- 1CSG EHV Power Transmission Company of China Southern Power Grid Co.,Ltd, Guangzhou, China
- 2Maintenance and Test Center of CSG EHV Power Transmission Company of China Southern Power Grid Co.,Ltd, Guangzhou, China
- 3CSG EHV Power Transmission Company of China Southern Power Grid Co.,Ltd, Dali Bureau, Dali, China
Knowledge graph (KG) has good knowledge expression ability and interpretation, and its application to power system fault diagnosis and disposal can effectively integrate data of the whole life cycle of equipment and form a novel knowledge-driven operation and maintenance management mode. This is crucial to assist dispatchers in fault disposal and effectively improve the power system emergency handling capability and dispatch intelligence level. This paper conducts a systematic review and summary of the application of KG in power system fault diagnosis and disposal, so as to provide an adequate and comprehensive guide for further research in this field. Firstly, the definition, status, and classification of KGs are systematically described, and the general process of KG construction is sorted out. Secondly, the basic framework, construction process, key technologies, and typical practices of KG for power system fault diagnosis and disposal are summarized and reviewed in detail. Ultimately, several challenges, opportunities, and perspectives of KG in this field are carefully presented.
1 Introduction
With the rapid development of ultra-high voltage (UHV) grids (Zhang et al., 2021), fault patterns of power system are becoming increasingly complex, which results in new tricky obstacles and challenges for precise fault diagnosis as well as appropriate and fast fault disposal (Barik et al., 2018). Traditional fault diagnosis and disposal methods generally depend on the experience and knowledge of maintenance personnel and specialists, which are tough for expansion and inheritance (Zhang and Zhao, 2017). Thus, various artificial intelligence and deep learning based approaches have been developed in recent years (Ajagekar and You, 2021; Van Gompel et al., 2022). Nevertheless, these methods are still in the simple application of power data and knowledge, which lack a comprehensive grasp of knowledge system and cognitive reasoning of power business (Pu et al., 2021). Therefore, it is an urgent and complex task to exploit a scientific and systematic fault diagnosis and disposal tool.
Knowledge graph (KG) also named multi-relation graph of semantic network is generally used to model the knowledge of complex concepts, entities, and relationships and to structurally represent nonlinear knowledge (Jayaram et al., 2015; Wang et al., 2021). It achieves efficient storage, query, computing, and other relational operations and analysis (Ji et al., 2022), which is widely applied in search engines (Ma and Zhang, 2018), social platforms (Mu et al., 2021), education and medical fields (Chen et al., 2018; Pham et al., 2022) thanks to its a series of practical superiorities, e.g., interpretability, universality, expansibility, etc. Meanwhile, KG has been introduced into the power system for equipment management (Tang et al., 2019; Wang et al., 2021), fault diagnosis and disposal (Qiao et al., 2020), electric marketing (Liang et al., 2021), grid information search (Hu et al., 2021), etc. Note that an advanced and efficient fault diagnosis and disposal technique is a critical safeguard for safe and steady operation as well as fault prevention of various power equipment and even power system (Wang and Peng, 2021). Besides, the application of KG in power system fault diagnosis and disposal is still in its infancy. For instance, reference (Zhang et al., 2015) developed a dynamic uncertain causality graph to explain and reveal the causal logic of various generator system faults in a nuclear power plant. Similarly, an improved signed directed graph was designed to diagnose different nuclear power plant faults in literature (Liu et al., 2019), upon which qualitative trend evaluation and granular computing algorithm were utilized to enhance its decision speed and resolution. Literature (Zhao et al., 2015; Yi and Jiang, 2020) applied a graph-based semi-supervised learning strategy to detect and classify short circuit and open circuit faults in photovoltaic (PV) arrays as well as blade icing faults in wind farms. Lv et al. (2020) established an inter-turn short-circuit location KG for direct-drive permanent magnet synchronous motor. In addition, literature (Liu and Wang, 2021) constructed a systematical transformer maintenance KG to guide fault analysis and disposal work. Reference (Liu et al., 2022a) created an electrical equipment fault KG according to operational and maintenance records, whereas the fault type and the correlation relationship between faults are regarded as node and edge, respectively.
Actually, numerous technical experience and documents for the power system have been accumulated in the past decades, e.g., equipment structure and corresponding fault mode, fault reasoning analysis, maintenance record, defect elimination record, etc., which provide the knowledge foundation for KG construction of fault diagnosis and disposal. Thus, more efficient comprehensive, and promising fault diagnosis and disposal techniques based on KG are urgent to develop and conduct. To tap the full potential of KG in fault diagnosis and disposal, this paper systematically and comprehensively overviews its current application state and provide several constructive and valuable perspectives/comments for future study.
The rest of this paper is designed as follows: Section 2 introduces KG in details. In Section 3, KG based fault diagnosis and disposal in power system are carefully discussed and evaluated. Lastly, Section 4 gives several critical challenges, opportunities and perspectives.
2 Knowledge graph
2.1 Definition and status of knowledge graph
For the sake of enhancing the intelligence level and efficiency of search engines, KG was first presented by Google in 2012, which is composed of conceptual entities, attributes, and relationships (Wang et al., 2012). Among which entity is defined as an object or thing that exists objectively and attributes are used to describe the features of entities. Additionally, the relationship is the most important feature of KG, which can realize the interconnection of everything and support semantic understanding, information retrieval, and other applications.
Specifically, KG can be concisely and transparently represented by a triple (Liu et al., 2022b; Tian et al., 2022), i.e., G=<E, R, S>. Among which G indicates KG; E = {e1, e2, e3, … , en} is a set of entities in the knowledge base; R = {r1, r2, r3, … , rm} represents a set of relationships; S = {s1, s2, s3, … , si} is the set of triple, and si = {en, rm, ej}, i.e., “entity-relationship-entity”, stands for fundamental units that constitute KG.
Furthermore, KG has been widely used in intelligent search, intelligent question and answer, text classification, and other fields (Li et al., 2022), thanks to its ability to better string and present knowledge via reticulated topology. For instance, YAGO (Suchanek et al., 2008), Wikidata (Turki et al., 2019), WordNet (AlMousa et al., 2022), FreeBase (Zhao et al., 2020), etc., are several successful and representative examples of applications of KG in other fields, and almost all of them are part of general-purpose KG. At present, however, KG is mostly promoted in the Internet field, but there are few applications in the power system, and KG is in the initial stage in the power industry.
2.2 Classification of knowledge graph
Whether divided by application fields or from the source of the required knowledge, KG can be classified into two major categories (Liu and Wang, 2018; Li et al., 2022), i.e., generic KG (also known as unrestricted domain KG or industry KG) and dedicated KG (alternate name restricted domain KG or domain KG). Among which, the generic KG involves a wide and diverse content and a complex network of relationships between knowledge, and is mainly oriented to intelligent search, intelligent question and answer, etc., (Li et al., 2022) While the dedicated KG targets specific domains with a low breadth and deep depth of knowledge, which provides business functions or is utilized to solve specific problems (Pu et al., 2021).
2.3 General process of knowledge graph construction
The construction procedure of KG mainly consists of knowledge extraction, knowledge fusion, knowledge storage, knowledge representation, knowledge verification, and knowledge reasoning. A standard KG can be acquired by the way of bottom-up or top-down, as illustrated in Figure 1 (Pu et al., 2021; Min et al., 2022). Bottom-up KG is constructed by knowledge extraction, knowledge fusion, knowledge storage, knowledge representation, knowledge verification, and knowledge reasoning, while the construction of top-down KG undertakes two critical steps, i.e., ontology learning and entity learning.
In particular, bottom-up is to extract entities, concepts, relationships, attributes, attribute values, and other information from open structured, semi-structured and unstructured data, then select objects with high confidence as candidates, analyze and summarize the underlying structural information, and finally form the knowledge ontology layer by layer and add it to the knowledge base. The top-down method means that the structure information of power domain knowledge ontology is obtained through the manual compilation or use of existing structured knowledge base by service experts, and then the knowledge ontology is added to the knowledge base.
3 Knowledge graph based fault diagnosis and disposal in power system
In the operation of the power system, such as generation, transmission, transformation, distribution, and many aspects of utilization of electricity, a large amount of data and information will be generated, thus forming a more complex power data resource system. However, traditional technologies have reached a bottleneck in the use and management of power data thanks to big data in the power grid having the typical “4 V” characteristics, i.e., large scale (volume), multiple types (variety), low value density (value) and fast change (velocity) (Song et al., 2013). Therefore, in order to achieve stable operation, electric power enterprises need to invest a lot of labor and funding. To address this obstacle, KG is a comparatively well-established and available technology. In contrast to data-driven-based approaches, KG as a symbolism-based artificial intelligence technique, has certain advantages in text data mining and interpretability (Li et al., 2022).
3.1 Basic framework for offline construction and online application of knowledge graphs in power system fault diagnosis and disposal
As shown in Figure 2, in the power grid, the application based on KG involves various aspects, including grid knowledge retrieval, cross-business connection, intelligent customer service, supplier evaluation, etc.
While this paper focuses on the application of KG in power system fault diagnosis and disposal. In particular, recording and retrieval of equipment defects (power equipment fault diagnosis), as well as automatic generation of security measures (fault disposal) using KG technique are reviewed and commented on emphatically according to available studies. It can provide guidance with important practical significance for the safe and reliable operation of the electric power system and the standardized and scientific management of the power supply system, such that the electrical equipment is maintained and operated in strict accordance with the safety operation management procedures.
Meanwhile, KG of fault diagnosis and disposal in power system is based on semi-structured and unstructured data (such as operation logs, inspection records, fault and disposal reports, operating standards, etc.), and structured data (such as equipment operation data, meteorological information, and experimental data, etc.) to form one or several graphs describing core elements (such as equipment name, equipment parameters, fault name, post-fault handling methods, fault disposal points, stable operation requirements, etc.) and their interrelationships, which are used to discriminate the causes of suspicious faults in equipment after an accident and give disposal suggestions and means (Guo et al., 2021). Ultimately, the software is used to convert the results into graphical form to visually display the contents of faulty equipment, causes of faults, disposal suggestions and means to assist operators in making efficient and accurate decisions through human-computer interaction. It is worth noting that in the practical application of KGs, certain permissions can be given to the operators to achieve efficient and secure maintenance and improvement of the KGs.
Therefore, the basic framework for offline construction and online application of knowledge graphs in power system fault diagnosis and disposal can be summarized in Figure 3. In particular, after the construction of domain KG for fault diagnosis and disposal in the power system is completed, which acquires to be iteratively updated in the practical application processes to effectively and adequately utilize generated new cases.
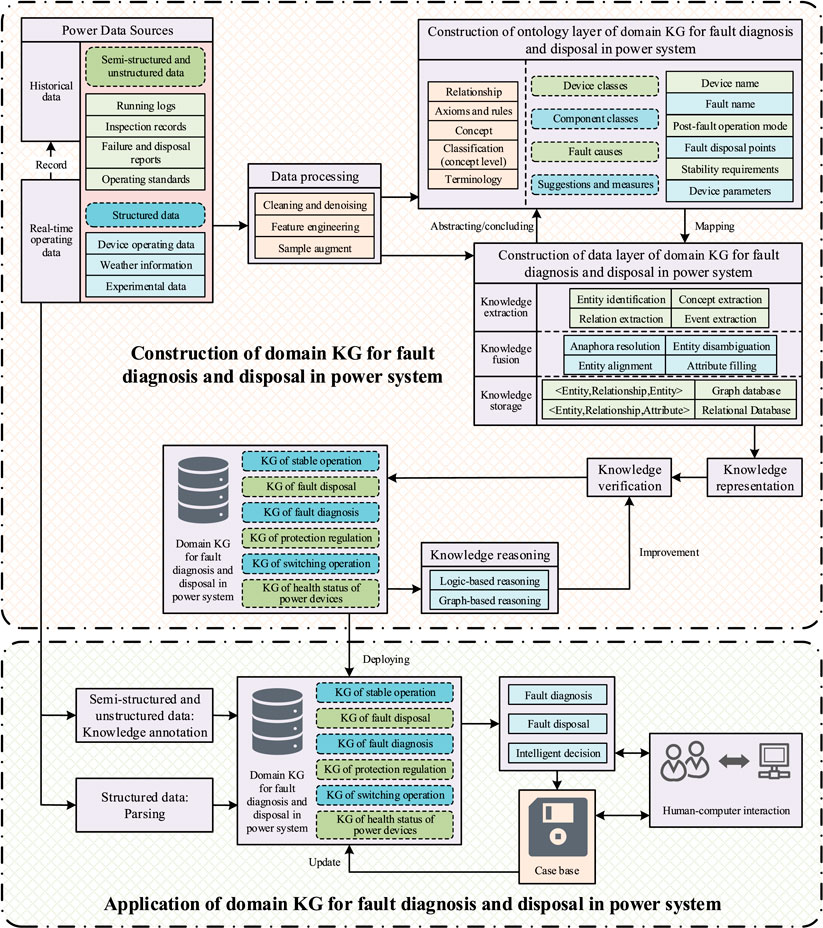
FIGURE 3. Basic framework for construction and application of KGs in power system fault diagnosis and disposal.
3.2 Knowledge graph of fault diagnosis and disposal in power system
As mentioned earlier, KG can adequately mine implicit information between the data and achieve efficient and structured data management, which undoubtedly provides a promising opportunity for fault diagnosis and disposal of power equipment. Rapid and reliable construction of a complete KG based on the available data of power equipment is the essential foundation of applying KG for fault diagnosis and disposal. Moreover, in the process of constructing KG in the electric power field, an appropriate data model is also crucial for the effective organization of knowledge thanks to involving massive data from heterogeneous sources. Particularly, the graph model uses the set of nodes and edges to portray the relationships between various things, which is extremely applicable to the construction of KG in the electric power field (Pu et al., 2021).
3.2.1 Construction of knowledge graph for fault diagnosis and disposal in power system
Consistent with the process of constructing domain KGs in other industries, the construction of KG for fault diagnosis and disposal in power system also involves two main parts, i.e., construction of the ontology layer and construction of the data layer.
Due to the domain nature of electric power data, the schema layer (ontology layer) and data layer of KG for power system fault diagnosis and disposal can be constructed using approaches of the top-down or the combination of top-down and bottom-up generally (Gao et al., 2020; Xiao et al., 2022). In this way, it is possible to pass on and solidify the relevant empirical knowledge in the existing database in the electric power field, but also to achieve the discovery of new knowledge.
For the ontology layer, the construction of ontology rules is an important task in building KGs for fault diagnosis and disposal of power systems. The existing data patterns, such as traditional power knowledge engineering systems, expert experience knowledge bases, and other structured data, can be directly used to guide the top-down construction of the ontology of KG. At the same time, various kinds of data generated in the actual business of electric power domain and semi/unstructured data such as the subjective experience of experts and technicians also contain rich industry knowledge in the power domain. Moreover, in order to ensure the completeness of the ontology of KG, information extraction technology is used to identify the power knowledge in such data, and the results of the identification are summarized and abstracted, and the abstracted concepts are finally mapped or supplemented into the ontology of power domain KG, so as to realize the bottom-up construction of the ontology (Pu et al., 2021). Partial ontology of KG for fault diagnosis and disposal in power system design can be designed as shown in Figure 4 (Guo et al., 2021; Li et al., 2021; Xiao et al., 2022).
For the data layer, its construction is realized by filling the content of the established ontology framework, which is mainly divided into three steps: knowledge extraction, knowledge fusion, and knowledge update. Among them, knowledge extraction is to obtain structured knowledge such as entities, inter-entity relationships, and attributes from non-(semi-)structured data through a series of knowledge extraction methods under the guidance of the ontology layer knowledge organization architecture. Knowledge fusion is to perform entity disambiguation and co-reference disambiguation processes on the entities obtained from knowledge extraction. While knowledge update is to evaluate the quality and timeliness of knowledge in the application process of KG and to update and revise in conjunction with the development of knowledge (Guo et al., 2021).
The composition of entities and relationships of devices in the grid can be represented by a semantic graph in the form of a network by using the triple < Entity, Relationship, Entity>, which can effectively and intuitively represent the relationship of the device components or ontology states in the scenario (Xiao et al., 2022). For instance, the relationship between the transformer and its casing pipe, and the casing pipe and its cracks can be represented in the data layer as illustrated in Figure 5, respectively.
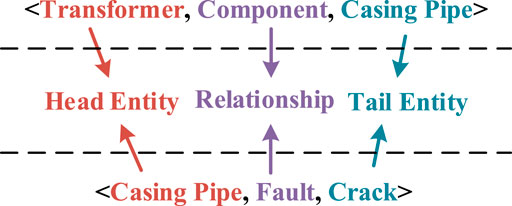
FIGURE 5. Representation of the relationship between transformer, casing pipe, and crack faults in the data layer.
3.2.2 Essential technologies
Entity extraction, relationship extraction are the essential technologies required to construct KGs (Pu et al., 2021; Li et al., 2022).
For knowledge extraction, it aims to automatically transform semi-structured data and unstructured data into structured data by information extraction from the massive power data. Traditionally, knowledge extraction in the electric power domain is mainly realized by using rule templates or domain dictionaries, however, it is highly dependent on manual and expert knowledge and has a limited scope of application. Deep learning-based methods are effective in circumventing the above problems, and thus its application to entity extraction has received attention. BiLSTM-CRF model (Ji et al., 2020; Dai et al., 2021), LR-CNN model (Guo et al., 2021), BERT-CRF model (Xiao et al., 2022), etc., are commonly used entity recognition techniques.
For relationship extraction, it aims to extract unknown entity relationships from the text and add them to KGs. Relationship extraction can also be divided into pattern matching-based relationship extraction and deep learning-based relationship extraction (Ji et al., 2022). Deep learning models such as BiGRU-Attention model (Guo et al., 2021), BERT-CNN-Attention model (Xiao et al., 2022), BiLSTM-GCN model (Song et al., 2022), BERT (Lü et al., 2021), etc., have gradually become a new research hotspot in the field of entity relation extraction due to their ability to automatically learn high-order features and high accuracy.
Eventually, the construction of KGs for power system fault diagnosis and disposal can be completed by continuously improving and training suitable power data models to realize the extraction of power knowledge such as entities, relations and attributes using the above-mentioned technologies.
3.3 Typical practices of knowledge graph for fault diagnosis and disposal in power system
At present, knowledge graph is more frequently used in power marketing, but its application in power dispatch, power operation and inspection, especially in power system fault diagnosis and disposal, is still in the initial stage.
In terms of power dispatch, the majority of business knowledge is fragmented and distributed, and it is characterized by complex relationships and a variety of categories. Therefore, related scholars have made some attempts in the field of power dispatching using natural language processing techniques and KG. For example, a topology identification method based on KG is proposed for the first time in Literature (Gao et al., 2020), which can overcome the drawbacks of online identification methods that require a large amount of high-quality operational data and occupy communication channels, and reduce the data dependence on topology identification.
Power operation and inspection have important practical significance to ensure the safe and stable operation of the power system. Literature (Liu and Wang, 2018) constructs a KG of power equipment defects using a corpus of equipment defect records, and proposes a method for power equipment defect retrieval using graph search. Meanwhile, Literature (Guo et al., 2021) designs a framework for the construction of domain KGs with the grid fault disposal plan text. Based on deep learning technology and KG, Literature (Xiao et al., 2022) proposes a fault intelligent diagnosis method that correlates multimodal information of substation equipment. Literature (Dai et al., 2021) established an intelligent diagnosis and auxiliary decision-making platform for secondary equipment functional defects based on BiLSTM-CRF model and knowledge graph using the text data of secondary equipment functional defects in power systems. Moreover, Literature (Zhang et al., 2022) presented a transfer learning based entity recognition technique for the grid domain and investigated the application of KG based grid fault disposal.
Overall, the current application scenarios of knowledge graph in power system fault diagnosis and disposal are relatively limited and slightly lacking in depth. Therefore, the development prospect of knowledge graph in power system fault diagnosis and disposition is promising.
4 Challenges, opportunities, and perspectives
In this paper, the application of KG in power system fault diagnosis and disposal is fully reviewed, and several challenges, opportunities, and prospects can be outlined as follows:
• Although the power system has accumulated a huge amount of data at present, the effective data available in certain areas (e.g., fault diagnosis and disposal of high-voltage DC system) are still limited. Therefore, to cope with the problem of uneven distribution of power data, it is the focus of research to use small-sample learning techniques to expand data directly on existing data and provide methodological guarantees for the construction of KGs;
• Deep learning is a black-box model and it is often difficult to interpret its results. By integrating KG with deep learning and applying knowledge inference techniques, explainable operation and maintenance solutions can be obtained, thus improving the credibility of operation and maintenance personnel for decisions based on KG;
• The performance of KG for power system fault diagnosis and disposal needs further practice in a situation where multiple faults are coupled with each other;
• Moreover, the construction of the current KG model requires a high cost to acquire samples and relies on experts using prior knowledge to analyze and judge relevant data. Thus, how to use artificial intelligence technology to achieve efficient and reliable utilization of power data will be a research direction in the field of fault diagnosis and disposal of power system based on KG.
Author contributions
QC: Writing-Original Draft; QL: Project administration, Resources; JW: Writing-Review and; Editing; CM: Investigation, Visualization; GP: Supervision, Writing-Review and; Editing; DW: Writing-Reviewing and Editing, Methodology.
Acknowledgments
The authors gratefully acknowledge the support of Key Science and Technology Projects of China Southern Power Grid Company Limited (CGYKJXM20210309).
Conflict of interest
QC, QL, JW, CM, GP and DW was employed by CSG EHV Power Transmission Company of China Southern Power Grid Co.,Ltd. Maintenance and Test Center of CSG EHV Power Transmission Company of China Southern Power Grid Co.,Ltd.
Publisher’s note
All claims expressed in this article are solely those of the authors and do not necessarily represent those of their affiliated organizations, or those of the publisher, the editors and the reviewers. Any product that may be evaluated in this article, or claim that may be made by its manufacturer, is not guaranteed or endorsed by the publisher.
References
Ajagekar, A., and You, F. (2021). Quantum computing based hybrid deep learning for fault diagnosis in electrical power systems. Appl. Energy 303, 117628. doi:10.1016/j.apenergy.2021.117628
AlMousa, A., Benlamri, B., and Khoury, K. (2022). A novel word sense disambiguation approach using WordNet knowledge graph. Comput. Speech & Lang. 74, 101337. doi:10.1016/j.csl.2021.101337
Barik, M. A., Gargoom, A., Mahmud, M. A., Haque, M. E., Al-Khalidi, H., and Than Oo, O. (2018). A decentralized fault detection technique for detecting single phase to ground faults in power distribution systems with resonant grounding. IEEE Trans. Power Deliv. 33 (5), 2462–2473. doi:10.1109/TPWRD.2018.2799181
Chen, P. H., Lu, Y., Zheng, V. W., Chen, X. Y., and Yang, B. D. (2018). KnowEdu: A system to construct knowledge graph for education. IEEE Access 6, 31553–31563. doi:10.1109/ACCESS.2018.2839607
Dai, Y., Zhang, J., Ji, Z., Liu, M., Gao, T., Zheng, Y., et al. (2021). Intelligent diagnosis and auxiliary decision of power system secondary equipment based on functional defect text. Electr. Power Autom. Equip. 41 (6), 184–194. doi:10.16081/j.epae.202106006
Gao, Z. P., Zhao, Y., Yu, Y. L., Luo, Y. J., Xu, Z. W., and Zhang, L. M. (2020). Low-voltage distribution network topology identification method based on knowledge graph. Power Syst. Prot. Control 48 (2), 34–43. doi:10.19783/j.cnki.pspc.190379
Guo, R., Yang, Q., Liu, S., Li, W., Yuan, X., and Huang, X. (2021). Construction and application of power grid fault handing knowledge graph. Power Syst. Technol. 45 (6), 2092–2100. doi:10.13335/j.1000-3673.pst.2021.0065
Hu, J. Y., Zhao, S. C., and Nie, Q. (2021). “Research on modeling of power grid information system based on knowledge graph,” in 2021 IEEE International Conference on Power Electronics, Computer Applications (ICPECA), Shenyang, China, 22-24 Jan. 2021 (IEEE), 648–651. doi:10.1109/ICPECA51329.2021.9362503
Jayaram, N., Khan, A., Li, C., Yan, X., and Elmasri, R. (2015). Querying knowledge graphs by example entity tuples. IEEE Trans. Knowl. Data Eng. 27 (10), 2797–2811. doi:10.1109/TKDE.2015.2426696
Ji, S. X., Pan, S. R., Cambria, E., Marttinen, P., and Yu, P. S. (2022). A survey on knowledge graphs: Representation, acquisition, and applications. IEEE Trans. Neural Netw. Learn. Syst. 33 (2), 494–514. doi:10.1109/TNNLS.2021.3070843
Ji, Z., Wang, X., Cai, C., and Sun, H. (2020). Power entity recognition based on bidirectional long short-term memory and conditional random fields. Glob. Energy Interconnect. 3 (2), 186–192. doi:10.1016/j.gloei.2020.05.010
Li, G., Li, Y. Q., Wang, H. T., Xie, Q., Huang, W. Q., and Hou, J. X. (2022). Knowledge graph of power equipment health management: Basic concepts, key technologies and research progress. Automation Electr. Power Syst. 46 (3), 1–13. doi:10.7500/AEPS20210804001
Li, J. L., Zhang, C. C., Zhao, H. R., Huang, T., Wan, Y., and Wang, X. W. (2021). Construction of power grid equipment technical supervision knowledge graph based on multi-source data fusion. Electrotech. Electr. 2021 (9), 60–63+68. doi:10.3969/j.issn.1007-3175.2021.09.013
Liang, H., Peng, X. J., Zhao, N. N., Duan, L. G., Yu, P. F., and Fu, D. (2021). “An approach of top-down electric generation knowledge graph construction,” in 2020 2nd International Conference on Energy, Power, Environment and Computer Application, Beijing, China, 22-23 Nov. 2020, 012021. doi:10.1088/1755-1315/661/1/012021
Liu, H., Zhou, S., Chen, C., Gao, T., Xu, J., and Shu, M. (2022). Dynamic knowledge graph reasoning based on deep reinforcement learning. Knowledge-Based Syst. 241, 108235. doi:10.1016/j.knosys.2022.108235
Liu, L. Q., Wang, B., Ma, F. Q., Zheng, Q., Yao, L. Z., Zhang, C., et al. (2022). A concurrent fault diagnosis method of transformer based on graph convolutional network and knowledge graph. Front. Energy Res. 10, 837553. doi:10.3389/fenrg.2022.837553
Liu, X. Y., and Wang, H. W. (2021). “Knowledge graph construction and decision support towards transformer fault maintenance,” in 2021 IEEE 24th International Conference on Computer Supported Cooperative Work in Design (CSCWD), Dalian, China, 05-07 May 2021 (IEEE), 661–666. doi:10.1109/CSCWD49262.2021.9437847
Liu, Y. K., Ai, X., Ayodeji, A., Wu, M. P., Peng, M. J., Xia, H., et al. (2019). Enhanced graph-based fault diagnostic system for nuclear power plants. Nucl. Sci. Tech. 30 (12), 174. doi:10.1007/s41365-019-0708-x
Liu, Z., and Wang, H. (2018). Retrieval method for defect records of power equipment based on knowledge graph technology. Automation Electr. Power Syst. 42 (14), 158–164. doi:10.7500/AEPS20180103007
Lü, M., Duan, B., Jiang, H., and Deng, D. (2021). Research on management and application of wind power data based on knowledge graph technology. Power Syst. Prot. Control 49 (6), 167–173. doi:10.19783/j.cnki.pspc.200608
Lv, K., Gao, C. X., Si, J. K., Feng, H. C., and Cao, W. P. (2020). Fault coil location of inter‐turn short‐circuit for direct‐drive permanent magnet synchronous motor using knowledge graph. IET Electr. Power Appl. 14 (9), 1712–1721. doi:10.1049/iet-epa.2020.0123
Ma, C., and Zhang, B. (2018). A new query recommendation method supporting exploratory search based on search goal shift graphs. IEEE Trans. Knowl. Data Eng. 30 (11), 2024–2036. doi:10.1109/TKDE.2018.2815544
Wang, M., Hao Li, H., Dacheng Tao, D. C., Ke Lu, K., and Xindong Wu, X. D. (2012). Multimodal graph-based reranking for web image search. IEEE Trans. Image Process. 21 (11), 4649–4661. doi:10.1109/TIP.2012.2207397
Min, W., Liu, C., Xu, L., and Jiang, S. (2022). Applications of knowledge graphs for food science and industry. Patterns 3 (5), 100484. doi:10.1016/j.patter.2022.100484
Mu, L., Jin, P. Q., Zhao, J., and Chen, E. H. (2021). Detecting evolutionary stages of events on social media: A graph-kernel-based approach. Future Gener. Comput. Syst. 123, 219–232. doi:10.1016/j.future.2021.05.006
Pham, T., Tao, X. H., Zhang, J., Yong, J. M., Li, Y. F., and Xie, H. R. (2022). Graph-based multi-label disease prediction model learning from medical data and domain knowledge. Knowledge-Based Syst. 235, 107662. doi:10.1016/j.knosys.2021.107662
Pu, T. J., Tan, Y. P., Peng, G. Z., Xu, H. F., and Zhang, Z. H. (2021). Construction and application of knowledge graph in the electric power field. Power Syst. Technol. 45 (6), 2080–2091. doi:10.13335/j.1000-3673.pst.2020.2145
Qiao, J., Wang, X. Y., Min, R., Bai, S. H., Yao, D., and Pu, T. J. (2020). Framework and key technologies of knowledge-graph-based fault handling system in power grid. Proc. CSEE 40 (18), 5837–5849. doi:10.13334/j.0258-8013.pcsee.200033
Song, W., Han, L., Xian, H., Yao, S., and Guo, S. (2022). Construction and application of GCN⁃based distribution network knowledge graph. Electron. Des. Eng. 30 (7), 160–164. doi:10.14022/j.issn1674-6236.2022.07.033
Song, Y. Q., Zhou, G. L., and Zhu, Y. L. (2013). Present status and challenges of big data processing in smart grid. Power Syst. Technol. 37 (4), 927–935. doi:10.13335/j.1000-3673.pst.2013.04.004
Suchanek, M. S., Kasneci, K., and Weikum, W. Y. A. G. O. (2008). Yago: A large ontology from wikipedia and WordNet. J. Web Semant. 6 (3), 203–217. doi:10.1016/j.websem.2008.06.001
Tang, Y., Liu, T., Liu, G., Li, J., Dai, R., and Yuan, C. (2019). “Enhancement of power equipment management using knowledge graph” in 2019 IEEE Innovative Smart Grid Technologies-Asia (ISGT Asia), Chengdu, China, 21-24 May 2019, IEEE, 905–910. doi:10.1109/ISGT-Asia.2019.8881348
Tian, L., Zhou, X., Wu, Y. P., Zhou, W. T., Zhang, J. H., and Zhang, T. S. (2022). Knowledge graph and knowledge reasoning: A systematic review. J. Electron. Sci. Technol. 20 (2), 100159. doi:10.1016/j.jnlest.2022.100159
Turki, T., Shafee, S., Hadj Taieb, A. H. T., Ben Aouicha, B. A., Vrandečić, V., Das, D., et al. (2019). Wikidata: A large-scale collaborative ontological medical database. J. Biomed. Inf. 99, 103292. doi:10.1016/j.jbi.2019.103292
Van Gompel, J. V., Spina, D., and Develder, C. (2022). Satellite based fault diagnosis of photovoltaic systems using recurrent neural networks. Appl. Energy 305, 117874. doi:10.1016/j.apenergy.2021.117874
Wang, J. D., Yang, J., Pei, Y. Z., Zhan, X. P., Zhou, T., and Xie, P. Y. (2021). Distribution network fault assistant decision-making based on knowledge graph. Power Syst. Technol. 45 (6), 2101–2112. doi:10.13335/j.1000-3673.pst.2020.1677
Wang, Z., and Peng, L. (2021). Grouping capacitor voltage estimation and fault diagnosis with capacitance self-updating in modular multilevel converters. IEEE Trans. Power Electron. 36 (2), 1532–1543. doi:10.1109/TPEL.2020.3011131
Xiao, F. L., Wu, Y. Z., Shen, X. H., He, Z. K., and Qin, Y. (2022). Intelligent fault diagnosis of substation equipment on the basis of deep learning and knowledge graph. Electr. Power Constr. 43 (3), 66–74. doi:10.12204/j.issn.1000-7229.2022.03.008
Yi, H. K., and Jiang, Q. C. (2020). Graph-based semisupervised learning for icing fault detection of wind turbine blade. Meas. Sci. Technol. 32 (3), 035117. doi:10.1088/1361-6501/abb166
Zhang, Q., Dong, C. L., Cui, Y., and Yang, Z. H. (2015). Dynamic uncertain causality graph for knowledge representation and probabilistic reasoning: Directed cyclic graph and joint probability distribution. IEEE Trans. Neural Netw. Learn. Syst. 26 (4), 1503–1517. doi:10.1109/TNNLS.2015.2402162
Zhang, C. H., Song, G. B., and Dong, X. Z. (2021). Non-unit ultra-high-speed DC line protection method for HVDC grids using first peak time of voltage. IEEE Trans. Power Deliv. 36 (3), 1683–1693. doi:10.1109/TPWRD.2020.3013021
Zhang, R., Liu, J., Zhang, B., Li, J., Gao, T., and Zhang, J. (2022). Research on grid fault handling knowledge graph construction and real-time auxiliary decision based on transfer learning. Electr. Power Inf. Commun. Technol. 20 (6), 24–34. doi:10.16543/j.2095-641x.electric.power.ict.2022.06.003
Zhang, Z. P., and Zhao, J. S. (2017). A deep belief network based fault diagnosis model for complex chemical processes. Comput. Chem. Eng. 107, 395–407. doi:10.1016/j.compchemeng.2017.02.041
Zhao, Y., Ball, R., Mosesian, J., de Palma, J. F., and Lehman, B. (2015). Graph-based semi-supervised learning for fault detection and classification in solar photovoltaic arrays. IEEE Trans. Power Electron. 30 (5), 2848–2858. doi:10.1109/TPEL.2014.2364203
Keywords: knowledge graph, artificial intelligence, power system, fault diagnosis, fault disposal
Citation: Chen Q, Li Q, Wu J, Mao C, Peng G and Wang D (2022) Application of knowledge graph in power system fault diagnosis and disposal: A critical review and perspectives. Front. Energy Res. 10:988280. doi: 10.3389/fenrg.2022.988280
Received: 07 July 2022; Accepted: 29 July 2022;
Published: 08 September 2022.
Edited by:
Bo Yang, Kunming University of Science and Technology, ChinaReviewed by:
Yiyan Sang, Shanghai University of Electric Power, ChinaXueqian Fu, China Agricultural University, China
Copyright © 2022 Chen, Li, Wu, Mao, Peng and Wang. This is an open-access article distributed under the terms of the Creative Commons Attribution License (CC BY). The use, distribution or reproduction in other forums is permitted, provided the original author(s) and the copyright owner(s) are credited and that the original publication in this journal is cited, in accordance with accepted academic practice. No use, distribution or reproduction is permitted which does not comply with these terms.
*Correspondence: Qiang Li, TGlRaWFuZ19FSFZfQ1NHQG91dGxvb2suY29t