a greater predictive weight of the teacher’s performance in the explicitness of criteria, the variable that has to do with the teaching and presentation of content (illustration) also plays an important role in influencing student participation and its subsequent application in learning assessment contexts. In other words, both the explicitness of achievement criteria and the illustration (presentation of contents) are important variables for developing an instructional practice focusing on the understanding of contents (Bell et al., 2012), to promote constructive and critical interactions between them (Serbati et al., 2020), to structure didactic interactions (teacher- and/or student-centered), and to model the skills to be developed by their students (Bazán–Ramírez et al., 2021a). Moreover, the findings of this study regarding aspects of illustration (teaching performance) match teacher performance characteristics previously reported by Liu and Cohen (2021). Analysis and problem solving, instructional dialog, engaging students in learning, modeling, strategy use, and instruction and representation of content (Liu and Cohen, 2021) imply teaching characteristics on the teacher’s side.
These results with graduate students of educational sciences describe teachers’ performances in virtual classes due to the pandemic, as perceived by the students. Therefore, the assessment made by the students implies didactic performances usually deployed in the face-to-face modality, which were adapted for their classes in virtual modality. In this regard, Loose and Ryan (2020) found that the transition to remote learning due to the pandemic led teachers to modify and innovate their instructional practices and develop ways to equalize positions between the teachers and students. In this way, the teaching and feedback performances on the part of the teacher reflect the possibility of moving content entirely from the face-to-face modality to an online course, facilitating a version of distance learning (George, 2020).
The variables of didactic performance on the part of the teacher and performance on the part of the students that we have included in the present work can be useful to assess synchronous (in class activity) and asynchronous learning (virtual learning environment, VLE) (Rehman and Fatima, 2021). Our results coincide with the proposal of (Abrahamsson and Dávila López, 2021) for the improvement of online learning, based on teaching strategies, monitoring, and feedback (Abrahamsson and Dávila López, 2021). Likewise, our results agree with the findings of Wang et al. (2021) that in virtual classrooms, teachers’ instructional performance plays a fundamental role in facilitating students’ learning outcomes in higher education.
An important limitation in this study is that the variable to predict was a latent factor that considers only the students’ self-assessment of their own didactic performance related to the application of acquired knowledge and the adjustment to the assessments made by the teacher. Although most studies relying on self-reporting on the perception of the teacher’s performance according to university students have included indicators of academic satisfaction or achievements as dependent variables (albeit self-perceived by the students), it would be relevant for future studies to include measurements of the actual academic achievements of undergraduate and/or postgraduate students as one of the latent variables to predict from teaching and feedback variables mediated by the student’s performance self-assessment.
4.1 Conclusion
The study hereby presented has found that teaching and feedback teacher performance variables, as perceived by educational sciences postgraduate students during virtual classes (in-person before the COVID-19 pandemic), significantly influence three student performance variables: illustration–participation (St_Participated), practice (St_Practice), and feedback–improvement (St_Feedback). This significant effect can be observed either when the five teacher performance variables are included as predictor variables or when arranged into two second-order factors (teaching and feedback).
It was also found that the evaluation–application student latent variable was significantly and equally influenced by the illustration–participation, and feedback–improvement student performance variables. Moreover, the pertinent practice performance had a significant effect, although to a lesser extent than the former two, on the evaluation–application student latent variable: evaluation–application.
Data Availability Statement
The raw data supporting the conclusion of this article will be made available by the authors, without undue reservation.
Ethics Statement
Ethical review and approval was not required for the study on human participants in accordance with the local legislation and institutional requirements. The patients/participants provided their written informed consent to participate in this study.
Author Contributions
AB-R developed and directed the research project and led the writing of this manuscript. He did the search and analysis of the literature on this topic, also contributed to the analysis and interpretation of data, and to the revision of the English version and writing in Frontiers format. WC-L directed the analysis and interpretation of data and contributed to the writing of the manuscript. He also helped in the review the English version and the writing in Frontiers format. CB-V was in charge of writing the English version of this manuscript. Also, she helped in the writing in Frontiers format. RQ-M contributed to the development and execution of the research project; assisted in collection, analysis, and interpretation of data; and supported the search for additional bibliographic information.
Conflict of Interest
The authors declare that the research was conducted in the absence of any commercial or financial relationships that could be construed as a potential conflict of interest.
Publisher’s Note
All claims expressed in this article are solely those of the authors and do not necessarily represent those of their affiliated organizations, or those of the publisher, the editors, and the reviewers. Any product that may be evaluated in this article, or claim that may be made by its manufacturer, is not guaranteed or endorsed by the publisher.
Supplementary Material
The Supplementary Material for this article can be found online at: https://www.frontiersin.org/articles/10.3389/feduc.2022.818209/full#supplementary-material
References
Abrahamsson, S., and Dávila López, M. (2021). Comparison of Online Learning Designs during the COVID-19 Pandemic within Bioinformatics Courses in Higher Education. Bioinformatics 37 (Suppl. 1), i9–i15. doi:10.1093/bioinformatics/btab304
Aidoo, A., and Shengquan, L. (2021). The Conceptual Confusion of Teaching Quality and Teacher Quality, and a Clarity Pursuit. IJAE.j.asia.edu 2 (2), 98–119. doi:10.46966/ijae.v2i2.168
Bazán, A., and Velarde, N. (2021). Auto-reporte del estudiantado en criterios de desempeño didáctico en clases de Psicología [Students Self-report within didactic performances criteria in Psychology classes]. J. Behav. Health Soc. Issues 13 (1), 22–35. doi:10.22201/fesi.20070780e.2021.13.1.75906
Bazán-Ramírez, A., Hernández, E., Castellanos, D., and Backhoff, E. (2021a). Oportunidades para el aprendizaje, contexto y logro de alumnos mexicanos en matemáticas [Opportunities to learn, contexts and achievement of mexican students in mathematics]. profesorado 25 (2), 327–350. doi:10.30827/profesorado.v25i2
Bazán-Ramírez, A., Pérez-Morán, J. C., and Bernal-Baldenebro, B. (2021b). Criteria for Teaching Performance in Psychology: Invariance According to Age, Sex, and Academic Stage of Peruvian Students. Front. Psychol. 12, 764081. doi:10.3389/fpsyg.2021.764081
Bell, C. A., Gitomer, D. H., McCaffrey, D. F., Hamre, B. K., Pianta, R. C., and Qi, Y. (2012). An Argument Approach to Observation Protocol Validity. Educ. Assess. 17, 62–87. doi:10.1080/10627197.2012.715014
Blumenfeld, A., Velic, A., Bingman, E. K., Long, K. L., Aughenbaugh, W., Jung, S. A., et al. FACS (2020). A Mastery Learning Module on Sterile Technique to Prepare Graduating Medical Students for Internship. MedEdPORTAL 16, 10914. doi:10.15766/mep_2374-8265.10914
Borges, Á., Falcón, C., and Díaz, M. (2016). Creation of an Observational Instrument to Operationalize the Transmission of Contents by university Teachers. Int. J. Soc. Sci. Stud. 4 (7), 82–89. doi:10.11114/ijsss.v4i7.1596
Carpio, C., and Irigoyen, J. J. (2005). Psicología y Educación. Aportaciones desde la teoría de la conducta. [Psychology and Education. Contributions from the theory of behavior]. Universidad Nacional Autónoma de México.
Carpio, C., Pacheco, V., Canales, C., and Flores, C. (1998). Comportamiento inteligente y juegos de lenguaje en la enseñanza de la psicología [Intelligent behavior and language games in the teaching of psychology]. Acta comportamentalia 6 (1), 47–60.
Chen, F. F. (2007). Sensitivity of Goodness of Fit Indexes to Lack of Measurement Invariance. Struct. Equation Model. A Multidisciplinary J. 14 (3), 464–504. doi:10.1080/10705510701301834
Cheung, G. W., and Rensvold, R. B. (2002). Evaluating Goodness-Of-Fit Indexes for Testing Measurement Invariance. Struct. Equation Model. A Multidisciplinary J. 9 (2), 233–255. doi:10.1207/S15328007SEM0902_5
Dees, D. M., Ingram, A., Kovalik, C., Allen-Huffman, M., McClelland, A., and Justice, L. (2007). A Transactional Model of College Teaching. Int. J. Teach. Learn. Higher Edu. 19 (2), 130–140.
Dimitrov, D. M. (2010). Testing for Factorial Invariance in the Context of Construct Validation. Meas. Eval. Couns. Dev. 43 (2), 121–149. doi:10.1177/0748175610373459
Finney, S. J., and DiStefano, C. (2008). “Non-normal and Categorical Data in Structural Equation Modeling,” in Structural Equation Modeling: A Second Course. Editors G. R. Hancock, and R. D. Mueller (Information Age Publishing), 269–314.
Fischer, J., Praetorius, A.-K., and Klieme, E. (2019). The Impact of Linguistic Similarity on Cross-Cultural Comparability of Students' Perceptions of Teaching Quality. Educ. Asse Eval. Acc. 31, 201–220. doi:10.1007/s11092-019-09295-7
Forsythe, A., and Jellicoe, M. (2018). Predicting Gainful Learning in Higher Education; a Goal-Orientation Approach. Higher Edu. Pedagogies 3 (1), 103–117. doi:10.1080/23752696.2018.1435298
George, M. L. (2020). Effective Teaching and Examination Strategies for Undergraduate Learning during COVID-19 School Restrictions. J. Educ. Tech. Syst. 49 (1), 23–48. doi:10.1177/0047239520934017
Gitomer, D., Bell, C., Qi, Y., McCaffrey, D., Hamre, B. K., and Pianta, R. C. (2014). The Instructional challenge in Improving Teaching Quality: Lessons from a Classroom Observation Protocol. Teach. Coll. Rec. 116 (6), 1–32. doi:10.1177/016146811411600607
Gitomer, D. H. (2018). Evaluating Instructional Quality. Sch. Effectiveness Sch. Improvement 30, 68–78. doi:10.1080/09243453.2018.1539016
Gopal, R., Singh, V., and Aggarwal, A. (2021). Impact of Online Classes on the Satisfaction and Performance of Students during the Pandemic Period of COVID 19. Educ. Inf. Technol. 26, 6923–6947. doi:10.1007/s10639-021-10523-1
Grosse, C., Kluczniok, K., and Rossbach, H.-G. (2017). Instructional Quality in School Entrance Phase and its Influence on Students' Vocabulary Development: Empirical Findings from the German Longitudinal Study BiKS. Early Child. Dev. Care 189 (9), 1561–1574. doi:10.1080/03004430.2017.1398150
Hafen, C. A., Hamre, B. K., Allen, J. P., Bell, C. A., Gitomer, D. H., and Pianta, R. C. (2014). Teaching through Interactions in Secondary School Classrooms: Revisiting the Factor Structure and Practical Application of the Classroom Assessment Scoring System-Secondary. J. Early Adolesc. 35, 651–680. doi:10.1177/0272431614537117
Hayes, L. J., and Fryling, M. J. (2018). Psychological Events as Integrated fields. Psychol. Rec. 68 (2), 273–277. doi:10.1007/s40732-018-0274-3
Howe, E. R., and Watson, G. C. (2021). Finding Our Way through a Pandemic: Teaching in Alternate Modes of Delivery. Front. Educ. 6, 661513. doi:10.3389/feduc.2021.661513
Hu, L. T., and Bentler, P. M. (1999). Cutoff Criteria for Fit Indexes in Covariance Structure Analysis: Conventional Criteria versus New Alternatives. Struct. Equation Model. A Multidisciplinary J. 6 (1), 1–55. doi:10.1080/10705519909540118
Irigoyen, J., Acuña, K., and Jiménez, M. (2011). “Interacciones didácticas en educación superior. Algunas consideraciones sobre la evaluación de desempeño [Didactic interactions in higher education. Some considerations about performance evaluation],” in Evaluación de desempeños académicos [Performance evaluation academics]. Editors J. Irigoyen, K. Acuña, and M. Jiménez (Universidad de Sonora), 73–96.
Jellicoe, M., and Forsythe, A. (2019). The Development and Validation of the Feedback in Learning Scale (FLS). Front. Educ. 4, 84. doi:10.3389/feduc.2019.00084
Joyce, J., Gitomer, D. H., and Iaconangelo, C. J. (2018). Classroom Assignments as Measures of Teaching Quality. Learn. Instr. 54, 48–61. doi:10.1016/j.learninstruc.2017.08.001
Kantor, J. R. (1975). Education in Psychological Perspective. Psychol. Rec. 25 (3), 315–323. doi:10.1007/BF03394321
Kaplan, L. S., and Owings, W. A. (2001). Teacher Quality and Student Achievement: Recommendations for Principals. NASSP Bull. 85, 64–73. doi:10.1177/019263650108562808
Keith, T. Z. (2019). Multiple Regression and beyond. An Introduction to Multiple Regression and Structural Equation Modeling. 3a ed. Routledge.
Klieme, E., Pauli, C., and Reusser, K. (2009). “The Pythagoras Study-Investigating Effects of Teaching and Learning in Swiss and German Mathematics Classrooms,” in The Power of Video Studies in Investigating Teaching and Learning in the Classroom. Editors T. Janik, and T. Seidel (Waxmann), 137–160.
Kozuh, A., and Maksimovic, J. (2020). Ways to Increase the Quality of Didactic Interactions. World J. Educ. Technol. Curr. Issues 12 (3), 179–191. doi:10.18844/wjet.v%vi%i.4999
Krijgsman, C., Mainhard, T., Borghouts, L., van Tartwijk, J., and Haerens, L. (2021). Do goal Clarification and Process Feedback Positively Affect Students' Need-Based Experiences? A Quasi-Experimental Study Grounded in Self-Determination Theory. Phys. Edu. Sport Pedagogy 26 (5), 483–503. doi:10.1080/17408989.2020.1823956
Krijgsman, C., Mainhard, T., van Tartwijk, J., Borghouts, L., Vansteenkiste, M., Aelterman, N., et al. (2019). Where to Go and How to Get There: Goal Clarification, Process Feedback and Students' Need Satisfaction and Frustration from Lesson to Lesson. Learn. Instruction 61, 1–11. doi:10.1016/j.learninstruc.2018.12.005
Li, C. H. (2016). Confirmatory Factor Analysis with Ordinal Data: Comparing Robust Maximum Likelihood and Diagonally Weighted Least Squares. Behav. Res. Methods 48 (3), 936–949. doi:10.3758/s13428-015-0619-7
Liu, J., and Cohen, J. (2021). Measuring Teaching Practices at Scale: A Novel Application of Text-As-Data Methods. Educ. Eval. Pol. Anal. 43, 587–614. First Published May 24, 2021. doi:10.3102/01623737211009267
Loose, C. C., and Ryan, M. G. (2020). Cultivating Teachers when the School Doors Are Shut: Two Teacher-Educators Reflect on Supervision, Instruction, Change and Opportunity during the Covid-19 Pandemic. Front. Educ. 5, 582561. doi:10.3389/feduc.2020.582561
Morales, G., Peña, B., Hernández, A., and Carpio, C. (2017). Competencias didácticas y competencias de estudio: su integración funcional en el aprendizaje de una disciplina [Didactic competencies and competencies of study: its functional integration in the learning of a discipline]. Alternatives Psychology/Alternativas en Psicología 21 (37), 24–35.
Nugroho, I., Paramita, N., Mengistie, B. T., and Krupskyi, O. P. (2021). Higher Education Leadership and Uncertainty during the COVID-19 Pandemic. JSeD 4 (1), 1–7. doi:10.31328/jsed.v4i1.2274
Organization for Economic Cooperation and Development. OECD (2017). PISA 2015 Assessment and Analytical Framework. Science, Reading, Mathematic, Financial Literacy and Collaborative Problem Solving. Revised edition. doi:10.1787/9789264281820-en
Organization for Economic Cooperation and Development, OECD (2013). PISA 2012 Assessment and Analytical Framework: Mathematics, Reading, Science. Problem Solving and Financial Literacy. doi:10.1787/9789264190511-en
Peralta, N. S., and Roselli, N. D. (2015). Los sistemas de interacción generados por la impronta didáctica del docente [The interaction systems generated by the teacher’s didactic imprinting]. Propós. Represent. 3 (2), 155–178. doi:10.20511/pyr2015.v3n2.85
Pop, C., and Khampirat, B. (2019). Self-assessment Instrument to Measure the Competencies of Namibian Graduates: Testing of Validity and Reliability. Stud. Educ. Eval. 60, 130–139. doi:10.1016/j.stueduc.2018.12.004
Prince, M., Felder, R., and Brent, R. (2020). Active Student Engagement in Online STEM Classes: Approaches and Recommendations. Adv. Eng. Edu. 8 (4), 1–25.
R Development Core Team (2020). R: A Language and Environment for Statistical Computing. R Foundation for Statistical Computing. https://cran.r-projet.org/.
Rehman, R., and Fatima, S. S. (2021). An Innovation in Flipped Class Room: A Teaching Model to Facilitate Synchronous and Asynchronous Learning during a Pandemic. Pak J. Med. Sci., 37(1):131–136. doi:10.12669/pjms.37.1.3096
Ribes, E. (1990). Problemas conceptuales en el análisis del comportamiento humano [Conceptual problems in the analysis of human behavior]. Trillas.
Sarkar, M., Gibson, S., Karim, N., Rhys-Jones, D., and Ilic, D. (2021). Exploring the Use of Self-Assessment to Facilitate Health Students' Generic Skills Development. Jtlge 12 (2), 65–81. doi:10.21153/jtlge2021vol12no2art976
Satorra, A., and Bentler, P. M. (2010). Ensuring Positiveness of the Scaled Difference Chi-Square Test Statistic. Psychometrika 75, 243–248. doi:10.1007/s11336-009-9135-y
Schumacker, R. E., and Lomax, R. G. (2016). A Beginner’s Guide to Structural Equation Modeling. 4a ed. Routledge. doi:10.4324/9781315749105A Beginner's Guide to Structural Equation Modeling
Serbati, A., Aquario, D., Da Re, L., Paccagnella, O., and Felisatti, E. (2020). Exploring Good Teaching Practices and Needs for Improvement: Implications for Staff Development. J. Educ. Cult. Psychol. Stud. (ECPS Journal) 21, 43–64. doi:10.7358/ecps-2020-021-serb
Silva, H. O., Morales, G., Pacheco, V., Camacho, A. G., Garduño, H. M., and Carpio, C. A. (2014). Didáctica Como Conducta: Una Propuesta Para La Descripción De Las Habilidades De Enseñanza [Didactic as behavior: a proposal for the description of teaching skills]. Rmac 40 (3), 32–46. doi:10.5514/rmac.v40.i3.63679
Sumer, M., Douglas, T., and Sim, K. (2021). Academic Development through a Pandemic Crisis: Lessons Learnt from Three Cases Incorporating Technical, Pedagogical and Social Support. J. Univ. Teach. Learn. Pract. 18 (5). doi:10.53761/1.18.5.1
Thomas, M. B. (2020). Virtual Teaching in the Time of COVID-19: Rethinking Our WEIRD Pedagogical Commitments to Teacher Education. Front. Educ. 5, 595574. doi:10.3389/feduc.2020.595574
Velarde Corrales, N. M., and Bazán-Ramírez, A. (2019). Sistema observacional para analizar interacciones didácticas en clases de ciencias en bachillerato. Rev. Investig. Psicol. 22 (2), 197–216. doi:10.15381/rinvp.v22i2.16806
Wang, R., Han, J., Liu, C., and Xu, H. (2021). How Do University Students' Perceptions of the Instructor's Role Influence Their Learning Outcomes and Satisfaction in Cloud-Based Virtual Classrooms during the COVID-19 Pandemic. Front. Psychol. 12, 627443. doi:10.3389/fpsyg.2021.627443
Winstone, N. E., Mathlin, G., and Nash, R. A. (2019). Building Feedback Literacy: Students' Perceptions of the Developing Engagement with Feedback Toolkit. Front. Educ. 4, 39. doi:10.3389/feduc.2019.00039
Wisniewski, B., Zierer, K., and Hattie, J. (2020). The Power of Feedback Revisited: A Meta-Analysis of Educational Feedback Research. Front. Psychol. 10, 3087. doi:10.3389/fpsyg.2019.03010.3389/fpsyg.2019.03087
Keywords: teaching, feedback, didactic performance, virtual classes, pandemic
Citation: Bazán-Ramírez A, Capa-Luque W, Bello-Vidal C and Quispe-Morales R (2022) Influence of Teaching and the Teacher’s Feedback Perceived on the Didactic Performance of Peruvian Postgraduate Students Attending Virtual Classes During the COVID-19 Pandemic. Front. Educ. 7:818209. doi: 10.3389/feduc.2022.818209
Received: 19 November 2021; Accepted: 03 January 2022;
Published: 25 January 2022.
Edited by:
Gavin T. L. Brown, The University of Auckland, New ZealandReviewed by:
Marcela Dávila, University of Gothenburg, SwedenJorge Carlos Aguayo Chan, Universidad Autónoma de Yucatán, Mexico
Copyright © 2022 Bazán-Ramírez, Capa-Luque, Bello-Vidal and Quispe-Morales. This is an open-access article distributed under the terms of the Creative Commons Attribution License (CC BY). The use, distribution or reproduction in other forums is permitted, provided the original author(s) and the copyright owner(s) are credited and that the original publication in this journal is cited, in accordance with accepted academic practice. No use, distribution or reproduction is permitted which does not comply with these terms.
*Correspondence: Aldo Bazán-Ramírez, YWJhemFucmFtaXJlekBnbWFpbC5jb20=