- School of Economics and Management, Shanghai University of Electric Power, Shanghai, China
With the continuous advancement of the new generation of information technology revolution, digital economy is gradually becoming an important driving force for energy transition while restructuring the new form of the economy. This paper selects panel data of 30 provinces in China from 2011 to 2021, empirically verifies the special impacts of digital economy on green-oriented transition of energy by using the spatial Durbin model, and further constructs a multi-intermediary model to verify its transition pathways. The main findings are as follows: First, the overall level of green-oriented transition of energy in China’s eastern coastal areas is relatively high, and the western regions have been more capable of catching up in recent years. Second, digital economy has played a significant role in promoting green-oriented transition of energy, and there is a positive spatial spillover effect. Third, digital economy could indirectly promote the green-oriented transition of energy by promoting the upgrading of industrial structure, improving the level of technological innovation and strengthening environmental regulations. This study provides empirical insights and reference for policymakers in accelerating the comprehensive green-oriented transition of energy.
1 Introduction
The massive consumption of fossil fuels such as coal in recent decades has brought huge challenges to the global environment: excessive carbon dioxide emissions have led to global climate change, depletion of fossil energy reserves, and increasing imbalances in energy markets. The 27th session of the Conference of the Parties (COP27) to the United Nations Framework Convention on Climate Change in 2022 launched the Planning for Climate Commission, aiming to accelerate the deployment of renewable energy sources such as hydrogen and increase investment in renewable energy technologies to gradually reduce dependence on fossil fuels (Bashir et al., 2023). China is the world’s top energy consumer and carbon emitter and has been the fastest rising energy consumption for 20 consecutive years, whose primary energy consumption increased from 600 million tons of standard coal in 1981 to 5.41 billion tons in 2022, with coal consumption accounting for 56.2% of total primary energy consumption. To reduce energy consumption, ensure energy security, and maintain environmental sustainability, the Chinese government has consistently prioritized energy transition, namely, the shift from fossil fuels to renewable energies (De La Peña et al., 2022). In September 2020, at the general debate of the 75th session of the United Nations General Assembly, Chinese President Xi Jinping proposed that China’s carbon dioxide emissions should peak before 2030 and China will strive to achieve carbon neutrality by 2060. This is a significant strategic decision made by the Chinese government based on its resolution to promote a global community of shared future, and it is the domestic requirement for achieving sustainable development. The COVID-19 pandemic that broke out in 2020 had a profound impact on energy demand, although the growth rate of renewable energy generation set a new record, due to weakened control over the energy market, the continuous decline in oil prices made traditional energy more competitive than renewable energy, and posed a serious threat to the global renewable energy supply chain (Tian et al., 2022). In 2021, carbon dioxide emissions during the economic recovery period were about 180 Mt higher than in 2019 before the pandemic, and China remains the country with the highest carbon dioxide emission intensity among major economies, due to the dominance of coal in China’s energy structure and the high proportion of industry in its GDP (IEA, 2022). Therefore, how to accelerate the “decarbonization” of the energy system and achieve the clean and low-carbon energy transition is a major issue that needs to be addressed urgently.
The digital economy is driven by emerging technologies such as big data, cloud computing, blockchain, and artificial intelligence, and it optimizes the allocation of economic and social resources through data. In recent years, supported by national policies, China’s digital economy has flourished, expanding nearly 400% compared to a decade ago, with its proportion in GDP increasing by 18.2% (Ran et al., 2023). As the next-generation information and communication technologies accelerate their penetration into the real economy, traditional factors are activated by data factors, and traditional industrial processes are optimized, thereby reducing information collection and transaction-related costs and improving total factor productivity. The development of digital economy continuously generates new digital business models and forms, such as smart logistics, industrial internet, and intelligent manufacturing, promoting the agglomeration, integration, and ecological development of various industries. Furthermore, the digital economy simplifies the procedures for generating renewable energy, which is a necessary path for decarbonizing the power sector (Ahmadpour et al., 2021). The integration of digital economy with the economic and social system breaks the original industrial layout, reshapes the mode of value creation, and extends the industrial chain, promoting the integration and development of industries. By integrating big data, cloud computing, artificial intelligence and other digital technologies into the production and consumption of energy, it drives enterprises to achieve intelligent production and efficient coordination, enabling flexible allocation of various energy sources and increasing the proportion of clean energy in energy consumption. Therefore, exploring whether digital economy could drive the green and low-carbon transition of energy is the focus of this study. Since there is a certain spatial correlation between things in different regions, the spatial correlation is also included in the study scope to reduce the bias of the estimation results (You and Lv, 2018). Moreover, the development of digital economy is closely related to industrial structure, technological innovation, and environmental regulations, which may exert mediating effects on green-oriented transition of energy. This paper will also discuss these three possible transition mechanisms to provide a more comprehensive explanation of the relationship between China’s digital economic development and green-oriented transition of energy.
The potential marginal contributions of this paper are mainly as follows: First, based on the connotation, laws and trends of green-oriented transition of energy, this paper comprehensively measures the level of China’s green-oriented transition of energy from four different dimensions, increasing the accuracy and comprehensiveness of the assessment results. Second, based on the spatial Durbin model, this paper explores the spatial effects of digital economy on green-oriented transition of energy, which could help to formulate relevant policies on the green-oriented transition of energy from the perspective of regional collaboration. Third, this paper conducts an in-depth analysis of the role mechanism of digital economy in green-oriented transition of energy, and uses the stepwise regression method to analyze the mediating effects of industrial structure upgrading, technological innovation, and environmental regulations.
The rest of the article is divided into the following sections. A review of the literature is presented in Section 2. The mechanism analysis and research hypotheses are presented in Section 3. In Section 4, the methodology and data sources are presented. The empirical analysis, results, and discussion are presented in Section 5. The article’s conclusions and policy suggestions are presented in Section 6.
2 Literature review
2.1 Energy transition and its influencing factors
Energy transition, as a global trend in energy development, has actively been studied by numerous scholars. Conceptually, the most intuitive form of energy transition is the change in energy structure. Hefner (2013) characterizes energy transition as a shift from solid to liquid and then to gaseous form of energy. Li et al. (2020) view green energy transition as the replacement of dominant energy sources, namely, replacing high-carbon fossil fuels with clean energy. The long-term changes in energy structure will impact the energy system in all kinds of aspects, including economic, political, social, and environmental aspects, which implies that energy transition is a significant shift towards a sustainable system (Markard et al., 2012). Regarding the driving forces and factors influencing energy transition, previous scholars have primarily conducted qualitative and quantitative studies. Millot and Maïzi (2021) find in their study of the historical characteristics of energy transition that its driving factors are multiple and interpenetrating, including national policies, technological innovation, and market mechanisms. By comparing and analyzing different energy scenarios in Europe, Hainsch et al. (2022) discover that energy transition is driven by a combination of technological support, policy strength, and social attitudes. Quantitative studies have also emerged, for instance, Guo et al. (2019) use a structural equation model to analyze the factors influencing sustainable energy transition in China, and find that energy technology innovation, energy markets, and socio-technical systems are the main drivers of energy transition. Huang and Zou (2020) study the impact of cross-regional electricity transmission and environmental regulations on energy transition based on panel data from 30 provinces in China, and find that significant heterogeneity in energy transition across different transmission regions, and that environmental regulations positively promote energy transition. Huang (2022) finds that natural resource prices, economic growth, energy imports, and population growth are all positively correlated with energy transition by using an ARDL model boundary co-integration test for empirical analysis.
2.2 Digital economy and its impact effects
Specifically, this means handling important affairs of various economic sectors through computer-assisted data digitization (Williams, 2021). Digital economy represents a force of technology that could boost economic development and technological innovation, reduce operational costs for businesses, and increase productivity (Yuan et al., 2021). Many studies have shown that the integration of digital economy with industries has optimized industrial chains, making industrial structures more advanced and rational (Zhang, 2018; Li et al., 2020; Wang et al., 2023). The new models and forms created by digital economy also stimulate economic growth (Fernández-Portillo et al., 2020) and enhance total factor productivity by promoting technological progress (Loebbecke and Picotb, 2015; Lyu et al., 2023). Additionally, the impact of digital economy on the environment has also attracted widespread attention. Numerous studies have confirmed that the development of digital economy could significantly reduce carbon emission intensity, including empirical studies based on global panels (Dong et al., 2022), as well as analyses from provincial and city levels in China (Li and Wang, 2022; Yi et al., 2022). However, the digital economy may have a potential rebound effect that offsets its positive impact on energy demand and environmental quality (Ozturk and Ullah, 2022). Some scholars have studied major urban agglomerations in China, for instance, Luo et al. (2022) found that the development of digital economy could significantly enhance the green development efficiency of the Yangtze River Economic Belt, and Ma and Zhu (2022) found that digital economy could obviously drive high-quality green development and further explore its nonlinear effects and spatial spillover effects.
2.3 The nexus of the digital economy and energy resources
The development of digital economy is driving changes in overall production methods, lifestyles, and governance methods, as well as reshaping energy production, distribution, and consumption patterns. Firstly, the widespread application of digital technology in the energy sector optimizes the energy supply chain and innovates energy consumption forms (Li and Zhang, 2017). Loock (2020) studies the value of digitization for energy transition in Europe and finds that digitization is conductive to business model innovation and energy transition promotion. In similar studies, Veskioja et al. (2022) discuss the role of digitization in the socio-technical energy transition in Europe, finding that digitization changes energy production and consumption behaviors, thereby strengthening environmental concepts to promote energy transition. Secondly, digital economy is beneficial for the development of renewable energies, which is key to achieving low-carbon energy transition. Digital technology has a strong advantage in assessing renewable energy investment projects, helping to reduce risks and attract foreign capital, thus increasing corporate investment in renewable energy projects (Mazzucato and Semieniuk, 2018). Shahbaz et al. (2022) empirically verify the impact of digital economy on renewable energy generation and consumption structures based on panel data from 72 countries, and find that digital economy significantly promotes energy transition, with government governance playing a mediating role. Additionally, digital economy promotes energy efficiency, which positively affects energy transition. Survey results on energy policy by Winskel and Kattirtzi (2020) show that digital technology aids in the intelligent management of energy systems, improving energy efficiency to some extent. Further, Wang and Shao (2023) finds in a nonlinear regression based on 282 prefecture-level cities in China that digital economy significantly improves energy efficiency, and this promoting effect gradually strengthens as the level of digital economy increases.
While existing literature provides a theoretical foundation and useful references for this study on digital economy and green-oriented transition of energy, there are still some inadequacies. First, although the theoretical and empirical analysis of the green-oriented transition of energy is relatively complete, there is still research space for applying quantitative methods measure the level of green-oriented transition of energy from different perspectives and dimensions. Second, few studies have explored the spatial relationship between digital economy and green-oriented transition of energy, and this paper considers the spatial dependencies and linkages between regions both geographically and economically. Third, digital economy may influence the green-oriented transition of energy through various intermediary effects, with its transmission mechanisms unclear. This paper explores from three aspects: upgrading of industrial structure, technological innovation, and environmental regulations.
3 Theoretical mechanism
3.1 Direct impacts of the digital economy on the green-oriented transition of energy
The digital economy, as a new economic form, has become a new driver for promoting changes in energy production and consumption patterns. On the one hand, digital economy promotes the intelligent retrofit of production processes in energy enterprises, promoting the optimization and upgrading of the energy production structure. Digital technologies enable energy enterprises to achieve automation and efficient management in processes such as raw material extraction, processing, inspection, and assembly, for instance, by utilizing high-precision sensor measurements and intelligent decision-making, the efficiency of renewable energy production, transmission, and storage can be enhanced, thereby promoting the development of renewable energy (Wang et al., 2023), leading to a further increase in the proportion of clean energy within overall energy production. On the other hand, digital economy is conducive to improving energy utilization efficiency, reducing energy consumption levels, and reshaping energy consumption pattern and structure. The digital transition of the energy industry promotes the research and application of new technology equipment, increases electricity consumption, and reduces the proportion of high-carbon fossil energy use, thereby enhancing the level of electrification (Kim et al., 2021). The large-scale substitution of electricity can optimize the energy consumption structure, which promotes the green-oriented transition of energy.
As the strong connectivity and shareability of digital economy can break through spatial and temporal restrictions, a large amount of data elements can be more easily transferred between enterprises, effectively improving the energy utilization efficiency of enterprises in production and operation and reducing the demand of high-energy-consuming enterprises for low-quality energy sources such as coal. Additionally, the internet and information technology strengthen the flow of technology, capital, and human resources across different regions, effectively enhancing the rationality of enterprise resource allocation and the flexibility of energy supply and distribution, thus promoting the spatial spillover effect of digital economy on green-oriented transition of energy. Based on the above analysis, we propose hypothesis 1 and hypothesis 2.
H1. The digital economy has a positive impact on the green-oriented transition of energy.
H2. The impact of the digital economy on the green-oriented transition of energy has a positive spatial spillover effect.
3.2 Digital economy, industrial structure upgrading and green-oriented transition of energy
The structural transition theory describes the process of changing the industrial structure to a more advanced, more complex and more adaptable direction to market needs, and the digital economy plays an important role in this process. Firstly, digital economy breaks the independence of industry sectors under traditional economic development models, with different industry sectors gradually integrating and developing under the integration of digital technologies. The circulation of data elements between industries transforms industries from simple chain organizations to multi-dimensional networks, promoting the diversification and innovative development of industries. Moreover, digital technologies break the spatial and temporal restrictions of product transactions, enhancing corporate competitiveness while increasing the added value and derivative value of products. The significant reduction in the cost of production and circulation of products allows for more effective allocation of materials, manpower, and social resources, thereby forming a new industrial chain, changing the logic and organizational forms of industrial development, and achieving the upgrading of industrial structure.
The upgrading of industrial structure can empower the green-oriented transition of energy, the essence of which lies in the overall improvement of the production efficiency of all kinds of factors, including the elimination of high energy consuming industries as well as the enhancement of the proportion of green industries, which implies that the industry is being transformed in the direction of lower-carbon and cleaner (Xue et al., 2022). The industrial structure has become more rational while it reduces dependency on fossil energy and forms a low-carbon and sustainable energy consumption structure (Cheng et al., 2023). Based on the above analysis, we propose hypothesis 3.
H3. Digital economy can indirectly promote the green-oriented transition of energy through industrial structure upgrading.
3.3 Digital economy, technological innovation and green-oriented transition of energy
According to the new growth theory, the core of technological innovation is knowledge accumulation, and the digital economy will enhance the knowledge stock of the entire economy through diversified information dissemination methods, thus promoting technological innovation. First, digital economy enhances the overall production capacity of the economy. When information technology products are integrated into the economic system as intermediate elements of the production process, new combinations are generated (Du et al., 2023), triggering technological innovation. Second, the network connection of the Internet of Everything (IoE) under the digital economy allows for real-time interactions between people and people, people and things, and things and things, making knowledge sharing and thinking collaboration among innovation agents possible. This flexible process of technological innovation not only saves material and time costs but also increases the applicability of technological innovations (Saldanha and Mithas, 2017).
Breaking through core technologies and promoting innovative development play a key role in green-oriented transition of energy, mainly reflected in three aspects: the first is technological innovation in energy production. Continuous innovation of new energy technologies leads to a continuous reduction in the cost of kW hours, especially for wind and solar power, which are now cheaper than traditional coal-fired power generation, promoting the rapid development of new energies. The second is technological innovation in energy transmission and conversion. Innovations in the energy internet, new storage technologies, hydrogen energy, and other areas will promote the development of intermittent and fluctuating renewable energies such as wind energy and solar energy, enhancing the capacity to consume a high proportion of renewable energies. This positive feedback further promotes the development of renewable energies, ultimately achieving a clean and low-carbon transition of energy production. The third is technological innovation in energy consumption. Innovative applications like energy-saving technology, fuel substitution technology, and resource-recycling coupling technology not only reduce the consumption of fossil energy but also significantly improve overall energy efficiency, accelerating the green transition of the energy consumption structure. Based on the above analysis, we propose hypothesis 4.
H4. Digital economy can indirectly promote the green-oriented transition of energy through technological innovation.
3.4 Digital economy, environmental regulations and green-oriented transition of energy
The deep integration of digital technology with ecological and environmental protection is beneficial to the supervision of environmental protection departments and the improvement of environmental regulations. To be specific, all kinds of data from production and operation processes of enterprises can be collected and recorded in real-time through devices and summarized into corresponding systems, thereby making problems be identified and solved promptly (Wu et al., 2023). Additionally, due to the drawbacks of previous empirical decision-making such as lengthy processes, inaccurate judgments, and weak targeting, it is difficult to trace the root causes, while big data can provide precise, comprehensive information, enhance the transparency of the execution, and solve the problem of information asymmetry (Dutil and Williams, 2017), thus providing the best decision-making basis for government departments to formulate environmental regulation policies and conduct real-time supervision, and using historical data for prediction and prevention, which enhances the levels of environmental regulations.
As a strong administrative control, environmental regulations play an important role in promoting the green-oriented transition of energy. Under the pressure of laws and regulations, enterprises respond by approaches such as adjusting the ratio of production factors, improving production equipment, and reducing the use of high-pollution raw materials to bring various economic activities up to the industry standard, thereby reducing the proportion of fossil energy used in production processes. For some incentive policies, such as carbon taxes and environmental subsidies, enterprises may be encouraged to increase their efforts in technological innovation, developing more green and environmentally friendly products (Pan et al., 2023), which not only helps enterprises reduce energy consumption and costs, but also helps enterprises establish a good public image, creating a brand effect and forming a virtuous cycle that empowers green-oriented transition of energy. Based on the above analysis, we propose hypothesis 5.
H5. Digital economy can indirectly promote the green-oriented transition of energy through industrial structure upgrading, technological innovation and environmental regulations.
In summary, the influential mechanism of the digital economy on green-oriented transition of energy is constructed in Figure 1.
4 Method and data
4.1 Empirical model
Due to the uneven spatial distribution of energy resources in various provinces of China, there is a spatial dependence in green-oriented transition of energy. to reduce estimation bias, this study performs an empirical investigation using the spatial Durbin model, and the model is constructed as:
Where EGT denotes the level of green-oriented transition of energy, DIG denotes digital economy, i denotes the area and t denotes year, C is a series of control variablesis a spatial weight matrix, μit represents the random disturbance term. To reduce heteroscedasticity in the data, all variables were transformed using logarithmic scales.
Furthermore, to verify whether the mediating effect of industrial structure upgrading, technological innovation and environmental regulations on green-oriented transition of energy is established, the mediating variables are added to Eq. 1 to construct a multiple mediation model, as shown in Eqs 2-5:
Where IS, TI and ENV denote industrial structure upgrading, technological innovation, and environmental regulations, respectively, b1, c1, and d1 are the estimated coefficients, X denotes each different mediating variable, and ε1it - ε4it are the random disturbance terms.
4.2 Variables and data
4.2.1 Dependent variable
From an intuitive point of view, energy transition is a structural change in production and consumption, but as a whole, it should be considered from a systematic perspective, including economic, political, demographic, social environment, etc. Based on defining the connotation of green-oriented transition of energy (EGT) and summarizing its status quo, laws and trends, we use four factors to construct an index system for green-oriented transition of energy: energy production structure, energy consumption structure, residents’ concept of consumption, and environmental coordination, as shown in Table 1. This paper adopts the coefficient of variation method to weight the four categories, and then measure the comprehensive green-oriented transition of energy index. To further characterize the spatial pattern of China’s green-oriented transition of energy, we draw the distribution maps of the comprehensive index in 2011, 2016, and 2021 (see Figure 2). Overall, the green-oriented transition of energy index of each province is gradually increasing, and the southeast coastal regions have relatively higher levels, which stems from its leading level of economic development and its emphasis on the development of green energy, thus has a decarbonized energy structure. In addition, western provinces such as Qinghai, Sichuan and Yunnan have strong catching-up ability and potential for improvement due to their rich renewable energy resources.
4.2.2 Independent variable
The digital economy is supported by the development of digital industries and focuses on the deep integration of industrial digitalization, constantly expanding the breadth and depth of integration of the digital economy. By taking into account the concept of the digital economy (DIG) and drawing on the digital economy index system established by other scholars, we use four factors to construct an index system for the digital economy: digital infrastructure, digital industry development, industrial digitization, and digital innovation (details are shown in Table 2). Entropy method is a method used for multi-index comprehensive evaluation. It can avoid the influence of subjective factors and assign different weights according to the entropy of indicators. The entropy value method is used to assign weights to each indicator.
4.2.3 Mediating variable
Industrial structure upgrading (IS): as the scale of the tertiary industry gradually expands, its output level will also be higher than that of the secondary industry, which reflects the change in industrial structure. We adopt the ratio of the value added of the tertiary industry to that of the secondary industry to measure industrial structure upgrading (Luo et al., 2022).
Technological innovation (TI): technological innovation is measured in both inputs and outputs, and innovation outputs better reflect the real innovation capacity of a region than innovation inputs. We refer to the study of Cheng et al. (2023), and adopts the total number of domestic invention patents, utility model patents and design patents applied for authorization to indicate the level of technological innovation.
Environmental regulations (ENV): Pollution emissions can reflect the environmental regulation of a region. We calculate the environmental composite index through the industrial wastewater emissions, industrial sulfur dioxide emissions and industrial soot emissions of the three indicators (Ran et al., 2023), and then take the inverse to get the strength of ENV, the larger the value of ENV, the stronger the environmental regulations.
4.2.4 Control variables
Urbanization level (URB): The urbanization process implies the transfer of rural labor force to cities and towns, which leads to systematic changes in the economic system, social structure and spatial functioning, which involves the choice and use of energy resources, and may affect the green-oriented transition of energy. The ratio of urban population to total population is utilized as a proxy variable for urbanization level.
Foreign direct investment (FDI): On the one hand, FDI can improve energy efficiency and technology level to promote the green-oriented transition of energy (Pan et al., 2020), but on the other hand, it may also increase the dependence on fossil energy. We use the proportion of FDI in GDP to measure this indicator.
Government involvement (GOV): The government has introduced policies, macro-control and other measures to guide enterprises and the public to change the way they use energy, and has increased the development and utilization of green energy. We use the percentage of government expenditure in GDP to measure government involvement.
Marketization level (ML): An increase in the level of marketization is conducive to improving the efficiency of resource allocation and thus the flexibility of energy system operation, and making the production and manufacturing process more green and low-carbon. We adopt the index of China’s marketization process compiled by Fan et al. (2011) as a proxy variable for the marketization level.
4.2.5 Spatial weighting matrices
As the coefficient matrix in the operation process, the spatial weight matrix has an important influence on the result. Considering the representativeness and effectiveness of the spatial weight matrix, the adjacency weight matrix and geographical distance matrix are used to measure the spatial relations of different regions, and the matrices are standardized to carry out spatial correlation test and regression analysis.
Adjacency weight matrix (W1): according to the definition of Queen Neighborhood, if two regions have common edges or common vertices, the weight value is taken to be 1, otherwise the weight is 0. The expression is shown in Eq. 6:
Geographic distance matrix (W2): based on the Euclidean distance between the administrative centers of the two provinces (dij) to construct a geographic distance matrix, the closer the distance the greater the spatial weighting coefficient, the expression is shown in Eq. 7:
4.2.6 Data sources
We use provincial panel data for China from 2011 to 2021 for calculations and empirical analysis. The research excludes Tibet, Hong Kong, Macao, and Taiwan due to the substantial quantity of missing data in these regions. The digital inclusive finance index is measured by the Digital Finance Research Center of Peking University and Ant Financial Services Group (Guo et al., 2020). The number of listed companies in e-commerce parks and intelligent business is calculated by the author from the public website. Data on energy production and consumption were obtained from the “China Energy Statistics Yearbook,” and the remaining indicators and control variables are sourced from the “China Statistical Yearbook,” “China Electronic Information Industry Statistical Yearbook,” “China Science and Technology Statistical Yearbook,” “China Environmental Statistical Yearbook,” China Stock Market & Accounting Research Database, statistical yearbooks of Chinese provinces, autonomous regions, and municipalities. Limited by the availability of data, we used linear interpolation method to complete a few missing data. In addition, all value variables are deflated from the 2011 base period. Table 3 displays the descriptive statistical results of the variables.
5 Results and discussion
5.1 Spatial autocorrelation test
Before conducting spatial econometric analysis, we need to test whether each variable has spatial correlation. Global spatial correlation mainly displays the overall spatial attributes of observed variables, usually expressed by global Moran’s index. Moran’s index has a range of [−1, 1], and a positive (negative) spatial correlation exists between areas when the value of I is larger (lesser) than 0. Table 4 displays the global Moran’s index using the adjacency weight matrix (W1) and geographic distance matrix (W2) of the digital economy and green-oriented transition of energy. Obviously, at the 1%, 5%, or 10% levels, the majority of Moran’s I indexes from 2011 to 2021 are significantly positive, suggesting that the digital economy and the green-oriented transition between regions have obvious positive spatial correlations.
To further verify the spatial connection between the digital economy and green-oriented transition of energy, the observed variables were tested using the local Moran index. We plot the local Moran index scatter plots for 2011 and 2021 for two variables in different matrices, as shown in Figure 3 and Figure 4. (A, B respectively refer to the Moran scatter plot of EGT in 2011 and 2021, and C, D respectively refer to the Moran scatter plot of DIG in 2011 and 2021). It is clear that the first or third quadrants contain the majority of the data, indicating that the level of green-oriented transition of energy and digital economy presents a spatial distribution state of high-high and low-low agglomeration, and the spatial association is significantly positive.
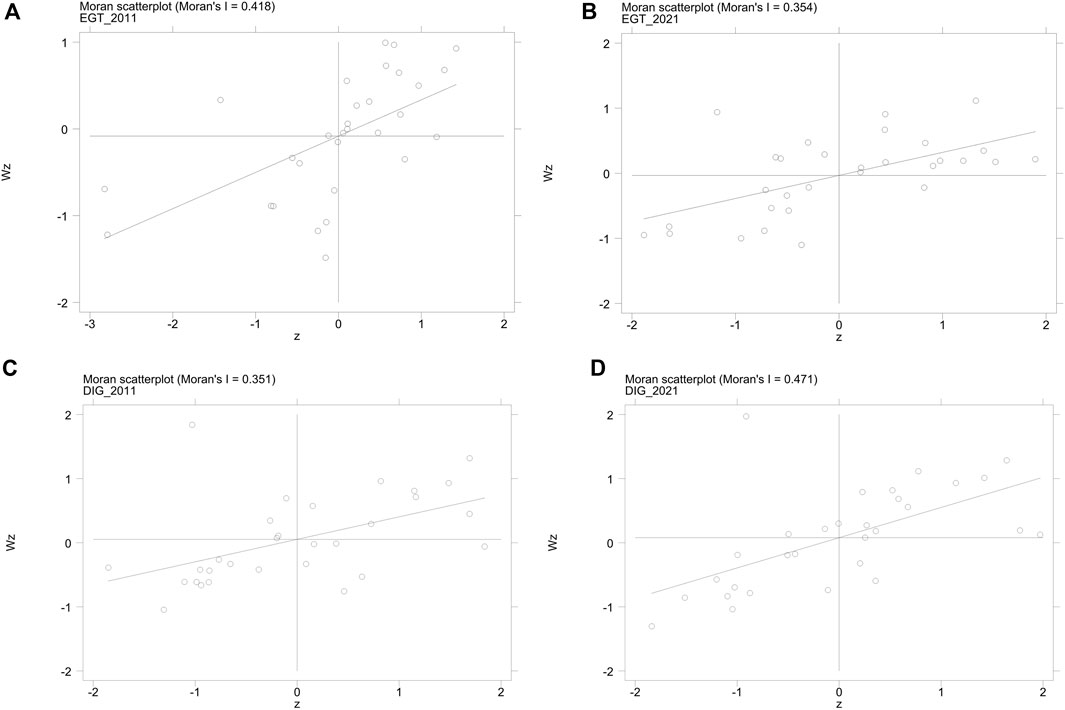
Figure 3. Moran’s I of variables in 2011 and 2021 (W1). (A) Moran scatter plot of EGT in 2011; (B) Moran scatter plot of EGT in 2021; (C) Moran scatter plot of DIG in 2011; (D) Moran scatter plot of DIG in 2021.
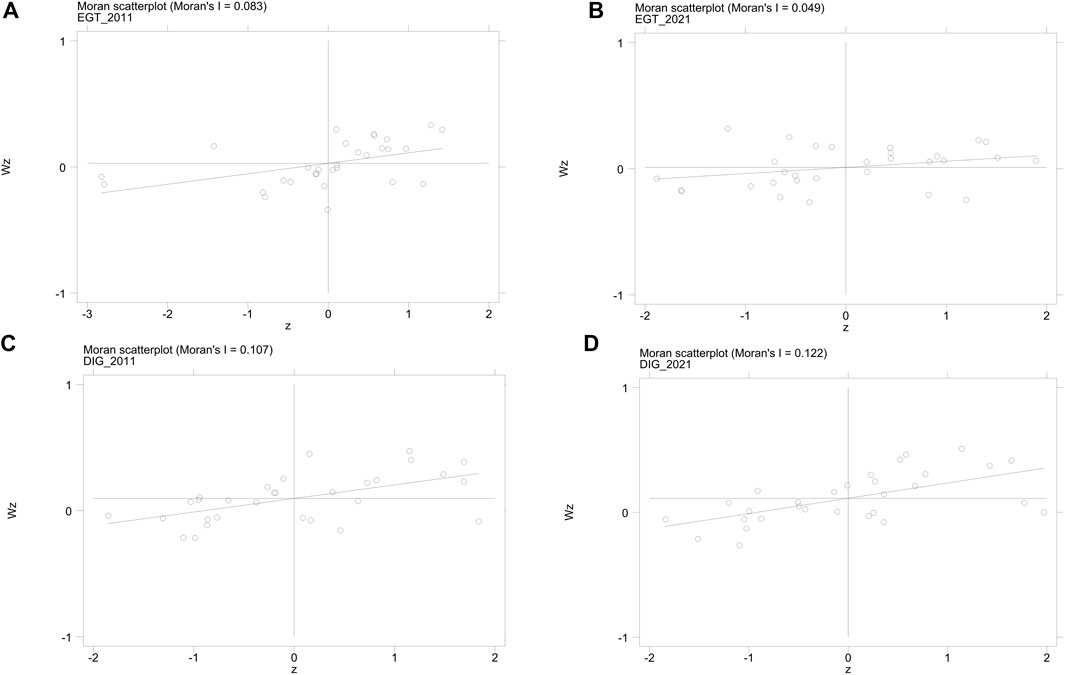
Figure 4. Moran’s I of variables in 2011 and 2021 (W2). (A) Moran scatter plot of EGT in 2011; (B) Moran scatter plot of EGT in 2021; (C) Moran scatter plot of DIG in 2011; (D) Moran scatter plot of DIG in 2021.
5.2 Benchmark regression results
We used the Lagrange multiplier (LM), likelihood ratio (LR), Wald, and Hausman tests in turn to choose an appropriate spatial economic model. First, the LM test indicated that the null hypothesis should be rejected and the spatial econometric model should be adopted instead of the OLS. According to the LR and Wald tests, the SDM was the best choice and it would not degenerate into SAR or SEM. The maximum likelihood estimation (MLE) approach based on the conditional log-likelihood function is used to estimate the SDMs to lessen the bias in the results caused by endogeneity concerns (Anselin, 1988). In addition, the Hausman test result indicates that the fixed effects SDM was the right adoption. Compared to the spatial fixed and double fixed effects, the SDM with time fixed effects provided a superior fit for the model regressions. The estimation results are shown in Table 5.
From the benchmark results of SDM, it can be seen that before and after adding the control variables, the coefficients of lnDIG pass the 1% significance test and are positive under both weight matrices, the hypothesis H1 is accepted, which indicates that the improvement of the digital economy can positively promote green-oriented transition of energy of the region. In columns (2) and (4), the spatial lag coefficient of lnDIG is significantly positive, indicating that the digital economy can also promote the green-oriented transition of energy in neighboring areas, which is consistent with hypothesis H2. Digital technology is profoundly changing the production, distribution, and consumption patterns of energy, constantly giving rise to new models and formats such as smart energy systems, comprehensive energy systems that complement multiple energy sources, and collaborative systems for renewable energy and electric vehicles. This provides a broad development market for renewable energy and expands its power generation scale, leading energy towards clean and low-carbon development. This research refers to LeSage and Pace (2010), using partial differential methods to decompose the spatial effects into direct, indirect, and total effects to correctly depict the spatial spillover effects between the variables. Table 6 displays the results.
The results indicate that the direct effect coefficient of lnDIG is significantly positive under both spatial matrices, indicating that the development of the digital economy in general has a significant role in promoting the green-oriented transition of energy. Digital means play an important role in the planning, design, operation and other stages of energy systems. By creating virtual physical system models, physical systems can be simulated, verified, predicted, and controlled, providing decision support for energy system optimization and energy-saving transformation, and helping clean, low-carbon, and efficient use of energy. In addition, digitalization is the mainstream trend of China’s industrial transformation, and the government has increased investment in energy digitalization projects to encourage energy companies to accelerate the pace of industrial digital transformation (Li and Zhan, 2018), promoted the proportion of clean energy such as electricity in energy consumption, and further optimized the energy structure. the coefficients of the indirect effect of lnDIG under both matrices pass the significance test and is positive, indicating that the development of the digital economy can have an impact on neighboring regions through resource sharing, talent exchange and trade circulation. On the energy production side, the investment in green projects and the introduction of technical talents will accelerate the development and utilization of clean energy, thus increasing the proportion of renewable energy generation. On the energy consumption side, the application of digital technology will make cross-regional cooperation and communication smarter and more convenient, shorten the response time of enterprises and change the allocation and use ratio of energy resources, thus promoting the further optimization of the energy structure of neighboring regions.
According to the control variables, the coefficients of the direct, indirect and total effects of the level of urbanization are all negative, and one possible explanation is that, because China is in the process of urbanization, there is a greater demand for infrastructure materials in the expansion of the city scale, and most of the energy used in the production of construction materials is dominated by low-quality energy sources, such as coal, which impedes the green-oriented transition of energy. The direct effect of FDI is positive, while the indirect and total effects are negative, probably because FDI is conducive to the introduction of new equipment and technology to promote the low-carbon development of the energy industry in the region, while the existence of industry competition and the adoption of technological blockades and other measures by enterprises in different regions will be detrimental to the green-oriented transition of energy in the neighboring regions. The coefficients of government involvement on green-oriented transition of energy are all positive, indicating that government policy measures and related financial expenditures can promote the development of renewable energy industry (Pan et al., 2023), and thus have a positive impact on the green-oriented transition of energy. In addition, the continuous improvement of the degree of marketization can further realize the optimal allocation of energy resources and improve the flexibility of the inter-regional energy system, thus promoting the green-oriented transition of energy.
5.3 Robustness test
In the above analysis, different spatial weight matrices were applied to regress the spatial Durbin model, and the estimated coefficients of the core explanatory variables were relatively similar in terms of significance and direction, and we will further test the robustness of the empirical results using three methods. First, we re-measure the level of digital economy. The White Paper on China’s Digital Economy Development (2022) released by the China Academy of Information and Communication Research (CAICR) points out that the scale of China’s digital economy reaches 45.5 trillion yuan in 2021, while the scale of industrial digitization reaches 37.2 trillion yuan, and its contribution to the total digital economy exceeds 80%, that is to say, industrial digitization is a key force for the accelerated development of the digital economy. Therefore, we apply the entropy method to measure the four indicators in industrial digitization and use them as proxy variables for digital economy. The regression results are shown in Table 7. It can be found that the digital economy can significantly promote the green-oriented transition of energy and produce spatial spillover effects, which means the results are robust. Second, the generalized spatial two-stage least squares (GS2SLS) method is used for estimation to attenuate the endogeneity problem arising in the model (Kelejian and Prucha, 1998). Since SDM already contains the first-order spatial lag term of each explanatory variable, which means that the number of potential strong instrumental variables in the model is small, so the instrumental variables should contain at least the second-order spatial lag term of the explanatory variables. To avoid the problems caused by weak instrumental variables, we choose the highest third-order spatial lag term as the instrumental variable. The results of column (2) and column (5) in Table 7 indicate that the green-oriented transition of energy has significant spatial spillovers, and that the digital economy plays a significant contributing role, which is consistent with the previous results and confirms the robustness of the results. Third, given that the outbreak of the New Crown Pneumonia epidemic at the beginning of 2020 had a large impact on energy demand, it may affect the green-oriented transition of energy to a certain extent. After re-estimating the model by setting the sample years as 2011-2019, the digital economy and its lagged term coefficients are significantly positive and the size of the coefficients have increased, which again verifies the reliability of the conclusions.
5.4 Mechanism verification
This paper draws on the stepwise test method of Wen et al. (2004) to analyze the multiple mediating effects under the spatial Durbin model, and the decomposition results of the spatial spillover effects of each mediating variable are shown in Table 8 and Table 9. The regression results of Formula 1 show that the development of digital economy has a significant positive impact on the green-oriented transition of energy, so it satisfies the preconditions of the stepwise regression method.
As evident from the findings presented in Table 8, The coefficient of lnDIG in column (1) is 0.326, which is significant at the level of 1%, indicating that the development of digital economy can effectively promote the upgrading of industrial structure. The coefficients of lnDIG and lnIS in column (2) both pass the significance test, and the coefficient of lnIS is 0.072, indicating that hypothesis H3 is verified. The digital economy can indirectly promote the green-oriented transition of energy in the region through promoting the upgrading of its industrial structure, and the indirect effect accounted for 15.3%. The coefficient of lnDIG in column (3) is 1.122, which implies that the development of digital economy has a significant positive impact on technological innovation. The coefficients of lnDIG and lnTI in column (4) are both significantly positive, and the coefficient of lnTI is 0.035, which proves that hypothesis H4 is verified and part of the mediating effect of technological innovation is established, the proportion is 25.7%. When environmental regulation is used as a mediating variable, the coefficient of lnDIG in column (5) is 0.443 and passes the significance test, indicating that hypothesis H5 is verified, the digital economy development can effectively strengthen the environmental regulation. The coefficients of lnDIG and lnENV in column (6) are both significantly positive, and the coefficient of lnENV is 0.080, which indicates that the development of the digital economy strengthens the environmental regulation and further promotes the process of the green-oriented transition of energy. Among them, the indirect effect of environmental regulation is 23.1%. In summary, the digital economy can indirectly promote the green-oriented transition of energy in the region through the three paths of industrial structure upgrading, technological innovation and environmental regulation. Furthermore, it can be seen that the size of the indirect effect of the three intermediary variables is in the following order: technological innovation, environmental regulations, industrial structure upgrading.
6 Conclusion and policy implications
Based on the standardized analysis of the theoretical mechanism of the impact of digital economic development on green-oriented transition of energy, this paper takes the panel data of 30 provinces in China from 2011 to 2020 as a sample to comprehensively measure China’s green-oriented transition of energy level and digital economy development index by constructing a comprehensive index system. Spatial Durbin model and multiple mediation model are used to verify the spatial effect and transmission mechanism of digital economy development affecting green-oriented transition of energy. The following conclusions are drawn: First, the overall level of China’s green-oriented transition of energy is on a rising trend year by year, and the transition level of the eastern coastal regions is generally high, while some western provinces and regions rich in renewable resources have outstanding catching-up ability. Second, there is an obvious spatial correlation between the development of digital economy and green-oriented transition of energy in different regions. Digital economy not only has a significant positive contribution to the green-oriented transition of energy in its own region, but also promotes the green-oriented transition of energy process in neighboring provinces. Finally, the development of digital economy can promote the green-oriented transition of energy through three mediation pathways, including industrial structure upgrading, technological innovation, and environmental regulations, which has been further confirmed by robustness tests.
Based on the above conclusions, this paper puts forward the following policy recommendations:
First, on the energy supply side, it is necessary to deeply promote the in-depth integration and application innovation of digital technology and the energy industry by widely applying digital technology to the energy system, to break the vertical development pattern between the energy systems of electricity, petroleum, natural gas, and coal, accelerate the development of energy grid, new power system, smart energy, and other energy systems, and promote the efficient development and utilization of renewable energy sources such as wind and solar energy and their substitution for fossil energy sources, thus promoting the green-oriented transition of energy production. On the energy consumption side, we should accelerate the integration of digital economy and high energy-consuming industries, to enhance the efficiency of the whole industry and the allocation of all factors as well as the innovation of the value creation model, and promote quality change, efficiency change, power change of industrial development and drive the transition of energy consumption, which stimulates the transition of energy production in return.
Second, given that the positive impact of digital economy on the green-oriented transition of energy has a spatial spillover effect, each region should firstly formulate a synergistic optimization strategy based on the differences in the level of green-oriented transition of energy, draw on and absorb the beneficial experience of neighboring regions, and strengthen cooperation and exchanges in green energy innovation and application while continuing to improve the construction of their own digital infrastructures. In addition, spatial spillover effect of digital economy should be made full use of to form a regional linkage to promote the green synergistic development of industries, thus promoting the green-oriented transition of energy in each region.
Finally, promoting green-oriented transition of energy can also be considered from three aspects: industrial structure upgrading, technological innovation and environmental regulations. The depth and breadth of the integration of digital technology and industry should be accelerated in order to reshape the industrial ecology and coordinate supply and demand, further extend the digital industrial chain, and promote the transformation and upgrading of industry in the direction of higher-end, green-oriented, and intelligent. It is necessary to break through the barriers of technological innovation in energy production, promote the conversion and utilization of renewable energy in energy production, and accelerate the introduction of green intelligent equipment, low-carbon production processes, new energy storage, etc., so as to provide strong technical support for the optimization of energy consumption structure. To accelerate the construction of a digital energy supervisory platform, strengthen the intelligent monitoring of energy production, storage and consumption, dynamically formulate and implement control measures in relevant areas to accelerate the consumption of clean energy on the production side and the substitution of electric energy on the consumption side, and promote the comprehensive and synergistic promotion of the green-oriented transition of energy.
Although this article has explored the impact mechanism of the digital economy on green-oriented transition of energy, there are several limitations that need to be noted. First, the definition of the digital economy is broad, and there is still room for improvement in the establishment of the index system and its measurement. Second, considering the completeness and availability of data source, this article selects samples at the provincial level for empirical analysis. Future research could further explore the relationship between the digital economy and green-oriented transition of energy at the urban level. Third, due to the methodological limitations of this paper, the impact of the digital economy on green-oriented transition of energy has not been fully explained from the perspectives of heterogeneity, nonlinear effect, or policy impact, and this is a direction worth exploring in the future.
Data availability statement
The raw data supporting the conclusion of this article will be made available by the authors, without undue reservation.
Author contributions
JH: Conceptualization, Methodology, Supervision, Writing–original draft, Writing–review and editing. SY: Data curation, Formal Analysis, Methodology, Software, Writing–original draft.
Funding
The author(s) declare that financial support was received for the research, authorship, and/or publication of this article. The article is sponsored by the National Social Science Foundation of China (Grant No. 23BJY196).
Conflict of interest
The authors declare that the research was conducted in the absence of any commercial or financial relationships that could be construed as a potential conflict of interest.
Publisher’s note
All claims expressed in this article are solely those of the authors and do not necessarily represent those of their affiliated organizations, or those of the publisher, the editors and the reviewers. Any product that may be evaluated in this article, or claim that may be made by its manufacturer, is not guaranteed or endorsed by the publisher.
References
Ahmadpour, A., Mokaramian, E., and Anderson, S. (2021). The effects of the renewable energies penetration on the surplus welfare under energy policy. Renew. Energy 164, 1171–1182. doi:10.1016/j.renene.2020.10.140
Anselin, L. (1988). Spatial econometrics: methods and models. Dordrecht: Kluwer Academic Publishers. doi:10.2307/143780
Bashir, M. F., Pan, Y., Shahbaz, M., and Ghosh, S. (2023). How energy transition and environmental innovation ensure environmental sustainability? Contextual evidence from Top-10 manufacturing countries. Renew. Energy 204, 697–709. doi:10.1016/j.renene.2023.01.049
Cheng, Y., Zhang, Y., Wang, J., and Jiang, J. (2023). The impact of the urban digital economy on China’s carbon intensity: spatial spillover and mediating effect. Resour. Conserv. Recycl. 189, 106762. doi:10.1016/j.resconrec.2022.106762
De La Peña, L., Guo, R., Cao, X., Ni, X., and Zhang, W. (2022). Accelerating the energy transition to achieve carbon neutrality. Resour. Conserv. Recycl. 177, 105957. doi:10.1016/j.resconrec.2021.105957
Dong, F., Hu, M., Gao, Y., Liu, Y., Zhu, J., and Pan, Y. (2022). How does digital economy affect carbon emissions? Evidence from global 60 countries. Sci. Total Environ. 852, 158401. doi:10.1016/j.scitotenv.2022.158401
Du, J., Shen, Z., Song, M., and Zhang, L. (2023). Nexus between digital transformation and energy technology innovation: an empirical test of A-share listed enterprises. Energy Econ. 120, 106572. doi:10.1016/j.eneco.2023.106572
Dutil, P., and Williams, J. (2017). Regulation governance in the digital era: a new research agenda. Can. Public Adm. 60, 562–580. doi:10.1111/capa.12226
Fan, G., Wang, X., and Ma, G. (2011). Contribution of marketization to China’s economic growth. Econ. Res. J. 46, 4–16.
Fernández-Portillo, A., Almodóvar-González, M., and Hernández-Mogollón, R. (2020). Impact of ICT development on economic growth. A study of OECD European Union countries. Technol. Soc. 63, 101420. doi:10.1016/j.techsoc.2020.101420
G20 Research Group (2016). G20 digital economy development and cooperation initiative. Canada: University of Toronto. G20 Research Group.
Guo, F., Wang, J., Wang, F., Kong, T., Zhang, X., and Cheng, Z. (2020). Measuring China’s digital financial inclusion: index compilation and spatial characteristics. China Econ. Q. 19, 1401–1418.
Guo, P., Kong, J., Guo, Y., and Liu, X. (2019). Identifying the influencing factors of the sustainable energy transitions in China. J. Clean. Prod. 215, 757–766. doi:10.1016/j.jclepro.2019.01.107
Hainsch, K., Löffler, K., Burandt, T., Auer, H., Crespo Del Granado, P., Pisciella, P., et al. (2022). Energy transition scenarios: what policies, societal attitudes, and technology developments will realize the EU Green Deal? Energy 239, 122067. doi:10.1016/j.energy.2021.122067
Hefner, R. A. (2013). The grand energy transition: the rise and the next great economic expansion. Beijing, China: CITIC Press Group.
Huang, L., and Zou, Y. (2020). How to promote energy transition in China: from the perspectives of interregional relocation and environmental regulation. Energy Econ. 92, 104996. doi:10.1016/j.eneco.2020.104996
Huang, S.-Z. (2022). The effect of natural resources and economic factors on energy transition: new evidence from China. Resour. Policy 76, 102620. doi:10.1016/j.resourpol.2022.102620
International Energy Agency (2022). Global energy review: CO2 emissions in 2021. Paris: International Energy Agency.
Kelejian, H. H., and Prucha, I. R. (1998). A generalized spatial two-stage least squares procedure for estimating a spatial autoregressive model with autoregressive disturbances. J. Real Estate Finance Econ. 17, 99–121. doi:10.1023/A:1007707430416
Kim, J., Park, J. C., and Komarek, T. (2021). The impact of Mobile ICT on national productivity in developed and developing countries. Inf. Manage. 58, 103442. doi:10.1016/j.im.2021.103442
LeSage, J. P., and Pace, R. K. (2010). “Spatial econometric models,” in Handbook of applied spatial analysis. Editors M. M. Fischer, and A. Getis (Berlin, Heidelberg: Springer Berlin Heidelberg), 355–376. doi:10.1007/978-3-642-03647-7_18
Li, B. J., and Zhang, Q. Q. (2017). Analysis of the balance of regional energy supply and demand structure and economic impact in China. Econ. Inq., 39–46.
Li, J., and Zhan, K. (2018). Intelligent mining technology for an underground metal mine based on unmanned equipment. Engineering 4, 381–391. doi:10.1016/j.eng.2018.05.013
Li, J., Zhou, S., Wei, W., Qi, J., Li, Y., Chen, B., et al. (2020a). China’s retrofitting measures in coal-fired power plants bring significant mercury-related health benefits. One Earth 3, 777–787. doi:10.1016/j.oneear.2020.11.012
Li, K., Kim, D. J., Lang, K. R., Kauffman, R. J., and Naldi, M. (2020b). How should we understand the digital economy in Asia? Critical assessment and research agenda. Electron. Commer. Res. Appl. 44, 101004. doi:10.1016/j.elerap.2020.101004
Li, Z., and Wang, J. (2022). The dynamic impact of digital economy on carbon emission reduction: evidence city-level empirical data in China. J. Clean. Prod. 351, 131570. doi:10.1016/j.jclepro.2022.131570
Loebbecke, C., and Picot, A. (2015). Reflections on societal and business model transformation arising from digitization and big data analytics: a research agenda. J. Strateg. Inf. Syst. 24, 149–157. doi:10.1016/j.jsis.2015.08.002
Loock, M. (2020). Unlocking the value of digitalization for the European energy transition: a typology of innovative business models. Energy Res. Soc. Sci. 69, 101740. doi:10.1016/j.erss.2020.101740
Luo, K., Liu, Y., Chen, P.-F., and Zeng, M. (2022). Assessing the impact of digital economy on green development efficiency in the Yangtze River Economic Belt. Energy Econ. 112, 106127. doi:10.1016/j.eneco.2022.106127
Lyu, Y., Wang, W., Wu, Y., and Zhang, J. (2023). How does digital economy affect green total factor productivity? Evidence from. China. Sci. Total Environ. 857, 159428. doi:10.1016/j.scitotenv.2022.159428
Ma, D., and Zhu, Q. (2022). Innovation in emerging economies: research on the digital economy driving high-quality green development. J. Bus. Res. 145, 801–813. doi:10.1016/j.jbusres.2022.03.041
Markard, J., Raven, R., and Truffer, B. (2012). Sustainability transitions: an emerging field of research and its prospects. Res. Policy 41, 955–967. doi:10.1016/j.respol.2012.02.013
Mazzucato, M., and Semieniuk, G. (2018). Financing renewable energy: who is financing what and why it matters. Technol. Forecast. Soc. Change 127, 8–22. doi:10.1016/j.techfore.2017.05.021
Millot, A., and Maïzi, N. (2021). From open-loop energy revolutions to closed-loop transition: what drives carbon neutrality?. Technol. Forecast. Soc. Change. 172, 121003. doi:10.1016/j.techfore.2021.121003
Ozturk, I., and Ullah, S. (2022). Does digital financial inclusion matter for economic growth and environmental sustainability in OBRI economies? An empirical analysis. Resour.Conserv. Recycl. 185, 106489. doi:10.1016/j.resconrec.2022.106489
Pan, X., Guo, S., Han, C., Wang, M., Song, J., and Liao, X. (2020). Influence of FDI quality on energy efficiency in China based on seemingly unrelated regression method. Energy 192, 116463. doi:10.1016/j.energy.2019.116463
Pan, X. Y., Shen, Z. Y., Song, M. L., and Shu, Y. L. (2023a). Enhancing green technology innovation through enterprise environmental governance: a life cycle perspective with moderator analysis of dynamic innovation capability. Energy Policy 182, 113773. doi:10.1016/j.enpol.2023.113773
Pan, X.-Y., Yuan, G., Wu, X.-H., and Xie, P.-J. (2023b). The effects of government subsidies on the economic profits of hydrogen energy enterprises-An analysis based on A-share listed enterprises in China. Renew. Energy 211, 445–451. doi:10.1016/j.renene.2023.04.093
Ran, Q., Yang, X., Yan, H., Xu, Y., and Cao, J. (2023). Natural resource consumption and industrial green transformation: does the digital economy matter? Resour. Policy 81, 103396. doi:10.1016/j.resourpol.2023.103396
Saldanha, T. J., and Mithas, S. (2021). Leveraging Customer Involvement for Fueling Innovation: The Role of Relational and Analytical Information Processing Capabilities. MIS Q. 41, 367–396. doi:10.25300/MISQ/2017/41.1.14
Shahbaz, M., Wang, J., Dong, K., and Zhao, J. (2022). The impact of digital economy on energy transition across the globe: the mediating role of government governance. Renew. Sustain. Energy Rev. 166, 112620. doi:10.1016/j.rser.2022.112620
Tian, J., Yu, L., Xue, R., Zhuang, S., and Shan, Y. (2022). Global low-carbon energy transition in the post-COVID-19 era. Appl. Energy 307, 118205. doi:10.1016/j.apenergy.2021.118205
Veskioja, K., Soe, R.-M., and Kisel, E. (2022). Implications of digitalization in facilitating socio-technical energy transitions in Europe. Energy Res. Soc. Sci. 91, 102720. doi:10.1016/j.erss.2022.102720
Wang, B., Wang, J., Dong, K., and Dong, X. (2023). Is the digital economy conducive to the development of renewable energy in Asia? Energy Policy 173, 113381. doi:10.1016/j.enpol.2022.113381
Wang, L., and Shao, J. (2023). Digital economy, entrepreneurship and energy efficiency. Energy 269, 126801. doi:10.1016/j.energy.2023.126801
Wen, Z., Zhang, L., Hou, J., and Liu, H. (2004). Testing and application of the mediating effects. Acta Psychol. Sin. 36, 614–620.
Williams, L. D. (2021). Concepts of digital economy and industry 4.0 in intelligent and information systems. Int. J. Intell. Netw. 2, 122–129. doi:10.1016/j.ijin.2021.09.002
Winskel, M., and Kattirtzi, M. (2020). Transitions, disruptions and revolutions: expert views on prospects for a smart and local energy revolution in the UK. Energy Policy 147, 111815. doi:10.1016/j.enpol.2020.111815
Wu, L., Wan, X., Jahanger, A., Li, M., Murshed, M., and Balsalobre-Lorente, D. (2023). Does the digital economy reduce air pollution in China? A perspective from industrial agglomeration. Energy Rep. 9, 3625–3641. doi:10.1016/j.egyr.2023.02.031
Xue, Y., Tang, C., Wu, H., Liu, J., and Hao, Y. (2022). The emerging driving force of energy consumption in China: does digital economy development matter? Energy Policy 165, 112997. doi:10.1016/j.enpol.2022.112997
Yi, M., Liu, Y., Sheng, M. S., and Wen, L. (2022). Effects of digital economy on carbon emission reduction: new evidence from China. Energy Policy 171, 113271. doi:10.1016/j.enpol.2022.113271
You, W., and Lv, Z. (2018). Spillover effects of economic globalization on CO2 emissions: a spatial panel approach. Energy Econ. 73, 248–257. doi:10.1016/j.eneco.2018.05.016
Yuan, S., Musibau, H. O., Genç, S. Y., Shaheen, R., Ameen, A., and Tan, Z. (2021). Digitalization of economy is the key factor behind fourth industrial revolution: how G7 countries are overcoming with the financing issues? Technol. Forecast. Soc. Change 165, 120533. doi:10.1016/j.techfore.2020.120533
Keywords: digital economy, energy transition, multiple mediation effect, spatial effects, spatial Durbin model
Citation: Hou J and Ye S (2024) The impact of digital economy on China’s green-oriented transition of energy: multiple mediation effects from a spatial perspective. Front. Energy Res. 12:1429276. doi: 10.3389/fenrg.2024.1429276
Received: 07 May 2024; Accepted: 04 June 2024;
Published: 01 July 2024.
Edited by:
Haonan Zhang, North China Electric Power University, ChinaReviewed by:
Pin Li, Xi’an University of Science and Technology, ChinaJuntao Du, Anhui University of Finance and Economics, China
Zhengmao Li, Aalto University, Finland
Copyright © 2024 Hou and Ye. This is an open-access article distributed under the terms of the Creative Commons Attribution License (CC BY). The use, distribution or reproduction in other forums is permitted, provided the original author(s) and the copyright owner(s) are credited and that the original publication in this journal is cited, in accordance with accepted academic practice. No use, distribution or reproduction is permitted which does not comply with these terms.
*Correspondence: Jianchao Hou, aGRzaHVveXVAMTI2LmNvbQ==