- 1Department of Pathology, Microbiology and Forensic Medicine, School of Medicine, The University of Jordan, Amman, Jordan
- 2Department of Clinical Laboratories and Forensic Medicine, Jordan University Hospital, Amman, Jordan
Background: The transformative potential of artificial intelligence (AI) in higher education is evident, with conversational models like ChatGPT poised to reshape teaching and assessment methods. The rapid evolution of AI models requires a continuous evaluation. AI-based models can offer personalized learning experiences but raises accuracy concerns. MCQs are widely used for competency assessment. The aim of this study was to evaluate ChatGPT performance in medical microbiology MCQs compared to the students’ performance.
Methods: The study employed an 80-MCQ dataset from a 2021 medical microbiology exam at the University of Jordan Doctor of Dental Surgery (DDS) Medical Microbiology 2 course. The exam contained 40 midterm and 40 final MCQs, authored by a single instructor without copyright issues. The MCQs were categorized based on the revised Bloom’s Taxonomy into four categories: Remember, Understand, Analyze, or Evaluate. Metrics, including facility index and discriminative efficiency, were derived from 153 midterm and 154 final exam DDS student performances. ChatGPT 3.5 was used to answer questions, and responses were assessed for correctness and clarity by two independent raters.
Results: ChatGPT 3.5 correctly answered 64 out of 80 medical microbiology MCQs (80%) but scored below the student average (80.5/100 vs. 86.21/100). Incorrect ChatGPT responses were more common in MCQs with longer choices (p = 0.025). ChatGPT 3.5 performance varied across cognitive domains: Remember (88.5% correct), Understand (82.4% correct), Analyze (75% correct), Evaluate (72% correct), with no statistically significant differences (p = 0.492). Correct ChatGPT responses received statistically significant higher average clarity and correctness scores compared to incorrect responses.
Conclusion: The study findings emphasized the need for ongoing refinement and evaluation of ChatGPT performance. ChatGPT 3.5 showed the potential to correctly and clearly answer medical microbiology MCQs; nevertheless, its performance was below-bar compared to the students. Variability in ChatGPT performance in different cognitive domains should be considered in future studies. The study insights could contribute to the ongoing evaluation of the AI-based models’ role in educational assessment and to augment the traditional methods in higher education.
1 Introduction
The revolutionary influence of artificial intelligence (AI) is gaining a profound recognition, particularly in the scope of higher education (Grassini, 2023; Kamalov et al., 2023). The advent of AI conversational models (e.g., ChatGPT, Bard, and Bing Chat) offers an opportunity to fundamentally transform the entire educational landscape including various aspects such as teaching methods, curriculum development, educational policies, and practices (Michel-Villarreal et al., 2023; Sallam, 2023; Southworth et al., 2023). On the other hand, AI is dynamic field, characterized by rapid expansion and evolution (Dwivedi et al., 2023). Therefore, it is important to continuously evaluate the suitability of AI-based models for integration into higher education (Chan, 2023). This is particularly relevant considering the already extensive utilization of AI-models among university students (Abdaljaleel et al., 2023; Ibrahim et al., 2023), with the need for revising higher education policies and practices that are guided by evidence (Newton et al., 2020). Thus, the implementation of AI-based models in higher education has the potential to revolutionize inquiry-based learning (Chang et al., 2023). In turn, this transformation can culminate in inclusive and equitable quality education (Ramírez-Montoya et al., 2023).
One particular aspect where AI-based tools can be helpful is the easy access to customized educational content that matches the specific needs and preferences of students; thereby, offering personalized learning experiences (Huang et al., 2023; Kamalov et al., 2023; Sallam, 2023; Sallam et al., 2023d). Nevertheless, there are valid concerns that arose regarding the perspectives of AI-models in higher education, specifically concerns involving the inaccuracy of the information provided by these models as well as privacy concerns (Kimmerle et al., 2023; Michel-Villarreal et al., 2023; Moldt et al., 2023; Sallam, 2023). In higher education, multiple-choice questions (MCQs) are well-recognized as an objective method to evaluate the students’ achievement of required competencies and intended learning outcomes despite its known limitations (Brown and Abdulnabi, 2017; Newton, 2020; Liu et al., 2023). Categorization of MCQs can be done based on the Bloom’s cognitive taxonomy (Bloom and Krathwohl, 1956; Mohammed and Omar, 2020). The Bloom’s framework enables educators frame MCQs in a way suitable to evaluate diverse aspects of student learning effectively as follows: (1) knowledge (recall), (2) comprehension (understanding), (3) application (problem-solving), (4) analysis (information breakdown), (5) synthesis (creative solutions), and (6) evaluation (judgment) (Seaman, 2011). Additionally, MCQs can be categorized into different difficulty levels, based on the complexity of the question and the cognitive skills needed for their solution (Rauschert et al., 2019).
An emerging research field that gained a huge momentum recently involved the exploration of AI-based conversational models’ ability to answer MCQs (Alfertshofer et al., 2023; Chang et al., 2023; Fuchs et al., 2023; Giannos and Delardas, 2023; Oztermeli and Oztermeli, 2023). The motivation of this research endeavor can be related to several aspects as follows. This inquiry can facilitate the understanding of AI capabilities to process and interpret educational materials. Thus, the investigation of AI-based models’ performance in MCQs-based exams can help to improve the AI technologies through identification of its current weaknesses. Subsequently, the accumulating evidence can help in effective integration of these AI tool in higher education (Southworth et al., 2023). Additionally, the identification of weakness in the current cognitive abilities of the AI-based models can guide targeted training and updates, reinforcing reliability of these AI tools. Moreover, exploring AI-models’ ability to pass the MCQs-based exams highlights critical ethical considerations in education mainly in relation to the academic dishonesty and emphasizes the threats these tools pose to the current assessment methods in education (Newton and Xiromeriti, 2023). Furthermore, this investigation can highlight the ability of AI-based models to provide interactive learning experience. In turn, this can help to offer education in a more interactive and accessible manner. This is particularly beneficial in resource-limited settings where the subscription to MCQs banks cannot be afforded; therefore, these AI-based models can offer a cost-effective alternative to traditional MCQ resources (Cheung et al., 2023).
Previous studies have evaluated the efficacy of AI-based models in addressing MCQs across diverse healthcare disciplines, yielding varying outcomes (Newton and Xiromeriti, 2023). These assessments encompassed the utilization of ChatGPT in scenarios such as United States Medical Licensing Examination (USMLE) exam, as well as the performance within fields such as parasitology and ophthalmology, among others in various languages (e.g., Japanese, Chinese, German, Spanish) (Antaki et al., 2023; Carrasco et al., 2023; Friederichs et al., 2023; Huh, 2023; Kung et al., 2023; Takagi et al., 2023; Xiao et al., 2023). A recent scoping review examined the influence of the ChatGPT model on examination outcomes, including instances where ChatGPT demonstrated superior performance compared to students, albeit in a minority of cases (Newton and Xiromeriti, 2023). In the context of assessing the performance of ChatGPT in MCQs based on the Bloom’s taxonomy, a comprehensive study by Herrmann-Werner et al. tested ChatGPT-4 performance on a large dataset comprising 307 psychosomatic medicine MCQs (Herrmann-Werner et al., 2023). The results showed the ability of ChatGPT to pass the exam regardless of the prompting used. Additionally, the study showed variability in cognitive errors being more common in the “Remember” and “Understand” domains (Herrmann-Werner et al., 2023). Nevertheless, no other studies were found utilizing the same approach highlighting a gap in literature on the investigation of cognitive abilities of these AI-based models.
Medical microbiology is a complex and dynamic field, and a meticulous approach to teaching and assessment is necessary to provide students with a thorough understanding of microbiological concepts, practical skills, and ethical considerations while keeping up with the latest developments in the field (Rutherford, 2015; Stevens et al., 2017; Joshi, 2021). Therefore, the current study aimed to provide a descriptive assessment of ChatGPT ability to accurately solve MCQs in medical microbiology through controlled prompts and the use of assessment of the content based on expert review of ChatGPT responses. Specifically, the study questions were as follows: (1) Can ChatGPT pass a medical microbiology examination? (2) How does ChatGPT performance compares to human students? And (3) Are there any differences in ChatGPT performance based on the category of MCQs?
The current study was motivated by the ongoing debate regarding the implementation of AI models in higher education (Rudolph et al., 2023). This controversy involved concerns regarding academic dishonesty which forced some institutions to ban the use of these AI models in campuses. This study could also contribute to the collective efforts aiming to assess the applicability of AI-based models in higher education. Specifically, delivering comprehensive insights into ChatGPT performance across diverse cognitive levels, guided by the revised Bloom’s taxonomy. The selection of this taxonomy as the theoretical framework in this study was based on several considerations as follows. The revised Bloom’s taxonomy, with its well-defined cognitive learning levels from basic understanding to advanced analysis, enables the evaluation of higher-order cognitive skills in AI-based models like ChatGPT. This framework aligns with the established educational practices for meaningful comparison with human performance and provides an objective framework for identifying AI-based models’ cognitive strengths and weaknesses, guiding its targeted development and training in various educational contexts.
2 Methods
2.1 Study design
The study utilized a dataset comprising 80 MCQs extracted from a medical microbiology exam administered during the Spring Semester of the academic year 2021/2022. This exam, pertaining to Doctor of Dental Surgery (DDS) Medical Microbiology 2 course at the University of Jordan, consisted of 40 MCQs in the midterm exam, each carrying a weight of 1 grade, and 40 final MCQs, each with a weight of 1.5 grades. The exam was conducted online in the second semester of the academic year 2021/2022. Data pertaining to the difficulty and discrimination indices of these questions were collected based on the DDS students’ performance during the exams. The MCQs employed in the examination were authored by the sole exam instructor (the first author: M.S.) and were free from any copyright issues. The choice of the year 2021 was made in consideration of the extent of knowledge available to ChatGPT 3.5 (September 2021) (OpenAI, 2023). The MCQs were presented in English, as it is the official language of instruction for the DDS program at the University of Jordan (Sallam et al., 2019).
In retrospect, we evaluated the quality of the study design, methodologies, and reporting of findings using the novel METRICS checklist for standardized reporting of AI-based studies (Sallam et al., 2023b).
The ethical approval for this study was deemed unnecessary as the data were completely anonymous and the results of the university exams were public and open, and the questions were generated by the first author with no copyright issues.
2.2 Classification of MCQs based on the revised Bloom’s classification
The MCQs were subjected to a categorization process by two microbiologists, a Consultant in Clinical Pathology (Microbiology/Immunology, the first author: M.S.) and a Microbiology/Immunology specialist (K.A.). This categorization was guided by the cognitive dimensions of the Revised Bloom’s Taxonomy, resulting in the following categories: (1) Remember, (2) Understand, (3) Analyze, and (4) Evaluate (Anderson and Krathwohl, 2001).
The ascending classification hierarchy based on the need for cognitive efforts was as follows: First, the “Remember” level entailed the requirement of retrieval of factual information devoid of a need for deeper comprehension, demanding minimal cognitive effort. Second, the “Understand” level indicated the need for comprehending the meaning of concepts and establishing connections between related ideas. Third, the “Analyze” level entailed the deconstruction of information into discern patterns, the need to conduct comparisons, or disassembly of complex problems into related segments. Fourth, the “Evaluate” level involved the need to make informed judgments and decisions, and the assessment of the value or quality of information, arguments, or solutions reflecting the highest degree of critical thinking.
2.3 MCQ metrics
In assessing the MCQs, metrics were derived from the performance of 153 DDS students in the midterm exam and 154 students in the final exam. These metrics encompassed both the facility index and discriminative efficiency. The facility index, expressed as the percentage of students who correctly answered a given question, elucidated the question level of difficulty. On the other hand, discriminative efficiency aimed to highlight the distinguishing ability of the MCQ based on varying levels of proficiency among students, with higher values indicating superior discrimination. A range of 30 to 50% denoted satisfactory discrimination, while values exceeding 50% indicated higher discriminatory power. The discriminative efficiency index was computed as a ratio, involving the discrimination index divided by the maximum discrimination. In addition, we collected the MCQ stem word count and choices’ word count data.
2.4 ChatGPT 3.5 query and prompt construction
The 80 MCQs were promoted on ChatGPT (model GPT-3.5 and date on 11 March 2023) is available to Free and Plus users (OpenAI, 2023). Then, these questions were subjected to ChatGPT 3.5 specific prompt as follows: “Select the most appropriate answer for the following MCQ with rationale for selecting this choice and excluding the other choices.”
2.5 Assessment of ChatGPT responses
First, ChatGPT responses were scored as either correct or incorrect based on the key answers. Then, the whole response was scored independently by the two expert raters for two aspects: (1) Correctness and (2) Clarity as follows: Completely correct or clear scored as “4”; almost correct or clear scored as “3”; partially correct or clear scored as “2”; and (4) completely incorrect or unclear scored as “1” (Sallam et al., 2023c).
The average correctness and clarity scores comprised the sum of the score of the two raters divided by 2 with a range of 0–4 for each score, and the sum of these two averages comprised the final correctness/clarity score with a range of 0–8.
2.6 Statistical analysis
Statistical analysis was conducted using IBM SPSS v26.0 for Windows. Descriptive statistics (mean and median) were used to assess central tendency, while standard deviation (SD) and interquartile range (IQR) were used to assess data dispersion. Association of the categorical variables was tested using the chi-squared test (χ2). As the scale variables exhibited non-normal distributions, as indicated by the Shapiro–Wilk (S-W) test, the Mann–Whitney U (M-W) and Kruskal-Wallis H (K-W) tests were applied. Specifically, the following scale variables showed the following skewness and kurtosis: MCQ stem word count (skewness = 2.051, kurtosis = 3.26, p < 0.001), MCQ choices word count (skewness = 0.816, kurtosis = −0.092, p < 0.001), facility index (skewness = −2.344, kurtosis = 6.474, p < 0.001), discriminative efficiency (skewness = −1.113, kurtosis = 3.464, p < 0.001), average correctness score (skewness = −1.756, kurtosis = 1.855, p < 0.001), average clarity score (skewness = −2.54, kurtosis = 6.363, p < 0.001), and the average correctness/clarity score (skewness = −2.004, kurtosis = 3.29, p < 0.001).
The Cohen kappa (κ) statistic was employed to assess inter-rater agreement. A significance level of p < 0.05 was set for all statistical assessments.
3 Results
3.1 General features of the MCQs and its metrics
To get an overview of the features of the MCQs dataset used in this study, categorization based on the revised Bloom’s taxonomy was done. A total of 80 MCQs were included classified as Analyze (n = 12, 15.0%), Evaluate (n = 25, 31.3%), Remember (n = 26, 32.5%), and Understand (n = 17, 21.3%). Of these, 76 MCQs were related to medical virology, while two questions were on medical mycology (one Remember, and one Understand) and two questions on oral parasitology (Evaluate). The features of the included MCQs is shown in (Table 1).
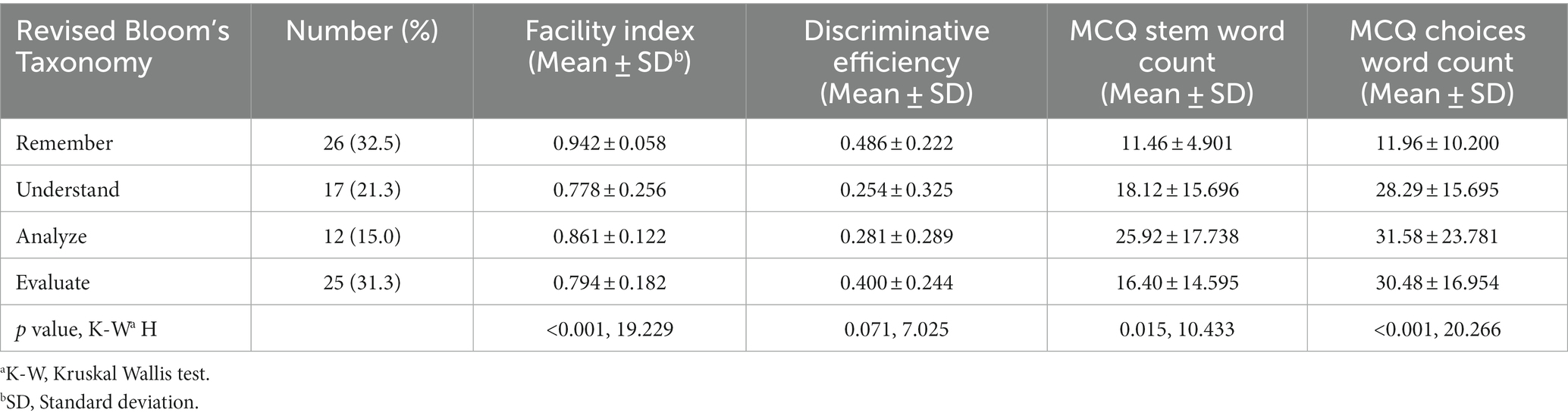
Table 1. Characteristics of the included multiple-choice questions (MCQs) stratified by the revised Bloom’s taxonomy.
To get better insights into the features of the MCQs dataset, we used the revised Bloom’s categorization as well as the facility index and discriminative efficiency. The mean facility index for the whole MCQ dataset was 0.848652 ± 0.1772418 (median = 0.911, IQR: 0.812–0.961). Stratified per Bloom’s revised domains, the highest median was seen for the Remember domain (0.961, IQR = 0.927–0.980), followed by the Analyze domain (median = 0.893, IQR = 0.811–0.956), the Understand domain (median = 0.900, IQR = 0.669–0.941), and the Evaluate domain (median = 0.850, IQR = 0.700–0.918). The mean discriminative efficiency was 0.376141 ± 0.2750766. Stem word count and choices word count showed statistically significant differences based on the Bloom’s revised domains (Table 1).
Based on the discriminative efficiency category, 27 MCQs had discriminative efficiency >0.500 (34.6%), 25 MCQs were 0.500–0.300 (32.1%) and 26 MCQs were < 0.300 (33.3%). Box plots illustrating the distribution of the facility index and discriminative efficiency across the revised Bloom’s cognitive domains is shown in (Figure 1).
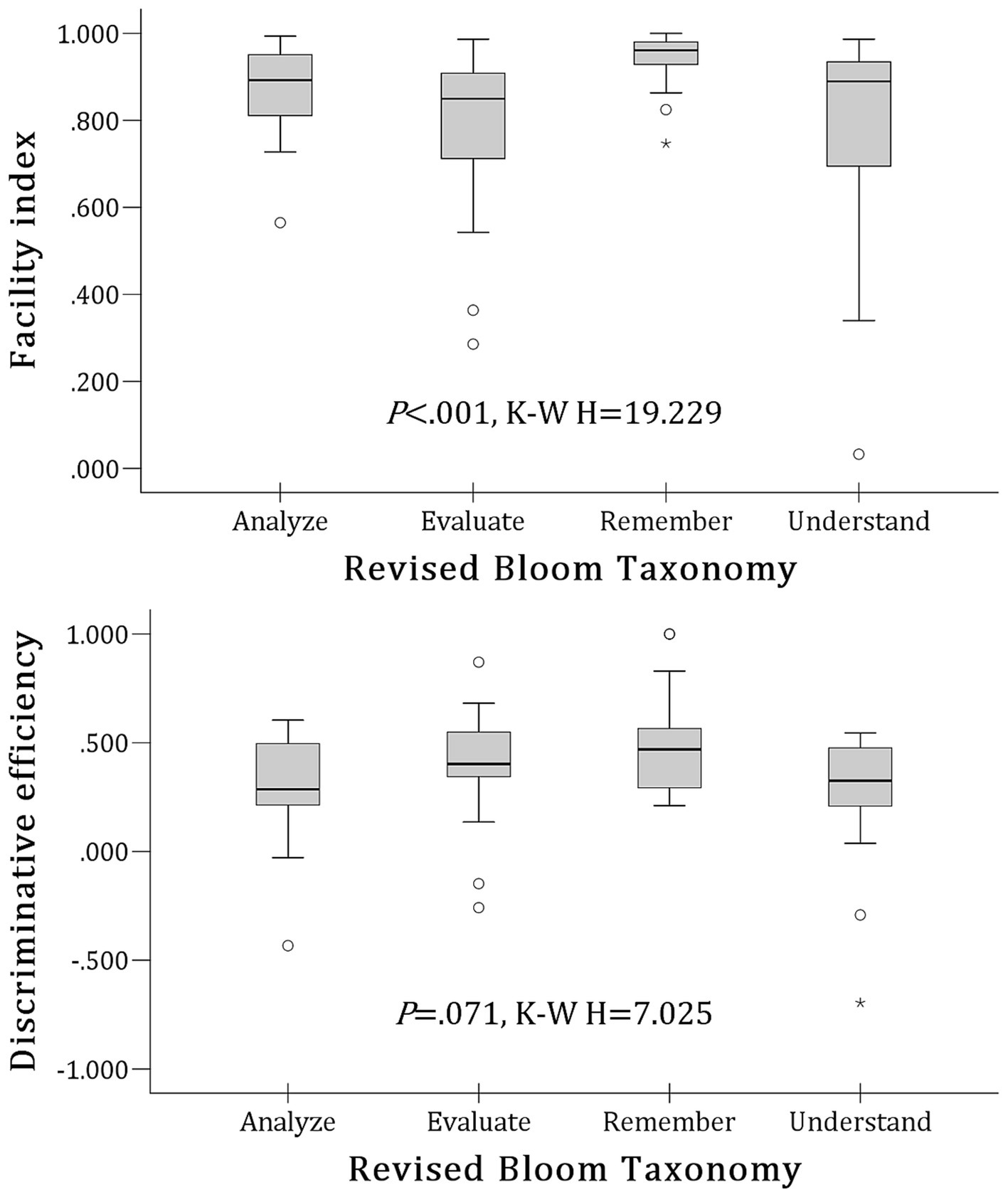
Figure 1. Box plots of the facility index and discriminative efficiency of the included multiple-choice questions (MCQs) stratified per the Bloom’s revised domains. K-W: Kruskal Wallis H test.
3.2 ChatGPT performance in the MCQs
To assess the performance of ChatGPT in the exam, the answers provided were compared to the key answers. Overall, ChatGPT 3.5 provided 64 correct responses out of the 80 MCQs (80.0%). Based on the weight of the answers, the ChatGPT 3.5 score was 80.5 out of 100 grades. Stratified per the revised Bloom’s domains, the best performance was observed for the Remember domain (23/26 correct responses, 88.5%), followed by Understand domain (14/17 correct responses, 82.4%), followed by Analyze domain (9/12 correct responses, 75.0%), and finally the Evaluate domain (18/25 correct responses, 72.0%, p = 0.492, χ23 = 2.41).
Upon comparing the correct MCQs responses by ChatGPT (n = 64) vs. incorrect responses (n = 16), the facility index was higher for those with correct responses (mean: 0.857 ± 0.184, median: 0.922, IQR = 0.825–0.961) compared to incorrect responses (mean: 0.816 ± 0.149, median: 0.853, IQR = 0.690–0.950, p = 0.103, M-W U = 376.5).
Then, the ChatGPT responses were scored per the two raters for correctness and clarity and the results are shown in (Table 2).
When categorized into two groups using the median of stem word count in the MCQs (≤11 words vs. >11 words), there was no statistically significant difference observed between ChatGPT correct and incorrect responses (8/40 for both, p = 1.00). However, when considering the word count of choices (≤15 words vs. >15 words), it was noted that incorrect responses were more frequent among choices with a higher number of words (4/40 vs. 12/40, p = 0.025, χ21 = 5.0).
3.3 Descriptive evaluation of ChatGPT responses based on the raters’ scores
To delineate the performance of ChatGPT across different cognitive domains, and MCQs’ metrics were compared the ChatGPT performance across correct vs. incorrect responses. In the descriptive analysis of ChatGPT 3.5 performance across different cognitive domains, the facility indices and discriminative efficiencies were higher for the MCQs answered correctly as opposed to those answered incorrectly; however, these differences lacked statistical significance (Table 3).
Upon comparing the average raters scores for clarity and correctness across the four domains, statistically significant differences were observed with higher scores for the correct ChatGPT answers. Upon grouping the Understand and Remember as one group (lower cognitive) vs. Evaluate and Analyze (higher cognitive), a higher percentage of correct responses was observed for the lower cognitive category (27/37 vs. 37/43, p = 0.145, χ21 = 2.124).
Finally, in comparison to the students’ performance, where the mean was 86.21 ± 8.04, median = 89.00, range: 42.00–98.00, ChatGPT 3.5 performance can be considered below average with a final score of 80.5 out of 100 grades.
3.4 Evaluation of the quality of study design and reporting of findings using the METRICS checklist
To assess the quality of study design, methods, and reporting of the findings, we used the METRICS checklist. Answering the checklist questions revealed one weakness in terms of absence of randomization in selecting the dataset. The overall attributes of the study design and quality of reporting is shown in (Table 4).
4 Discussion
The current study was based on a meticulous evaluation of ChatGPT 3.5 capacity to respond to medical microbiology subject in higher education assessed through a dataset of MCQs mostly in the medical virology topics. Despite passing the exam with a score of 80.5/100, ChatGPT 3.5 demonstrated a sub-optimal level of performance, compared to the students. This highlights the accuracy issues of ChatGPT 3.5 which was highlighted previously in various recent studies (Giansanti, 2023; Li et al., 2023; Roumeliotis and Tselikas, 2023; Sallam, 2023).
In the context of MCQs, it is important to scrutinize ChatGPT performance through the lens of cognitive domains. This is relevant since these distinctions could reveal minor variations in ChatGPT abilities with subsequent implications on its potential use as a powerful tool in higher education (Bai et al., 2023). Thus, the major contribution of the current study in relation to the existent literature is the use of the revised Bloom’s taxonomy as the framework to dissect the performance of ChatGPT based on different cognitive domains. The added value was also related to the comparative analysis of human students vs. ChatGPT performance as well as the focus on medical microbiology topic which gave insights on ChatGPT unique performance characteristics in a specialized topic.
Notably, in this study, the highest degree of correctness was observed in the Remember domain, where ChatGPT 3.5 answered 88.5% MCQs correctly. In contrast, a marginal decline in performance, albeit lacking statistical significance, was observed across the MCQs that required higher cognitive abilities. Speifically, ChatGPT 3.5 correctly answered 82.4% of the MCQs in the Understand domain, 75% in the Analyze domain, and 72.0% in the Evaluate domain. Herrmann-Werner et al. initiated the use of Bloom’s taxonomy in assessment of ChatGPT cognitive abilities in psychosomatic medicine and psychotherapy MCQ-based exams using a large dataset (Herrmann-Werner et al., 2023). Compared to our findings that showed better ChatGPT performance in lower cognitive domains —albeit without statistical significance— Herrmann-Werner et al. revealed worse ChatGPT performance in lower cognitive skills manifested in errors predominantly in the “Remember” and “Understand” domains, with fewer errors in “Apply,” and very few in the “Analyze” and “Evaluate” domains (Herrmann-Werner et al., 2023). This highlights the necessity for an ongoing assessment of AI-based models, considering variations in subjects and evaluation methods to ensure reliable conclusions about ChatGPT performance across different cognitive domains. Additionally, the accuracy of ChatGPT was clearly affected by the number of words in the MCQ choices which should be considered in the future studies to confirm this tentative link.
In this study, when compared against the performance of human students, ChatGPT 3.5 final score of 80.5 out of 100 grades assumes a position below the average achieved by human students. While ChatGPT demonstrated a level of competency in addressing a substantial proportion of the MCQs, it did not ascend to a level that would equate or surpass the achievements of students. This was shown in various studies across different tested subjects, where ChatGPT was unable to pass these exams highlighting the accuracy issues and the need to continuously improve these models. Specifically, ChatGPT failed to pass Section 1 of the Fellowship of the Royal College of Surgeons (FRCS) examination in Trauma and Orthopaedic Surgery, with the results indicating ChatGPT deficits in the advanced judgment and multidimensional thinking necessary to pass the exam (Cuthbert and Simpson, 2023). Another study showed that ChatGPT proficiency in parasitology lagged behind that of medical students (Huh, 2023).
In contrast, other studies indicated the superior performance of ChatGPT compared to the students. For example, a study evaluating ChatGPT performance in the Self-Assessment Neurosurgery Exams (SANS) of the American Board of Neurological Surgery found that ChatGPT 3.5 and ChatGPT 4 achieved scores of 73.4 and 83.4%, respectively, compared to the user average of 73.7% (Rohaid et al., 2023). Additionally, ChatGPT successfully achieved a passing score in the German state licensing exam at the Progress Test Medicine level and demonstrated superior performance compared to the majority of medical students in their first to third years of study (Friederichs et al., 2023). Therefore, more studies are needed to reach reliable conclusions about the performance of AI-based models in comparison to the performance of students in higher education. Additionally, it is important to consider the performance of the AI-based models taking into account the different language and cultural factors similar to the approach taken by Alfertshofer et al. that tested ChatGPT performance across the US, Italian, French, Spanish, UK, and Indian medical licensing exams (Alfertshofer et al., 2023).
To gain a comprehensive perspective on the study findings, a recent scoping review demonstrated the diverse performance of ChatGPT, influenced by various evaluation methods and varying tested subjects (Newton and Xiromeriti, 2023). This scoping review showed that ChatGPT 3 achieved a passing rate of 20.3%, while ChatGPT 4 excelled with an impressive 92.9% passing rate in the included exams (Newton and Xiromeriti, 2023). Of note, ChatGPT 3 outperformed human students in 10.9% of exams, and ChatGPT 4 surpassed human performance in 35% of the exams (Newton and Xiromeriti, 2023). These comparisons highlighted the potential of ChatGPT in higher educational assessments; nevertheless, it showed the importance of ongoing refinements of these models and the dangers of inaccuracies it poses (Lo, 2023; Sallam, 2023; Sallam et al., 2023d; Gill et al., 2024). However, making direct comparisons across variable studies can be challenging due to differences in models implemented, subject fields of the exams, test dates, and the exact approaches of prompt construction (Holmes et al., 2023; Huynh Linda et al., 2023; Meskó, 2023; Oh et al., 2023; Skalidis et al., 2023; Yaa et al., 2023).
To achieve a comprehensive understanding of ChatGPT capabilities, it is important to test the performance of this AI-based model across different disciplines. In this study, we opted to test ChatGPT performance in Medical Microbiology which is a complex and continuously evolving scientific field. Variability in ChatGPT performance across varying disciplines was shown in previous studies as follows. A recent study by Lai et al. showed that ChatGPT-4 had an average score of 76.3% in the United Kingdom Medical Licensing Assessment, a national undergraduate medical exit exam (Lai et al., 2023). Importantly, the study revealed varied performance across medical specialties, with weaker results in gastrointestinal/hepatology, endocrine/metabolic, and clinical hematology domains as opposed to better performance in the mental health, cancer, and cardiovascular domains (Lai et al., 2023). Additionally, a similar discrepancy in ChatGPT-4 performance across medical subjects (albeit lacking statistical significance) was noticed in a study by Gobira et al. which utilized the 2022 Brazilian National Examination for Medical Degree Revalidation, with worse performance in preventive medicine (Gobira et al., 2023).
In a study by Baglivo et al. (2023) that utilized various styles of vaccination-related questions, different AI-based models outperformed medical students. This contrasts our finding of below average performance of ChatGPT compared to the students. A plausible explanation for this discrepancy can be related to different question styles and different exam settings. Taken together, this highlights the need to assess the performance of AI-based models in various disciplines, using different questions’ format, and compared to human performance (Borchert et al., 2023; Chen et al., 2023; Deiana et al., 2023; Flores-Cohaila et al., 2023; Puladi et al., 2023).
Finally, it is important to acknowledge the limitations inherent in this study. The limited number of MCQs, though a deliberate choice to ensure standardization, may limit the comprehensiveness of the performance evaluation. The subjective nature of evaluating clarity and overall correctness introduces an element of bias, warranting caution in the interpretation of results. Employing standardized tools for evaluating AI-generated output presents a superior alternative strategy (Sallam et al., 2023a). Furthermore, the study exclusively focused on medical microbiology, particularly medical virology, which warrants consideration, as generalizability to other academic disciplines may be restricted. Additionally, this study could not fully capture the potential improvements or updates in ChatGPT performance over time, as large language models continue to evolve. External factors like online exam conditions and format may have influenced the exam metrics and these conditions were not fully considered in the analysis conducted in this study (Newton, 2023; Newton and Essex, 2023).
5 Conclusion
ChatGPT successfully passed a medical microbiology exam but performed below the human students. The study findings highlighted ChatGPT 3.5 ability to solve MCQs across different cognitive domains, with the highest level of accuracy in the Remember domain. However, improvement is needed, especially for MCQs with longer choices, to match the human performance. This study could have implications for using AI-model like ChatGPT in higher education and emphasizes the need for ongoing improvement for better performance highlighting the issue of inaccuracy. With updates much needed to improve ChatGPT abilities in higher cognitive domains, the AI-based models can be a valuable tool for personalized learning through providing accurate explanation and reasoning for selecting the key answers. Lastly, the study opens the door to a broader inquiry into the validity and reliability of MCQ-based assessments in higher education since passing these exams can be achieved by AI-models. Thus, refined approaches for effective design of MCQs is needed to maintain the reliability of MCQs as an assessment method in higher education (Gonsalves, 2023). Future studies are recommended taken into consideration the issues of rigorous design, variable tested subjects, different language and cultural aspects, and different exam settings.
Data availability statement
The data analyzed in this study is subject to the following licenses/restrictions: These are exam questions that cannot be made available publicly. The data presented in this study are available on request from the corresponding author (MS). Requests to access these datasets should be directed to bWFsaWsuc2FsbGFtQGp1LmVkdS5qbw==.
Ethics statement
Ethical approval was not required for the study involving human data in accordance with the local legislation and institutional requirements. Written informed consent to participate in this study was not required from the participants in accordance with the national legislation and the institutional requirements.
Author contributions
MS: Conceptualization, Data curation, Formal analysis, Investigation, Methodology, Project administration, Resources, Software, Supervision, Validation, Visualization, Writing – original draft, Writing – review & editing. KA-S: Formal analysis, Investigation, Methodology, Writing – review & editing.
Funding
The author(s) declare that no financial support was received for the research, authorship, and/or publication of this article.
Conflict of interest
The authors declare that the research was conducted in the absence of any commercial or financial relationships that could be construed as a potential conflict of interest.
The author(s) declared that they were an editorial board member of Frontiers, at the time of submission. This had no impact on the peer review process and the final decision.
Publisher’s note
All claims expressed in this article are solely those of the authors and do not necessarily represent those of their affiliated organizations, or those of the publisher, the editors and the reviewers. Any product that may be evaluated in this article, or claim that may be made by its manufacturer, is not guaranteed or endorsed by the publisher.
References
Abdaljaleel, M., Barakat, M., Alsanafi, M., Salim, N. A., Abazid, H., Malaeb, D., et al. (2023). Factors influencing attitudes of university students towards ChatGPT and its usage: a multi-National Study Validating the TAME-ChatGPT survey instrument. Preprints [Epub ahead of preprint] doi: 10.20944/preprints202309.1541.v1
Alfertshofer, M., Hoch, C. C., Funk, P. F., Hollmann, K., Wollenberg, B., Knoedler, S., et al. (2023). Sailing the seven seas: a multinational comparison of ChatGPT's performance on medical licensing examinations. Ann. Biomed. Eng., 1–4. doi: 10.1007/s10439-023-03338-3 [Online ahead of print].
Anderson, L. W., and Krathwohl, D. R. (2001). A taxonomy for learning, teaching, and assessing: A revision of Bloom's taxonomy of educational objectives. Longman: Addison Wesley Longman, Inc.
Antaki, F., Touma, S., Milad, D., El-Khoury, J., and Duval, R. (2023). Evaluating the performance of ChatGPT in ophthalmology: an analysis of its successes and shortcomings. Ophthalmol. Sci. 3:100324. doi: 10.1016/j.xops.2023.100324
Baglivo, F., De Angelis, L., Casigliani, V., Arzilli, G., Privitera, G. P., and Rizzo, C. (2023). Exploring the possible use of AI Chatbots in public health education: feasibility study. JMIR Med. Educ. 9:e51421. doi: 10.2196/51421
Bai, L., Liu, X., and Su, J. (2023). ChatGPT: the cognitive effects on learning and memory. Brain-X 1:e30. doi: 10.1002/brx2.30
Bloom, B. S., and Krathwohl, D. R. (1956). Taxonomy of educational objectives: The classification of educational goals: Longmans, Green. Longmans, Green.
Borchert, R. J., Hickman, C. R., Pepys, J., and Sadler, T. J. (2023). Performance of ChatGPT on the situational judgement test-a professional dilemmas-based examination for doctors in the United Kingdom. JMIR Med. Educ. 9:e48978. doi: 10.2196/48978
Brown, G. T. L., and Abdulnabi, H. H. A. (2017). Evaluating the quality of higher education instructor-constructed multiple-choice tests: impact on student grades. Front. Educ. 2:24. doi: 10.3389/feduc.2017.00024
Carrasco, J. P., García, E., Sánchez, D. A., Porter, E., De La Puente, L., Navarro, J., et al. (2023). ¿Es capaz “ChatGPT” de aprobar el examen MIR de 2022? Implicaciones de la inteligencia artificial en la educación médica en España. Revista Española de Educación Médica 4, 55–69. doi: 10.6018/edumed.556511
Chan, C. K. Y. (2023). A comprehensive AI policy education framework for university teaching and learning. Int. J. Educ. Technol. High. Educ. 20:38. doi: 10.1186/s41239-023-00408-3
Chang, J., Park, J., and Park, J. (2023). Using an artificial intelligence Chatbot in scientific inquiry: focusing on a guided-inquiry activity using Inquirybot. Asia Pac. Sci. Educ. 9, 44–74. doi: 10.1163/23641177-bja10062
Chen, T. C., Multala, E., Kearns, P., Delashaw, J., Dumont, A., Maraganore, D., et al. (2023). Assessment of ChatGPT's performance on neurology written board examination questions. BMJ Neurol. Open 5:e000530. doi: 10.1136/bmjno-2023-000530
Cheung, B. H. H., Lau, G. K. K., Wong, G. T. C., Lee, E. Y. P., Kulkarni, D., Seow, C. S., et al. (2023). ChatGPT versus human in generating medical graduate exam multiple choice questions-a multinational prospective study (Hong Kong S.A.R., Singapore, Ireland, and the United Kingdom). PLoS One 18:e0290691. doi: 10.1371/journal.pone.0290691
Cuthbert, R., and Simpson, A. I. (2023). Artificial intelligence in orthopaedics: can chat generative pre-trained transformer (ChatGPT) pass section 1 of the fellowship of the Royal College of surgeons (trauma & Orthopaedics) examination? Postgrad. Med. J. 99, 1110–1114. doi: 10.1093/postmj/qgad053
Deiana, G., Dettori, M., Arghittu, A., Azara, A., Gabutti, G., and Castiglia, P. (2023). Artificial intelligence and public health: evaluating ChatGPT responses to vaccination myths and misconceptions. Vaccines (Basel) 11:1217. doi: 10.3390/vaccines11071217
Dwivedi, Y. K., Sharma, A., Rana, N. P., Giannakis, M., Goel, P., and Dutot, V. (2023). Evolution of artificial intelligence research in technological forecasting and social change: research topics, trends, and future directions. Technol. Forecast. Soc. Chang. 192:122579. doi: 10.1016/j.techfore.2023.122579
Flores-Cohaila, J. A., García-Vicente, A., Vizcarra-Jiménez, S. F., De la Cruz-Galán, J. P., Gutiérrez-Arratia, J. D., Quiroga Torres, B. G., et al. (2023). Performance of ChatGPT on the Peruvian National Licensing Medical Examination: cross-sectional study. JMIR Med. Educ. 9:e48039. doi: 10.2196/48039
Friederichs, H., Friederichs, W. J., and März, M. (2023). ChatGPT in medical school: how successful is AI in progress testing? Med. Educ. Online 28:2220920. doi: 10.1080/10872981.2023.2220920
Fuchs, A., Trachsel, T., Weiger, R., and Eggmann, F. (2023). ChatGPT's performance in dentistry and allergy-immunology assessments: a comparative study. Swiss Dent. J. 134.
Giannos, P., and Delardas, O. (2023). Performance of ChatGPT on UK standardized admission tests: insights from the BMAT, TMUA, LNAT, and TSA examinations. JMIR Med. Educ. 9:e47737. doi: 10.2196/47737
Giansanti, D. (2023). The Chatbots are invading us: a map point on the evolution, applications, opportunities, and emerging problems in the health domain. Life 13:1130. doi: 10.3390/life13051130
Gill, S. S., Xu, M., Patros, P., Wu, H., Kaur, R., Kaur, K., et al. (2024). Transformative effects of ChatGPT on modern education: emerging era of AI Chatbots. Internet Things Cyber-Physical Syst. 4, 19–23. doi: 10.1016/j.iotcps.2023.06.002
Gobira, M., Nakayama, L. F., Moreira, R., Andrade, E., Regatieri, C. V. S., and Belfort, R. Jr. (2023). Performance of ChatGPT-4 in answering questions from the Brazilian National Examination for medical degree revalidation. Rev. Assoc. Med. Bras. 69:e20230848, e20230848. doi: 10.1590/1806-9282.20230848
Gonsalves, C. (2023). On ChatGPT: what promise remains for multiple choice assessment? J. Learn. Dev. Higher Educ. 27:9. doi: 10.47408/jldhe.vi27.1009
Grassini, S. (2023). Shaping the future of education: exploring the potential and consequences of AI and ChatGPT in educational settings. Educ. Sci. 13:692. doi: 10.3390/educsci13070692
Herrmann-Werner, A., Festl-Wietek, T., Holderried, F., Herschbach, L., Griewatz, J., Masters, K., et al. (2023). Assessing ChatGPT’s mastery of Bloom’s taxonomy using psychosomatic medicine exam questions. medRxiv, [Epub ahead of preprint]. doi: 10.1101/2023.08.18.23294159
Holmes, J., Liu, Z., Zhang, L., Ding, Y., Sio, T. T., McGee, L. A., et al. (2023). Evaluating large language models on a highly-specialized topic, radiation oncology physics. Front. Oncol. 13:1219326. doi: 10.3389/fonc.2023.1219326
Huang, A. Y. Q., Lu, O. H. T., and Yang, S. J. H. (2023). Effects of artificial intelligence–enabled personalized recommendations on learners’ learning engagement, motivation, and outcomes in a flipped classroom. Comput. Educ. 194:104684. doi: 10.1016/j.compedu.2022.104684
Huh, S. (2023). Are ChatGPT’s knowledge and interpretation ability comparable to those of medical students in Korea for taking a parasitology examination?: a descriptive study. J. Educ. Eval. Health Prof. 20:1. doi: 10.3352/jeehp.2023.20.1
Huynh Linda, M., Bonebrake Benjamin, T., Schultis, K., Quach, A., and Deibert Christopher, M. (2023). New artificial intelligence ChatGPT performs poorly on the 2022 self-assessment study program for urology. Urol. Pract. 10, 409–415. doi: 10.1097/UPJ.0000000000000406
Ibrahim, H., Liu, F., Asim, R., Battu, B., Benabderrahmane, S., Alhafni, B., et al. (2023). Perception, performance, and detectability of conversational artificial intelligence across 32 university courses. Sci. Rep. 13:12187. doi: 10.1038/s41598-023-38964-3
Joshi, L. T. (2021). Using alternative teaching and learning approaches to deliver clinical microbiology during the COVID-19 pandemic. FEMS Microbiol. Lett. 368:fnab103. doi: 10.1093/femsle/fnab103
Kamalov, F., Santandreu Calonge, D., and Gurrib, I. (2023). New era of artificial intelligence in education: towards a sustainable multifaceted revolution. Sustainability 15:12451. doi: 10.3390/su151612451
Kimmerle, J., Timm, J., Festl-Wietek, T., Cress, U., and Herrmann-Werner, A. (2023). Medical students’ attitudes toward AI in medicine and their expectations for medical education. medRxiv, [Epub ahead of preprint] doi: 10.1101/2023.07.19.23292877
Kung, T. H., Cheatham, M., Medenilla, A., Sillos, C., De Leon, L., Elepaño, C., et al. (2023). Performance of ChatGPT on USMLE: potential for AI-assisted medical education using large language models. PLOS Digit. Health 2:e0000198. doi: 10.1371/journal.pdig.0000198
Lai, U. H., Wu, K. S., Hsu, T. Y., and Kan, J. K. C. (2023). Evaluating the performance of ChatGPT-4 on the United Kingdom medical licensing assessment. Front. Med. (Lausanne) 10:1240915. doi: 10.3389/fmed.2023.1240915
Li, J., Dada, A., Kleesiek, J., and Egger, J. (2023). ChatGPT in healthcare: a taxonomy and systematic review. medRxiv, [Epub ahead of preprint]. doi: 10.1101/2023.03.30.23287899
Liu, Q., Wald, N., Daskon, C., and Harland, T. (2023). Multiple-choice questions (MCQs) for higher-order cognition: perspectives of university teachers. Innov. Educ. Teach. Int. 1-13, 1–13. doi: 10.1080/14703297.2023.2222715
Lo, C. K. (2023). What is the impact of ChatGPT on education? A rapid review of the literature. Educ. Sci. 13:410. doi: 10.3390/educsci13040410
Meskó, B. (2023). Prompt engineering as an important emerging skill for medical professionals: tutorial. J. Med. Internet Res. 25:e50638. doi: 10.2196/50638
Michel-Villarreal, R., Vilalta-Perdomo, E., Salinas-Navarro, D. E., Thierry-Aguilera, R., and Gerardou, F. S. (2023). Challenges and opportunities of generative AI for higher education as explained by ChatGPT. Educ. Sci. 13:856. doi: 10.3390/educsci13090856
Mohammed, M., and Omar, N. (2020). Question classification based on Bloom's taxonomy cognitive domain using modified TF-IDF and word2vec. PLoS One 15:e0230442. doi: 10.1371/journal.pone.0230442
Moldt, J.-A., Festl-Wietek, T., Madany Mamlouk, A., Nieselt, K., Fuhl, W., and Herrmann-Werner, A. (2023). Chatbots for future docs: exploring medical students’ attitudes and knowledge towards artificial intelligence and medical chatbots. Med. Educ. Online 28:2182659. doi: 10.1080/10872981.2023.2182659
Newton, P. M. (2020). “Guidelines for creating online MCQ-based exams to evaluate higher order learning and reduce academic misconduct” in Handbook of academic integrity. ed. S. E. Eaton (Singapore: Springer Nature Singapore), 1–17.
Newton, P. M. (2023). The validity of unproctored online exams is undermined by cheating. Proc. Natl. Acad. Sci. 120:e2312978120. doi: 10.1073/pnas.2312978120
Newton, P. M., Da Silva, A., and Berry, S. (2020). The case for pragmatic evidence-based higher education: a useful way forward? Front. Educ. 5:583157. doi: 10.3389/feduc.2020.583157
Newton, P. M., and Essex, K. (2023). How common is cheating in online exams and did it increase during the COVID-19 pandemic? A systematic review. J. Acad. Ethics, 1–21. doi: 10.1007/s10805-023-09485-5
Newton, P. M., and Xiromeriti, M. (2023). ChatGPT performance on MCQ exams in higher education. A pragmatic scoping review. EdArXiv, [Epub ahead of preprint]. doi: 10.35542/osf.io/sytu3
Oh, N., Choi, G.-S., and Lee, W. Y. (2023). ChatGPT goes to the operating room: evaluating GPT-4 performance and its potential in surgical education and training in the era of large language models. Ann. Surg. Treat. Res. 104, 269–273. doi: 10.4174/astr.2023.104.5.269
OpenAI. (2023). ChatGPT. Available at: https://openai.com/chatgpt
Oztermeli, A. D., and Oztermeli, A. (2023). ChatGPT performance in the medical specialty exam: an observational study. Medicine (Baltimore) 102:e34673. doi: 10.1097/md.0000000000034673
Puladi, B., Gsaxner, C., Kleesiek, J., Hölzle, F., Röhrig, R., and Egger, J. (2023). The impact and opportunities of large language models like ChatGPT in oral and maxillofacial surgery: a narrative review. Int. J. Oral Maxillofac. Surg. 1–11.[Online ahead of print]. doi: 10.1016/j.ijom.2023.09.005
Ramírez-Montoya, M. S., Rodríguez-Abitia, G., Hernández-Montoya, D., López-Caudana, E. O., and González-González, C. (2023). Editorial: open education for sustainable development: contributions from emerging technologies and educational innovation. Front. Educ. 8:1131022. doi: 10.3389/feduc.2023.1131022
Rauschert, E. S. J., Yang, S., and Pigg, R. M. (2019). Which of the following is true: we can write better multiple choice questions. Bull. Ecol. Soc. America 100:e01468. doi: 10.1002/bes2.1468
Rohaid, A., Oliver, Y. T., Ian, D. C., Patricia, L. Z. S., John, H. S., Jared, S. F., et al. (2023). Performance of ChatGPT and GPT-4 on neurosurgery written board examinations. medRxiv, [Epub ahead of preprint]. doi: 10.1101/2023.03.25.23287743
Roumeliotis, K. I., and Tselikas, N. D. (2023). ChatGPT and open-AI models: a preliminary review. Future Internet 15:192. doi: 10.3390/fi15060192
Rudolph, J., Tan, S., and Tan, S. (2023). ChatGPT: bullshit spewer or the end of traditional assessments in higher education? J. Appl. Learn. Teach. 6, 342–363. doi: 10.37074/jalt.2023.6.1.9
Rutherford, S. (2015). E pluribus unum: the potential of collaborative learning to enhance microbiology teaching in higher education. FEMS Microbiol. Lett. 362:fnv191. doi: 10.1093/femsle/fnv191
Sallam, M. (2023). ChatGPT utility in healthcare education, research, and practice: systematic review on the promising perspectives and valid concerns. Healthcare 11:887. doi: 10.3390/healthcare11060887
Sallam, M., Al-Fraihat, E., Dababseh, D., Yaseen, A., Taim, D., Zabadi, S., et al. (2019). Dental students' awareness and attitudes toward HPV-related oral cancer: a cross sectional study at the University of Jordan. BMC Oral Health 19:171. doi: 10.1186/s12903-019-0864-8
Sallam, M., Barakat, M., and Sallam, M. (2023a). Pilot testing of a tool to standardize the assessment of the quality of health information generated by artificial intelligence-based models. Cureus. 15:e49373. doi: 10.7759/cureus.49373
Sallam, M., Barakat, M., and Sallam, M. (2023b). METRICS: establishing a preliminary checklist to standardize design and reporting of artificial intelligence-based studies in healthcare. JMIR Preprints. doi: 10.2196/preprints.54704
Sallam, M., Salim, N. A., Al-Tammemi, A. B., Barakat, M., Fayyad, D., Hallit, S., et al. (2023c). ChatGPT output regarding compulsory vaccination and COVID-19 vaccine conspiracy: a descriptive study at the outset of a paradigm shift in online search for information. Cureus 15:e35029. doi: 10.7759/cureus.35029
Sallam, M., Salim, N. A., Barakat, M., and Al-Tammemi, A. B. (2023d). ChatGPT applications in medical, dental, pharmacy, and public health education: a descriptive study highlighting the advantages and limitations. Narra J. 3:e103. doi: 10.52225/narra.v3i1.103
Seaman, M. (2011). BLOOM'S TAXONOMY: its evolution, revision, and use in the field of education. Curric. Teach. Dialog., 13. Available at: https://www.proquest.com/scholarly-journals/blooms-taxonomy-evolution-revision-use-field/docview/1017893795/se-2?accountid=27719
Skalidis, I., Cagnina, A., Luangphiphat, W., Mahendiran, T., Muller, O., Abbe, E., et al. (2023). ChatGPT takes on the European exam in Core cardiology: an artificial intelligence success story? Eur. Heart J. Digit. Health 4, 279–281. doi: 10.1093/ehjdh/ztad029
Southworth, J., Migliaccio, K., Glover, J., Glover, J. N., Reed, D., McCarty, C., et al. (2023). Developing a model for AI across the curriculum: transforming the higher education landscape via innovation in AI literacy. Comput. Educ. Artif. Intell. 4:100127. doi: 10.1016/j.caeai.2023.100127
Stevens, N. T., McDermott, H., Boland, F., Pawlikowska, T., and Humphreys, H. (2017). A comparative study: do "clickers" increase student engagement in multidisciplinary clinical microbiology teaching? BMC Med. Educ. 17:70. doi: 10.1186/s12909-017-0906-3
Takagi, S., Watari, T., Erabi, A., and Sakaguchi, K. (2023). Performance of GPT-3.5 and GPT-4 on the Japanese medical licensing examination: comparison study. JMIR. Med. Educ. 9:e48002. doi: 10.2196/48002
Xiao, L., Changchang, F., Ziwei, Y., Xiaoling, L., Yuan, J., Zhengyu, C., et al. (2023). Performance of ChatGPT on clinical medicine entrance examination for Chinese postgraduate in Chinese. medRxiv, [Epub ahead of preprint]. doi: 10.1101/2023.04.12.23288452
Keywords: educational research, artificial intelligence, MCQ, cognitive, reasoning
Citation: Sallam M and Al-Salahat K (2023) Below average ChatGPT performance in medical microbiology exam compared to university students. Front. Educ. 8:1333415. doi: 10.3389/feduc.2023.1333415
Edited by:
Carina Soledad González González, University of La Laguna, SpainReviewed by:
Pinaki Chakraborty, Netaji Subhas University of Technology, IndiaBrett Bligh, Lancaster University, United Kingdom
Copyright © 2023 Sallam and Al-Salahat. This is an open-access article distributed under the terms of the Creative Commons Attribution License (CC BY). The use, distribution or reproduction in other forums is permitted, provided the original author(s) and the copyright owner(s) are credited and that the original publication in this journal is cited, in accordance with accepted academic practice. No use, distribution or reproduction is permitted which does not comply with these terms.
*Correspondence: Malik Sallam, bWFsaWsuc2FsbGFtQGp1LmVkdS5qbw==