- 1Department of Management, College of Business Administration, Princess Nourah Bint Abdulrahman University, Riyadh, Saudi Arabia
- 2Department of Information Systems, Sultan Qaboos University, Muscat, Oman
- 3Department of Pharmaceutical Sciences, College of Pharmacy, King Saud bin Abdulaziz University for Health Sciences, Riyadh, Saudi Arabia
Introduction: In an era where artificial intelligence (AI) is rapidly transforming the healthcare sector, understanding the perceptions of future healthcare professionals is vital. This study aims to assess the attitudes of healthcare students in Saudi Arabia toward AI and to evaluate their views on its impact on medical education and future healthcare careers.
Methods: A nationwide survey was conducted across 21 universities in Saudi Arabia. The study targeted healthcare students from various academic years and disciplines. Data were collected on students' exposure to AI, their educational backgrounds, and their perceptions of AI's role in healthcare.
Results: The survey revealed significant gender-based differences in perceptions of AI. There was a strong consensus on the importance of integrating AI into healthcare curricula. However, respondents also expressed caution regarding the application of AI in clinical practice. Attitudes varied based on students' year of study, level of AI exposure, and educational background, indicating a complex set of influences on their views.
Discussion: The findings highlight the multifaceted perspectives of future healthcare professionals regarding AI. The results suggest the necessity of developing tailored educational strategies that incorporate AI into the curriculum while addressing concerns about its clinical implementation. These insights are essential for preparing students for an AI-integrated healthcare system in the Kingdom of Saudi Arabia.
1 Introduction
In the dynamic landscape of healthcare, the advent of artificial intelligence (AI) has ushered in a new era of innovation and transformation. As AI continues to evolve, it is imperative to understand its implications on various aspects of healthcare, particularly in the context of education (Chen et al., 2020). This study aims to delve into the perceptions of healthcare students in the Kingdom of Saudi Arabia (KSA) toward AI, providing valuable insights that can guide the integration of AI in healthcare curricula.
1.1 Background
Artificial intelligence, with its ability to mimic human intelligence and automate complex tasks, has made significant strides in various sectors, and healthcare is no exception (Han et al., 2020). From predictive analytics and precision medicine to automated diagnostics and patient care, AI is revolutionizing the way healthcare is delivered (Alowais et al., 2023).
In the realm of medical education, AI has the potential to enhance learning experiences, facilitate personalized learning, and prepare students for a future where AI will be an integral part of healthcare. However, the successful integration of AI in medical education hinges on the perceptions and attitudes of the learners themselves–the future healthcare professionals (Buabbas et al., 2023).
Understanding their perceptions toward AI, their apprehensions and expectations can provide valuable insights for educators and policymakers, helping them tailor AI education strategies that are effective and well-received (Jones et al., 2021; Hardiker and Grant, 2011). This is particularly relevant in the context of KSA, where AI is increasingly recognized for its potential to enhance healthcare delivery and outcomes.
1.2 Significance of the study
The significance of this study lies in its potential to influence the integration of AI in healthcare education in Saudi Arabia. As AI continues to permeate various aspects of healthcare, it is crucial for future healthcare professionals to be well-versed with its applications and implications (Hassan et al., 2023). However, the successful integration of AI in healthcare curricula depends largely on the perceptions and attitudes of the students themselves. By exploring these perceptions, this study can provide valuable insights to guide educators and policymakers in developing effective AI education strategies. Furthermore, by focusing on healthcare students from diverse disciplines across 21 universities in Saudi Arabia, this study can help ensure that the AI education strategies are inclusive and cater to the diverse needs of all healthcare students.
1.3 Objectives of the study
The main objectives of this study are:
1. To understand the perceptions of healthcare students in Saudi Arabia toward AI and its impact on their education and future careers.
2. To explore the factors that influence these perceptions, such as the students' year of study, exposure to AI, and educational backgrounds.
3. To identify any gender-based differences in the perceptions of AI among healthcare students.
4. To gauge the perceived importance of AI in healthcare careers and its necessity in healthcare curricula.
5. To provide insights that can guide the development of effective and inclusive AI education strategies for healthcare students in Saudi Arabia.
1.4 Scope of the study
The scope of this study is broad yet focused, encompassing the perceptions of healthcare students toward AI across 21 universities in Saudi Arabia. The study is not limited to medical students but includes students from diverse healthcare disciplines such as pharmacy, nursing, dental hygiene, medical engineering, physical therapy, and clinical nutrition. This inclusive approach ensures a comprehensive understanding of AI perceptions across the healthcare education landscape in Saudi Arabia.
The study aims to explore various factors that influence these perceptions, including the students' year of study, exposure to AI, and educational backgrounds. It also seeks to identify any gender-based differences in these perceptions. However, the study does not delve into the technical aspects of AI or its specific applications in healthcare.
By defining this scope, the study ensures a focused yet comprehensive exploration of the research objectives, providing valuable insights that can guide the integration of AI in healthcare education in Saudi Arabia.
1.5 Structure of the article
The article unfolds in a structured manner to comprehensively explore healthcare students' perceptions of artificial intelligence (AI). Section 2 critically examines existing literature. This includes sub-sections that delve into AI in healthcare, survey-based research in medical education and AI, and attitudes and perceptions toward AI in education.
Moving forward, Section 3 elucidates the research approach. It encompasses survey design, participant details, data collection methods, the chosen approach to data analysis, and a candid discussion about the study's limitations.
Following the methodology, Section 4 employs rigorous statistical methods. This includes demographic analysis, an exploration of participants' views on AI, insights derived from surveyed student data, a nuanced examination of diverse perceptions of artificial intelligence, and an assessment of sentiment toward AI in the field.
Following this, Section 5 meticulously examines the obtained results, providing a comprehensive analysis and deriving meaningful findings. The subsequent conclusion is drawn based on these insights, which contribute to a nuanced understanding of the research outcomes.
Finally, Section 6 artfully ties together key findings. It encapsulates the implications drawn from the study, offering a robust conclusion and pointing toward potential avenues for future research.
2 Literature review
2.1 AI in healthcare
Artificial intelligence (AI) is no longer a futuristic vision; it is rapidly transforming the healthcare landscape (Kumar et al., 2023). From enhancing diagnostic accuracy through algorithms that analyze medical images to predicting patient outcomes and assisting in treatment plans, AI offers a powerful arsenal for improved healthcare delivery (Pierre et al., 2023; Edison, 2023). Its applications extend beyond clinical practice, revolutionizing medical education by providing students with immersive simulations and personalized learning experiences (Guze, 2015). However, this technological surge also necessitates critical consideration. Ethical implications surrounding data privacy, algorithmic bias, and the human–machine interface require careful thought and responsible implementation. As we navigate this transformative era, understanding the potential and pitfalls of AI in healthcare is crucial. This study delves into this complex terrain, exploring both the promises and challenges of integrating AI into healthcare education and practice, ultimately aiming to shape a future where technology elevates the quality of care without compromising ethical principles.
Over the past decade, a robust body of research has emerged exploring the myriad applications of artificial intelligence in healthcare, paving the way for a potential transformation in medical diagnosis, treatment, and practice. The outbreak of the COVID-19 pandemic has led to a growing body of research explicitly highlighting the significant contribution of artificial intelligence in healthcare (Piccialli et al., 2021; Rodriguez-Rodriguez et al., 2021). This emphasis stems from the pandemic's unique challenges, where restrictions on social interaction necessitated a heightened focus on the utilization of AI and robotics to maintain quality patient care (Wang and Wang, 2021; Getson and Nejat, 2021; Bhaskar et al., 2020).
According to Rajpurkar et al. (2022), AI has the potential to improve healthcare and revolutionize medicine. The authors provide their 2-year learning on medical AI's major changes. New study methods include leveraging non-image data sources and increasing human–AI collaboration. It also addresses scientific and moral challenges such as data scarcity and racial prejudice, demonstrating how difficult AI in healthcare is?
The study by Choi et al. (2020) introduces an AI-based clinical decision support system for heart failure diagnosis, demonstrating high accuracy in tests with 1198 patients. The hybrid approach combines expert knowledge and machine learning, highlighting AI's role in enhancing diagnostics for complex medical conditions. In Dave et al. (2020), the authors emphasize enhancing transparency in healthcare AI, particularly for heart disease diagnosis, using explainable AI techniques. The study explores feature-based and example-based methods, highlighting their role in making AI predictions more interpretable and trustworthy for medical practitioners. A new framework called HealthFaaS was proposed for AI-based smart healthcare for heart patients using serverless computing (Golec et al., 2023). An IoT data analytics solution utilizing machine learning models focuses on dynamic scalability and efficient resource usage. In the study, the LightGBM model is demonstrated as effective in predicting heart disease and evaluated in terms of throughput, latency, and quality of service (QoS). In the study by Keshri et al. (2022), the authors explore the application of AI to diagnose and treat brain disorders. AI methods are discussed in neurology along with their clinical applications, including diagnosis, surgery, and postoperative assessment. It also discusses AI's role in improving medical professionals' judgment and overcoming existing obstacles to its effective implementation in brain care. In the study by Naghshvarianjahromi et al. (2021), they discuss the application of brain-inspired intelligence systems in healthcare. It delves into how these systems can mimic natural brain processes to improve healthcare screening and diagnostics, offering more efficient, accurate, and cost-effective solutions than traditional methods. Vinokurtseva and Veettil (2020) explore the use of artificial intelligence in mental healthcare. It discusses how AI can improve diagnosis and treatment processes by analyzing data from personal digital devices. This study addresses the potential benefits and challenges of implementing AI in mental healthcare, emphasizing the importance of policy-making to address privacy and confidentiality concerns. In another study, Johnson et al. (2022) highlight the growing potential of AI in enhancing healthcare practices by predicting lung cancer prognosis with high accuracy. By identifying crucial factors for survivability, it paves the way for personalized treatment plans and improved patient outcomes in future. In another research, Esteva et al. (2017) presented a convolutional neural network (CNN) trained on a large dataset of 129,450 clinical images, achieving accuracy comparable to dermatologists. CNN's potential for extending dermatological diagnostics beyond the clinic is highlighted, offering the prospect of affordable universal access to vital diagnostic care. A study by Sharma et al. (2020) examines the effects and potential developments of artificial intelligence (AI) technology in cardio-thoracic diagnostic imaging. In diagnostic imaging, AI is discussed at several stages, including image collection, preparation, reading, reporting, prediction, and prescription. The article examines the challenges and opportunities in radiology and explores the potential impact of artificial intelligence on efficiency, accuracy, and patient outcomes. Li et al. (2021) systematically reviewed 38 studies on AI's impact in diagnosing thoracic pathologies using chest X-ray and CT. AI as a concurrent reader improved physicians' diagnostic performance, but limitations include varying study quality, a lack of standardization, diverse settings, and ethical concerns about reliance on AI in clinical practice.
In Winkel et al. (2022), authors presented and validated a fully automated, deep learning-based branch-wise coronary artery calcium (CAC) scoring algorithm on a multi-center dataset. The objective of the study was to demonstrate that fully automated total and vessel-specific CAC scoring is feasible using a DL-based algorithm. The study has some limitations, such as the retrospective nature of the study and the fact that the algorithm was tested on a single vendor's scanner.
In another study, Wang et al. (2023) presented a novel method for automatic coronary artery segmentation in CCTA images. The suggested method uses an algorithm based on deep learning called DR-LCT-UNet. It has two new parts: the Dense Residual (DR) module and the Local Contextual Transformer (LCT) module. The objective of the study was to demonstrate that automatic coronary artery segmentation is feasible using a DL-based algorithm. The study has some limitations, such as the fact that it was tested on a single dataset.
Muscogiuri et al. (2022) explore the use of artificial intelligence (AI) in cardiovascular imaging. The authors discuss the potential of AI to improve the accuracy and efficiency of cardiovascular imaging, which can lead to better diagnosis and treatment of cardiovascular diseases. The study also highlights the challenges and limitations of AI in this field, such as the need for large datasets and the potential for bias.
In a different research, Cellina et al. (2022) discuss the potential applications of artificial intelligence (AI) in emergency radiology. The authors highlight the benefits of AI in improving image acquisition, workflow efficiency, and detection of emergency disorders such as intracranial hemorrhage, bone fractures, and pneumonia. The study also emphasizes the importance of smart reporting, which can provide an objective indicator of the disease's severity and optimize treatment planning. Overall, this study provides a comprehensive overview of the current state of AI in emergency radiology.
In another study, the authors investigate physicians' views on incorporating AI into diagnostic pathology (Sarwar et al., 2019). Conducted through a survey sent to pathologists and pathology residents in Canada and the United States, the study reveals physician optimism about AI's potential in diagnostic pathology. However, concerns include the necessity for increased education and training, potential errors, and the impact on the physician–patient relationship.
Furthermore, research conducted by Rezvantalab et al. (2018) explores the application of convolutional neural networks (CNNs), including DenseNet 201, ResNet 152, Inception v3, and InceptionResNet v2, in the dermoscopic classification of eight skin diseases. The study aims to evaluate the effectiveness of these deep learning models on a dataset comprising various diagnostic categories. While the CNNs demonstrated superior classification accuracy compared to dermatologists, potential limitations include the need for further validation and consideration of dataset biases.
2.2 Survey-based research in medical education and AI
In the evolving landscape of medical education, the integration of artificial intelligence (AI) has become a focal point, prompting a surge in survey-based research endeavors to understand its impact. This section explores the intersection of medical education and AI through the lens of survey-based studies. These investigations delve into the perceptions, attitudes, and experiences of educators, students, and healthcare professionals regarding the incorporation of AI technologies in medical training. By synthesizing the findings of diverse surveys, we aim to gain valuable insights into the current landscape of AI in medical education, elucidating both the challenges and opportunities that shape the future of this dynamic and transformative field.
Nikiforova et al. (2021)'s study provides a detailed look at survey design and administration of best practices in medical education research. The authors discuss survey design procedures, identifying well-written and badly written questions, and using survey design concepts. The authors advise using verified survey instruments and emphasizes transparent reporting of survey methodology and findings. This article helps researchers and educators conduct high-quality medical education surveys.
Using consumer perspectives on AI-based medical devices with clinical decision assistance, Esmaeilzadeh and Pouyan found that technological, ethical, and regulatory issues increase perceived risks. Technology concerns such as performance and communication predict risk beliefs. According to the study by Esmaeilzadeh (2020), healthcare AI deployments require normative standards, evaluation methods, and continual monitoring to assure safety, quality, and ethics.
In their study Castagno and Khalifa (2020), Castagno and Khalifa look at how healthcare workers feel about AI in healthcare. Th study included a detailed poll of 98 doctors, nurses, therapists, and managers. The data show that most health workers do not understand how AI works and are worried about how it will be used in clinical settings, but they all agree that AI has benefits in the medical field. To address the concerns of healthcare workers, the study stresses the need for more education and clear rules. Healthcare workers need to work together to add AI to professional practice. If they do not work together, the medical field could miss out on a great chance. This study stresses the need for more research.
Ezzaim et al. (2022) evaluate research publications on ScienceDirect from 2019 to 2021 on AI in education (AIEd). The essay finds that AIEd can improve teaching and learning, but it also notes its limitations and ethical issues. The authors suggest more study on AIEd's capabilities and ramifications.
Lee et al. (2021) conducted a scoping review on the impacts of AI on undergraduate medical education (UMED). The study divides UMED AI applications into learning assistance, assessment, curriculum design, and research. It discusses the pros and cons of AI implementation, including improved student engagement, personalized learning, feedback, accessibility, cost reduction, quality assurance, and ethical and technical issues. The conclusion underlines AI's revolutionary potential in UMED to improve student outcomes and the need for further research and assessment to maximize its impact.
The author of Masters (2019) looks at the pros and cons of using AI in medical education, focusing on how it can help with teaching, learning, and managing. The study stresses the importance of preparing medical students for a healthcare system that is affected by AI by teaching them about AI and its ethical effects. The material that was summed up fits with conversations about the pros and cons of AI in medical education. These have focused on personalized learning, adaptive assessment, and ethical use during the COVID-19 pandemic, calling for more study, collaboration, and integration for better results.
Sun et al. (2023) conducted a systematic review to assess the current state and challenges of AI in healthcare and medical education. They utilized databases such as PubMed, Embase, Cochrane, and CNKI, focusing on literature from 2017 to July 2022. Their objective was to provide an overview of AI applications in these fields, including issues and potential directions. The study acknowledges limitations, such as the inclusion of only English and Chinese literature and the generally low quality of current research on AI in medical education.
Chassignol et al. (2018) presented a comprehensive overview of the role of AI in education. They explore how AI influences various aspects of the educational process, including customized content, innovative teaching methods, technology-enhanced assessment, and communication between students and educators. The study aims to identify how AI reshapes the educational landscape, focusing on the impact of AI on student–teacher interaction and learning efficiency. Limitations include a lack of detailed examination of specific AI technologies and their direct effects on educational outcomes.
In this narrative study, Cadamuro (2021) examine the use of artificial intelligence (AI) in medical laboratories. The review describes how AI can be used in healthcare and lab science. Moreover, it discusses AI algorithms' problems, such as data quality, ethics, human control, and rules and regulations. The article emphasizes that AI is not a substitute for doctors but an additional tool. AI could combine clinical and diagnostic data, providing insights for diagnosis, prognosis, and treatment. Nevertheless, there is a notable lack of a comprehensive analysis of all existing or emerging AI applications and specific examples of implementation or evaluation.
Ouyang et al. (2022) conducted a systematic review analyzing 32 articles on the applications of artificial intelligence (AI) in online higher education from 2011 to 2020. Employing PRISMA principles, the authors assess functions, algorithms, effects, and implications of AI in online higher education, aiming to provide an overview, identify gaps, and propose future research directions. However, a review may not encompass all relevant studies that are not indexed or meet inclusion criteria.
Sallam et al. (2023) evaluated Jordanian healthcare students– approach to using ChatGPT. Based on the Technology Acceptance Model (TAM), they make and test the TAME-ChatGPT poll tool to find out how people feel about and use ChatGPT. In an online poll of 458 Jordanian healthcare students, the authors used exploratory factor analysis to test the validity and reliability of the survey tool. The authors discuss the implications for educational interventions using ChatGPT based on their findings.
Several additional studies have examined the use of AI in specific areas of medical education, such as feedback provision, personalized learning pathways, and assessment tools (Zhang et al., 2023; Civaner et al., 2022; Gillissen et al., 2022; Boillat et al., 2022). These investigations further support the promising potential of AI to enhance medical student learning and improve educational outcomes.
These survey-based studies paint a promising picture for AI's integration into medical education, showcasing its potential to improve learning outcomes and address various educational challenges. However, beyond the quantitative data, understanding the individual perspectives and perceptions toward AI plays a critical role in its successful implementation. The next section delves into this, exploring the diverse range of attitudes and perceptions held by both students and educators regarding AI's place in medical education.
2.3 Attitudes and perceptions toward AI in education
The landscape of medical education is undergoing a transformative shift with the increasing potential of artificial intelligence (AI). While survey-based research has provided valuable insights into the various applications and outcomes of AI integration, understanding the human element remains crucial. This section explores the diverse spectrum of attitudes and perceptions held by both students and educators toward AI in medical education. Examining these perspectives is crucial for ensuring that AI development and implementation in medical education align with educational goals, optimize learning outcomes, and address student needs effectively.
Embarking on a detailed examination of existing literature, we now turn our attention to specific research studies that illuminate the intricate tapestry of attitudes and perceptions toward AI in medical education. These studies offer diverse perspectives from both students and educators, shedding light on the multifaceted dimensions that influence the reception and integration of AI technologies in the educational realm.
Chan and Hu (2023) interviewed university students about generative AI (GenAI) in higher education. They focused on the familiarity, willingness to engage, potential benefits, and challenges of GenAI, using technologies such as ChatGPT as a reference. The study examined how these perspectives affect GenAI integration in schooling. While extensive, the research is constrained by its demographic focus and self-reported data, which may introduce biases.
A quantitative study by Obenza et al. (2024) examined university students' ChatGPT use and perceptions. They surveyed 500 students to examine their comprehension, perception, advantages, downsides, and intention to employ generative AI technologies. The study found that students understood and liked generative AI but were moderately concerned. The research, however, is limited by its focus on a specific regional demographic and reliance on self-reported data.
Busch et al. (2023a) conducted an international survey to understand medical students' attitudes toward AI in education and practice. The survey, involving participants from various countries, aimed to explore their knowledge, attitudes, and the current state of AI education. The study found generally positive attitudes toward AI but also noted limited AI knowledge among students. Limitations of the study include a low response rate per institution and potential selection bias.
Li et al. (2022) conducted a survey-based study to understand medical students' perceptions and behavioral intentions toward learning artificial intelligence (AI) in clinical practice. The study employed the Theory of Planned Behavior to examine factors influencing students' attitudes toward AI, involving 274 participants. The research aimed to bridge the gap in knowledge about medical students' intentions to adopt AI technologies. However, it was limited to a specific demographic and might not represent broader student populations.
Gillissen et al. (2022) investigated the perspectives of medical students toward AI and digitization through a mixed-methods methodology. The research employed semi-structured interviews and a subsequent survey to investigate the impact of curriculum type, gender, and educational level on the perceptions of AI among German medical students. The discussion unveiled diverse perspectives on the usefulness of AI in diagnostics and the level of readiness for integrating AI into medicine. The study is limited by its narrow emphasis on a particular national environment and its dependence on self-reported data.
Bisdas et al. (2021) conducted a global poll to find out the perspectives of medical and dental students on AI. Over 3,000 students from 63 countries took part in the study, which looked at what they knew, how they felt about AI in their area, and how AI might change future practice. Even though there was a lot of study, there were some problems. For example, the fact that the survey was sent out online and relied on self-reported data could have led to selection bias.
To ascertain the perspectives of international pharmacy students regarding AI in medicine, Busch et al. (2023b) conducted a survey. Utilizing an online survey, the research endeavored to evaluate the technological proficiency, awareness, and perspectives of the students with regard to the incorporation of AI in pharmacy education and their careers. There are some problems with the study, like the fact that it only looked at a certain group of students, which could have caused reaction bias.
A survey was conducted by Sit et al. (2020) among medical students in the United Kingdom to ascertain their perspectives on the integration of AI into healthcare and its potential influence on their career decisions within the field of radiology. Using an anonymous electronic survey, the research uncovered concerns regarding the impact of AI on radiology careers while emphasizing a general belief in the significance of AI in healthcare. Limitations include a potential selection bias and a non-representative sample of all UK medical students.
Liu et al. (2022) surveyed medical students in the United States in an effort to ascertain their perspectives on AI in medicine. The purpose of the research was to determine which learning formats and subjects medical students found most agreeable in relation to AI in medicine, as well as to evaluate their familiarity, knowledge, and attitudes toward AI. The study's limitation includes potential selection bias due to a relatively small sample size that is not fully representative of the entire US medical student body.
Galán and Portero (2022) assess Spanish medical students about AI's impact on radiology. The main goals are to test students' awareness and grasp of AI in radiology, evaluate their thoughts and expectations regarding its significance in their future professions, and determine their agreement or disagreement with AI-related statements. The non-representative sample, which only comprises students who completed the online survey, may restrict the generalizability of findings to Spanish medical students.
In their study, Li and Qin (2023) explore medical students' views on integrating AI into medical education. The authors identify significant elements impacting medical students' acceptance and intention to utilize AI using the Unified Theory of Acceptance and the utilization of Technology model. The main goals are to assess students' AI awareness and understanding, evaluate their thoughts and expectations about its significance in their future jobs, and offer a curriculum and design for teaching AI abilities.
In their study, Teng et al. (2022) examine Canadian healthcare students' understanding and attitudes toward AI. The research of health profession students from numerous institutions found that most of them expect AI to affect their occupations within 10 years. Overall, these students view AI positively in their fields. However, attitudes vary by field, emphasizing the need to incorporate AI basics into educational curricula. Due to the diverse perspectives and knowledge gaps of healthcare students, the study stresses the importance of AI literacy in healthcare education.
While current literature extensively explores the perceptions of medical students toward artificial intelligence (AI) in various countries such as the USA, Germany, Canada, the UK, and China, a notable gap exists in the context of Saudi Arabia (KSA). To address this gap, our study embarks on a comprehensive survey covering 21 universities across KSA. This research goes beyond the conventional focus on medicine students, encompassing diverse healthcare disciplines such as pharmacy, nursing, dental hygiene, medical engineering, physical therapy, and clinical nutrition. By including students across all academic years and departments, our inclusive methodology ensures a holistic representation of AI perceptions in the healthcare education landscape of KSA. This nuanced approach distinguishes our study, offering a unique contribution to the understanding of AI perceptions, which is particularly crucial for tailoring AI integration strategies to the diverse needs of healthcare students in KSA. In the upcoming methodology section, we detail our survey design, data collection process, and analytical approach, providing insights into the robust methodology employed for this comprehensive study.
3 Methodology
In the rapidly evolving field of healthcare, the integration of artificial intelligence (AI) has become a topic of significant interest. This study aims to explore this interest further by delving into the perspectives of healthcare students across the Kingdom of Saudi Arabia (KSA). Drawing upon diverse medical disciplines and universities within KSA's healthcare landscape, this study sheds light on future healthcare professionals' perspectives on AI and their call for AI education, informing approaches to equip the Kingdom's medical workforce for the AI-driven future of healthcare. The following sections detail the meticulous design and execution of the survey, the rigorous data cleaning process, and the insightful analysis of the collected data.
3.1 Survey design
Our study's methodology was influenced by the research conducted by Teng et al. (2022). Their extensive survey, which included several types of questions such as multiple-choice, pick-group-rank, 11-point Likert scale, slider scale, and narrative questions, provided a great framework for our data collection procedure.
We modified their questionnaire, customizing it to align with the particular requirements and goals of our study. By doing so, we were able to gather a diverse array of responses, which in turn provided us with a comprehensive dataset for analysis. Utilizing a comparable questionnaire facilitates the possibility of comparing our study with the research conducted by Teng et al., thus enhancing our comprehension of the topic at hand.
It is crucial to acknowledge that although our questionnaire was influenced by the one utilized in the Teng et al. (2022), our research inquiries, analysis, and interpretations are unique to our study.
The survey, prioritizing participant confidentiality and anonymity, adhered to ethical guidelines with explicit informed consent. The questionnaire design, meticulously crafted after a thorough narrative literature review of attitudinal surveys on artificial intelligence (AI), aimed to capture nuanced perspectives, drawing inspiration from successful elements found in prior surveys, including those discussed by Teng et al. It was tailored to specific demographics and underwent a pilot phase. Input from a diverse group, including students and clinicians, during this phase, played a crucial role in refining question clarity. This final iteration comprised 16 items, utilizing diverse question types, and explored six key areas–demographics, self-reported understanding of AI, attitudes toward AI's impact on their field, preferences for AI literacy incorporation in the curriculum, priorities in AI literacy education, and willingness to allocate time for acquiring basic AI literacy. As discussed earlier, this comprehensive approach ensured a thorough understanding of healthcare students' views on AI.
3.2 Participants and data collection
The survey targeted healthcare students in the Kingdom of Saudi Arabia (KSA) across diverse disciplines, including pharmacy, nursing, and allied health fields. The inclusion criteria comprised participants aged 18 years or older currently enrolled in healthcare programs in KSA. Responses from students studying outside the country or those not enrolled in entry-to-practice programs were excluded to maintain relevance.
The study involved a total of 400 participants from 21 universities in Saudi Arabia offering healthcare and medical-related degrees. Data collection commenced in February 2023 and extended over 6 months. A diverse group of participants from various academic backgrounds ensured a representative sample. Responses were meticulously cleaned to maintain data uniformity, and incomplete responses (those with a completion rate below 90%) were excluded from the analysis. Moreover, the survey was administered in both Arabic and English to accommodate linguistic diversity. Subsequently, the dataset underwent thorough cleaning to ensure data consistency and reliability.
3.3 Data analysis
A comprehensive statistical analysis was conducted, covering diverse dimensions such as demographic profiles, attitudes toward AI, and preferences for AI education. Descriptive statistics and inferential analyses were applied to derive meaningful insights from the dataset.
3.4 Limitations
Acknowledging potential limitations, including self-reporting biases and exclusion based on completion rates, the study provides valuable insights into healthcare students' perspectives on AI education and its impact on their careers.
This comprehensive methodology ensured the ethical conduct of the study, a diverse and representative study cohort, and rigorous data collection and analysis procedures. The findings contribute to a deeper understanding of healthcare students' perspectives on AI in the Kingdom of Saudi Arabia.
4 Statistical analysis
In this section, we present a comprehensive statistical analysis of the dataset obtained from healthcare students in the KSA. Our examination encompasses a broad spectrum of themes, spanning demographics, AI attitudes, and preferences for AI education.
As outlined in the preceding section, our meticulously designed questionnaire sought to capture the diverse viewpoints of healthcare students in Saudi Arabia. Encompassing a wide array of academic disciplines such as pharmacy, nursing, and allied fields, the survey aimed to provide a realistic cross-section of healthcare education in the country. This holistic approach ensures that our analysis not only delves into the statistical intricacies of the dataset but also reflects the rich diversity inherent in the perspectives of healthcare students across various domains.
The subsequent subsections delve into specific facets of our statistical analysis, shedding light on demographic patterns, students' data and perspectives, varied perceptions of artificial intelligence, and the prevailing sentiments toward AI within the healthcare field. Each subsection offers a nuanced exploration of the dataset, providing valuable insights into the intricate interplay between demographics and attitudes toward artificial intelligence among healthcare students in KSA.
4.1 Demographic analysis and participants' views on AI
This section provides an in-depth examination of the participants' demographics and their perceptions about artificial intelligence (AI) as it applies to healthcare education and practice. A detailed demographic profile of the participants includes information on their age, gender, greatest level of education, and the academic program in which they were enrolled. These demographics provide a more comprehensive picture of the sample composition since they reflect the various healthcare disciplines covered. The age distribution of participants was divided into eight categories, including those under the age of 21 years and individuals aged 21–25, 26–30, 31–35, 36–40, 41–45, 46–50, and above 50 years. This classification gives a thorough examination of the age distribution of participants across various healthcare programs.
This part also dives into healthcare students' opinions of AI and desires for AI education. It delves into the many perspectives stated by participants on the incorporation of AI in their respective healthcare professions. The study focuses on their feelings, attitudes, and expectations about using artificial intelligence in healthcare education and practice.
We continue by reviewing the substantial survey data, which reveals the participants' expectations and readiness for integrating AI in healthcare education and practice.
4.2 Surveyed student data and perspectives
In this section, we will go over some of the significant parameters that were included in the survey to obtain insight into the opinions of healthcare students across several areas. The survey parameters included information about the university, college, and program in which participants were enrolled, as well as their program year, age, and gender. Furthermore, the study sought to determine the respondents' greatest degree of education.
The survey's most important part was defining artificial intelligence (AI) in a single statement. Participants were also polled on the significance of AI in their individual fields of study and their perceptions on AI's impact on their future careers. Participants were asked to rate their belief in AI's involvement on a scale of 0–10. Furthermore, the study investigated whether healthcare students believed that a core knowledge of AI was required for their education and if they comprehended the ethical implications surrounding AI in their areas.
Participants were also asked to score their optimism about AI in their industries, their reservations about AI's role, and their conviction in the importance of proper AI management. Responses were recorded on a scale of 0–10.
The poll also went into the emotional aspects, with participants asking how they felt about AI's integration into their various areas. They were also urged to outline their main goals and indicate when they thought AI might impact their careers.
A substantial portion of the study focused on AI education, namely its inclusion in the curriculum verses extracurricular learning. Participants were also questioned about their desired lengths for AI learning, considering both in-curriculum and extracurricular possibilities.
Finally, participants were asked to discuss their career aspirations and their top three AI-related goals. This extensive study provides useful insights into healthcare students' perspectives and aspirations regarding AI across all facets of their educational and professional experience.
These components include a wide range of critical criteria influencing healthcare students' opinions on AI in their different areas. It is worth noting that several of these characteristics are investigated and built on more in this section, providing a more complete knowledge of the participants' perspectives and expectations.
4.3 Diverse perceptions of artificial intelligence
In this part, we look at the different ideas and thoughts that people have about artificial intelligence (AI). The answers that people gave show the different ways that they see what AI means to them. It is clear that AI is a complex and changing idea that can be understood in different ways depending on one's background, knowledge, and experience in the field. When asked to describe artificial intelligence (AI), most of the people who took the survey said, “I Don't Know,” which made up a big 27.91% of the total participants. After looking into this group more, we found that 18.86% were women and 9.04% were men. Moreover, approximately 5.67 percent of the people who answered gave answers such as “NA” or said that the question did not apply or be important to them.
Approximately 3% of the people who took part were excited about AI. Some answers were positive about AI's potential, seeing it as something to be excited about and look forward to.
Another important theme that approximately 3.6% of people who took part said they thought about was “The Future.” People in this group thought that AI was a very important tool that would shape the future.
Some of the people who answered were worried about AI, especially how it would affect jobs. These people talked a lot about how AI could take over jobs that people used to do, which is how some people feel.
Many people who took part talked about AI in terms of speed and automation. They talked about how AI can improve accuracy, make work easier, and make fields such as medicine and tech more efficient.
For some people who answered, the most important thing about AI was that it could learn and change. People who took part in the discussion stressed that AI is not just pre-programmed code but can change over time by learning from new data.
Another thing that people talked about was how they used data. Some stressed how important it is for AI to use data and algorithms. Some of the people who spoke also said that AI can solve problems through machine learning.
Some people who took the survey said that AI is a technology miming human intelligence for different purposes. The person explained that AI is a type of software that can think and act like a human.
Artificial intelligence (AI) was often linked to the areas of medicine and technology. Many people talked about how AI can be used to improve medical methods and other areas of technology.
Interactions between people and machines came up a lot in the answers. Some people talked about how AI makes it possible for people and machines to talk to each other, which could lead to machines that can understand and react to what people want and do.
One thing that came up over and over again was how people saw AI as a force for progress and new ideas. Many people saw AI as a way to improve technology and make new things in many areas of life.
People also talked about how AI makes daily life easier. They thought of AI as a technology that makes daily jobs and activities easier and more efficient.
In conclusion, the people who took part had different ideas about AI. Some were excited about it, while others stressed how important it would be in changing the future. Some people were worried that AI would take away jobs, while others were interested in how it could improve speed, learning, and the use of data. People also talked about AI's ability to mimic human intelligence, its uses in health and technology, how it can help people and machines work together, and its growth and innovation potential. In general, these answers show how complex AI is and how important it is in many areas of modern life.
4.4 Sentiment toward AI in the field
The participants exhibited a diverse array of opinions with regard to the incorporation of artificial intelligence (AI) inside their various domains. Significantly, a considerable proportion, roughly 20% of the participants, expressed a sense of hope and enthusiasm. The individuals in question have a sense of anticipation regarding the potential good impacts and breakthroughs that would be facilitated by artificial intelligence (AI) inside their respective domains. This sentiment fostered an atmosphere characterized by hope and enthusiasm for the forthcoming future.
Furthermore, a considerable proportion of the participants, approximately 9.77%, expressed their response as “I Don't Know” or “NA”. The members of this group exhibited a degree of uncertainty regarding the potential influence of artificial intelligence (AI) or expressed the view that AI may not be applicable to their respective areas. This observation may suggest a limited level of acquaintance with AI or a perception that AI is currently not pertinent to their unique professional responsibilities.
The research additionally indicated that a subset of participants, approximately 4.4%, held the perception that AI possesses utility and advantages, acknowledging its capacity to augment diverse facets inside their respective domains. A small percentage of individuals recognized the significance of artificial intelligence (approximately 3.1%), underscoring its substantial contribution to facilitating advancements.
In contrast, a minority of individuals expressed ambivalence toward AI, recognizing both its potential advantages and drawbacks. In contrast, an additional cohort of participants voiced apprehensions and anxieties pertaining to artificial intelligence (AI), specifically in regard to the preservation of employment stability and apprehensions surrounding potential fallibility. A subset of the participants expressed reservations regarding the integration of artificial intelligence (AI) within their respective domains. These reservations were mostly rooted in uncertainty regarding the efficacy of AI and apprehensions surrounding potential job displacement. Another cohort of participants employed terminology such as “concerned” or “anxious” to articulate their emotions around artificial intelligence, signifying a certain level of trepidation.
The results of the study shed light on the wide range of emotions and attitudes expressed by experts from many disciplines in relation to the changing role of artificial intelligence. These thoughts exemplify the intricate interplay between individuals and AI technology. The comments provided by most participants demonstrate the presence of diversity and highlight the diverse perspectives on AI held by individuals from different professional backgrounds.
Upon the conclusion of our extensive statistical examination, it becomes apparent that healthcare students in the Kingdom of Saudi Arabia possess a varied array of perspectives and emotions with regard to the utilization of AI within their different domains. The aforementioned thoughts serve as a great basis for our following discourse. In the subsequent section, we conduct a more comprehensive analysis of the primary discoveries, scrutinizing the ramifications of these varied viewpoints on healthcare education and practice. The analysis presented in this study provides insight into the intricate nature of AI roles and the intricate relationship between optimism, concerns, and expectations in this ever-evolving field.
5 Results and discussion
This section contains a presentation of the results and an extensive analysis of the survey findings. The research we conducted unveiled a plethora of information pertaining to the beliefs, expectations, and perceptions of healthcare students concerning AI. The study's data collection and analysis have created a unique opportunity to look into the ever-evolving landscape of AI in healthcare education and practice. We hope that this combined discussion and interpretation of our findings will shed light on the main findings, their ramifications, and the multifaceted nature of healthcare students' attitudes regarding AI, contributing to a better knowledge of this critical field.
5.1 Gender-based variances in perceived importance of AI
The survey findings provide valuable insights into the opinions of healthcare students, specifically focusing on gender-based distinctions within the context of the significance of AI. To assess the level of significance ascribed to AI within their particular domains, participants were requested to assign a rating on a numerical scale ranging from 0 to 10. A rating of 0 represented minimal relevance, while a rating of 10 denoted utmost importance. The replies were classified into five distinct categories based on their numerical values: extremely low (0–3), low (4–5), moderate (6–7), high (8–9), and very high (10). A comprehensive examination of these assessments reveals significant disparities between male and female participants.
Among male respondents, 8.96% considered AI to have very low importance in their fields, signifying a rating of 0–3. In contrast, 7.91% of female participants expressed similar sentiments, suggesting that a slightly higher proportion of male participants found AI to be of very low importance. Furthermore, 13.43% of male and 18.58% of female participants regarded AI with low importance (a rating of 4–5), indicating a more pronounced inclination among female participants toward this category as shown in Figure 1.
The moderate importance category (ratings 6–7) saw 15.67% of male and 20.16% of female participants. Interestingly, a higher percentage of female participants found AI to be moderately important in their respective fields. On the other hand, 23.13% of male and 16.21% of female participants believed AI to be highly important (ratings 8–9), demonstrating that a greater proportion of male participants attributed high importance to AI.
A striking commonality between male and female participants was found in the very high importance category (rating of 10), with 38.81% of male and 37.15% of female participants sharing the belief that AI holds the utmost importance in their fields.
An intriguing observation from the data is the existence of a minority, comprising approximately 2.06% of the total participants (eight individuals), who assigned a rating of 0 to AI, essentially considering it to have no importance in their field. Of these eight participants, five were female and three were male, suggesting a marginally higher representation of female participants among those who found AI to be utterly unimportant. A closer examination revealed that five of these participants were aged 20 years or less, suggesting that age might play a role in shaping these opinions. Furthermore, seven out of these eight participants, or 87.5%, had their highest education level listed as high school, potentially implying a correlation between educational background and the perception of AI's significance in their respective fields. These intriguing findings emphasize the varied spectrum of opinions among healthcare students regarding AI's importance and its nuanced relationship with gender, age, and educational background.
In our analysis, we also found it interesting that there are noticeable differences based on gender in how healthcare students perceive the importance of AI in their respective fields. It is intriguing to note that approximately 62% of male participants express that AI holds high or very high importance in their healthcare careers. Conversely, approximately 53.35% of female participants share a similar perspective, deeming AI as having high or very high importance in their respective fields.
While it is significant to acknowledge that a considerable proportion of both male and female participants attribute high or very high importance to AI in their healthcare professions, the difference in these percentages implies a slightly higher prevalence of such beliefs among male participants.
These varying perceptions may be influenced by a range of factors, including differences in exposure to AI-related information, levels of engagement with AI technologies, and variations in career aspirations and expectations among male and female healthcare students.
The presence of these variations underscores the importance of tailoring educational approaches to ensure that all healthcare students, irrespective of gender, receive the requisite AI-related knowledge and skills to excel in their future careers. Addressing these disparities is crucial to prepare healthcare professionals adequately for the evolving healthcare landscape, where AI is anticipated to play a substantial role.
Future research endeavors could delve deeper into the specific determinants of these gender-based differences in AI perception among healthcare students. This deeper understanding will enable the development of targeted educational strategies that bridge the gender gap in healthcare AI perceptions.
In conclusion, our findings highlight the significance of recognizing and addressing gender-based variations in AI perceptions among healthcare students, facilitating the design of effective educational programs that equip future healthcare professionals with the skills necessary to thrive in a technologically evolving healthcare landscape.
5.2 Gender-based perspectives on the imperative of cautious AI management
In this subsection, we explore and analyze the perspectives of healthcare students with regard to the necessity for careful AI management within their respective fields. The analysis specifically considers gender-based distinctions among survey respondents. To gauge the perceived importance of cautious AI management, participants were asked to rate it on a scale of 0–10, with 0 signifying very low importance and 10 indicating very high importance. These responses were categorized into five groups: very low (0–3), low (4–5), medium (6–7), high (8–9), and very high (10). A comprehensive examination of these ratings illuminates noteworthy variations based on gender.
As shown in Figure 2, among male respondents, 35.1% expressed that AI management is of very low importance (ratings 0–3) in their healthcare careers. In contrast, 22.5% of female participants shared a similar viewpoint, indicating a higher proportion of male participants who found AI management to be of very low importance.
The category of low importance (ratings 4–5) was chosen by 4.5% of male participants and 5.5% of female participants, demonstrating a similar perspective between genders regarding AI management.
Moving on to the medium importance category (ratings 6–7), 9.0% of male and 6.3% of female participants fell into this group, indicating that a greater percentage of male participants perceived medium importance associated with AI management.
Interestingly, in the high importance category (ratings 8-9), 10.4% of male participants and 11.9% of female participants conveyed a belief in the importance of AI management. These percentages demonstrate a relatively equivalent view between genders in recognizing AI management as important.
Conversely, in the very high importance category (rating 10), a significant 41.0% of male and a remarkable 53.8% of female participants expressed the highest level of importance assigned to AI management. These findings suggest that both male and female participants overwhelmingly consider AI management as imperative in their healthcare fields.
The data highlights the importance of gender-specific insights into healthcare students' perceptions of AI management and how they view its significance within their fields. This nuanced analysis reveals the multifaceted nature of attitudes toward AI management, reinforcing the need for tailored educational approaches to ensure that all healthcare students, regardless of gender, are well-prepared for the evolving healthcare landscape. Further research may explore the factors contributing to these gender-based differences in AI management perception among healthcare students, ultimately enhancing the development of targeted educational strategies.
Overall, this examination of gender-based perspectives underscores the relevance of understanding how healthcare students perceive the imperative of cautious AI management, offering valuable insights for educational programs and the future healthcare workforce.
5.3 Gender-based perspectives on the impact of AI on career
In this subsection, we examine how healthcare students' gender influences their perceptions regarding the impact of artificial intelligence (AI) on their future careers. Participants were asked to rate this impact on a scale of 0–10, with 0 indicating very low impact and 10 representing very high impact. Responses were categorized into five groups: very low (0–3), low (4–5), moderate (6–7), high (8–9), and very high (10).
Figure 3 illustrates disparities in responses between male and female participants, as revealed by the data analysis. Among male respondents, 9% consider AI to have a very low impact on their careers (ratings 0–3), while 7.9% of female participants share a similar perspective. On the low impact scale (ratings 4–5), the difference is more pronounced, with 13.4% of male and 18.6% of female participants expressing this view.
In the moderate impact category (ratings 6–7), 15.7% of male and 20.2% of female participants believe AI will have a moderate impact on their careers. A higher proportion of female participants perceive AI's moderate impact.
Conversely, 23.1% of male participants and 16.2% of female participants regard AI as having a high impact on their careers (ratings 8–9), with a greater number of male participants attributing high importance to AI's role in their future careers.
A notable commonality between male and female participants is their belief in the very high impact of AI on their careers (rating of 10), with 38.8% of male and 37.2% of female participants sharing this perspective.
These variations in gender-based perceptions raise intriguing questions about the factors influencing the participants' views, such as differences in exposure to AI, educational backgrounds, or career aspirations. Bridging these perception gaps is essential to provide effective AI education that aligns with the diverse perspectives and expectations of healthcare students.
Notably, only one participant was assigned a rating of 0, indicating that AI will have no impact on their career. The gender distribution of this one participant is approximately equal, with three male and five female participants sharing this view. A closer examination reveals that five of these participants are aged 20 years or younger, suggesting age as a potential factor influencing this perception. Furthermore, 87.5% of these participants hold a high school-level education as their highest qualification, indicating a potential correlation between educational background and the perceived impact of AI on their careers.
The data also reveal that approximately 26.5% of female participants perceive very low or low impact (ratings 0-5) of AI on their careers, while 22.4% of male participants hold a similar belief. In contrast, a significant majority, comprising approximately 62% of male participants and approximately 53% of female participants, believe AI will have an important or very important impact on their careers. This significant gender gap in the perception of AI's impact on careers underscores the importance of considering gender-based variations in healthcare education.
When analyzing the responses without gender differentiation, some interesting trends emerge. Among respondents who rated the impact as low or very low (0–5), a significant 82% hold a high school-level educational qualification. Furthermore, 62.3% of participants in this category are 20 years or younger. Interestingly, 63% of students from University A rate the impact as low or very low.
An interesting finding is that 17% of participants who aim to “start their own practice/business in the future” perceive a low or very low impact of AI on their careers. Similarly, 19.32% of students aspiring to “do research as part of their career in the future” rank the impact as low or very low. Among those who aim to “focus on clinical work only,” 15% perceive low or very low impact.
These findings suggest a complex interplay of factors, including gender, educational background, career aspirations, and age, influencing healthcare students' perceptions of the impact of AI on their future careers. Addressing these perceptions in healthcare education is vital to prepare students effectively for the evolving landscape of AI in the field. Further research is needed to gain a deeper understanding of the multifaceted elements that shape these perspectives.
5.4 Gender-based variations in perceived importance of AI basics in healthcare education
In this subsection, we analyze gender-based differences in healthcare students' beliefs regarding the necessity of AI basics in their education and discuss the implications of these perspectives. Participants were asked to rate the importance of healthcare students having a fundamental understanding of AI, which was categorized into five groups: very low, low, medium, high, and very high.
Among female participants, 13.4% considered AI basics as having very low importance, while 18.2% found it to be of low importance. In comparison, a slightly lower percentage of male participants, 14.2% and 10.4%, held similar beliefs in the very low and low categories.
For the medium importance category, 13% of female participants and 14.9% of male participants regarded AI basics as moderately important. Interestingly, a higher proportion of female participants leaned toward this category.
The high importance category included 26.1% of female and 18.7% of male participants, indicating a more pronounced inclination among female participants toward considering AI basics as highly important.
In the very high importance category, 29.2% of female participants believed that healthcare students must have a deep understanding of AI basics, while a higher proportion of male participants, 41.8%, shared this view.
Notably, 5.93% of the total participants rated AI basics as having very low importance, suggesting that they do not perceive a significant need for basic AI knowledge in healthcare education. These respondents had an average impact on their career, rated at 4.83. Among this subgroup, 95.65% had their highest educational qualification listed as high school. When examining gender differences within this subgroup, 69.57% of female and 30.43% of male participants held the view that AI basics have very low importance.
The existence of this subgroup warrants further investigation into their rationale and the potential implications of their perspectives on the integration of AI basics into healthcare education. It may also inform the development of educational programs that address the diverse range of views within the healthcare student population.
To provide a visual representation of these gender-based perspectives, please refer to Figure 4 for the belief in healthcare students needing AI basics and Figure 5 for the gender ratio of healthcare students who believe AI basics understanding is not required. These figures offer a graphical overview of the variations in perceptions among healthcare students regarding AI basics.
5.5 Variations in perceptions of AI education necessity by program year
The necessity of AI education in healthcare varies by students' program year. Understanding these variations is essential for tailoring education strategies to meet students' evolving needs and perspectives. This section explores students' beliefs at different stages of their education, shedding light on AI education dynamics in healthcare.
5.5.1 Factors shaping pre-1 students' AI education beliefs
The diverse range of beliefs among Pre-Professional Year 1 (Pre-1) students regarding the importance of AI basics in healthcare education can be influenced by several factors.
First, 21.8% of Pre-1 students consider the need for AI basics to be “very low,” while 9.2% of students find it “low”. This may reflect a degree of skepticism or limited prioritization of AI education in this group.
Second, the presence of 21.8% of students who hold the belief that AI basics are of “medium” importance could be indicative of confusion or uncertainty. These students might be experiencing mixed feelings or a lack of clarity regarding the role of AI in healthcare, potentially benefiting from further clarification about the importance of AI basics.
Moreover, 16.1% of students in this group believe AI basics are “high,” demonstrating that some students have more balanced or nuanced views about the importance of AI knowledge in healthcare.
Finally, a significant 31% of Pre-1 students consider AI basics to be of “very high” importance, reflecting a substantial endorsement of AI knowledge within this junior program year.
These factors collectively shape the beliefs of Pre-1 students and underscore the importance of considering these variables in educational strategies and curriculum enhancements.
5.5.2 Factors shaping pre-2 students' AI education beliefs
On the other hand, in Pre-Professional Year 2 (Pre-2), students exhibit diverse beliefs concerning the importance of AI basics in healthcare education, categorized into five levels: very low, low, medium, high, and very high.
A substantial 31.5% of Pre-2 students perceive the need for AI basics as “very low,” reflecting a significant portion with skeptical viewpoints or limited prioritization of AI education.
Furthermore, 12.3% of students find AI basics “low,” indicating a group with reservations or those who perceive lower importance.
Moreover, 15.1% of Pre-2 students consider AI basics to be of “medium” importance, showing recognition of the relevance of AI knowledge in healthcare education.
Additionally, 20.5% of students believe AI basics are “high,” reflecting a substantial portion that endorses AI education.
An equal 20.5% of Pre-2 students consider AI basics to be of “very high” importance, indicating a strong endorsement of AI knowledge in healthcare.
The analysis of Pre-2 students' beliefs underscores the need for addressing skepticism, accommodating varied perspectives, and creating a comprehensive AI education strategy within this program year.
5.5.3 Factors shaping first year students' AI education beliefs
In the first year, students' beliefs regarding the necessity of AI basics in healthcare education vary across five levels: very low, low, medium, high, and very high.
Among first-year students, 26.7% consider the need for AI basics to be “very low,” 9.3% find it “low,” 22.7% consider it “medium,” 14.7% believe it is “high,” and 26.7% consider it “very high.”
One possible reason for these readings could be the varying exposure and awareness of AI in healthcare among first-year students. Those who rate it as “very low” or “low” may have limited exposure or understanding of AI's significance in healthcare. In contrast, those who rate it as “high” or “very high” may have a more informed perspective or recognize the growing importance of AI in healthcare education.
5.5.4 Factors shaping first year students' AI education beliefs
In the second year, students' beliefs regarding the necessity of AI basics in healthcare education span across five levels: very low, low, medium, high, and very high.
Among second-year students, 22.8% consider the need for AI basics to be “very low,” 14% find it “low,” 17.5% consider it “medium,” 17.5% believe it is “high,” and 28.1% consider it “very high”.
A noteworthy pattern emerges, where the combined percentage of “high” and “very high” (45.6%) is slightly lower than the combined percentage of “low,” “very low,” and “medium” (54.3%). This pattern, consistent with observations in previous years (year 1, Pre-1, and Pre-2), raises questions about the factors influencing these beliefs.
A possible reason for this pattern could be linked to the student's age, level of exposure, and prior experience with AI knowledge. Younger students in their early years of healthcare education might have limited exposure to AI concepts, potentially leading to undervaluing the importance of AI basics.
Additionally, misperceptions or a lack of accurate information about AI's role in healthcare might contribute to this pattern, as students may not fully comprehend the transformative potential of AI in healthcare education and practice.
The persistence of this pattern across multiple program years highlights the need for targeted educational initiatives to enhance AI awareness and knowledge among healthcare students. It also underscores the importance of providing clear and comprehensive information to help students make informed judgments about the necessity of AI basics in their education.
5.5.5 Factors shaping third year students' AI education beliefs
In the third year, students' beliefs regarding the necessity of AI basics in healthcare education encompass five levels: very low, low, medium, high, and very high. Among third-year students, 12.8% consider the need for AI basics to be “very low,” 10.3% find it “low,” 28.2% consider it “medium,” 20.5% believe it is “high,” and 28.2% consider it “very high”.
5.5.6 Factors shaping fourth year students' AI education beliefs
In the fourth year, students' beliefs follow the same five-level categorization. Among fourth-year students, 34.8% consider the need for AI basics to be “very low,” 13% find it “low,” 8.7% consider it “medium,” 13% believe it is “high,” and 30.4% consider it “very high”.
5.5.7 Factors shaping fifth year students' AI education beliefs
In the fifth year, medical students' beliefs span across the five levels. Among fifth-year students,33.3% consider the need for AI basics to be “very low,” 16.7% find it “low,” and interestingly, no students consider it “medium”; 16.7% believe it is “high” and 33.3% consider it “very high”.
5.5.7.1 Pattern analysis and tentative reason
A noticeable pattern in both the fourth- and fifth-year students is the absence of students rating AI basics as “medium”. This suggests that a significant proportion of these students have well-defined opinions, tending toward either “very low” or “very high”. This pattern may be indicative of these students having clear views and being less likely to remain in the “middle ground”.
In contrast, the third-year students demonstrate a more evenly distributed range of beliefs, including a notable percentage rating it as “medium”. This suggests that third-year students might be at a stage where they are considering various factors and have not yet formed extremely strong opinions.
Additionally, the prevalence of “very low” ratings in the fourth and fifth years could be attributed to a potential lack of awareness or a perception that AI basics are not necessary in their advanced stages of education.
Overall, this pattern may reflect a maturation of perspectives over the course of their education. While third-year students are still exploring the concept, fourth- and fifth-year students may have developed clearer, albeit polarized, views on the importance of AI basics in healthcare education. These findings highlight the importance of timely and comprehensive AI education and the need to address students' evolving perspectives.
5.5.8 Factors shaping sixth year and senior students' AI education beliefs
In the sixth year, students' beliefs regarding the necessity of AI basics in healthcare education encompass five levels: very low, low, medium, high, and very high. Among sixth-year students, 12.5% consider the need for AI basics to be “very low,” 12.5% find it “low,” 12.5% consider it “high,” and a significant 62.5% believe it is “very high.” Interestingly, there are no students in this group who consider AI basics to be of “medium” importance.
In senior students, beliefs regarding the necessity of AI basics follow the same five-level categorization. Among senior students, 15.4% consider the need for AI basics to be “very low,” 30.8% find it “low,” 7.7% consider it “high,” and 46.2% believe it is “very high”. Similar to the sixth-year students, there are no students in this group who consider AI basics to be of “medium” importance.
A distinctive pattern emerges across both the sixth-year and senior students, characterized by the absence of students rating AI basics as “medium.” This suggests that the majority of these students have clear, well-defined views, leaning either toward “very low,” “low,” “high,” or “very high.”
In the case of sixth-year students, the prevalence of “very high” ratings at 62.5% is particularly significant. This indicates a strong consensus among these students regarding the importance of AI basics in healthcare education, potentially influenced by their advanced stage of education and extensive exposure to healthcare practices.
For senior students, the slight variation in “low” ratings may be attributed to a smaller sample size or the nature of their current activities. It is possible that these senior students are engaged in post-graduate research or projects where the use of AI in healthcare may be less relevant, leading them to rate it as “low.” However, the majority of them still recognize the importance of AI basics, rating it as “very high.”
The absence of “medium” ratings in both groups indicates that they have well-considered opinions on the topic and are less likely to remain in the middle ground. These findings emphasize the significance of advanced education and practical experience in shaping students' perspectives on the role of AI basics in healthcare education.
5.6 Gender disparities in perceptions of the need for careful AI management
In the following lines, we analyze the data regarding the need for careful AI management by gender and discuss why there are notably higher responses in the “very low” and “very high” categories, while the “low” and “medium” categories have relatively fewer responses.
Among male participants, 35.1% consider the need for careful AI management to be “very low.” In contrast, 4.5% of male participants find it “low,” 9.0% rate it as “medium,” and 10.4% consider it “high.” A significant 41% of male participants believe it is “very high.”
For female participants, 22.5% consider the need for careful AI management to be “very low,” while 5.5% find it “low,” 6.3% rate it as “medium,” 11.9% consider it “high,” and a majority of 53.8% of female participants believe it is “very high.”
The data show a clear pattern where a majority of both male and female participants lean toward extreme positions, either “very low” or “very high,” when it comes to the need for careful AI management.
One potential reason for the higher responses in the “very low” category could be a perception among participants that AI management is not a significant concern in their view. They might feel that the current state of AI management in healthcare is adequate and does not require significant attention.
On the other hand, the substantial number of responses in the “very high” category may indicate a recognition of the potential risks and importance of careful AI management. Participants, particularly female, might emphasize the need for a robust and cautious approach to AI management in healthcare, given the sensitivity and potential impact of healthcare decisions.
The comparatively lower responses in the “low” and “medium” categories suggest that participants are less likely to hold a moderate view on this topic. It could be that they either perceive the issue as being of high importance or of little to no concern, leading to polarized responses.
These findings highlight the significance of addressing the varying levels of concern and recognition of the importance of AI management in healthcare, as well as the need for a nuanced approach to accommodating different perspectives.
5.7 Importance in healthcare studies by career goals
In this section, we delve into how healthcare students' perceptions of the importance of their studies vary based on their career goals. Participants were given the option to select up to three career goals from four available options: starting their own practice/business, pursuing research, focusing on clinical work, or pursuing academics.
In Figure 6, the options refer to participants' stated career goals. Option 1 corresponds to “I would like to start my own practice/business in the future,” Option 2 indicates “I would like to do research as part of my career in the future,” Option 3 represents “I want to focus on clinical work only,” and Option 4 pertains to “Academics”. The data reveals interesting patterns. Students who select only one career goal appear to have a clear vision of their future career path, which is reflected in their ratings of AI importance. For instance, those who aspire to start their own practice/business in future rate the importance of AI very high, with 28.9% considering it high and 43.4% considering it very high, as shown in Figure 6. This clarity in career goals corresponds to higher ratings for AI importance.
Additionally, it is worth noting that among participants who selected only one career goal, only 22.4% rated AI importance as very low or low. This suggests that students with a single career goal tend to recognize the significance of AI in their field of study.
Furthermore, a noteworthy trend emerges when considering the maximum rating of 10 for AI importance. A substantial 52.8% of participants who provided this maximum rating have only one career goal. This high percentage underscores the strong belief in the importance of AI education among students with a singular, well-defined career goal.
Intriguingly, when examining the participants who perceive AI importance as low or very low, it becomes apparent that female students are more prevalent in this category, constituting 65.52% of the total respondents. This gender-based variation raises questions about the factors influencing female students' perceptions of AI education.
These findings indicate the complex interplay between career goals, clarity of vision, and perceptions of AI importance. It suggests that students with a single, distinct career goal tend to emphasize the importance of AI education, while gender disparities also play a role in these perceptions. Further research could explore the underlying factors contributing to these variations in more detail.
5.8 Anticipated AI impact on career across program years
In this section, we explore how healthcare students across different program years perceive the timeline for AI's influence on their future careers. We have delved into their expectations over a spectrum of timeframes, spanning from 5 years into the future to a lifetime perspective. The information presented in the following sections provides a holistic understanding of how the changing landscape of AI technologies influences the career prospects of these students. This offers valuable insights into incorporating AI in healthcare education and practice.
5.9 Program year vs. expected AI impact on career
In this subsection, we explore the students' perceptions of when artificial intelligence (AI) will have an impact on their careers. Participants from various program years, including first to sixth Year, Senior, Pre-1, and Pre-2, provided insights into their expectations regarding the timeline for AI's influence on their professional lives.
5.9.1 Anticipated AI impact on career within 5 years
The data concerning students' expectations of how AI will impact their careers in the coming 5 years provides valuable insights into their perceptions across different program years.
First Year students express a moderate expectation of 28.0%, showcasing a realistic outlook on AI's imminent influence on their careers. In the second Year, this expectation slightly increases to 36.8%, reflecting a growing awareness and understanding of AI technologies.
Surprisingly, the third year students report a 30.8% expectation, indicating that they maintain a similar level of anticipation as the first year, potentially due to their specific career goals.
However, as we progress, a significant dip is observed in the fourth year, where the anticipation drops to 17.4%. This could be attributed to students focusing more on immediate career concerns.
In the fifth Year, there is a noticeable increase in the expectation of AI's influence at 33.3%. Students in this stage of their education might be gearing up for the transformation AI is poised to bring to healthcare.
The sixth year students show an expectation of 37.5%, possibly a reflection of their readiness to embrace AI technologies. However, the senior students are the most anticipatory at 61.5%. This is likely due to their extensive exposure, understanding, and awareness of the imminent integration of AI in healthcare.
The Pre-1 and Pre-2 students report expectations of 27.6% and 38.4%, respectively. This could be due to differences in the curriculum and exposure to AI concepts in the two pre-professional years.
This section illustrates how students' anticipation of AI's impact varies with their program year. Higher readings in most years indicate increased awareness, guidance, and an evolving understanding of AI's imminent role in healthcare education and practice, while the senior students show the highest values due to their extensive exposure and experience in the field.
5.9.2 Anticipated AI impact on career within 10 years
The data, organized by the expected timeframe, reveal interesting trends. In the shortest span of “In 10 Years,” a significant majority of students anticipate that AI will impact their careers within this timeframe. This is indicative of the rapid evolution of AI technologies and their integration into healthcare practices. The breakdown by program year is as follows:
1st Year: 54.7%, 2nd Year: 52.6%, 3rd Year: 53.8%, 4th Year: 60.9%, 5th Year: 50%, 6th Year: 37.5%, Senior: 30.8%, Pre-1: 55.2%, and Pre-2: 46.6%
Notably, fourth year students exhibit the highest anticipation, with 60.9% believing AI will impact their careers within a decade. It is important to recognize that these students are closer to entering the workforce and may be more aware of the ongoing developments in healthcare technology.
In contrast, sixth year and senior students express significantly lower expectations, with 37.5% and 30.8%, respectively, believing AI will influence their careers within 10 years. This divergence may reflect a sense of immediacy among the younger program years and, conversely, a recognition by sixth-year and senior students that AI's impact is not as distant as a 10-year horizon suggests.
There are several reasons behind these expectations. In a healthcare context, the rapid growth of the internet of things (IoT) plays a pivotal role. Healthcare professionals are increasingly utilizing IoT devices and sensors that provide real-time patient data. For instance, sensors measuring parameters such as blood pressure, heart rate, and blood oxygen levels are now common in both clinical and home settings. These sensors, along with AI, enable automated monitoring, early detection of health issues, and timely interventions.
Furthermore, researchers are continuously developing solutions that leverage IoT and AI to automate medication administration, connect hospitals and emergency medical staff through real-time data sharing, and provide immediate alerts to family members or remote personal doctors in case of health emergencies. Additionally, IoT technology facilitates the use of implanted sensors for basic bodily functions, enabling a deeper level of healthcare monitoring and intervention.
The diverse perceptions across program years illustrate the dynamic nature of AI's role in healthcare education and practice. It highlights the importance of addressing these expectations in curriculum design and educational strategies.
The visual representation of this data is available in Figure 7, offering an overview of students' beliefs regarding AI's influence on their future careers.
5.9.3 Anticipated AI impact on career within 20 years
When considering the expectations regarding how AI will impact their careers within the next 20 years, the data reveal an interesting perspective across different program years.
First year students show a modest expectation of 14.7%, suggesting that they perceive the impact of AI as a gradual and long-term transformation in the healthcare field.
Similarly, second year students express an expectation of 8.8%, indicating a somewhat reserved outlook on the speed of AI integration in healthcare.
In the third year, there is a slightly higher expectation at 12.8%, reflecting a somewhat increased awareness and anticipation of AI's role in the coming two decades.
The fourth year students show a 17.4% expectation, potentially indicating their preparation to adapt to AI-driven changes in healthcare.
Similarly, the fifth year students display a 16.7% expectation, suggesting a readiness to embrace AI technologies in their future careers.
Notably, the sixth year students stand out with a high expectation of 25%. This surprising figure might be attributed to various factors, including confusion among some students about the timespan involved or a divergence in their perceptions of AI's importance and significance.
In contrast, senior students show the lowest expectation within this 20-year timeframe at 7.7%. It is essential to recognize that the number of senior students and research-oriented students in the participant pool is comparatively smaller, which could account for this lower value.
The Pre-1 and Pre-2 students report expectations of 12.6% and 11%, respectively, aligning with the relatively modest anticipations within this category.
Overall, the data indicate that while the expectations about AI's impact within 20 years are on the lower side, they reflect the students' diverse perceptions and readiness to adapt to AI-driven changes in the healthcare industry.
5.10 Preferred AI learning duration vs. importance in healthcare studies
In this section, we explore the relationship between participants' preferred AI learning durations and their perceived importance of AI in healthcare studies. The options for preferred AI learning duration are explained in Figure 8 as follows:
• Option 1: One Day Course
• Option 2: Two-hour Workshop
• Option 3: Multiple Workshop Series
• Option 4: Graduate-level Education (Master's, PhD)
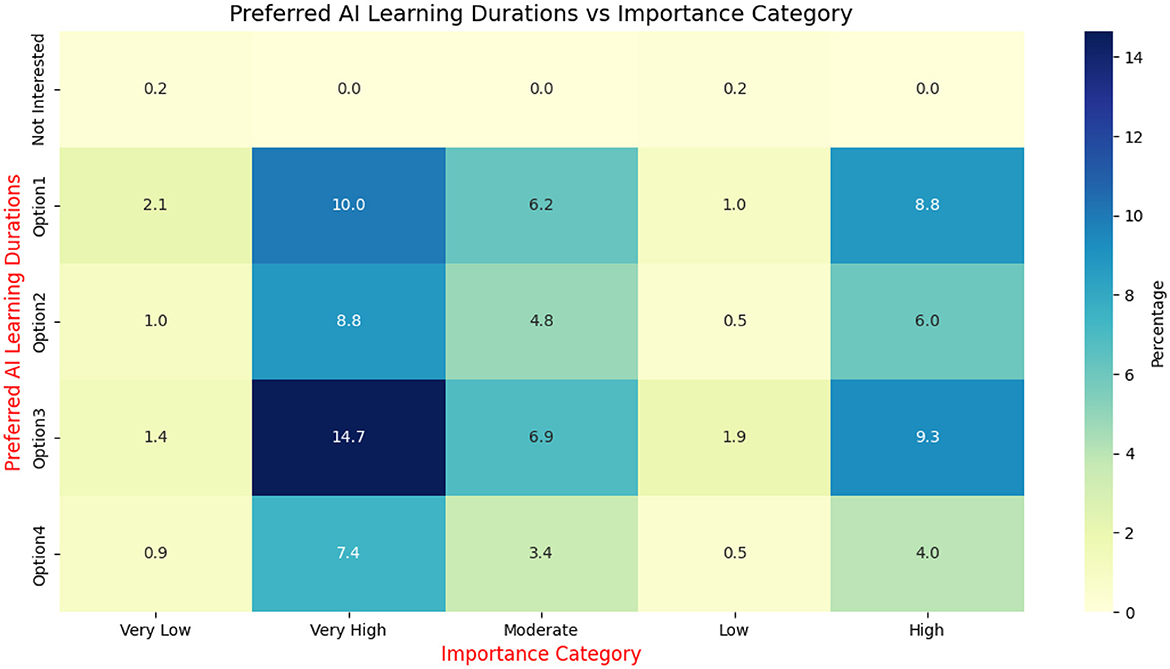
Figure 8. Heatmap illustrating the relationship between preferred AI learning duration and importance of AI in healthcare studies.
The heat map presents an interesting comparison, revealing insights into the participants' choices and preferences. Here are some observations:
The results indicate that a majority of students who prefer very high importance in AI also prefer multiple workshop series for understanding AI concepts, constituting 14.7% of the total participants. Additionally, 10% of participants believe that a 1-day course is sufficient for grasping the basics of AI. This finding is intriguing and may be attributed to participants either underestimating the complexity of AI concepts or desiring only fundamental knowledge.
Similar patterns emerge for participants who consider AI to be of high importance. Notably, there are very few participants who believe that, regardless of the learning duration, AI is of low or very low importance. The majority in this category also favors multiple workshop series, reflecting a consistent preference for extended learning formats.
In the moderate importance category, entries are significantly higher, suggesting potential confusion or uncertainty among participants regarding the optimal learning period for understanding AI. Despite this uncertainty, a substantial number of participants prefer multiple workshop series, indicating a consistent trend across importance levels.
Surprisingly, even in the low- and very-low-importance categories, there are participants who express interest in AI learning. This could be driven by factors such as curiosity or a desire for a foundational understanding despite their perceived lower importance of AI in their careers.
These observations highlight the complex interplay between preferred AI learning duration and participants' perceptions of AI importance in healthcare studies. The nuanced patterns observed underscore the need for tailored educational approaches that consider participants' preferences and align with their perceived importance of AI in their respective fields.
6 Conclusion
As artificial intelligence (AI) reshapes the healthcare landscape, our study pioneers an exploration of Saudi healthcare students' attitudes toward its integration into their education. Spanning 21 universities and engaging participants from diverse healthcare disciplines, this nationwide survey provides nuanced insights into the multifaceted perceptions that shape the future of AI integration in healthcare education and practice in KSA.
Our study identified and bridged a critical gap in the existing literature by focusing on the perceptions of healthcare students in KSA, a region where the transformative impact of AI on medical education had not been comprehensively explored. While previous research extensively covered various countries, our inclusive methodology distinguished our study by encompassing diverse healthcare disciplines and students across all academic years.
The findings shed light on gender-based differences in perceptions, emphasizing the recognized importance of AI in healthcare careers. Notably, our study revealed a general consensus on the necessity of AI in healthcare curricula, underlining its role in shaping future healthcare professionals. However, the study also emphasized the need for cautious AI management in clinical settings, demonstrating the complex interplay of factors that influence students' attitudes.
The results of our comprehensive statistical analysis further underscored the variations in perceptions across different demographic factors, program years, and career goals. This granular understanding of the diverse perspectives of healthcare students on AI sets the stage for tailoring effective educational strategies that align with the unique needs of the KSA healthcare education landscape.
As we conclude, the implications of this study extend beyond academic discourse. Our research provides actionable insights for educators, policymakers, and healthcare institutions, offering a foundation for the strategic integration of AI into healthcare curricula. By understanding the intricate web of perceptions held by future healthcare professionals, we contribute to the preparation of a workforce capable of navigating the AI-integrated future of healthcare in the Kingdom of Saudi Arabia.
Data availability statement
The raw data supporting the conclusions of this article will be made available by the authors, without undue reservation.
Ethics statement
Ethical review and approval was not required for the study on human participants in accordance with the local legislation and institutional requirements. Written informed consent from the participants was not required to participate in this study in accordance with the national legislation and the institutional requirements.
Author contributions
AA: Conceptualization, Funding acquisition, Supervision, Writing – original draft. AS: Data curation, Formal analysis, Writing – original draft, Writing – review & editing. RA: Data curation, Supervision, Validation, Writing – review & editing, Resources. OA: Project administration, Resources, Software, Visualization, Writing – review & editing.
Funding
The author(s) declare that no financial support was received for the research and/or publication of this article.
Conflict of interest
The authors declare that the research was conducted in the absence of any commercial or financial relationships that could be construed as a potential conflict of interest.
Generative AI statement
The author(s) declare that no Gen AI was used in the creation of this manuscript.
Publisher's note
All claims expressed in this article are solely those of the authors and do not necessarily represent those of their affiliated organizations, or those of the publisher, the editors and the reviewers. Any product that may be evaluated in this article, or claim that may be made by its manufacturer, is not guaranteed or endorsed by the publisher.
References
Alowais, S. A., Alghamdi, S. S., Alsuhebany, N., Alqahtani, T., Alshaya, A. I., Almohareb, S. N., et al. (2023). Revolutionizing healthcare: the role of artificial intelligence in clinical practice. BMC Med. Educ. 23:689. doi: 10.1186/s12909-023-04698-z
Bhaskar, S., Bradley, S., Sakhamuri, S., Moguilner, S., Chattu, V. K., Pandya, S., et al. (2020). Designing futuristic telemedicine using artificial intelligence and robotics in the covid-19 era. Front. Public Health 708:556789. doi: 10.3389/fpubh.2020.556789
Bisdas, S., Topriceanu, C.-C., Zakrzewska, Z., Irimia, A.-V., Shakallis, L., Subhash, J., et al. (2021). Artificial intelligence in medicine: a multinational multi-center survey on the medical and dental students' perception. Front. Public Health 9:795284. doi: 10.3389/fpubh.2021.795284
Boillat, T., Nawaz, F. A., and Rivas, H. (2022). Readiness to embrace artificial intelligence among medical doctors and students: questionnaire-based study. JMIR Med. Educ. 8:e34973. doi: 10.2196/34973
Buabbas, A. J., Miskin, B., Alnaqi, A. A., Ayed, A. K., Shehab, A. A., Syed-Abdul, S., et al. (2023). Investigating students' perceptions towards artificial intelligence in medical education. Healthcare 11:1298. doi: 10.3390/healthcare11091298
Busch, F., Hoffmann, L., Truhn, D., Ortiz-Prado, E., Makowski, M. R., Bressem, K. K., et al. (2023a). Medical students' perceptions towards artificial intelligence in education and practice: a multinational, multicenter cross-sectional study. medRxiv, 2023–12. doi: 10.1101/2023.12.09.23299744
Busch, F., Hoffmann, L., Truhn, D., Palaian, S., Alomar, M., Shpati, K., et al. (2023b). International pharmacy students' perceptions towards artificial intelligence in medicine—a multinational, multicentre cross-sectional study. Br. J. Clini. Pharmacol. 90, 649–661. doi: 10.1111/bcp.15911
Cadamuro, J. (2021). Rise of the machines: The inevitable evolution of medicine and medical laboratories intertwining with artificial intelligence—a narrative review. Diagnostics 11:1399. doi: 10.3390/diagnostics11081399
Castagno, S., and Khalifa, M. (2020). Perceptions of artificial intelligence among healthcare staff: a qualitative survey study. Front. Artif. Intellig. 3:578983. doi: 10.3389/frai.2020.578983
Cellina, M., Cè, M., Irmici, G., Ascenti, V., Caloro, E., Bianchi, L., et al. (2022). Artificial intelligence in emergency radiology: where are we going? Diagnostics 12:3223. doi: 10.3390/diagnostics12123223
Chan, C. K. Y., and Hu, W. (2023). Students' voices on generative AI: perceptions, benefits, and challenges in higher education. arXiv [preprint] arXiv:2305.00290. doi: 10.1186/s41239-023-00411-8
Chassignol, M., Khoroshavin, A., Klimova, A., and Bilyatdinova, A. (2018). Artificial intelligence trends in education: a narrative overview. Procedia Comput. Sci. 136, 16–24. doi: 10.1016/j.procs.2018.08.233
Chen, L., Chen, P., and Lin, Z. (2020). Artificial intelligence in education: a review. IEEE Access 8, 75264–75278. doi: 10.1109/ACCESS.2020.2988510
Choi, D.-J., Park, J. J., Ali, T., and Lee, S. (2020). Artificial intelligence for the diagnosis of heart failure. NPJ Digit. Med. 3:54. doi: 10.1038/s41746-020-0261-3
Civaner, M. M., Uncu, Y., Bulut, F., Chalil, E. G., and Tatli, A. (2022). Artificial intelligence in medical education: a cross-sectional needs assessment. BMC Med. Educ. 22:772. doi: 10.1186/s12909-022-03852-3
Dave, D., Naik, H., Singhal, S., and Patel, P. (2020). Explainable ai meets healthcare: a study on heart disease dataset. arXiv [preprint] arXiv:2011.03195. doi: 10.48550/arXiv.2011.03195
Edison, G. (2023). The integration of ai in the doctor's toolkit: Enhancing medical decision-making. Bullet: Jurnal Multidisiplin Ilmu 2, 604–613.
Esmaeilzadeh, P. (2020). Use of ai-based tools for healthcare purposes: a survey study from consumers' perspectives. BMC Med. Inform. Decis. Mak. 20, 1–19. doi: 10.1186/s12911-020-01191-1
Esteva, A., Kuprel, B., Novoa, R. A., Ko, J., Swetter, S. M., Blau, H. M., et al. (2017). Dermatologist-level classification of skin cancer with deep neural networks. Nature 542, 115–118. doi: 10.1038/nature21056
Ezzaim, A., Kharroubi, F., Dahbi, A., Aqqal, A., Haidine, A., et al. (2022). Artificial intelligence in education-state of the art. Int. J. Comp. Eng. Data Sci. (IJCEDS) 2:2.
Galán, G. C., and Portero, F. S. (2022). Medical students' perceptions of the impact of artificial intelligence in radiology. Radiología 64, 516–524. doi: 10.1016/j.rxeng.2021.03.008
Getson, C., and Nejat, G. (2021). Socially assistive robots helping older adults through the pandemic and life after COVID-19. Robotics 10:106. doi: 10.3390/robotics10030106
Gillissen, A., Kochanek, T., Zupanic, M., and Ehlers, J. (2022). Medical students' perceptions towards digitization and artificial intelligence: a mixed-methods study. Healthcare 10:723. doi: 10.3390/healthcare10040723
Golec, M., Gill, S. S., Parlikad, A. K., and Uhlig, S. (2023). Healthfaas: Ai based smart healthcare system for heart patients using serverless computing. IEEE Internet Things J. 1, 18469–18476. doi: 10.1109/JIOT.2023.3277500
Guze, P. A. (2015). Using technology to meet the challenges of medical education. Trans. Am. Clin. Climatol. Assoc. 126:260.
Han, C., Rundo, L., Murao, K., Nemoto, T., and Nakayama, H. (2020). “Bridging the gap between AI and healthcare sides: towards developing clinically relevant ai-powered diagnosis systems,” in Artificial Intelligence Applications and Innovations: 16th IFIP WG 12.5 International Conference, AIAI 2020 (Neos Marmaras: Springer), 320–333.
Hardiker, N. R., and Grant, M. J. (2011). Factors that influence public engagement with ehealth: a literature review. Int. J. Med. Inform. 80, 1–12. doi: 10.1016/j.ijmedinf.2010.10.017
Hassan, N., Aazam, M., Tahir, M., and Yau, K.-L. A. (2023). Floating fog: extending fog computing to vast waters for aerial users. Cluster Comput. 26, 181–195. doi: 10.1007/s10586-022-03567-6
Johnson, M., Albizri, A., and Simsek, S. (2022). Artificial intelligence in healthcare operations to enhance treatment outcomes: a framework to predict lung cancer prognosis. Ann. Operat. Res. 308, 275–305. doi: 10.1007/s10479-020-03872-6
Jones, K. F., Paal, P., Symons, X., and Best, M. C. (2021). The content, teaching methods and effectiveness of spiritual care training for healthcare professionals: a mixed-methods systematic review. J. Pain Symptom Manage. 62, e261–e278. doi: 10.1016/j.jpainsymman.2021.03.013
Keshri, S., Kumar, R., Kumar, D., Singhal, T., Giri, S., Sharma, I., et al. (2022). Insights of artificial intelligence in brain disorder with evidence of opportunity and future challenges. J. Pharmaceut. Negat. Results 13, 10853–10867.
Kumar, P., Chauhan, S., and Awasthi, L. K. (2023). Artificial intelligence in healthcare: review, ethics, trust challenges & future research directions. Eng. Appl. Artif. Intell. 120:105894. doi: 10.1016/j.engappai.2023.105894
Lee, J., Wu, A. S., Li, D., and Kulasegaram, K. M. (2021). Artificial intelligence in undergraduate medical education: a scoping review. Acad. Med. 96, S62–S70. doi: 10.1097/ACM.0000000000004291
Li, D., Pehrson, L. M., Lauridsen, C. A., Tøttrup, L., Fraccaro, M., Elliott, D., et al. (2021). The added effect of artificial intelligence on physicians' performance in detecting thoracic pathologies on ct and chest x-ray: a systematic review. Diagnostics 11:2206. doi: 10.3390/diagnostics11122206
Li, Q., and Qin, Y. (2023). Ai in medical education: medical student perception, curriculum recommendations and design suggestions. BMC Med. Educ. 23:852. doi: 10.1186/s12909-023-04700-8
Li, X., Jiang, M. Y.-c., Jong, M. S.-y., Zhang, X., and Chai, C.-s. (2022). Understanding medical students' perceptions of and behavioral intentions toward learning artificial intelligence: a survey study. Int. J. Environ. Res. Public Health 19:8733. doi: 10.3390/ijerph19148733
Liu, D. S., Sawyer, J., Luna, A., Aoun, J., Wang, J., Boachie, L., et al. (2022). Perceptions of us medical students on artificial intelligence in medicine: mixed methods survey study. JMIR Med. Educ. 8:e38325. doi: 10.2196/38325
Masters, K. (2019). Artificial intelligence in medical education. Med. Teach. 41, 976–980. doi: 10.1080/0142159X.2019.1595557
Muscogiuri, G., Volpato, V., Cau, R., Chiesa, M., Saba, L., Guglielmo, M., et al. (2022). Application of ai in cardiovascular multimodality imaging. Heliyon. 8:e10872. doi: 10.1016/j.heliyon.2022.e10872
Naghshvarianjahromi, M., Majumder, S., Kumar, S., Naghshvarianjahromi, N., and Deen, M. J. (2021). Natural brain-inspired intelligence for screening in healthcare applications. IEEE Access 9, 67957–67973. doi: 10.1109/ACCESS.2021.3077529
Nikiforova, T., Carter, A., Yecies, E., and Spagnoletti, C. L. (2021). Best practices for survey use in medical education: how to design, refine, and administer high-quality surveys. South. Med. J. 114, 567–571. doi: 10.14423/SMJ.0000000000001292
Obenza, B. N., Salvahan, A., Rios, A. N., Solo, A., Alburo, R. A., and Gabila, R. J. (2024). University students' perception and use of ChatGPT: Generative artificial intelligence (AI) in higher education. Int. J. Hum. Comput. Stud. 5, 5–18.
Ouyang, F., Zheng, L., and Jiao, P. (2022). Artificial intelligence in online higher education: a systematic review of empirical research from 2011 to 2020. Educ. Inform. Technol. 27, 7893–7925. doi: 10.1007/s10639-022-10925-9
Piccialli, F., Di Cola, V. S., Giampaolo, F., and Cuomo, S. (2021). The role of artificial intelligence in fighting the COVID-19 pandemic. Inform. Syst. Front. 23, 1467–1497. doi: 10.1007/s10796-021-10131-x
Pierre, K., Gupta, M., Raviprasad, A., Sadat Razavi, S. M., Patel, A., Peters, K., et al. (2023). Medical imaging and multimodal artificial intelligence models for streamlining and enhancing cancer care: opportunities and challenges. Expert Rev. Anticancer Ther. 23, 1265–1279. doi: 10.1080/14737140.2023.2286001
Rajpurkar, P., Chen, E., Banerjee, O., and Topol, E. J. (2022). AI in health and medicine. Nat. Med. 28, 31–38. doi: 10.1038/s41591-021-01614-0
Rezvantalab, A., Safigholi, H., and Karimijeshni, S. (2018). Dermatologist level dermoscopy skin cancer classification using different deep learning convolutional neural networks algorithms. arXiv [preprint] arXiv:1810.10348. doi: 10.48550/arXiv.1810.10348
Rodriguez-Rodriguez, I., Rodriguez, J.-V., Shirvanizadeh, N., Ortiz, A., and Pardo-Quiles, D.-J. (2021). Applications of artificial intelligence, machine learning, big data and the internet of things to the covid-19 pandemic: A scientometric review using text mining. Int. J. Environ. Res. Public Health 18:8578. doi: 10.3390/ijerph18168578
Sallam, M., Salim, N. A., Barakat, M., Al-Mahzoum, K., Ala'a, B., Malaeb, D., et al. (2023). Assessing health students' attitudes and usage of chatgpt in jordan: validation study. JMIR Medical Education 9:e48254. doi: 10.2196/48254
Sarwar, S., Dent, A., Faust, K., Richer, M., Djuric, U., Van Ommeren, R., et al. (2019). Physician perspectives on integration of artificial intelligence into diagnostic pathology. NPJ digit. Med. 2:28. doi: 10.1038/s41746-019-0106-0
Sharma, P., Suehling, M., Flohr, T., and Comaniciu, D. (2020). Artificial intelligence in diagnostic imaging: status quo, challenges, and future opportunities. J. Thorac. Imaging 35, S11–S16. doi: 10.1097/RTI.0000000000000499
Sit, C., Srinivasan, R., Amlani, A., Muthuswamy, K., Azam, A., Monzon, L., et al. (2020). Attitudes and perceptions of uk medical students towards artificial intelligence and radiology: a multicentre survey. Insights Imag. 11, 1–6. doi: 10.1186/s13244-019-0830-7
Sun, L., Yin, C., Xu, Q., and Zhao, W. (2023). Artificial intelligence for healthcare and medical education: a systematic review. Am. J. Transl. Res. 15:4820.
Teng, M., Singla, R., Yau, O., Lamoureux, D., Gupta, A., Hu, Z., et al. (2022). Health care students' perspectives on artificial intelligence: countrywide survey in canada. JMIR Med. Educ. 8:e33390. doi: 10.2196/33390
Vinokurtseva, A., and Veettil, S. (2020). Fake brain helping human brain: Artificial intelligence in mental healthcare. Univ. West. Ont. Med. J. 88, 25–27. doi: 10.5206/uwomj.v88i2.7304
Wang, Q., Xu, L., Wang, L., Yang, X., Sun, Y., Yang, B., et al. (2023). Automatic coronary artery segmentation of ccta images using unet with a local contextual transformer. Front. Physiol. 14:1138257. doi: 10.3389/fphys.2023.1138257
Wang, X. V., and Wang, L. (2021). A literature survey of the robotic technologies during the covid-19 pandemic. J. Manuf. Syst. 60, 823–836. doi: 10.1016/j.jmsy.2021.02.005
Winkel, D. J., Suryanarayana, V. R., Ali, A. M., Görich, J., Buß, S. J., Mendoza, A., et al. (2022). Deep learning for vessel-specific coronary artery calcium scoring: validation on a multi-centre dataset. Eur. Heart J.-Cardiovasc. Imag. 23, 846–854. doi: 10.1093/ehjci/jeab119
Keywords: teaching, learning, healthcare, data science applications in education, lifelong learning, artificial intelligence, medical
Citation: Almusharraf A, Shaikh AK, Attar RW and Alwassil O (2025) Perceptions of artificial intelligence in healthcare curricula: insights from a nationwide survey of medical students. Front. Educ. 10:1550671. doi: 10.3389/feduc.2025.1550671
Received: 15 January 2025; Accepted: 14 April 2025;
Published: 09 May 2025.
Edited by:
Chayanika Uniyal, University of Delhi, IndiaReviewed by:
Hemant Beniwal, Sawai ManSingh Medical College, IndiaUdit Choudhary, Mahatma Gandhi Institute of Medical Sciences, India
Copyright © 2025 Almusharraf, Shaikh, Attar and Alwassil. This is an open-access article distributed under the terms of the Creative Commons Attribution License (CC BY). The use, distribution or reproduction in other forums is permitted, provided the original author(s) and the copyright owner(s) are credited and that the original publication in this journal is cited, in accordance with accepted academic practice. No use, distribution or reproduction is permitted which does not comply with these terms.
*Correspondence: Abdul Khalique Shaikh, c2hhaWtoQHNxdS5lZHUub20=