- Harvard Medical School, Boston, MA, United States
Annotating a text while reading is commonplace and essentially as old as printed text itself. Collaborative online annotation platforms are enabling this process in new ways, turning reading from a solitary into a collective activity. The platforms provide a critical discussion forum for students and instructors that is directly content-linked, and can increase uptake of assigned reading. However, the student viewpoint regarding collaborative online annotation platforms remains largely unexplored, as do comparisons between annotation and traditional reading assessment methods, and comparisons between the two leading platforms (Hypothes.is vs. Perusall) for annotation by the same student population. The results in this study indicate that collaborative online annotation is largely preferred by students over a traditional reading assessment approach, that students regularly exceed annotation requirements indicated by an instructor, and that overall annotation quality increased as the students gained experience with the platforms. The data analysis in this study can serve as a practical exemplar for measurement of student annotation output, where baselines have yet to be established. These findings link the established research areas of peer learning, formative assessment, and asynchronous learning, with an emerging educational technology.
Introduction
Hovering over a text with a pencil, adding a sticky note to a page, or making digital document highlights and comments, are natural and familiar practices. Some readers feel that they are not giving a text their full attention without adding annotations (O’Connell, 2012). Centuries-old text annotations feature drawings, critical explanation, corrections, and comments to other readers, at times exceeding the amount of primary text itself (Wolfe and Neuwirth, 2001; Wolfe, 2002). An annotator might also leave memory prompts, questions, predictions, and connections to other work. For the consumer, the annotations of another student or scholar can be mined for insights that might go unappreciated if reading an unannotated text. Some students prefer second hand textbooks that have already been annotated by previous readers, for precisely this reason (Van Dam, 1988; November, 2020). Wolfe and Neuwirth (2001) propose four main functions of annotation: to facilitate reading and later writing tasks by making self-directed annotations, to eavesdrop on the insights of other readers, to provide feedback to writers or promote communication with collaborators, and to call attention to topics and important passages. In Kalir and Garcia’s (2019) comprehensive work, annotation is defined broadly as a note added to a text, which can provide information, share commentary, express power, spark conversation, and aid learning. Accordingly, the online draft copy of their book includes annotations by other scholars that provide extended critical thoughts for any reader willing to consume them. Suggestions for annotations to be positioned as a third independent component of a text (Bold and Wagstaff, 2017), prompt us to consider not only the medium and the message (McLuhan, 1964), but also the marginalia (Jackson, 2001) in all of our reading.
A lack of student attention to assigned reading can be problematic for teachers. In a study of multiple physics courses, only 37% of students regularly read the textbook, and less than 13% read often and before the relevant lecture was occurring (Podolefsky and Finkelstein, 2006). This is in accord with a study of psychology courses, where a similarly low 28% of students did the assigned reading before it was covered in class (Clump et al., 2004). The importance that professors attach to reading appears to be much higher than the importance attached by students. In a Business School study, only 4% of professors thought that a student could score an A or B grade without doing the assigned reading for a course, while 34% of the students thought they could do so (Braguglia, 2006). Furthermore, only 20% of students identified “not having done the reading” as a reason to not participate in discussions (Howard and Henney, 1998), so tutorial-based discussion may not be as strong of a motivator for reading as an instructor would like.
In addition to reading uptake problems, a student’s first experience reading primary literature (i.e., journal research articles, historical documents) can be challenging. They need to adjust to a format that is often less accommodating to the reader, and in the sciences, may have difficulty grasping technical details in experimental protocols and numerous data figures. It may also be greatly rewarding as students gain an appreciation for the structure of an inquiry and how it led to a particular finding, which is often absent when consuming information from a textbook (Wenk and Tronsky, 2011). For article interpretation in the sciences, there is often a focus on the figures, on questions that elicit student confusion, and on questions that would be good follow-up experiments given the data in the article at hand. Approaches for humanities and social science primary source documents may have a distinct, but similarly critical focus. Reading guidance can be provided to students as fillable templates containing thought-prompts from an instructor, an approach that has been repeatedly covered as a beneficial learning scaffold [see Create framework (Hoskins et al., 2011), Figure facts template (Round and Campbell, 2013), and the templates of others (Wenk and Tronsky, 2011; Yeong, 2015)]. The templates also relate to the process of Just in Time Teaching (Marrs and Novak, 2004), where student misconceptions about a particular reading can be obtained via a template or pre-class questions, so they can be adequately aired and addressed during a subsequent in-person session. Annotation can also be seen as an aid in primary literature comprehension with the Science in the Classroom approach (Kararo and McCartney, 2019), which uses papers pre-annotated by scientific professionals that define key technical terms, highlight previous work, and include news and policy links, in “lenses” that can be toggled on and off.
Distinct from pre-annotated papers is a social or collaborative online annotation approach where the students and instructors input commentary themselves to illuminate and debate various aspects of a text. Two of the leading collaborative online annotation platforms in current use are Hypothes.is and Perusall. Hypothes.is aims ‘‘to enable a conversation over the world’s knowledge,’’1 while Perusall positions itself as “the only truly social e-reader,’’ with ‘‘every student prepared for every class2.” Since educational technology platforms can change their interfaces and features regularly, going to the platform website will contain the most up to date and complete information for functionality, usage, and implementation tips. Additional guidance for Perusall may be found in King (2016). Both platforms enable collaborative online annotation of a text. Any part of a text that is highlighted is then linked to a marginal comment box, which can include not only commentary, but also tags, diagrams, and hyperlinks. Both platforms also support Latex for annotating with mathematical notation. Collaborative annotation is possible with any type of material that can be found as a webpage for Hypothes.is, or uploaded as a PDF for Perusall. Textbook material could be annotated if in an online eBook for Hypothes.is, or from Perusall’s catalogue of available textbooks. The two platforms differ in how they are accessed by students, user interface, social functionality, potential audience participation size, and annotation machine learning measurement capabilities.
In contrast to discussion forums that might appear on a learning management system, annotation platform discussions are grounded at a specific place within the document (highlighted word, sentence, paragraph, or figure region) rather than from a description of a figure or reference to a paragraph that is needed to establish context in a discussion forum post. Grounding in a primary document reduces the number of explicit references needed in order for comments to be understood (Honeycutt, 2001). If the source text is absent and not connected to a discussion, participants have to reconstruct the context, which has been referred to as communication overhead (Weng and Gennari, 2004).
Instructional goals in collaborative annotation may change according to the source material. One might expect collaborative annotation of textbook material to have a stronger focus on understanding the fundamental knowledge that the book provides. Annotating research articles may allow for additional goals that build on fundamental knowledge and consider the structure of an inquiry, along with its implications and possible pitfalls.
Prior work on collaborative online annotation (Miller et al., 2018; Lee et al., 2019) positions the research alongside theoretical frameworks of: Peer Instruction (Fagen et al., 2002), where students are collaboratively solving problems often focusing on common areas of misconception; Student-Centered Open Learning Environments (Hannafin et al., 2014) where students negotiate complex, open-ended problems in a largely independent manner with web resources and technology tools to complement sense-making; and Social Constructivism (Vygotsky, 1978; Adams, 2006), where cognitive functions originate in social interactions and learners integrate into a knowledge community. These three fields hold students’ prior experiences and co-construction of knowledge in high regard.
Theory developed in the formative assessment field also connects naturally to collaborative online annotation. For students to close in on a desired landmark skill for a course, they need to know what they are shooting for and what good practice of that skill looks like (Sadler, 1989). In collaborative online annotation, student thoughts about a text are out in the open. Another student’s reasoning and sense-making on a difficult article can be compared, and the instructor’s reasoning is also there to serve as a model for what criticism in an academic discipline looks like. For this reason, collaborative annotation has been suggested as a signature pedagogy for literary criticism courses, as it embodies the routines and value commitments in that field (Clapp et al., 2020); the sciences and social sciences can surely follow suit. The timing of feedback, another possible weakness in the assessment process, comes in a steady flow in collaborative annotation, as a text is read and analyzed by the instructor and students within a defined time window (less than 1 week in the current study), and the student can expect threads to build and their annotations to be commented upon within hours to days, and occasionally even in real-time if multiple students are active on the platform simultaneously. Peer to peer exchanges may also decrease some of the workload on instructors for feedback provision. Participation norms that used to focus on hands up in a lecture hall are now shifting to other forms of participation in a modern, technology-enhanced classroom (Jackson et al., 2018), if they have not already done so. Asynchronous teaching tools have become increasingly important with transitions to blended and fully online learning environments coming abruptly during a viral pandemic, and can be a welcome remedy for time zone and other technical issues that affect synchronous teaching.
Annotation as an aid for learning has prior support in various settings, both with and without any technological scaffolding. In a pen and paper setting where students were trained in effective textbook annotation routines by their instructors, student annotation was found to be better than a control non-annotation condition on later test performance and self-reported studying efficiency (Simpson and Nist, 1990). In a setting where instructors added marginal notes to course readings, the notes were overwhelmingly affirmed by students as a helpful study aid, and missed when they were absent from other non-annotated course readings (Duchastel and Chen, 1980). In a collaborative synchronous annotation setting using Google docs in English literature classes, annotation was viewed as a technique that allowed instructors to effectively highlight what good performance in literary analysis looks like, and students also felt greatly aided by reading the annotations of others in understanding a given text (Clapp et al., 2020). Collaborative annotation with Hypothes.is facilitated “close reading” with difficult texts (Kennedy, 2016). Perusall provided a stimulus for reading uptake, where 90–95% of students completed all but a few of the reading assignments before class if using Perusall, and also performed better on an exam than those students who took the same class without using Perusall (Miller et al., 2018). Novak et al. (2012) provide an excellent review of research on social annotation platforms; however, many of the platforms they analyzed have relatively small user bases, or are now defunct. Ghadirian et al. (2018) review social annotation tools and suggest that prior research has failed to capture students’ experiences while participating in social annotation activities, and that understanding of how to implement social annotation from disciplines outside of education and computer science is lacking. Wolfe and Neuwirth (2001) have pointed to the absence of studies focusing on participants to solicit their impressions of the technological environments during collaborative annotation. These gaps, coupled with the emergence of Hypothes.is and Perusall as key platforms, should drive new qualitative and quantitative investigation of collaborative online annotation.
There have been no published comparisons of student output and usage preferences between the two leading online annotation platforms, nor direct comparisons of annotation platforms to more traditional classroom assessment techniques such as reading templates, for the same type of content with the same population of students. Furthermore, the student viewpoint regarding collaborative online annotation remains relatively unexplored in prior publications, and pedagogical best practices are still emerging. Instructors and students familiar with more than one annotation platform are well-positioned to provide feedback on the annotation process as a whole. Establishing quantitative baselines for student output on an annotation platform will hold value for instructors to gauge activity in their own classes. To address the above gaps, and situate online annotation platforms for better use in the classroom, this study posed the following research questions:
1. Qualitatively, from the student viewpoint:
a. How do collaborative online annotation platforms compare to a more traditional templated assessment method for the same type of reading content?
b. How do the two leading collaborative online annotation platforms (Hypothes.is and Perusall) compare to each other?
2. Quantitatively, how do Hypothes.is and Perusall compare on student output for the following measures:
a. Number of annotations made per student per paper?
b. Character volume of a student’s annotations per paper?
c. Annotation content quality?
d. Percentage of isolated vs. collaborative threaded annotations?
Also captured are the changes over time in the quantitative measures as students proceed through successive paper analyses on a platform, and then move onto the next platform. The answers to the previous questions are further considered in order to shape more effective pedagogy for collaborative annotation. The educational technology, peer learning, and assessment fields stand to gain valuable insight from readers’ responses to dynamically annotated text.
Research Methods
Participant Details and Overall Workflow
The study took place with first year Master’s students, in a university in the northeastern United States, in a course focused on the analysis of scientific research papers. Two student cohorts participated: a 2019–2020 cohort of 18 students, and a 2020–2021 cohort of 21 students. Synchronous class sessions were held in-person for the 2019–2020 cohort during the months that the template completion and annotation activities were proceeding, and were held virtually for the 2020–2021 cohort. Annotation and template completion were done by all students on their own time, asynchronously, outside of any synchronous class sessions, with both cohorts. Figure 1 shows the paper analysis routine of students occurring under three different conditions: a traditional assessment template, the Hypothes.is annotation platform, and the Perusall annotation platform. There was 1 week of time allotted for each paper analysis. Both the 2019–2020 and 2020–2021 cohorts used the traditional template first, as it provided an established scaffold for beginners in paper analysis. After completing four assigned papers with the traditional template analysis, the students then used collaborative online annotation for another eight papers. With the 2019–2020 cohort, the Hypothes.is platform (four papers) was used first, followed by Perusall (four papers). The platform order was reversed with the 2020–2021 cohort – Perusall first, and Hypothes.is second. As such, the 2019–2020 cohort analyzed articles A, B, C, D via the traditional template, articles E, F, G, H with Hypothes.is, and articles I, J, K, L with Perusall; the 2020–2021 cohort analyzed articles M, N, O, P with the traditional template, articles Q, R, S, T with Perusall, and articles U, V, W, X with Hypothes.is. The bibliography for all articles A to X is available in Supplementary Table 1. All papers were recently published (2017–2020) biomedical science research journal articles, deemed to be roughly equivalent in scope and difficulty by the instructor (GWP). The research proposal was reviewed by the Harvard Human Research Protection Program and received the lowest risk categorization. Aid in the informed consent process for students was provided by a program administrator. All student data in this study has been anonymized.
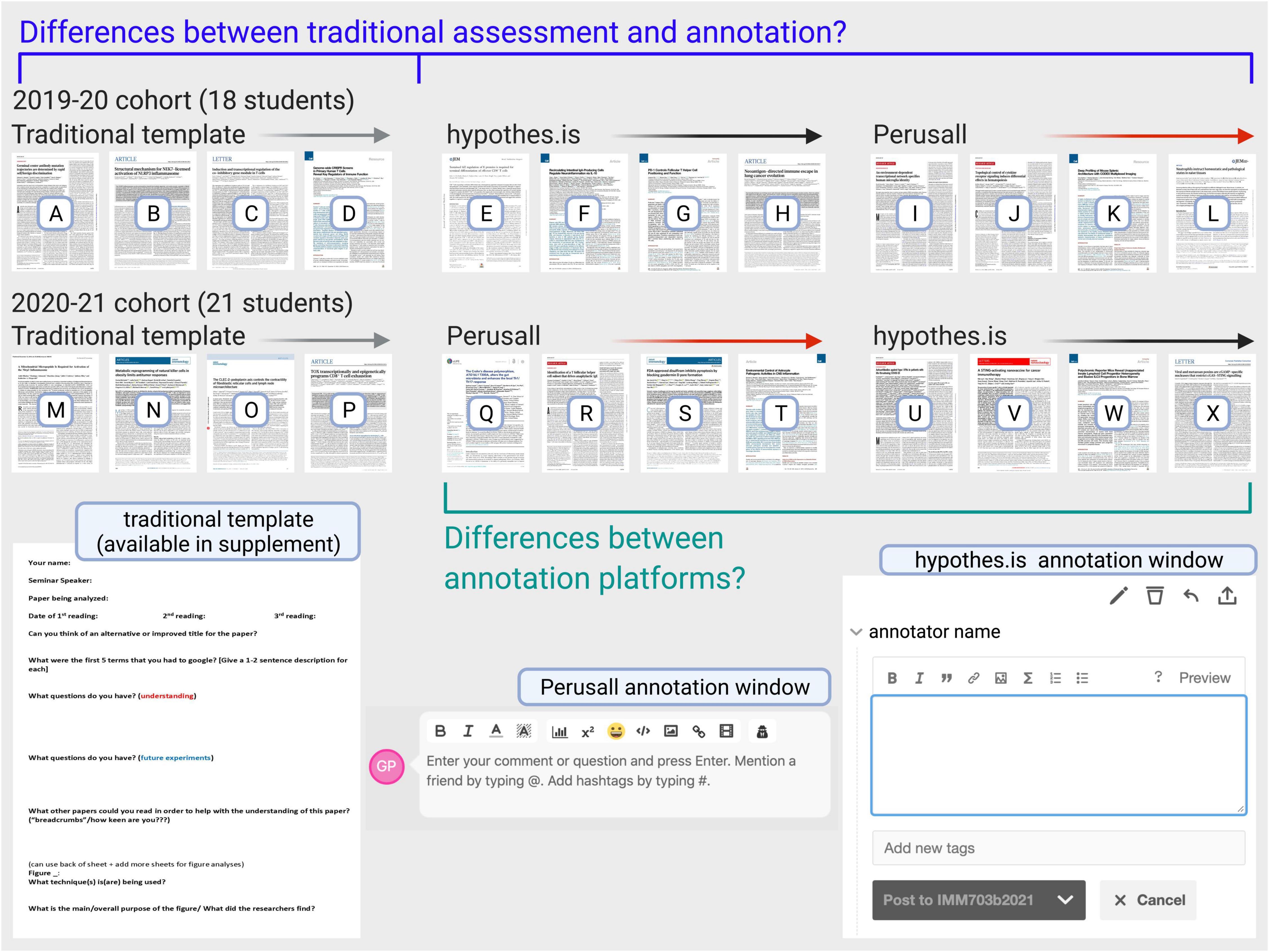
Figure 1. Study design and student reading assessment overview. Each student cohort analyzed four papers through a traditional reading assessment template, and four papers through each of the two collaborative online annotation platforms. This enabled two comparisons: the traditional template vs. annotation (as reading assessment methods), and Hypothes.is vs. Perusall (as annotation platforms). Full template prompts are available in section “Research Methods,” and the template is also available for download from Supplementary Material. Figure created with Biorender.com.
Research on technological and pedagogical innovations in student-centered open learning environments is thought to be best positioned within authentic classroom settings (Hannafin et al., 2014). This study follows an action research approach in education, as it is instructor-initiated, focused on practical elements of classroom function, and their future improvement (McMillan, 2015; Beck, 2017). With template vs. annotation, and Hypothes.is vs. Perusall comparisons, this study also invokes A/B testing. This has grown in popularity as a research method not only in massively open online classes (Chen et al., 2016; Renz et al., 2016), but also when testing two instructional approaches with the same or similar student populations, such as comparing two versions of open eTextbooks for readability/user perceptions of quality (Kimmons, 2021), comparing two learning management systems for accessing the same course content (Porter, 2013), or comparing different question formats for impacts on learning (Van Campenhout et al., 2021). Open source A/B testing platforms for education have recently been embraced by major philanthropic foundations (Carnegie Learning, 2020), as a way to aid decision-making surrounding educational practices.
Traditional Assessment Template
In the traditional reading assessment template, for each of the assigned papers, the students filled in the following information/answered the following questions:
• The dates that the paper was read.
• Can you think of an alternative/improved title for the paper?
• What were the first 5 terms that you had to Google? [give a 1–2 sentence description for each].
• What questions do you have related to understanding the paper?
• What questions do you have that could serve as future experiments?
• What other papers could have helped with the understanding of the current paper? (a means to indicate reading breadth around a particular research topic; students give references to these papers and brief summarizing information on the template).
and,
• Analyze all figures regarding:
◦ What technique(s) is(are) being used?
◦ What is the main purpose of the figure/what did the researchers find?
This template was considered to be an established form of assessment in a course focused on improving the understanding of primary scientific literature, and is similar to other reading templates referenced in the introduction in that it focuses on figure interpretation, airing student understanding/misunderstanding, and possible future lines of inquiry. It is also included in Supplementary Material, for any instructor to use or adapt.
Annotation Platform Usage
Students were briefed regarding online annotation platform usage and made simple trial annotations when the platforms were first introduced, in order to ensure they could access the articles, highlight and then annotate a piece of text. None of the trial annotations were counted as student output. Examples of annotation from previous students were shared in the briefing so the current students could envision what a collective annotation process entailed. The examples included students posing and answering questions, commenting on annotations of others to agree/disagree/add nuance, adding links to other articles to aid in comprehension, defining key terms, adding diagrams, adding tags, pointing out shortcomings or missing controls in the article, and suggesting future lines of inquiry.
Students were given a guideline of making five annotations per paper, and were given a rubric from Miller et al. (2016), that the instructor was also following for grading individual annotations. Each annotation was scored 0, 1, or 2 for quality (0 = no demonstration of thoughtful reading of the text, 1 = demonstrates reading of text, but only superficial interpretation, 2 = demonstrates thorough and thoughtful reading and insightful interpretation of the text). There were no pre-existing annotations made by the instructor, so all the annotations were initiated by the students. However, the instructor did occasionally participate in annotation threads to answer a question, clear up a misconception, etc., as was a normal occurrence outside of a research setting. When classifying threaded vs. isolated annotations, instructor comments in threads were excluded. For example, if a thread of 6 annotations had 2 instructor annotations, and 4 student annotations, the length would be counted as 4, and those 4 annotations would be considered to be part of a thread. An annotation with no other student additions is counted as isolated. An annotation with one instructor addition is still counted as isolated if there is no student follow-up after the instructor addition.
In prior studies using student annotation, some instructors gave a weighting of 6% per annotated article (Lee et al., 2019), or 15% of an overall course grade in an undergraduate physics course (Miller et al., 2016). In prior studies using templates, analysis of a paper via the Figure Facts template counted 10% for each paper analyzed (Round and Campbell, 2013). Since the students were expending a considerable amount of effort in reading long and technically challenging papers, the assessment of each paper in this study, either via traditional template or by annotation, carried a 10% weighting in the final course grade.
To sum up the major attributes of the annotation process in this study according to an existing typology of social annotation exercises (Clapp et al., 2020), the annotations were asynchronous as opposed to synchronous (students annotated on their own time); unprompted as opposed to prompted (other than the number of annotations [five] and the shared grading rubric, no specific requests were placed on annotation content); authored as opposed to anonymous (students knew the identity of their classmates making the annotations, and could use the identity in @call outs); and finally, marked as opposed to unmarked (the annotations counted toward course credit). This typology can serve as a useful comparative tool for future collaborative annotation research.
Distinctions Between the Annotation Platforms
The students accessed the Hypothes.is platform as a web browser plug-in. URLs to all the articles for Hypothes.is annotation were given to students through a learning management system module. Although Hypothes.is annotations have the potential for an internet-wide audience, the class grouping for Hypothes.is limited annotation to only the students of the class and the instructor. Public visitors to a particular article’s URL could not access the student annotations because they were not part of the student group. The students accessed Perusall as a stand-alone online platform with a code given by the instructor. Again, the annotations were only available among the students of the course and the instructor. Perusall annotations are generally limited to a course or subgroup within a course.
When this study took place, one could annotate text or a part of a figure with Perusall, but only text annotation was possible with Hypothes.is. Perusall also included some social functions such as student avatars which would let one know when someone else (student or instructor) was also using the platform at the same time, the ability to “upvote” an annotation (express agreement or support), automatic labeling of annotations that were phrased as questions, emoji icons, @student call outs, which will alert someone that they have been mentioned in an annotation, and email notifications for annotation responses. Hypothes.is included tagging and email notification of annotation responses, but did not have the other social-type functionality. Perusall has an additional machine learning capability for grading annotation output in large enrollment classes, as well as a “confusion report” to assess major areas of student confusion, but these were not used and thus not evaluated in the current study.
Survey Questions for Annotation Platform Comparison, and Annotation vs. Traditional Template Comparison
At the end of the academic year, students were given a voluntary, anonymous survey prompting comparison of the collaborative online annotation process to the traditional reading assessment template, and comparison of Hypothes.is to Perusall. For the 2019–2020 cohort, the survey completion rate was 15 out of 18 students. For the 2020–2021 cohort, the survey completion rate was 19 out of 21 students. The overall survey completion rate for all participants was 34/39, or 87%. Survey questions were as follows:
1. Compared to the MS word templated approach, did you find the annotation platform a better or worse tool for your learning of the biology content and experimental procedures in each paper?
2. Which annotation platform, Hypothes.is or Perusall, did you prefer, and why?
3. What did you like about the Hypothes.is platform?
4. What did you dislike about the Hypothes.is platform?
5. What did you like about the Perusall platform?
6. What did you dislike about the Perusall platform?
7. Did you feel that the guideline of 5 annotations per week, with the supplied rubric, was enough guidance in the annotation process? Why or why not?
8. Identify a useful annotation that you came across. What was it about the annotation that made it useful?
9. Identify a useless annotation that you came across. What was it about the annotation that made it useless?
10. How could the annotation platforms and related teaching and learning processes be improved (i.e., features, workflow, teacher prompts, etc.)?
The survey data is available to the reader in full in Supplementary Table 2, as an unedited student-by-question matrix (Kuckartz, 2014). Categorization of responses for the first two survey items was straightforward, falling into only three categories (Question 1—annotation preferred, template preferred, or no clear preference; Question 2—Hypothes.is preferred, Perusall preferred, or no clear preference). More than three categories were needed to adequately summarize responses for items 3–10, and owing to space constraints in this manuscript, those can be found in Supplementary Table 3. A few responses were uncategorizable, and occasionally, some questions were left blank by a student. Representative responses for each survey question are included in the body of the paper, with some occasional light editing for clarity. The words “article” and “paper” are used interchangeably throughout.
Annotation Output Analysis and Figure Generation
Quantitative student annotation output measurements included:
i. The number of annotations made per student per paper (how many times does a student annotate?)
ii. The annotation character volume per student per paper (how much do students contribute in their body of annotations for a given paper?)
iii. The annotation character volume per student per annotation per paper (how much do students contribute in each individual annotation?)
iv. The individual annotation quality, as assessed by the course instructor according to the rubric of Miller et al. (2016)
v. Whether annotations were isolated (defined as one solitary annotation) or part of a collaborative thread (defined as two or more annotations)
Anonymized student annotations are available on a student by student basis and on a threaded basis for each cohort and platform as excel files in the Harvard Dataverse. The anonymization replaced names with numbered student labels (student 1, student 2, student 19, etc.). Annotation of one paper was missed by two students (student 19-paper V, student 33-paper Q) in the 2020–2021 cohort due to excused medical absences, so means are calculated for those students with an accordingly adjusted denominator. Character counts were taken for annotations as they appeared, with the name substitutions. If a student typed in a URL or DOI in their annotation, it is included in the character count. If a student included a hyperlink in their annotation, the URL was extracted and placed in a separate column in the excel analysis file, but not counted toward the character length. This approach preserves the links to other resources made by students, but treats the annotation content with as little manipulation as possible. Repeating the character count analyses with URL and DOI text excluded, did not affect any conclusions regarding platform differences (or lack of differences) in annotation output character volumes. Emoji characters in annotations have also been preserved, but were used sparingly by students. Data analysis was performed using a combination of MS Excel and Graphpad Prism 9 software, and figures were generated using Biorender.com (Figure 1), MS Word (Table 1 and Supplementary Tables 1–3), or Graphpad Prism (Figures 2–5). The number of observations for (i–iv) depended on student cohort size: 2019–2020 cohort (n = 18), 2020–2021 cohort (n = 21), or with the students from the two cohorts combined (n = 39). T-tests for comparing means are paired (Hypothes.is mean vs. Perusall mean) and p values are indicated on the graphs or bar charts. For threaded annotation observations, a threaded vs. isolated percentage was measured for annotation output on each paper, thus there are only four observations (four papers) for a paired t-test within a cohort to compare platforms. Readers should consider whether the difference in means, or data trends in the charts, could be pedagogically significant in their classrooms, along with any consideration of the mean comparison p value. No comparisons for annotation counts, character counts, or annotation quality were undertaken between specific papers, rather the analysis focused on differences between the two annotation platforms. The effect of an individual paper is indeed diluted, as means across four papers for each student within each platform were used to obtain annotation number, character volume, and annotation quality scores which then fed into the comparison of the platforms.
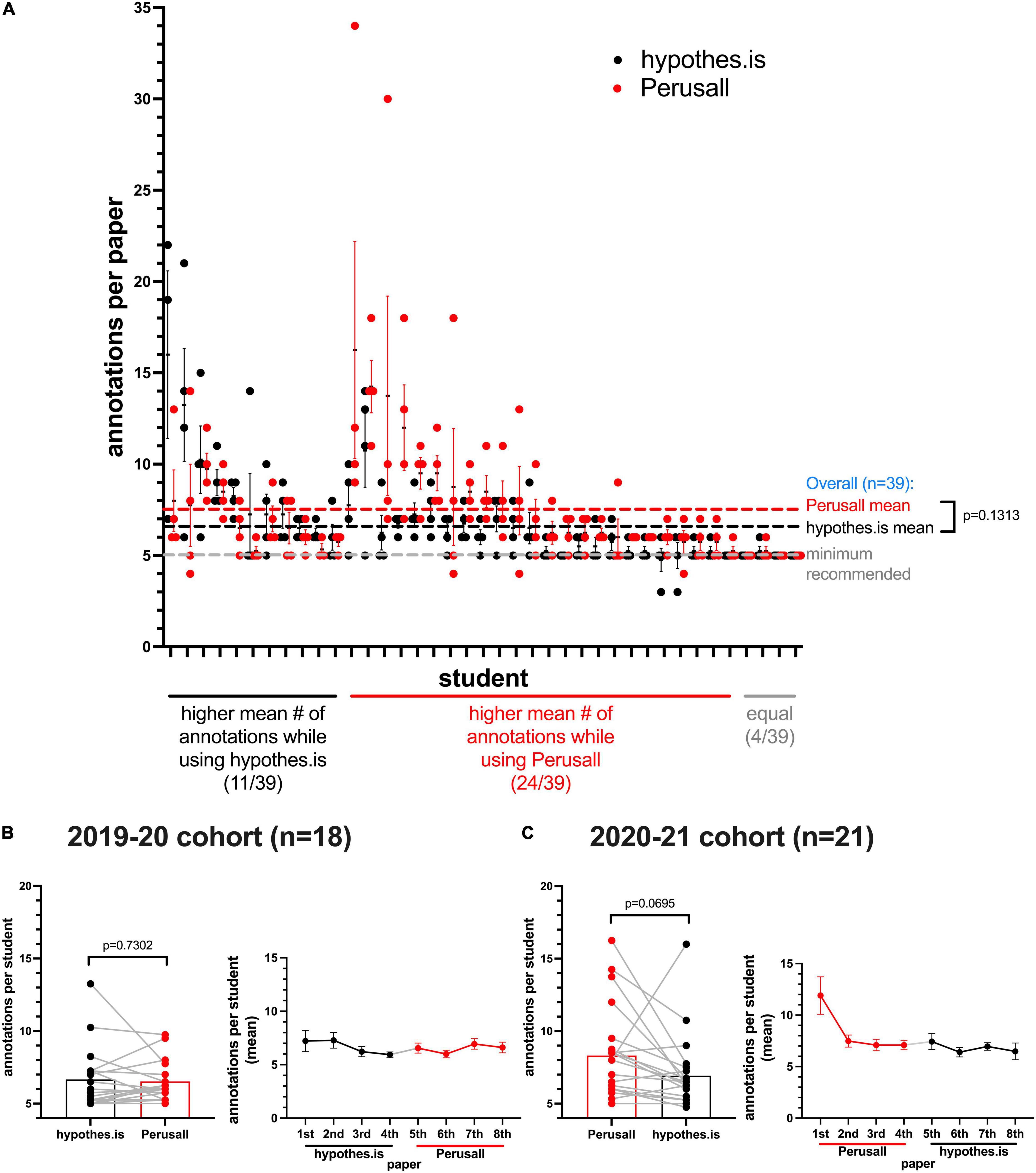
Figure 2. Most students exceed the instructor-stipulated annotation requirement. (A) Each data point represents the number of annotations made by a student for each paper. The X-axis is organized to group students together according to platform output means being higher on Hypothes.is, or Perusall, or equal. Lines next to data points indicate standard error of the mean (SEM) of the measurement for each student. Dashed lines indicate the global means for all students, or the minimum stipulated number (5). (B) 2019–2020 cohort (18 students), and (C) 2020–2021 cohort (21 students) mean number of annotations per student (from the four papers on each platform), along with pairing (gray lines) to indicate an individual student’s output on each platform. To the right of each bar chart is a timeline tracking the mean number of annotations per student from the first to the eighth paper annotated, error bars: SEM.
Results
Student Survey Responses
Key Comparisons: Template vs. Annotation, Hypothes.is vs. Perusall
Students strongly favored the collaborative online annotation process compared to the traditional paper analysis template (Table 1, Q1). 25/34 students (∼74%) felt that the online annotation process was a better content-learning tool compared to the traditional template. Only 6/34 students (∼18%) preferred the template, and 3/34 (∼9%) students had a mixed response with no clear preference for either process.
Those in favor of the online annotation approach indicated that looking through the annotations brought new insights based on the thinking of others, and enabled interaction that was not possible with the traditional reading template.
The annotation platforms were a better tool than the template approach. Having to read through it and analyze it myself, and then re-synthesize it with other people’s comments forced me to go back to the paper more than once and dive in.
Annotation was much better than the templates. Promotes critical thinking and importantly, discussion. With the templates I would never even think about some of the things my classmates bring up.
The power of the annotation platform lays in its capacity to serve as a collective real-time inter-phase in which one can comment, review, and interact with other students. This enables a deeper conversation with respect to questions, concerns, or the analysis of a particular piece of discussion, figure, experimental methodology, and is as a result superior to conventional note-taking which is static by nature.
I thought that the annotation platforms were a lot more helpful because I could see what other students were saying and it wasn’t just my ideas. I felt like I did a lot more thinking when I read the threads of other students.
For those in favor of the traditional template approach, they felt that it prompted a more complete and thorough analysis of the paper, because each figure had to be analyzed.
I personally preferred the templated approach, although it was more difficult and took up significantly more time. It caused me to examine each figure in a lot more detail. With the annotation platforms, it was much easier to “slack off.”
I think the template was better. It gave me a framework for how I’m supposed to learn from and critique a paper. I still follow the template even when I have to use the annotation platform.
Preference between the platforms had a relatively even split (Table 1, Q2); 14/34 students (∼41%) preferred Perusall, 12/34 students (∼35%) preferred Hypothes.is, and 8/34 students (∼24%) indicated no clear preference for either platform.
Remaining Qualitative Survey Data
Specifics for Platform Preference: Commentary on Hypothes.is
Students commented favorably on Hypothes.is regarding its simplicity in using the annotation window, overall reading experience with the article being annotated, and having less log in prompts.
I liked Hypothes.is because of its inherent simplicity. You annotate and/or highlight a particular section, can see any replies immediately underneath the annotation, and can in turn can click within the text or within the annotation to go back and forth between the text and comment of interest.
Hypothes.is, because it’s much easier to locate the annotations. In Perusall we have to click on each highlight to navigate to the specific annotation.
Hypothes.is was easy to see all the threads and I didn’t have to login every time I wanted to access it.
I liked in Hypothes.is how hierarchy can be established within a thread of comments when necessary. You can reply to any comment in a particular thread and the system will make it clear which comment you are responding to by adding an indentation before your reply. That makes annotations very neat and organized.
Students disliked Hypothes.is for the inability to annotate figures, and the lack of an upvote button. Some viewed the plug-in nature of Hypothes.is as a positive, because it brought them directly to the paper’s URL, while others viewed this as a negative because they were accessing Hypothes.is through Google Chrome, which was not their preferred browser. As a change from when this data was collected, it appears that Hypothes.is now supports all browsers with the exception of Internet Explorer.
Hypothes.is has fewer functions in the comment section than Perusall.
I wish Hypothes.is had an upvote/question button for my peers’ responses.
I definitely preferred the website nature of Perusall over the plugin of Hypothes.is.
It is less interactive than Perusall, like marking annotation as helpful or marks it as when I have the same question.
There was not a graph annotation function like Perusall, which made it more difficult to annotate figures. The plugin format of Hypothes.is was a bit hard to figure out at times.
I don’t see an option to upvote or mark my favorite annotations. I once clicked on the flag button at the bottom of someone’s annotation and I then realized that it reported the annotation to the moderator, as if there is something inappropriate. That’s not what I meant to do and I couldn’t undo the “reporting.” That was pretty awful.
I do not like how I have to download it and use it as a Google Chrome (not my favorite browser) extension. I also dislike how I cannot label the figures directly—can only highlight text.
Specifics for Platform Preference: Commentary on Perusall
Students favored Perusall’s allowance for annotating images, annotation up-voting, the more social feel and appearance, seeing the presence of other students, and the ease of access via one online platform with all course article PDFs present.
I preferred Perusall over Hypothes.is. It seems like a more user-friendly platform, it allows inserting images (and emojis!) and has good filter functions (e.g., for unread replies, comments by instructor etc.).
Perusall seems to be a more well-polished annotation tool. You can temporarily hide other people’s annotation and focus on the paper only, which gives you a cleaner environment.
Preferred Perusall because it is easier for me to access the papers. With Hypothes.is, I often have to switch from Safari to Chrome or vice versa before it lets me view the paper. Also, with Perusall, I get to draw a highlighted box for annotating figures, with Hypothes.is, I can only highlight text.
I personally preferred Perusall because we can annotate on a figure by highlighting the image and also upvote the threads we think are particularly good.
I like the organization of the Perusall platform, specifically the page by page conversation tracking as well as the up-voting feature.
Perusall’s interface reminds me of social media a bit more than Hypothes.is, which is at least refreshing when going through what could be difficult material.
There is no confusion about what link to follow to annotate the paper because it’s already uploaded. It’s easy to see each person’s annotations because they’re color coded and you also get to see who’s online. You can react to people’s annotations.
Student dislikes of Perusall included problems with highlighting text, having to do frequent sign-ins when accessing the platform, occasional inability to read both the paper and the annotations simultaneously on their screens, and the lack of being able to use the tool themselves for independent study groups.
I always had issues highlighting discrete pieces of the text, whereby my highlighter function would inadvertently highlight an entire page but not allow me to highlight a particular sentence or words.
It’s quite difficult to see the content of annotation without clicking on the highlight. Also can’t download the paper directly from the Perusall platform.
It was hard to have the comments section open and be zoomed in on the paper enough to read it. Additionally, there were a few times where comments would get put on top of each other by different students and the comment that was placed first was not seen.
One suggestion I would make is that I hope I can upload papers myself and set up study groups. I tried to discuss one of the papers with just a small group of students but unfortunately I could not do that.
The final comment is suggestive of the utility of collaborative annotation outside of an instructor-guided setting (i.e., student study groups, group project collaborations).
Instructor Guideline for Numbers of Annotations Made
The majority of students (70%) found the guidelines regarding the number of annotations to be sufficient, although they could perceive the arbitrary nature of the guideline in that five or even fewer annotations could be lengthy and insight-rich, while a higher number of annotations could be terse and not provide as much insight. The annotation number stipulation is straightforward and easy for both instructors and students to keep track of which is likely one of the reasons that it is commonly used as either a guideline or output measurement (Miller et al., 2018; Lee et al., 2019; Singh, 2019).
5 annotations are more than enough. I think the nature of the platforms naturally enforce conversations and interactions, that in my mind without thinking about it, usually go beyond the suggested 5 annotations.
Yes, I think five annotations are a good amount. But maybe some clarifications on how long those annotations should be. Since some students have five really long annotations, and some have 10 short annotations. Otherwise, the guidance is clear.
I think so; the real power of these guidelines came from the variety of annotations that were given by each of the students. Between background information on methods, critiques of the authors’ analysis, and questions about the scientific background of the papers, I felt like the five annotations per week were sufficient for a robust conversation.
I think the guideline was reasonable. As a suggestion, perhaps the assignment could include introducing a certain number of comments as well as responding to other comments. The goal of using an annotation platform vs. the template is to encourage discussion with other people.
Which Annotations Were Most Useful?
Annotations explaining some aspect of the source text were the most frequently mentioned as useful in the survey (40% of responses). Not surprisingly, the students also found value in annotations encouraging dialogue and raising additional concerns and questions. Corrections from either the instructor or other students, and linkage to other applications or course content, rounded out the categories for useful annotations.
We have seen several times circumstances where multiple people will enter into a conversation and we end up with a whole thread of annotations. I think these can be extremely helpful and also just make reading the paper more interesting. Especially when people argue about a certain point, as getting to see people’s arguments often helps to better understand the author’s motivation behind doing something a certain way.
Corrections from other students and the instructor if I misunderstand something. Connections between the paper we are annotating with lecture materials or other research papers.
Annotations that synthesized something from the paper and then asked a question about it. What I appreciated was that it was sometimes a comprehension question (why would they use X method, not Y?) but sometimes it linked to outside ideas or thoughts (would this translate to Z?).
Sometimes I come across annotations that describe a method, and those are helpful because they make it easier to understand the results. However, this only applies to annotations where someone took the time to make a clear and concise annotation rather than copy-pasting a description from a webpage or linking a paper.
Which Annotations Were Useless?
The most frequent response for this question in the survey was that there was no such thing as a useless annotation (31%). Students placed less value on the re-stating of anything obvious, terse agreement annotations, or information that was easily found through an internet search. They favored annotations that were dialogic. There were some differences in opinion in regards to definition-type annotations; for some they made the reading process easier, while others viewed definitions as a dialogic dead-end and something that they can easily obtain on their own.
Some annotations were superfluous in nature and defined terms and or processes that were canonical and did not need a one paragraph explanation.
Definitions—especially in the beginning were very frustrating. There is no response to them, they don’t make you think any more or differently about the paper.
I dislike annotations that only link to another paper, like ‘Excellent review article (link). What is it about the review article that makes it excellent? What did the student learn from that review article? What about the review article complements this specific paper? Just even a single sentence would be a big improvement.
Annotations that describe straightforward results. Like if there is a graph that shows that some parameter increased with a treatment, then an annotation stating just that is useless. If the annotation links it to the other results and explains the conclusion, that’s useful. However, it shouldn’t be too long and convoluted.
I can’t remember any useless annotation I have come across. I don’t think there is or can be any useless annotation—I think what may seem obvious to one may actually be something that is completely missed by another.
Further Pedagogy-Related Feedback From Students
In the survey responses, students are thinking of ways to operationalize dialogic feedback and achieve “revisits” to the platform after an annotation requirement has been fulfilled. Some students were daunted by the vast amount of annotations on a given paper in a group of approximately 20 students and one instructor annotating. Reading the full body of annotations is a fairly large time commitment for the students, who would also spend a great deal of time reading the content of the paper itself.
I feel like having your recaps in class helps, because I rarely read all of the annotations, or feel overwhelmed doing so.
I think at times there were just too many comments on a paper. It became a race for people to read and annotate the papers early so there was enough left to comment on, without being repetitive. If I was late to the game, sometimes it was easier to just read the comments and find an outside/related subject to Google and link to instead of reading the paper and really thinking about it. I think lowering the number of comments we need to make would help with that.
What happens a lot with me and some of my friends is that by the time we’re done reading and making our annotations, someone else on the platform has already commented what we wanted to say. Then it becomes stressful to think of new things just to stand out. I feel like commenting “I had the same question” on another person’s comment makes it seem like I was lazy and didn’t read the paper when in fact I really did have the same question.
I think it would be interesting to assign different figures to different groups of students, it might allow for more in depth critique of certain sections. Additionally, it would be an opportunity for students to work in groups and get to know each other.
Again, I like the “back and forth” discussions in the annotation. It is like a debate in the annotation form. I think I’ve seen too much “I agree” (though I used it a lot, too). We might be better off to give contrary opinions and then defend each other’s view using lecture or outside source knowledge. I’m sure we’ve all come across some annotations to which we hold completely different opinions. For these annotations, after we’ve given our feedback, I’d expect the other people to defend their ideas too.
I think that perhaps there could be an incentive provided for people to actively go back to the platform (after they made their annotations) to discuss with people who annotate after them—perhaps like extra marks? Because once one makes their annotations, there isn’t really a need for one to go back and “interact.” So perhaps this would encourage more interaction? but I also feel that this may lead to “flooding” of annotations.
The annotation platforms have adopted technical solutions to enhance returns to the platform via email notifications when one has been tagged in an annotation, or had their annotation further commented on. Additional return incentive could be built-in pedagogically by the instructor, perhaps encouraging responses in dialogic threads, or suggesting that while a certain number of responses can be “initiative” (start a new thread), other responses should continue from an existing annotation to make a constructive dialogic thread. Assessment routines could perhaps be shifted away from individual annotations and toward the overall quality of collaborative threaded contributions.
Students suggest some prompting such that all of an individual’s annotations are not directed in one section of a paper, instead being divided among introduction, methods, results, and discussion sections. Teacher prompts taking the form of “seed” annotations could also guide students by superimposing a templated approach onto the annotation approach, if certain seed annotations are regularly included (i.e., Are the researchers missing any controls? Do the conclusions feel supported by the existing data?). In another study, anonymous seed annotations generated from a previous year’s more intriguing threaded discussions, had future value to prompt better annotation quality and more elaborative-generative type of threads in a subsequent class (Miller et al., 2016).
Teacher prompts could be helpful, though I also worry that then students may focus on answering just those prompts and not branching out to really critically analyze the rest of the paper.
I think it worked really well overall, however, it would help to have more guidance/requirements on the types of annotations students should be leaving. Annotation platforms make it really easy to “skim’ the paper, rather than really read into it.
Simply writing five annotations would be very generic. It may be better to restrain, for example, one annotation at least to comment on a new term that wasn’t familiar to you before, three annotations at least to comment on the results/experiments, and perhaps one annotation at least to comment on the biggies (significance? results sound or not? future directions? etc.).
Since the body of annotations can grow to a large size in a group of 20 students, the notion of going to upvoted responses might be a way for students to consume the annotations more selectively. The upvote button on the Perusall platform should help to limit sparse “I agree” type annotations, as the upvote accomplishes the same function. However, there was some concern that threads or comments that were really insightful did not get upvotes, whereas some threads that were viewed as not being particularly helpful did receive multiple upvotes. This is an area where instructor curation and recaps are needed to prevent the loss of quality annotation work from student consideration.
On Hypothes.is, I can’t see which comments get the most “upvotes” or “likes.” Sometimes I don’t have the time to read through every comment, but it’d be helpful to look at comments that were most helpful to a lot of students.
I read some really thoughtful Perusall annotations from other people that didn’t get upvoted. I also read some less thoughtful Perusall annotations from other people that got relatively heavily upvoted.
Quantitative Data: Annotation Output for Hypothes.is vs. Perusall Platforms
Instructors getting started with a collaborative annotation platform may look toward quantitative metrics suggestive of student engagement. Perhaps the platforms themselves will come up with more sophisticated indicators, but some basic usage indicators that are easy for an instructor to grasp include: the number of annotations made by a student (How often does it meet or exceed instructor-stipulated minimum?); annotation character volume per student per paper (Has a student contributed sparse or more lengthy content in their annotations for a paper?); annotation content quality; and the degree to which annotations are isolated (did not receive any further response) or threaded (received at least one response). Looking at these metrics over time, as students progress from one paper to the next, and then from one platform to the next, may also be beneficial to gauge overall student progress.
Number of Annotations per Student per Paper
In considering each data point in Figure 2A, representing the number of annotations made by a student on a given paper, the vast majority of students exceed the five annotation instructor stipulation on a consistent basis. Only 4/39 students consistently adhered to the minimum recommended number. This exceeding of the instructor-stipulated minimum is in line with a study by Lee et al. (2019), where a mean number of 16.4 annotations per student per paper exceeded a 12 annotation stipulation. 24/39 students had more annotations per paper using Perusall, while 11/39 students had more annotations per paper when using Hypothes.is. 4/39 students annotated to the same degree using both platforms, likely out of habit of sticking to the minimum stipulated annotation number. In considering the output from both cohorts (n = 39), the mean number of annotations per paper per student using Perusall (7.49, SEM:0.427), was higher than the mean number of annotations per paper per student using Hypothes.is (6.81, SEM:0.375), although the difference was less than one annotation per paper, not statistically significant, and unlikely to be pedagogically significant for reasons mentioned previously.
In Figures 2B,C, means for the number of annotations made per paper were similar when comparing output on the two platforms for either cohort. The mean number of annotations made per student per paper stayed relatively stable over time as the students progressed within and between annotation platforms in the 2019–2020 cohort. There was an initial high activity on the first paper annotated for the 2020–2021 cohort, which then stabilized between 6.5 and 7.5 annotations per paper, similar to the 2019–2020 cohort. An abnormal initial output makes sense, if one considers that students are adjusting to the platforms and may not yet have a good sense of output norms among their peers.
Character Volume in Annotations
In Figure 3A, 28/39 students had higher annotation character volumes per paper using Perusall, while 11/39 students had higher annotation character volumes with Hypothes.is. In combining data from the two cohorts (n = 39), the overall mean for Perusall was 3,205 characters per paper (SEM: 206), and 2,781 characters per paper (SEM: 210) for Hypothes.is (p = 0.0157).
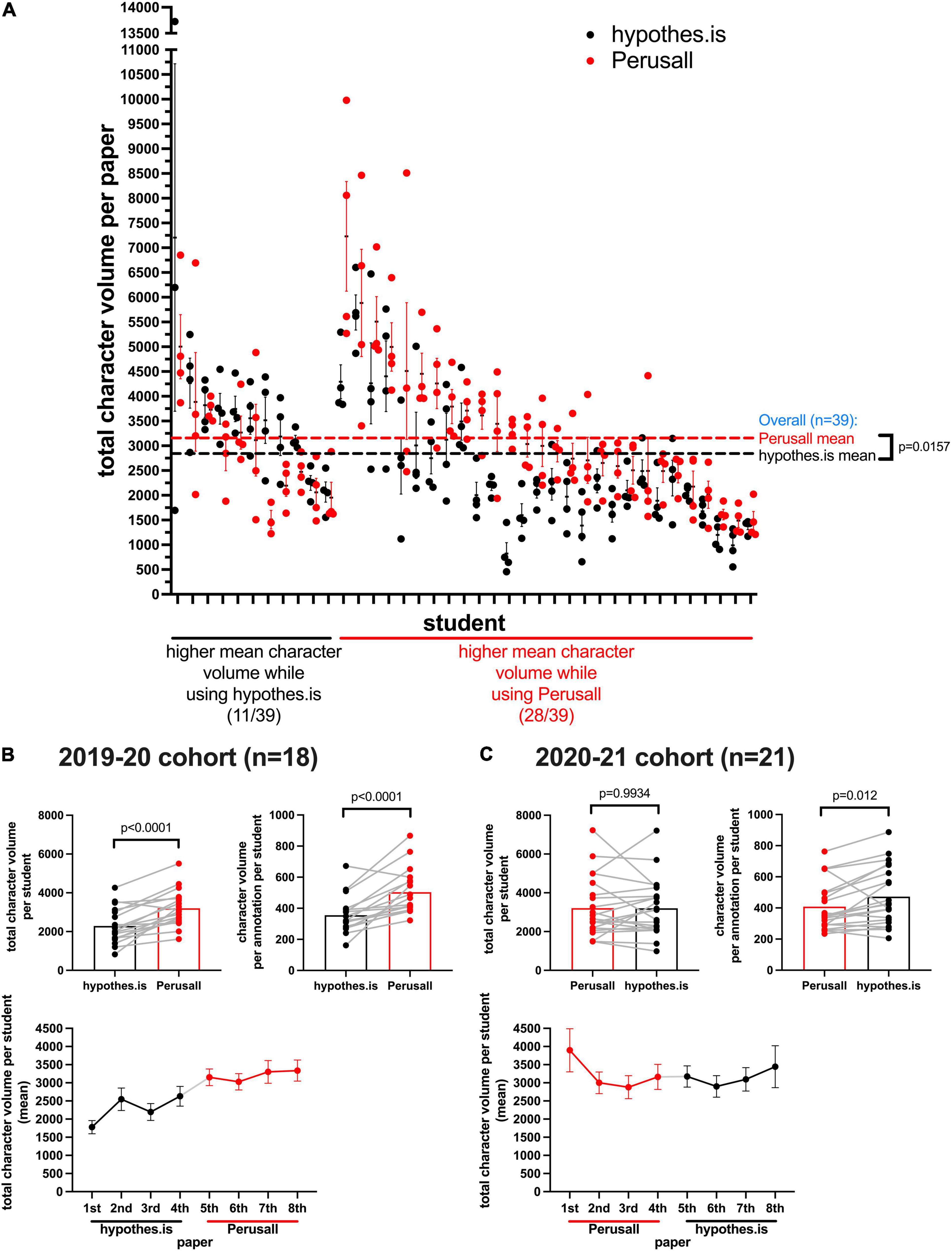
Figure 3. Character volume in annotations. (A) Each data point represents the total character volume within all annotations made by a student for each paper. The X-axis is organized to group students together according to platform output means being higher on Hypothes.is or Perusall. Lines next to data points: SEM. Dashed lines indicate global means. (B) For the 2019–2020 cohort (18 students), and (C) the 2020–2021 cohort (21 students), the mean total character volume for all annotations per student (from four papers on each platform), and mean character volume per annotation are indicated, along with the pairing (gray lines) to indicate an individual student’s output on each platform. Below the bar chart is a timeline tracking the mean total character volume of annotations per student from the first to the eighth paper, error bars: SEM.
In Figure 3B, the 2019–2020 cohort had a higher mean total character volume output per student per paper for Perusall (3,205, SEM:220), than for Hypothes.is (2,287, SEM:215) (p < 0.0001). They also had a higher mean character volume per annotation for Perusall (503, SEM:19.7), than for Hypothes.is (355, SEM:17.7) (p < 0.0001). This cohort showed a steady increase in character volume output per student over time.
In Figure 3C, there was no significant difference seen in mean total character volume for the 2020–2021 cohort between the platforms, although Hypothes.is had a higher character volume output per student when looking on a per annotation basis (p = 0.012). Mean character volume output per student over time was steadier and did not show the same consistently rising pattern as the 2019–2020 cohort. One potential explanation is that the user interface or social nature of the Perusall platform encourages a higher output and this inertia remains when the students transition to Hypothes.is.
Annotation Quality Scores Increase Over Time, Regardless of Platform Order
In keeping with prior studies (Miller et al., 2018), individual annotation quality was generally quite high, with the vast majority of annotations scoring full marks (two out of a possible two in an individual annotation). Figures 4A,B, indicate a decrease in low scoring annotations (0 or 1), as the students go from the first to the second annotation platform. Figures 4C,D indicate an increase in mean annotation quality score as students progressed from one platform to the next, regardless of platform order. Mean annotation quality score for the 2019–2020 cohort went from 1.70 to 1.84 from the first to the second platform (Hypothes.is to Perusall) (p = 0.0176). For the 2020–2021 cohort, mean annotation quality went from 1.57 to 1.71 from the first to the second platform (Perusall to Hypothes.is) (p = 0.011). In considering progression over time for annotation quality in Figures 4E,F, there was some fluctuation on a per paper basis, but the trends indicate an improvement from the beginning to the end of the annotation exercise. This data combined, is consistent with a growing fluency with annotation practices and de-emphasizes any platform influence on annotation quality. It is reasonable to conjecture that different attributes of a platform may change student behavior, and this can be seen in regards to annotation lengths. Since both platforms enable an essential basic annotation function, student insight shines through and does not necessarily depend on annotation length. Thus, it is reassuring that the mean quality score measured per student globally (n = 39) was almost identical (1.71 Hypothes.is, SEM:0.04, 1.70 Perusall, SEM:0.05).
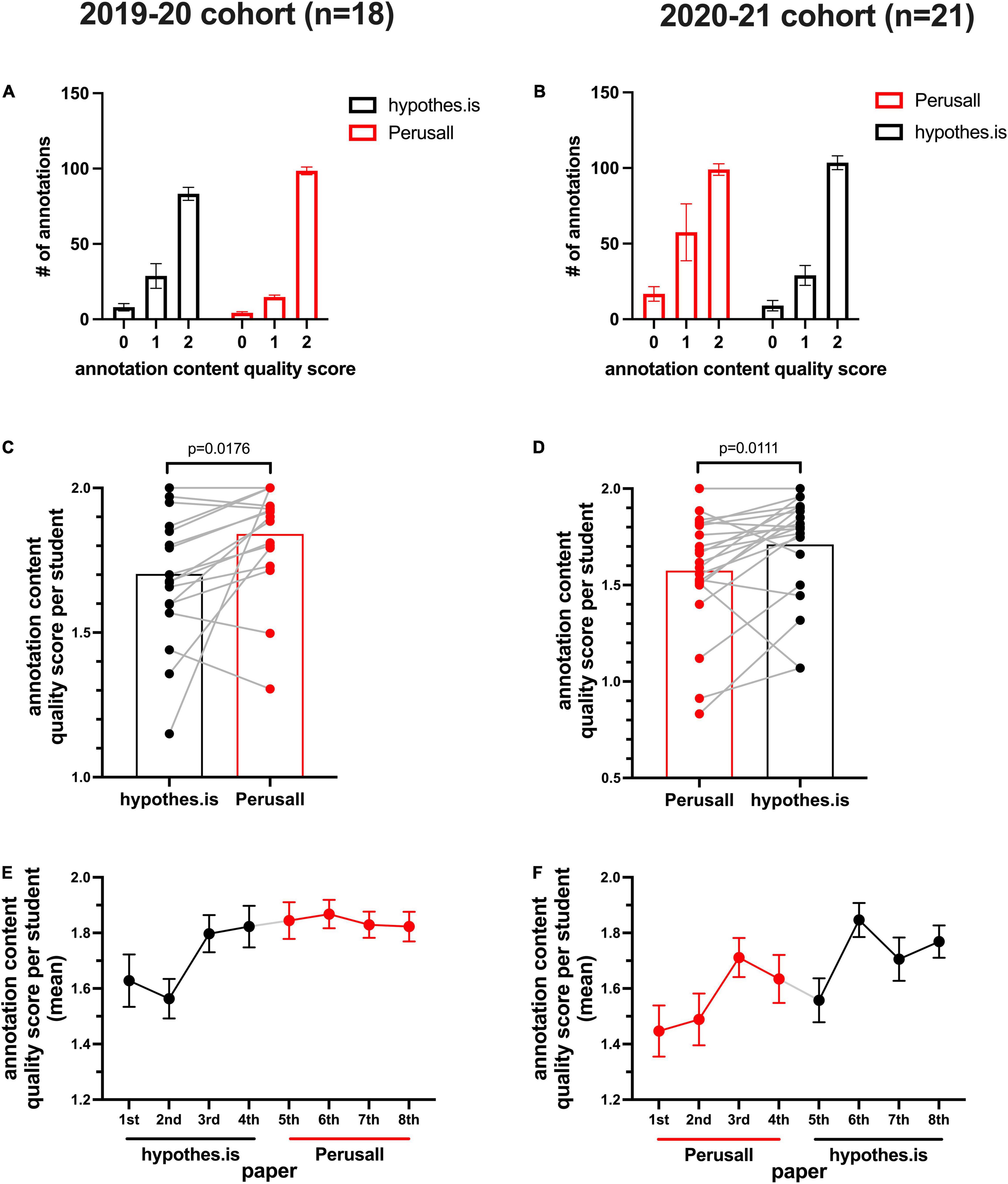
Figure 4. Annotation quality scores increase from the first to second annotation platform for each cohort, regardless of the platform order. (A) 2019–2020 cohort, and (B) 2020–2021 cohort, mean number of annotations scoring a 0, 1, or 2 (among four papers on each platform), error bars: SEM. (C) 2019–2020 cohort, and (D) 2020–2021 cohort, mean annotation quality score per student (among four papers on each platform), with the pairing (gray lines) to indicate an individual student’s score on each platform. (E) 2019–2020 cohort, and (F) 2020–2021 cohort, timeline tracking the mean annotation quality score per student from the first to the eighth paper, error bars: SEM.
Isolated vs. Threaded Annotations
Threaded annotations can be viewed as preferable to isolated annotations because they provide evidence that the initial annotation has been read and digested by the responder, and then spurred some dialogue for debate, additional nuance, or correction. In considering the percentage of total annotations that were isolated vs. those appearing in a thread, the only time that isolated annotations outnumbered threaded annotations was in the initial use of the Hypothes.is platform with the first assigned paper for the 2019–2020 cohort (Figure 5A). In all other papers, annotations that were part of a thread outnumbered those that were isolated. The 2019–2020 cohort showed a clear trend of increasing threaded annotations over time, and a higher mean of percentage threaded annotations in the second platform (Perusall, 80% threaded), vs. the first platform (Hypothes.is, 53% threaded) (p = 0.0108). The 2020–2021 cohort (Figure 5B) showed a relatively steady trend with a mean of ∼70% of annotations occurring in threads on each platform. The final paper annotated on each platform tended to have the highest percentage of collaborative annotations, again indicating an upward trend for dialogue.
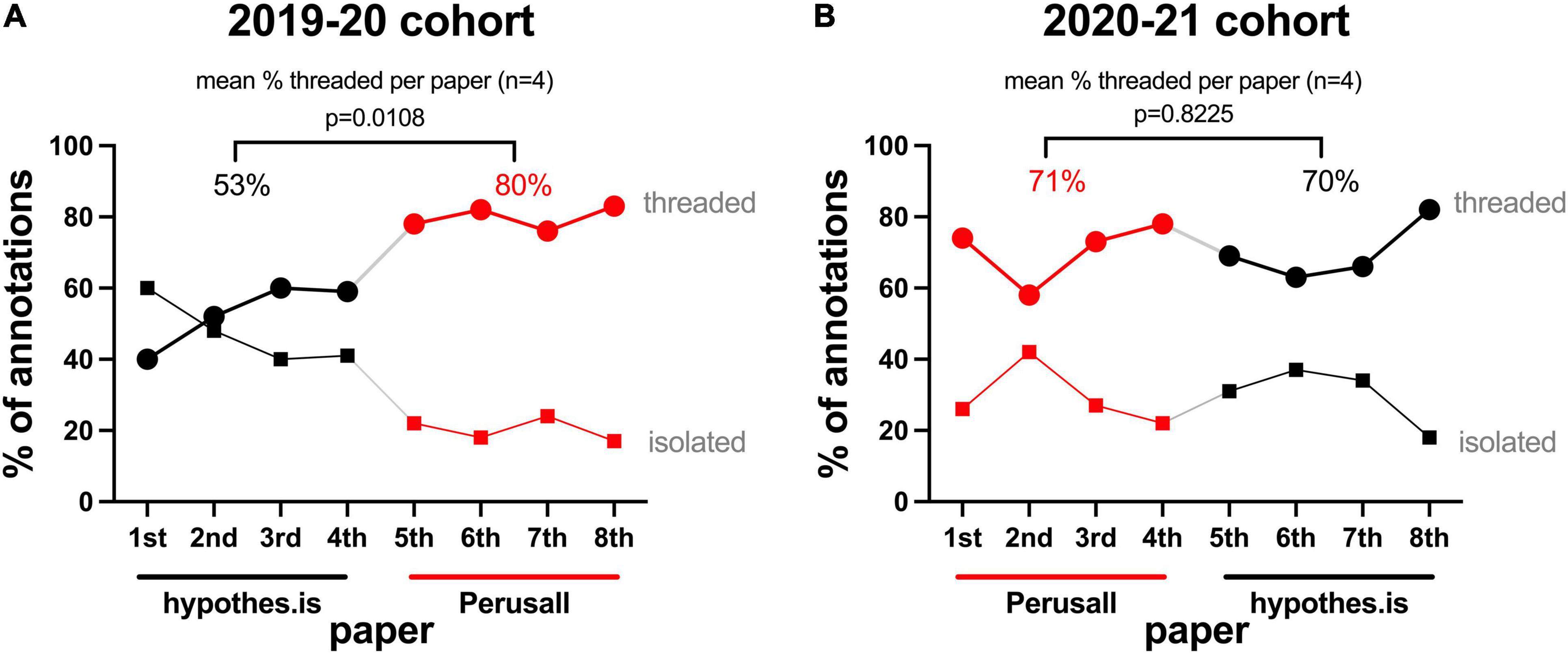
Figure 5. Annotations in collaborative threads over time. (A) 2019–2020 cohort, (B) 2020–2021 cohort, percentage of annotations classified as isolated (no further student responses) [squares], or accompanied by one or more responses (thread length two or greater) [circles] within a given paper. Above the graphs are the mean percentages of threaded responses among the four papers annotated on each platform within a given cohort.
Discussion
The trend toward an increase in the percentage of threaded annotations, and an increase in mean annotation quality scores over time is reassuring, as it suggests that even in a relatively unprompted setting, students have some natural fluency and become better annotators. This should be encouraging for both the annotation platform designers and for teachers considering a collaborative annotation approach in their courses. Prior studies have not followed the same student population from one platform to another, nor looked at output over time (threaded vs. isolated, annotation numbers, annotation character volume) within and between platforms. The quantitative analysis in this work provides a baseline upon which future quantitative studies on student annotation output can be compared or further built-upon in sophistication. The annotation character volume difference in the 2019–2020 cohort was in favor of output on the Perusall platform, which could suggest that social functionality of a platform may drive some additional engagement, however, that conclusion should be tempered by the data from the 2020–2021 cohort, which was more even. The survey data shows a slight preference for Perusall vs. Hypothes.is (41% vs. 35%).
Caveats and Limitations of Current Study
Since the class sizes in this study were relatively small (<25 students), the body of annotations for a weekly reading were still fully consumable by the instructor with the investment of roughly 4–5 h for reading, processing, grading, and engaging with a subset of those annotations. This does not include a thorough reading of the source document and the planning of the accompanying lecture, which took additional time. The reading time commitment for an entire body of annotations is perhaps even more daunting for students, as was indicated in some survey responses. With larger classes, one instructor may have difficulty managing the body of annotations, and if engaging with students on the platform, would likely be participating within a smaller percentage of the overall student body. Both platforms have the ability to divide a class into smaller subgroups. Perusall’s default setting is for groups of 20 students. If the readings are annotated in assignment mode, Perusall also has a machine learning capability to analyze a large body of annotations that could accrue with a large class, but this was not evaluated in the current study. Annotations of poor quality can contribute noise to the reading experience, and contempt for the annotator (Wolfe and Neuwirth, 2001). In this study, low quality annotations were a relatively minor concern, but could be a greater concern with larger class sizes, or for classes where some subset of students approach the source material in superficial way (i.e., required class outside of student’s main interests, unreasonable difficulty for students in grasping the source material, or desire to troll/abuse other students in the class). In sum, even though the annotation approach worked well in the current study and student population, problems could emerge with another population.
Since the Hypothes.is annotations were occurring on article PDFs hosted as webpages, annotations can be temporarily lost if the article URL changes. This occurred with one article from the 2019 to 2020 cohort, and one article from the 2020 to 2021 cohort. With some technical support from Hypothes.is, the annotations were recovered by using a locally saved PDF where an underlying fingerprint could still be recognized in order to show the annotations. Individual annotations can also become “orphaned” if the text they were directed to disappears from the source webpage. These are listed under another tab in the annotation interface, so are not lost from consideration. If students are annotating web content that is more dynamic with many source edits, then this could be more problematic. In Perusall, the source documents were uploaded PDFs, so the underlying text never changed.
Ideally, the same articles would have been assigned to each cohort (2019–2020 and 2020–2021), however, that was not possible, as the articles needed to relate to a seminar speaker series where the invited speakers change from year to year. Instructors should keep in mind that when students first use an annotation platform, they do not yet have an impression of group output norms, so one might expect higher or lower output on the first paper annotated. This can be seen in both cohorts in this study, as the 2019–2020 cohort had a particularly low character volume on the first paper annotated, while the 2020–2021 cohort had a higher annotation number and character output on the first paper.
The mean number of annotations per paper are surely influenced by teacher guidelines. If one used the platforms with no minimum stipulation, or had a minimum stipulation count of 10 instead of 5, student behavior is likely to change. Some portion of the motivation is driven by instructor stipulation and the grading of the annotations, another portion of the motivation is coming from genuine engagement with a thought-provoking point made by another student, a refutation of one’s annotation, or taking a conversation in an unexpected direction. One cannot be sure of the balance between these forces, but there is prior research indicating that even in ungraded settings, collaborative annotation still appears to engage students with class-associated reading (Singh, 2019).
In retrospect, being able to link identity for student survey comments to the same student’s annotation output would have enabled additional research questions to be asked (i.e., do students that favor one platform in their survey response also make more annotations/have a higher character volume with that platform?). As the surveys in this study were answered anonymously by students, this was not possible.
Finally, the functionality of the platforms can change over time. This is an unavoidable problem for research on any type of educational technology. Some issues mentioned by students may already be in the process of being fixed by the platforms.
Emergence of Annotation Best Practices
The major areas for the shaping of annotation best practices appear to reside in:
1. Scaffolding for students in writing more effective annotations.
2. Affordances of asynchronous participation.
3. Measurement of annotation across texts vs. within texts.
4. Large data set mining/learning analytics approaches.
As the annotation platforms are relatively new to the education technology scene, instructors are now starting to consider what scaffolding is needed in order for students to write high quality annotations. Work by Jackson (2021) parallels two of the qualitative survey prompts here, in that it asks for students to elaborate on what makes for good quality and poor quality annotations, in hopes that they will apply that reflection toward their own annotation output later on. It includes an excellent clarify-connect-extend annotation rubric (Jackson, 2021), which instructors might find useful in an initial briefing of the annotation process for their students, or for remedial tune-ups for those who are contributing less than ideal output.
Asynchronous discussion allows for preparation and analysis not only for students, but also for the instructor. For example, in synchronous situations, the instructor cannot typically ask a student to wait for an hour for a reply to a comment/question, in order that the instructor can go read another article and make a more nuanced and accurate comment. Yet with an asynchronous approach, this is possible. Although one often thinks of how to motivate students, these asynchronous approaches provide a buffer of time that can motivate further engagement from the instructor with the source text or with other related materials. On the other hand, tardy feedback (>2 weeks after an assignment is completed) is detrimental to the feedback’s value and impact (Brown, 2007). With the annotation platforms in the current study, follow-up on student annotations occurred on the order of hours to days, well within the period of significance for feedback usefulness.
Annotation across various texts vs. within a given text both yield valuable information (Marshall, 2000). A student’s annotations across various texts during a semester, or during a degree program, could give some indication of intellectual growth over time. The body of annotations within a given text could provide an important indicator for instructors regarding engagement levels for an assigned text, with the assumption being that a text with a high volume of threaded annotations is more conducive to debate and collective meaning-making by the students than a text with a low volume. This may provide a signal for what reading should be kept or omitted in future course syllabi, while considering that some higher or lower numbers may occur in the initial introduction of the annotation platform, as students become familiar with the annotation routines. Similar consideration of individual student activity vs. course resource usage have been harnessed for LMS dashboards (Wise and Jung, 2019), and for annotations across course documents over time (Singh, 2019). Although just in time teaching was mentioned previously in regards to the traditional template assessment, it may equally apply to annotation output, particularly if collating tags indicating confusion. This could inform instructors on where students are having difficulties (Singh, 2019). Perusall also has the capability to generate a confusion report to summarize general areas of questions/confusion.
For learning analytics practitioners, a body of annotations holds not only the insight within it (i.e., what section of text is highlighted? what is expressed in the annotations added?), but where it was applied (which document or URL?), when it was applied (time stamps), and how students and scholars might form an effective network (who participates in whose threads?). This could collectively yield a staggering amount of data. An estimated 2,900,000 time-stamped learning “traces” were postulated to arise from a 200-student course using an nStudy collaborative annotation tool (Winne et al., 2019). The Hypothes.is and Perusall platforms have vastly larger student user bases, so collaborative online annotation seems ripe for learning analytics and big data inquiries. Statistical properties of online web page tagging practices (Halpin et al., 2007; Glushko et al., 2008), or the view of collaborative tagging as distributed cognition (Steels, 2006), may also apply to annotation content when larger groups of annotators are involved.
Annotation Platforms for Peer Review and Post-publication Peer Review
Although the user base for online annotation by students is large, collaborative text-linked annotation could find additional users in a journal’s peer review process or the post-publication peer review process (Staines, 2018), whereby commentary is collected for the purposes of re-contextualizing or further assessing the quality of previously published manuscript (Kriegeskorte, 2012). Some journals already include collaborative stages in peer review, but the discussion occurs in more of a forum type situation, where the commentary is not directly text-linked or marginal. Authors, reviewers, and editors should consider whether commentary that is directly text-linked or figure-linked is more beneficial, or whether they would like to continue to contextualize comments with line numbers and other source document referrals. Critical commentary on a published article may occur already within the introduction and discussion sections of other articles, or on web blogs, but assembling it can be difficult, as it is not anchored within the discussed document, but reconstructed in a labor-intensive way from citation trails. One can contemplate whether post-publication peer review initiatives like Pub Peer (Townsend, 2013), would be more streamlined if commentary was directly content-linked. This could perhaps be aided by a set of common tags among users.
Meta-Commentary, New Teaching Spaces
In reading the primary source paper and accrued commentary in the annotations, which often include praises, snipes, and how the authors “should have done things differently,” one is fairly confident that the commentary drives additional interest in the paper. Although they are not typically marginal or text-linked, comments in newspaper articles are generally supported by authors and may drive more interest in the article itself (Nielsen, 2012). To consider an example outside of academics, some television programs (i.e., Terrace House) include a surrogate audience of commentators to help a home audience interpret and judge the actions of characters on the show (Rugnetta, 2017). The audience tunes in not only to see what the main characters will do, but also how their behavior is commented upon by this panel of observers. Their commentary functions as highly engaging meta-content that indicates how a viewer should receive and process main events in the show (Urban, 2010). Some fear that the show would be mundane without the additional panel commentary which serves as a major engagement tool; the audience is treated to a meta-experience that filters their own experience, and it is this alternative reading that provides additional intrigue (Kyotosuki, 2018).
Consider reading your favorite movie script with annotations by the director, or a draft of your favorite novel including exchanges between the editor and the author, or a landmark scientific paper with annotations by current scientists. These would all inform the reader on the process involved in getting to the final product, or in the latter example could provide a contemporary lens for older content, and thus add value. Some critics have imagined bodies of annotations from a favorite book that could be shared (transportable social marginalia) in a literary communion through a series of “annotation skins” (Anderson, 2011). The collation and screening of quality annotations could also be a value-adding enterprise for those willing to participate.
While one can lament the loss of physical teaching spaces imposed by a viral pandemic or other virtual learning circumstances, new spaces are opened by new technologies (Pursell and Iiyoshi, 2021). The instructor and the student can “meet at the text” via collaborative online annotation, and engage in critical exchanges.
Future Research Questions
Collaborative annotation provides fertile ground for further study. First: To what degree do students read the primary source text on the annotation platform? Is the student marking up a paper copy or separate digital copy (i.e., personally downloaded PDF file) and then going to the annotation platform, or do they treat the platform as a packaged experience where they do both the initial reading and annotating? This question should be included in future annotation usage surveys and could inform platform designers, who would like to enable the smoothest experience in both source text reading and annotating on the same screen. One might expect dialogic interactions to decrease if users were annotating a paper or digital copy by themselves first and then just typing in those isolated points into the annotation platform interface. Second: What percentage of the total body of annotations on a given text are students consuming? To what extent do students revisit their own annotation threads to look for and address new responses, or revisit a growing body of annotations after they have fulfilled an instructor stipulated amount? Survey data in this study indicated that students found value in the annotations of others, which is in accord with the value of “eavesdropping” on the insights of other readers (Wolfe and Neuwirth, 2001), but currently, the only plausible indicator that an annotation has been read by another student is if they have then commented on it to extend the thread. Perhaps technical developments by the platforms might render some measurement on student consumption of annotations in the future. The consumption of high quality, but perhaps unseen, threads can be aided by an instructor’s curation of annotations for a subsequent class session. Third: How much value does a body of annotations hold over time? Although this has been considered for an annotation’s value relative to the potential permanence of the work itself (Marshall, 2000), one could also ponder how much value a body of annotations generated in one class could have for an instructor or group of students at another university who happen to be embarking on an annotation exercise on the same source text. This would seem to provide fertile ground for cross-cultural and cross-institutional comparisons. An instructor could give a series of richly annotated documents to a group of students and have them evaluate that reading experience vs. a set of unannotated documents to test the dirty textbook Hypothes.is (Van Dam, 1988) in the current online portable annotation environment. There will be opportunities for instructor curation and comparison that also relate to pedagogy, as was the strategy for a previous class’s annotations to function as “seed” annotations for the promotion of productive student dialogue by Miller et al. (2016). Fourth: Would the author of the source text ever wish to engage with the annotators? Some authors might discover new insights, research directions, and caveats for their published work in treating public annotation directly linked to the source text as a form of post-publication peer review. Textbook authors and editors might like to see sections of the book that generate many annotations indicative of confusion. Other authors are opposed, stating that commentary on their article is fine in other locations (separate blogs, twitter, etc.), but do not want any commentary to be superimposed upon their own website URLs (Watters, 2017). Constructive commentary is likely favorable to most, but it would need to also be free of the noise of useless comments, personal attacks, or factually false statements. This useful “wheat” vs. useless “chaff” concern affects all publication systems.
Conclusion
Collaborative online annotation can provide a means for creative discussion and better understanding of a text, including quite challenging primary research texts. As with any educational technology, pedagogical considerations will be of paramount importance. Students recognize and appreciate that an online annotation platform can make their thoughts, and those of their classmates, visible and actionable for an assigned text, thus providing a useful comparator. Also, some solace can be found in a struggle on tough material that is collective as opposed to isolated. Repetition in grading is cut down when collaborative annotation takes the place of an assignment where students are generating a relatively uniform assessment product. Some of the feedback burden on instructors is removed when students beat the instructor to the punch in responding to an annotation with quality feedback.
Early web browsers contemplated annotation as a feature, but were hampered by an inability to host the potentially huge scale of annotations on a proper server (Andreessen, 2014), so a realization of the power of online annotation is not new and has been around since the early 1990s. Now, because of the large and growing user bases, Perusall and Hypothes.is are opening up a new enterprise that classroom instructors, scholars, and learning analytics practitioners can all enter, and hopefully can all benefit students in the process.
In an address entitled: The Revolution will be annotated (Personal Democracy Forum, 2013), Whaley argued that “reasoning tends to work better as a team sport.” The student feedback in the current study supports that argument. In As we may think (Bush, 1945), where multiple aspects of the internet were presupposed, Vannevar Bush predicted:
There is a new profession of trail blazers, those who find delight in the task of establishing useful trails through the enormous mass of the common record. The inheritance from the master becomes, not only his additions to the world’s record, but for his disciples the entire scaffolding by which they were erected.
This idea may gain traction in the rapidly accruing mass of annotations and post-publication commentary. Since annotation platforms like Perusall are now serving students in the millions, and Hypothes.is annotators have made over 20 million annotations, approximately one year after they marked 10 million annotations,3 research into usage and impact of these platforms seems particularly pressing. Hypothes.is, through iAnnotate,4 and Perusall, through the Perusall Exchange5 are generating excitement in their own dedicated conferences. Learning Management Systems (LMSs) and Audience Response Systems (“clickers” or ARSs) have become so ubiquitous in higher education as to gain a common label. With the number of students currently served, it seems fitting that collaborative online annotation platforms (COAPs) acquire a common label too. To examine the scope of the current study, the students in two cohorts made altogether over 2,200 annotations, totaling over 920,000 characters. Although most of the focus in this and other annotation papers is how the collaborative annotation process helps the students, one can also consider how this spotlight into student thoughts helps the teacher. The students repeatedly had insights into the scientific content of the assigned papers which expanded the thinking of the instructor.
These annotation platforms are bringing new value to the educational technology landscape, new ways of achieving prompt and valuable feedback that is often dialogic in nature, may lessen instructor burden, and increase instructor and student motivation. The task we now face as educators is to make the annotation trails as useful as possible as we engage in the team sport of reasoning in the sciences, social sciences, and humanities.
Data Availability Statement
Anonymized datasets for this study can be found in the Harvard Dataverse online repository: Full survey responses (https://dataverse.harvard.edu/dataset.xhtml?persistentId=doi:10.7910/DVN/0GG53Z), and annotation content excel files (https://dataverse.harvard.edu/dataset.xhtml?persistentId=doi:10.7910/DVN/G8SR2G).
Ethics Statement
The studies involving human participants were reviewed and approved by the Harvard Human Research Protection Program. Written informed consent for participation was not required for this study in accordance with the National Legislation and the Institutional Requirements.
Author Contributions
GWP conceived the study, analyzed the data, and wrote the manuscript.
Conflict of Interest
The author declares that the research was conducted in the absence of any commercial or financial relationships that could be construed as a potential conflict of interest.
Publisher’s Note
All claims expressed in this article are solely those of the authors and do not necessarily represent those of their affiliated organizations, or those of the publisher, the editors and the reviewers. Any product that may be evaluated in this article, or claim that may be made by its manufacturer, is not guaranteed or endorsed by the publisher.
Acknowledgments
I would like to acknowledge the consistent group effort of the students in annotating challenging papers, and providing frank commentary on the annotation process. The author would also like to acknowledge the time spent with Harvard Medical School’s Curriculum Fellows for broadening his exposure to pedagogical approaches and tools. Valuable feedback on early drafts of this manuscript came from Dr. Taralyn Tan of Harvard’s Neuroscience Program, and Dr. Rachel Wright of Smith College. Valuable guidance on IRB navigation was provided by Dr. Madhvi Venkatesh, Vanderbilt University, formerly of Harvard.
Supplementary Material
The Supplementary Material for this article can be found online at: https://www.frontiersin.org/articles/10.3389/feduc.2022.852849/full#supplementary-material
Footnotes
- ^ https://web.Hypothes.is/about/
- ^ https://perusall.com/about
- ^ https://web.Hypothes.is/blog/our-view-from-20-million-annotations/
- ^ https://iannotate.org/
- ^ https://perusall.com/exchange
References
Adams, P. (2006). Exploring social constructivism: theories and practicalities. Education 34, 243–257. doi: 10.1080/03004270600898893
Anderson, S. (2011). What I Really Want is Someone Rolling Around in the Text. New York: New York Times,
Andreessen, M. (2014). Why Andreessen Horowitz Is Investing in Rap Genius. Available online at: http://genius.com/Marc-andreessen-why-andreessen-horowitz-is-investing-in-rap-genius-annotated [Accessed on July 19, 2021]
Beck, C. (2017). “Informal action research: The nature and contribution of everyday classroom inquiry,” in The Palgrave International Handbook of Action Research, eds L. Rowell, C. Bruce, J. Shosh, and M. Riel (New York: PalgraveMacmillan), 37–48. doi: 10.1111/1751-7915.13576
Bold, M. R., and Wagstaff, K. L. (2017). Marginalia in the digital age: are digital reading devices meeting the needs of today’s readers? Libr. Inf. Sci. Res. 39, 16–22. doi: 10.1016/j.lisr.2017.01.004
Carnegie Learning (2020). UpGrade: An Open Source Platform for A/B Testing in Education. Available online at: https://www.carnegielearning.com/blog/upgrade-ab-testing/ [Accessed on Jun 28, 2021]
Chen, Z., Chudzicki, C., Palumbo, D., Alexandron, G., Choi, Y.-J., Zhou, Q., et al. (2016). Researching for better instructional methods using AB experiments in MOOCs: results and challenges. Res. Pract. Technol. Enhanc. Learn. 11, 1–20. doi: 10.1186/s41039-016-0034-4
Clapp, J., DeCoursey, M., Lee, S. W. S., and Li, K. (2020). “Something fruitful for all of us”: social annotation as a signature pedagogy for literature education. Arts Humanit. High. Educ. 20, 147402222091512.
Clump, M. A., Bauer, H., and Bradley, C. (2004). The Extent to which Psychology Students Read Textbooks: a Multiple Class An. Discovery Service for Purdue University. J. Instr. Psychol. 31, 227–232.
Duchastel, P., and Chen, Y. P. (1980). The use of marginal notes in text to assist learning. Educ. Technol. 20, 41–45.
Fagen, A. P., Crouch, C. H., and Mazur, E. (2002). Peer instruction: results from a range of classrooms. Phys. Teach. 40, 206–209. doi: 10.1119/1.1474140
Ghadirian, H., Salehi, K., and Ayub, A. F. M. (2018). Social annotation tools in higher education: a preliminary systematic review. Int. J. Learn. Technol. 13, 130–162. doi: 10.1504/ijlt.2018.092096
Glushko, R. J., Maglio, P. P., Matlock, T., and Barsalou, L. W. (2008). Categorization in the wild. Trends Cogn. Sci. 12, 129–135. doi: 10.1016/j.tics.2008.01.007
Halpin, H., Robu, V., and Shepherd, H. (2007). The Complex Dynamics of Collaborative Tagging. in Proceedings of the 16th International Conference on World Wide Web. New York: ACM 211–220.
Hannafin, M. J., Hill, J. R., Land, S. M., and Lee, E. (2014). “Student-centered, open learning environments: Research, theory, and practice,” in Handbook of Research on Educational Communications and Technology, eds M. Spector, M. D. Merrill, J. Merrienboer, and M. Driscoll (London, UK: Routledge), 641–651. doi: 10.1007/978-1-4614-3185-5_51
Honeycutt, L. (2001). Comparing e-mail and synchronous conferencing in online peer response. Writ. Commun. 18, 26–60. doi: 10.1177/0741088301018001002
Hoskins, S. G., Lopatto, D., and Stevens, L. M. (2011). The CREATE approach to primary literature shifts undergraduates’ self-assessed ability to read and analyze journal articles, attitudes about science, and epistemological beliefs. CBE Life Sci. Educ. 10, 368–378. doi: 10.1187/cbe.11-03-0027
Howard, J. R., and Henney, A. L. (1998). Student participation and instructor gender in the mixed-age college classroom. J. Higher Educ. 69, 384–405. doi: 10.2307/2649271
Jackson, H., Nayyar, A., Denny, P., Luxton-Reilly, A., and Tempero, E. (2018). HandsUp: An In-Class Question Posing Tool. in 2018 International Conference on Learning and Teaching in Computing and Engineering (LaTICE). New Jersey: IEEE, 24–31.
Jackson, P. (2021). How to Write a High Quality Reading Annotation. Available online at: https://www.saltise.ca/wp-content/uploads/2021/03/How-to-write-high-quality-reading-annotations.pdf [Accessed on Jun 30, 2021]
Kalir, R., and Garcia, A. (2019). Annotation: The MIT Press Essential Knowledge Series. Annotation. Available online at: https://mitpressonpubpub.mitpress.mit.edu/annotation [Accessed on May 17, 2021].
Kararo, M., and McCartney, M. (2019). Annotated primary scientific literature: a pedagogical tool for undergraduate courses. PLoS Biol. 17:e3000103. doi: 10.1371/journal.pbio.3000103
Kennedy, M. (2016). Open annotation and close reading the Victorian text: using hypothes. is with students. J. Vic. Cult. 21, 550–558. doi: 10.1080/13555502.2016.1233905
Kimmons, R. (2021). A/B Testing on Open Textbooks. A Feasibility Study for Continuously Improving Open Educational Resources. J. Appl. Instr. Des. 10, 1–9. doi: 10.51869/102/rk
King, G. (2016). Introduction to Perusall. Available online at: https://perusall.com/downloads/gary-king-webinar-slides.pdf (accessed June 3, 2020).
Kriegeskorte, N. (2012). Open evaluation: a vision for entirely transparent post-publication peer review and rating for science. Front. Comput. Neurosci. 6:79. doi: 10.3389/fncom.2012.00079
Kuckartz, U. (2014). Qualitative Text Analysis: A Guide to Methods, Practice and Using Software. California: Sage.
Kyotosuki, N. (2018). Terrace House: Visualising ‘Asian Modernity. Available online at: https://atmafunomena.wordpress.com/2018/08/31/terrace-house-visualising-asian-modernity/ [Accessed on July 1, 2021].
Lee, S. C., Lee, Z.-W., and Yeong, F. M. (2019). “Using social annotations to support collaborative learning in a Life Sciences module,” in personalized Learing. Diverse Goals. One Heart, ASCILITE, ed. Y. W. Chew (Singapore: ASCILITE), 487–492.
Marrs, K. A., and Novak, G. (2004). Just-in-time teaching in biology: creating an active learner classroom using the internet. Cell Biol. Educ. 3, 49–61. doi: 10.1187/cbe.03-11-0022
Marshall, C. (2000). The Future of Annotation in a Digital (paper) World. Successes & Failures of Digital Libraries: [papers presented at the 1998 Clinic on Library Applications of Data Processing, March 22-24, 1998]. Available online at: http://hdl.handle.net/2142/25539
Miller, K., Lukoff, B., King, G., and Mazur, E. (2018). Use of a Social Annotation Platform for Pre-Class Reading Assignments in a Flipped Introductory Physics Class. Front. Educ. 3:1–12. doi: 10.3389/feduc.2018.00008
Miller, K., Zyto, S., Karger, D., Yoo, J., and Mazur, E. (2016). Analysis of student engagement in an online annotation system in the context of a flipped introductory physics class. Phys. Rev. Phys. Educ. Res. 12, 1–12. doi: 10.1103/PhysRevPhysEducRes.12.020143
Nielsen, C. (2012). Newspaper journalists support online comments. Newsp. Res. J. 33, 86–100. doi: 10.1093/geront/gns046
Novak, E., Razzouk, R., and Johnson, T. E. (2012). The educational use of social annotation tools in higher education: a literature review. Internet High. Educ. 15, 39–49. doi: 10.1016/j.iheduc.2011.09.002
November, A. (2020). Interview with Eric Mazur: Socrates Meets the Web! Novemb. Learn. Webpage. Available online at: https://novemberlearning.com/2020/04/29/interview-with-eric-mazur-socrates-meets-the-web/ [Accessed on May 25, 2020].
Personal Democracy Forum (2013). Dan Whaley: The Revolution Will Be Annotated. Available online at: https://www.youtube.com/watch?v=2jTctBbX_kw
Podolefsky, N., and Finkelstein, N. (2006). The Perceived Value of College Physics Textbooks: students and Instructors May Not See Eye to Eye. Phys. Teach. 44, 338–342. doi: 10.1119/1.2336132
Porter, G. W. (2013). Free choice of learning management systems: do student habits override inherent system quality? Interact. Technol. Smart Educ. 10, 84–94. doi: 10.1108/ITSE-07-2012-0019
Pursell, C., and Iiyoshi, T. (2021). Policy Dialogue: online Education as Space and Place. Hist. Educ. Q. 61, 534–545. doi: 10.1017/heq.2021.47
Renz, J., Hoffmann, D., Staubitz, T., and Meinel, C. (2016). Using A/B testing in MOOC environments. in Proceedings of the Sixth International Conference on Learning Analytics & Knowledge, New York: ACM. 304–313.
Round, J. E., and Campbell, A. M. (2013). Figure facts: encouraging undergraduates to take a data-centered approach to reading primary literature. CBE Life Sci. Educ. 12, 39–46. doi: 10.1187/cbe.11-07-0057
Rugnetta, M. (2017). How is Terrace House like a Let’s Play. Available online at: https://www.youtube.com/watch?v=24MWwO_Gpg8 [Accessed on July 1, 2021]
Sadler, D. R. (1989). Formative assessment and the design of instructional systems. Instr. Sci. 18, 119–144. doi: 10.1007/bf00117714
Simpson, M. L., and Nist, S. L. (1990). Textbook annotation: an effective and efficient study strategy for college students. J. Read. 34, 122–129.
Singh, S. (2019). Exploring the potential of social annotations for predictive and descriptive analytics. Annual Conference on Innovation and Technology in Computer Science Education. New York: Association for Computing Machinery 247–248. doi: 10.1145/3304221.3325547
Staines, H. R. (2018). Digital Open Annotation with Hypothes.is: Supplying the Missing Capability of the Web. J. Sch. Publ. 49, 345–365. doi: 10.3138/jsp.49.3.04
Steels, L. (2006). Collaborative tagging as distributed cognition. Pragmat. Cogn. 14, 287–292. doi: 10.1016/j.chb.2015.04.053
Townsend, F. (2013). Post-publication peer review: PubPeer. Ed. Bull. 9, 45–46. doi: 10.1080/17521742.2013.865333
Urban, G. (2010). A method for measuring the motion of culture. Am. Anthropol. 112, 122–139. doi: 10.1111/j.1548-1433.2009.01201.x
Van Campenhout, R., Brown, N., Jerome, B., Dittel, J. S., and Johnson, B. G. (2021). Toward Effective Courseware at Scale: Investigating Automatically Generated Questions as Formative Practice. in Proceedings of the Eighth ACM Conference on Learning@Scale. New York: ACM 295–298.
Van Dam, A. (1988). Hypertext’87: keynote address. Commun. ACM 31, 887–895. doi: 10.1145/48511.48519
Vygotsky, L. (1978). Mind in Society: The Development of Higher Psychological Processes.Cambridge: Harvard University Press
Watters, A. (2017). Un-Annotated. Available online at: http://hackeducation.com/2017/04/26/no-annotations-thanks-bye [Accessed on August 12, 2021]
Weng, C., and Gennari, J. H. (2004). Asynchronous Collaborative Writing through Annotations. in Proceedings of the 2004 ACM Conference on Computer Supported Cooperative Work.Washington: University of Washington 578–581.
Wenk, B. L., and Tronsky, L. (2011). First-Year Students Benefit From Reading Primary Research Articles. J. Coll. Sci. Teach. 40, 60–67.
Winne, P. H., Teng, K., Chang, D., Lin, M. P. C., Marzouk, Z., Nesbit, J. C., et al. (2019). nStudy: software for learning analytics about processes for self-regulated learning. J. Learn. Anal. 6, 95–106.
Wise, A. F., and Jung, Y. (2019). Teaching with analytics: towards a situated model of instructional decision-making. J. Learn. Anal. 6, 53–69.
Wolfe, J. (2002). Annotation technologies: a software and research review. Comput. Compos. 19, 471–497. doi: 10.1016/s8755-4615(02)00144-5
Wolfe, J. L., and Neuwirth, C. M. (2001). From the margins to the center: the future of annotation. J. Bus. Tech. Commun. 15, 333–371. doi: 10.1177/105065190101500304
Keywords: annotation, assessment, asynchronous, feedback, Hypothes.is, peer learning, Perusall
Citation: Porter GW (2022) Collaborative Online Annotation: Pedagogy, Assessment and Platform Comparisons. Front. Educ. 7:852849. doi: 10.3389/feduc.2022.852849
Received: 11 January 2022; Accepted: 23 March 2022;
Published: 10 May 2022.
Edited by:
Michael Kickmeier-Rust, University of Teacher Education St. Gallen, SwitzerlandReviewed by:
Paul Seitlinger, University of Vienna, AustriaAgnes D’Entremont, University of British Columbia, Canada
Copyright © 2022 Porter. This is an open-access article distributed under the terms of the Creative Commons Attribution License (CC BY). The use, distribution or reproduction in other forums is permitted, provided the original author(s) and the copyright owner(s) are credited and that the original publication in this journal is cited, in accordance with accepted academic practice. No use, distribution or reproduction is permitted which does not comply with these terms.
*Correspondence: Gavin W. Porter, Z2F2aW5fcG9ydGVyQGhtcy5oYXJ2YXJkLmVkdQ==