- 1Industrial Process and Energy Systems Engineering Group, Institute of Mechanical Engineering, École Polytechnique Fédérale de Lausanne (EPFL), Sion, Switzerland
- 2CIRAIG, Institute for Sustainable Energy, School of Engineering, University of Applied Sciences Western Switzerland, Sion, Switzerland
- 3CIRAIG, Montréal, QC, Canada
The global transition to decentralized energy systems signifies a fundamental transformation toward sustainable energy paradigms. This study specifically focuses on the Swiss energy system, analyzing how dynamic pricing influences the strategic decisions of different actors. The main contributions include 1) a detailed examination of pricing models tailored to the Swiss context, 2) an exploration of strategic financial burden shifts among end-users, TSOs, and DSOs, and 3) a comparison of decentralized versus centralized energy models, highlighting their respective efficiencies and resilience. This research differentiates from existing literature by providing an in-depth actor-based analysis within a Swiss context, offering valuable insights into decentralized energy system optimization. This study tackles the problem of how pricing influences strategic decisions across different actors in Switzerland’s evolving decentralized energy landscape. Here we show that a carefully tailored pricing model, designed for the Swiss context, enables optimized strategies that balance local efficiencies with systemic equity and resilience. The analysis reveals that decentralized approaches, in contrast to centralized models, not only accommodate diverse stakeholder preferences but also enhance system robustness against market and operational disruptions. Moreover, the study illustrates the strategic financial burden shifting where end-users compensate for cost shifts, with observed additional costs up to 5200 CHF/year cap when service providers are prioritized as objective actors. Notably, the most frequently selected system configuration in the primal problem, which optimizes the total system costs, aligns with the preferences of TSO and DSO for a 47.1 GW PV deployment. However, end-users demonstrate a preference for increased PV installations, constrained by urban grid capacities. Additionally, the study highlights significant regional disparities across Switzerland, necessitating tailored pricing approaches that reflect varied urban forms. The emergence of prosumers catalyzes new business models, redistributing investments across TSOs (256–261 CHF/cap/year), DSOs (244–413 CHF/cap/year), and prosumers (556–764 CHF/cap/year), showcasing the evolving dynamics of energy system economics.
Highlights
1 Introduction
1.1 Background
The transition from centralized to decentralized energy systems highlights a pivotal shift in the global pursuit of sustainable and resilient energy paradigms. This evolution is often modeled using optimization and simulation techniques and promises to redefine energy generation and consumption. However, such models are based on centralized decision-making objectives and fall short of capturing the nuanced roles and localized objectives of multiple actors inherent in energy systems. This approach assumes the existence of a single centralized actor primarily seeking system-wide optimal solutions. It does not adequately address individual stakeholders’ diverse and sometimes conflicting goals in the energy transition and, therefore, does not consider equity in the energy system design.
Switzerland serves as a prime example in this research, not as the sole focus but as a validation of methodologies applicable to decentralized energy systems. The Swiss strategic shift towards a decentralized, carbon-neutral energy future illustrates the transition from traditional, centralized energy infrastructures. This move towards local renewable energy sources and efficiency is driven by environmental imperatives and a more distributed system’s economic and societal advantages. Nonetheless, this transition transcends national boundaries, reflecting a global trend towards decentralization, with Switzerland’s experience offering valuable insights into addressing the associated financial, regulatory, and technological challenges.
Incorporating decentralized energy system options into the Swiss energy model demonstrates their attractiveness over centralized solutions, which naturally gravitates towards less expensive and more efficient alternatives (Chuat et al., 2024). This observation underscores the need to reevaluate financial mechanisms and regulatory frameworks to foster investments in renewable energy technologies and infrastructure. The shift to decentralization brings critical considerations about investment strategies, risk management, and the equitable distribution of financial benefits among various stakeholders, including individuals, communities, and small businesses.
Furthermore, the advent of decentralized energy production signifies a profound transformation in the roles of traditional energy system actors. The bidirectional energy flow in decentralized systems, where prosumers consume and generate renewable energy locally, challenges the conventional unidirectional grid. This shift necessitates a comprehensive reimagining of the energy landscape, including grid modernization to accommodate bidirectional flows, the development of smart grid technologies for real-time supply and demand balance, and the establishment of tariffs reflective of the variable nature of renewable energy production.
2 State of the art
2.1 Literature review
The field of energy system modeling has become increasingly important as the global community seeks sustainable solutions to meet energy demands. Integrating multiple actors within these systems presents a complex challenge, necessitating innovative approaches to ensure efficient, sustainable energy harvesting, distribution, and management (Wang et al., 2020). However, the depth of understanding regarding these actors’ economic and strategic interactions still needs to be improved, as given in the overview of selected models compared in Table 1. This study aims to fill this gap by exploring various stakeholders’ economic trade-offs and strategic decisions within the Swiss energy system.
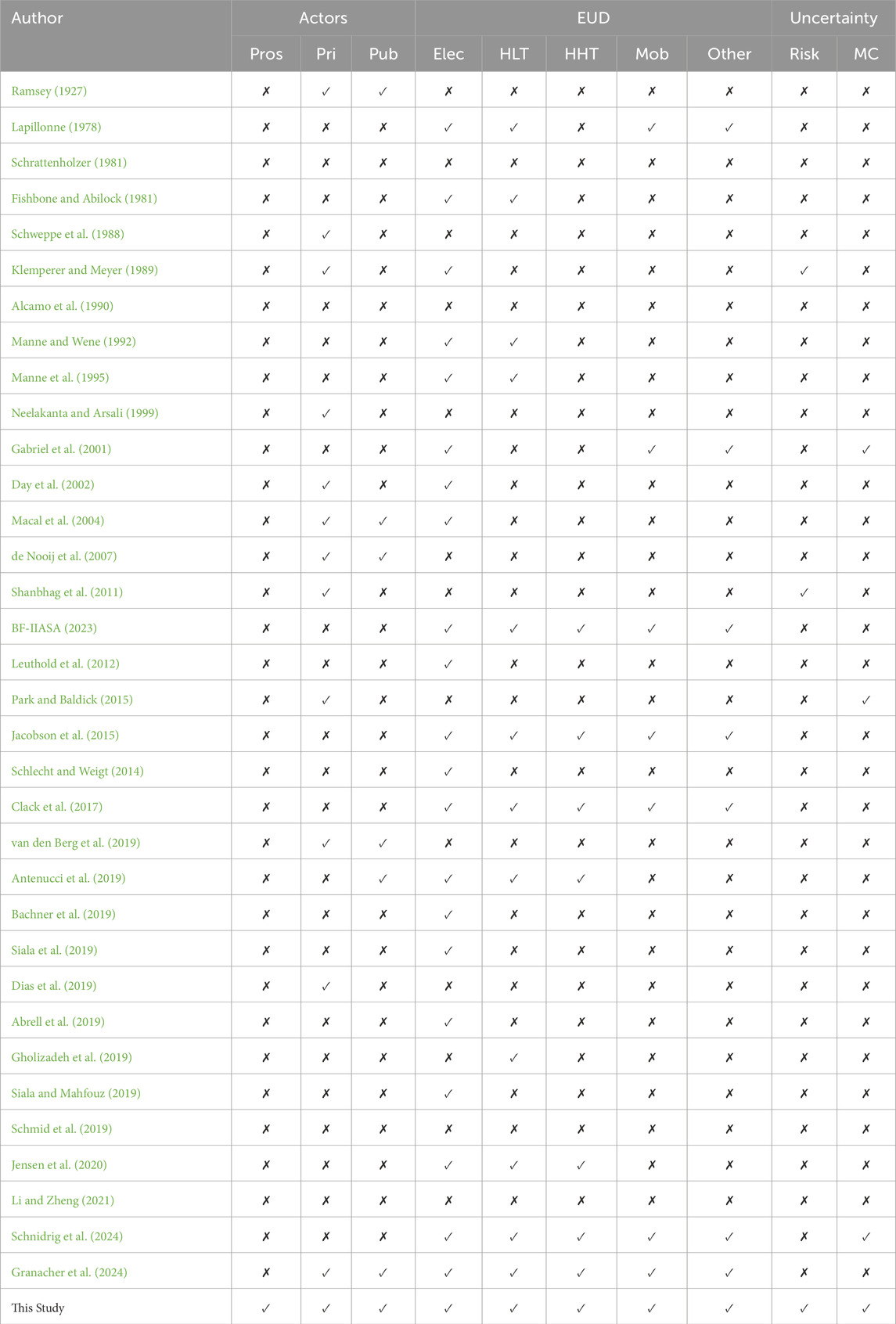
Table 1. National and international energy system model selection of actors integration overview, selected based on the actors and End-Uses Demands considered. Legend: ✗ feature not considered, ✓ feature considered. Acronyms: Prosumers (Pros), Private (Pri), Public (Pub), Electricity (Elec), Heat Low Temperature (HLT), Heat High Temperature (HHT), Mobility (Mob), Monte-Carlo Analysis (MC).
Optimization techniques have been applied to address the design and operation of energy systems. Traditionally, system design has been approached from a universal decision-maker perspective, using cost minimization to model society’s decisions without considering the impacts on individual system actors (Schär and Geldermann, 2021). This approach often yields optimal solutions on a system level but may not be practical or acceptable to all stakeholders (Stidham, 1992; Kelman et al., 2013). Recent advancements have introduced multi-actor models, which consider the diverse objectives and behaviors of different stakeholders (González-Briones et al., 2018; Roche et al., 2010). Despite these advancements, there remains a notable gap in the literature concerning integrating economic and market dynamics within these models, particularly in decentralized energy systems. Agent-based modeling introduces a multi-actor perspective, allowing for exploring individual needs and behaviors within the system. This approach has been instrumental in grid management and energy system optimization, acknowledging the growing complexity and interconnectedness of modern energy systems (González-Briones et al., 2018; Roche et al., 2010). Moreover, game theory, especially leader-follower models, provides a framework for understanding the strategic cooperative and competitive interactions between different actors within the energy system. These models help in identifying solutions that consider the responses and objectives of multiple agents in the system, facilitating a more cooperative approach to system design (Yu and Hong, 2017; Sarfarazi et al., 2020; Du et al., 2019). However, applying these theoretical models to real-world scenarios remains underexplored, particularly in pricing strategies and market dynamics in decentralized energy systems.
The development of optimization-based energy models has progressed from focusing on specific sectors to encompassing entire systems. However, these models often lack detailed consideration of economic and market influences on the system design. A selection of energy system models containing stakeholder features is represented in Table 1. Models such as EnergyScope (Moret et al., 2017; Limpens et al., 2019; Limpens, 2021; Li et al., 2020; Schnidrig et al., 2023), OEMOF (Hilpert et al., 2018), CALLIOPE (Pfenninger et al., 2014), and OSeMOSYS (Howells et al., 2011) have represented significant advancements in cross-sectoral energy system modeling. Still, integrating energy policy and market mechanisms within these models remains limited. Furthermore, there is a lack of comprehensive models that address the systemic impact of pricing, equitable resource distribution, and infrastructure investment in decentralized energy systems. Historical assessments and case studies have been employed to understand the impacts of energy policies and economic and market dynamics (Hudson and Jorgenson, 1974; Lee and Shih, 2010). However, there is a notable gap in models that simultaneously integrate economic and market dynamics with the design and operation of energy systems, considering the variety of novel technologies that can be activated at different scales.
The trend towards decentralized energy systems has highlighted the need for new models to effectively address the systemic impact of pricing, equitable resource distribution, and infrastructure investment (Wang et al., 2020). Notably, the relationship between infrastructure investment and resource pricing in decentralized systems has been under-explored, with existing studies not fully capturing the complexities of those interactions (Schär and Geldermann, 2021). Lastly, research into pricing strategies that enhance the resilience of decentralized energy systems against the market and physical disruptions is limited, indicating a significant gap in the current body of knowledge (Du et al., 2019). This study aims to address these gaps by developing and applying a nuanced pricing model tailored to the Swiss energy system’s unique context, uncovering optimal pricing strategies for energy services and vectors, and analyzing the preferences of different system actors for specific energy configurations.
2.2 Identified gaps and contribution
Despite significant advancements in energy system modeling, especially in transitioning towards decentralized systems, a considerable gap exists in comprehensively understanding and optimizing pricing mechanisms for energy flows. This research aims to bridge these gaps by employing a nuanced pricing model tailored to the Swiss energy system’s unique context. This model is designed to uncover the optimal pricing strategies for energy services and vectors, shedding light on the preferences of different system actors for specific energy configurations and their implications on the broader energy landscape.
This investigation identifies several critical areas that have been underexplored in existing literature:
To address these gaps, this study sets forth the following objectives:
1. Develop and apply a pricing model that captures the economic trade-offs and strategic interactions among key actors in the energy system, accommodating varying levels of decentralization and renewable energy integration.
2. Analyze the impact of spatial and energetic characteristics of prosumers on their preferences for energy system configurations and assess the consequences on required grid capacity, energy pricing, and the integration of renewable energy sources.
3. Evaluate how different energy system configurations align with or diverge from the interests of TSOs, DSOs, and prosumers, and explore the implications for economic dynamics, market strategies, and investment in infrastructure.
This approach targets the complexities inherent in decentralized energy systems and provides a pioneering analysis of the economic and strategic considerations influencing actor-specific preferences within the Swiss energy system. By focusing on these areas, our research provides valuable insights into optimizing energy system designs for sustainability, efficiency, and equity, offering guidance to policymakers, system operators, and market participants. These findings support informed decision-making as Switzerland progresses towards a decentralized, renewable energy future.
3 Methodology
This study adopts a multi-actor optimization framework, building on the research of Granacher et al. (2024), to examine the role of prosumers within Switzerland’s transitioning energy system. This model defines the value of flows exchanged between the actors of a system as a function of the investment made by the different actors. It is based on a two-stage method. First, a set of energy system configurations is generated by solving the primal problem as a system-level multi-objective optimization problem from the perspective of a universal decision-maker without considering the interests of individual actors within the system. Solving this problem defines a set of system configurations with the corresponding technologies, investments, and flows exchanged inside the system. The primal problem is solved using an
The optimization problem is formulated to minimize the costs for one actor. At the same time, a parameterized
This study focuses on the methodology to allocate prices to pre-calculated energy system configurations with flow exchanges. The sensitivity to other parameters is addressed within the primal problem, where alternative configurations are generated by varying key parameters such as energy demand, technology costs, and resource availability. This approach allows the dual problem to assess the impact of these variations on different actors, identifying the evolution of the exchanged flow values. Therefore, the sensitivity analysis to these parameters enhances the robustness of the methodology by capturing the potential shifts in optimal configurations and their implications for various system actors.
3.1 Primal problem: Swiss energy system
The energy system modeling framework EnergyScope (Schnidrig et al., 2024) has been selected as the primal problem as 1) it integrates the centralized and regionally distributed decentralized actors of the energy system and 2) due to its fast generation of a collection of system configurations. The case study assumes a carbon-neutral (Li et al., 2020) and energy-independent Swiss energy system (Schnidrig et al., 2023) in 2050.
EnergyScope is based on an optimization-based formulation, minimizing the total cost
We study the PV penetration by parametrizing the factor
3.2 Dual problem: the Swiss energy system actors
In our study, we consider that the Swiss energy system is realized and operated by three groups of types of actors
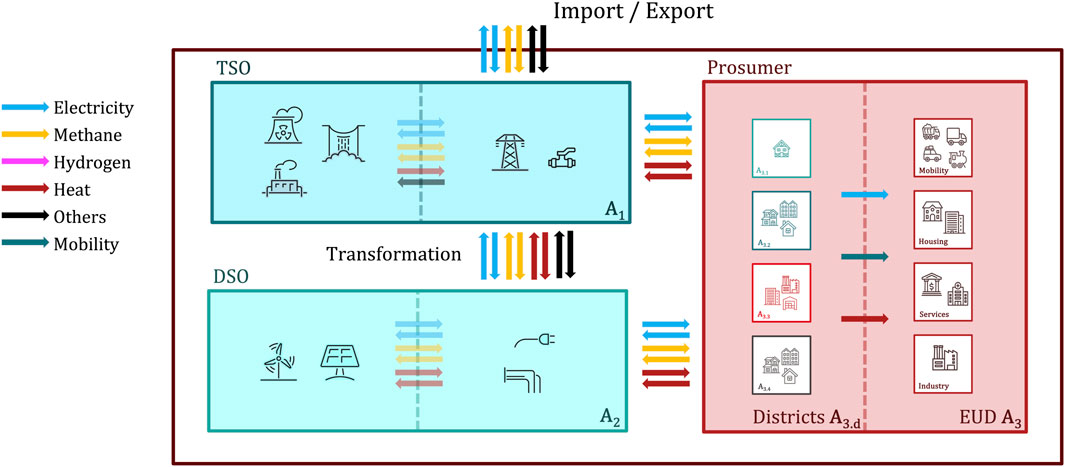
Figure 1. Illustration of the main actors in the Swiss national energy system and their interactions across different infrastructure levels. The technology-actor attribution figure is available in the Appendix Supplementary Figure S2.
3.2.1 Transmission system operators and large producers (TSO)
TSO are responsible for integrating renewable energy sources into the high-voltage grid while maintaining stability and ensuring the security of supply. Within this case study, we consider TSO as energy transporters and power operators at a size
3.2.2 Distribution system operators and small producers (DSO)
Swiss DSO are responsible for the medium and low voltage distribution systems. They face the challenge of adapting to the decentralized nature of renewable energy integration. Their role now encompasses integrating diverse renewable sources, such as solar and wind, into local grids and new energy efficiency and usages like building renovation, heat pump integration, and electric vehicles. They are also responsible for energy conversion and storage technologies at the Megawatt scale. This shift necessitates substantial investments in grid modernization, including developing innovative grid technologies for more efficient management of energy flows. DSO are also exploring innovative energy storage solutions to balance renewables production and demands. Additionally, DSO in Switzerland is facing challenges such as technical complexities in integrating renewables, evolving regulatory landscapes, and financial demands for upgrading infrastructure.
3.2.3 Prosumers and consumers
A distinct and growing group in the energy transition is the prosumers. As illustrated in Figure 1 in the red box, the prosumers
The additional sub-group EUD
3.3 Model adaptations for prosumer integration
Analyzing the role of prosumers within the energy system necessitates adapting the existing framework developed by Granacher et al. (2024) (Equations 2a–f), further referred to as the internal pricing approach. The internal pricing approach considers a set of pre-generated energy system configurations
The binary decision variable
Integrating prosumers’ demands expands the model to include prosumer-specific dynamics, such as decentralized energy production leading to self-consumption, storage capabilities, and the economic transactions involved with the actors. To capture the dynamics induced by prosumers, a set of actors
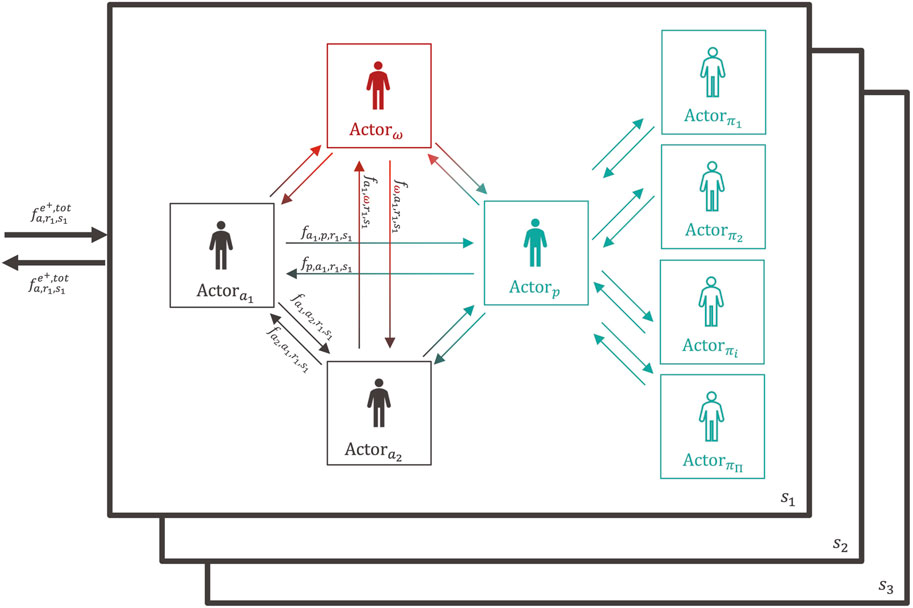
Figure 2. Illustration of the framework adaptation including the geographically dispersed prosumers
The enhancement in the framework adaptation is the consideration for the prosumers’ cost
The equations detailing internal exchange prices between actors (Equation 2c), such as
It should be noted that with this formulation, the result for the end-user actors defines the price of the energy vectors (e.g., retail and selling tariff of electricity) as well as the annual bill associated with the services (CHF/pkm or m2 heated), considered as tariffs subject to bounds related to resources (Equation 2d) and profitability limits to the actors (Equation 2e).
3.4 Costs space exploration - trade-offs
For a given configuration, the dual problem defines the profit repartition between the actors in the system by choosing a target actor who will maximise its profit under the constraints of the profitability of the others as defined by Equation 2e.
To explore the actors’ trade-offs, a quasi-Monte Carlo simulation as per Morokoff’s method (Morokoff and Caflisch, 1995) is used, to evaluate the variations in the solution space under the
Each sample
4 Results
The method is applied to investigate end-users role in the penetration of photovoltaic energy in the Swiss energy system. The EnergyScope decentralized model (Schnidrig et al., 2024) is used to parametrize the PV penetration. As system boundaries, we considered an independent and
4.1 Primal problem: analysis of calculated configurations
Figure 3A shows the evolution of the energy system configuration as a function of the PV penetration from the current deployment of 4 GW to the maximum potential of 165 GW. The evolution shows a shift between centralized and decentralized energy sources and reaches a minimum cost at 47.1 GW PV deployment, reaching 62% SC. The evolution also shows the interplay of wind and solar energy, with a decrease in wind power production correlated with the PV deployment. In a scenario with 30 GW of installed PV capacity (20% of the available PV potential) and 43% SC (Figure 3B), regional disparities in solar potential are leveraged, leading to annual energy production of around 30 TWh. However, this scenario also entails a 5% increase in system costs compared to the optimal configuration due to reliance on higher service cost resources like biomass and geothermal energy. The trade-off analysis for increasing PV penetration shows that decentralized PV installations, while reducing grid strain and promoting localized energy solutions, also introduce challenges related to overproduction, including curtailment with the need for grid reinforcement at high self-consumption levels. This necessitates innovative storage solutions and more flexible grid management to accommodate fluctuations inherent in a predominantly solar-based energy system. This underscores the need for strategic geographic planning and targeted investments in renewable energy to achieve Switzerland’s balanced, efficient, and sustainable energy system with a good understanding of the role of decentralized production and, therefore, of end-users. The annual costs for the TSO, DSO, and end-user actors reported in Figure 3A) show the critical role of the end-users for the PV deployment with an interplay between TSO and DSO actors with the increasing level of PV penetration.
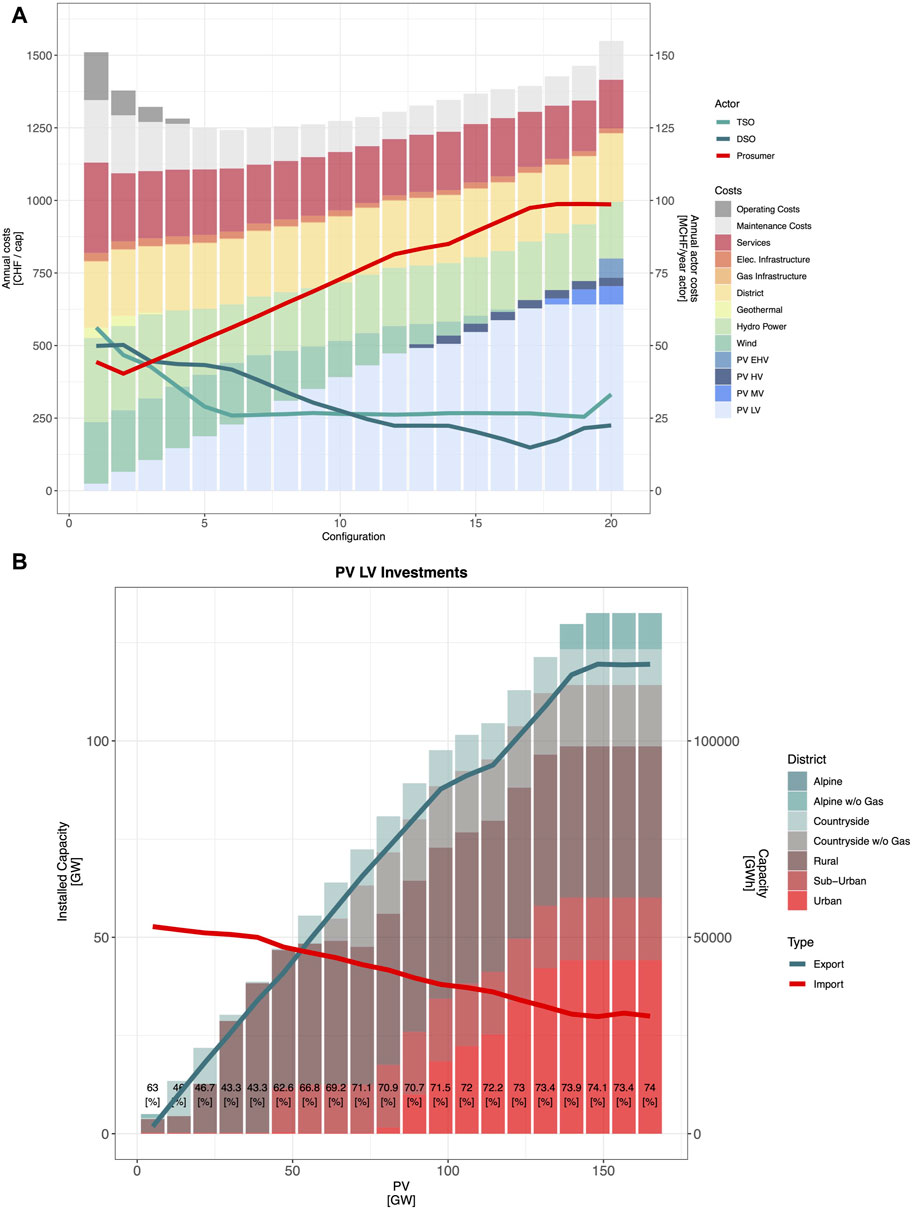
Figure 3. Primal energy system configurations based on the evolution of PV parametrization in the Swiss energy system 2050 with a population of 10 Million. The x-axis represents the numbered configurations (A) and the installed capacity of PV (B) ordered with increasing PV penetration from configuration 1 (5 GW) to configuration 20 (165 GW), as indicated at the bottom of the bars.
Switzerland’s regional variations in energy system configurations, driven by its diverse geographic and demographic landscape, are represented through the seven distinct district types (Terrier et al., 2024; Chuat et al., 2024; Schnidrig et al., 2024). Applying the primal’s problem in the PV parametrization allows us to identify the prioritization of PV deployment across the different district types. PV Rural areas are prioritized zones for PV installations due to their lower energy demand density than Urban districts. The latter have insufficient installed PV to fulfill their demand; therefore, Urban centers must import electricity. Alpine regions, with a PV potential exceeding demand by 43.1%, emerge as key areas for reducing annual grid energy demand significantly (Schnidrig et al., 2024). This classification underscores the importance of a tailored approach to PV deployment across different regions to enhance the national energy system’s efficiency.
4.1.1 Implications on system actors in the evolving Swiss energy landscape
The transition of the Swiss energy system towards increased PV integration and decentralized energy sources significantly reshape the roles and economic outcomes for the different system actors (see Supplementary Figure S1). This shift from traditional centralized production to more integrated, decentralized solutions opens new market opportunities and presents distinct challenges, altering the investment landscape considerably.
The increase in PV capacity correlates with increased financial commitments from end-users, who face optimal annual costs of 556 CHF/cap, accounting for 43% of the total costs. This proportion grows with further PV deployment, peaking at 63% in configuration 19 (165 GW).
With a low PV penetration, end-users face minimal investment needs, around 450 CHF/year cap (Figure 3A); however, as PV penetration intensifies, their investment steadily increases, reaching up to 540 CHF/year cap for a very high level of PV penetration. Concurrently, DSO and TSO experience a notable shift in their investment strategies. These actors invest in wind energy and biomass at low PV penetration. However, with a higher level of PV penetration, the investment pivots to integrating and managing decentralized end-user PV systems. The investment peak at 500 CHF/cap/year for DSO and 540 CHF/cap/year for TSO) for low PV penetration gradually decreasing to reach a plateau at 148.2 GW of PV deployed for the TSO and a minimum of 1480 CHF/cap/year at a very high level of penetration for the DSO actors. as depicted in Figure 3A) and S1 in the Supplementary Material.
4.2 Dual problem: price allocation
The configurations generated by the primal problem show that Switzerland’s net-zero target can be reached in very different ways, with relative importance given to different actors. The price allocation model (dual problem) will quantify how the different actors value the associated investment and the corresponding exchanges.
4.2.1 Extreme scenarios: single-actor optimization
First, we apply the dual problem by systematically selecting each system actor as the objective actor, i.e., maximizing its profit (or minimizing its cost) and letting the objective actor select its preferred configuration in the list of configurations generated by solving the primal problem. To solve this problem, we specified profitability limits for the different actors, with the present 2020 service costs for the end users and no profit for the other actors. Results that indeed correspond to an extreme situation where one single actor takes the maximum profit are presented in Table 2, each column refers to the selected actor and reports the preferred system configuration
From this first analysis, we can conclude that none of the actors select extreme configurations, demonstrating a preference for economically balanced approaches. The DSO and Distribution TSO favor configuration 6 (47.1 GW PV) and configuration 5 (38.7 GW PV), respectively, indicative of their strategic alignment with Urban and Sub-Urban settings with the annual system costs ranging from 1280 CHF/cap to 1340 CHF/cap, where configuration 6 sees the emerge of Countryside PV installation. The solution preferred by DSO corresponds to an end-user annual investment of 556 CHF/year/cap and a DSO and TSO contribution of 413 and 256 CHF/year/cap, respectively. Solution preferred by TSO features more PV (55.5 GW) with higher TSO investment (259 CHF/year/cap) and decreased DSO share (376 CHF/year/cap), while a solution that maximizes the profit of DSO corresponds to a PV deployment of 47.1 GW. In contrast, actors from isolated regions such as Alpine areas opt for lower PV penetration levels (13.5 GW), driven by a prioritization of local rooftop installations which escalate the total cost to 1460 CHF/cap with 339 CHF/year/cap investment allocated to the other end-users, 463 to the TSO and 439 to the DSO. The most remote areas without gas infrastructure prioritize maximum PV deployment (157 GW), culminating in the highest observed annual system costs of 1550 CHF/cap.
This analysis underscores the financial shifts among the actors, emphasizing that while the objective actor incurs minimal costs, other system participants face significant cost increases. For instance, minimizing TSO costs leads to an annual minimal cost of −7.45 kCHF/cap, yet Urban, Sub-Urban, and Rural areas experience dramatically higher energy service prices, recorded at annually 3410
4.2.2 Analysis of the systemic burden-shifting between actors
Acknowledging that single-actor optimization leads to financial burden-shifting, the
The multi-actor approach is applied by considering the following actors: DSO, TSO, regional end-users, and the consumers (industrial and mobility sectors) within the bundling actor, taking each of the three actors as objective actors
Figure 4 reports for each group of targeted actors the frequency of appearance of the actors’ total cost expressed as the variation with respect to the 2020 reference. It illustrates the financial burden-shifting towards the actors. The profit of the TSO can double [-269%;−36%] and is mainly transferred to the end-user with limited effect [−11%; 15%] on the DSO. For the DSO, the profit is again transferred to the end-user [256%; 33%] with a limited impact on the TSO [−41%; 52%]. While the profit of one actor can be shifted up to 250%, the same amount is compensated by a cost increase by the end-user. Irrespective of the scenario, selecting the End-Users as the objective actor group
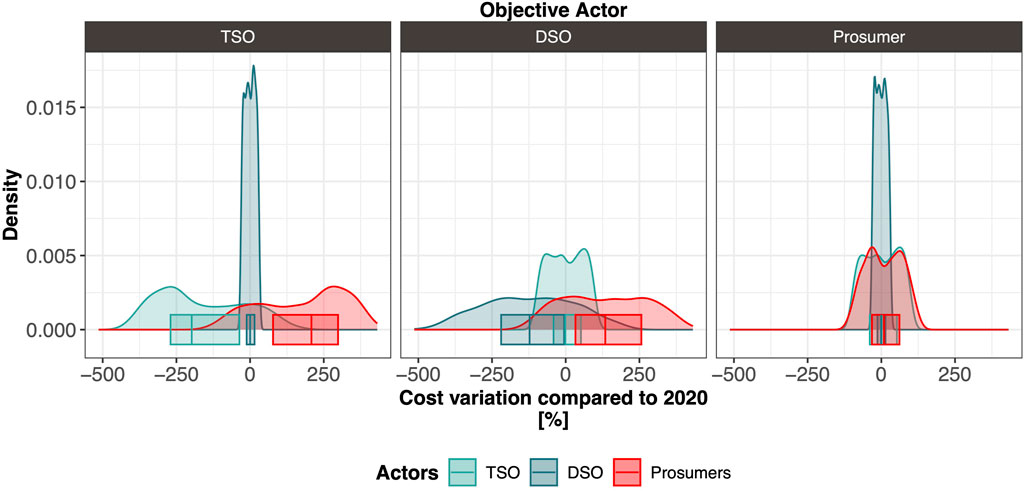
Figure 4. Relative actor’s annual cost distribution as a function of the objective actor 1)
4.2.3 Actor interactions
Figure 5 presents the annual energy bill for the same three groups. It identifies the selected configurations considering the Sobol sampling on the TSO and DSO bounds (0 −
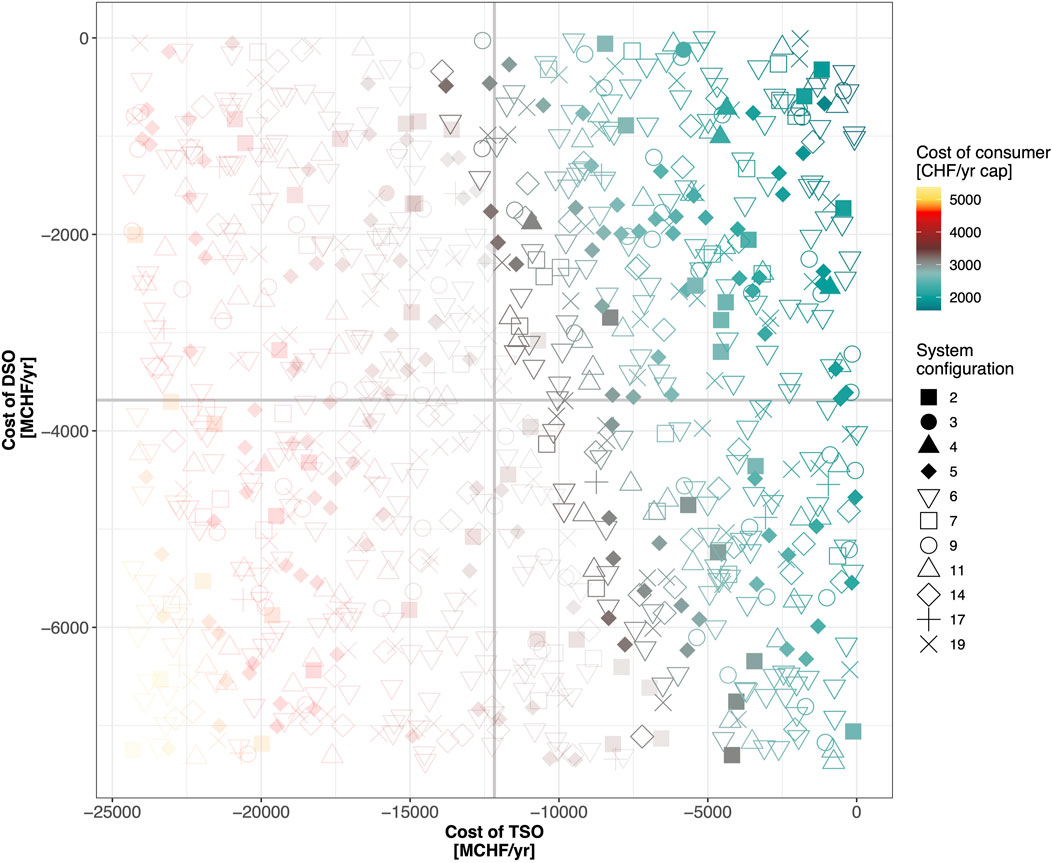
Figure 5. Actor’s cost of the selected configuration with the end-user as objective actor
The Sobol sampling demonstrates that there are more solutions where the TSO makes more profit than the reference case, corresponding to an annual profit of 12,400–12,800 CHF/cap. More solutions benefiting DSO and end-users can be found (42% of solutions in the right bottom quadrant of Figure 5) compared to benefiting TSO and end-users (1.8% of solutions in the left top quadrant of Figure 5). This discrepancy can be brought back to the flexibility of price dictation based on investments made. The higher the amount of energy transported through the actor, the more they can influence the total energy system. Despite TSO and DSO facing similar costs throughout the configurations (Figure 3A), the amount of transported energy is higher for the DSO, allowing them to have more flexibility to enhance their profit within the given price margin on the energy flow (Figures S1b and S1c). The trend is even more pronounced with the end-users investing heavily in new energy harvesting technologies (PV) with increased decentralization, thus having a more significant lever on the price design than the other actors.
The Sobol sampling identifies 11 out of the 20 configurations generated by the primal problem in the economically feasible domain for the prosumers. Four configurations appear in 73.2% of the feasible samples: 5 (38.7 GW PV) 14.2%, 6 (47.1 GW PV) 39.7%, 11 (89.2 GW PV) 8.4%, and 19 (156.6 GW PV) 11.4%1, configuration 6 (47.1 GW PV) corresponding as well to the minimum cost of the primal problem. It is interesting to note that DSO and TSO select configuration 6 (47.1 GW PV) most frequently within the feasible domain at their respective 10% minimum, while the end-users select configuration 11 (89.2 GW PV) 33% within their respective 10% minimum, followed by the economic optimum (22% within the minimum space).
Configuration 5 (38.7 GW PV) 14.2% deploys PV systems predominantly in rural areas. This configuration projects a PV capacity of 38.7 GW, generating 24.3 TWh annually with a self-consumption rate of 43.0%. The scenario achieves full potential utilization of wind energy (20 GW). The energy system imports 50 TWh centrally and exports 30 TWh from decentralized sources. Hydroelectric power contributions are maximized from both dams (8.9 GW) and river systems (4.4 GW).
Configuration 6 (47.1 GW PV) 39.7% extends PV deployments to both rural and suburban areas with a total capacity of 47.1 GW. This setup maintains an equilibrium between centralized imports and decentralized exports. The scenario supports a full wind potential (20 GW) and incorporates a methane storage capacity of 5 TWh, enhancing energy flexibility and storage.
Configuration 11 (89.2 GW PV) 8.4% focuses on PV system deployment across rural, suburban, and countryside areas with a limited 10% deployment in urban settings due to grid capacity constraints. This configuration supports a total PV capacity of 89.7 GW, with a curtailment rate of 17% due to excess generation. The scenario includes 7 TWh of methane storage and 8.2 TWh of hydro storage, with reduced wind capacity at 10 GW to align with grid capabilities.
Configuration 19 (156.6 GW PV) 11.4% maximizes PV installations on all eligible built surfaces except those requiring extra high voltage systems. This leads to an installed capacity of 133 GW, generating 281 TWh, with 23% of the production curtailed due to overproduction. This configuration excludes wind energy utilization and incorporates 7 TWh of hydro storage and 5 TWh of methane storage, focusing on enhancing storage capabilities to manage the extensive PV output effectively.
The actor’s burden-shifting in the identified configurations is illustrated in the Pearson distribution of Figure 6, which depicts the correlation between the end user actors and the DSO and TSO In these matrices, the lower section delineates the allocation of solutions among actors, the diagonal illustrates the distribution of costs per actor, and the upper section details the correlation metrics. The Pearson correlation coefficient (Silverman, 1986), symbolized by
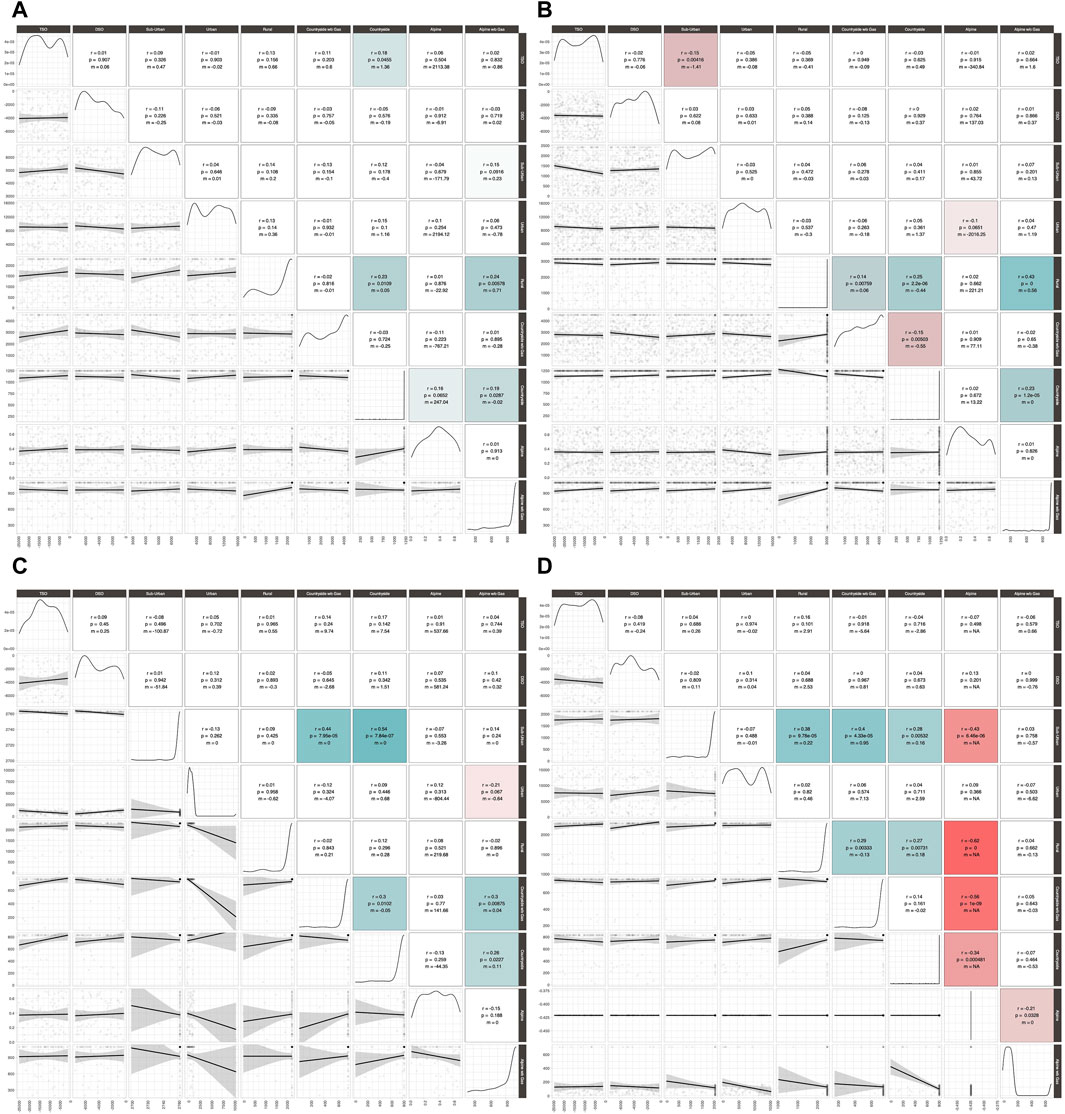
Figure 6. Pearson correlation coefficient matrix based on the ϵα constraint optimization for the four most frequent scenarios, respectively configurations 5, 6, 11 and 19. They represent 73.7 % of the solution space. The upper triangle depicts the correlation factor r with the color gradient and the significance p with the transparency. Red indicates a negative correlation, while green indicates a positive correlation. The transparency is set such as p ≤ 0.05 are not significant under the initial hypothesis of not correlating, thus leading to complete transparency over the latter value. Furthermore, the trend-line m gradient is expressed, indicating the annual price between the respective actors. The diagonal depicts the distribution of the appearance of the individual variables. The lower triangle represents the observation distribution with the corresponding trend line and 95% confidence interval. Each point corresponds to one distinct fiscal configuration.
Notably, specific correlations between actors are discernible in the upper section of the matrix; for example, a positive correlation exists between Rural and Sub-Urban actors in configuration 6, marked by a correlation coefficient
The diagonal further illustrates the distribution of costs among actors, showing the variability of a configuration’s sensitivity to specific scenarios. For instance, in configuration 6 (47.1 GW PV), the Countryside actor predominantly faces the highest costs, peaking annually at 30
While configuration 5 (38.7 GW PV) shows positive correlations between the districts Countryside, Rural, and Alpine w/o Gas
4.3 The role of the end-users
4.3.1 The distribution of energy prices
Figure 7 represents the distribution of the district’s energy exchange prices for the end-users and the DSO and the four identified configurations. The boxplots indicate the median and the 25% & 75% quantiles, indicating the probability of having a specific price at least in 75%, respectively at maximum 25% of the cases. Additionally, the figures are complemented with the cumulative classed prices, thus indicating the probability of reaching, at minimum, a specific price.
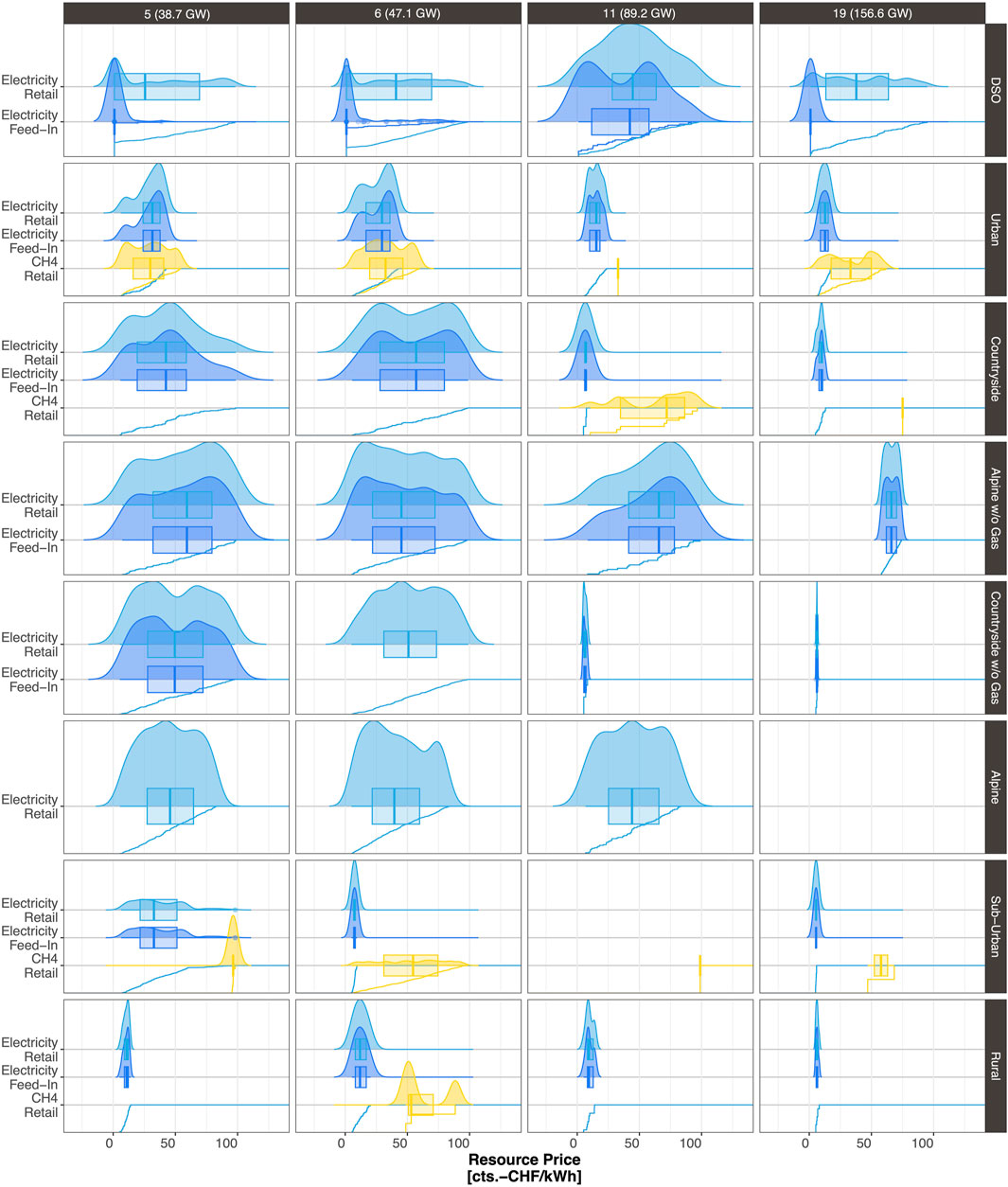
Figure 7. Energy price distribution, compared to 2020, in each district type for the selected system configurations. The cumulative probability distribution is located under the boxplot associated with the distribution.
The role of the actors within the energy system is made visible through the price design, as it is possible to identify actors importing only (Alpine for configurations 5 (38.7 GW PV), 6 (47.1 GW PV) and 11 (89.2 GW PV)), gas-depending districts as the Countryside districts within configuration 11. In our approach, the actors create their prices in the market without mutualization constraints. Consequently, the difference between feed-in and retail electricity prices is negligible for configurations of districts importing and exporting electricity, indicating price equality for feed-in and retail scenarios for the decentralized prosumers. Although one would expect a difference between retail and feed-in tariffs to reflect the energy management, this indeed is transferred to the feed-in and retail tariff for the DSO and the assumption in our model that the DSO will organize the market between the prosumers via the bundling actor
In configurations 5 and 6, it should be noted that there is a zero value for the feed-in price of the DSO, meaning that there are no exchanges from DSO to TSO but only TSO to DSO. This demonstrates that the DSO will organize the PV flows, while the TSO will provide the balance by the hydropower.
Additionally, Figure 7 further represents the classed price curves (bottom of each actor’s row), indicating the probability of encountering at least one specific price under various market designs of the energy system: Flat classed price curves are synonym to a wide price distribution and respectively a wide boxplot, while reversely steep curves indicate narrow distributions, as illustrated through the actor Countryside w/o Gas in configurations 5 ([27; 68] cts-CHF/kWh) and 6 ([31; 70] cts-CHF/kWh) for wide, and 11 ([5.2; 7.0] cts-CHF/kWh) and 19 ([5.8; 6.1] cts-CHF/kWh) for narrow distributions. Comparing the spread of price distributions with the PV installation in the respective districts (Figure 3B), one can observe that higher investments result in steeper curves, representative of a narrower price distribution, while lower PV shares exhibit flatter curves, indicative of a reduced dependency and a compensatory effect for the amortization of investments in other actors, distributed variably across districts as previously demonstrated.
The larger distributions indicate a lower lever on the market design, correlating with the installed PV amount (
Differences between feed-in and retail tariffs are visible for the DSO exchanging with the bundling actor
4.3.2 Regional variations in configurations
From the actor analysis, we can see that different actors are considering different investments and exchanges. The prosumer actors are indeed differentiating themselves by population density and climatic conditions. However, when viewed from a system-wide perspective, more optimal configurations emerge. Taking the market scenarios for the four selected configurations (5, 6, 11, 19) being closest to
Figure 8 show the geographic allocation of the end users by averaging the annual household bill per heated area in the regions of Switzerland. One can observe the energy price correlating with the population densities, as the highest energy prices can be observed throughout all scenarios in the densely populated areas around the major cities and their suburbs. A similar conclusion is observed in the valleys, where the population density is concentrated in the plain. In contrast, the remote areas in the side valleys (alpine) see low prices across all configurations.
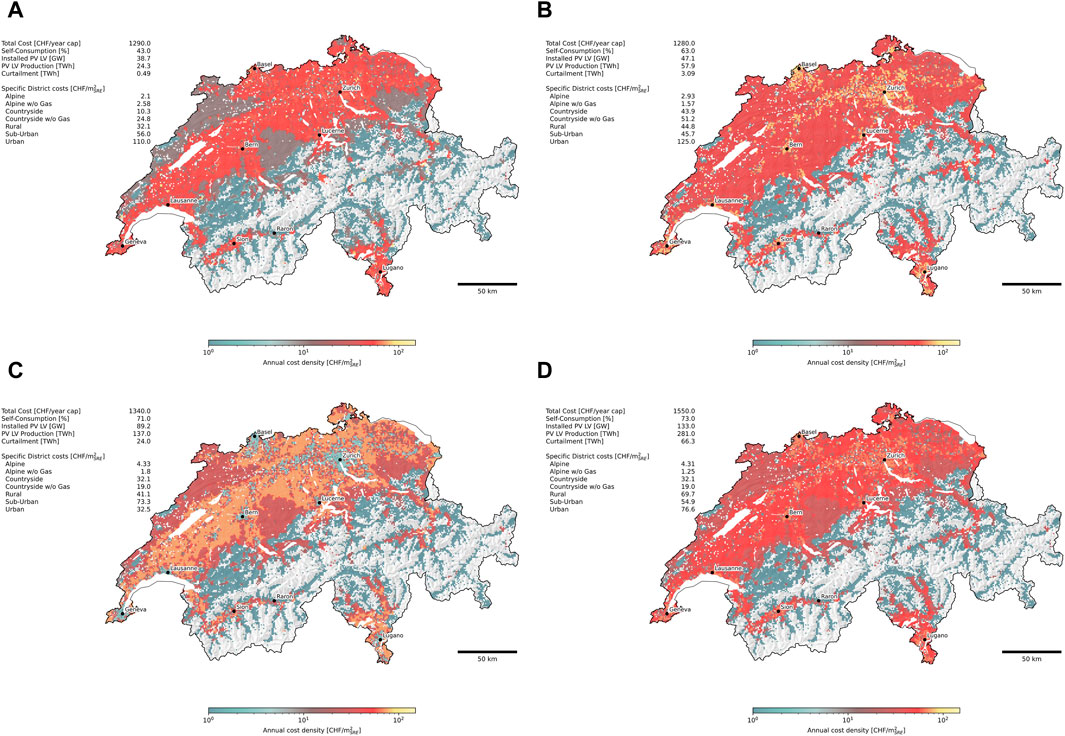
Figure 8. Representation of the geographic specific energy services price per energetic reference area evolution for the four most selected energy system configurations, respectively configurations 5, 6, 11 and 19.
Similarly to previously observed with the energy pricing (Figure 7), the investment in PV leads to favorable energy prices for the prosumers that have a high exportation rate at the detriment to the importers: A high PV/demand ratio is associated with increased energy prices, as visible in the maps 8 a)-c), where the different PV deployment configurations are translated in annual price evolutions: urban areas as Zürich, Lausanne, Basel, and Geneva have the most beneficial price design, once they deploy PV (Configuration 11 32.1 CHF/m2), compared to the configurations with low Urban-PV deployment (5 and 6 110–125 CHF/m2). Similarly, configuration 6 (Figure 8B)) is the most favorable one for the sub-urban districts (45.7 CHF/m2), as it is the first configuration seeing sub-urban-PV appear. As rural regions have PV fully deployed across all four scenarios, configuration 5 (Configuration 5, Figure 8A)) corresponds to the first configuration with Rural-PV deployed, and therefore reading to the economically most favorable design (32.1 CHF/m2) corresponds to the most favorable design.
Once the “optimal” configuration is reached, other districts start deploying their PV capacities, thus reducing their dependencies on electricity import. In contrast, the excess electricity is exported or curtailed (Figure 3B). Taking the rural regions as an example, with configuration 5 being optimal (and fully deploying the rural PV capacity), gradually increasing PV installation in other districts leads to price increases for the following configurations (44.8–69.7 CHF/m2). In configuration 19 (Figure 8D)), all districts reached their maximum PV deployment, and centralized PV is installed, thus increasing all end-users annual bills compared to their optimum.
Alpine districts display a unique trend, with electricity prices initially skewing high at low levels of PV production, detailed by an increase of 2.0 CHF/m2 year (Figure 8). This trend inverses as PV deployment increases, eventually disappearing at full PV installation stages, marking a transition to complete auto-sufficiency and minimal external energy exchanges. The transition point where prices begin to decrease can be quantified by 47.4 GW, illustrating the district’s journey towards energy independence.
5 Discussion
5.1 Burden shifting compensated by end-users
In the evolving landscape of Switzerland’s energy system, end-users increasingly shoulder significant financial responsibilities. The data reveals that end-users contribute between 556 and 764 CHF/cap/year towards PV installations. This range not only highlights the economic burden borne by consumers but also signals a transformative shift from traditional centralized energy provision, where TSOs and DSOs previously managed most infrastructural investments, to a model where individual and community investments become pivotal. For instance, investments by TSOs and DSOs are comparatively lower, ranging from 256 to 261 CHF/cap/year and 244 to 413 CHF/cap/year, respectively, underscoring a substantial shift towards decentralized financial responsibilities and empowerment of end-users in the energy market. This shift has broader implications for energy policy, suggesting the need for new regulatory frameworks that support and incentivize such decentralized investments. The data also indicate potential socio-economic impacts, as the financial burden on end-users may influence energy equity and access, highlighting the importance of policy measures to mitigate any negative effects on vulnerable populations.
5.2 Total cost minimization and configuration preferences
The optimal configurations derived from the primal problem analysis suggest a convergence in the cost minimization goals of TSOs, DSOs, and the overall system configuration. However, end-users exhibit a distinct set of preferences, favoring configurations that permit extensive PV installations, even when constrained by the capacity of urban distribution grids. This preference divergence indicates a potential conflict in objectives; while TSOs and DSOs focus on configurations that minimize system-wide costs, end-users prioritize energy autonomy and sustainability, even at potentially higher costs. This contrast is illustrated by the urban grid capacity constraints, which limit PV installation but are countered by end-user preferences for increased local generation capacity. The broader implications for theory and practice include the need for multi-objective optimization approaches that balance these competing interests and the development of technologies and policies that enhance grid flexibility and capacity to accommodate higher levels of PV penetration.
5.3 Regional disparities and pricing dynamics
The analysis reveals pronounced regional disparities in energy pricing influenced by urban forms and infrastructural capacities. Urban areas, constrained by space for PV installations, exhibit higher energy prices due to greater demand and limited supply capacity, contrasted with rural areas where extensive PV potentials lead to lower prices. For example, urban regions might face prices that reflect the higher cost of integrating limited renewable resources into a densely built environment. At the same time, rural areas can leverage their expansive areas for more significant installations, thus benefiting from economies of scale and reduced prices. This disparity underlines the critical impact of geographical and infrastructural factors on the economic and strategic planning within the decentralized energy systems of Switzerland. The broader implications for practice involve designing targeted policies and incentives that address these regional disparities, ensuring equitable access to renewable energy benefits across different geographic and socio-economic contexts. Additionally, these findings contribute to the theoretical understanding of regional energy system dynamics, highlighting the importance of incorporating spatial considerations into energy system models.
5.4 Emergence of new business models with prosumers
The rise of prosumers marks a significant evolution in the business models within the Swiss energy sector. A new financial dynamic is evident with investments ranging from 556 to 764 CHF/cap/year by prosumers compared to 256–261 CHF/cap/year by TSOs and 244–413 CHF/cap/year by DSOs. These investments not only support the infrastructure necessary for decentralized energy production but also foster a shift towards local energy autonomy. The significant capital influx from prosumers supports the development of technologies and systems that facilitate consumer-driven energy production, management, and consumption, reinforcing the transition from centralized to decentralized energy systems. This model challenges traditional utility operations and suggests a shift towards a more integrated and participatory approach to energy management, highlighting the proactive role of consumers in shaping the future energy landscape. The broader implications for theory include advancing the understanding of decentralized energy economics and the role of prosumers in energy system transformations. These findings indicate the need for utilities to adapt their business models and for policymakers to create supportive environments that encourage prosumer participation and investment.
These extended discussions integrate detailed financial data to provide a clearer, evidence-based view of the dynamics at play in Switzerland’s transition towards a more decentralized, consumer-empowered energy system. By focusing on specific numeric outcomes and trends, the analysis offers a comprehensive understanding of the economic shifts, regional disparities, and strategic preferences that define the current and future state of energy planning in Switzerland.
6 Limitations
While providing valuable insights into optimizing the Swiss energy system for a sustainable transition, the study encounters several limitations that merit consideration for future research and practical applications. These limitations are primarily related to the modeling approach, the assumptions made, and the scope of the analysis.
6.1 Modeling constraints and simplifications
The MILP model, although sophisticated, necessarily incorporates simplifications and assumptions to manage computational complexity. These may include linearizing non-linear processes, fixed efficiency rates, and omitting specific dynamic interactions within the energy system. Such simplifications can affect the granularity and accuracy of the model’s predictions, particularly in representing real-world operational challenges and the temporal variability of renewable energy sources.
6.2 Economic and policy assumptions
The economic analyses hinge on several assumptions regarding future costs, technological advancements, and policy developments. While grounded in current trends and expert forecasts, these assumptions are inherently uncertain and subject to change. Consequently, different energy system configurations’ economic viability and attractiveness may evolve as new information emerges and market conditions shift.
6.3 Stakeholder engagement and behavioral factors
The model primarily focuses on technical and economic parameters, with less emphasis on the behavioral aspects of energy system actors (e.g., consumers, prosumers, DSO, TSO). The adoption rates of renewable technologies, public acceptance, and the evolving role of prosumers are influenced by factors beyond economic rationality, such as cultural norms, social values, and policy incentives. These aspects are critical for successfully implementing energy transitions but are challenging to incorporate quantitatively in optimization models.
6.4 Point- and self-consumption
The primal problem (Schnidrig et al., 2024) used as a case study does not take into account the self-consumption of the MV, HV and EHV sectors, as it applies a copper-plate assumption for latter sectors, using an average grid model (Schnidrig et al., 2023). Therefore, no inter-region exchanges have been considered due to the geographic distribution of resources and demands.
Addressing these limitations requires a multifaceted approach involving refining modeling techniques, integrating higher-resolution data, and incorporating interdisciplinary perspectives. Engaging with a broader range of stakeholders, expanding the model to include intersectoral dynamics, and updating the analysis to reflect new technological and economic realities will be essential for advancing our understanding of energy system optimization in sustainable transitions.
7 Conclusion
Achieving the optimal Swiss energy system in the context of the energy transition necessitates the allocation of the financial means of the different actors of the energy system (TSOs, DSOs, end-users). This is especially true when one has to consider the integration of prosumers who are, at the same time, end-users and new major investors in renewable energy harvesting. We have proposed a method that enables the cost distribution between the actors, considering the possibility of cost shifting between them. The consideration of the prosumer actors highlights the importance of self-consumption and the geographical diversity in Switzerland’s landscape. Applying the national energy system model EnergyScope and an internal pricing approach have assessed the interdependencies and trade-offs among energy system actors, emphasizing the pivotal role of economic considerations in determining optimal configurations. These configurations aim to enhance system efficiency and align with the broader objectives of achieving a carbon-neutral and energy-independent Switzerland. The results indicate that decentralized PV deployment significantly reduces costs and increases energy self-sufficiency, highlighting the importance of strategic investments in decentralized infrastructure. The optimal configurations identified in the study provide actionable insights for policymakers and practitioners, emphasizing the need for targeted financial and regulatory support to facilitate the transition toward a sustainable energy system.
The findings highlight the significance of decentralization in the energy transition, where the strategic deployment of PV systems across different regions plays a critical role in reshaping the energy landscape. Urban areas, facing delays in reaching the economic optimum for PV installations due to high energy demand densities, underscore the challenge of energy self-sufficiency and the importance of strategic indigenous electricity imports. Conversely, rural and alpine districts demonstrate potential for leading the charge towards energy independence, leveraging their unique geographic and energy demand characteristics. Future research should focus on refining the economic and technical models to optimize decentralized energy systems further, considering different regions’ specific needs and potentials. Developing innovative grid management solutions and storage technologies will also be crucial in managing the variability and overproduction associated with increased PV penetration.
Moreover, the study has identified significant variations in resource prices across different energy system configurations, reflecting the impact of decentralized energy sources on the economic dynamics within the Swiss energy system. These variations emphasize the need for legislative frameworks and policy interventions tailored to regional disparities, ensuring a balanced and equitable distribution of costs among actors. The strategic implications for policy and infrastructure development are profound, necessitating targeted efforts to support grid enhancements, decentralized PV deployment, and innovative market solutions to foster a sustainable energy transition. Practical applications of these findings include the development of investment strategies that prioritize regions with the highest potential for PV deployment and creating policy incentives that encourage end-users to invest in renewable energy technologies. These efforts will support Switzerland’s transition to a carbon-neutral and energy-independent future and serve as a model for other regions aiming to achieve similar goals.
This research contributes to a deeper understanding of the Swiss energy transition, offering valuable insights into optimizing the integration and functionality of various energy system components. It underscores the importance of a comprehensive approach considering energy system optimization’s economic, technical, and geographical dimensions. As Switzerland continues its journey toward a sustainable and resilient energy future, the findings from this study provide a foundation for informed policy-making, strategic planning, and the development of forward-thinking solutions that align with the nation’s energy, environmental, and economic objectives.
This study demonstrates that decentralized PV deployment in Switzerland can significantly enhance the sustainability and resilience of the energy system. Key findings include the identification of optimal PV penetration levels that minimize costs and maximize self-consumption, the critical role of end-users in driving PV adoption, and the necessity of innovative grid management solutions to handle overproduction. These insights have important implications for future research, focusing on further refining economic and technical models to optimize decentralized energy systems. Additionally, practical applications of these findings include the development of targeted investment strategies and policy frameworks that support the transition to decentralized energy systems, both in Switzerland and other regions with similar energy profiles.
The findings underscore the necessity for strategic policy interventions to balance interests among actors and promote investments by prosumers instead of traditional electricity production actors. The significant impact of geographic diversity and energy system configurations on market dynamics emphasizes the need for targeted infrastructure development. Specifically, enhancements in DSO grid infrastructure and decentralized PV should be prioritized to realize Switzerland’s transition towards a carbon-neutral and energy-independent future.
These results have substantial implications for existing energy policies and practices. For policymakers, the shift towards decentralized energy systems necessitates revisions in regulatory frameworks to accommodate and incentivize decentralized energy production and consumption. The findings suggest a strategic shift towards investing in localized energy solutions and advanced grid management technologies for practitioners, especially those involved in energy system planning and management. Moreover, the methodologies and findings can be transferred to regions with analogous energy and geographic characteristics. This provides a template for enhancing energy system resilience and sustainability through increased PV penetration and decentralized energy solutions.
Data availability statement
The datasets presented in this study can be found in online repositories. The names of the repository/repositories and accession number(s) can be found in the article/Supplementary Material.
Author contributions
JS: Conceptualization, Data curation, Formal Analysis, Investigation, Software, Visualization, Writing–original draft, Writing–review and editing. AC: Conceptualization, Data curation, Formal Analysis, Methodology, Software, Validation, Writing–original draft, Writing–review and editing. JG: Conceptualization, Methodology, Validation, Writing–review and editing, Writing–original draft. CT: Formal Analysis, Validation, Writing–review and editing. FM: Supervision, Validation, Writing–review and editing, Writing–original draft. MM: Funding acquisition, Resources, Supervision, Validation, Writing–review and editing, Methodology, Project administration.
Funding
This research has received funding from the Flagship Initiative under the project “Flagship PFFS-21-03” Blue City and from the Swiss Federal Office of Energy SFOE as part of the SWEET consortium SWICE and CoSI. The authors bear sole responsibility for the conclusion and the results of the presented publication.
Conflict of interest
The authors declare that the research was conducted in the absence of any commercial or financial relationships that could be construed as a potential conflict of interest.
The author(s) declared that they were an editorial board member of Frontiers, at the time of submission. This had no impact on the peer review process and the final decision.
Publisher’s note
All claims expressed in this article are solely those of the authors and do not necessarily represent those of their affiliated organizations, or those of the publisher, the editors and the reviewers. Any product that may be evaluated in this article, or claim that may be made by its manufacturer, is not guaranteed or endorsed by the publisher.
Supplementary material
The Supplementary Material for this article can be found online at: https://www.frontiersin.org/articles/10.3389/fenrg.2024.1433921/full#supplementary-material
Abbreviations
DSO, Distribution system operator; EHV, Extra High Voltage; EUD, End Uses Demands; HV, High Voltage; MILP, Mixed Integer Linear Programming; PV, Photovoltaics; SC, Self-Consumption; TSO, Transmission System Operator.
Footnotes
1Despite reducing the solution space, no configuration distribution preference is visible (Supplementary Figure S3).
References
Abrell, J., Eser, P., Garrison, J. B., Savelsberg, J., and Weigt, H. (2019). Integrating economic and engineering models for future electricity market evaluation: a Swiss case study. Energy Strategy Rev. 25, 86–106. doi:10.1016/j.esr.2019.04.003
Alcamo, J., Shaw, R., and Hordijk, L. (1990). The RAINS model of acidification: science and strategies in europe. Netherlands: Springer.
Antenucci, A., Crespo del Granado, P., Gjorgiev, B., and Sansavini, G. (2019). Can models for long-term decarbonization policies guarantee security of power supply? A perspective from gas and power sector coupling. Energy Strategy Rev. 26, 100410. doi:10.1016/j.esr.2019.100410
Bachner, G., Mayer, J., and Steininger, K. W. (2019). Costs or benefits? Assessing the economy-wide effects of the electricity sector’s low carbon transition – the role of capital costs, divergent risk perceptions and premiums. Energy Strategy Rev. 26, 100373. doi:10.1016/j.esr.2019.100373
BF-IIASA (2023). Global biosphere management model (GLOBIOM) documentation 2023. Documentation version 1.0. Laxenburg, Austria: IIASA.
Chuat, A., Terrier, C., Schnidrig, J., and Maréchal, F. (2024). Identification of typical district configurations: a two-step global sensitivity analysis framework. Energy 296, 131116. doi:10.1016/j.energy.2024.131116
Clack, C. T. M., Qvist, S. A., Apt, J., Bazilian, M., Brandt, A. R., Caldeira, K., et al. (2017). Evaluation of a proposal for reliable low-cost grid power with 100% wind, water, and solar. Proc. Natl. Acad. Sci. 114, 6722–6727. doi:10.1073/pnas.1610381114
Day, C., Hobbs, B., and Pang, J.-S. (2002). Oligopolistic competition in power networks: a conjectured supply function approach. IEEE Trans. Power Syst. 17, 597–607. doi:10.1109/tpwrs.2002.800900
de Nooij, M., Koopmans, C., and Bijvoet, C. (2007). The value of supply security: the costs of power interruptions: economic input for damage reduction and investment in networks. Energy Econ. 29, 277–295. doi:10.1016/j.eneco.2006.05.022
Dias, L. P., Simões, S., Gouveia, J. P., and Seixas, J. (2019). City energy modelling - optimising local low carbon transitions with household budget constraints. Energy Strategy Rev. 26, 100387. doi:10.1016/j.esr.2019.100387
Du, G., Zhang, Y., Liu, X., Jiao, R. J., Xia, Y., and Li, Y. (2019). A review of leader-follower joint optimization problems and mathematical models for product design and development. Int. J. Adv. Manuf. Technol. 103, 3405–3424. doi:10.1007/s00170-019-03612-6
Fishbone, L. G., and Abilock, H. (1981). Markal, a linear-programming model for energy systems analysis: technical description of the bnl version. Int. J. Energy Res. 5, 353–375. doi:10.1002/er.4440050406
Gabriel, S. A., Kydes, A. S., and Whitman, P. (2001). The national energy modeling system: a large-scale energy-economic equilibrium model. Operations Res. 49, 14–25. doi:10.1287/opre.49.1.14.11195
Gholizadeh, N., Vahid-Pakdel, M. J., and Mohammadi, B. (2019). Enhancement of demand supply’s security using power to gas technology in networked energy hubs. Int. J. Electr. Power & Energy Syst. 109, 83–94. doi:10.1016/j.ijepes.2019.01.047
González-Briones, A., De La Prieta, F., Mohamad, M. S., Omatu, S., and Corchado, J. M. (2018). Multi-Agent systems applications in energy optimization problems: a state-of-the-art review. Energies 11, 1928. doi:10.3390/en11081928
Granacher, J., Schnidrig, J., Chuat, A., Lepour, D., Terrier, C., Castro-Amoedo, R., et al. (2024). A multi-actor approach for integrated energy system design with internal pricing. Front. Energy Res.
Hilpert, S., Kaldemeyer, C., Krien, U., Günther, S., Wingenbach, C., and Plessmann, G. (2018). The Open Energy Modelling Framework (oemof) - a new approach to facilitate open science in energy system modelling. Energy Strategy Rev. 22, 16–25. doi:10.1016/j.esr.2018.07.001
Howells, M., Rogner, H., Strachan, N., Heaps, C., Huntington, H., Kypreos, S., et al. (2011). OSeMOSYS: the open source energy modeling system. Energy Policy 39, 5850–5870. doi:10.1016/j.enpol.2011.06.033
Hudson, E. A., and Jorgenson, D. W. (1974). U. S. Energy policy and economic growth, 1975-2000. Bell J. Econ. Manag. Sci. 5, 461–514. doi:10.2307/3003118
Jacobson, M. Z., Delucchi, M. A., Cameron, M. A., and Frew, B. A. (2015). Low-cost solution to the grid reliability problem with 100% penetration of intermittent wind, water, and solar for all purposes. Proc. Natl. Acad. Sci. 112, 15060–15065. doi:10.1073/pnas.1510028112
Jensen, I. G., Wiese, F., Bramstoft, R., and Münster, M. (2020). Potential role of renewable gas in the transition of electricity and district heating systems. Energy Strategy Rev. 27, 100446. doi:10.1016/j.esr.2019.100446
Kelman, A., Ma, Y., and Borrelli, F. (2013). Analysis of local optima in predictive control for energy efficient buildings. J. Build. Perform. Simul. 6, 236–255. doi:10.1080/19401493.2012.671959
Kipfer, M., and Duffner, R. (2024). Die Schweiz in zahlen - statistisches jahrbuch 2022-2023. No. 129 in die schweiz in zahlen. Neuchâtel: BFS.
Klemperer, P. D., and Meyer, M. A. (1989). Supply function equilibria in oligopoly under uncertainty. Econometrica 57, 1243–1277. doi:10.2307/1913707
Lapillonne, B. (1978). Medee 2: a model for long-term energy demand evaluation. Tech. report. Laxenburg, Austria: International Institue for Applied Systems Analysis.
Lee, S.-C., and Shih, L.-H. (2010). Renewable energy policy evaluation using real option model — the case of Taiwan. Energy Econ. 32, S67–S78. doi:10.1016/j.eneco.2010.04.010
Leuthold, F. U., Weigt, H., and von Hirschhausen, C. (2012). A large-scale spatial optimization model of the European electricity market. Netw. Spatial Econ. 12, 75–107. doi:10.1007/s11067-010-9148-1
Li, A., and Zheng, H. (2021). Energy security and sustainable design of urban systems based on ecological network analysis. Ecol. Indic. 129, 107903. doi:10.1016/j.ecolind.2021.107903
Li, X., Damartzis, T., Stadler, Z., Moret, S., Meier, B., Friedl, M., et al. (2020). Decarbonization in complex energy systems: a study on the feasibility of carbon neutrality for Switzerland in 2050. Front. Energy Res. 8, 549615. doi:10.3389/fenrg.2020.549615
Limpens, G. (2021). “Generating energy transition pathways: application to Belgium,”. Ph.D. thesis Louvain (Belgium): Université catholique de Louvain.
Limpens, G., Moret, S., Jeanmart, H., and Maréchal, F. (2019). EnergyScope TD: a novel open-source model for regional energy systems. Appl. Energy 255, 113729. doi:10.1016/j.apenergy.2019.113729
Luginbühl, W., Altwegg, L., Vontobel, F., Delbiaggio, K., Rauchenstein, J., Mazumder, S., et al. (2024). Tätigkeitsbericht der ElCom 2023 m2023.
Macal, C. M., Boyd, G. A., Cirillo, R. R., Conzelmann, G., North, M. J., Thimmapuram, P. R., et al. (2004). Modeling the restructured illinois electricity market as a complex adaptive system.
Manne, A., Mendelsohn, R., and Richels, R. (1995). MERGE: a model for evaluating regional and global effects of GHG reduction policies. Energy Policy 23, 17–34. doi:10.1016/0301-4215(95)90763-w
Manne, A. S., and Wene, C. O. (1992). MARKAL-MACRO: a linked model for energy-economy analysis. Tech. Rep. BNL–47161. Washington, DC: USDOE.
Moret, S., Codina Girones, V., Bierlaire, M., and Maréchal, F. (2017). Characterization of input uncertainties in strategic energy planning models. Appl. Energy 202, 597–617. doi:10.1016/j.apenergy.2017.05.106
Morokoff, W. J., and Caflisch, R. E. (1995). Quasi-Monte Carlo integration. J. Comput. Phys. 122, 218–230. doi:10.1006/jcph.1995.1209
Neelakanta, P., and Arsali, M. (1999). Integrated resource planning using segmentation method based dynamic programming. IEEE Trans. Power Syst. 14, 375–385. doi:10.1109/59.744558
Park, H., and Baldick, R. (2015). Stochastic generation capacity expansion planning reducing greenhouse gas emissions. IEEE Trans. Power Syst. 30, 1026–1034. doi:10.1109/tpwrs.2014.2386872
Pfenninger, S., Hawkes, A., and Keirstead, J. (2014). Energy systems modeling for twenty-first century energy challenges. Renew. Sustain. Energy Rev. 33, 74–86. doi:10.1016/j.rser.2014.02.003
Ramsey, F. P. (1927). A contribution to the theory of taxation. Econ. J. 37, 47–61. doi:10.2307/2222721
Roche, R., Blunier, B., Miraoui, A., Hilaire, V., and Koukam, A. (2010). “Multi-agent systems for grid energy management: a short review,” in IECON 2010 - 36th Annual Conference on IEEE Industrial Electronics Society, Glendale, AZ, USA, 07-10 November 2010 (IEEE), 3341–3346.
Sarfarazi, S., Deissenroth-Uhrig, M., and Bertsch, V. (2020). Aggregation of households in community energy systems: an analysis from actors’ and market perspectives. Energies 13, 5154. doi:10.3390/en13195154
Schär, S., and Geldermann, J. (2021). Adopting multiactor multicriteria analysis for the evaluation of energy scenarios. Sustainability 13, 2594. doi:10.3390/su13052594
Schlecht, I., and Weigt, H. (2014). Swissmod - a model of the Swiss electricity market. SSRN Electron. J. doi:10.5451/unibas-ep34944
Schmid, D., Korkmaz, P., Blesl, M., Fahl, U., and Friedrich, R. (2019). Analyzing transformation pathways to a sustainable European energy system—internalization of health damage costs caused by air pollution. Energy Strategy Rev. 26, 100417. doi:10.1016/j.esr.2019.100417
Schnidrig, J., Cherkaoui, R., Calisesi, Y., Margni, M., and Maréchal, F. (2023). On the role of energy infrastructure in the energy transition. Case study of an energy independent and CO2 neutral energy system for Switzerland. Front. Energy Res. 11. doi:10.3389/fenrg.2023.1164813
Schnidrig, J., Chuat, A., Terrier, C., Maréchal, F., and Margni, M. (2024). Power to the people: on the role of districts in decentralized energy systems. Energies 17, 1718. doi:10.3390/en17071718
Schrattenholzer, L. (1981). The energy supply model MESSAGE. Tech. report. Laxenburg, Austria: IIASA.
Schweppe, F., Caramanis, M., Tobors, R., and Bohn, R. (1988). “Spot pricing of electricity,” in Power electronics and power systems. 1 edn. (Springer US).
Shanbhag, U. V., Infanger, G., and Glynn, P. W. (2011). A complementarity framework for forward contracting under uncertainty. Operations Res. 59, 810–834. doi:10.1287/opre.1110.0947
Siala, K., de la Rúa, C., Lechón, Y., and Hamacher, T. (2019). Towards a sustainable European energy system: linking optimization models with multi-regional input-output analysis. Energy Strategy Rev. 26, 100391. doi:10.1016/j.esr.2019.100391
Siala, K., and Mahfouz, M. Y. (2019). Impact of the choice of regions on energy system models. Energy Strategy Rev. 25, 75–85. doi:10.1016/j.esr.2019.100362
Silverman, B. (1986). “Density estimation dir statistics and data analysis,” in Monographs on statistics and applied probability (Springer).
Sobol, I. M. (1969). On the distribution of points in a cube and the approximate evaluation of integrals. U.S.S.R. Comput. Math. Math. Phys. 7, 86–112. doi:10.1016/0041-5553(67)90144-9
Stidham, S. (1992). Pricing and capacity decisions for a service facility: stability and multiple local optima. Manag. Sci. 38, 1121–1139. doi:10.1287/mnsc.38.8.1121
Terrier, C., Loustau, J. R. H., Lepour, D., and Maréchal, F. (2024). From local energy communities towards national energy system: a grid-aware techno-economic analysis. Energies 17, 910. doi:10.3390/en17040910
van den Berg, N. J., Hof, A. F., Akenji, L., Edelenbosch, O. Y., van Sluisveld, M. A. E., Timmer, V. J., et al. (2019). Improved modelling of lifestyle changes in Integrated Assessment Models: cross-disciplinary insights from methodologies and theories. Energy Strategy Rev. 26, 100420. doi:10.1016/j.esr.2019.100420
Wang, N., Heijnen, P. W., and Imhof, P. J. (2020). A multi-actor perspective on multi-objective regional energy system planning. Energy Policy 143, 111578. doi:10.1016/j.enpol.2020.111578
Yu, M., and Hong, S. H. (2017). Incentive-based demand response considering hierarchical electricity market: a Stackelberg game approach. Appl. Energy 203, 267–279. doi:10.1016/j.apenergy.2017.06.010
Nomenclature
Keywords: decentralized energy systems, renewable energy integration, dynamic pricing models, prosumer behavior, energy system optimization
Citation: Schnidrig J, Chuat A, Granacher J, Terrier C, Maréchal F and Margni M (2024) Power shift: quantifying the role of actors in the multi-actor Swiss energy system decentralization. Front. Energy Res. 12:1433921. doi: 10.3389/fenrg.2024.1433921
Received: 16 May 2024; Accepted: 29 July 2024;
Published: 16 August 2024.
Edited by:
Fang-Rong Ren, Nanjing Forestry University, ChinaReviewed by:
Yinhai Fang, Nanjing Forestry University, ChinaJiachao Peng, Wuhan Institute of Technology, China
Copyright © 2024 Schnidrig, Chuat, Granacher, Terrier, Maréchal and Margni. This is an open-access article distributed under the terms of the Creative Commons Attribution License (CC BY). The use, distribution or reproduction in other forums is permitted, provided the original author(s) and the copyright owner(s) are credited and that the original publication in this journal is cited, in accordance with accepted academic practice. No use, distribution or reproduction is permitted which does not comply with these terms.
*Correspondence: Jonas Schnidrig, am9uYXMuc2NobmlkcmlnQGhldnMuY2g=