- 1Department of Learning Sciences, Georgia State University, Atlanta, GA, United States
- 2Department of Psychology, University of Memphis, Memphis, TN, United States
Purpose: College placement assessments often overlook multilingual learners’ full linguistic abilities and literacy engagement, as standardized tests primarily assess English proficiency rather than how students interact with academic texts. Directed Self-Placement (DSP) offers an alternative approach through self-assessment, with some models incorporating post-task self-ratings of students’ competence beliefs. However, this approach does not fully capture how motivation (i.e., competence beliefs and task value) interacts with varying literacy skills within a task. This exploratory study applies Explanatory Item Response Models (EIRMs) to examine how self-rated motivation relates to vocabulary performance on higher-and lower-frequency words, offering insights to refine DSP frameworks for multilingual learners.
Method: A total of 39 multilingual learners and 249 monolingual English-speaking college students completed a vocabulary assessment and responded to self-report motivation scale questions assessing their reading motivation. Item-level analyses were implemented to examine the interaction between motivation and multilingual learner status on higher-and lower-frequency words.
Results: Lower-frequency words posed greater challenges across all participants but disproportionately undermined the performance of multilingual learners with lower reading competence beliefs. However, multilingual learners with higher reading competence performed comparably to monolingual English speakers on both higher and lower frequency words.
Conclusion: EIRM-based analyses offer novel insights into the ways that motivation interacts with different dimensions of literacy performance at the item level. Future research should develop validated self-assessment tools that incorporate additional aspects of motivation and multilingual learners’ linguistic strategies, which could inform more equitable placement practices.
Introduction
As linguistic diversity in U.S. postsecondary education continues to grow, multilingual learners now make up a significant portion of college students (Biondi et al., 2024; Aguirre-Muñoz et al., 2024). This population includes first-generation college students, recent immigrants, and those maintaining their heritage languages while navigating English-dominant academic environments, demonstrating varying levels of proficiency in both their home languages and English (de Kleine and Lawton, 2015). Despite their linguistic competencies, multilingual students often encounter biases in college placement, where high-stakes assessments such as the SAT, ACT, and ACCUPLACER play a dominant role (Hilgers, 2019; Llosa and Bunch, 2011). Standardized tests frequently fail to assess these students accurately, even when their spoken English proficiency is sufficient for credit-bearing coursework (Nelson et al., 2024). By 2018, multilingual students from immigrant families accounted for 28% (5 million) of U.S. college students, underscoring the need for placement assessments that better reflect their academic abilities and support their success in higher education (Nelson et al., 2024).
To reduce biases in standardized college placement testing for multilingual learners, some institutions have adopted Directed Self-Placement (DSP), an alternative approach that incorporates student self-reflection to guide placement decisions (e.g., Ferris et al., 2017; Baker et al., 2024). One common method involves students evaluating their self-efficacy, or their beliefs about their own literacy abilities (Aull, 2021; Jones, 2008; Reynolds, 2003; Toth and Aull, 2014), which aligns with theoretical models of motivation in education (Eccles et al., 1983; Eccles and Wigfield, 2002; Wigfield and Eccles, 2002). However, general motivational self-report measures often fail to capture how motivation influences academic performance in specific literacy contexts, underscoring the need for more refined self-assessment tools tailored to different learner populations (Aull, 2021; Toth and Aull, 2014). Analyzing how self-rated motivation corresponds with performance-based measures at the item level may address this need by revealing patterns in how students’ attitudes and motivation fluctuate with varying literacy task demands—insights often overlooked by traditional aggregate scores (Wilson et al., 2008).
To contribute to the growing literature on DSP, this exploratory study examined how self-rated motivation interacts with item-level performance on a vocabulary task. Participants included multilingual learners and monolingual English learners enrolled at a southeastern U.S. university, a region with growing multilingual K-12 populations. This diverse sample allows for a comparative analysis of how self-assessment, linguistic background, and motivation influence literacy performance. While all participants met English proficiency requirements for credit-bearing courses, this study identifies preliminary trends to inform future research on equitable testing frameworks for multilingual learners with varying levels of English proficiency. Because reading comprehension can be integrated into DSP practices for multilingual learners (e.g., Ferris et al., 2017), this study analyzes engagement with higher-and lower-frequency vocabulary words at the item level. These vocabulary types play distinct roles in text comprehension (Schmitt, 2014) and may offer insight into how motivation interacts with academic preparedness for multilingual learners in English-dominant settings.
The role of vocabulary in multilingual learners’ higher education outcomes
Testing frameworks, including standardized placement assessments and alternative non-standardized models, in higher education often prioritize reading comprehension as a key measure of academic readiness. However, reading comprehension in English-dominant settings relies on foundational language skills, particularly vocabulary knowledge, which plays a crucial role in processing complex, discipline-specific texts (Perfetti and Stafura, 2014; Kaldes et al., 2024; Magliano et al., 2023; Perin, 2020). Both English vocabulary breadth (the number of words a student knows) and vocabulary depth (the nuanced understanding of word meanings) are strongly associated with reading comprehension (Ibrahim et al., 2016; Şen and Kuleli, 2015) and are further linked to broader academic success for multilingual college students (Alsahafi, 2023; Loewen and Ellis, 2004; Masrai and Milton, 2021).
For college multilingual learners, developing vocabulary knowledge in English may represent both opportunities for growth and a means to leverage existing strengths by drawing on home language skills, regardless of their English proficiency (García and Wei, 2014). For example, academic texts often include low-frequency vocabulary words, which consist of specialized and uncommon terms that multilingual learners of all linguistic skill levels must continue acquiring beyond early grade-school years (Mancilla-Martinez et al., 2021; Nagy and Townsend, 2012). Additionally, college-level texts may present challenges because they incorporate low-incidence words, domain-specific vocabulary, and complex syntax (Burton, 2014). Nonetheless, research with child multilingual learners emphasizes significant benefits of multilingualism for vocabulary development, as multiple languages can help reading comprehension and academic achievement (Prevoo et al., 2016; Ramirez and Kuhl, 2017; Calafato, 2022). These skills enable them to navigate academic texts with flexibility and resilience, bridging gaps in their English vocabulary. For example, recognizing a word’s root in their home language can help infer its meaning in English, demonstrating their ability to integrate linguistic and cognitive resources across languages (Ramirez, 2009; Kieffer and Lesaux, 2012).
Rethinking placement assessment for multilingual learners in higher education
Multilingual learners bring strengths to literacy that are rooted in translanguaging theory, which posits that they fluidly leverage their entire linguistic repertoire to construct meaning (García and Wei, 2014). Standardized assessments that incorporate translanguaging practices can more accurately reflect multilingual learners’ abilities, yet such supports are rarely included in postsecondary placement assessments (Chalhoub-Deville, 2019; Gottlieb, 2023; Nelson et al., 2024; Sireci, 2020). This gap is significant because, even in untimed conditions, placement tests often fail to accommodate the longer, cross-linguistic processing multilingual learners use to construct meaning, instead focusing narrowly on surface-level accuracy (Alshammari, 2018; Grabe, 2009; Pitoniak et al., 2009; Ramírez, 2000; Suzuki and Sunada, 2018). As a result, standardized college placement assessments may highlight perceived deficits rather than recognizing metalinguistic strengths in reading comprehension, such as cross-linguistic strategy use and advanced conceptual knowledge (Nelson et al., 2024; Prevoo et al., 2016; Ramirez and Kuhl, 2017). This monolingual focus may not only lead to over-placement in remedial or developmental courses but may also delay access to required or advanced coursework, limiting academic progress (Ferris and Lombardi, 2020; Key, 2024; Melguizo et al., 2021).
Unfortunately, developing and implementing translanguaging in standardized assessments requires significant time, resources, and policy shifts, as translanguaging demands flexible test designs, bilingual resources, and innovative scoring systems (Badham and Furlong, 2023; Ferris and Lombardi, 2020; Lopez et al., 2017). Given these constraints, a more immediate and practical approach may involve using the Directed Self-Placement framework, which considers how multilingual college learners engage in self-reflection to guide the placement process (e.g., Ferris et al., 2017). Understanding student motivation is important to this process, as students’ confidence in their literacy skills and engagement levels influence their approach to reading and writing in academic contexts (Aull, 2021; Eccles and Wigfield, 2023, 2024).
The role of motivation
Motivation plays a critical role in college students’ academic success, particularly for studying and essay writing, which require active problem-solving and sustained use of metacognitive strategies (Britt et al., 2017; Rouet, 2006; Rouet et al., 2017; Snow, 2002). Theories of self-regulated learning emphasize the integration of cognitive and motivational factors in guiding students’ learning processes (Boekaerts, 1991, 1992; Efklides and Schwartz, 2024; Pintrich, 2000; Zimmerman, 1989). Expectancy-value theory (EVT) further delineates motivation by identifying two core components: competence beliefs (i.e., perceived self-efficacy in one’s abilities), which correspond to expectancy, and the perceived importance or enjoyment of tasks, which define task value (Eccles et al., 1983; Eccles and Wigfield, 2002; Wigfield, 1994; Wigfield and Eccles, 1992, 2002).
Translanguaging theory contextualizes how motivation fosters reading among multilingual learners, enabling them to navigate monolingual educational systems that often overlook their linguistic strengths (García and Wei, 2014; Gu et al., 2024). For example, Liu et al. (2024) examined how self-efficacy and engagement, two motivational constructs that align with EVT, influenced the relationship between home language and English reading among Chinese elementary school students. They found that self-efficacy in the home language significantly contributed to English reading comprehension through cross-linguistic transfer, highlighting the importance of leveraging home language literacy skills. Additionally, intrinsic motivation, which is linked to enjoyment and interest in reading, positively influenced comprehension in both the home language and English.
Previous research on multilingual college students suggests that translanguaging aligns with key motivational constructs in EVT. For example, Kamhi-Stein (2003) found that multilingual college students in the U.S. who viewed their home language as an asset were more likely to use cross-linguistic strategies, such as mental translation, to navigate complex academic texts. This positive perception reflects confidence in drawing on home language skills to support reading comprehension in English, aligning with competence beliefs in EVT. Additionally, students who recognized their cultural and linguistic knowledge as valuable for academic success found learning more engaging and meaningful, reinforcing the construct of task value (Eccles and Wigfield, 2002; Kamhi-Stein, 2003). This aligns with evidence that college students studying English as a foreign language make better progress when they demonstrate a growth mindset about their ability to improve (Calafato and Simmonds, 2023). Growth mindset is closely related to self-efficacy, as confidence in one’s ability to improve fosters resilience and persistence (Blackwell et al., 2007; Dweck, 2009; Rhew et al., 2018). Similarly, Rutgers et al. (2024) found that learners’ self-perceptions as multilinguals, including their linguistic, emotional, and evaluative connections to multiple languages, serve as a foundation for fostering resilience and creativity when engaging with complex texts in English.
Directed self-placement
Directed Self-Placement (DSP) is tied to concepts of motivation in academic success because it offers a student-centered approach to course placement by emphasizing self-assessment and agency (Royer and Gilles, 1998). Unlike standardized placement methods, DSP can incorporate motivation by using reflective questionnaires that prompt students to evaluate their competence, prior experiences, and readiness for specific courses (Ferris et al., 2017; Royer and Gilles, 1998). DSP can also validate the diverse linguistic and educational backgrounds of multilingual learners by encouraging them to reflect on their use of cross-linguistic strategies or home-language literacy skills to navigate academic texts (Johnson and Vander Bie, 2024; Ferris et al., 2017).
However, DSP may also introduce risks, particularly if students misjudge their capabilities, which can lead to potential misplacement (Ferris et al., 2017; Crusan, 2011). Incorporating performance-based reading and writing tasks alongside self-rating surveys may help address this issue for some groups of college students (Aull, 2021). For example, Aull (2021) found that students in 100-and 200-college level courses adjusted their self-assessments after completing a performance-based task, which required them to read an article on fake news and write a 250–300-word summary of its key points. Conversely, students placed in preparatory courses showed no significant changes in their self-ratings. Aull suggests that this may be because these students’ self-perceptions were shaped more by general messages about their abilities than by the task itself.
For multilingual learners, understanding how their perceptions of competence interact with specific task demands in English-dominant contexts is essential for accurately assessing their academic level (Baker et al., 2024). Although Aull (2021) did not specifically examine multilingual learners, the author found that students requiring additional support rated their abilities similarly before and after a writing task. This suggests some students may not need a proficiency test to adequately self-assess their general skill level. However, motivation may have influenced how they engaged with different aspects of the task. For instance, students who anticipated needing a preparatory course may have felt more confident and engaged when identifying key ideas in the article, but less so when summarizing main points or structuring sentences with appropriate syntax and cohesion. Extending this to multilingual learners, analyzing how their perceptions of ability and engagement relate to specific task demands at the item level (e.g., performance on individual reading questions or rubric criteria on an essay) could provide more targeted insights for instructional support as they navigate English-only academic contexts.
Building on this idea, Baker et al. (2024) required multilingual college students to rate their confidence on specific test items within their DSP framework. However, they did not examine whether students’ self-assessments on test items aligned with their actual performance, leaving open the question of how students with different motivation levels perform on item-level tasks. The current work employs Explanatory Item Response Models (EIRMs) to explore this gap in the literature, by linking students’ broader self-assessments of motivation to their actual performance on specific test items (De Boeck and Wilson, 2004; Maris and Bechger, 2009). Unlike aggregate scoring approaches, EIRMs analyze how motivation interacts with different linguistic and cognitive demands within a task, offering a more nuanced understanding of self-assessment accuracy across various task characteristics, and for diverse student groups. This approach could reveal patterns that placement assessments often overlook, providing deeper insight into the role of motivation in multilingual learners’ academic performance.
Current study
This exploratory study examines how DSP frameworks can integrate self-assessment tools with performance-based vocabulary measures in monolingual and multilingual learners who identified English as a non-native language. While DSP traditionally relies on writing performance for placement (Crusan, 2011), vocabulary assessments provide additional insight into academic success, particularly for multilingual learners (Alsahafi, 2023; Loewen and Ellis, 2004; Masrai and Milton, 2021). Vocabulary is foundational for reading comprehension in English (Perfetti and Stafura, 2014), which is critical for higher education (Ari, 2016; Kaldes et al., 2024; Perin, 2020). Some DSP studies have incorporated multiple-choice linguistic assessments that capture vocabulary performance (Baker et al., 2024). However, as an exploratory study, this research presents a model for integrating performance-based measures into DSP rather than prioritizing vocabulary assessments over other reading and writing measures used in prior DSP literature.
Reflective questionnaires are central to DSP, supporting multilingual learners by validating their linguistic and educational backgrounds to recognize them as strengths (Johnson and Vander Bie, 2024; Ferris et al., 2017). To align with DSP and motivational frameworks, this study examines self-reported reading motivation, focusing on competence beliefs and task value (Eccles et al., 1983; Royer and Gilles, 1998). While DSP survey questions vary by institution (Toth and Aull, 2014), this study broadly aligns with DSP by incorporating self-reports on years in college and years speaking English. Years in college serve as a proxy for academic exposure, while years speaking English provides insight into linguistic background, which some DSP frameworks assess through qualitative self-reflection surveys (Johnson and Vander Bie, 2024).
This study extends DSP research by applying Explanatory Item Response Models (EIRMs) to examine how self-report variables interact with specific test items, addressing the need for a more detailed understanding of literacy skills across learner groups (Aull, 2021). Because this is an exploratory study, word frequency serves as an example of a task characteristic that may influence vocabulary performance, given the role of low-frequency and discipline-specific terms in academic literacy (Mancilla-Martinez et al., 2021; Nagy and Townsend, 2012). While higher-frequency words are reinforced through regular exposure, lower-frequency words require deeper mastery and repeated encounters for retention (Schmitt, 2014). EIRMs provide a nuanced analysis of how individual background factors influence vocabulary assessment by assessing shifts in mastery of specific task demands rather than relying solely on aggregate scores. Additionally, we modeled item performance across the sample to identify systematically difficult or easy test items, establishing a baseline for item functioning before analyzing patterns across learner groups (De Boeck et al., 2011). Specifically, we asked the following research questions:
1. What is the relationship of self-reported language status (monolingual or multilingual) and reading motivation with item-level accuracy on an assessment? How does prior educational experience, such as years in college, relate to item-level accuracy?
2. How does self-reported reading motivation interact with word frequency on vocabulary items (lower-frequency versus higher-frequency) in relation to item-level accuracy for monolingual and multilingual learners?
Finally, we conducted a follow-up analysis to examine the diversity in multilingual learners’ linguistic and educational experiences among those with higher and lower levels of reading motivation. Specifically, we analyzed self-reported years speaking English and years spent in college to explore how these variations, combined with differing motivation levels, influenced vocabulary performance.
Method
Participants
Participants included 288 college students from a large university in the southeast region of the United States (M age = 20.31, SD = 4.25). Of the 288 college students, 249 students responded “Yes” to a survey item that asked whether English was their native or home language (M age = 21.20; SD = 4.18). There were 39 students who responded “No” to the survey item, whom we refer to as multilingual learners (M age = 21.05; SD = 4.68). Table 1 provides additional demographic information for the total sample, native, monolingual English speakers, and multilingual learners. Details regarding the languages spoken by the 39 multilingual learners can be found in Supplementary Table S1.
Multilingual learners were asked to report the number of years they had been speaking English. To contextualize this data, an exposure rate was calculated, representing the proportion of each student’s life (in years) spent speaking English. This method aligns with approaches in count data modeling, whereby exposure rates account for differences in exposure periods across individuals (Gardner et al., 1995). For example, a student reporting 10 years of English exposure at age 18 has been exposed to English for most of their life, whereas 10 years of exposure for a 38-year-old represents a smaller proportion of their lifespan.
The average exposure rate among multilingual learners was 0.71 (SD = 0.24, min–max = 0.1–0.97). Thus, on average, participating students spent 71% of their lives speaking English. While this measure provides a standardized way to compare participants, it does not capture variability in daily exposure (e.g., frequency or intensity of use) or the contexts in which English was spoken (e.g., academic, social, or home settings). Rather, it serves as a coarse-grained proxy for relative lifetime exposure.
Measures
Reading vocabulary assessment
The reading vocabulary assessment comprised 20 items selected from the reading vocabulary subtest of the GMRT-4 (Level 6; MacGinitie et al., 2002). The GMRT-4 reading vocabulary assessment evaluates both the depth and breadth of vocabulary knowledge. Specifically, it assesses participants’ comprehension of individual words within specific contexts, indicating depth, while also encompassing a wide variety of vocabulary words across multiple items, offering insight into breadth.
This assessment was designed to evaluate receptive (passive) vocabulary knowledge, meaning it measured the participants’ ability to recognize and understand words in context rather than produce them actively. Participants silently read short phrases or sentences presented on a computer screen, each containing a single underlined word, followed by five answer options. Their task was to select the option that best matched the meaning of the underlined word in context, which included both higher-and lower-frequency words. A higher-frequency word is one that appears more commonly in daily conversation (e.g., “They want to inspire him”; Responses: express concern for, motivate, attempt to persuade, frighten, save; Answer: motivate). In contrast, a lower-frequency word is less commonly used in everyday speech and may be more typical of academic or specialized language (e.g., “The scientist attempted to extrapolate the data”; Responses: confirm, estimate beyond, analyze, refute, compare; Answer: estimate beyond). The reliability of the items for the current study sample was excellent (Cronbach’s alpha coefficient = 0.92).
We determined word frequency score for each of the 20 underlined words using the Zipf scale from the SUBTLEX-US word frequency database (van Heuven et al., 2014). Zipf scale values range from 1 to 7 (1 = lowest frequency, 7 = highest frequency). The Zipf scores for reading vocabulary items ranged from 1.59 to 3.83. Item 4 had no assigned score in the database and was therefore excluded from analyses. From the 19 items, a score of 2.63 represented the median frequency score across all items. Thus, items with a score of 2.63 and above were considered higher frequency and items below 2.63 were considered the lower frequency items1.
Reading motivation
Reading motivation was assessed with two 5-point Likert-scale items, measuring the two critical dimensions of (a) competence beliefs and (b) task value that are central to EVT (Eccles and Wigfield, 2002; Wigfield and Eccles, 2002). The item assessing competence beliefs prompted students to self-assess confidence in their reading ability with the statement “I am confident in my reading ability,” rated on a scale from 1 (not like me at all) to 5 (very much like me). The item measuring task value for reading asked students to rate reading enjoyment with the statement “I enjoy reading,” also on a scale from 1 (not like me at all) to 5 (very much like me).
Analytic strategy
We first explored the overall correlations among the variables and examined the distribution of self-rated reading competence and reading enjoyment. Additionally, we conducted item-level analyses for the reading assessment, including item-total correlations and percent correct scores for each item. We conducted analyses of the distribution of self-rated competence and enjoyment as well as item-level analyses, for both the entire sample and separately for students categorized as monolingual English speakers and multilingual learners.
Explanatory item response models
To address our primary research questions, we conducted Explanatory Item Response Modeling (EIRM) using the lme4 package in R Studio (Bates et al., 2015). In line with the assumptions of the Item Response Theory (IRT) framework, where the intercept typically represents item easiness, we opted not to include an intercept in our models (De Boeck et al., 2011). By excluding the intercept, we aimed to directly estimate item parameters and simplify the interpretation of model coefficients.
Three models were estimated to explore person-and item-level effects on students’ probability of correctly answering reading vocabulary items (coded as 0 = incorrect, 1 = correct). The first model (i.e., Model 1) estimated person-level factors at level two, including year in college (γ01), monolingual English speaker status with multilingual learner status as the reference group (γ02), high reading competence beliefs (γ03), and high reading enjoyment (γ04) with lower reading competence and enjoyment as the reference (i.e., score of 3 and below). Additionally, this model included item-level parameters at level 1 (γ10). The specification for Model 1 is shown below:
In the subsequent two models, we investigated combined person-by-item effects alongside the main effects, which encompassed either reading competence or enjoyment, monolingual English speaker status, and lower-frequency words. Due to the inherent limitations in estimating both item parameters and traditional interaction effects across item and person level effects within a single model (De Boeck et al., 2011), we employed subset differential item functioning (DIF) modeling. Subset DIF requires the creation of new covariates representing the combined effect between dichotomous item and person-level variables (see De Boeck et al., 2011).
In this study, item frequency and motivation were measured on continuous and ordinal scales, respectively. Therefore, we created dichotomous categories for word frequency, reading competence, and reading enjoyment. Items with a word frequency below the median of 2.63 were categorized as lower frequency. As in Model 1, reading competence and enjoyment were classified into high (ratings of 4 and 5) versus relatively lower scores (3 and below). The equation for Model 2 below illustrates the three new dummy-coded covariates with person-by-item effects derived from reading competence, multilingual learner status, with lower frequency words (γ11 – γ13)2. A similar third model (Model 3) included person-by-item effects with reading enjoyment and multilingual learner status with lower frequency words.
Despite the inherent limitations of dichotomizing continuous data and data with multiple levels, we chose this method to gain deeper insights into the combined effects of multilingual learner status, motivation, and word frequency, over and above item-level parameters. Moreover, dichotomization of self-reported reading competence and enjoyment addressed the heavily skewed distribution of self-reported ratings, predominantly ranging from 3 to 5, with only a few students rating themselves a 1 or 2 (as explained later in the preliminary findings).
Analysis of model fit
We used multiple indices to evaluate the overall goodness of fit, including deviance, Akaike Information Criterion (AIC), Bayesian Information Criterion (BIC), and log-likelihood difference tests. Additionally, we reported the sample-adjusted BIC (SABIC) to address the penalty BIC imposes on smaller sample sizes (Sclove, 1987). Lower AIC and BIC values indicate better model fit, with the selected model being the one with the lowest criterion value. Significant log-likelihood difference tests suggest that the more complex model explains more variance in the outcome than the simpler model. Variables that did not significantly contribute to better model fit, as evidenced by minimal improvements in the model fit indices, were excluded from the final model to ensure parsimony and interpretability.
If both person-by-item effects from Models 2 and 3, involving reading competence and reading enjoyment, contributed to better model fit separately over the main effect model (Model 1), we planned to fit a final model, Model 4. This model would include both sets of person-by-item-effects estimated in Models 2 and 3. The final model would account for the combined effects of both dimensions of motivation (enjoyment, competence) with multilingual learner status and item frequency, thereby controlling for one another.
Follow-up analysis of English exposure rate and years in college
If the person-by-item effects showed significant results indicating a meaningful relationship between different levels of motivation on multilingual learner status and word frequency, we conducted a planned follow-up analysis. This analysis aimed to further explore whether English exposure among multilingual learners varied between groups characterized by differing levels of reading competence and enjoyment. We examined descriptive statistics, including the mean, standard deviation, and distribution of English exposure rates among multilingual learners across high and lower reading competence beliefs and enjoyment. To assess potential differences in mean English exposure across these groups, we used a Wilcoxon rank-sum test, a non-parametric test selected due to the relatively small sample size of multilingual learners (N = 39), which were divided into lower and high motivation groups.
Next, we examined whether the number of students with only 1 year of college (Freshmen) differed among multilingual learners with varying levels of reading competence and enjoyment. Given the small sample size of multilingual learners and the limited number of students in years 2–4 of college (see Table 1), we compared the number of Freshmen to a combined group of students with more than 1 year of college. This analysis utilized Fisher’s exact test, comparing frequencies in a two-by-two table of multilingual learners with high and lower motivation, categorized by whether they were Freshmen or had more college experience.
Results
Preliminary analyses
Table 2 displays correlations among years in college, monolingual English speaker status, high reading competence rating, high reading enjoyment rating, total scores on lower-and higher-frequency words in the reading vocabulary assessment, and overall scores on the assessment (See Supplementary Table S2 for separate correlation coefficients by monolingual English speakers and multilingual learners). The magnitudes of the correlations between the variables were small to moderate (rs ranging from 0.12 to 0.24), with significant associations observed between year in college and both lower-frequency (r = 0.13, p < 0.05) and higher-frequency word scores (r = 0.14, p < 0.05). High reading enjoyment was negatively correlated with monolingual English speaker status (r = −0.12, p < 0.05), suggesting that multilingual learners reported higher reading enjoyment compared to their monolingual peers. Additionally, high reading enjoyment was positively correlated with high reading competence (r = 0.24, p < 0.001), while high reading competence beliefs was positively correlated with high-frequency word scores (r = 0.12, p < 0.05). The strongest relationships were found between total reading vocabulary scores and both lower-frequency (r = 0.86, p < 0.001) and higher-frequency word scores (r = 0.95, p < 0.001).
Table 3 displays the distribution of self-rated reading competence and enjoyment. A significant proportion of participants in the total sample reported high levels of competence and enjoyment, with 37 and 43% assigning themselves ratings of 4 or 5, respectively. Additionally, many students reported higher levels of reading enjoyment, with 27 and 38% of students rating themselves as either a 4 or 5, respectively. Ratings below 3 were rare, with only 2 and 0.40% of students rating their reading competence as a 2 or 1, respectively. Likewise, 13 and 5% of students rated themselves as 2 or 1 on reading enjoyment. Monolingual English speakers and multilingual learners also mostly reported high ratings of reading competence and enjoyment (4 or 5) as opposed to rating themselves lower.
Table 4 includes item-total correlations and percent correct responses for each item in the vocabulary assessment, categorized by word frequency and by monolingual English and multilingual learner groups. Many item-total correlations exceeded 0.20, suggesting a moderate to strong relation between the items and overall test score. While students tended to score low on most items, they appeared to exhibit slightly stronger performance on the higher (M = 0.31) compared to lower frequency items (M = 0.25).
Approximately 6% of the reading vocabulary items, totaling 352 out of 5,472 observations at the item level, contained missing data. According to Table 4, items later in the assessment sequence exhibited more frequent missing values, suggesting incomplete responses may have been due to time constraints. To assess whether these missing data met the assumption of missing at random (MAR), which allows missingness to depend on observed variables, we examined the relationship between item order and missingness using a mixed-effects model (See Supplementary Tables S3, S4 for model fit information and parameter estimates, respectively). The results indicated that item order was significantly associated with missing responses (Odds Ratio = 1.42, 95% CI = 1.35–1.49, p < 0.001), meaning missingness was predictable based on an observed factor (item order), rather than unobserved participant characteristics. Consequently, we addressed missing data using multiple imputation with the mice package in R Studio (van Buuren and Groothuis-Oudshoorn, 2011).
Relations of linguistic and educational background, motivation, and word frequency with reading vocabulary performance
To ensure that parameter estimates were interpretable, we first examined model fit indices before proceeding with interpretation. Table 5 contains model fit indices for all models. Model 2 yielded the lowest AIC value, suggesting superior model performance with the added person-by-item effects of reading competence beliefs and multilingual learner status with lower frequency items (Vrieze, 2012). Moreover, Model 2 explained significant variance in response accuracy over and above Model 1, as evidenced by the significant log-likelihood ratio statistic (p = 0.005). Finally, the SABIC for Model 2 (4449.05) decreased compared to Model 1 (4451.13), although this decrease was marginal, suggesting that this model offered the most parsimonious fit to the data.
Taken together, these indices suggest that Model 2 offered the best balance of both explanatory accuracy and model simplicity compared to the null model. Thus, we reported and interpreted person-by item effects across reading competence, multilingual learner status, and lower frequency items. Model 3 did not show evidence of improvement in model fit, thus person-by-item effects between reading enjoyment, multilingual learner status, and lower-frequency items were not reported or interpreted. Additionally, a fourth model was not estimated, which would have contained both the person-by-item effects from Model 2 and Model 3.
Model results
The parameter estimates from Model 2 are shown in Table 6. A significant number of the item-level parameter estimates were negative (ps < 0.001), indicating that students were generally less likely to answer items correctly. Years in college (γ01) was significantly related to item-level performance (OR = 1.24, 95% CI = 1.02–1.52, p = 0.038), suggesting that students with more years in college had a higher probability of answering items correctly. However, reading competence beliefs (γ04) did not significantly relate to item-level performance beyond students’ years in college (OR = 1.75, 95% CI = 0.99–3.10, p = 0.08). Similarly, neither monolingual English speaker status (γ02) nor reading enjoyment (γ03) were significantly related to students’ probability of correctly answering the items (ps > 0.05).
Figure 1 illustrates the nature of the combined effect between multilingual status, reading competence, and word frequency. Compared to monolingual English speakers with higher reading competence, multilingual learner students with lower competence exhibited significantly lower probability of success on lower frequency words compared to higher frequency words (OR = 0.17, 95% CI = 0.06–0.54, p = 0.003). This effect was not observed for monolingual English speakers with lower reading competence or multilingual learners with high reported reading competence.
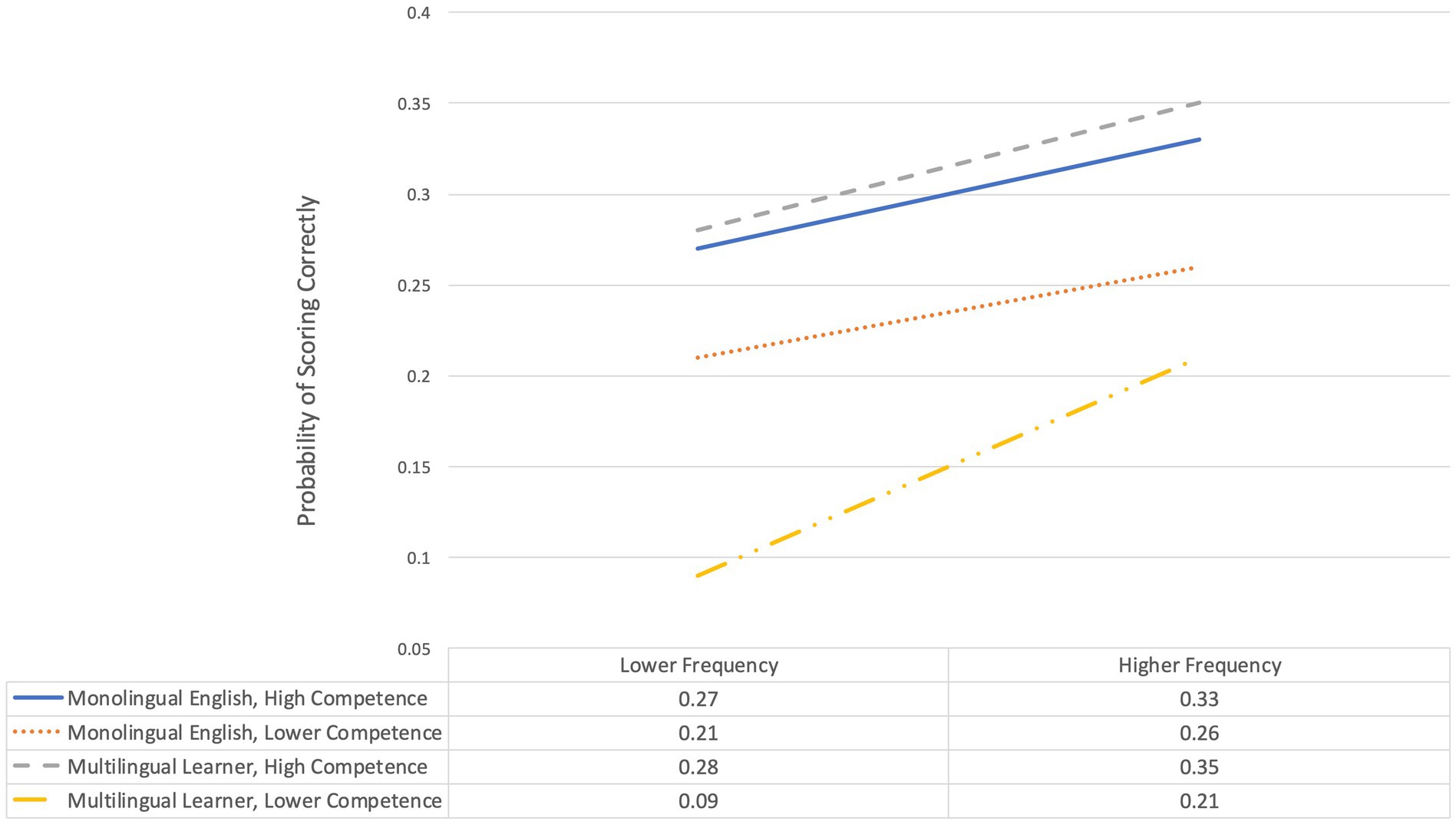
Figure 1. Item-level probabilities by word frequency, English exposure, and reading competence beliefs.
Differences in multilingual learner’s English exposure and years in college by reading competence
This follow-up analysis examined potential differences in the duration of English exposure and number of years in college between two groups of multilingual learners, categorized by higher and lower reading competence (M[SD] age = 21.7[5.29] and 19.4[1.80], respectively; see Supplementary Table S5 for demographic details). Both groups exhibited similar rates of English exposure, with multilingual learners who reported higher reading competence having an average exposure rate of 0.71 (SD = 0.25; Min - Max = 0.1–0.97), and those with lower competence showing an identical average exposure rate of 0.71 (SD = 0.22; Min - Max = 0.21–0.95). A subsequent Wilcoxon rank-sum analysis confirmed that there were no significant differences in English exposure rates between these groups (p = 0.764; see Supplementary Table S6 for the complete Wilcoxon rank-sum results). Figure 2 illustrates this with a cloud plot, showing that despite the non-normal distribution of English exposure across the two groups, both groups display similar distribution trends with a negative skew.
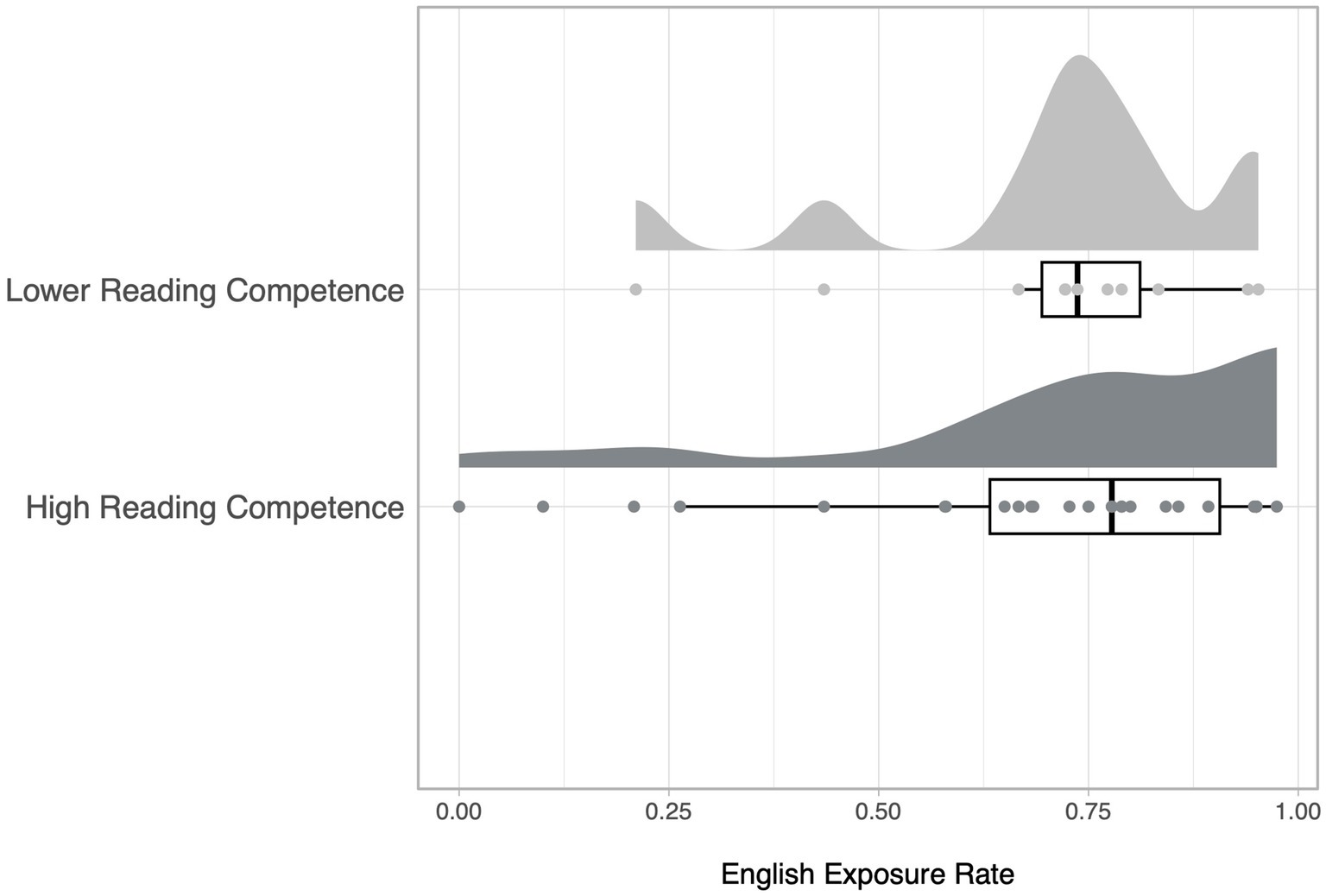
Figure 2. Cloud plot of multilingual learners’ English exposure rates by high and lower reading competence beliefs.
Next, we used Fisher’s Exact Test to examine the association between group membership (lower motivation vs. higher motivation) and year in college (year 1 vs. years 2–4) among multilingual learners. The test yielded a p-value of 0.301, indicating no statistically significant association between group membership and having more years in college. However, there was an observed trend with fewer Freshman students in the higher motivation group (12 out of 28, 43%) compared to their less motivated peers (7 out of 11, 64%).
Discussion
This exploratory study utilized novel item-level analyses to inform Directed Self-Placement (DSP) frameworks by examining how educational and linguistic background, self-reported motivation, and reading vocabulary performance on lower-and higher-frequency items interact, with a specific focus on multilingual learners. Across the entire sample, years in college significantly related to performance on item-level performance. Word frequency emerged as a critical factor influencing performance, with lower-frequency items posing greater challenges across all participants but disproportionately affecting some multilingual learners. However, the degree to which people endorsed more motivated dispositions appeared to moderate the different patterns across the language groups. Specifically, although multilingual learners with lower self-reported reading self-efficacy performed significantly worse on lower-frequency vocabulary items compared to both their monolingual peers, multilingual learners with higher self-efficacy performed similarly to their monolingual peers. These findings suggest that reading motivation factors may influence DSP assessments, particularly in how multilingual college students’ approach and engage with academic tasks in U.S. higher education settings. Multilingual students with higher motivation may have performed better by employing compensatory mechanisms, such as translanguaging strategies (e.g., Gu et al., 2024).
As an exploratory study using an extant dataset to inform DSP rather than a fully implemented DSP process, several limitations should be considered. First, motivation was assessed using only two broad questions—one on competence beliefs (“I am confident with my reading”) and one on task value (“I enjoy reading”)—which did not capture task-specific motivation after reading and writing. Future DSP assessments could further develop these items by including multiple, more refined items that assess a broader range of motivational factors across different language and literacy contexts. Second, vocabulary assessments are not typically included in DSP, which primarily emphasizes writing (Crusan, 2011). Instead, this study loosely aligns with Baker et al. (2024), which incorporated a multiple-choice linguistic task in DSP with multilingual learners, though their assessment included syntax as well as vocabulary. This highlights the variability in DSP tasks and the need to explore which assessment types are most effective in assessing multilingual learners’ success in college. Third, this study did not examine how multilingual learners use their home languages to support vocabulary performance, despite translanguaging being a key academic strategy that enhances motivation (Gu et al., 2024; García and Wei, 2014). The lack of differences in self-reported years speaking English between motivation groups suggests that English exposure alone may be an insufficient measure of language experience, reinforcing the need for self-assessment tools that capture strategic home language use and the interplay between home language and English across various contexts. Fourth, as an initial investigation of item-level assessments to inform DSP, the small number of multilingual learners in the sample (N = 39) limits generalizability across linguistic backgrounds. Reading development in English may vary based on students’ first languages and literacy histories (Jiang, 2016), emphasizing the need for DSP adaptations at institutions serving specific multilingual populations (e.g., Hispanic-Serving Institutions).
Finally, the data in this study were not collected directly from a DSP process but from college students already enrolled in a four-year institution. As a result, the study primarily emphasizes data-driven methods that could inform DSP but require further implementation in practice. While these limitations reflect the study’s exploratory nature and the challenges of applying a new methodology to DSP, its use of EIRMs and expectancy-value theory (EVT) provides a promising direction for refining placement frameworks. The next section explores how EVT and translanguaging theory can guide DSP assessment design, as well as how EIRMs can improve placement decisions for multilingual learners.
Developing a more comprehensive DSP assessment
The results of the EIRM analysis and study limitations highlight key areas for improving DSP assessment. While DSP research has emphasized motivation (e.g., Aull, 2021) and the importance of recognizing linguistic diversity in placement (e.g., Ferris et al., 2017), motivation measures in DSP tend to be broad, assessing general feelings and attitudes rather than task-specific literacy engagement (Toth and Aull, 2014). Additionally, prior studies on DSP frameworks with multilingual learners have not systematically drawn on theoretical models to inform self-report tools and interview-based assessments (e.g., Ferris et al., 2017; Johnson and Vander Bie, 2024; Baker et al., 2024), such as translanguaging theory (García and Wei, 2014). Incorporating theoretical models that explicitly highlight multilingual students’ cognitive and linguistic assets could enhance DSP tools by providing a more comprehensive understanding of their academic and language abilities. This section explores three key areas for improving DSP assessment frameworks by drawing on theory and preliminary findings from the current study: refining motivation measures through Situated Expectancy-Value Theory (SEVT), incorporating translanguaging strategies into DSP assessment, and using EIRMs to provide deeper, data-driven placement insights.
Refining motivation measures
Existing DSP models often cite Bandura’s self-efficacy theory, which focuses on competence beliefs, or students’ self-efficacy in their ability to complete a task successfully (Bandura, 1989). While self-efficacy is a critical factor in motivation, it does not account for task value, or the extent to which students perceive a task as meaningful, useful, or engaging. Task value is particularly important in literacy assessments because students are more likely to engage deeply with reading and writing tasks when they see them as relevant to their academic goals (Eccles and Wigfield, 2023, 2024). However, DSP assessments do not typically include task value explicitly, limiting the ability to capture how all aspects of motivation vary across literacy settings.
The motivation measures in the current study aligned more closely with the original Expectancy-Value Theory (EVT) framework, as they broadly assessed competence beliefs and task value without situating them in specific literacy contexts (Eccles et al., 1983). However, motivation should be assessed in specific scenarios that allow students to reflect on different literacy experiences across languages, as well as immediately after completing a literacy task in English. More recent developments in EVT, specifically Situated Expectancy-Value Theory (SEVT), emphasize that motivation is shaped by more specific academic and social contexts (Eccles and Wigfield, 2023, 2024). This means that competence beliefs and task value fluctuate depending on the literacy setting and task demands. For multilingual learners, motivation may differ based on whether they are reading and writing in English or their home language across different literacy contexts in day-to-day life. Incorporating these variations into DSP assessments would allow for a more accurate understanding of how multilingual students engage with academic literacy tasks.
To better assess competence beliefs and task value across different literacy settings, DSP assessment frameworks could consider including measures that capture motivation in both broader language and literacy contexts and task-specific situations. Students could reflect on their competence beliefs across different language and literacy scenarios by responding to statements such as, “I am confident understanding novels in English” or “I am confident reading academic texts in my home language.” Similarly, task value could be assessed with items like, “I enjoy reading newspapers in English” or “I enjoy reading newspapers in my home language.” These measures capture students’ general engagement with literacy across languages but do not fully account for how motivation fluctuates in response to specific academic tasks.
To align motivation measures with specific task demands, students could self-rate their competence beliefs and task value on a Likert scale after completing a performance-based measure during the DSP process. For example, after summarizing a reading, students could evaluate their confidence in the task by responding to statements such as, “How confident were you in identifying the main idea of the article?” or “How confident were you in summarizing the article in your own words?.” Task value could also be further refined by asking students not only whether they found the task engaging but also whether they believed that certain aspects of the task were a necessary part of the overall task goal, as indicated by statements such as, “Understanding the main points of the article was an important part of successfully completing the summary.”
In the current study, the lack of significant findings for task value in relation to vocabulary performance may reflect the broad nature of the motivation questions, which did not distinguish between different aspects of task value or account for how motivation shifts in response to specific tasks. By refining how motivation is measured across different literacy experiences and immediately after performance-based tasks, DSP assessments may better capture the relationship between different aspects of motivation and literacy assessment performance.
Integrating translanguaging strategy questions
Multilingual students employ their full linguistic repertoires in academic settings, using their home languages to aid comprehension and meaning making. Rather than viewing languages as separate systems, multilingual learners blend their linguistic resources to engage with academic tasks. For example, Canagarajah (2011) argues that multilingual students fluidly integrate their languages in academic writing, challenging traditional monolingual standards that dominate assessment practices. Similarly, García and Wei (2014) emphasize that translanguaging is not simply code-switching but an active process of constructing meaning across languages. However, DSP frameworks have not systematically incorporated these perspectives into self-assessments. While some placement models acknowledge multilingual backgrounds, self-assessments primarily focus on English proficiency and self-perceived academic skills rather than the cognitive and linguistic strategies that students use across languages (e.g., Baker et al., 2024; Ferris et al., 2017; Horton, 2022; Johnson and Vander Bie, 2024). Expanding DSP assessments to include translanguaging strategies could provide a more accurate and equitable understanding of multilingual students’ literacy engagement.
Recent studies have developed self-assessment questionnaires that measure translanguaging strategies in academic literacy. For example, Öztürk and Çubukçu (2022) used a questionnaire to examine how college students incorporate translanguaging into reading tasks, finding that students frequently used their home languages for comprehension, paraphrasing, and critical thinking. Similarly, Ali (2024) employed survey-based measures with college students to analyze how translanguaging supports academic literacy, showing that students used it to improve reading, writing, and note-taking. Champlin (2016) also used self-assessments to explore translanguaging in bilingual instruction, emphasizing the need for structured support and training to maximize its benefits. A common finding across these studies is that multilingual students consistently use translanguaging as an academic resource.
To capture translanguaging practices effectively, DSP assessments could incorporate two distinct but complementary approaches. The first focuses on students’ broader use of translanguaging in daily literacy activities, asking them to reflect on how they engage with reading and writing across languages in both academic and non-academic contexts. For example, students could respond to statements such as “When you read for school, do you mentally translate difficult words into your home language?” or “How often do you switch between English and your home language when reading news articles, texting, or writing notes?” These questions provide insight into how students integrate multiple languages as part of their regular literacy habits, not just in formal academic settings.
The second approach situates translanguaging within specific DSP tasks, allowing students to reflect on their language use immediately after completing a reading or writing assignment. After summarizing an article, students could indicate whether they relied on their home language for comprehension by answering questions such as, “Did you mentally translate any parts of the article into your home language while reading?” or “While writing your summary, did you think in your home language before translating your ideas into English?” By assessing translanguaging both broadly and in direct relation to performance-based measures, DSP frameworks can gain a more comprehensive understanding of how multilingual students navigate academic literacy in English while drawing on their full linguistic resources.
Integrating explanatory item response models into DSP research
Traditional DSP frameworks incorporate a range of assessment methods, including broad self-assessments, holistic writing samples, and institution-specific placement models (e.g., Baker et al., 2024; Ferris et al., 2017). While some DSP surveys include post-task self-assessments (e.g., Ferris et al., 2017), others rely on more general measures of self-confidence that do not capture how motivation interacts with specific literacy tasks (Toth and Aull, 2014). Aull (2021) has emphasized the need for more refined and task-specific DSP assessments, particularly those that account for the nuanced ways multilingual students engage with academic literacy.
EIRMs provide a more detailed understanding of how motivation, linguistic background, and literacy performance interact at the item level (Wilson et al., 2008). Traditional assessments often produce broad placement decisions, but EIRMs allow for a more precise analysis of how different learner profiles engage with specific dimensions of a literacy task. For example, Magliano et al. (2007) emphasized that multiple-choice reading assessments require various skills, including both text matching and inferencing. Similarly, rather than treating reading proficiency as a single construct, EIRMs distinguish between these subskills to identify how learner characteristics, such as self-efficacy levels or translanguaging tendencies, relate to specific aspects of reading comprehension and task performance.
Findings from the current study highlight the value of this approach. The EIRM analysis suggested that multilingual students with lower reading self-efficacy performed significantly worse on lower-frequency vocabulary items than both their monolingual peers and multilingual students. However, our findings also suggested that some multilingual students may perform just as well as their monolingual English speaking peers if they endorse high reading competence beliefs. While Aull (2021) and Ferris et al. (2017) took a step toward more accurate self-assessment by having students rate their competence immediately after a literacy task, our results suggest that incorporating data-driven methods such as EIRM analyses can provide a more precise understanding of how learner characteristics relate to different components of a literacy task. For example, a student may report feeling less confident in their reading ability on a vocabulary assessment, which aligns with their overall score, but may not recognize that their difficulties are specifically in decoding lower-frequency vocabulary. Similarly, another student may rate themselves as having high competence on a reading comprehension task but could still require support in areas such as inferencing. Rather than relying solely on self-reported general skill levels, these methods help anticipate how different students engage with specific literacy demands, allowing DSP frameworks to more effectively identify and support multilingual learners in targeted areas.
Expanding EIRMs beyond vocabulary to writing placement could further enhance DSP frameworks. Traditional writing assessments often rely on binary placement decisions, such as assigning students to remedial or standard courses based on a single aggregate cut-off score, but EIRMs can evaluate performance across multiple dimensions, including organization, coherence, and grammar. Uto (2021) applied a multidimensional Item Response Theory (IRT) approach to rubric-based performance assessments and demonstrated that writing ability consists of distinct yet interrelated competencies rather than a single unified skill. While IRT provides a more detailed understanding of how students perform across these dimensions, EIRM analysis builds on this by examining how learner characteristics, such as multilingual background or motivation levels, influence performance on specific aspects of writing. Incorporating EIRMs into DSP would not only allow for a more precise assessment of writing proficiency but also help identify how multilingual learners and students with varying levels of motivation engage with different writing demands.
Applications in research and practice
While EIRMs offer a more precise understanding of how motivation, linguistic background, and literacy performance interact at the item level, current DSP models lack a standardized, validated self-assessment tool and instead rely on a mix of broad self-efficacy measures, holistic writing samples, and institution-specific placement methods (Toth and Aull, 2014). This variability makes it difficult for DSP frameworks to consistently capture task-specific motivation and translanguaging strategies across institutions, which may lead to inconsistencies in placement accuracy and student support. Before EIRM-based analyses can be effectively integrated into DSP, research must first establish a validated self-assessment scale that reliably measures motivation and translanguaging across diverse literacy contexts and institutional settings.
Future research could focus on establishing the validity and reliability of such a scale by employing confirmatory factor analysis (CFA) to determine whether different facets of motivation and translanguaging function as distinct but related dimensions. For example, Belova and Kharkhurin (2024) validated the Plurilingual and Pluricultural Competence (PPC) scale by conducting psychometric analyses to assess its factor structure, reliability, and applicability across different linguistic populations. Similarly, a DSP-focused self-assessment scale could be developed and tested across multilingual student samples to ensure its construct validity and cross-institutional relevance. This process would involve examining whether different facets of motivation and translanguaging operate as independent yet interrelated constructs, ensuring that the scale accurately reflects students’ engagement with academic literacy across languages and institutions.
With a validated self-assessment scale in place, research on Explanatory Item Response Models (EIRMs) can offer valuable insights into how motivation and translanguaging interact with literacy performance, ultimately refining DSP placement recommendations. Research on Informed Self-Placement (ISP) emphasizes the importance of providing students with feedback on their self-assessments to improve placement accuracy and support academic success (Morton, 2022). When students receive structured feedback during the placement process, they can more accurately evaluate their strengths and areas for improvement, leading to better-informed course decisions. Similarly, EIRM research enhances this process by identifying trends in student responses, allowing universities to anticipate areas where students may need additional support. Instead of solely assigning students to a course level, EIRM-informed DSP frameworks could generate individualized feedback reports, offering insights into specific literacy skills, motivation levels, and translanguaging strategies that shape academic performance.
Finally, long-term tracking can refine placement accuracy by identifying which self-assessment measures are most relevant to students’ academic growth. Gere (2019) emphasized that students’ writing confidence and self-perceptions evolve across disciplines, while Mayo et al. (2023) found that factors beyond placement accuracy, such as parental education, also shape long-term outcomes in English composition courses. Extending this to multilingual learners, combining EIRM item-level analyses with longitudinal DSP data could help determine which aspects of motivation and translanguaging align most closely with long-term writing success. If EIRM research highlights that students who report using their home language for comprehension tend to perform better in advanced writing tasks, longitudinal research could refine these self-assessment tools to better understand if these strategies help inform long-term outcomes. Similarly, if task value (i.e., students’ belief that certain aspects of a task are essential to overall learning) is strongly associated with writing performance over time, DSP frameworks could emphasize these specific aspects of motivation to inform student placement.
Conclusion
As DSP continues to evolve, placement frameworks must be grounded in robust theoretical models of motivation and translanguaging, alongside more advanced, data-driven methodologies. While existing studies, such as Aull (2021) and Ferris et al. (2017), have begun to explore task-focused self-reflection in self-assessment, DSP models still largely rely on broad self-efficacy ratings that may not fully capture how students engage with specific literacy tasks (Toth and Aull, 2014). This study provides an exploratory look into how EIRM-based analyses could refine these approaches by offering a more precise method for examining how motivation influences different dimensions of literacy performance at the item level. However, further research is needed to evaluate the role of translanguaging strategies in DSP and refine self-assessments through more targeted motivation and translanguaging measures. Specifically, before EIRM-informed DSP models can be effectively implemented, a self-assessment scale integrating motivation and translanguaging should be developed, validated, and standardized to ensure consistent measurement across institutional contexts. Additionally, longitudinal research is needed to determine whether different aspects of motivation, translanguaging, and item-level literacy performance align with long-term academic outcomes, further refining how DSP frameworks support multilingual learners.
Data availability statement
The raw data supporting the conclusions of this article will be made available by the authors, without undue reservation.
Ethics statement
The studies involving humans were approved by Institutional Review Board (IRB) of the University of Memphis (IRB #2491). The studies were conducted in accordance with the local legislation and institutional requirements. The participants provided their written informed consent to participate in this study.
Author contributions
GK: Conceptualization, Formal analysis, Writing – original draft, Writing – review & editing. JB: Resources, Data curation, Writing – review & editing. EK: Data curation, Writing – review & editing.
Funding
The author(s) declare that financial support was received for the research and/or publication of this article. The research reported here was supported by the Institute of Education Sciences, U.S. Department of Education, through Training Grant #R305B200007, awarded to Georgia State University.
Conflict of interest
The authors declare that the research was conducted in the absence of any commercial or financial relationships that could be construed as a potential conflict of interest.
Generative AI statement
The authors declare that no Generative AI was used in the creation of this manuscript.
Publisher’s note
All claims expressed in this article are solely those of the authors and do not necessarily represent those of their affiliated organizations, or those of the publisher, the editors and the reviewers. Any product that may be evaluated in this article, or claim that may be made by its manufacturer, is not guaranteed or endorsed by the publisher.
Author disclaimer
The opinions expressed are those of the authors and do not represent views of the Institute or the U.S. Department of Education.
Supplementary material
The Supplementary material for this article can be found online at: https://www.frontiersin.org/articles/10.3389/feduc.2025.1521482/full#supplementary-material
Footnotes
1. ^In standardized reading assessments like the Gates-MacGinitie Reading Tests (GMRT), the vocabulary words selected are often of lower frequency compared to everyday language usage. This is intentional, as the aim is to challenge the test-takers’ vocabulary knowledge and ensure a comprehensive assessment of their reading abilities. The use of lower frequency words helps to differentiate between varying levels of reading proficiency among students. According to the GMRT technical report, “the selection of test words in the Word Knowledge and Vocabulary tests involves using typical classroom materials and the expertise of skilled educators to ensure the words are relevant yet challenging for the intended grade levels” (Houghton Mifflin Harcourt, 2017). This approach ensures that the vocabulary items are appropriate for educational assessment while being more challenging than commonly encountered words in everyday reading.
2. ^Monolingual English speakers and High Reading competence by Lower Word Frequency served as the comparison group.
References
Aguirre-Muñoz, Z., Pando, M., and Liu, C. (2024). Enhancing bilingual/ESL teachers’ STEM instruction with targeted content and disciplinary literacy professional development: A study on knowledge and practice outcomes. Educ. Sci. 14:745. doi: 10.3390/educsci14010050
Ali, A. D. (2024). Translanguaging in multilingual university classrooms: Effects on students’ language skills and perceptions. Biling. Res. J. 47, 186–210. doi: 10.1080/15235882.2024.2336942
Alsahafi, M. (2023). The Relationship Between Depth of Academic English Vocabulary Knowledge and Academic Success of Second Language University Students. SAGE Open 13. doi: 10.1177/21582440231153342
Alshammari, H. (2018). The effect of educational background on second language reading. Doctoral dissertation. Available online at: https://digitalcommons.memphis.edu/etd/1951 (Accessed January 25, 2025).
Ari, O. (2016). Word recognition processes in college-age students' reading comprehension achievement. Commun. College J. Res. Pract. 40, 718–723. doi: 10.1080/10668926.2015.1098578
Aull, L. L. (2021). Integrating constructed response tasks into directed self-placement: Exploring impacts on students' self-assessment of proficiency and autonomy. Assess. Writ. 47:100511. doi: 10.1016/j.asw.2021.100511
Badham, L., and Furlong, A. (2023). Summative assessments in a multilingual context: What comparative judgment reveals about comparability across different languages in literature. Int. J. Test. 23, 111–134. doi: 10.1080/15305058.2022.2149536
Baker, B., Arias, A., Bibeau, L.-D., Qin, Y., Norenberg, M., and St-John, J. (2024). Rethinking student placement to enhance efficiency and student agency. Lang. Test. 41, 181–191. doi: 10.1177/02655322231179128
Bandura, A. (1989). Regulation of cognitive processes through perceived self-efficacy. Dev. Psychol. 25, 729–735. doi: 10.1037/0012-1649.25.5.729
Bates, D., Maechler, M., Bolker, B., and Walker, S. (2015). Fitting Linear Mixed-Effects Models Using lme4. J. Stat. Softw. 67, 1–48. doi: 10.18637/jss.v067.i01
Belova, S. S., and Kharkhurin, A. V. (2024). Analysing the factor structure of the plurilingual and pluricultural competence scale: Dimensionality, reliability, and validity of the adapted Russian version. Int. J. Multilingual. 1–24. doi: 10.1080/14790718.2024.2326001
Biondi, M., Lesce, L., and Finn, H. B. (2024). Supporting the Academic Literacy Development of English Learners: Bridging High School and College. NECTFL Review.
Blackwell, L. S., Trzesniewski, K. H., and Dweck, C. S. (2007). Implicit theories of intelligence predict achievement across an adolescent transition: A longitudinal study and an intervention. Child Dev. 78, 246–263. doi: 10.1111/j.1467-8624.2007.00995.x
Boekaerts, M. (1991). Subjective competence, appraisals and self-assessment. Learn. Instr. 1, 1–17. doi: 10.1016/0959-4752(91)90016-2
Boekaerts, M. (1992). The adaptable learning process: Initiating and maintaining behavioural change. Appl. Psychol. 41, 377–397. doi: 10.1111/j.1464-0597.1992.tb00713.x
Britt, M. A., Rouet, J.-F., and Durik, A. (2017). Literacy Beyond Text Comprehension: A Theory of Purposeful Reading. 1st Edn. New York: Routledge.
Burton, R. S. (2014). Readability, Logodiversity, and the Effectiveness of College Science Textbooks. Bioscene 40, 3–10.
Calafato, R. (2022). Fidelity to participants when researching multilingual language teachers: A systematic review. Rev. Educ. 10:e3344. doi: 10.1002/rev3.3344
Calafato, R., and Simmonds, K. (2023). The impact of multilingualism and learning patterns on student achievement in English and other subjects in higher education. Camb. J. Educ. 53, 705–724. doi: 10.1080/0305764X.2023.2206805
Canagarajah, S. (2011). Translanguaging in the classroom: Emerging issues for research and pedagogy. Appl. Linguist. Rev. 2, 1–28. doi: 10.1515/9783110239331.1
Chalhoub-Deville, M. B. (2019). Multilingual testing constructs: Theoretical foundations. Lang. Assess. Q. 16, 472–480. doi: 10.1080/15434303.2019.1671391
Champlin, M. J. (2016). Translanguaging and bilingual learners: A study of how translanguaging promotes literacy skills in bilingual students (Master’s thesis, St. John Fisher University). Fisher Digital Publications. Available online at: https://fisherpub.sjf.edu/education_ETD_masters/323 (Accessed January 13, 2025).
Crusan, D. (2011). The promise of directed self-placement for second language writers. TESOL Q. 46, 119–134. doi: 10.5054/tq.2012.174239
De Boeck, P., Bakker, M., Zwitser, R., Nivard, M., Hofman, A., Tuerlinckx, F., et al. (2011). The Estimation of Item Response Models with the lmer Function from the lme4 Package in R. J. Stat. Softw. 39, 1–28. doi: 10.18637/jss.v039.i12
De Boeck, P., and Wilson, M. (2004). Explanatory item response models: A generalized linear and nonlinear approach. New York, NY: Springer.
de Kleine, C., and Lawton, R. (2015). Meeting the needs of linguistically diverse students at the college level (White paper). Retrieved from College Reading & Learning Association website. Available online at: http://www.crla.net/index.php/publications/crla-whitepapers (Accessed January 7, 2025).
Eccles, J. S., Adler, T. F., Futterman, R., Goff, S. B., Kaczala, C. M., Meece, J. L., et al. (1983). “Expectancies, values, and academic behaviors” in Achievement and achievement motivation. ed. J. T. Spence (San Francisco, CA: W. H. Freeman), 75–146.
Eccles, J. S., and Wigfield, A. (2002). Motivational beliefs, values, and goals. Annu. Rev. Psychol. 53, 109–132. doi: 10.1146/annurev.psych.53.100901.135153
Eccles, J. S., and Wigfield, A. (2023). Expectancy-value theory to situated expectancy-value theory: Reflections on the legacy of 40+ years of working together. Motiv. Sci. 9, 1–12. doi: 10.1037/mot0000275
Eccles, J. S., and Wigfield, A. (2024). The development, testing, and refinement of Eccles, Wigfield, and colleagues’ situated expectancy-value model of achievement performance and choice. Educ. Psychol. Rev. 36:51. doi: 10.1007/s10648-024-09888-9
Efklides, A., and Schwartz, B. L. (2024). Revisiting the metacognitive and affective model of self-regulated learning: Origins, development, and future directions. Educ. Psychol. Rev. 36:61. doi: 10.1007/s10648-024-09896-9
Ferris, D. R., Evans, K., and Kurzer, K. (2017). Placement of multilingual writers: Is there a role for student voices? Assess. Writ. 32, 1–11. doi: 10.1016/j.asw.2016.10.001
Ferris, D., and Lombardi, A. (2020). Collaborative placement of multilingual writers: Implications for fairness, validity, and student success. eScholarship, University of California. Available online at: https://escholarship.org/uc/item/7z6683m6 (Accessed January 13, 2025).
García, O., and Wei, L. (2014). Translanguaging: Language, bilingualism, and education. Palgrave Macmillan. doi: 10.1057/9781137385765
Gardner, W., Mulvey, E. P., and Shaw, E. C. (1995). Regression analyses of counts and rates: Poisson, overdispersed Poisson, and negative binomial models. Psychol. Bull. 118, 392–404. doi: 10.1037/0033-2909.118.3.392
Gere, A. R. (ed.). (2019). Developing writers in higher education: A longitudinal study. Ann Arbor, MI: University of Michigan Press.
Gottlieb, M. (ed.). (2023). “Issues in assessment for multilingual learners” in Assessing multilingual learners: Bridges to empowerment. 3rd ed (Thousand Oaks, CA: Corwin), 45–75.
Grabe, W. (2009). Reading in a second language: Moving from theory to practice. Cambridge, United Kingdom: Cambridge University Press.
Gu, M., Jiang, G. L., and Chiu, M. M. (2024). Translanguaging, motivation, learning, and intercultural citizenship among EMI students: A structural equation modelling analysis. Int. J. Intercult. Relat. 100:101983. doi: 10.1016/j.ijintrel.2024.101983
Hilgers, A. (2019). Placement testing instruments for modality streams in an English language program. Master's Thesis. Moorhead, MN: Minnesota State University Moorhead. Available online at: https://red.mnstate.edu/thesis/218 (Accessed January 13, 2025).
Horton, A. E. (2022). Two sisters and a heuristic for listening to multilingual, international students’ directed self-placement stories. Journal of Writing. Assessment 15. doi: 10.5070/W4JWA.222
Houghton Mifflin Harcourt. (2017). Gates-MacGinitie Reading Tests Technical Report. Available online at: https://www.ode.state.or.us/wma/teachlearn/testing/resources/gmrt_9_40364_techreport_questions_33b_and_34c.pdf (Accessed November 12, 2024).
Ibrahim, E., Sarudin, I., and Muhamad, A. (2016). The relationship between vocabulary size and reading comprehension of ESL learners. Engl. Lang. Teach. 9, 116–123. doi: 10.5539/elt.v9n2p116
Jiang, X. (2016). The role of oral reading fluency in ESL reading comprehension among learners of different first language backgrounds. Reading Matrix 16, 227–242.
Johnson, K., and Vander Bie, S. (2024). Directed self-placement for multilingual, multicultural international students. J. Writ. Assess. 17. doi: 10.5070/W4JWA.1550
Kaldes, G., Higgs, K., Lampi, J., Santuzzi, A., Tonks, S. M., O’Reilly, T., et al. (2024). Testing the model of a proficient academic reader (PAR) in a postsecondary context. Read. Writ. 38, 37–76. doi: 10.1007/s11145-023-10500-9
Kamhi-Stein, L. D. (2003). Reading in two languages: How attitudes toward home language and beliefs about reading affect the behaviors of “underprepared” L2 college readers. TESOL Q. 37, 35–71. doi: 10.2307/3588465
Key, W. Jr. (2024). The effects of placement method on multilingual learner success in higher education. TESOL J. 15:e835. doi: 10.1002/tesj.835
Kieffer, M. J., and Lesaux, N. K. (2012). Direct and indirect roles of morphological awareness in the English reading comprehension of native English, Spanish, Filipino, and Vietnamese speakers. Lang. Learn. 62, 1170–1204. doi: 10.1111/j.1467-9922.2012.00722.x
Liu, Y., Cheong, C. M., Ng, R. H. W., and Tse, S. K. (2024). The role of L1 self-efficacy in L2 reading comprehension: an exploration of L1–L2 cross-linguistic transfer. Int. J. Biling. Educ. Biling. 27, 883–897. doi: 10.1080/13670050.2023.2290478
Llosa, L., and Bunch, G. C. (2011). What's in a test? ESL and English placement tests in California's community colleges and implications for U.S.-educated language minority students. TESOL Q. 45, 1–26. doi: 10.5054/tq.2011.244024
Loewen, S., and Ellis, R. (2004). The relationship between English vocabulary knowledge and the academic success of second language university students. New Zealand Stud. Appl. Linguist. 10, 1–29.
Lopez, A. A., Turkan, S., and Guzman-Orth, D. (2017). Conceptualizing the use of translanguaging in initial content assessments for newly arrived emergent bilingual students (ETS Research Report No. RR-17-07). Educational Testing Service.
MacGinitie, W. H., MacGinitie, R. K., Maria, K., and Dreyer, L. G. (2002). Gates-MacGinities Reading Tests (4th edition): Technical manual, Forms S & T. Riverside, IL: Rolling Meadows.
Magliano, J. P., Millis, K., Ozuru, Y., and McNamara, D. S. (2007). “A multidimensional framework to evaluate reading assessment tools,” in Reading comprehension strategies: Theories, interventions, and technologies. ed. D. S. McNamara (Mahwah, NJ: Lawrence Erlbaum Associates Publishers), 107–136.
Magliano, J. P., Talwar, A., Feller, D. P., Wang, Z., O’Reilly, T., and Sabatini, J. (2023). Exploring Thresholds in the Foundational Skills for Reading and Comprehension Outcomes in the Context of Postsecondary Readers. J. Learn. Disabil. 56, 43–57. doi: 10.1177/00222194221087387
Mancilla-Martinez, J., Hwang, J. K., and Oh, M. H. (2021). Assessment selection for multilingual learners’ reading development. Read. Teach. 75, 351–362. doi: 10.1002/trtr.2053
Maris, G., and Bechger, T. M. (2009). On interpreting the model parameters for explanatory item response models. Measurement 7, 1–18. doi: 10.1080/15366360902717261
Masrai, A., and Milton, J. (2021). Vocabulary knowledge and academic success: A study of Arabic-speaking learners of English. Lang. Teach. Res. 25, 575–595. doi: 10.1177/1362168819859294
Mayo, M., Cox, S., Hernandez, M., Lares, J., Woodyard, S., Luksetich, A., et al. (2023). Empowering first-year students in English: Assessing the validity and reliability of directed self-placement [Poster presentation]. Long Beach: California State University.
Melguizo, T., Flores, S. M., and Velasquez, D. (2021). Lost in the transition: The cost of college-readiness English standards misalignment for students initially classified as English learners. J. High. Educ. 92, 815–846. doi: 10.1080/00221546.2021.1888634
Morton, T. (2022). Reviewing the research on informed self-placement: Practices, justifications, outcomes, and limitations. Center for the Analysis of Postsecondary Readiness (CAPR). Available online at: https://files.eric.ed.gov/fulltext/ED622178.pdf (Accessed January 13, 2025).
Nagy, W., and Townsend, D. (2012). Words as tools: Learning academic vocabulary as language acquisition. Read. Res. Q. 47, 91–108. doi: 10.1002/RRQ.011
Nelson, R., Nichols-Besel, K., and Tahtinen-Pacheco, S. (2024). Testing support for multilingual college students: Providing an equitable pathway. J. Multilingual Educ. Res. 54, 161–171. doi: 10.1080/10790195.2024.2384347
Öztürk, S. A., and Çubukçu, F. (2022). Exploring student-directed translanguaging in the English-centric classroom. Lang. Teach. Res. Q. 31, 159–173. doi: 10.32038/ltrq.2022.31.11
Perfetti, C. A., and Stafura, J. (2014). Word knowledge in a theory of reading comprehension. Sci. Stud. Read. 18, 22–37. doi: 10.1080/10888438.2013.827687
Perin, D. (2020). “Reading, writing, and self-efficacy of low-skilled postsecondary students” in The Wiley handbook of adult literacy. ed. D. Perin (Hoboken, NJ: Wiley Blackwell), 237–260.
Pintrich, P. R. (2000). “The role of goal orientation in self-regulated learning” in Handbook of self-regulation. eds. M. Boekaerts, P. R. Pintrich, and M. Zeidner (San Diego, CA: Academic Press), 451–502.
Pitoniak, M. J., Young, J. W., Martiniello, M., King, T. C., Buteux, A., and Ginsburgh, M. (2009). Guidelines for the Assessment of English Language Learners. Princeton, New Jersey: Educational Testing Service.
Prevoo, M. J. L., Malda, M., Mesman, J., and van IJzendoorn, M. H. (2016). Within-and cross-language relations between oral language proficiency and school outcomes in bilingual children with an immigrant background: A meta-analytical study. Rev. Educ. Res. 86, 237–276. doi: 10.3102/0034654315584685
Ramírez, J. D. (2000). “Bilingualism and literacy: Problem or opportunity? A synthesis of reading research on bilingual students” in A Research Symposium on High Standards in Reading for Students from Diverse Language Groups: Research, Practice & Policy (Washington, DC: U.S. Department of Education, Office of Bilingual Education and Minority Languages Affairs (OBEMLA)), 9–41.
Ramirez, G. (2009). Cross-linguistic influence in second language acquisition: The impact of first language morphology on second language derivational morphology. Biling. Lang. Congn. 12, 25–37. doi: 10.1017/S1366728908003303
Reynolds, E. (2003). “The role of self-efficacy in Writing and Directed Self-Placement” in Directed self-placement: Principles and practices. eds. D. J. Royer and R. Gilles (Cresskill, NJ: Hampton Press), 73–103.
Rhew, E., Piro, J. S., Goolkasian, P., and Cosentino, P. (2018). The effects of a growth mindset on self-efficacy and motivation. Cogent Educ. 5:1492337. doi: 10.1080/2331186X.2018.1492337
Rouet, J.-F. (2006). The Skills of Document Use: From Text Comprehension to Web-Based Learning. 1st Edn. New York, NY: Routledge.
Rouet, J.-F., Britt, M. A., and Durik, A. (2017). RESOLV: Readers' representation of reading contexts and tasks. Educ. Psychol. 52, 200–215. doi: 10.1080/00461520.2017.1329015
Royer, D. J., and Gilles, R. (1998). Directed self-placement: An attitude of orientation. Coll. Compos. Commun. 50, 54–70. doi: 10.58680/ccc19981316
Rutgers, D., Evans, M., Fisher, L., Forbes, K., Gayton, A., and Liu, Y. (2024). Multilingualism, multilingual identity and academic attainment: Evidence from secondary schools in England. J. Lang. Identity Educ. 23, 210–227. doi: 10.1080/15348458.2021.1986397
Schmitt, N. (2014). Size and depth of vocabulary knowledge: What the research shows. Lang. Learn. 64, 913–951. doi: 10.1111/lang.12077
Sclove, S. L. (1987). Application of model-selection criteria to some problems in multivariate analysis. Psychometrika 52, 333–343. doi: 10.1007/BF02294360
Şen, Y., and Kuleli, M. (2015). The Effect of Vocabulary Size and Vocabulary Depth on Reading in EFL Context. Procedia Soc. Behav. Sci. 199, 555–562. doi: 10.1016/j.sbspro.2015.07.546
Sireci, S. G. (2020). Standardization and UNDERSTANDardization in educational assessment. Educ. Meas. Issues Pract. 39, 100–105. doi: 10.1111/emip.12377
Snow, C. (2002). Reading for Understanding: Toward an R&D Program in Reading Comprehension. Santa Monica, CA: RAND Corporation.
Suzuki, Y., and Sunada, M. (2018). Automatization in second language sentence processing: Relationship between elicited imitation and maze tasks. Biling. Lang. Congn. 21, 32–46. doi: 10.1017/S1366728916000857
Toth, C., and Aull, L. (2014). Directed self-placement at 20: A narrative analysis of research trends. Writ. Program Admin. 38, 56–84. doi: 10.1016/j.asw.2013.11.006
Uto, M. (2021). A multidimensional generalized many-facet Rasch model for rubric-based performance assessment. Behaviormetrika 48, 425–457. doi: 10.1007/s41237-021-00144-w
van Buuren, S., and Groothuis-Oudshoorn, K. (2011). mice: Multivariate Imputation by Chained Equations in R. J. Stat. Softw. 45, 1–67. doi: 10.18637/jss.v045.i03
van Heuven, W. J. B., Mandera, P., Keuleers, E., and Brysbaert, M. (2014). Subtlex-UK: A new and improved word frequency database for British English. Q. J. Exp. Psychol. 67, 1176–1190. doi: 10.1080/17470218.2013.850521
Vrieze, S. I. (2012). Model selection and psychological theory: A discussion of the differences between the Akaike information criterion (AIC) and the Bayesian information criterion (BIC). Psychol. Methods 17, 228–243. doi: 10.1037/a0027127
Wigfield, A. (1994). Expectancy value theory of achievement motivation: A developmental perspective. Educ. Psychol. Rev. 6, 49–78. doi: 10.1007/BF02209024
Wigfield, A., and Eccles, J. S. (1992). The development of achievement task values: A theoretical analysis. Dev. Rev. 12, 265–310. doi: 10.1016/0273-2297(92)90011-P
Wigfield, A., and Eccles, J. S. (2002). “The development of competence beliefs, expectancies for success, and achievement values from childhood through adolescence,” in Educational Psychology: Development of Achievement Motivation. eds. A. Wigfield and J. S. Eccles (San Diego, CA: Academic Press), 91–120.
Wilson, M., De Boeck, P., and Carstensen, C. H. (2008). “Explanatory item response models: A brief introduction” in Assessment of competencies in educational contexts. eds. J. Hartig, E. Klieme, and D. Leutner (Boston, MA: Hogrefe & Huber Publishers), 91–120.
Keywords: multilingual learners, directed self placement, reading motivation, vocabulary, explanatory item response models
Citation: Kaldes G, Braasch JLG and Kessler E (2025) The role of motivation in literacy assessment: using item-level analyses to inform college placement for multilingual learners. Front. Educ. 10:1521482. doi: 10.3389/feduc.2025.1521482
Edited by:
Catalina Lomos, Luxembourg Institute of Socio-Economic Research, LuxembourgReviewed by:
Semirhan Gökçe, Niğde Ömer Halisdemir University, TürkiyeFei Tang, Yunnan University, China
Copyright © 2025 Kaldes, Braasch and Kessler. This is an open-access article distributed under the terms of the Creative Commons Attribution License (CC BY). The use, distribution or reproduction in other forums is permitted, provided the original author(s) and the copyright owner(s) are credited and that the original publication in this journal is cited, in accordance with accepted academic practice. No use, distribution or reproduction is permitted which does not comply with these terms.
*Correspondence: Gal Kaldes, Z2thbGRlczFAZ3N1LmVkdQ==