- 1Aerospace Systems Design, Analysis, and Optimization Lab, School of Aeronautics & Astronautics, Purdue University, West Lafayette, IN, United States
- 2Center of Integrated Systems in Airspace (System-of-Systems Lab), School of Aeronautics & Astronautics, Purdue University, West Lafayette, IN, United States
With rising concerns over commercial aviation’s contribution to global carbon emissions, the aviation industry faces tremendous pressure to adopt advanced solutions for reducing its share of CO2 emissions. One near-term potential solution to mitigate this global emissions situation is to operate existing aircraft with sustainable aviation fuel (SAF); this solution requires almost no modification to current aircraft, making it the “quickest” approach to reduce aviation carbon emissions, albeit the actual impact will be determined by the degree to which airlines adopt and use SAF, the ticket price impact of SAF, and the future growth of travel demand. This article presents results that estimate the expected fleet-wide emissions of future airline operations using SAF considering various projected traveler demand and biofuel penetration/utilization levels. The work demonstrates an approach to make these predictions by modeling the behavior of a profit-seeking airline using the Fleet-Level Environmental Evaluation Tool (FLEET). Considering five future SAF scenarios and two future passenger demand projection scenarios, FLEET estimates future fleet-level CO2 emissions, showcasing the possible upper and lower bounds on future aviation emissions when SAF is introduced for use in airline fleets. Results show that the future fleet-level CO2 emissions for all scenarios with SAF are lower than the baseline scenario with no SAF, for all demand projection scenarios. The passenger demand served and the trips flown for a given SAF scenario depends on the SAF price and the biofuel penetration levels. This shows that even if airlines serve a higher passenger demand for some future scenarios, the carbon emissions could still be lower than the current baseline scenario where airlines only use conventional jet fuel.
1 Introduction
The Paris Agreement, a multinational treaty that intends to confine the temperature growth to 2°C from pre-industrial levels by the year 2050 (United Nations Framework Convention on Climate Change, 2021), impacts all industries. The United States recently re-signed the Paris Agreement (U.S. Department of State, Office of the Spokesperson, 2021) and set 2030 emission reduction targets to accomplish its goals (The White House, 2021). The aviation industry is responsible for about 2.5% of global carbon emissions (Ritchie, 2020). Although this figure is relatively low, it is reasonable to assume that aviation will have to do its part to meet the agreement’s goals. In Europe, the “Destination 2050” report outlines a vision for European Aviation to attain net-zero CO2 emissions by the year 2050 (van der Sman et al., 2021). Additionally, the International Civil Aviation Organization (ICAO) (International Civil Aviation Organization, 2016) launched the Carbon Offsetting and Reduction Scheme for International Aviation (CORSIA) in 2021. The ICAO CORSIA monetizes the carbon emissions from international routes and it creates incentives for airline operators to use SAF with a premium price (Chao et al., 2019a; Chao et al., 2019b) to confine the carbon emissions from the aviation sector to the 2020 level (IATA, 2016; International Civil Aviation Organization, 2016; Chao et al., 2019c).
Achieving these goals will require technological improvements as well as policy changes. Sustainable Aviation Fuel (SAF) is one technology that has been under development for several years and that has been used in operation by some airlines (Csonka, 2016; United Airlines, 2021). SAF is a mixture of biofuels and conventionally petroleum-derived jet fuel (CJF) that has a lower life-cycle carbon footprint than conventional jet fuel. Because biofuels, commonly made from crops, absorb carbon dioxide when crops are grown, they can have reduced net carbon emissions; i.e. life-cycle emissions. Although biofuels tend to be priced higher than CJF, adopting SAF is one of the most straight-forward actions commercial aviation could take to reduce carbon emissions and meet the emission reduction targets (The White House, 2021). Moolchandani et al. (Moolchandani et al., 2011) show that the use of SAF can potentially reduce 2050 emissions in the U.S. by 55–92% of a 2005 baseline level. The resulting variation in CO2 reduction levels is governed by the sensitivity of the SAF adoption rate to the CJF prices. The Destination 2050 report (van der Sman et al., 2021) attributes a 34% reduction in future CO2 emissions for European Aviation from the use of SAF and an additional 12% reduction from the “effect of SAF on demand.”
Studies into the feasibility of SAF to achieve these emission goals include analysis of SAF production pathways, fleet penetration of leading aircraft technologies, and economic interactions between SAF and commercial aviation industries. Winchester et al. show the economic and environmental impacts of Hydro-processed Ester and Fatty Acids (HEFA) biofuels on U.S. commercial aviation (Winchester et al., 2013). Haller defined and explored future aircraft technologies for environmental improvement under NASA’s Subsonic Fixed Wing project (Haller, 2012). In the Renewable Fuel Standard Program (RFS2) Regulatory Impact Analysis from EPA, Sissine showed the properties of biofuels from different pathways in different regions (Sissine, 2010). Sun et al. (2020) and Sun et al. (2021) already showed that stringent environmental policies can enhance domestic innovation and improve energy efficiency however, the impacts of the environmental policies on the aviation industry are still unclear.
The aviation trade organization, Airlines for America, the U.S. Department of Agriculture, and the aircraft manufacturer, Boeing, established the Farm to Fly initiatives to help develop the U.S. SAF industry. Farm to Fly was later extended with the addition of the Department of Transportation’s Federal Aviation Administration (FAA) and major private partners such as the Commercial Aviation Alternative Fuels Initiative (CAAFI), as well as the Department of Energy and the Department of Defense. The new initiative, which is called Farm to Fly 2.0 (F2F2), set a goal to supply about 1 billion gallons of SAF as drop-in aviation biofuels in 2018. CAAFI kept fostering supply chain development activities in several states of the U.S. through the F2F2 Public-Private-Partnership efforts. Therefore, the industry is growing towards a sustainable commercial industry.
The year 2016 was the first year for commercial scale biofuel production; the U.S. aviation sector used over a million gallons of biofuel. The AltAir facility dominated the delivery of tallow HEFA fuel to Los Angeles Airport (LAX) for that year. There exist about 19 biofuel production facilities in the U.S., including those that are already producing biofuel for commercial usage and those that have plans to begin commercial operations soon, with an expected combined production capacity of about 1 billion gallons per year (CAAFI, 2018).
With this backdrop, airlines are looking at SAF as a feasible option for meeting the Paris Agreement and ICAO CORSIA emission goals. United Airlines started using SAF on a trial-basis for outbound flights from Los Angeles in 2016 (United Airlines, 2021), while Southwest Airlines and Alaska Airlines have established agreements with SAF producers (Csonka, 2016). However, because SAF have a higher production cost than CJF (Doliente et al., 2020) and their production capacity is still to be determined, the degree of utilization of SAF by commercial airlines and their ability to meet the emission goals is uncertain. Fuel demands of airlines may dwarf SAF production capacity and its higher production cost could increase ticket prices, which–in turn–could reduce the passenger demand and potentially hurt their bottom line, albeit lower travel demand would reduce emissions. Hence, there is a need to assess the effectiveness of introducing and utilizing SAF across commercial air transportation in achieving the carbon reduction emissions. This is relevant because the pricing of SAF, the level of introduction across the fleet of aircraft, and the fleet-level life-cycle emissions all work together to influence the utilization of SAF as an aviation fuel and aviation emissions.
Several studies using different models and analytical approaches have ventured to estimate the environmental impact of commercial aviation and the impact of potential mitigation strategies. For example, Kim et al. (Kim et al., 2007) and Lee et al. (Lee et al., 2007) used the system tool for assessing aviation’s global emission (SAGE)—commissioned by the FAA—to assess global commercial aviation fuel usages and emissions. Li et al. (2016) studied 22 airlines over the 2008–2012 period and found that European airlines have higher efficiency than non-European ones due to higher operational and business efficiency, similar to the results of European Union Emission Trading Scheme. Implementing aviation emission taxes could reduce emissions due to higher ticket fares and lower passenger demands; however, Hofer et al. (2010) show that these emission reductions can be offset as people divert to other modes of transportation. Hassan et al. (2018) provide a modeling framework that accounts for biofuel availability, fuel price, and inverse demand effects, and provide a probabilistic assessment of the achievability of CO2 targets in the US; however, the assessments date the pre-COVID era and do not take into account the sharp dip in demand in 2020.
The work summarized in this paper presents an approach that uses a more realistic operations-based model where an aircraft allocation problem is solved while satisfying passenger demand and fleet-level operational constraints for different future scenarios. The research assesses the expected fleet-wide emissions of future airline operations for various projected demand, levels of penetration/utilization of biofuels, and the price of biofuels and its impact on ticket prices. Projected demand is based on assumptions about future demand growth in an existing network of operations; levels of penetration of biofuels in airline operations are based on estimated biofuel production capacity; and prices of biofuels are based on estimations of potential future cost reductions, either through technology advancements, production capacity improvements, or competition with conventional fuels. Note that higher fuel prices are likely to affect air travel demand and reduce airline profits. We assume that new policies to either encourage or to force airlines to achieve the aforementioned emission goals will be in affect and proceed to assess the potential reduction in emissions that can be achieved if airlines abide by these regulations. The authors assess the impact of using SAF (starting in year 2020) on airline emissions using the Fleet-Level Environmental Evaluation Tool (FLEET) (Moolchandani et al., 2017), in which the biofuel and the CJF have the emission intensity 2.31 and 3.67 lb CO2-equivalent per lb consumed fuel, respectively. FLEET simulates the behavior of a profit-seeking airline and uniquely combines an airline fleet operations model with the assessment of the environmental impacts of US-touching commercial aviation. By exploring different future scenarios of SAF utilisation and travel demand, the results provide bounds on potential future fleet-level emissions and the ability of airlines to reduce emissions by the year 2050.
2 Sustainable Aviation Fuel
Sustainable Aviation Fuel (SAF) is a mixture of biofuels and CJF and has different properties depending on the type biofuel. The SAFs from different production pathways and feedstocks have different production costs and life-cycle carbon emission intensities. According to the American Society for Testing and Materials (ASTM) International Specification D7566 (Sissine, 2010), the SAF is a mixture between biomass-derived synthesized paraffinic kerosene (SPK) and the CJF. SPK usually includes biofuels based on biomass feedstocks. Although aircraft emit similar amounts of carbon emissions by using both CJF or SAFs (Stratton et al., 2010), the biomass feedstocks from SPK production pathways can capture carbon dioxide from the atmosphere. Hence, SAFs have lower carbon emission intensity than CJF when considering the life cycle of both types of fuels, which include the net carbon emissions from “well to wake” in CJF and “seed to wake” in SAF. Similarly, to assess SAF economic competitiveness, different SPK production pathways can result in various compositions of production costs, e.g. feedstock acquisition cost, feedstock transportation cost, fuel transportation cost, and bio-refinery operational cost, etc.
The blending ratio of SPKs should also be lower than 50% (Sissine, 2010). Then, the SAF production costs and life-cycle emission intensities depend on the types of SPK and the blending ratios. Doliente et al. (2020) thoroughly reviewed production costs and life-cycle emission intensities of SPKs from the HEFA, the Fischer-Tropsch production pathway (FT), and the alcohol-to-jet production pathway (ATJ). These production pathways convert different feedstocks to the SPKs for SAF production. For example, HEFA uses oils, like vegetable oil, as the feedstock; while the FT uses lignocellulosic feedstocks.
The common feedstocks for HEFA production pathways are camelina, algae, and used cooking oil (UCO). Even though camelina is not the most popular oilseed grown in the U.S., commercial airlines have used SJF developed from this feedstock (Hileman et al., 2009). For algae, the open pond approach and the photo-bio-reactor are the two most common ways to cultivate algae. The open pound approach is more attractive to the photo-bio-reactor for biofuel productions, because it requires less capital investments, operation costs, and life-cycle carbon emissions (Jorquera et al., 2010; Stephenson et al., 2010). Finally, Doliente et al. (2020) mention that the UCO has relatively low feedstock acquisition cost and will not create land competition with edible feedstock. However, the uncertainty and variability of UCO waste stream are current challenges for SAF.
At the time of this paper, HEFA-based SAF is the only SAF reaching commercial production; the AltAir facility delivered a million gallons of tallow HEFA fuel in 2019 to Los Angeles Airport (LAX) for U.S. airline operations (CAAFI, 2018). Doliente et al. (2020) also reveal that the HEFA fuels have the lowest production cost (68.70 ¢/lb), which includes the feedstock costs, among the other studied SAFs. Additionally, the HEFA fuels based on conventional oil crops have the emission intensity of 2.312 lb CO2-equivalent per lb consumed fuel (Doliente et al., 2020). For the comparison, the production cost of CJF is 26.42 ¢/lb (Doliente et al., 2020) with emission intensity of 3.775 lb CO2-equivalent per lb consumed fuel (de Jong et al., 2017). The ICAO CORSIA supporting document shows the slightly lower carbon emission intensity of conventional oil crops (Soybean, Rapeseed, and Camelina in Table 1) (ICAO, 2019). Hence, the authors also include a study to identify how the different carbon emission intensity settings might affect the evolution of future fleet-level emissions.
2.1 Future Scenarios
The work presented in this article considers multiple possibilities for biofuel market penetration levels, biofuel price, and future travel demand to create five possible scenarios of biofuel utilization (Table 2). The HEFA fuel market penetration level affects the SAF price and the carbon emission intensities. Because the biofuel industry is in its infancy, the high risk and high production costs depress the initial penetration level (Chao et al., 2019a). Additionally, due to the ASTM regulations, the penetration level of biofuel is confined to 50%. Feuvre (Le Feuvre, 2019) estimates that the SPK penetration level will be about 19% in 2040. Based on the available biofuel penetration level information, the authors consider three potential penetration level scenarios. The “Reference” penetration level case follows the prediction of Feuvre (Le Feuvre, 2019). The “Low” penetration level case assumes that the biofuel penetration increases linearly to 10% by 2050. Finally, the “High” penetration level case assumes that the penetration level follows prediction of Feuvre (Le Feuvre, 2019) until year 2030, increasing linearly to 50% by year 2050. Figure 1 shows the different biofuel penetration levels—the blue line shows the “Reference” penetration case, the grey line shows the “Low” penetration level, and the “High” penetration level is depicted by the orange line; the stair-step looking line shape represents the discrete leaps in production facilities. The 2016 Billion-Ton report conservatively estimates that the U.S. biomass can produce biofuel meeting more than 30% of 2005 U.S. petroleum consumption (Langholtz et al., 2016). Considering that the US petroleum consumption in 2019 was 61% of petroleum consumed in 2005 (Administration, 2021a), this means that using the Longholtz et al. estimates, the U.Ss biomass can produce biofuel meeting 49% of U.S. petroleum needs (30%/61% = 49%) of 2019. Because the U.S. aviation sector is responsible for about 6.5% of U.S. petroleum fuel consumption, according to U.S. Energy Information Administration (EIA) estimates for 2020 (Administration, 2021b,c), this means that there is sufficient biomass to supply the SAF needs of aviation, even at 2020 levels.
These different penetration levels lead to lower carbon emission intensities for SAF compared to CJF. The carbon emission intensity for each penetration level is calculated using Eq. 1, where the CJF emission intensity is 3.67 lb CO2-equivalent per lb consumed fuel, and the biofuel emission intensity is 2.312 lb CO2-equivalent per lb consumed fuel (Doliente et al., 2020). Figure 2 shows the SAF carbon emission intensity for the “Reference,” “High,” and “Low” penetration level cases.
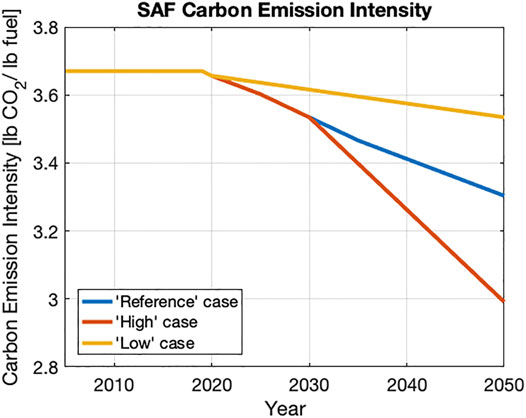
FIGURE 2. SAF carbon emission intensities for different biofuel penetration levels considered in this study.
For biofuel price, the authors consider three different pricing levels—“Reference,” “Constant,” and “Special.” The “Reference” biofuel price case assumes that the price difference between biofuel and CJF reduces linearly from the current differential to zero from years 2019–2050. The decreasing price difference reflects that the potential technology improvements and the scale of the economy reduce the biofuel production costs. The “Constant” biofuel price case assumes that the biofuel price stays constant at the 2020 value. In the “Special” biofuel price case, the authors assume that the biofuel price reduces linearly to 75¢/gallon. Figure 3 shows the CJF cost, “Reference” biofuel cost, “High” biofuel cost, and “Low” biofuel cost values in fixed 2005 U.S. dollars; the simulation used in the studies for this paper uses 2005 as the initial year. The CJF fuel price is based on U. S. Energy Information Administration, 2011 Annual Energy Outlook (U. S. Energy Information Administration, 2011).
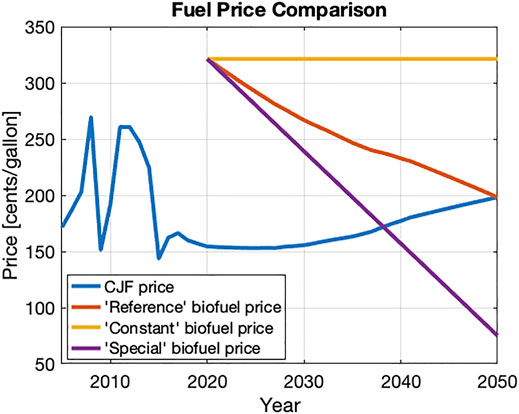
FIGURE 3. Comparison of Conventional Jet Fuel (CJF) price in FLEET with “Reference,” “Constant,” and “Special” biofuel price (in 2005 U.S. Dollars).
The authors construct a set of five scenarios using different combinations of the three biofuel price cases and the three biofuel penetration level cases (as listed in Table 2). The first three scenarios consider all possible combinations of the “Reference” biofuel price with the different biofuel penetration levels. The last two scenarios consider only the “High” penetration level, combined with “Constant” and “Special” biofuel price cases.
For each scenario, the SAF price calculation considers the biofuel price and the biofuel penetration level. Eq. (2) depicts the SAF price calculation, where the CJF price and biofuel price are adapted from Figure 3 and the penetration levels are adapted from Figure 1 based on the scenario under consideration. For example, the SAF price for scenario 1 (“Reference” biofuel price + “Reference” penetration level) in the year 2040 is given by (1−0.19)x177.12 + 0.19 × 232.7 = 187.7¢/gallon. Figure 4 shows the SAF price for all the scenarios. The SAF price for all scenarios follows the CJF price trend shown in Figure 3 for years 2005–2019 because there is no biofuel present in the fuel mix. After 2019, the SAF price deviates from CJF price trends due to the addition of biofuels in the fuel mix. Scenarios 4 and 5 lead to the highest and the lowest SAF prices in the year 2050, respectively, with the 2050 SAF prices for scenarios 1, 2, and 3 matching the 2050 CJF prices.
3 Modeling Tool—FLEET
To analyze the environmental impact of SAF on commercial airline travel, there is a need to—1) model airline operations, 2) model and project passenger demand into the future, and 3) model the introduction and use of different aircraft types into the future. Fleet-Level Environmental Evaluation Tool (FLEET) is a system dynamics-inspired simulation that combines all these models into a single tool; Figure 5 provides a representation of FLEET (Moolchandani et al., 2017).
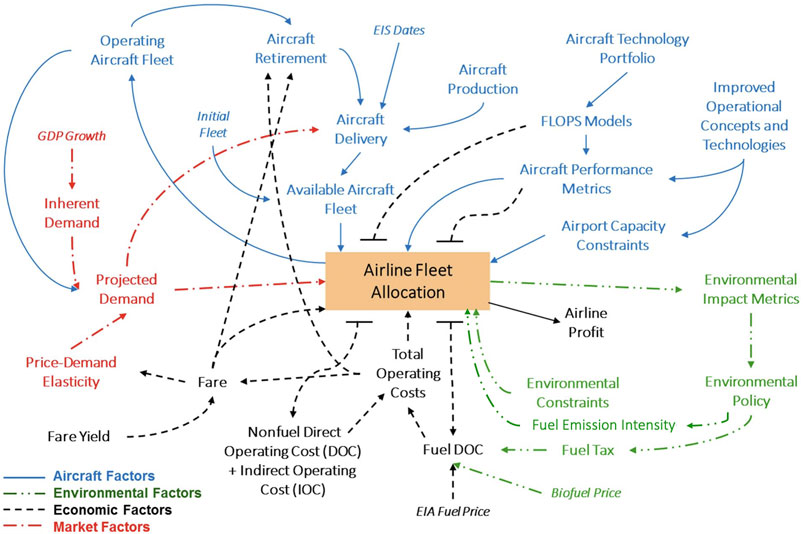
FIGURE 5. System-dynamics-inspired representation of FLEET [adapted with permission from (Moolchandani et al., 2017)].
FLEET simulation enables the prediction of the environmental impacts of commercial aviation by evolving a mix of aircraft in a notional airline’s fleet and passenger demand over time (Moolchandani et al., 2017); the primary environmental impact considered here is CO2 emissions. At the heart of FLEET is an optimization algorithm that solves an allocation problem to maximize airline profit while satisfying passenger demand and operational constraints over its route network. The tool can reflect the performance of new technology aircraft that are predicted to consume less fuel and generate less noise than current aircraft; with these aircraft models, FLEET simulates how an airline would use these new aircraft to meet passenger demand on a route network. The predicted usage of these new aircraft drives the fleet-level environmental impacts. Many studies exist that discuss the various studies conducted with FLEET considering only subsonic aircraft operations (Moolchandani et al., 2011; Moolchandani et al., 2012; Moolchandani et al., 2013; Chao, 2016; Chao et al., 2016; Chao et al., 2017; Moolchandani et al., 2017; Ogunsina et al., 2017; Ogunsina et al., 2018; Jain and Crossley, 2020; Jain et al., 2021a). Recent FLEET studies considering a mixture of supersonic and subsonic commercial aircraft in airline fleet also exist (Jain et al., 2020; Jain et al., 2021b; Mane et al., 2021).
As discussed by the authors in (Jain et al., 2021b, Jain et al., 2021a; Moolchandani et al., 2017), FLEET represents aircraft by class (based on number of seats) and by technology age. There are six different classes of subsonic aircraft in FLEET—1) Small Regional Jet (up to 50 seats), 2) Regional Jet, 3) Small Single Aisle, 4) Large Single Aisle, 5) Small Twin Aisle, and 6) Large Twin Aisle. There are four different technology ages in FLEET—1) Representative-in-class (most flown aircraft in 2005), 2) Best-in-class (aircraft with most recent entry into service dates as of 2005), 3) New-in-class (aircraft currently under development that will enter service in near future), and 4) Future-in-class (aircraft that will enter into service after new-in-class aircraft). FLEET uses year 2005 as the first year of simulation because many future goals for aviation CO2 emissions use 2005 as a reference year. Table 3 lists the subsonic aircraft available in FLEET; Mavris et al. (Mavris et al., 2017) provide details about these aircraft. These different classes and technology of aircraft are modeled using the Flight Optimization Software (FLOPS) (McCullers, 2016) and represent the mix of aircraft sizes and technologies in the airline fleet.
In the FLEET allocation problem, the notional airline could best be thought of as an aggregate airline representing all US flag carrier airlines. Jain et al. (2021a) discuss that FLEET predictions for routes and passenger demand build upon reported data from the Bureau of Transportation Statistics (BTS) (U.S Dept. of Transportation, Bureau of Transportation Statistics, 2017). For historical years, FLEET uses a dynamic route network that follows how US flag carrier airlines updated their route networks from 2005 to 2018—as reported in the BTS data. This is followed by a static route network from 2018 and beyond (i.e., FLEET does not predict the addition or deletion of routes in the future). FLEET also uses BTS reported values of historical passengers carried as the passenger demand from 2005 to 2018, followed by passenger demand predictors using economic and price factors for years 2019 and beyond. In 2018 (and all the subsequent years), there are 1,974 routes in the FLEET network that connect a subset of WWLMINET 257 airports (Kim et al., 2005). All these routes are either US domestic routes or international routes with direct flights originating or ending at a US airport, because these are the only routes that appear in the BTS database.
The FLEET simulation output provides information about the type(s) of and number of aircraft allocated to routes to meet passenger demand based on a number of scenarios. The scenarios are essentially a combination of low, nominal, and high values for aircraft technology, economic growth rate, and energy price. More details about the subsonic-only FLEET scenarios are available in (Mavris et al., 2017; Ogunsina et al., 2018). This work considers only the “Current Trends Best Guess (CTBG)” scenario of technology development and economic conditions from the previous work; this scenario comprises nominal aircraft technology development, nominal economic growth, and nominal energy price evolution.
4 Future Passenger Demand Projections
The passenger demand forecast in FLEET is modeled is a function of two factors: the demand changes due to broad economic factors, referred to here as the “inherent demand growth,” and the demand change due to passenger response to changes in ticket prices charged by the airlines, called the “elastic growth.” In the inherent demand growth model, the demand growth is a function of GDP growth, while the elastic growth model incorporates the effects of range and availability of alternative modes of transport into its calculation to determine whether demand might increase or decrease on a given route as airline ticket prices change. More information about passenger demand modeling in FLEET is available in (Moolchandani et al., 2017).
The novel coronavirus (COVID-19) pandemic has induced one of the sharpest declines in air travel demand in aviation history; full-year global passenger traffic results from both the International Air Transport Association (IATA) and the International Civil Aviation Organization (ICAO) indicate that 2020 was the worst year in the history for air travel demand (IATA, 2021a; Hasegawa, 2021). There is an uncertainty about how the air travel demand recovery will look like in the near future, with complete demand recovery to pre-COVID-19 levels (2019) expected by year 2023 or 2024 (IATA, 2021b; Pearce, 2021), depending on the continuation of travel restrictions imposed world-wide due to the spread of more contagious COVID-19 variants.
To account for the impact of the COVID-19 pandemic on future passenger demand, the authors consider two different future demand projection scenarios for this article. These demand projection scenarios assume airline operations recovery to pre-COVID-19 (2019) levels in year 2023 and 2024, along with variations in the GDP growth rates–starting from the year of passenger demand recovery to 2019 levels to the year 2030. The two demand projection scenarios considered here are a subset of the six scenarios identified by the authors in (Jain et al., 2021a). Table 4 summarizes all six future demand scenarios; the authors only consider two scenarios marked with red font in this article—scenario 1 (“2023 recovery”) and scenario 5 (“2024 recovery + GDP slowdown to 75% until 2030”). In the table, the passenger demand for different years is listed as a percentage of pre-COVID-19 levels (2019) and the GDP growth rate is listed as a percentage of the “Nominal” GDP growth rate in FLEET (Moolchandani et al., 2017; Mavris et al., 2017). The total passenger demand in 2020 for all scenarios is set to be 34% of the passenger demand levels in 2019, signifying a 66% drop in total passenger demand (IATA, 2021b; Jain et al., 2021a). Figure 6 shows the historical and projected demand in FLEET for the two projected demand scenarios in consideration (Jain et al., 2021a).
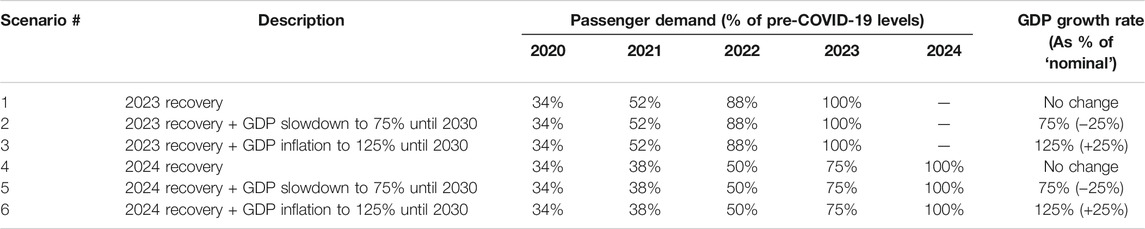
TABLE 4. Future demand projection scenarios; the ones marked in red font are considered in this study (Jain et al., 2021a).
The “2023 recovery” scenario is the primary scenario for this work; this represents an optimistic view that the airline operations will recover to pre-COVID-19 (2019) levels by 2023, with minimal impact on GDP growth. The total passenger demand is set to recover to 52% of pre-COVID-19 levels by 2021, 88% of pre-COVID-19 levels by 2022, and 100% of pre-COVID-19 levels by 2023 (Jain et al., 2021a; IATA, 2021b), along with the assumption that the passenger demand in FLEET continues to grow based on FLEET’s GDP growth rate beyond 2023. The “2024 recovery + GDP slowdown to 75% until 2030” acts as an additional scenario for this work, taking into account the possibility of lower passenger demand recovery due to the spread of new COVID-19 variants. Also, this scenario assumes that the passenger demand grows at 75% of FLEET’s GDP growth rate until year 2030, representing the worst case scenario for future passenger demand growth. The total passenger demand is set to recover to 38% of pre-COVID-19 levels by 2021 (IATA, 2021a), 50% of pre-COVID-19 levels by 2022, 75% of pre-COVID-19 levels by 2023, and to pre-COVID-19 levels by 2024 (Jain et al., 2021a).
5 Results
The FLEET simulation is run from years 2005–2050. The results presented here use the previously developed “Current Trends Best Guess (CTBG)” scenario (Mavris et al., 2017) as the baseline scenario, using the subsonic CTBG results (with no SAF) for comparing the current results. The five future SAF scenarios (discussed in Section 2.1) are input into FLEET to estimate the changes in fleet-level CO2 emissions and airline operations with the introduction of SAF to the airline fleet in year 2020. As mentioned above, this article considers two future passenger demand projection scenarios—“2023 recovery” and “2024 recovery + GDP slowdown to 75% until 2030”—this leads to a total of ten scenarios. The authors consider the “2023 recovery” scenario to be the primary simulation scenario, with the “2024 recovery + GDP slowdown to 75% until 2030” scenario acting as an additional scenario that simulates changes in CO2 emissions when SAF are introduced considering the worst case passenger demand growth.
5.1 Primary Simulation Scenario (2023 Passenger Demand Recovery)
This subsection presents FLEET simulation results considering the “2023 recovery” passenger demand projection—recovery to pre-COVID-19 (2019) levels by 2023, with no impact on GDP growth. Figure 7 shows the normalized CO2 emissions predicted by FLEET for the SAF scenarios along with the no SAF baseline scenario. As visible in the figure, there is a slump in fleet-level CO2 emissions in the year 2020 due to the COVID-19 pandemic-related travel restrictions. The fleet-level emissions for all five SAF scenarios are always lower than the no-SAF baseline scenario. The minimum reduction in 2050 fleet-level CO2 emissions is 4.4% (for scenario 3) and the maximum reduction is 22.5% (for scenario 4). With the current modeling, FLEET simulation results show that the fleet-level CO2 emissions could go below 2005 levels if scenario 2 (“Reference” biofuel price + “High” penetration level), scenario 4 (“Constant” biofuel price + “High” penetration level), or scenario 5 (“Special” biofuel price + “High” penetration level) were to materialize in reality; these are depicted by green, red, and purple color solid lines, respectively, in Figure 7 (and in all subsequent figures in this section).
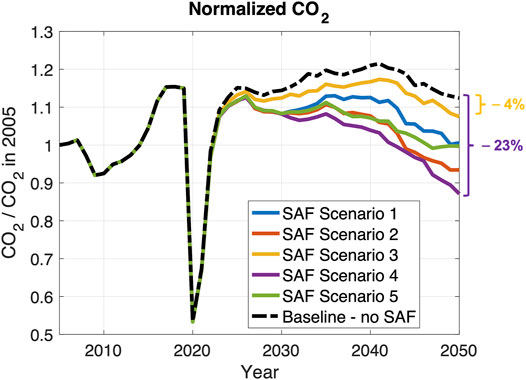
FIGURE 7. Normalized fleet-level CO2 emissions for SAF scenarios (considering passenger demand recovery to pre-COVID-19 levels by 2023); biofuel emission intensity: 2.312 lb CO2-equivalent per lb consumed fuel; CJF emission intensity: 3.67 lb CO2-equivalent per lb consumed fuel.
Perhaps not surprisingly, FLEET predictions show that the scenario with the highest SAF price (refer to Figure 4)—scenario 4 (“Constant” biofuel price + “High” penetration level)—leads to the lowest CO2 emissions. This reduction in emissions can be explained by looking at the passenger demand served and the trips flown in scenario 4. The high SAF price leads to an increase in the airline ticket prices, which causes the air travel demand to shrink, leading to lesser trips and subsequently lesser emissions. Figures 8A,B show the normalized passenger demand and trips flown, respectively, for the SAF scenarios along with the baseline scenario. There is a 3.7% reduction in the 2050 passenger demand for scenario 4 and a 5.5% reduction in the 2050 trips flown compared to the baseline scenario (depicted by purple solid line in Figures 8A,B), indicating that the reduced emissions are a combination of using SAF and the consequent reduction in the number of passengers and trips flown by the airline.
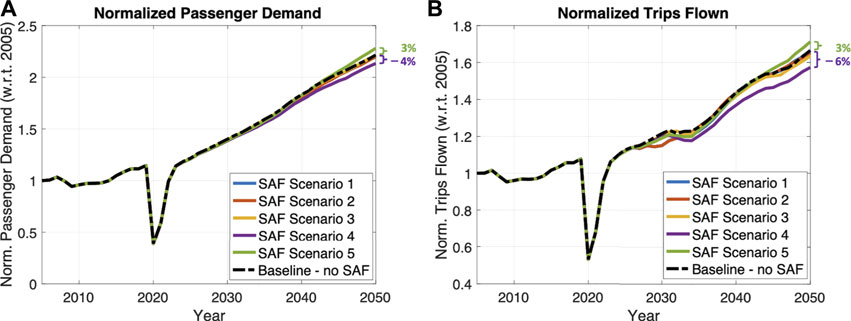
FIGURE 8. (A) Normalized passenger demand, (B) Normalized trips flown, for SAF scenarios (considering passenger demand recovery to pre-COVID-19 levels by 2023).
Similarly, the scenario with the lowest SAF price—scenario 5 (“Special” biofuel price + “High” penetration level)—leads to CO2 emissions that are higher than the other scenarios with “High” biofuel penetration levels, i.e., scenarios 2 and 4. The reason for this behavior can be traced back to the increased passenger demand (3.0%) and trips flown (2.9%) by the airline for scenario 5 (depicted by green solid line in Figures 8A,B); the reduced SAF prices lead to lower ticket prices, causing a surge in air travel demand, leading to more trips and, subsequently, more emissions.
The authors note that scenarios with ‘High’ biofuel penetration levels lead to a higher reduction in the fleet-level CO2 emissions, followed by scenarios with “Reference” and “Low” biofuel penetration levels. This indicates that higher biofuel penetration levels could lead to lower fleet-level CO2 emissions, even if the airline ends up serving higher passenger demand. The FLEET-predicted maximum 22.5% CO2 reduction by 2050 relative to the non-SAF baseline from introducing SAF and the price-elastic demand effects of SAF, while for the US-touching based network and airlines discussed above, is notably lower than the cumulative 46% predicted for European Aviation by (van der Sman et al., 2021).
5.2 Additional Simulation Scenario (2024 Passenger Demand Recovery With GDP Slowdown to 75%)
This subsection talks about FLEET simulation results considering the “2024 recovery + GDP slowdown to 75% until 2030” passenger demand projection—recovery to pre-COVID-19 (2019) levels by 2024 (a year later than previous demand projection), with GDP slowdown to 75% until year 2030. Figure 9 shows the normalized CO2 emissions for the SAF scenarios along with the no SAF baseline scenario in FLEET. As with the previous set of results, the emissions from all five scenarios are always lower than the baseline scenario, with a minimum reduction of 1.9% (for scenario 3) and a maximum reduction of 23.4% (for scenario 4) in 2050 fleet-level CO2 emissions.
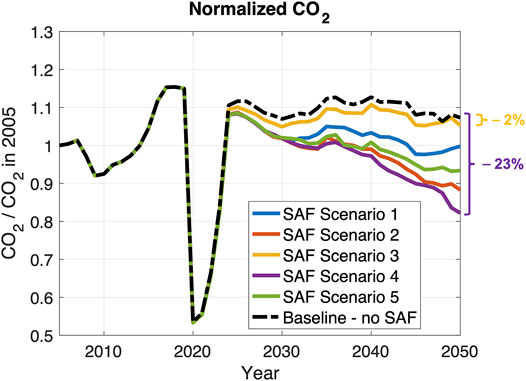
FIGURE 9. Normalized fleet-level CO2 emissions for SAF scenarios (considering passenger demand recovery to pre-COVID-19 levels by 2024 and GDP slowdown to 75% until 2030); biofuel emission intensity: 2.312 lb CO2-equivalent per lb consumed fuel; CJF emission intensity: 3.67 lb CO2-equivalent per lb consumed fuel.
With the current modeling, FLEET simulation results show that the fleet-level CO2 emissions could go below 2005 levels if four out of the five SAF scenarios were to materialize in reality (compared to the only three for the demand projections considered in Section 5.1)—scenario 1 (“Reference” biofuel price + “Reference” penetration level), scenario 2 (“Reference” biofuel price + “High” penetration level), scenario 4 (“Constant” biofuel price + “High” penetration level), and scenario 5 (“Special” biofuel price + “High” penetration level). For the current demand projection scenario, the authors note that 2005 emission levels (or lower) could be achieved for “High” and “Reference” biofuel penetration levels. For the previous demand projection case, these levels could only be obtained using the “high” biofuel penetration level.
Similar to the previous set of results, the airline ends up serving the highest demand for the SAF scenario with lowest SAF price (scenario 5), leading to CO2 emissions that are higher than the other scenarios with “High” biofuel penetration levels, i.e., scenarios 2 and 4. Figure 10 shows the normalized passenger demand and trips flown for the SAF scenarios along with the baseline scenario. For scenario 5, there is a 3.1% increase in 2050 passenger demand and a 3.4% increase in trips flown by the airline compared to the baseline scenario. Scenario 4 leads to the lowest fleet-level CO2 emissions due a combination of using SAF and a 3.7% reduction in the passenger demand served (along with a 7.5% reduction in trips flown), depicted the purple solid lines in Figure 10.
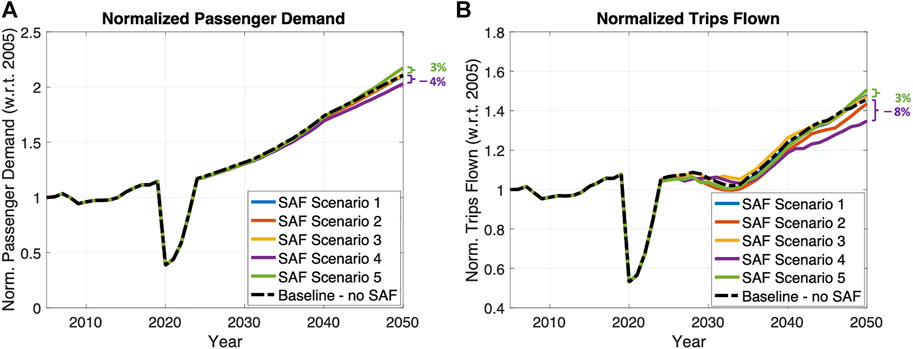
FIGURE 10. (A) Normalized passenger demand, (B) Normalized trips flown, for SAF scenarios (considering passenger demand recovery to pre-COVID-19 levels by 2024 and GDP slowdown to 75% until 2030).
Interestingly, the predictions show that a delay in the passenger demand recovery from COVID-19 (recovery in 2024) due to extended travel restrictions and a GDP slowdown until 2030 could lead to lower overall fleet-level emissions (comparing Figures 7, 9). The widened gap in passenger demand recovery after the demand slump in 2020—visible when comparing Figure 10A with Figure 8A—contributes positively to CO2 emission reductions, and the usage of SAF instead of CJF helps to pull down emissions even further.
5.3 Biofuel Alternatives
The results presented in Sections 5.1, 5.2 are based on a biofuel emission intensity of 2.312 lb CO2-equivalent per lb consumed fuel (based on HEFA oil crops in (Doliente et al., 2020)) and a CJF emission intensity of 3.67 lb CO2-equivalent per lb consumed fuel. The authors note that the biofuel and CJF emission intensities vary from study to study, making it a good parameter for sensitivity analysis. For this sensitivity study, two biofuel emission intensity values are considered—) 1.87 lb CO2-equivalent per lb consumed fuel—this is an average value for HEFA oil crops (Soybean, Rapeseed, and Camelina; shown in Table 1) based on (ICAO, 2019), and 2) 0.60 lb CO2-equivalent per lb consumed fuel—value for used cooking oil based on (ICAO, 2019). These emission intensities are chosen so that they can help us set bounds on future fleet-level CO2 emissions, with the assumption that the biofuel cost evolution stays the same for all the different biofuels considered here. The CJF emission intensity is also updated to 3.775 lb CO2-equivalent per lb consumed fuel based on (de Jong et al., 2017).
Figure 11 shows the normalized CO2 emissions for all five SAF scenarios while considering different biofuel emission intensities (2.312, 1.87, and 0.6 lb CO2-equivalent per lb consumed fuel) and different future demand growth projections (“2023 recovery” and “2024 recovery + GDP slowdown to 75% until 2030”). The figure clearly shows the impact of biofuel selection and demand projection on future aviation emissions. As expected, reductions in biofuel emission intensity and future passenger demand could lead to lower emissions. Scenario 4 (“Constant” biofuel price + “High” penetration level)—shown in Figure 11D—leads to the lowest emissions among all scenarios, with a maximum possible reduction of 48% for the case with biofuel emission intensity of 0.6 lb CO2-equivalent per lb consumed fuel (used cooking oil used as biofuel) and passenger demand recovery in 2024 (with GDP slowdown to 75% until 2030).
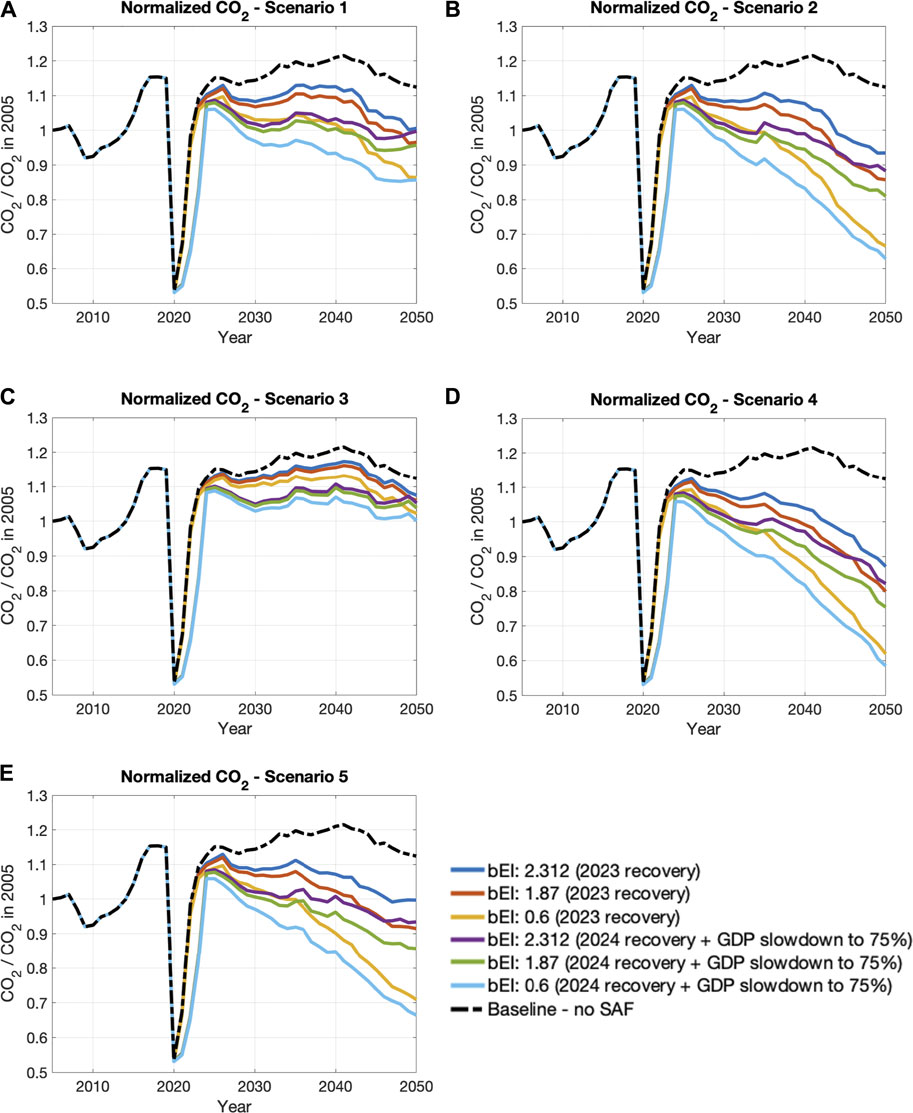
FIGURE 11. Normalized fleet-level CO2 emissions for different SAF scenarios considering multiple biofuel emission intensities and future demand growth projections – (A) Scenario 1: reference biofuel price, reference penetration level, (B) Scenario 2: reference biofuel price, high penetration level, (C) Scenario 3: reference biofuel price, low penetration level, (D) Scenario 4: constant biofuel price, high penetration level, (E) Scenario 5: special biofuel price, high penetration level; ‘bEI’ refers to ‘biofuel emission intensity’ (in lb CO2-equivalent per lb consumed fuel.
6 Conclusion
This article discusses the possible impact of using SAF on fleet-level CO2 emissions and airline operations, while taking into account the air travel demand disruption due to the COVID-19 pandemic and various possibilities of the introduction and use of biofuels by airlines. The authors consider five SAF scenarios (listed in Table 2) along with two COVID-19-related demand projection scenarios (listed using red font in Table 4). The SAF scenarios are based on a combination of different biofuel prices and different biofuel penetration levels; the future demand projection scenarios use a combination of different passenger demand recovery possibilities and different GDP growth rates. The authors used FLEET to model the behavior of a profit-seeking airline for different SAF and projected demand scenarios and estimate changes in future fleet-level CO2 emissions, along with predicting the future passenger demand and trips flown. In addition, because of the numerous sources of biofuel, the study explores the potential future emission levels if any of these biofuels were used by airlines at the assumed penetration levels and prices.
The results indicate that the introduction SAF for use in airline fleets and the projected demand scenarios could notably impact the future fleet-level aviation CO2 emissions. Considering a biofuel emission intensity of 2.312 lb CO2-equivalent per lb consumed fuel, the total CO2 emissions from all five SAF scenarios are always lower than the no-SAF baseline scenario, for both the COVID-19-related projected demand scenarios. For the “2023 recovery” scenario, a minimum of 4.4% reduction (for scenario 3) and a maximum of 22.5% reduction (for scenario 4) is possible in the 2050 fleet-level emissions. For the “2024 recovery + GDP slowdown to 75% until 2030” scenario, the maximum possible reduction in fleet-level emissions is higher—23.4%, but the minimum possible reduction is lower—1.9%; the late recovery of passenger demand to pre-COVID-19 levels along with a GDP slowdown until year 2030 causes the emissions from the no-SAF baseline case to decrease, diminishing the benefits of using SAF with “Low” biofuel penetration levels. However, when FLEET evaluates SAF usage with “Reference” and “High” biofuel penetration levels, the predicted benefits of SAF are amplified, leading to even lower future emissions when compared to the “2023 recovery” scenario.
The authors note that the SAF scenarios with low SAF price lead to higher fleet-level emissions for both future demand projections scenarios; this happens because low fuel prices lead to low ticket prices, which causes a surge in demand, and the airline ends up flying more trips—leading to higher emissions. For SAF scenarios with high SAF price, the opposite occurs—high fuel prices push ticket prices up, shrinking demand, causing the airline to fly lesser number of trips, leading to lower fleet-level emissions.
Looking at the biofuel penetration levels, current modeling suggests that the “High” penetration level leads to 2050 emissions that are lower than the 2005 emissions levels, for both future demand projections scenarios. For the “2024 recovery + GDP slowdown to 75% until 2030” scenario, the 2050 emissions from the “Reference” penetration level are also lower than the 2005 emission levels. These results show that the reason for the reduction in fleet-level emissions for the SAF scenarios is a combination of the reduced overall CO2 emissions from using SAF and reduced passenger demand (and hindered demand growth for one of the COVID-19-related demand scenarios).
Additionally, the type of biofuel selected (and subsequently its carbon emission intensity) also impacts the future aviation emissions; biofuels with lower carbon emission intensities lead to lower emissions. The reduction in emissions could be as high as 48% compared to the baseline scenario with no SAF, when using a biofuel with an emission intensity of 0.6 lb CO2-equivalent per lb consumed fuel (used cooking oil used as biofuel) along with passenger demand recovery in 2024 (with GDP slowdown to 75% until 2030).
The CO2 emission predictions presented in this work—considering five scenarios combining different biofuel prices and biofuel penetration levels—show that future emissions can decrease when SAF with high biofuel penetration levels are introduced for use in airline fleets. The results do not intend to show the exact CO2 emission levels, but provide upper and lower bounds on possible future aviation emissions. As expected, not introducing SAF will lead to the highest CO2 emissions possible, followed by introducing SAF with “Low” penetration levels; these scenarios could act as the upper bounds for without SAF and with SAF future aviation emission predictions, respectively. With the current modeling, introducing SAF with “High” penetration levels could lead to the lowest possible emissions, serving as the “best case scenario” for future aviation CO2 emissions.
Data Availability Statement
The raw data supporting the conclusion of this article will be made available by the authors upon request, without undue reservation.
Author Contributions
SJ was the lead author, providing the overall outline for the article and conducting most of the FLEET simulations. HC provided inputs for the paper and for FLEET regarding SAF costs and penetration rates. MM provided major revisions and editing oversight for the article and developed demand scenarios reflecting COVID-19 impacts. WC provided final revisions to the article and oversight of the FLEET simulation tool. DD also provided reviews of the article and oversight of the FLEET simulation tool.
Funding
The open access publication fees are provided from discretionary funds of the authors generated at Purdue University.
Conflict of Interest
The authors declare that the research was conducted in the absence of any commercial or financial relationships that could be construed as a potential conflict of interest.
Publisher’s Note
All claims expressed in this article are solely those of the authors and do not necessarily represent those of their affiliated organizations, or those of the publisher, the editors and the reviewers. Any product that may be evaluated in this article, or claim that may be made by its manufacturer, is not guaranteed or endorsed by the publisher.
References
Administration, T. U. E. I. (2021a). Adjusted Distillate Fuel Oil Sales for Residential Use. Dataset.
Administration, T. U. E. I. (2021c). Use of Energy for Transportation - U.S. Energy Information Administration. EIA. Dataset.
Administration, T. U. E. I. (2021b). U.S. Fossil Fuel Consumption Fell by 9nearly 30 Years - Today in Energy - U.S. Energy Information Administration. EIA. Dataset.
CAAFI (2018). Alternative Jet Fuel Production Facilities Status. Commercial Aviation Alternative Fuels Initiative (CAAFI), Available at: http://www.caafi.org/focus_areas/docs/Alternative_Jet_Fuel_Production_Facilities_Status.pdf (Accessed June 2018).
Chao, H., Agusdinata, D. B., and DeLaurentis, D. A. (2019a). The Potential Impacts of Emissions Trading Scheme and Biofuel Options to Carbon Emissions of U.S. Airlines. Energy Policy 134, 110993. doi:10.1016/j.enpol.2019.110993
Chao, H., Agusdinata, D. B., and DeLaurentis, D. A. (2019c). The Potential Impacts of Emissions Trading Scheme and Biofuel Options to Carbon Emissions of U.S. Airlines. Energy Policy 134, 110993. doi:10.1016/J.ENPOL.2019.110993
Chao, H., Agusdinata, D. B., DeLaurentis, D., and Stechel, E. B. (2019b). Carbon Offsetting and Reduction Scheme with Sustainable Aviation Fuel Options: Fleet-Level Carbon Emissions Impacts for U.S. Airlines. Transportation Res. D: Transport Environ. 75, 42–56. doi:10.1016/j.trd.2019.08.015
Chao, H., DeLaurentis, D., and Agusdinata, B. (2017). “Sensitivity Analysis of Fleet-Level Life Cycle Carbon Emissions to Biofuel Options and Emission Policy Schemes for the U.S. Commercial Airlines,” in 17th AIAA Aviation Technology, Integration, and Operations Conference. doi:10.2514/6.2017-3768
Chao, H. (2016). Fleet Level Environmental Evaluation of Emission Taxing Scheme and Biofuel: A Combined Optimization and Multi-Actor ApproachMaster’s Thesis. West Lafayette, Indiana: Purdue University, School of Aeronautics and Astronautics. URL: https://docs.lib.purdue.edu/open_access_theses/932.
Chao, H., Kolencherry, N., Ogunsina, K., Moolchandani, K., Crossley, W. A., and DeLaurentis, D. A. (2017). “A Model of Aircraft Retirement and Acquisition Decisions Based on Net Present Value Calculations,” in 17th AIAA Aviation Technology, Integration, and Operations Conference. doi:10.2514/6.2017-3600
Chao, H., Ogunsina, K. E., Moolchandani, K., DeLaurentis, D. A., and Crossley, W. A. (2016). “Airline Competition in Duopoly Market and its Impact on Environmental Emissions: A Game Theory Approach,” in 16th AIAA Aviation Technology, Integration, and Operations Conference, Reston, Virginia (American Institute of Aeronautics and Astronautics). doi:10.2514/6.2016-3759
Csonka, S. (2016). “CAAFI: Ten Years and Growing! (Opening Remarks),” in 5th CAAFI Biennial General Meeting, October 25, 2016. Washington, DC: Commercial Aviation Alternative Fuels Initiative. URL: https://caafi.org/information/pdf/Biennial_Meeting_Oct252016_Opening_Remarks.pdf.
de Jong, S., Antonissen, K., Hoefnagels, R., Lonza, L., Wang, M., Faaij, A., et al. (20172017). Life-cycle Analysis of Greenhouse Gas Emissions from Renewable Jet Fuel Production. Biotechnol. Biofuels 10 (1 10), 1–18. doi:10.1186/S13068-017-0739-7
Doliente, S. S., Narayan, A., Tapia, J. F. D., Samsatli, N. J., Zhao, Y., and Samsatli, S. (2020). Bio-aviation Fuel: A Comprehensive Review and Analysis of the Supply Chain Components. Front. Energ. Res. 8, 110. doi:10.3389/fenrg.2020.00110
Haller, B. (2012). Overview of Subsonic Fixed Wing Project: Technical Challenges for Energy Efficient, Environmentally Compatible Subsonic Transport Aircraft. Tech. Lead Syst. Anal. 1–23.
Hasegawa, T. (2021). Effects of Novel Coronavirus (Covid-19) on Civil Aviation: Economic Impact Analysis. ICAO Economic Impacts of COVID-19 on Civil Aviation. Available at: https://www.icao.int/sustainability/Documents/COVID-19/ICAO COVID 2021 06 23 Economic Impact TH Toru.pdf (Accessed 22-June-2021).
Hassan, M., Pfaender, H., and Mavris, D. (2018). Probabilistic Assessment of Aviation Co2 Emission Targets. Transportation Res. Part D: Transport Environ. 63, 362–376. doi:10.1016/j.trd.2018.06.006
Hileman, J. I., Wong, H. M., Waitz, I. A., Ortiz, D. S., Bartis, J. T., Weiss, M. A., et al. (2009). Near-Term Feasibility of Alternative Jet Fuels. Santa Monica: Aviation.
Hofer, C., Dresner, M. E., and Windle, R. J. (2010). The Environmental Effects of Airline Carbon Emissions Taxation in the US. Transportation Res. Part D: Transport Environ., 15, 37–45. doi:10.1016/j.trd.2009.07.001
IATA (2021a). 2020 Worst Year in History for Air Travel Demand. Geneva: IATA Press Release. Available at: https://www.iata.org/en/pressroom/pr/2021-02-03-02/(Accessed February 3, 2021).
IATA (2016). Offsetting CO2 Emissions with Corsia. International Air Transport Association. Geneva: IATA. Available at: https://www.iata.org/en/programs/environment/corsia/(accessed Aug, 2021).
IATA (2021b). Optimism when Borders Reopen, 33. Geneva: IATA Press Release. Available at: https://www.iata.org/en/pressroom/pr/2021-05-26-01/(Accessed May 26, 2021).
ICAO (2019). CORSIA SUPPORTING DOCUMENT, CORSIA Eligible Fuels-Life Cycle Assessment Methodology. Tech. rep. Montreal: ICAO.
Jain, S., and Crossley, W. (2020). “Predicting Fleet-Level Carbon Emission Reductions from Future Single-Aisle Hybrid Electric Aircraft,” in AIAA Propulsion and Energy 2020 Forum. doi:10.2514/6.2020-3554
Jain, S., Mane, M., Chao, H., Crossley, W. A., and DeLaurentis, D. A. (2021a). “Estimating the Impact of Novel Coronavirus (Covid-19) on Future Fleet-Level Co2 Emissions and Airline Operations,” in 21st AIAA Aviation Technology, Integration, and Operations Conference (Reston: AIAA AVIATION).
Jain, S., Mane, M., Crossley, W. A., and DeLaurentis, D. A. (2021b). “Investigating How Commercial Supersonic Aircraft Operations Might Impact Subsonic Operations and Total Co2 Emissions,” in 21st AIAA Aviation Technology, Integration, and Operations Conference (Reston: AIAA AVIATION).
Jain, S., Ogunsina, K. E., Chao, H., Crossley, W. A., and DeLaurentis, D. A. (2020). Predicting Routes for, Number of Operations of, and Fleet-Level Impacts of Future Commercial Supersonic Aircraft on Routes Touching the United States. doi:10.2514/6.2020-2878
Jorquera, O., Kiperstok, A., Sales, E. A., Embiruçu, M., and Ghirardi, M. L. (2010). Comparative Energy Life-Cycle Analyses of Microalgal Biomass Production in Open Ponds and Photobioreactors. Bioresour. Tech. 101, 1406–1413. doi:10.1016/J.BIORTECH.2009.09.038
Kim, B., Fleming, G. G., Balasubramanian, S. N., Malwitz, A., Lee, J., Waitz, I. A., et al. (2005). System for Assessing Aviation’s Global Emissions. version 1.5. Washington, DC: SAGE. technical manual. Tech. Rep. DOT-VNTSC-FAA-05-14. URL: https://rosap.ntl.bts.gov/view/dot/8909.
Kim, B. Y., Fleming, G. G., Lee, J. J., Waitz, I. A., Clarke, J.-P., Balasubramanian, S., et al. (2007). System for Assessing Aviation's Global Emissions (SAGE), Part 1: Model Description and Inventory Results. Transportation Res. Part D: Transport Environ. 12, 325–346. doi:10.1016/J.TRD.2007.03.007
Langholtz, M. H., Stokes, B. J., and Eaton, L. M. (2016). BILLION-TON REPORT Advancing Domestic Resources for a Thriving Bioeconomy, 1160. Washington, DC: Oak Ridge National Laboratory, 2016.
Le Feuvre, P. (2019). Are Aviation Biofuels Ready for Take off? International Energy Agency. Commentary. Paris: IEA. Available at: https://www.iea.org/commentaries/are-aviation-biofuels-ready-for-take-off (Accessed March 18, 2019).
Lee, J. J., Waitz, I. A., Kim, B. Y., Fleming, G. G., Maurice, L., and Holsclaw, C. A. (2007). System for Assessing Aviation's Global Emissions (SAGE), Part 2: Uncertainty Assessment. Transportation Res. Part D: Transport Environ. 12, 381–395. doi:10.1016/J.TRD.2007.03.006
Li, Y., Wang, Y.-z., and Cui, Q. (2016). Has Airline Efficiency Affected by the Inclusion of Aviation into European Union Emission Trading Scheme? Evidences from 22 Airlines during 2008-2012. Energy, 96, 8–22. doi:10.1016/j.energy.2015.12.039
Mane, M., Jain, S., Crossley, W. A., and DeLaurentis, D. A. (2021). “Speed and Cost Impact on Market success of Supersonic Passenger Transport Aircraft,” in 21st AIAA Aviation Technology, Integration, and Operations Conference (Reston: AIAA AVIATION).
Mane, M., Tetzloff, I., Crossley, W., Agusdinata, D., and DeLaurentis, D. (2011). “Impact of Development Rates of Future Aircraft Technologies on Fleet-wide Environmental Emissions,” in 11th AIAA Aviation Technology, Integration, and Operations (ATIO) Conference, 1–13. doi:10.2514/6.2011-6843
Mavris, D., Delaurentis, D., Crossley, W., and Alonso, J. J. (2017). Project 10 Aircraft Technology Modeling and Assessment: Phase I Report.
McCullers, L. (2016). Flight Optimization Software FLOPS v.9, Software Package. NASA Langley Research Center, Hampton, VA, release 9.0.0 edn. URL: https://software.nasa.gov/software/LAR-18934-1.
Moolchandani, K. A., Agusdinata, D. B., DeLaurentis, D. A., and Crossley, W. A. (2012). “Airline Competition in Duopoly Market and its Impact on Environmental Emissions,” in 12th AIAA Aviation Technology, Integration, and Operations (ATIO) Conference, 1–11. doi:10.2514/6.2012-5466
Moolchandani, K., Agusdinata, D. B., Mane, M., DeLaurentis, D., and Crossley, W. (2013). “Assessment of the Effect of Aircraft Technological Advancement on Aviation Environmental Impacts,” in 51st AIAA Aerospace Sciences Meeting including the New Horizons Forum and Aerospace Exposition, Reston, Virigina (American Institute of Aeronautics and Astronautics). 13. doi:10.2514/6.2013-652
Moolchandani, K., Govindaraju, P., Roy, S., Crossley, W. A., and DeLaurentis, D. A. (2017). Assessing Effects of Aircraft and Fuel Technology Advancement on Select Aviation Environmental Impacts. J. Aircraft 54, 857–869. doi:10.2514/1.C033861
Ogunsina, K., Chao, H., Kolencherry, N., Jain, S., Moolchandani, K., Crossley, W. A., et al. (2018). “Fleet-level Environmental Assessments for Feasibility of Aviation Emission Reduction Goals,” in Proceedings of CESUN Global Conference, Tokyo, Japan.
Pearce, B. (2021).Covid-19: An Almost Full Recovery of Air Travel in prospect. IATA Economic Reports, Available at: https://www.iata.org/en/iata-repository/publications/economic-reports/an-almost-full-recovery-of-air-travel-in-prospect/(Accessed May 26, 2021).
Ritchie, H. (2020). Climate Change and Flying: what Share of Global CO2 Emissions Come from Aviation? Published online at OurWorldInData.org. Available at: https://ourworldindata.org/co2-emissions-from-aviation.
Sissine, F. (2010). Renewable Fuel Standard Program (RFS2) Regulatory Impact Analysis.Tech. rep.. Washington, DC.
Stephenson, A. L., Kazamia, E., Dennis, J. S., Howe, C. J., Scott, S. A., and Smith, A. G. (2010). Life-Cycle Assessment of Potential Algal Biodiesel Production in the United Kingdom: A Comparison of Raceways and Air-Lift Tubular Bioreactors. Energy Fuels 24, 4062–4077. doi:10.1021/ef1003123
Stratton, R. W., Min Wong, H., and Hileman, J. I. (2010). Life Cycle Greenhouse Gas Emissions from Alternative. Jet Fuels 571, 1–133. PARTNER-COE-2010-001.
Sun, H., Edziah, B. K., Kporsu, A. K., Sarkodie, S. A., and Taghizadeh-Hesary, F. (2021). Energy Efficiency: The Role of Technological Innovation and Knowledge Spillover. Technol. Forecast. Soc. Change 167, 120659. doi:10.1016/j.techfore.2021.120659
Sun, H., Kporsu, A. K., Taghizadeh-Hesary, F., and Edziah, B. K. (2020). Estimating Environmental Efficiency and Convergence: 1980 to 2016. Energy 208, 118224. doi:10.1016/j.energy.2020.118224
The White House (2021). FACT SHEET: President Biden Sets 2030 Greenhouse Gas Pollution Reduction Target Aimed at Creating Good-Paying Union Jobs and Securing U.S. Leadership on Clean Energy Technologies. The White House Statements and Releases, Available at: https://www.whitehouse.gov/briefing-room/statements-releases/2021/04/22/fact-sheet-president-biden-sets-2030-greenhouse-gas-pollution-reduction-target-aimed-at-creating-good-paying-union-jobs-and-securing-u-s-leadership-on-clean-energy-technologies/(Accessed April 22, 2021).
United Airlines (2021). Sustainable Fuel Sources. Available at: https://www.united.com/ual/en/us/fly/company/global-citizenship/environment/sustainable-fuel-sources.html.
United Nations Framework Convention on Climate Change (2021). The Paris Agreement. Available at: https://unfccc.int/process-and-meetings/the-paris-agreement/the-paris-agreement.
U.S Dept. of Transportation, Bureau of Transportation Statistics (2017). Airline Origin and Destination Survey (DB1B) Available at: http://www.transtats.bts.gov/DatabaseInfo.asp?DB_ID=125&Link=0, Accessed Dec. 2017. [Dataset].
U.S. Department of State, Office of the Spokesperson (2021). U.S.-China Joint Statement Addressing the Climate Crisis - United States Department of State. Media Note Available at: https://www.state.gov/u-s-china-joint-statement-addressing-the-climate-crisis/(Accessed April 17, 2021).
U. S. Energy Information Administration (2011). Annual Energy Outlook 2011. Available at: www.eia.gov/forecasts/aeo/, Accessed Oct, 2016. [Dataset].
van der Sman, E., Peerlings, B., Kos, J., Lieshout, R., and Boonekamp, T. (2021). Destination 2050–A Route to Net Zero European Aviation. Tech. Rep. NLR-CR-2020-510, Netherlands Aerospace Centre NLR. Available at: https://reports.nlr.nl/handle/10921/1555/.
Keywords: commercial aviation CO2 emissions, sustainable aviation fuels (SAF), airline fleet-level predictions, future aviation CO2 scenarios, model-based prediction method
Citation: Jain S, Chao H, Mane M, Crossley WA and DeLaurentis DA (2021) Estimating the Reduction in Future Fleet-Level CO2 Emissions From Sustainable Aviation Fuel. Front. Energy Res. 9:771705. doi: 10.3389/fenrg.2021.771705
Received: 06 September 2021; Accepted: 29 October 2021;
Published: 29 November 2021.
Edited by:
Kristin C. Lewis, Volpe National Transportation Systems Center, United StatesReviewed by:
Huaping Sun, Jiangsu University, ChinaMohammed Hassan, Georgia Institute of Technology, United States
Copyright © 2021 Jain, Chao, Mane, Crossley and DeLaurentis. This is an open-access article distributed under the terms of the Creative Commons Attribution License (CC BY). The use, distribution or reproduction in other forums is permitted, provided the original author(s) and the copyright owner(s) are credited and that the original publication in this journal is cited, in accordance with accepted academic practice. No use, distribution or reproduction is permitted which does not comply with these terms.
*Correspondence: William A. Crossley , Y3Jvc3NsZXlAcHVyZHVlLmVkdQ==