- 1Universidad Privada del Norte, Facultad de Ciencias de la Salud, Lima, Peru
- 2Universidad Autónoma de Madrid, Madrid, Spain
- 3Universidad Tecnológica del Perú, Lima, Peru
- 4Universidad Peruana de Ciencia Aplicadas, Lima, Peru
Problem-solving is becoming more and more seen as an important skill for college students to learn to build metacognitive skills, critical thought, and the ability to learn on their own. Even though this skill is very important, there aren’t many approved tools that can be used to test it in schools, especially in Peru. The goal of this study is to fill in that gap by creating and testing a short problem-solving scale based on the Rational Problem-Solving Style, which stresses taking a planned and organized approach to problems. 733 Peruvian college students (mean age: 21.56 years, standard deviation: 4.15 years; 59.89% female) took part. A 15-item Problem-Solving Questionnaire and used experimental (EFA) and confirmatory factor analysis (CFA) to test it. The scale’s validity and reliability were checked, along with its link to academic self-efficacy. There were four parts to the Problem-Solving Questionnaire: Solution Analysis and Planning, Critical Evaluation of Solutions, Generation and Evaluation of Alternatives, and Prioritization and Review of Alternatives. Fit scores from CFA (like CFI = 0.98 and RMSEA = 0.062) and reliability coefficients (ω = 0.73–0.90) showed that it was a reliable educational tool. There was proof of concept validity in the form of correlations with academic self-efficacy (r = 0.36–0.80). The scale is a validity and effective way to test the problem-solving skills of university students in Peru. Due to its brevity and emphasis on logical methods, it is suitable for use in both education and research, aligning with global goals for quality education.
1 Introduction
In recent decades, problem-solving has emerged as a crucial ability in higher education, driven by the growing need for workers capable of addressing the problems of a worldwide environment (Castellanos and Rojas, 2023). This ability is intricately associated with the enhancement of metacognitive skills, which are crucial for independent learning and self-regulation (Covarrubias-Apablaza et al., 2019; Guamán-Ledesma and Rivera, 2024). However, there is still a lack of adequate instruments to assess this competency within current educational contexts (Ilbay, 2024). Lack of such tools hinders attempts to match educational practices with worldwide goals like Sustainable Development Goal 4 (SDG 4), which stresses inclusive and fair quality education and lifelong learning possibilities for everyone (Dastyari and Jose, 2024).
Although the Ministry of Education has pushed active approaches that support critical thinking and creativity by means of problem-solving in Peru, there is still needed to create and validate a particular instrument to gauge this competency in higher education (Velázquez-Tejeda and Goñi Cruz, 2024). Closing this gap is key to developing skills that improve academic performance and employability, promoting innovation and equity in education (Haxhiu, 2023). This underscores the pressing need for instruments to address global inequalities in educational results, especially in marginalized areas (Mavangere et al., 2022).
D’Zurilla and Goldfried (1971) developed the concept of problem-solving as then understood in psychology as a self-directed cognitive-behavioral process wherein people try to find and create workable answers to specific daily problems. Seeing it as a skill that can be developed, the authors presented the Social Problem-Solving Model, a methodological framework for analyzing problem-solving in daily life (D’Zurilla and Maydeu-Olivares, 1995; Maydeu-Olivares and D’Zurilla, 1996). This paradigm is founded on three essential concepts: issue-solving, problem, and solution (D’Zurilla et al., 2004). An issue is characterized as a circumstance where an adaptive reaction is not readily evident, necessitating the use of a problem-solving procedure. Conversely, a solution is the result of this process a reaction that ameliorates the problematic circumstance or mitigates related discomfort (D’Zurilla and Maydeu-Olivares, 1995). For the purposes of this study, problem-solving is defined as the deliberate, methodical, and logical process by which individuals identify, analyze, and address problematic situations, generating and evaluating alternative solutions to implement effective strategies.
Later, D’Zurilla and Nezu (1982) refined and expanded this model, indicating that the capacity for social problem-solving is not a unitary construct but rather comprises two general dimensions: (a) problem orientation, referring to a metacognitive process that reflects an individual’s beliefs, attitudes, and emotions about life problems and their ability to solve them; and (b) problem-solving skills (later called problem-solving styles), which refer to the cognitive and behavioral activities that enable a person to understand a problem and find effective solutions (Chang et al., 2004). Within this framework, four main skills were identified: problem definition and formulation; generation of solution alternatives; decision-making; and solution implementation and verification (D’Zurilla and Goldfried, 1971). However, this categorization of dimensions has evolved over the years as measurement studies have developed.
Based on this theoretical model, D’Zurilla and Nezu (1990) developed a preliminary version of the Social Problem-Solving Inventory (SPSI), containing 70 items, which included the Problem Orientation Scale (POS) and the Problem-Solving Skills Scale (PSSS). These scales demonstrated adequate reliability across their factors. For instance, POS consisted of three factors: cognition (α = 0.74), emotion (α = 0.90), and behavior (α = 0.86), while PSSS included four factors: problem definition (α = 0.85), generation of alternatives (α = 0.78), decision-making (α = 0.75), and solution implementation and verification (α = 0.65). Maydeu-Olivares and D’Zurilla (1996) later created a revised version (SPSI-R) based on exploratory and confirmatory component analysis results showing a five-factor model fit enough (RMSEA = 0.048; RMSR = 0.060). Factor analysis uncovered five elements: (1) positive issue orientation; (2) negative problem orientation; (3) logical problem-solving approach; (4) impulsive/careless style; and (5) avoidant style. Ultimately, the writers pointed out that these findings have notable consequences for theory and the evaluation of social problem-solving as they provide a more thorough knowledge of its fundamental aspects.
For the development of the problem-solving scale proposed in this article, the focus was exclusively placed on the Rational Problem-Solving Style. D’Zurilla and Goldfried (1971) concept of problem-solving which they characterize as a deliberate, aware, logical, effortful activity basis this option. Considered as a constructive approach, rational problem-solving is therefore described as the deliberate, methodical, and logical use of successful abilities to address difficulties (D’Zurilla et al., 2004). The rational style’s incorporation of the four fundamental skills of the theoretical model problem description and formulation; generation of solution alternatives; decision-making; and solution implementation and verification D’Zurilla and Goldfried, 1971, helps one to choose to concentrate the scale on the rational style. Earlier studies also classified these skills under the rational style (D’Zurilla and Nezu, 1990; Maydeu-Olivares and D’Zurilla, 1996).
One must define each one if one wants to fully appreciate the degree of these abilities. In this sense, the process of addressing issues depends critically on the capacity to identify and analyze problems, create and assess alternative solutions, make wise judgments, and check the implementation process. This methodical technique guarantees a logical and methodical strategy of handling problems, which corresponds with the theoretical framework suggested by D’Zurilla and Goldfried (1971). This kind of organization of problem-solving techniques guarantees a consistent and useful evaluation instrument relevant in many educational environments.
In this regard, rational problem-solving is linked to the student’s ability to reflect on their processes and apply metacognitive strategies, enhancing academic performance and enabling successful coping with challenges (Astuhuaman and Cristóbal, 2021). Additionally, this constructive style is associated with the development of critical and creative skills, which are essential both in academia and in everyday life (Makoviichuk et al., 2020). By fostering these competencies, educational institutions can help reduce dropout rates and improve equity in learning outcomes, aligning with SDG 4 and broader education goals (Albert et al., 2023). Furthermore, these efforts can support systemic reforms aimed at addressing educational inequities in marginalized communities worldwide (Meng, 2024).
Historically, there have been several instruments that measure problem-solving; a notable example is the Problem-Solving Scale (SPSI, D’Zurilla et al., 1999). The original version contained 70 items that were analyzed with exploratory and confirmatory factor analysis among university students to determine its internal structure. Its validity concerning other variables was also examined, and its reliability was demonstrated through internal consistency and test–retest methods. Results indicated that the two theoretically proposed dimensions had moderate support using confirmatory factor analysis and showed values from 0.83 to 0.88 and 0.92–0.94 in test–retest and internal consistency, respectively. Additionally, D’Zurilla et al. (1999) demonstrated that the inventory scores for each dimension correlated with the Problem-Solving Scale and variables such as stress, anxiety, depression, hopelessness, suicidality, life satisfaction, self-esteem, extraversion, social adjustment, and social skills.
Based on this version, a 25-item version (SPSI-S) was proposed, which showed a five-factor structure in the university population, as the goodness-of-fit index values were optimal and reliability by internal consistency showed values from 0.74 to 0.89. Every element, thus, connected to sadness, anxiety, despair, suicidality, and life satisfaction (D’Zurilla et al., 1999). Results were comparable in the official Spanish translation version because ranging from 0.68 to 0.83 (Maydeu-Olivares et al., 2000) revealed appropriate levels of dependability and excellent fit indices.
Although these tools exist, it is essential to create a new one as their simplicity will help them to be used in environments with little time and minimize participant tiredness (Rammstedt and Beierlein, 2014). Furthermore, important is its possibility to increase measurement and assessment efficiency without sacrificing validity and dependability (Kemper et al., 2019). Thus, in addition to being succinct, this tool is meant to catch the main traits of problem-solving. This makes it ideal for research that requires shorter, more accurate assessments in university settings, as students frequently face situations requiring problem-solving skills both academically and in their everyday lives.
The need for a problem-solving measurement tool in a higher education context lies in the ability to assess these competencies, which are critical to the learning process (Sotomayor and Águila, 2022). Additionally, having an instrument that accurately measures this phenomenon enables educators, counselors, pedagogues, and educational psychologists to effectively assess this skill in their students, providing valuable information that can guide decision-making and curriculum improvement (Maydeu-Olivares et al., 2000). Furthermore, having a tool adapted to the university context helps identify areas for improvement to tailor pedagogical strategies to students’ requirements, fostering meaningful learning, creativity, and critical thinking (Akpur, 2020; Aslan, 2021; Sari et al., 2021; Simanjuntak et al., 2021). Given that one of the main skills of university students is problem-solving, this becomes even more crucial since handling difficult circumstances affects their welfare, academic performance, even future employment (Demirhan and Şahin, 2021; Dikmen, 2022; Korkmaz et al., 2020; van Laar et al., 2020).
Empirical data shows that elements supporting students’ academic success and development problem-solving is tightly related to academic motivation, creative and critical thinking, and self-directed learning factors that support students’ academic success and development (Hwang and Oh, 2021; Orakci, 2023; Ramos and Hayward, 2018). It is also associated with greater confidence, persistence, and the use of adaptive strategies to tackle complex problems (Yilmaz, 2022). However, its impact goes beyond academic performance, influencing motivational processes, promoting resilience in challenging educational contexts, facilitating the adoption of metacognitive strategies, and reducing procrastination (Kozikoglu, 2019). Furthermore, problem-solving is significantly related to academic self-efficacy, as confirmed by a correlational study indicating a direct and robust relationship (r > 0.50) with academic variables, such as inquiry community, reflective academic thinking, and metacognitive awareness (Karaoglan-Yilmaz et al., 2023). In other contexts, such as the workplace, problem-solving self-efficacy is based on personal belief in the ability to perform necessary actions in specific situations (van Laar et al., 2020). Nevertheless, it is considered that research in work settings differs from academic contexts, as studying problem-solving in educational environments is less straightforward.
This study is justified by the need to develop an instrument to measure problem-solving ability in higher education students. This measure is relevant due to the high rates of university dropout in Latin America, reaching 46% (Mellado et al., 2018) and in Peru, where it stands at 16.2% (Ministerio de la Mujer y Poblaciones Vulnerables – MIMP, 2019). Dropout is associated with personal factors, such as academic self-efficacy and emotional exhaustion, as well as interactive factors related to teaching processes (Améstica-Rivas et al., 2020; Fernández-Martín et al., 2019). In this context, problem-solving, as defined in this study, includes both intrinsic student factors and external factors derived from the educational environment. Including this variable in academic analysis could facilitate understanding the causes of university dropout, which leads to losses in public investment in education (Dominguez-Lara, 2016; Pal, 2012) and increases the population without professional competencies (Rocha et al., 2017). A validated and reliable instrument to measure problem-solving would contribute to mitigating these rates, supporting the development of academic self-efficacy.
The purpose of this study is to develop and validate an instrument to measure problem-solving in higher education students, according to international standards, and to provide evidence related to its content, internal structure, and relationships with other variables.
2 Method
2.1 Participants
The sample size was calculated using the ‘semPower’ package (Moshagen and Bader, 2023) with an a priori analysis. Parameters were set to 86 degrees of freedom, RMSEA = 0.05, power = 0.95, and alpha = 0.05, yielding a minimum required sample size of 250 participants. The study exceeded this requirement, including a total of 733 students. Table 1 provides a summary of the participants’ sociodemographic characteristics under the conditions Total, EFA, and CFA. The majority were female (59.89%), with similar distributions in EFA (58.23%) and CFA (60.69%). The average age was 21.56 years (SD = 4.15), slightly higher in CFA (21.59) and lower in EFA (21.5). The health college had the highest representation (53.07% overall), followed by Business (17.46%). Most students were in semesters 4–6 (60.30%) and resided in Lima (55.66%). The vast majority belonged to the Regular Undergraduate program (94.68%), with a small proportion of Working Adults (5.32%).
2.2 Instrument
Participant Demographic Information. A detailed demographic information form was used to collect data on the participants in this study. Variables included gender, age, college, academic semester, place of residence, and the educational program in which they were enrolled.
Problem-Solving Questionnaire (PSQ). Designed to gauge how people handle everyday obstacles, this is a 15-item measure. The following four-point Likard-type answer structure is used: Rarely is my case (1), Sometimes is my case (2), Frequently is my case (3), and Always is my case (4). The questionnaire assesses many facets of problem-solving behavior; this paper investigates its psychometric qualities. For a complete list of the items, refer to Appendix A.
Academic Self-Efficacy Scale (EAPESA; Palenzuela, 1983). Modified for use with Peruvian university students (Dominguez-Lara and Fernández-Arata, 2019), this scale includes nine items that assess students’ belief in their ability to successfully perform academic tasks. Responses are recorded on a four-point Likert scale, ranging from Never to Always, where higher scores indicate greater academic self-efficacy. The EAPESA has demonstrated strong psychometric validity across different cultural contexts, including Peru, and consistently shows high reliability in various studies. In this study, the scale’s reliability was excellent, with a Cronbach’s alpha of 0.91. The availability of normative data for Peruvian university students further enhances its suitability for educational research.
2.3 Procedure
The test construction process was conducted in three phases. In Phase 1, referred to as the theoretical framework, an extensive review of the scientific literature on satisfaction and problem-solving in specialized texts was carried out, enabling a deep understanding of the phenomenon under study. In Phase 2, titled test development, the construct was operationalized using an operationalization table (see Table 2). This process facilitated the creation of 15 items focused on key aspects of problem-solving, aligning with the goal of building a brief measure (Ziegler et al., 2014). The choice to employ only 15 elements was driven by the need to create a quick, simple tool that would reduce participant weariness and be appropriate for time-limited situations.
Regarding the “Generation and Evaluation of Alternatives” dimension, it consists of three items, unlike the other dimensions with four. This reduction followed expert review, where one item was removed for redundancy to maintain clarity and focus. For instance, an item such as “I explore various ways to address the problem” was considered redundant as it overlapped conceptually with “I create as many alternatives as possible.” Although having fewer items may limit the detailed exploration of this dimension, the remaining items effectively represent its critical aspects. Future studies could address this by adding items to ensure a more balanced representation across dimensions.
To ensure content validity, three expert judges reviewed the instrument, evaluating each item based on representativeness and relevance criteria, following international technical recommendations (American Educational Research Association et al., 2014; Clark and Watson, 2016). This procedure ensured that the items were conceptually coherent and appropriately aligned with the theoretical aspects of the construct, providing a solid foundation for test quality.
The instrument was administered collectively through an online form, using a snowball sampling method in which university students shared the form with other students. Due to the virtual nature of the process, an Internet-Mediated Research (IMR; Hoerger and Currell, 2012) methodology was implemented. Prior to participation, an informed consent form was presented, which included essential information on the study’s objective, anonymity, and data processing. The study was approved by the ethics committee of the authors’ university (N° 0132-2024-CIE) and adhered to the guidelines of the Declaration of Helsinki (World Medical Association, 1964). The virtual form was available from October 7 to October 16, 2024, with an approximate completion time of 10 min.
In Phase 3, a preliminary review of the items was conducted. Given the ordinal nature of the observable variables, bar charts were used for the initial visualization of the data. Additionally, in accordance with international standards, dimensionality (internal structure of the test), reliability/precision, and validity in relation to other variables were examined. These analyses are described in detail in the data analysis section.
Finally, all research materials, including (a) the database, (b) the R code, and (c) the test format used, were deposited in the open-access repository OSF, ensuring the study’s accessibility and transparency: https://osf.io/k3qv8/?view_only=c525fb67e0b946efad25516a498283ee.
2.4 Data analysis
The R programming language running in the RStudio environment (RStudio Team, 2022) was used to carry out all data analysis. Data organizing and model estimate were aided by many packages including ‘psych’ (Revelle, 2021), ‘lavaan’ (Rosseel, 2012), ‘semPlot’ (Epskamp, 2015), and ‘PsyMetricTools’ (Ventura-León, 2024).
Given the ordinal nature of the variables, a preliminary evaluation of response rates for Likert-type items was conducted to ensure that each response option had a minimum frequency of 10%, as lower values could negatively impact model estimation (Linacre, 2002).
The data analysis process was divided into several stages. First, an Exploratory Factor Analysis (EFA) was conducted. The number of factors was determined by setting an initial number and evaluating model fit as factors were added. A four-factor structure was found to provide acceptable fit values. Following the suggested cutoffs by Hu and Bentler (1999), where SRMR and RMSEA values below 0.08 and CFI and TLI values over 0.95 indicate satisfactory fit, model fit was assessed using indices including RMSEA, SRMR, CFI, and TLI. Also taken into account were factor loadings above 0.30 and inter-factor correlations above 0.32 (Tabachnick and Fidell, 2019).
All models were evaluated with an oblimin rotation and using the mean and variance-adjusted weighted least squares (WLSMV) method, implemented in ‘lavaan’ (Rosseel, 2022). This estimator was chosen for its demonstrated effectiveness in handling ordinal data (Li, 2016). Additionally, a three-factor model was tested on a secondary dataset to evaluate its performance, as the original proposal suggested three factors (Silvera et al., 2001).
During the EFA, items with factorial complexity (items with loadings above 0.30 on multiple factors; Lloret et al., 2014) were removed, which improved model fit.
In the Confirmatory Factor Analysis (CFA), models from previous studies were initially tested to determine if existing structures performed well with the WLSMV estimator. Generally, these models did not meet acceptable fit criteria (CFI ≤ 0.95, RMSEA g ≥ 0.08). As a result, data were re-specified based on modification indices (MI > 10), expected parameter changes (EPC > 0.2), and high standardized residual covariances (>0.2). These thresholds served as guides, and theoretical reasoning was applied to justify the changes made to the scale, which was structured around the theoretical model of problem-solving. It was ensured that each subscale retained at least three items for model identification purposes. Factor loadings above 0.30 and inter-factor correlations greater than 0.32 were also applied as standards (Tabachnick and Fidell, 2019).
Reliability was assessed using the omega coefficient (ω), recommended for factorial models, especially congeneric models with unequal factor loadings (McDonald, 2013; Savalei and Reise, 2019; Ventura-León and Caycho-Rodríguez, 2017).
To validate the scales in relation to another variable, a structural equation modeling approach was used. Specifically, a CFA model was employed to explore the interrelationships among various constructs (Raykov and Marcoulides, 2006). One of the main advantages of CFA models over simple correlations is their ability to account for item weights, measurement errors, and indirect measures, providing a more accurate representation of the latent variable. Academic Self-Efficacy was selected as a convergence measure due to its strong empirical support.
3 Results
3.1 Preliminary analysis
Figure 1 displays the frequency distribution of responses for items RP1 to RP15. A clear variation in responses is observed, with a strong preference for the “Always is my case” category (Option 4) in items like RP7 (46.66%) and RP6 (46.52%). In contrast, the “Rarely is my case” category (Option 1) has its highest frequency in RP1 (13.23%) and RP9 (9.14%). The intermediate categories, “Sometimes is my case” (Option 2) and “Frequently is my case” (Option 3), also reveal different distributions, especially in items like RP9, where “Sometimes is my case” hits 37.24%, and RP3, where “Frequently is my case” accounts for 16.51%. Responses often cluster at the extremes for certain items, so stressing the different views recorded by every item on the scale.
3.2 Exploratory factor analysis
Table 3 presents the goodness-of-fit indices for the exploratory factor analysis (EFA) model applied to the Problem-Solving Scale. The model was evaluated using χ2, degrees of freedom (df), SRMR, CFI, TLI, and RMSEA. Overall, the model shows a good fit, with a CFI of 0.989 and a TLI of 0.978, indicating strong data alignment. The RMSEA of 0.075 is also within acceptable limits, suggesting a reasonable, though slightly elevated, fit. The SRMR of 0.026 further reinforces the model’s fit quality.
Table 4 shows the factorial structure derived from the exploratory factor analysis (EFA) applied to the Problem-Solving Scale along with the factor loadings of every item on the four found factors: f1, f2, f3, and f4. The bolded loadings show how often an item is assigned to a certain factor, like RP1 in f1 or RP6 in f2. Many items have notable loadings on a single factor, which supports the validity of the factorial structure. RP1, for instance, has a significant loading on f1 (0.82), whereas RP5 is strongly correlated with f2 (0.81). Indicated at the bottom of the table, the modest correlations between the variables point to a sufficient variation between the measured constructs. With values between 0.78 and 0.90, the omega coefficients (ω) also demonstrate strong internal dependability for every component.
3.3 Confirmatory factor analysis
Figure 2 illustrates the final Confirmatory Model structure of the Problem-Solving Scale, with four factors: Prioritization and Review of Alternatives (PRA), Generation and Evaluation of Alternatives (GEA), Critical Evaluation of Solutions (CES), and Solution Analysis and Planning (SAP). The item factor loadings are above 0.68 and the inter-factor correlations vary from 0.84 to 0.92, suggesting strong interrelationships across dimensions. Excellent fit indices corroborate these findings: χ2 (84) = 244, CFI = 0.98, TLI = 0.975, RMSEA = 0.062, SRMR = 0.034, and CRMR = 0.03. Additionally, omega coefficients show acceptable to good reliability: ω_PRA = 0.79, ω_GEA = 0.82, ω_CES = 0.73, and ω_SAP = 0.81. It is important to note that, the solid lines represent the factor loadings of each item on its respective latent factor, indicating the relationship between the observed variables and the theoretical constructs. On the other hand, the dashed lines represent factor loadings that were restricted or set to fixed values during the estimation process.
3.4 Evidence of validity in relation to another variable
Ranging from 0.65 to 0.86, Figure 3 shows the relationship between academic self-efficacy (SE) and the components of the problem-solving scale. With χ2 (242) = 496.039, SRMR = 0.037, WRMS = 1.013, CFI = 0.984, TLI = 0.981, and RMSEA = 0.486, the confirmatory factor analysis fit indices show an outstanding model fit. With CFI and TLI above 0.95 and an RMSEA around 0.05, these data indicate a great model fit. The correlations between Academic Self-Efficacy and the problem-solving elements show a strong interrelationship among these dimensions: 0.64 with Prioritizing and Review of Alternatives (PRA), 0.57 with Generation and Evaluation of Alternatives (GEA), 0.36 with Critical Evaluation of Solutions (CES), and 0.80 with Solution Analysis and Planning (SAP). Emphasizing that the solid lines show the standardized factor loadings of the items on their respective latent factors, the dashed lines show factor loadings limited or fixed throughout the estimate process. This difference helps us to see the links between the theoretical constructions as well as the factorial structure of the scale.
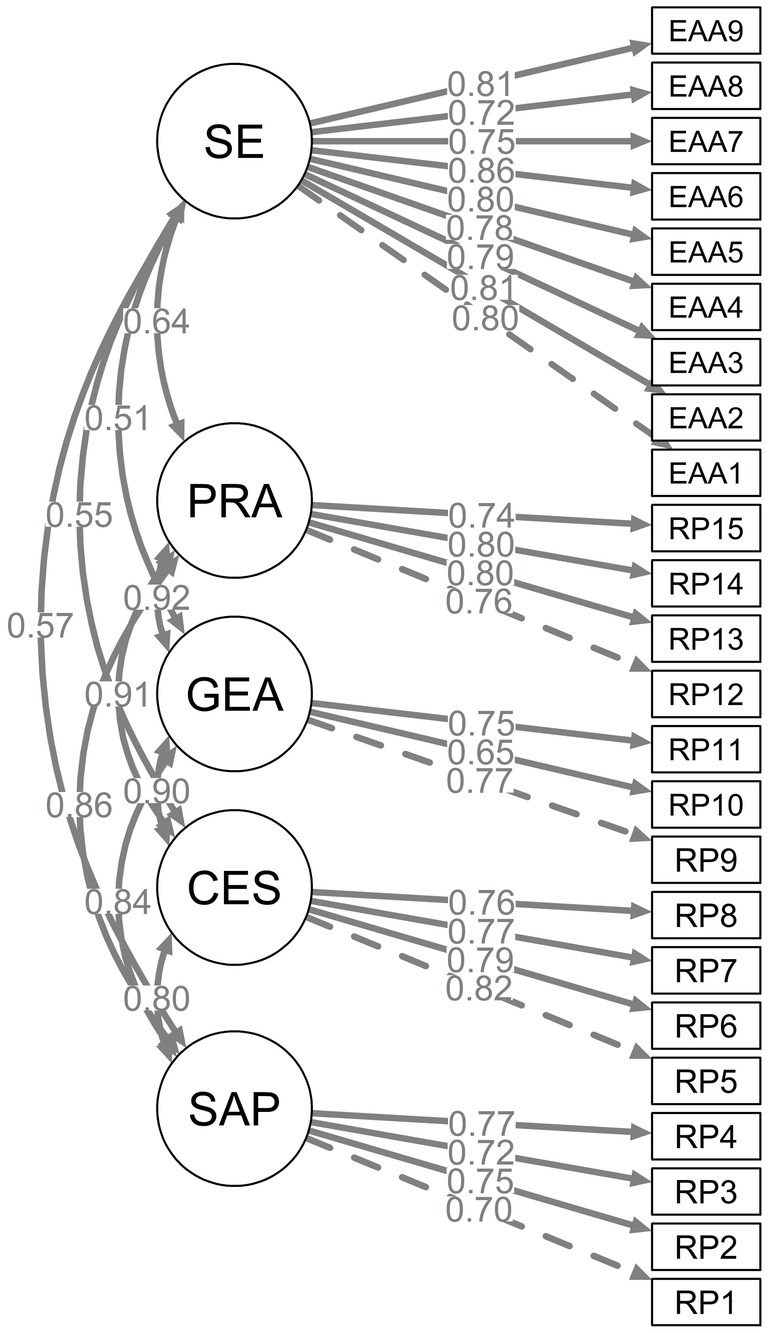
Figure 3. Relationship between the factors of the problem-solving scale and academic self-efficacy. Solution Analysis and Planning (SAP), Critical Evaluation of Solutions (CES), Generation and Evaluation of Alternatives (GEA), Prioritization and Review of Alternatives (PRA), Self-Efficacy (SE). The solid lines represent the standardized factor loadings of the items, whereas dashed lines indicate factor loadings that were constrained or fixed during the estimation process.
4 Discussion
Appropriate instruments to evaluate problem-solving in educational environments are still much needed (Ilbay, 2024). In the Peruvian setting, there is still needed to create and validate a particular instrument to evaluate this ability at the university level even if active approaches that foster critical thinking and creativity are being tried (Espinoza, 2021; Velázquez-Tejeda and Goñi Cruz, 2024; Hortigüela-Alcalá et al., 2019). The research of the problem-solving construct has become more important since it is regarded as a basic competency for addressing higher education challenges and is also connected with metacognitive skills fundamental for promoting autonomous and self-regulated learning (Monroy and Villamil, 2023; Guamán-Ledesma and Rivera, 2024; Covarrubias-Apablaza et al., 2019). In this regard, it is essential to create a legitimate, accurate, and succinct instrument to evaluate university students’ aptitude for solving problems. An innovative approach using the WLSMV estimator was employed for the CFA, recognized for its effectiveness in analyzing ordinal variables (Li, 2016). This kind of tool is crucial for catching the essence of problem-solving and thus a perfect choice for research requiring exact and quick evaluations in learning environments.
Lack of such tools hinders attempts to match educational practices with global goals like Sustainable Development Goal 4 (SDG 4), which stresses inclusive and fair quality education and lifetime learning possibilities for everyone (Dastyari and Jose, 2024). Development of skills that increase academic performance and employability depends on closing this disparity, therefore fostering innovation and equality in education (Haxhiu, 2023). This also highlights the urgent need for tools that bridge global disparities in educational outcomes, particularly in underserved regions (Mavangere et al., 2022). These considerations underscore the necessity of contextually relevant tools that not only measure but also facilitate the development of problem-solving competencies aligned with both local and global educational objectives.
The descriptive analysis shows significant variability in participants’ responses, with a tendency toward high scores, such as “Always is my case,” especially in items 7 (“I propose ideas before deciding”) and 6 (“I identify the obstacles of the problem”). Similarly, intermediate scores, particularly “Sometimes is my case,” are predominant in item 9 (“I generate the maximum number of alternatives”). This variability may be influenced by the participants’ specific experiences and contexts, aligning with problem-solving models.
Regarding the internal structure of the scale, exploratory factor analysis findings indicated that the four-dimensional model was most suitable for the 15 items, as the fit indices were optimal. This structure was confirmed by the CFA, yielding satisfactory results with excellent fit indices. Theoretical assumptions are supported by these findings because conceptual methods of problem-solving suggest four primary talents (D’Zurilla and Goldfried, 1971; D’Zurilla and Nezu, 1980; Maydeu-Olivares and D’Zurilla, 1996). Thus, there is enough evidence to claim that the problem-solving scale has an underlying structure made up of four theoretically supported components (D’Zurilla et al., 2004; D’Zurilla and Goldfried, 1971).
By fostering these competencies, educational institutions can help reduce dropout rates and improve equity in learning outcomes, aligning with SDG 4 and broader education goals (Albert et al., 2023). Moreover, these initiatives may assist structural changes meant to solve educational disparities in underprivileged populations all around (Meng, 2024). Including problem-solving techniques into curricula not only meets immediate academic demands but also helps society by enabling students to properly negotiate difficult obstacles.
Emphasizing how this interaction helps kids become resilient and achieve academically, the empirical data show the great link between academic self-efficacy and problem-solving abilities. Studies reveal that successful confrontation of challenges depends on self-efficacy (van Laar et al., 2020). Indeed, a higher level of problem-solving capacity is linked to a greater sense of self-efficacy, helping students approach academic tasks with confidence and persistence (Yilmaz, 2022). This connection aligns with educational psychology findings that link metacognitive awareness with problem-solving skills, promoting self-directed learning and resilience in challenging academic settings (Kozikoglu, 2019; Hwang and Oh, 2021). Consequently, self-efficacy not only predicts students’ ability to manage and solve complex academic problems but also correlates with adaptive coping mechanisms, enhancing persistence and reducing procrastination (Karaoglan-Yilmaz et al., 2023). This relationship is particularly relevant in Latin American contexts, where high university dropout rates prevail; developing self-efficacy through problem-solving skills could mitigate the negative impact of these rates by fostering continued engagement and successful academic trajectories (Mellado et al., 2018).
Reliability was demonstrated through internal consistency using the omega coefficient, as this is preferable when the factor models are congeneric (Savalei and Reise, 2019; Ventura-León and Caycho-Rodríguez, 2017). The results showed values between 0.78 and 0.90, exceeding the recommended 0.70 threshold (Ventura-León and Caycho-Rodríguez, 2017). This is like the reliability reported in other tests that also measure problem-solving (D’Zurilla et al., 1999; Maydeu-Olivares et al., 2000). Therefore, the scale items consistently measure each aspect that constitutes problem-solving.
This study is significant because it focuses on constructing a scale to assess problem-solving in the Peruvian university context, where specific tools for measuring this competency are still lacking (Ilbay, 2024). Additionally, future research should explore the instrument’s applicability in diverse educational settings, thereby informing curriculum development and policymaking and extending its benefits beyond the local context. By providing a measure of this skill, the research offers educators and education professionals valuable data for optimizing curricula and guiding pedagogical decisions, with the development and validation of this instrument being among its main contributions (Maydeu-Olivares et al., 2000; Sotomayor and Águila, 2022). In this sense, the study expands the understanding of problem-solving, as while consolidated theories exist regarding this skill in other contexts, it has not yet been thoroughly explored from a rational approach. Thus, the proposed scale is based on the Rational Problem-Solving Style, defined by D’Zurilla and Goldfried (1971) as a conscious, deliberate, and rational process that encompasses essential skills such as problem definition, alternative generation, decision-making, and solution verification. Additionally, the scale includes a convergence analysis with academic satisfaction, which adds a more comprehensive perspective on the impact of this competency on student wellbeing. This tool, therefore, allows for adapting pedagogical strategies to the actual needs of students, promoting meaningful learning and developing skills such as creativity and critical thinking (Akpur, 2020; Aslan, 2021; Sari et al., 2021; Simanjuntak et al., 2021). This comprehensive approach also contributes to a limited regional literature and highlights the relationship between rational problem-solving and students’ ability to reflect and employ metacognitive strategies, thereby enhancing their performance and capacity to face challenges (Astuhuaman and Cristóbal, 2021).
The development and validation of the problem-solving scale faced limitations. The use of snowball sampling limited generalizability, emphasizing the need for random sampling in future research. Although the sample size exceeded the minimum requirement of 250 participants, with 733 students, expanding the sample further could improve robustness and allow for detailed subgroup analyses. The “Generation and Evaluation of Alternatives” dimension has fewer items than other dimensions but meets Brown’s (2015) recommendation of at least three items per factor, ensuring reliability and validity. Future research could add items to enhance its comprehensiveness and balance across factors.
5 Conclusion
In conclusion, this study provides higher education with a valid and reliable tool for assessing problem-solving in Peruvian university contexts, addressing a significant gap in measuring essential competencies for autonomous learning and self-regulation. Strong psychometric qualities and a reasonable capture of the logical aspect of problem-solving define the scale. Research and instructional practice both benefit much from these results. First, the tool helps teachers to methodically evaluate students’ ability to solve problems, therefore enabling evidence-based curriculum design enhancements. Second, it offers a way to find students who could benefit from focused treatments meant to improve cognitive and metacognitive abilities. At last, the creation of a theory-driven, succinct, culturally appropriate instrument supports worldwide attempts to standardize the evaluation of 21st-century abilities in various educational environments.
Data availability statement
The datasets presented in this study can be found in online repositories. The names of the repository/repositories and accession number(s) can be found at: https://osf.io/k3qv8/?view_only=c525fb67e0b946efad25516a498283ee.
Ethics statement
The studies involving humans were approved by Universidad Privada del Norte Ethics Committee. The studies were conducted in accordance with the local legislation and institutional requirements. The participants provided their written informed consent to participate in this study.
Author contributions
JV-L: Conceptualization, Formal analysis, Investigation, Project administration, Supervision, Writing – original draft, Writing – review & editing. CL-C: Data curation, Methodology, Writing – original draft, Writing – review & editing. ST-M: Data curation, Investigation, Writing – original draft, Writing – review & editing. AS-V: Investigation, Methodology, Validation, Writing – original draft, Writing – review & editing. GG-M: Data curation, Formal analysis, Methodology, Writing – original draft, Writing – review & editing.
Funding
The author(s) declare that financial support was received for the research and/or publication of this article. The authors declare that this research received funding from the Universidad Privada del Norte.
Conflict of interest
The authors declare that the research was conducted in the absence of any commercial or financial relationships that could be construed as a potential conflict of interest.
Generative AI statement
The authors declare that no Gen AI was used in the creation of this manuscript.
Publisher’s note
All claims expressed in this article are solely those of the authors and do not necessarily represent those of their affiliated organizations, or those of the publisher, the editors and the reviewers. Any product that may be evaluated in this article, or claim that may be made by its manufacturer, is not guaranteed or endorsed by the publisher.
References
Akpur, U. (2020). Critical, reflective, creative thinking and their reflections on academic achievement. Think. Skills Creat. 37:100683. doi: 10.1016/j.tsc.2020.100683
Albert, J. R., Basillote, L., Alinsunurin, J., Vizmanos, J. F., Muñoz, M., and Hernandez, A. (2023). Sustainable development goal 4 on quality education for all: how does the Philippines fare and what needs to be done? PIDS Discussion Paper Series. doi: 10.62986/dp2023.16
American Educational Research Association, American Psychological Association, & National Council on Measurement in Education (2014). Standards for educational and psychological testing. 7th Edn. Washington DC: American Educational Research Association.
Améstica-Rivas, L., King-Domínguez, A., Gutiérre, D. A. S., and González, V. R. (2020). Efectos económicos de la deserción en la gestión universitaria: el caso de una universidad pública chilena. Hallazgos 18, 209–231. doi: 10.15332/2422409x.5772
Aslan, A. (2021). Problem-based learning in live online classes: learning achievement, problem-solving skill, communication skill, and interaction. Comput. Educ. 171:104237. doi: 10.1016/j.compedu.2021.104237
Astuhuaman, G. G., and Cristóbal, O. E. P. (2021). Resolución de problemas, habilidades y rendimiento académico en la enseñanza de la matemática. Revista Educación 45, 170–182. doi: 10.15517/revedu.v45i1.41237
Brown, T. A. (2015). Confirmatory factor analysis for applied research. New York, United States of America: Guilford Publications.
Castellanos, N. E., and Rojas, Y. P. (2023). Competencias del siglo XXI en educación: una revisión sistemática durante el periodo 2014-2023. Ciencia Latina Revista Científica Multidisciplinar 7, 219–249. doi: 10.37811/cl_rcm.v7i4.6869
Chang, E. C., D’Zurilla, T. J., Sanna, L. J., D’Zurilla, T. J., and Sanna, L. J. (2004). Resolución de problemas sociales: teoría, investigación y formación. Washington DC, United States of America: American Psychological Association.
Clark, L. A., and Watson, D. (2016). “Constructing validity: basic issues in objective scale development” in Methodological issues and strategies in clinical research. ed. J. A. Kazdin. 4th ed (Washington DC, United States of America: American Psychological Association), 187–203.
Covarrubias-Apablaza, C. G., Acosta-Antognoni, H., and Mendoza-Lira, M. (2019). Self-regulation learning and general self-efficacy and their relation with academic goals in university students. Formacion Universitaria 12, 103–114. doi: 10.4067/S0718-50062019000600103
D’Zurilla, T. J., and Goldfried, M. R. (1971). Problem solving and behavior modification. J. Abnorm. Psychol. 78, 107–126. doi: 10.1037/h0031360
D’Zurilla, T. J., and Maydeu-Olivares, A. (1995). Conceptual and methodological issues in social problem-solving assessment. Behav. Ther. 26, 409–432. doi: 10.1016/S0005-7894(05)80091-7
D’Zurilla, T. J., and Nezu, A. (1980). A study of the generation-of-alternatives process in social problem solving. Cogn. Ther. Res. 4, 67–72. doi: 10.1007/BF01173355
D’Zurilla, T. J., and Nezu, A. (1982). “Social problem-solving in adults” in Advances in cognitive-behavioral research and therapy. ed. P. C. Kendall (New York, United States of America: Academic Press).
D’Zurilla, T. J., and Nezu, A. M. (1990). Development and preliminary evaluation of the social problem-solving inventory. Psychol. Assess. 2, 156–163. doi: 10.1037/1040-3590.2.2.156
D’Zurilla, T. J., Nezu, A. M., and Maydeu-Olivares, A. (1999). Manual for the social problem-solving inventory-revised. New York, United States of America: Multi-Health Systems.
D’Zurilla, T. J., Nezu, A. M., and Maydeu-Olivares, A. (2004). Social problem solving: theory and assessment. In D’Zurilla, T. J., Sanna, T. J., and Chang, L. J., Resolución de problemas sociales: Teoría, investigación y formación. Washington DC, United States of America: American Psychological Association.
Dastyari, A., and Jose, C. (2024). Achieving inclusive and equitable quality education for all: the importance of digital inclusion. Alternat. Law J. 49, 282–287. doi: 10.1177/1037969X241295798
Demirhan, E., and Şahin, F. (2021). The effects of different kinds of hands-on modeling activities on the academic achievement, problem-solving skills, and scientific creativity of prospective science teachers. Res. Sci. Educ. 51, 1015–1033. doi: 10.1007/S11165-019-09874-0
Dikmen, M. (2022). Mindfulness, problem-solving skills and academic achievement: do perceived stress levels matter? Kuramsal Eğitimbilim 15, 42–63. doi: 10.30831/akukeg.945678
Dominguez-Lara, S. A. (2016). Normative data of an academic self-efficacy scale in college students from Lima. Interacciones 2, 91–98. doi: 10.24016/2016.v2n2.31
Dominguez-Lara, S., and Fernández-Arata, M. (2019). Autoeficacia académica en estudiantes de Psicología de una universidad de Lima. Revista Electrónica de Investigación Educativa 21, 1–13. doi: 10.24320/redie.2019.21.e32.2014
Epskamp, S. (2015). semPlot: unified visualizations of structural equation models. Struct. Equ. Model. Multidiscip. J. 22, 474–483. doi: 10.1080/10705511.2014.937847
Espinoza, E. E. (2021). El aprendizaje basado en problemas, un reto a la enseñanza superior. Conrado. 17, 295–303.
Fernández-Martín, T., Solís-Salazar, M., Hernández-Jiménez, M. T., and Moreira-Mora, T. E. (2019). Un análisis multinomial y predictivo de los factores asociados a la deserción universitaria. Revista Electrónica Educare 23, 1–25. doi: 10.15359/ree.23-1.5
Guamán-Ledesma, J. L., and Rivera, Y. V. (2024). Fomentando el pensamiento reflexivo: estrategias para mejorar las habilidades de metacognición. Esprint Investigación 3, 28–38. doi: 10.61347/ei.v3i1.63
Haxhiu, Z. (2023). Closing the achievement gap: an analysis of equity-based educational interventions. J. Educ. Rev. Provis. 2, 32–35. doi: 10.55885/jerp.v2i2.154
Hoerger, M., and Currell, C. (2012). “Ethical issues in internet research” in APA handbook of ethics in psychology (Vol. 2): practice, teaching, and research. eds. S. Knapp, M. Gottlieb, M. Handelsman, and L. VandeCreek (Washington DC, United States of America: American Psychological Association), 385–400.
Hortigüela-Alcalá, D., Palacios Picos, A., and López Pastor, V. (2019). The impact of formative and shared or co-assessment on the acquisition of transversal competences in higher education. Assess. Eval. High. Educ. 44, 933–945. doi: 10.1080/02602938.2018.1530341
Hu, L., and Bentler, P. M. (1999). Cutoff criteria for fit indexes in covariance structure analysis: conventional criteria versus new alternatives. Struct. Equ. Model. Multidiscip. J. 6, 1–55. doi: 10.1080/10705519909540118
Hwang, Y., and Oh, J. (2021). The relationship between self-directed learning and problem-solving ability: the mediating role of academic self-efficacy and self-regulated learning among nursing students. Int. J. Environ. Res. Public Health 18, 1–9. doi: 10.3390/ijerph18041738
Ilbay, E. (2024). La importancia del pensamiento crítico y la resolución de problemas en la educación contemporánea. Revista Científica Kosmos 3, 4–18. doi: 10.62943/rck.v3n1.2024.50
Karaoglan-Yilmaz, F. G., Ustun, A. B., Zhang, K., and Yilmaz, R. (2023). Metacognitive awareness, reflective thinking, problem solving, and community of inquiry as predictors of academic self-efficacy in blended learning: a correlational study. Turk. Online J. Dist. Educ. 24, 20–36. doi: 10.17718/tojde.989874
Kemper, C. J., Trapp, S., Kathmann, N., Samuel, D. B., and Ziegler, M. (2019). Short versus long scales in clinical assessment: exploring the trade-off between resources saved and psychometric quality lost using two measures of obsessive--compulsive symptoms. Assessment 26, 767–782. doi: 10.1177/1073191118810057
Korkmaz, S., Keleş, D. D., Kazgan, A., Baykara, S., Gürok, M. G., Demir, C. F., et al. (2020). Emotional intelligence and problem solving skills in individuals who attempted suicide. J. Clin. Neurosci. 74, 120–123. doi: 10.1016/j.jocn.2020.02.023
Kozikoglu, I. (2019). Investigating critical thinking in prospective teachers: metacognitive skills, problem solving skills and academic self-efficacy. J. Soc. Stud. Educ. Res. 10, 111–130. Available at: https://jsser.org/index.php/jsser/article/view/362/371
Li, C. H. (2016). Confirmatory factor analysis with ordinal data: comparing robust maximum likelihood and diagonally weighted least squares. Behav. Res. Methods 48, 936–949. doi: 10.3758/s13428-015-0619-7
Linacre, J. M. (2002). Optimizing rating scale category effectiveness. J. Appl. Meas. 3, 85–106. Available at: https://www.researchgate.net/publication/292264351_Optimizing_rating_scale_category_effectiveness
Lloret, S., Ferreres, A., Hernández, A., and Tomás, I. (2014). Exploratory item factor analysis: a practical guide revised and updated. Anales de Psicología 30, 1151–1169. doi: 10.6018/analesps.30.3.199361
Makoviichuk, O., Shulha, A., Shestobuz, O., Pits, I., Prokop, I., and Byhar, H. (2020). Training future primary school teachers in the context of developing constructive skills in younger pupils. Revista Romaneasca Pentru Educatie Multidimensionala 12, 232–250. doi: 10.18662/rrem/12.1sup1/233
Mavangere, N., Edifor, E. E., Adedoyin, F., Apeh, E., and Owusu, A. (2022). Education inequality in underserved regions: exploring the role of technology to promote diversity and inclusivity. In 2022 IEEE international conference on E-business engineering (ICEBE), (pp. 288–293).
Maydeu-Olivares, A., and D’Zurilla, T. J. (1996). A factor-analytic study of the social problem-solving inventory: an integration of theory and data. Cogn. Ther. Res. 20, 115–133. doi: 10.1007/BF02228030
Maydeu-Olivares, A., Rodriguez-Fornells, A., Gomez-Benito, J., and D’Zurilla, T. (2000). Psychometric properties of the Spanish adaptation of the social problem-solving inventory-revised (SPSI-R). Personal. Individ. Differ. 29, 699–708. doi: 10.1016/S0191-8869(99)00226-3
Mellado, F. R. M., Orellana, M. B. C., and Gabrie, A. J. B. (2018). Student retention and dropout in higher education in Latin America and the Caribbean: a systematic review. Educ. Policy Anal. Arch. 26, 1–36. doi: 10.14507/epaa.26.3348
Meng, X. (2024). Education policy and social justice: a policy framework analysis for improving educational opportunities for marginalized groups. J. Educ. Educ. Policy Stud. 2, 5–11. doi: 10.54254/3049-7248/2/2024008
Ministerio de la Mujer y Poblaciones Vulnerables – MIMP (2019). “Política Nacional de Igualdad de Género” in El Peruano.
Moshagen, M., and Bader, M. (2023). semPower: general power analysis for structural equation models. Behav. Res. Methods 56, 2901–2922. doi: 10.3758/s13428-023-02254-7
Monroy, N. E. C., and Villamil, Y. P. R. (2023). Competencias del siglo XXI en educación: una revisión sistemática durante el periodo 2014-2023. Ciencia Latina Revista Científica Multidisciplinar. 7, 219–249.
Orakci, Ş. (2023). Structural relationship among academic motivation, academic self-efficacy, problem solving skills, creative thinking skills, and critical thinking skills. Psychol. Sch. 60, 2173–2194. doi: 10.1002/pits.22851
Pal, S. (2012). Mining educational data to reduce dropout rates of engineering students. Int. J. Inf. Eng. Electron. Bus. 4, 1–7. doi: 10.5815/ijieeb.2012.02.01
Palenzuela, D. L. (1983). Construcción y validación de una escala de autoeficacia percibida específica de situaciones académicas. Análisis y Modificación de Conducta 9, 185–219.
Rammstedt, B., and Beierlein, C. (2014). Can’t we make it any shorter? J. Individ. Differ. 35, 212–220. doi: 10.1027/1614-0001/a000141
Ramos, L., and Hayward, S. L. (2018). An examination of college students’ problem-solving self-efficacy, academic self-efficacy, motivation, test performance, and expected grade in introductory-level economics courses. Decis. Sci. J. Innov. Educ. 16, 217–240. doi: 10.1111/dsji.12161
Raykov, T., and Marcoulides, G. A. (2006). A first course in structural equation modeling. 2nd Edn. New Jersey, United States of America: Lawrence Erlbaum Associates.
Revelle, W. (2021). psych: Procedures for psychological, psychometric, and personality research. Illinois, United States of America: Northwestern University.
Rocha, C. F., Zelaya, Y. F., Sánchez, D. M., and Pérez, A. F. (2017). Prediction of university desertion through hybridization of classification algorithms. CEUR workshop proceedings, 2029, 215–222.
Rosseel, Y. (2012). Lavaan: an R package for structural equation modeling and more. J. Stat. Softw. 48, 1–36. doi: 10.18637/jss.v048.i02
Rosseel, Y. (2022). lavaan: Latent variable analysis. Available online at: https://lavaan.ugent.be/development.html
RStudio Team. (2022). RStudio: Integrated Development for R. PBC, Boston, MA: RStudio. Available online at: http://www.rstudio.com/
Sari, Y. I., Sumarmi, S., Utomo, D. H., and Astina, I. K. (2021). The effect of problem based learning on problem solving and scientific writing skills. Int. J. Instr. 14, 11–26. doi: 10.29333/iji.2021.1422a
Savalei, V., and Reise, S. P. (2019). Don’t forget the model in your model-based reliability coefficients: a reply to McNeish (2018). Collabra Psychol. 5:5. doi: 10.1525/collabra.247
Silvera, D. H., Martinussen, M., and Dahl, T. I. (2001). The TromsøSocial intelligence scale, a self-report measure of social intelligence. Scand. J. Psychol. 42, 313–319. doi: 10.1111/1467-9450.00242
Simanjuntak, M. P., Hutahaean, J., Marpaung, N., and Ramadhani, D. (2021). Effectiveness of problem-based learning combined with computer simulation on students’ problem-solving and creative thinking skills. Int. J. Instr. 14, 519–534. doi: 10.29333/iji.2021.14330a
Sotomayor, D. R., and Águila, A. (2022). Competencia resolución de conflictos: pautas teórico-metodológicas para su formación en estudiantes de Sociología. Mendive. Revista de Educación 20, 69–82. Available at: https://mendive.upr.edu.cu/index.php/MendiveUPR/article/view/2661/html
van Laar, E., van Deursen, A. J. A. M., van Dijk, J. A. G. M., and de Haan, J. (2020). Determinants of 21st-century skills and 21st-century digital skills for workers: a systematic literature review. SAGE Open 10:215824401990017. doi: 10.1177/2158244019900176
Velázquez-Tejeda, M. E., and Goñi Cruz, F. F. (2024). Modelo de estrategia metacognitiva para el desarrollo de la resolución de problemas matemáticos. Páginas de Educación 17:e3313. doi: 10.22235/pe.v17i1.3313
Ventura-León, J. (2024). PsyMetricTools [software]. GitHub. Available at: https://github.com/jventural/PsyMetricTools
Ventura-León, J., and Caycho-Rodríguez, T. (2017). El coeficiente Omega: un método alternativo para la estimación de la confiabilidad. Revista Latinoamericana de Ciencias Sociales, Niñez y Juventud 15, 625–627. Available at: https://www.redalyc.org/journal/773/77349627039/html/
World Medical Association. (1964). Declaración de Helsinki. Available online at: http://www.conamed.gob.mx/prof_salud/pdf/helsinki.pdf (Accessed January 3, 2025).
Yilmaz, F. G. K. (2022). Utilizing learning analytics to support students’ academic self-efficacy and problem-solving skills. Asia-Pacific Educ. Res. 31, 175–191. doi: 10.1007/s40299-020-00548-4
Ziegler, M., Poropat, A., and Mell, J. (2014). Does the length of a questionnaire matter?: expected and unexpected answers from generalizability theory. J. Individ. Differ. 35, 250–261. doi: 10.1027/1614-0001/A000147
Appendix A
Problem-Solving Questionnaire (PSQ)
Instrucciones: Lee cada afirmación y selecciona la opción que mejor describa cómo ENFRENTAS LOS PROBLEMAS EN TU DÍA A DÍA. No hay respuestas correctas o incorrectas, solo elige lo que más se asemeje a tu comportamiento habitual. Tomate tu tiempo, no marques por marcar.
Keywords: problem-solving, validation, scale development, higher education, academic self-efficacy, metacognition
Citation: Ventura-León J, Lino-Cruz C, Tocto-Muñoz S, Sánchez-Villena A and Gamboa-Melgar G (2025) Problem-solving: development and validation of a short instrument for higher education. Front. Educ. 10:1555167. doi: 10.3389/feduc.2025.1555167
Edited by:
Aurora Dimache, Atlantic Technological University, IrelandReviewed by:
C. Paul Morrey, Utah Valley University, United StatesOtang Kurniaman, Riau University, Indonesia
Prasetyo Listiaji, University of Szeged, Hungary
Nataša Nikolić, University of Belgrade, Serbia
Copyright © 2025 Ventura-León, Lino-Cruz, Tocto-Muñoz, Sánchez-Villena and Gamboa-Melgar. This is an open-access article distributed under the terms of the Creative Commons Attribution License (CC BY). The use, distribution or reproduction in other forums is permitted, provided the original author(s) and the copyright owner(s) are credited and that the original publication in this journal is cited, in accordance with accepted academic practice. No use, distribution or reproduction is permitted which does not comply with these terms.
*Correspondence: José Ventura-León, am9zZS52ZW50dXJhQHVwbi5wZQ==