- Ubiquitous and Personal Computing Laboratory, Faculty of Engineering, Kyoto University of Advanced Science (KUAS), Kyoto, Japan
This study delves into the relationship between students' response time and response accuracy in Kahoot! quiz games, within the context of an app development course for first year university students. Previous research on response time in standardized tests has suggested that longer response time are often linked to correct answers, a phenomenon known as speed-accuracy trade-off. However, our study represents one of the initial investigations into this relationship within gamified online quizzes. Unlike standardized tests, each of our Kahoot! quiz modules consisted of only five single choice or true/false questions, with a time limit for each question and instantaneous feedback provided during game play. Drawing from data collected from 21 quiz modules spanning from 2022 to 2023, we compiled a dataset comprising 4640 response-time/accuracy entries. Mann-Whitney U tests revealed significant differences in normalized response time between correct answers and wrong answers in 17 out of the 21 quiz modules, with wrong answers exhibiting longer response time. The effect size of this phenomenon varied from modest to moderate. Additionally, we observed significant negative correlations between normalized overall response time and overall accuracy across all quiz modules, with only two exceptions. These findings challenge the notion of the speed-accuracy trade-off but align with some previous studies that have identified heterogeneity speed-accuracy relationships in standardized tests. We contextualized our findings within the framework of dual processing theory and underscored their implications to learning assessment in online quiz environments.
1 Introduction
Quizzes serve as brief and informal assessment aimed at quickly gauging students' comprehension of learning materials. They are widely employed across all educational levels and are recognized as valuable pedagogical tools for fostering learning (Lee et al., 2012; Cook and Babon, 2016; Cavadas et al., 2017; Egan et al., 2017; Liang, 2019). In line with advancements in learning technologies, quizzes in the modern educational landscape have evolved to become increasingly cloud-based, interactive, and gamified. Kahoot! stands out as one of the most popular quiz game platforms globally. As of 2019, Kahoot! boasts over 250 million users worldwide, with its usage surging further during the pandemic (Martń-Sómer et al., 2021).
One major advantage of Kahoot! quiz games over traditional quizzes is their ability to be played synchronously in real-time with the entire class. The system automatically evaluates students' responses, providing immediate feedback on the correctness of their answers. This instant feedback allows students to promptly identify errors and enables instructors to address mistakes or pose follow-up questions as necessary. Research indicates that such instantaneous feedback is often more educationally effective compared to feedback delivered after a delay, as commonly seen in traditional quizzes (Thelwall, 2000; Cutri et al., 2016). Another advantage of Kahoot! is its gamification approach. Gamification involves the use of game design elements, such as points, badges, and level-up, in non-game contexts (Deterding et al., 2011). Kahoot! incentives students by rewarding correct and swift responses with higher scores, ranking participants at the top of the leaderboard. Rankings are continuously updated throughout the game, with the top three players displayed on a podium at the end, fostering a sense of competition that can enhance motivation and engagement among students (Licorish et al., 2017; Wang and Tahir, 2020; Liang, 2023). Moreover, Kahoot! quiz games are easily scalable to accommodate large classes, making them suitable for use in classrooms with hundreds of students (Castro et al., 2019; Pfirman et al., 2021; Cortés-Pérez et al., 2023). Additionally, the Kahoot! platform captures a wealth of data on students' behavior and performance during game play. Following each session, a comprehensive report is generated, offering aggregated class statistics and individual student performance breakdowns for each question item. By analyzing the report, instructors can gain deeper insights into students' progress and comprehension. Prior research has demonstrated that Kahoot! positively impacts various aspects of classroom dynamics (Cameron and Bizo, 2019; Castro et al., 2019), including student engagement (Licorish et al., 2017; Cameron and Bizo, 2019; Wang and Tahir, 2020), collaborative learning (Liang, 2023), and learning consolidation (Ismail et al., 2019; Cortés-Pérez et al., 2023) across diverse subjects.
In this study, we aim to explore the relationship between the students' response time and response accuracy in Kahoot! quizzes in an undergraduate course for first year engineering students. We define response time as the duration it takes for a student to formulate an answer to a question item within a Kahoot! quiz game. Historically, response time has held a central role in cognitive and psychometric research (Thorndike et al., 1926; Gulliksen, 1950; Lohman, 1989). Numerous studies have utilized response time as a proxy to study the cognitive processes underlying test performance (Kyllonen and Thomas, 2020), as an indicator of motivation in computer-based tests (Wise and Kong, 2005), and as a parameter in test design (van der Linden, 2009; Ranger et al., 2020; He and Qi, 2023). Response time can be influenced by various factors, including individual differences in motivation, cognitive capacity, attitudes, personality, question difficulty, and trade-offs related to accuracy (Thurstone, 1937; Lohman, 1989; Ferrando and Lorenzo-Seva, 2007; Ranger and Kuhn, 2012; Partchev et al., 2013; Goldhammer et al., 2014). On the other hand, response accuracy reflects a student's ability to answer an item correctly (Goldhammer, 2015). It has been hypothesized that unmotivated students may hastily response without fully considering the question item, and hence short response time implies that the students did not give best effort (Wise and Kong, 2005). This notion aligns with the speed-accuracy trade-off (Heitz, 2014), which suggests a negative relationship between speed and accuracy (Luce, 1991). According to the speed-accuracy trade-off, an increase in one variable (e.g., response time) is often associated with a decrease in the other (e.g., accuracy). However, the speed-accuracy relation can be moderated by individual abilities and traits, as well as the characteristics of question items (Goldhammer et al., 2014).
Despite abundant studies on the speed-accuracy relationship in standard tests and exams, this relationship within the context of gamified online quizzes remains relatively unexplored, with only a few studies addressing this topic (Castro et al., 2019; Neureiter et al., 2020; Martń-Sómer et al., 2021). Given the increasing adoption of gamified online quizzes such as Kahoot! across the educational levels, it is important to understand the relationship between these two potential learning assessment measures. Research on response time holds significant relevance for learning assessment conducted through gamified quiz game systems, as response time can serve as an indicator of students' level of mastery and confidence. Particularly, for easier questions, studies suggest that students' proficiency may be more closely associated with response speed rather than accuracy (Dodonova and Dodonov, 2013). In addition, obtaining correct answers on single-choice or true/false questions may sometimes be attributed to chance rather than genuine understanding. Thus, response time could potentially offer a more nuanced evaluation of learning in Kahoot! quizzes. Our study seeks to contribute new insights into this area of research. In particular, we set out to answer the following research questions: (1) Do correct answers exhibit longer response time than wrong answers in Kahoot! quiz games? and (2) Is there a positive correlation between response time and response accuracy, as suggested by the speed-accuracy trade-off? We investigated these questions by analyzing the response time and response accuracy of first year university engineering students enrolled in an Android app development course.
2 Method
2.1 Design of Kahoot! quiz modules
The analysis encompassed 21 original Kahoot! quiz modules utilized in an Android app development course designed for first-year engineering students during the academic years 2022 and 2023. The course is one of the three tracks offered under the umbrella course titled “Introduction to Design,” alongside “LEGO Mindstorm” and “Micro-Controller and Interfacing.” The objective of these courses is to introduce students to the design process in engineering. Students are required to select one of the three tracks. We explicitly designed the Andriod app development track for students with no prior experience in app development and minimal experience in coding. Consequently, the students enrolled in this course were beginners in the subject. To better accommodate their skill level, we used the more beginner-friendly visual programming environment MIT APP Inventor, rather than the official development environment Android Studio. Detailed information regarding the course design can be referenced in Liang et al. (2021) and Liang (2022).
Each quiz module consisted of five question items, which were either single-choice or true-false types, and typically required approximately 10 min for completion. The majority of the quiz games were centered on the factual and conceptual knowledge of app development, making most of them entry level and not particularly difficult. All question items were covered in the lecture sessions, encompassing fundamental aspects of app development such as UI/UX design, basic programming concepts (variables, loops, conditions, etc.), database management, data visualization, application programming interface, and embedded smartphone sensors. Nonetheless, we acknowledge that correctly answering the questions often required knowledge retrieval, which may be challenging for some students with limited capacity in short-term memory. To accommodate varying difficulty levels, each question item was allotted a fixed duration ranging from 20 to 120 seconds. These design choices align with the prevailing format and instructional use of Kahoot! across diverse educational contexts (Cutri et al., 2016; Castro et al., 2019; Martń-Sómer et al., 2021; Pfirman et al., 2021).
2.2 Data collection process
We employed a convenience sampling approach and included students who enrolled in the Android development course in 2022 and 2023. The inclusion criterion was a maintenance of a higher than 70% attendance rate, resulting in a total of 98 participants (46 Japanese students and 52 international students). The number of students participating in each quiz module ranged from 29 to 58. All participants were 1st-year engineering majors enrolled in an English-medium instruction program at a private university in Japan. The Kahoot! quiz modules were played at the end of each teaching session to facilitate review and reflection on the material covered in the lectures, with all students participating synchronously. Students participated in the quiz game by accessing it via a unique game PIN and inputting a nickname on their smartphones or laptops. During the quiz sessions, question items were projected onto the classroom displays, allowing all students to view and respond to them individually using their own devices. The distribution of students' answers as well as the correct answer were shown every time after all students submitted their answers to a question. This interactive approach encouraged active engagement and provided immediate feedback on understanding and retention of the lecture content. Notably, these quizzes were not utilized for formal evaluation purposes. Nonetheless, the gamification elements of the Kahoot! quizzes, such as the leaderboard, battles, and bonus points for streak, served as external motivators for students to engage with the quiz games and aim for higher scores. Our previous study showed that students found the quiz games enjoyable and fun to compete against their peers. Many students expressed desire to score top three so that their names could appear on the Podium at the end of the gameplay, and they felt frustrated when they did not score well (Liang, 2023). These observations were consistent with the benefits of gamified learning technologies (Wang and Tahir, 2020). This study was approved by the Ethics Review Board at the Kyoto University of Advanced Science.
Following the conclusion of each quiz module, the Kahoot! platform automatically generated a comprehensive report encompassing descriptive statistics, individual student responses to each question item, and their corresponding response times in seconds. This report could be exported into a spreadsheet format with multiple tabs, and our analysis focused primarily on the “RawReportData” tab. A raw report contains a total of N×M records, where N represents the total number of students who participated in a quiz game, and M denotes the number of quiz items. Each record within the raw report contained details of a player's response to a specific quiz item, including their chosen answer, response time, score, and the accuracy of their response.
2.3 Statistical analysis and data visualization
The first step in analyzing the exported report involved the removal of dummy players and unanswered records, as they may skew the data distribution. We opted not to utilized the “Scores (points)” provided in the Kahoot! reports because they already factored in both time and accuracy (Castro et al., 2019). Instead, we derived secondary variables to separately characterize response time and accuracy using variables such as “Answer Time (%),” “Answer Time (seconds),” and “Correct.” For the implementation of the analysis, Python 3.10.5 was employed. We utilized various Python modules, including Pandas, Pingouin, Matplotlib, and Seaborn, to perform data cleaning, statistical analysis, and visualization.
To address RQ1, Mann-Whitney U (MWU) tests (Kerby, 2014) were conducted on each quiz module to explore the disparity in response time between correct responses and wrong responses. The MWU test makes no assumption regarding the underlying data distribution and is suitable for both ordinal and continuous data. It evaluates differences in the overall distribution across groups. As the time allocated to each question varied, response time was normalized over the allotted duration. The normalized response time (NRT) was defined as the response time divided by the total time allocated to a quiz item. Specifically, the variable “Answer Time (%)” from the raw report served as NRT in the MWU tests. The MWU test yielded the U statistic and corresponding p-value at a 95% confidence level. Additionally, two effect size measures, namely rank-biserial correlation (RBC) and common language effect size (CLES), were employed. RBC quantifies the effect size of the MWU test within the range [–1, 1]. We calculated the RBC using the simple difference formula as defined in Kerby (2014). CLES, on the other hand, with a value of 0.50 suggesting no difference between groups (Brooks et al., 2013; Kerby, 2014). Box-and-whisker plots were generated to visually inspect the distribution of NRT for correct and wrong answers to enhance the interpretation of results.
To address RQ2, Pearson's correlation analysis was applied to explore the quantitative relationship between overall score and overall response time for each module. Scatter plots were generated for intuitive visual inspection. For each student participating in a quiz module, the normalized score was computed as the ratio of correct answers, while the normalized overall response time (NORT) was defined as the total answer time for all question items divided by the total time allocated to the entire quiz module.
3 Results
The histogram of the NRT for correct and wrong answers are depicted in Figure 1. The distribution of NRT for wrong answers exhibits a bimodal patterns across many quiz modules, characterized by two distinct peaks at opposite ends. Modules 1, 3-10 displayed a prominent peak on the left side, indicative of rapid guessing behavior among some students. Conversely, Modules 11-13, 19 and 20 showcased a prevalence of slower responses among wrong answers, suggesting a lower level of mastery of the quiz questions among students. In contrast, the distribution of NRT for correct answers predominantly follows a geometric distribution. Correct responses were predominantly swift, with only occasional deviations. Modules 14 and 18 displayed distributions akin to a left-skewed normal distribution, indicating that while most correct answers were not slow, they were not as swift as in other quiz modules. Interestingly, Modules 12 and 20 exhibited distributions close to uniform, implying a wide range of response speeds in obtaining correct answers in these two modules.
Table 1 provides descriptive statistics of NRT in each Kahoot! quiz module, along with the results of MWU test and correlation analysis. In the table, NRTcorrect and NRTwrong represent the NRT of correct and wrong answers in the form of average plus or minus standard deviation, respectively. UMWU, pMWU, RBCMWU, and CLESMWU denote the U statistic, p-value, RBC and CLES of the MWU test. Additionally, rcor and pcor indicate Pearson's correlation coefficient and its corresponding p-value. The average NRT for correct answers was consistently lower than that for wrong answers across all quiz modules. Specifically, the average NRT for correct answers was predominantly below 50%, with only one exception. Conversely, the average NRT for wrong answers exceeded 50% in eight quiz modules. Results from the MWU test revealed significant between-group differences in 17 out of the 21 quiz modules. Among these 17 modules, RBCMWU and CLESMWU ranged between [–0.60, –0.20] and [0.60, 0.80], respectively, indicating modest to moderate effects. The box-and-whisker plots in Figure 2 provide additional confirmation that correct answers are associated with shorter NRT compared to wrong answers.
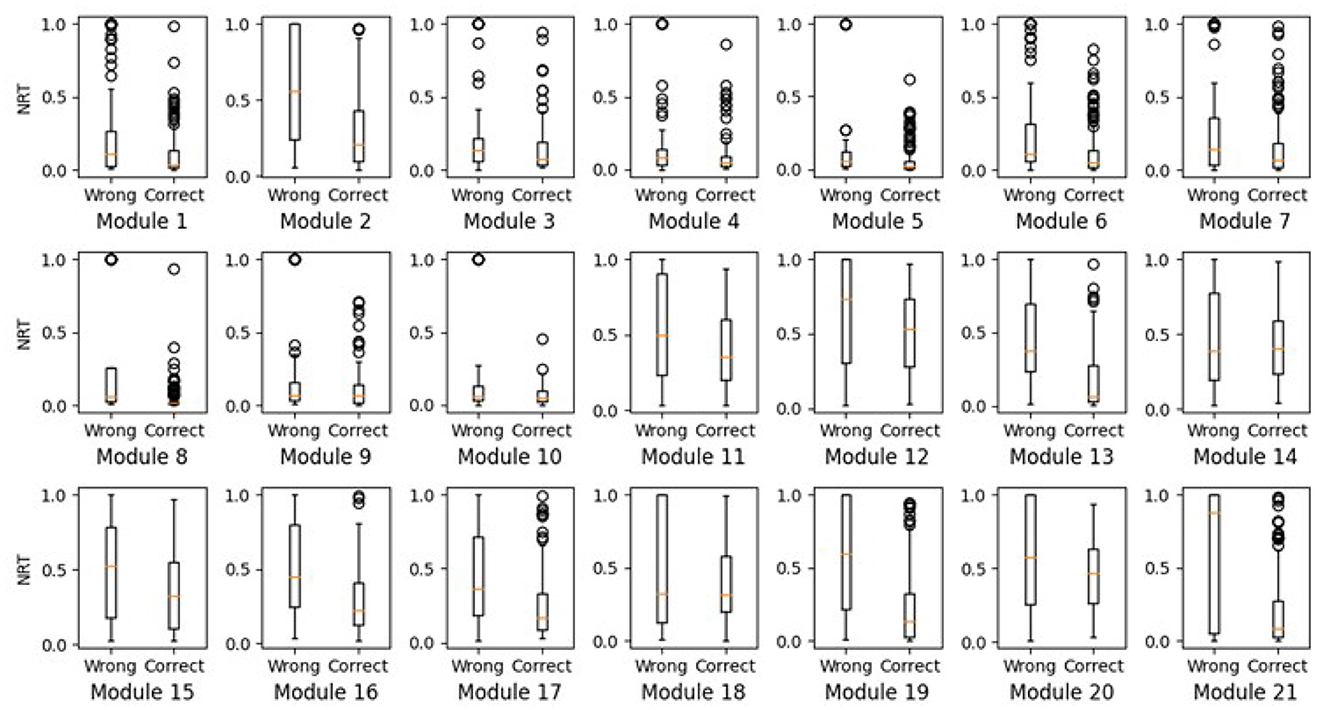
Figure 2. Distributions of normalized answer time of wrong answers and correct answers for each quiz module.
Pearson's correlation analysis uncovered significant and negative correlations between the normalized score and NORT in 13 quiz modules. As shown in Table 1, the Pearson's correlation coefficient ranged between [–0.78, –0.37], indicating modest to strong negative correlations between the two variables.The inverse proportional relationship between the normalized score and NORT was further illustrated by the linear regression lines in the scatter plots displayed in Figure 3, where the shaded regions surrounding the regression lines represent the 95% confidence intervals.
Upon synthesizing the results of the MWU test and correlation analysis, several notable findings emerged. Specifically, Modules 8, 19, and 21 exhibited significant between-group differences with moderate effect sizes, alongside strong and negative correlations between response time and accuracy. Module 8, for instance, displayed a particularly striking pattern, with the average NRT of wrong answers (0.28) nearly ten times that of correct answers (0.03). This module primarily focused on factual knowledge related to the animation components in app development using MIT App Inventor. Sample questions included inquiries about the standard frame per second (FPS) for 2D movies and identifying animation components in MIT App Inventor. Students who possessed the requisite information in their working memory could swiftly retrieve it to answer the questions accurately. Conversely, those lacking such information were more likely to resort to random guessing, resulting in wrong answers. Similar trends were observed in Module 21, where the average NRT for wrong answers (0.60) was three times that of correct answers (0.20). In Module 18, which primarily focused on procedural knowledge, students were prompted to mentally simulate and predict the behavior of simple programs. Despite the cognitive demands of this task, correct answers (0.24) were obtained in less than half the time compared to incorrect answers (0.59). The significant and strong negative correlation (rcor=-0.70) further underscored the relationship between response time and accuracy in this module.
Two modules, Module 9 and 18, displayed no significant between-group differences and lacked correlations between response time and accuracy. Module 9 comprised five questions focusing on factual knowledge of multimedia components in MIT App Inventor, such as identifying media components and the capabilities of the “VideoPlayer” component. Despite the presence of factual questions, Module 9 posed greater difficulty compared to Module 8, as evidenced by the average NRT of correct answers (0.03 in Module 9 vs. 0.11 in Module 8). Similarly, Module 18 primarily examined procedural knowledge related to databases. Three of the five questions required students to mentally simulate program behavior to predict variable values. For example, one question prompted students to predict the value displayed in a text label on the user interface after clicking the 'Save' button. These questions proved to be challenging for all students, as indicated by the extended response times for both correct (0.40 ± 0.25) and wrong (0.47 ± 0.39) answers.
4 Discussion
Our study delved into the speed-accuracy dynamics within Kahoot! quiz games. Across most quiz modules, we observed that incorrect answers were associated with longer response times compared to correct answers, with between-group differences exhibiting modest to moderate effects. Moreover, significant negative correlations between response time and accuracy were evident in the majority of modules, ranging from weak to strong correlations. Echoing prior studies on traditional standardized test (Goldhammer et al., 2014), we observed that the speed-accuracy relationship was moderated by the difficulty of quiz items, which is, in turn, related to the specific topic covered in the lectures. For example, the topic of database in our course appeared to be challenging for all students, resulting in no significant between-group difference in the corresponding quiz module.
The relationship between response time and accuracy in Kahoot! quiz games has not been well-studied and only three prior studies were identified in this space. Comparisons with prior research underscored consistent findings regarding between-group differences, aligning with previous studies across different educational contexts. For instance, an early study found that the question item that showed the highest correct answer rate also registered the lowest answer time (Castro et al., 2019). A more recent study revealed that the response time was significantly lower for the correct answers in each quiz module in a medical course (Neureiter et al., 2020), reflecting a pattern mirrored in our findings. Another study found that the average response time of incorrect answers was slightly longer in a chemical engineering course, but the difference was not significant (Martń-Sómer et al., 2021). In a broader scope, the between-group difference was observed in computer-based test on basic computer skills (Goldhammer et al., 2012) and physics (Lasry et al., 2013). Our study adds further evidence to the between-group difference in the context of engineering education. The effect sizes observed in our study shed light on the nontrivial impact of this phenomenon.
The quantitative relation between response time and accuracy in Kahoot! quiz games was only explored in one prior study where no correlation was found (Neureiter et al., 2020). In contrast, we found primarily negative correlations in our quiz modules, with only two exceptions of no correlation. Positive correlation was not observed in any quiz module.
Interestingly, our findings diverged from the traditional speed-accuracy trade-off, which posits that increased response time correlates with improved accuracy (Luce, 1991). Several factors unique to the setting of Kahoot! quiz games may contribute to this disparity. Unlike traditional tests, Kahoot! imposes time limits on individual questions rather than the entire assessment. This approach ensures that all students are paced at each quiz item, mitigating the need for rushed responses toward the end of the assessment (Wise, 2015). The scoring system incentivizes rapid, accurate responses, deviating from the typical trade-off observed in traditional test settings. Moreover, prior studies have emphasized that the speed-accuracy trade-off is primarily applicable to speeded tasks, where students could potentially provide correct answers given sufficient time. However, for power tasks, where not everyone can arrive at the correct answer even with unlimited time, the traditional speed-accuracy trade-off may not hold. In our Kahoot! quizzes, a majority of the questions focus on factual and conceptual knowledge, representing a form of power task, as knowledge recall plays a pivotal role here.
The variability in the strength and direction of the relationship between response time and accuracy in Kahoot! quiz games, as observed in our study and prior research, may be explained through the lens of dual processing theory (Schneider and Shiffrin, 1977; Shiffrin and Schneider, 1977; Schneider and Chein, 2003). This theory posits that the speed-accuracy relationship is contingent upon the relative degrees of controlled and automatic processing involved in generating responses. In line with this theory, easy questions are typically conducive to automatic processing, whereas difficult questions necessitate more controlled processing (Goldhammer et al., 2014).
The ratio of the two types of processing depends on a person's ability and familiarity with the relevant knowledge, and a negative relation between response time and accuracy can be expected if a person works in the mode of automatic processing to a great extent (Goldhammer et al., 2014). Previous research has highlighted the role of strong memory traces in facilitating quick correct responses (Sporer, 1993). Similarly, we hypothesize that the prolonged response times for incorrect answers in our quiz modules may stem from weak memory representations. Students who struggle to retrieve the necessary knowledge for generating correct responses may become ensnared in cognitive uncertainty, leading to prolonged decision times (Hornke, 2005). This ongoing rumination process may exacerbate confusion, ultimately prompting some students to resort to random guessing as time elapses.
While our study offers valuable insights into the speed-accuracy relationship in Kahoot! quiz games, several limitations warrant consideration and present opportunities for future research. Firstly, our study employed an interpersonal design, focusing primarily on between-subject variations. The neglect of within-subject variations represents a notable limitation. Future studies may benefit from adopting a multilevel approach, which considers both between-subject and within-subject variations (Klein Entink et al., 2009). Secondly, our analysis treated all question items as homogeneous in terms of the relationship between response time and accuracy, overlooking potential differences between speeded tasks and power tasks. Future investigations could delve deeper into the distinct effects of these task types on the speed-accuracy relationship. Understanding how different question types influence students' response strategies and performance could yield valuable insights for educators and instructional designers. Additionally, the sample of our study comprised primarily 1st-year engineering majors, predominantly male. This homogeneity in demographic representation limits the generalizability of our findings to broader student populations. Furthermore, our analysis did not account for potential confounding factors such as motivation and personality, despite these being considered influential on the speed-accuracy relationship in prior studies (Ferrando and Lorenzo-Seva, 2007; Ranger and Kuhn, 2012). Future research should aim to diversify the participant pool to capture a more representative sample, encompassing students from diverse backgrounds and academic disciplines, and factor in potential confounding factors in the analysis. Lastly, the instantaneous feedback provided by Kahoot! quizzes may influence students' response strategies, potentially altering their approach based on the accuracy of previous questions. The order of questions within quizzes could also impact students' performance and response behaviors. Investigating the effects of question order and feedback mechanisms on the speed-accuracy relationship constitutes a promising avenue for future inquiry.
5 Conclusion
Our study offers valuable insights into the complex interplay between response time and accuracy in real-time gamified response systems within higher engineering educational contexts. Through comprehensive analysis, we observed consistent trends wherein incorrect answers were associated with longer response times compared to correct answers. Moreover, significant negative correlations between response time and accuracy were prevalent across most modules. Our discussion underscores the importance of considering response time in addition to response accuracy as a measure to evaluate students' learning in online quiz environments.
Data availability statement
The raw data supporting the conclusions of this article will be made available by the authors, without undue reservation.
Ethics statement
The study was approved by the Ethics Review Board at Kyoto University of Advanced Science. The study was conducted in accordance with the local legislation and institutional requirements. The participants provided their written informed consent.
Author contributions
ZL: Writing – review & editing, Writing – original draft, Visualization, Validation, Supervision, Software, Resources, Project administration, Methodology, Investigation, Formal analysis, Data curation, Conceptualization.
Funding
The author(s) declare that no financial support was received for the research, authorship, and/or publication of this article.
Conflict of interest
The author declares that the research was conducted in the absence of any commercial or financial relationships that could be construed as a potential conflict of interest.
Publisher's note
All claims expressed in this article are solely those of the authors and do not necessarily represent those of their affiliated organizations, or those of the publisher, the editors and the reviewers. Any product that may be evaluated in this article, or claim that may be made by its manufacturer, is not guaranteed or endorsed by the publisher.
References
Brooks, M., Dalal, D., and Nolan, K. (2013). Are common language effect sizes easier to understand than traditional effect sizes? J. Appl. Psychol. 99:332. doi: 10.1037/a0034745
Cameron, K., and Bizo, L. (2019). Use of the game-based learning platform KAHOOT! to facilitate learner engagement in animal science students. Res. Learn. Technol. 27, 1–14. doi: 10.25304/rlt.v27.2225
Castro, M. J., Lopez, M., Cao, M.-J., Fernández-Castro, M., García, S., Frutos, M., et al. (2019). Impact of educational games on academic outcomes of students in the degree in nursing. PLoS ONE 14:e0220388. doi: 10.1371/journal.pone.0220388
Cavadas, C., Godinho, W., Machado, C., and Carvalho, A. (2017). “Quizzes as an active learning strategy: A study with students of pharmaceutical sciences,” in 2017 12th Iberian Conference on Information Systems and Technologies (CISTI), 1–6. doi: 10.23919/CISTI.2017.7975894
Cook, B., and Babon, A. (2016). Active learning through online quizzes: better learning and less (busy) work. J. Geogr. High. Educ. 41, 24–38. doi: 10.1080/03098265.2016.1185772
Cortés-Pérez, I., Zagalaz-Anula, N., Ruiz, C., Daz, A., Obrero-Gaitan, E., and Osuna-Pérez, M. (2023). Study based on gamification of tests through KAHOOT! and reward game cards as an innovative tool in physiotherapy students: a preliminary study. Healthcare 11:578. doi: 10.3390/healthcare11040578
Cutri, R., Marim, L. R., Cordeiro, J. R., Gil, H. A. C., and Guerald, C. C. T. (2016). “KAHOOT, a new and cheap way to get classroom-response instead of using clickers,” in 2016 ASEE Annual Conference and Exposition Proceedings.
Deterding, S., Dixon, D., Khaled, R., and Nacke, L. (2011). “From game design elements to gamefulness: defining “gamification”,” in Proceedings of the 15th International Academic MindTrek Conference: Envisioning Future Media Environments, MindTrek '11 (New York, NY, USA: Association for Computing Machinery), 9–15. doi: 10.1145/2181037.2181040
Dodonova, Y. A., and Dodonov, Y. S. (2013). Faster on easy items, more accurate on difficult ones: cognitive ability and performance on a task of varying difficulty. Intelligence 41, 1–10. doi: 10.1016/j.intell.2012.10.003
Egan, R., Fjermestad, J., and Scharf, D. (2017). “The use of mastery quizzes to enhance student preparation,” in Western Decision Sciences Institute (WDSI) Conference.
Ferrando, P., and Lorenzo-Seva, U. (2007). An item response theory model for incorporating response time data in binary personality items. Appl. Psychol. Meas. 31, 525–543. doi: 10.1177/0146621606295197
Goldhammer, F. (2015). Measuring ability, speed, or both? Challenges, psychometric solutions, and what can be gained from experimental control. Measurement 13, 133–164. doi: 10.1080/15366367.2015.1100020
Goldhammer, F., Naumann, J., and Keýel, Y. (2012). Assessing individual differences in basic computer skills psychometric characteristics of an interactive performance measure. Eur. J. Psychol. Assess. 29:263. doi: 10.1027/1015-5759/a000153
Goldhammer, F., Naumann, J., Stelter, A., Toth, K., Rlke, H., and Klieme, E. (2014). The time on task effect in reading and problem solving is moderated by task difficulty and skill: Insights from a computer-based large-scale assessment. J. Educ. Psychol. 106, 608–626. doi: 10.1037/a0034716
He, Y., and Qi, Y. (2023). Using response time in multidimensional computerized adaptive testing. J. Educ. Measur. 60, 697–738. doi: 10.1111/jedm.12373
Heitz, R. (2014). The speed-accuracy tradeoff: History, physiology, methodology, and behavior. Front. Neurosci. 8. doi: 10.3389/fnins.2014.00150
Hornke, L. (2005). Response time in computer-aided testing: a “verbal memory” test for routes and maps. Psychol. Sci. 47, 280–293.
Ismail, M. A.-A., Ahmad, A., Mohammad, J., Mohd Fakri, N. M. R., Nor, M., and Mat Pa, M. (2019). Using KAHOOT! as a formative assessment tool in medical education: a phenomenological study. BMC Med. Educ. 19, 1–8. doi: 10.1186/s12909-019-1658-z
Kerby, D. (2014). The simple difference formula: an approach to teaching nonparametric correlation. Compr. Psychol. 3:11. doi: 10.2466/11.IT.3.1
Klein Entink, R., Fox, J.-P., and Linden, W. (2009). A multivariate multilevel approach to the modeling of accuracy and speed of test takers. Psychometrika 74, 21–48. doi: 10.1007/s11336-008-9075-y
Kyllonen, P., and Thomas, R. (2020). “Using response time for measuring cognitive ability illustrated with medical diagnostic reasoning tasks,” in Integrating Timing Considerations to Improve Testing Practices (Routledge), 122–141. doi: 10.4324/9781351064781-9
Lasry, N., Watkins, J., Mazur, E., and Ibrahim, A. (2013). Response times to conceptual questions. Am. J. Phys. 81:703. doi: 10.1119/1.4812583
Lee, L., Nagel, R., and Gould, D. (2012). The educational value of online mastery quizzes in a human anatomy course for first-year dental students. J. Dent. Educ. 76, 1195–1199. doi: 10.1002/j.0022-0337.2012.76.9.tb05374.x
Liang, Z. (2019). “Enhancing active learning in web development classes using pairwise pre-and-post lecture quizzes,” in 2019 IEEE International Conference on Engineering, Technology and Education (TALE), 1–8. doi: 10.1109/TALE48000.2019.9225896
Liang, Z. (2022). “Contextualizing introductory app development course for first-year engineering students,” in 2022 IEEE International Conference on Teaching, Assessment and Learning for Engineering (TALE), 86–92. doi: 10.1109/TALE54877.2022.00022
Liang, Z. (2023). “Enhancing learning experience in university engineering classes with KAHOOT! quiz games,” in The 31st International Conference on Computers in Education (ICCE 2023).
Liang, Z., Nishi, M., and Kishida, I. (2021). “Teaching android app development to first year undergraduates: textual programming or visual programming?” in 2021 IEEE International Conference on Engineering, Technology and Education (TALE), 1–8. doi: 10.1109/TALE52509.2021.9678602
Licorish, S., George, J., Owen, H., and Daniel, B. (2017). “go KAHOOT!” enriching classroom engagement, motivation and learning experience with games,” in The 25th International Conference on Computers in Education.
Lohman, D. F. (1989). Individual differences in errors and latencies on cognitive tasks. Learn. Individ. Differ. 1, 179–202. doi: 10.1016/1041-6080(89)90002-2
Luce, R. D. (1991). Response Times: Their Role in Inferring Elementary Mental Organization. Oxford: Oxford University Press. doi: 10.1093/acprof:oso/9780195070019.001.0001
Martín-Sómer, M., Moreira, J., and Casado, C. (2021). Use of KAHOOT! to keep students motivation during online classes in the lockdown period caused by covid 19. Educ. Chem. Eng. 36, 154–159. doi: 10.1016/j.ece.2021.05.005
Neureiter, D., Klieser, E., Neumayer, B., Winkelmann, P., Urbas, R., and Kiesslich, T. (2020). Feasibility of KAHOOT! as a real-time assessment tool in (histo-)pathology classroom teaching. Adv. Med. Educ. Pract. 11, 695–705. doi: 10.2147/AMEP.S264821
Partchev, I., Boeck, P. D., and Steyer, R. (2013). How much power and speed is measured in this test? Assessment 20, 242–252. doi: 10.1177/1073191111411658
Pfirman, S., Hamilton, L., Turrin, M., Narveson, C., and Lloyd, C. A. (2021). Polar knowledge of us students as indicated by an online KAHOOT! quiz game. J. Geosci. Educ. 69, 150–165. doi: 10.1080/10899995.2021.1877526
Ranger, J., and Kuhn, J.-T. (2012). Improving item response theory model calibration by considering response times in psychological tests. Appl. Psychol. Meas. 36, 214–231. doi: 10.1177/0146621612439796
Ranger, J., Kuhn, J.-T., and Ortner, T. (2020). Modeling responses and response times in tests with the hierarchical model and the three-parameter lognormal distribution. Educ. Psychol. Meas. 80:001316442090891. doi: 10.1177/0013164420908916
Schneider, W., and Chein, J. M. (2003). Controlled automatic processing: behavior, theory, and biological mechanisms. Cogn. Sci. 27, 525–559. doi: 10.1207/s15516709cog2703_8
Schneider, W., and Shiffrin, R. M. (1977). Controlled and automatic human information processing: 1 detection, search, and attention. Psychol. Rev. 84, 1–66. doi: 10.1037/0033-295X.84.1.1
Shiffrin, R., and Schneider, W. (1977). Controlled and automatic human information processing: II perceptual learning, automatic attending and a general theory. Psychol. Rev. 84, 127–190. doi: 10.1037/0033-295X.84.2.127
Sporer, S. (1993). Eyewitness identification accuracy, confidence, and decision times in simultaneous and sequential lineups. J. Appl. Psychol. 78, 22–33. doi: 10.1037/0021-9010.78.1.22
Thelwall, M. (2000). Computer-based assessment: a versatile educational tool. Comput. Educ. 1, 37–49. doi: 10.1016/S0360-1315(99)00037-8
Thorndike, E. L., Bregman, E. O., Cobb, M. V., and Woodyard, E. (1926). The Measurement of Intelligence. New York, NY: Teachers College Bureau of Publications. doi: 10.1037/11240-000
Thurstone, L. L. (1937). Ability, motivation, and speed. Psychometrika 2, 249–254. doi: 10.1007/BF02287896
van der Linden, W. J. (2009). Conceptual issues in response-time modeling. J. Educ. Measur. 46, 247–272. doi: 10.1111/j.1745-3984.2009.00080.x
Wang, A., and Tahir, R. (2020). The effect of using KAHOOT! for learning a literature review. Comput. Educ. 149:103818. doi: 10.1016/j.compedu.2020.103818
Wise, S., and Kong, X. (2005). Response time effort: a new measure of examinee motivation in computer-based tests. Appl. Meas. Educ. 18, 163–183. doi: 10.1207/s15324818ame1802_2
Keywords: speed-accuracy, response time, quiz, Kahoot!, gamification, engineering, higher education, app development
Citation: Liang Z (2024) More haste, less speed? Relationship between response time and response accuracy in gamified online quizzes in an undergraduate engineering course. Front. Educ. 9:1412954. doi: 10.3389/feduc.2024.1412954
Received: 06 April 2024; Accepted: 03 June 2024;
Published: 19 June 2024.
Edited by:
Pedro Tadeu, CI&DEI-ESECD-IPG, PortugalReviewed by:
Mohammed F. Farghally, Virginia Tech, United StatesWanda Nugroho Yanuarto, Muhammadiyah University of Purwokerto, Indonesia
Copyright © 2024 Liang. This is an open-access article distributed under the terms of the Creative Commons Attribution License (CC BY). The use, distribution or reproduction in other forums is permitted, provided the original author(s) and the copyright owner(s) are credited and that the original publication in this journal is cited, in accordance with accepted academic practice. No use, distribution or reproduction is permitted which does not comply with these terms.
*Correspondence: Zilu Liang, bGlhbmcuemlsdUBrdWFzLmFjLmpw