- 1Department of Rheumatology and Immunology, The First Affiliated Hospital of Anhui University of Traditional Chinese Medicine, Hefei, Anhui, China
- 2Center for Xin’an Medicine and Modernization of Traditional Chinese Medicine of Institute of Health and Medicine (IHM), The First Affiliated Hospital of Anhui University of Traditional Chinese Medicine, Hefei, Anhui, China
- 3Department of Orthopedics, The First Affiliated Hospital of Anhui University of Traditional Chinese Medicine, Hefei, Anhui, China
Objective: Autoimmune diseases have long been recognized for their intricate nature and elusive mechanisms, presenting significant challenges in both diagnosis and treatment. The advent of artificial intelligence technology has opened up new possibilities for understanding, diagnosing, predicting, and managing autoimmune disorders. This study aims to explore the current state and emerging trends in the field through bibliometric analysis, providing guidance for future research directions.
Methods: The study employed the Web of Science Core Collection database for data acquisition and performed bibliometric analysis using CiteSpace, HistCite Pro, and VOSviewer.
Results: Over the past two decades, 1,695 publications emerged in this research field, including 1,409 research articles and 286 reviews. This investigation unveils the global development landscape predominantly led by the United States and China. The research identifies key institutions, such as Brigham & Women’s Hospital, influential journals like the Annals of the Rheumatic Diseases, distinguished authors including Katherine P. Liao, and pivotal articles. It visually maps out the research clusters’ evolutionary path over time and explores their applications in patient identification, risk factors, prognosis assessment, diagnosis, classification of disease subtypes, monitoring and decision support, and drug discovery.
Conclusion: AI is increasingly recognized for its potential in the field of autoimmune diseases, yet it continues to face numerous challenges, including insufficient model validation and difficulties in data integration and computational power. Significant advancements have been demanded to enhance diagnostic precision, improve treatment methodologies, and establish robust frameworks for data protection, thereby facilitating more effective management of these complex conditions.
1 Introduction
Autoimmune diseases (AID) encompass a spectrum of conditions instigated by an anomalous immune reaction to internal antigens (1, 2). The intricacies and fundamental mechanisms of these disorders pose challenges in diagnosis and treatment. Although substantial headway has been achieved in comprehending the pathophysiology of AID in recent years, there remains a need for more refined diagnostic modalities and efficacious therapeutic approaches. The advent of artificial intelligence (AI) technology offers promising new avenues for advancing our understanding, diagnosis, and management of AID. AI algorithms have the capacity to scrutinize copious datasets from diverse origins such as electronic health records, laboratory indicators, medical imaging, and genomic information (3–5). Through discerning subtle patterns and associations, AI facilitates disease prognosis, early detection, and refinement of treatment modalities (5–8). Despite having demonstrated significant potential in the field of AID, AI has encountered numerous challenges related to models, data, and treatment. Future advancements are expected to be driven by data integration and algorithm optimization, aimed at enhancing diagnosis, treatment, and monitoring capabilities. The integration of AI in AID research has proliferated in tandem with advancements in AI technology in the last two decades. The objective of our investigation is to explore the evolution of this field over the past twenty years through a bibliometric lens.
Bibliometrics is a method that reveals the research patterns and trends in a specific field through quantitative and qualitative analysis of literature data (9, 10). While traditional review articles exist to summarize research progress, each review article focuses differently. Currently, there is still a lack of comprehensive, objective, and intuitive analysis of the evolution and trends of AI applications in AID. Bibliometric analysis based on quantitative analysis of literature can objectively and comprehensively describe the historical characteristics and development trends of this field (11).
In this study, based on bibliometric analysis tools, we provided the research contents as follows (Figure 1): (1) comprehensively summarized the global development status in that field; (2) identified the high-productivity institutions, interested journals, highly cited authors, and pivotal milestone articles in that field; (3) visualized the evolution trajectory of research clusters in that field in a timeline format; (4) explored the current state of AI applications in the AID; (5) detailed the challenges and prospects.
2 Methods
2.1 Data collection
The Web of Science Core Collection (WoSCC) is a highly regarded database in the field of bibliometrics (11, 12), having gained significant recognition among scholars and serving as the primary data source informing this study. The search term strategy in this study was formulated by integrating reviews, bibliometric studies related to artificial intelligence and autoimmune diseases, as Medical Subject Headings (MeSH) terms in PubMed (5, 13). Supplementary Table S1 provides a list of the search terms employed in this study.
The search was conducted on July 1, 2024, with the search terms limited to English language articles and reviews published between 2003 and the search date. After the retrieval and screening process, a total of 1,695 publications were identified, comprising 1,409 research articles and 286 reviews. The WoSCC was used to systematically collect data on publication countries/regions, institutions, journals, authors, articles, and keywords. Excel software (version 2021) was used to conduct summary and analysis.
2.2 Bibliometric analysis
CiteSpace is a Java application created by the research team led by Chen Chaomei at Drexel University (14). This software offers visualization techniques for publication data and has been widely acknowledged in the field of bibliometrics for providing objective analyses of academic frontiers (15, 16). In the CiteSpace analysis, co-occurrence networks were used to distinguish merged networks via color-coded nodes and edges. Burst detection, based on Kleinberg’s algorithm, was employed as an indicator of active topics (17). In this study, CiteSpace charted the literature network within the relevant field, offering analyses on research hotspots, frontier trends, and the evolution of knowledge structures.
VOSviewer, developed by Leiden University in the Netherlands, was utilized to extract and analyze elements within literature data such as authors, keywords, and institutions (18–20). It calculated the strength of their associations and presented them in a visual format. This process involved data extraction and preprocessing, association calculation, and visualization mapping to facilitate co-occurrence analysis, citation analysis, and clustering analysis. VOSviewer was utilized to create a node network, and relevant information was obtained through parameters such as link strength, cluster color, and node size analysis.
HistCite Pro 2.1 was employed to manage and analyze a large volume of literature data, excelling particularly in citation analysis (21). By utilizing HistCite, citation relationships between documents could be traced, elucidating the knowledge dissemination pathways and developmental trends within the research domain, thereby identifying highly significant literature in the field. In HistCite Pro 2.1, the “Limit” was set to 30, with the remaining settings kept at their default values. Subsequently, the “Make graph” option was selected to effectively and visually represent the interconnectedness within the research field, facilitating the efficient identification of key literature.
3 Results
3.1 General features of publications
Bibliometric analysis was able to quantitatively portray the developmental status within a specific academic research field. Our study revealed that over the past two decades, the total number of publications in this research field amounted to 1,695. Among these, 1,409 were research articles and 286 were reviews. These publications include the contributions of 10,915 authors from 7,070 institutions across 703 journals (Supplementary Table S2).
As depicted in Figure 2A, we observed a rising trend in the number of publications over the past two decades, with particularly accelerated growth in the last five years. The count surpassed 100 for the first time in 2020, reaching 139 publications. By 2023, it had reached 341 publications, and in the first half of 2024 alone, the count had already reached 222 publications. In terms of citations, there was a noticeable upward trend in the number of citations in 2017, surpassing 1,000 and reaching 1,152. It is noteworthy that by 2023, the number of citations had reached 5,777.
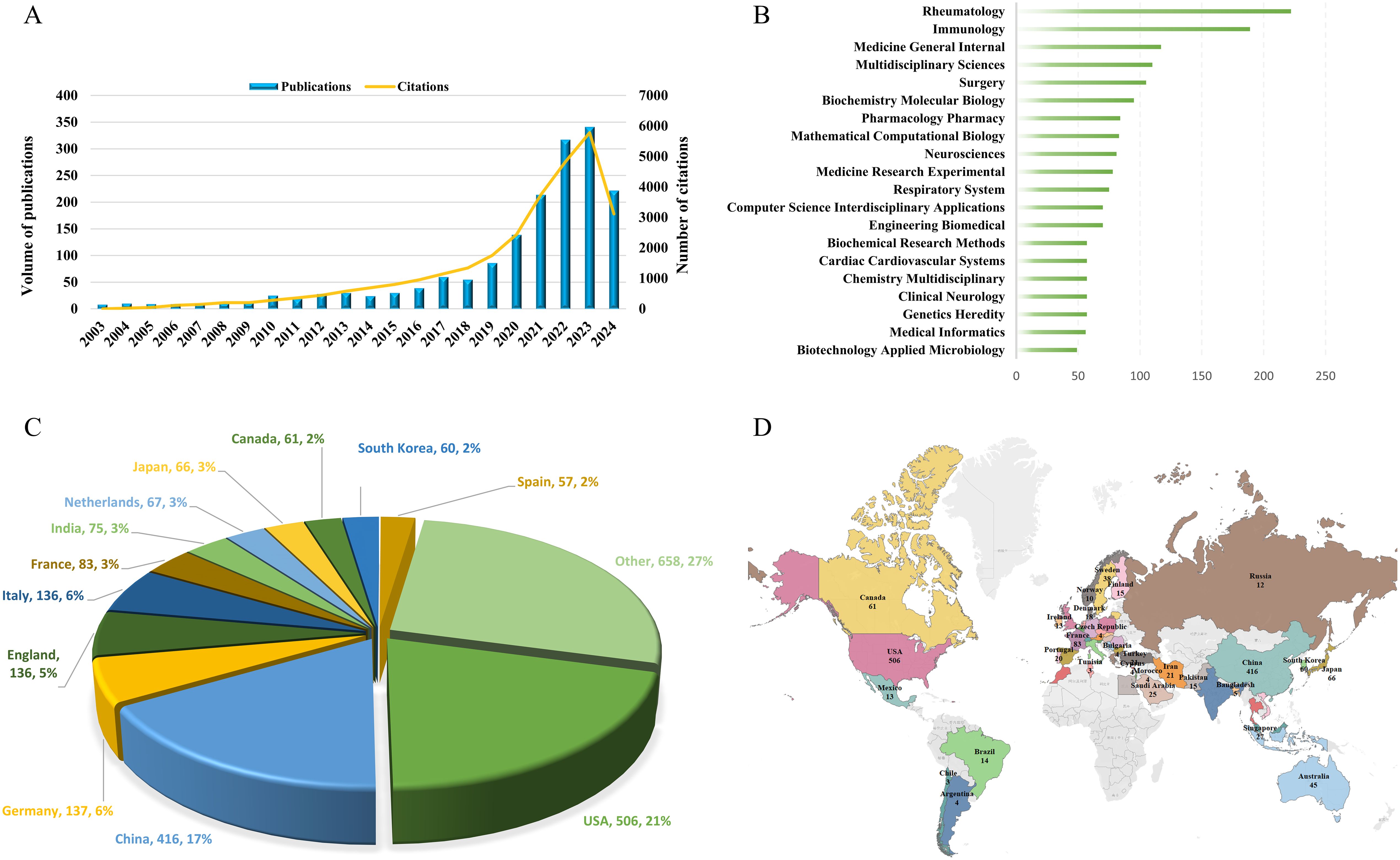
Figure 2. The evolution and distribution characteristics of publications. (A) The annual number of publications and citations over the past 20 years. (B) Top 20 Web of Science Categories for Publications. (C) Analysis of the publication numbers by country/region. (D) Global Distribution Overview of Publications.
3.2 Categories of publications
A thorough examination revealed that over the past two decades, publications on AI in AID spanned 124 Web of Science Categories. As illustrated in Figure 2B, the top 20 Categories with the highest publication counts are showcased. Among these, there are 5 Categories with over 100 publications, with Rheumatology leading at 222 publications, followed by Immunology with 189 publications. Subsequently, there were 117 publications in Medicine General Internal, 110 publications in Multidisciplinary Sciences, and 105 publications in Surgery.
3.3 Publication analysis of countries/regions
In the field of research, a total of 57 countries/regions contributed publications, with the USA having the highest number of publications at 506, accounting for 21% of the total, followed by China with 416 publications, making up 17%. Additionally, Germany had 137 publications, while England and Italy each had 136 publications. The pie chart in Figure 2C illustrates the distribution of publications by different countries/regions, revealing that the top 12 countries accounted for 73% of the total publications. The global distribution of publications by countries/regions, as depicted in Figure 2D, overall indicates that the United States and China made the most significant contributions to the field in terms of publication output. Unfortunately, it is noted that countries in Central Asia and regions in Africa have not yet ventured into this research field.
Through the analysis of co-authorship relationships among countries/regions using VOSviewer, it was found that the USA had the highest total link strength, reaching 437, indicating a higher frequency and wider scope of collaboration with other countries (Figure 3A). In terms of publication citations, a minimum citation threshold of no less than 5 times was set, and a country/region citation network map was generated (Figure 3B). In this network, node size represents citation counts, with the top five countries in citation counts being the USA, Germany, China, England, and Italy, with citation frequencies of 13,515, 3,425, 3,298, 3,277, and 2,436 respectively. Furthermore, through the analysis of total link strength, the USA, Italy, and China ranked in the top three with values of 1,849, 1,040, and 1,023 respectively, indicating the wider dissemination of publication citations from these three countries/regions.
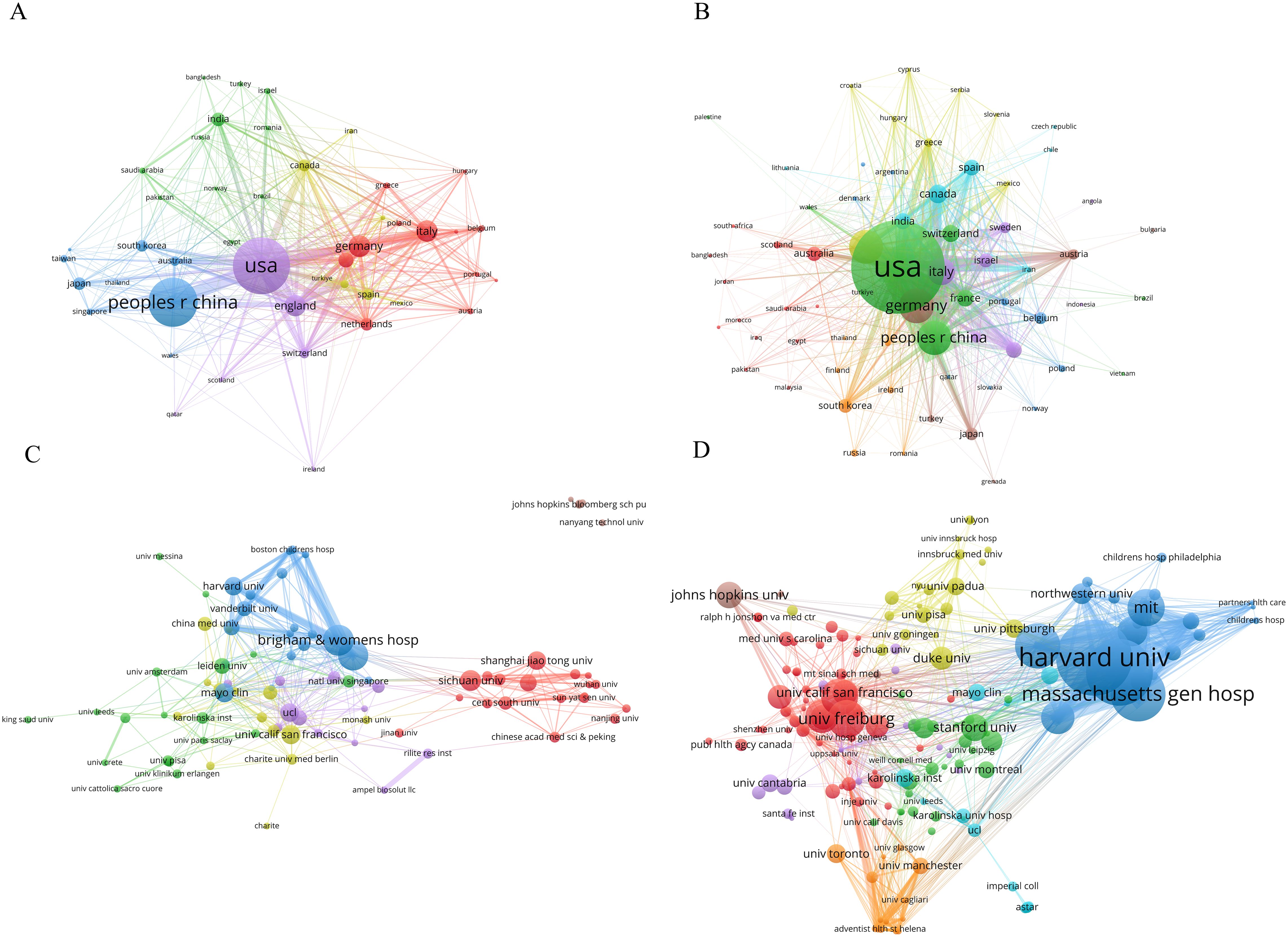
Figure 3. The co-authorship network among countries/regions and academic institutions. (A) Co-authorship Network of Countries/Regions. (B) Publication Citation Network of Countries/Regions. (C) Co-authorship Network of academic institutions. (D) Publication Citation Network of academic institutions. Network nodes symbolize publication or citation values, with larger nodes signifying higher values; Links between nodes reflect collaboration or citation strength, where thicker and darker lines denote stronger connections; Nodes of the same color are part of the same cluster, indicating similar characteristic.
3.4 Publication analysis of academic institutions
Various institutions leverage their expertise in clinical medicine, data algorithms, and biological research to collaboratively consolidate resources and knowledge. Setting the publication threshold at a minimum of 8 articles, a collaboration network comprising 88 academic institutions was established (Figure 3C). Node size represented publication output, with Brigham & Women’s Hospital, Harvard Medical School, Sichuan University, Mayo Clinic, and University of California San Francisco ranking as the top five institutions, with publication counts of 37, 36, 25, 23, and 23 respectively. Moreover, in this network, Brigham & Women’s Hospital, Harvard Medical School, and Massachusetts General Hospital ranked among the top three for total link strength, with values of 91, 67, and 60 consecutively.
By focusing on citations, with a minimum threshold of 100 citations, a total of 223 academic institutions were identified in the network graph (Figure 3D). The analysis revealed that Harvard University claimed the top position with 1,567 citations, followed by Brigham & Women’s Hospital with 1,372 citations and Massachusetts General Hospital with 1,129 citations. In the citation network graph, Brigham & Women’s Hospital was identified as the institution with the highest total link strength, with a value of 743. Massachusetts General Hospital and Harvard University recorded 638 and 597, respectively. These findings indicate that these three institutions occupy a dominant position within the field.
3.5 Publication analysis of authors
Interdisciplinary collaboration among authors overcomes professional barriers and drives progress in the field. Based on a minimum threshold of 3 publications, an analysis was performed to construct a co-authorship network graph for 323 authors (Figure 4A). The analysis revealed the existence of distinct co-author clusters, with notable prominence observed in clusters spearheaded by Gainer, Vivian S. and Karlson, Elizabeth W., Cai, Tianxi and Liao, Katherine P., as well as Kleyer, Arnd, Simon, David, and Schett, Georg. These findings suggest that the aforementioned individuals demonstrated higher levels of involvement and collaboration in research activities within their respective clusters. With regard to the presentation of citations, Figure 4B depicts the citation network graph, wherein the nodes represent the citation counts. Cai, Tianxi was the most highly cited author, with 955 citations, and also ranked first in total link strength within the network, with a value of 106. A co-citation network graph was constructed based on a minimum citation frequency threshold of 20 times, comprising a total of 219 authors (Figure 4C). In this co-citation network, the highest number of citations were attributed to Smolen, JS; Breiman, L.; and Aletaha, D., with respective citation counts of 177, 136, and 129.
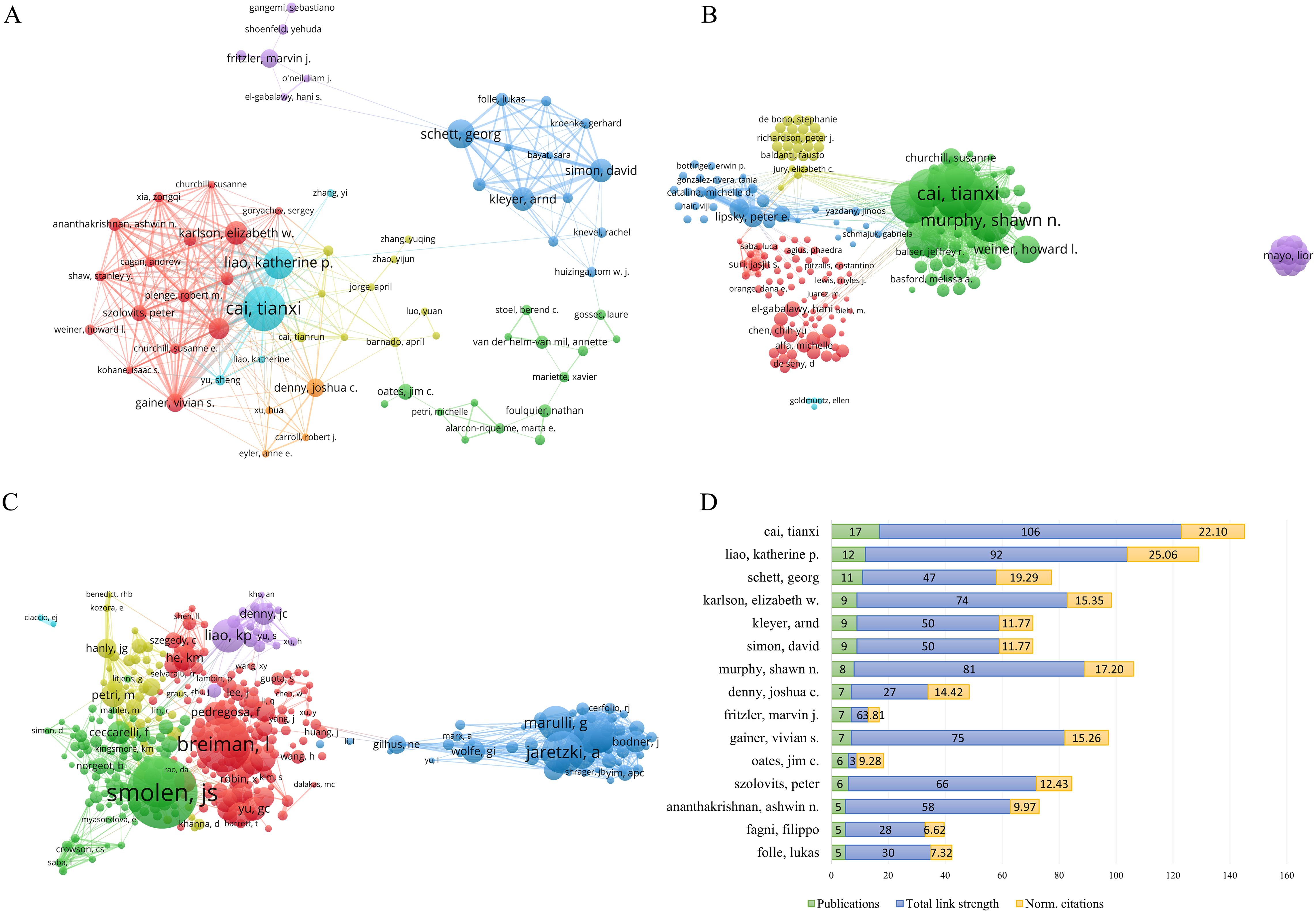
Figure 4. Author analysis of publications. (A) Network of co-authors of publications. (B) Citation network of authors in the field. (C) Co-citation network of authors. (D) Analysis of Total link strength and Normalized citations among the top 15 authors based on publication count.
We also analyzed the total link strength and norm. Citations of the top 15 authors in terms of publication volume (Figure 4D). Our results revealed that the author with the highest publication volume was Cai, Tianxi, with 17 articles, and the highest total link strength of 106. However, Cai, Tianxi ranked second in the norm. Citations with 22.10. The author ranked first in the norm. The citation was Liao, Katherine P., with a publication volume of 12 and a total link strength of 92, which placed them second. This indicates the significant influence of Cai, Tianxi, and Liao, Katherine P. in the field of study.
3.6 Publication analysis of journals
Through an analysis utilizing VOSviewer of journal citation networks, it was observed that Autoimmunity Reviews, Annals of Thoracic Surgery, European Journal of Cardio-Thoracic Surgery, Scientific Reports, and Lupus Science & Medicine emerged as the top five journals based on total link strength, achieving scores of 134, 114, 111, 105, and 99 respectively (Figure 5A). These findings suggest a pronounced citation relationship with other journals. Moreover, an examination of the temporal aspect of journal citations, as illustrated in Figure 5B, revealed that yellow nodes denote journals that have displayed heightened activity in the field in recent years, whereas progressively bluer nodes signify earlier periods of relative activity for these journals. Furthermore, by setting the minimum citation threshold to no fewer than 100, a total of 154 core journals were utilized to construct the co-citation network (Figure 5C). The results indicated that the total link strengths for Annals of the Rheumatic Diseases, Nature, Proceedings of the National Academy of Sciences of the United States of America, PLOS One, and Journal of Immunology were 88,303, 72,645, 60,534, 58,811, and 57,919, respectively.
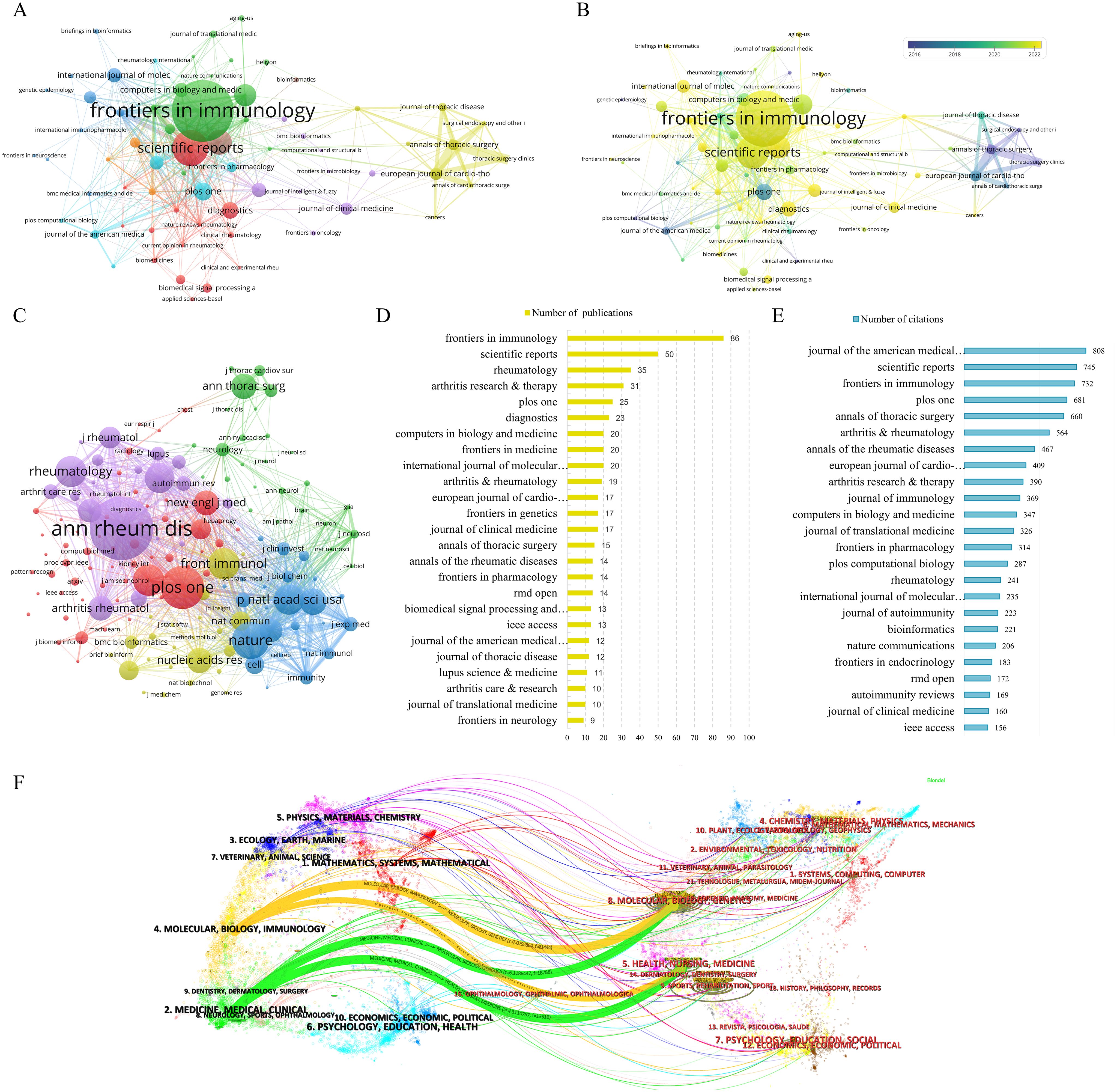
Figure 5. Journals analysis of publications in research fields. (A) Citation network analysis of journals. (B) Analysis of Journal Citation Years in the Field. (C) Co-citation network analysis of journals. (D) The 25 Journals with the Highest Number of Publications. (E) Top 25 journals in terms of citations. (F) The dual-map overlay of journals.
In the realm of academic publication output, Frontiers in Immunology emerged as the frontrunner with 86 articles, closely trailed by Scientific Reports with 50 articles, Rheumatology with 35 articles, Arthritis Research & Therapy with 31 articles, and PLOS One with 25 articles. Additionally, in terms of scholarly impact measured by citations, the Journal of the American Medical Informatics Association claimed the top position with 808 citations, followed by Scientific Reports with 745 citations, Frontiers in Immunology with 732 citations, PLOS One with 681 citations, and Annals of Thoracic Surgery with 660 citations (Figures 5D, E).
The dual-map overlay function illustrated the distribution of citing and cited journals, revealing the interdisciplinary connections between the research field and other disciplines. In Figure 5F, the dual-map overlay showed citing journals on the left side and cited journals on the right side. Two significant citation pathways were observed. The yellow path indicated that journals in the fields of molecular biology and immunology tended to cite publications from molecular biology, genetics, as well as health, nursing, and medical disciplines. The green path, on the other hand, suggested that medicine, medical, and clinical journals were inclined to cite publications from molecular, biology, genetics, as well as health, nursing, and medical fields.
3.7 Historical trajectory of the research field
3.7.1 Analysis of influential literature
Using HisCite, a citation history map of research articles was generated. Supplementary Table S3 listed literature of significant reference value based on the Local Citation Score (LCS) and Global Citation Score (GCS). The LCS indicates the frequency of citations a work receives within a specific research database or field, showcasing its influence within that scope. The study by Rea F et al. in 2006 reported the experience of thymectomy in myasthenia gravis patients using the “da Vinci” robotic system, which received the highest LCS of 45 (22). This was followed by the comparative study of robotic versus non-robotic thoracoscopic thymectomy by Rückert JC et al. in 2010, and the research by Yuanfang Guan et al. in 2019 on predicting the response to anti-tumor necrosis factor drugs in rheumatoid arthritis patients by integrating clinical and genetic markers using machine learning, with LCS values of 38 and 34, respectively (23, 24).
In VOSviewer, with the citation threshold set to no fewer than 25 times, a citation network graph comprising 309 publications was constructed (Figure 6A). The nodes in the graph represent the citation counts, with Forbes (2018) (25), Ritchie (2010) (26), and Ford (2016) (27) ranking top three with 254, 240, and 220 citations, respectively. Additionally, we analyzed the co-citation of literature in the research field by setting the citation frequency threshold to no fewer than 20 times, resulting in the selection of 80 publications for creating a co-citation network (Figure 6B). Through the analysis of the total link strength, it was revealed that the publications by Rea F (2006) (22), Ritchie ME (2015) (28), and Rückert JC (2011) (24) held prominent positions in the network, with total link strengths of 270, 235, and 204, respectively.
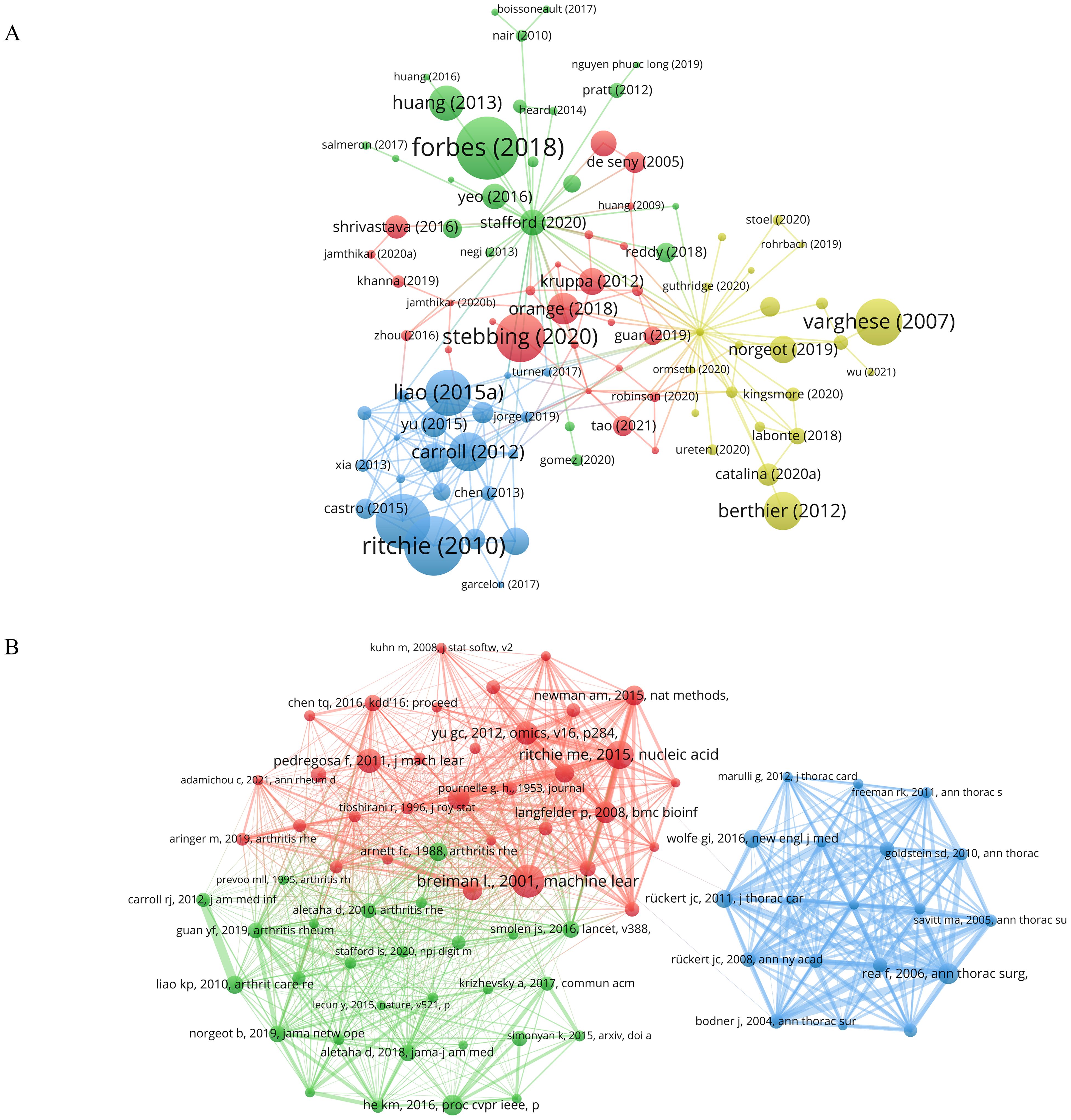
Figure 6. Analysis of publications in the field of research. (A) Network diagram of literatures citation analysis. (B) Network diagram for co-citation analysis of literatures. Node size indicates the citation count of the literature. The same color represents the same cluster.
3.7.2 Analysis of literature development characteristics
In bibliometrics, Citespace was used to cluster all references using the log-likelihood ratio (LLR) algorithm, resulting in the visualization of the top 9 clusters in Figure 7A. By plotting the average cluster years, we mapped the developmental trajectory of clusters in this research field. Furthermore, based on Citespace’s clustering criteria, silhouette values close to 1 indicated high cohesion and separation within the clusters, while values above 0.7 signified convincing clustering. Supplementary Table S4 detailed the cluster names chronologically.
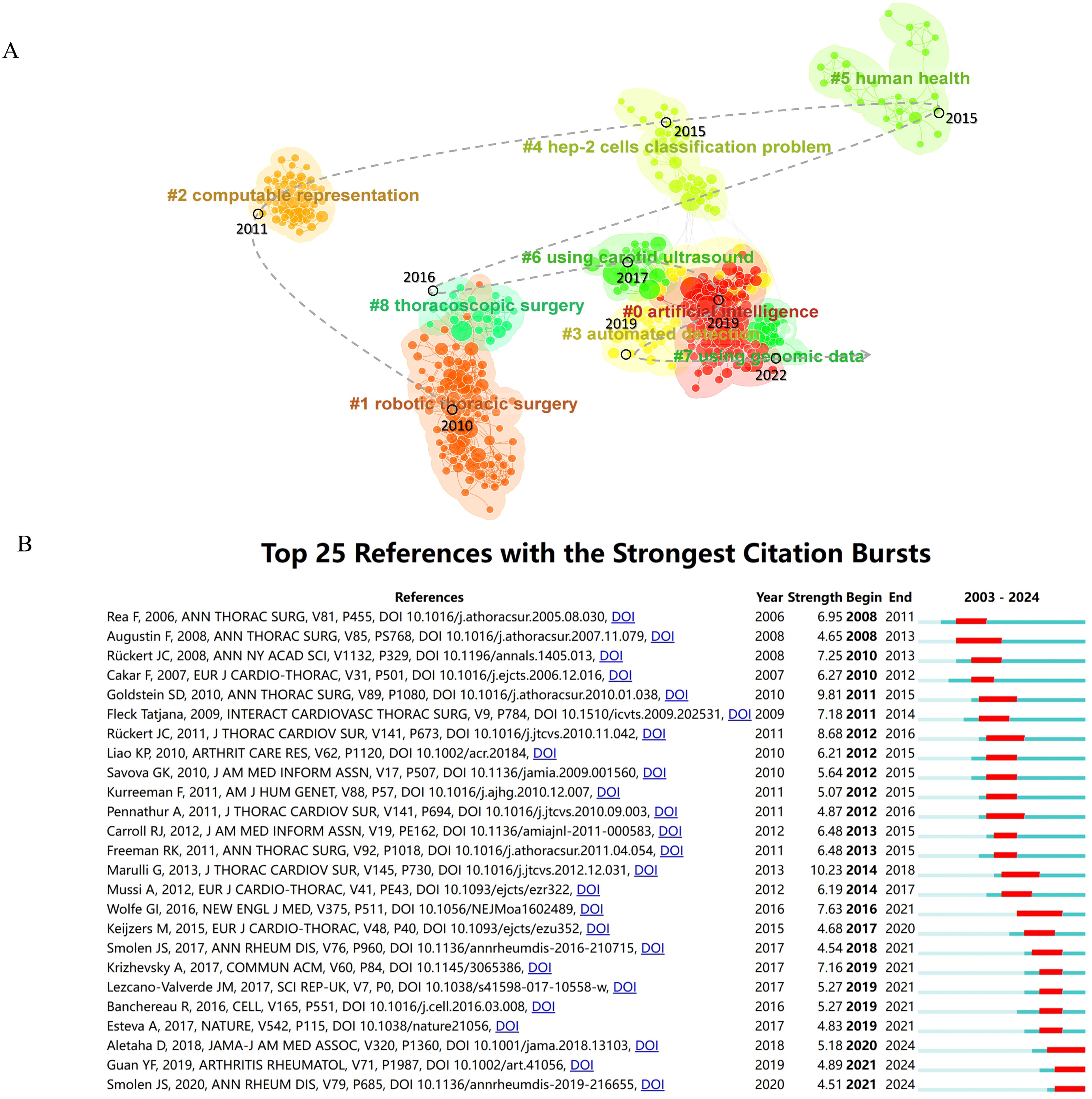
Figure 7. Analysis of the development characteristics of literature clusters. (A) Literature clustering analysis and its evolutionary trajectory. (B) Top 25 References with the Strongest Citation Bursts.
In 2010, the establishment of Cluster #1, Robotics Thoracic Surgery, marked a significant milestone in the field, with a primary focus on the utilization of robotic thymectomy in patients with myasthenia gravis (24, 29, 30). The subsequent year, 2011, witnessed the emergence of Cluster #2, Computable Representation, which delved into the utilization of electronic health records and algorithms to elevate the precision in the identification of conditions such as rheumatoid arthritis (31, 32). Fast forward to 2015, Clusters #4 and #5 came into existence. The former, Cluster #4, highlighted the pivotal role of HEp-2 cell classification in the realm of autoimmune disease diagnosis (33), whereas Cluster #5, Human Health, provided insights into the intricate relationship between the immune epitope database (IEDB) and gut microbiota. This cluster shed light on the dynamic interplay between gut microbiota and the host immune system, contributing to a deeper comprehension of immune system functionality (34–36). In 2016, Cluster #8 (Thoracoscopic Surgery) was established, focusing on conducting thymectomy using a combination of robotic and video-assisted thoracoscopic surgery (VATS) techniques (37). Cluster #6 (Using Carotid Ultrasound) emerged in 2017, with a focus on reporting the cardiovascular risk assessment in autoimmune disease patients through the use of carotid ultrasound B-mode imaging (38). Cluster #0 (Artificial Intelligence) and Cluster #3 (Automated Detection) shared many similar research foundations and were simultaneously formed in 2019. Cluster #0 focused on machine learning, aiming to better understand the complex mechanisms of autoimmune diseases by organizing and analyzing large volumes of clinical and immunological data to enhance diagnostic and predictive capabilities (23, 39, 40). Additionally, Cluster #3 centered on the identification of biomarkers associated with autoimmune diseases to gain further insights into the mechanisms of disease onset (39, 41). The final cluster to emerge in 2022, Cluster #7 (Using Genomic Data), originated from the machine learning cluster. The primary objective of this cluster was to employ machine learning techniques for the analysis of both traditional clinical data and novel genomic data, with the aim of developing predictive models that could enhance the clinical diagnosis and treatment of autoimmune diseases such as lupus nephritis and systemic lupus erythematosus (39, 42–44).
3.7.3 References with the strongest citation bursts
CiteSpace utilized an algorithm to identify the top 25 references with the strongest citation bursts, setting a threshold for a minimum burst duration of 3 years (Figure 7B). The case report by Rea F (2006) on thymectomy using the “da Vinci” robot for treating myasthenia gravis patients marked the earliest citation burst, lasting for 3 years (22). In 2013, Marulli G reported on the surgical and neurologic outcomes after robotic thymectomy in 100 consecutive patients with myasthenia gravis, which achieved the highest burst strength in the field at 10.23 and lasted for 4 years (30). Additionally, ongoing momentum was observed in some references, including recommendations by Aletaha D (2018) (45) and Smolen JS (2020) (46) on the diagnosis and management of rheumatoid arthritis, including personalized therapy through predictive markers, and Guan YF (2019) utilizing machine learning to predict drug responses in rheumatoid arthritis patients (23).
3.8 Keyword-based topic evolution and frontiers
3.8.1 Co-occurrence network analysis of keywords
The co-occurrence analysis reveals the hotspots and overall correlations in the research field. Utilizing VOSviewer, a co-occurrence network consisting of 130 keywords is constructed with a minimum co-occurrence frequency of not less than 15 words (Figure 8A). Upon analysis, it is observed that the most frequent co-occurring keywords in the network are “machine learning” with 372 occurrences and “rheumatoid arthritis” with 208 occurrences. Furthermore, the network exhibits distinct clustering, with a total of 5 clusters identified. Among these, 4 clusters demonstrate extensive interconnections, while the cluster represented by purple nodes, centered around “myasthenia gravis,” shows limited intersection with other clusters. This cluster primarily highlights the application of AI-based robotics in thymectomy procedures.
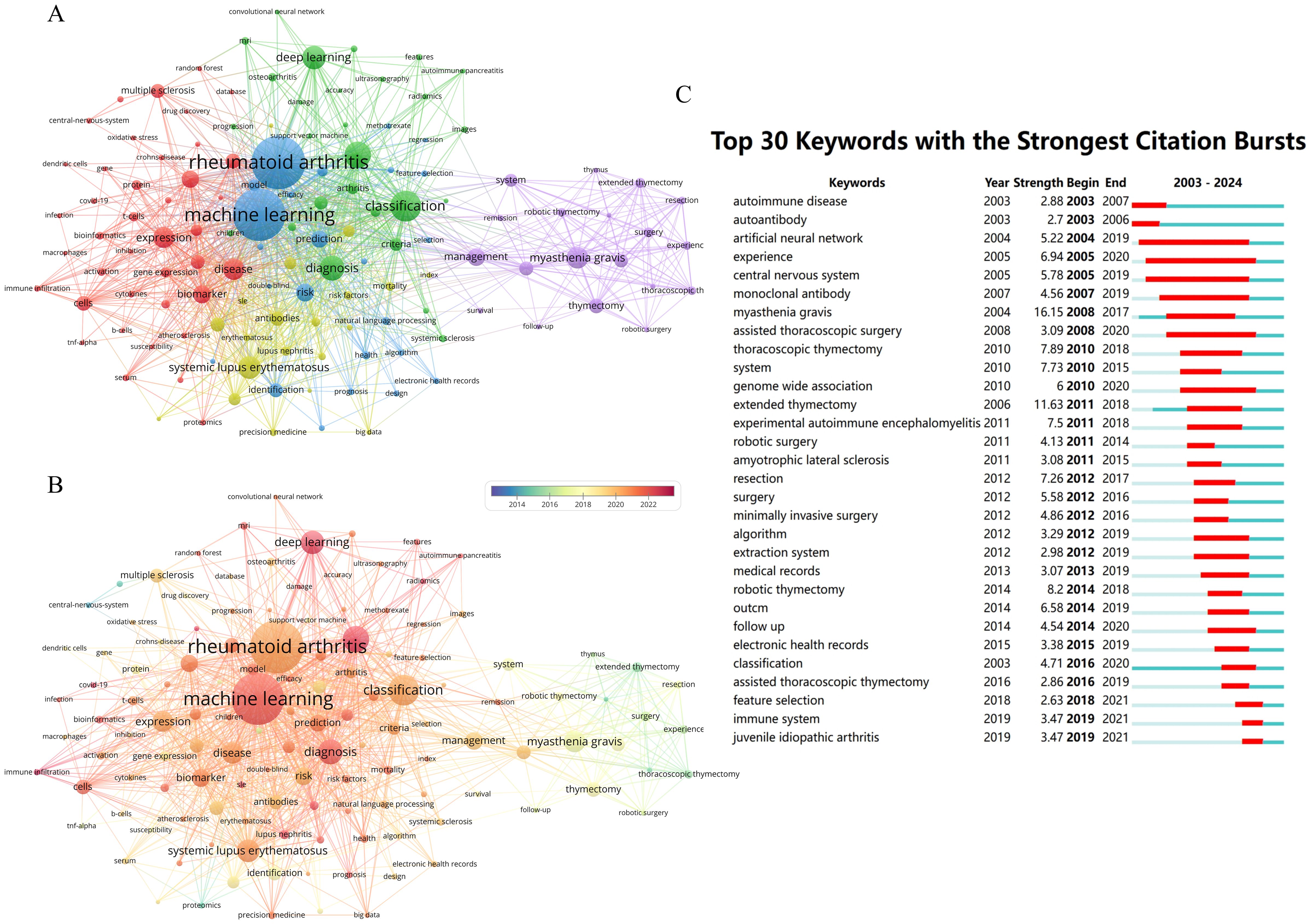
Figure 8. Keywords network analysis in the field. (A) Keyword co-occurrence network analysis. (B) Analysis of Keywords co-occurrence networks in a temporal perspective. (C) Top 30 Keywords with the Strongest Citation Bursts.
Due to different time periods, the academic frontiers focus on different hot topics. By analyzing the annual changes of the co-occurrence network, we found that the cluster represented by “thymectomy” was generally active before 2018, as shown in Figure 8B. Additionally, we have also observed that “machine learning” and “deep learning” are becoming hot topics in this research field, particularly in the study of key diseases such as rheumatoid arthritis, systemic lupus erythematosus, lupus nephritis, multiple sclerosis, among others.
3.8.2 Strongest citation bursts analysis of keywords
Keyword bursts signify a significant increase in the frequency of specific terms during a defined time period. Analyzing these bursts aids researchers in identifying emerging trends, predicting future directions, and guiding research decisions. Applying a threshold of no less than 3 years, Citespace conducted an analysis of the Strongest citation bursts of keywords based on Kleinberg’s burst detection algorithm (47). In Figure 8C, the top 30 keywords were listed, with “autoimmune disease” and “autoantibody” displaying the earliest onset of Strongest citation bursts, dating back to 2003. The keyword showing the highest burst strength was “myasthenia gravis” at 16.15. Furthermore, the keywords “artificial neural network” and “experience” maintained the longest durations, lasting for 15 years with burst intensity values of 5.22 and 6.94, respectively. Over the past five years, “immune system” and “juvenile idiopathic arthritis” have also emerged as focal points of research interest in the field.
3.8.3 Dynamic process of research topics
Clustering was performed using the LLR algorithm on a keyword co-occurrence network. The top 9 clusters depicted in Figure 9A include: #0 thymectomy, #1 mortality, #2 artificial intelligence, #3 immune infiltration, #4 risk factors, #5 ulcerative colitis, #6 hyposalivation, #7 rheumatoid arthritis, and #8 multiple sclerosis. The central item in Cluster #8 is (2005) with a centrality of 0.26, followed by (2005) in Cluster #7 with a centrality of 0.20, and (2005) in Cluster #1 with a centrality of 0.17. Figure 9B displayed the cluster landscape based on keyword co-occurrence, allowing for the observation of the distribution changes of each cluster from 2003 to 2024.
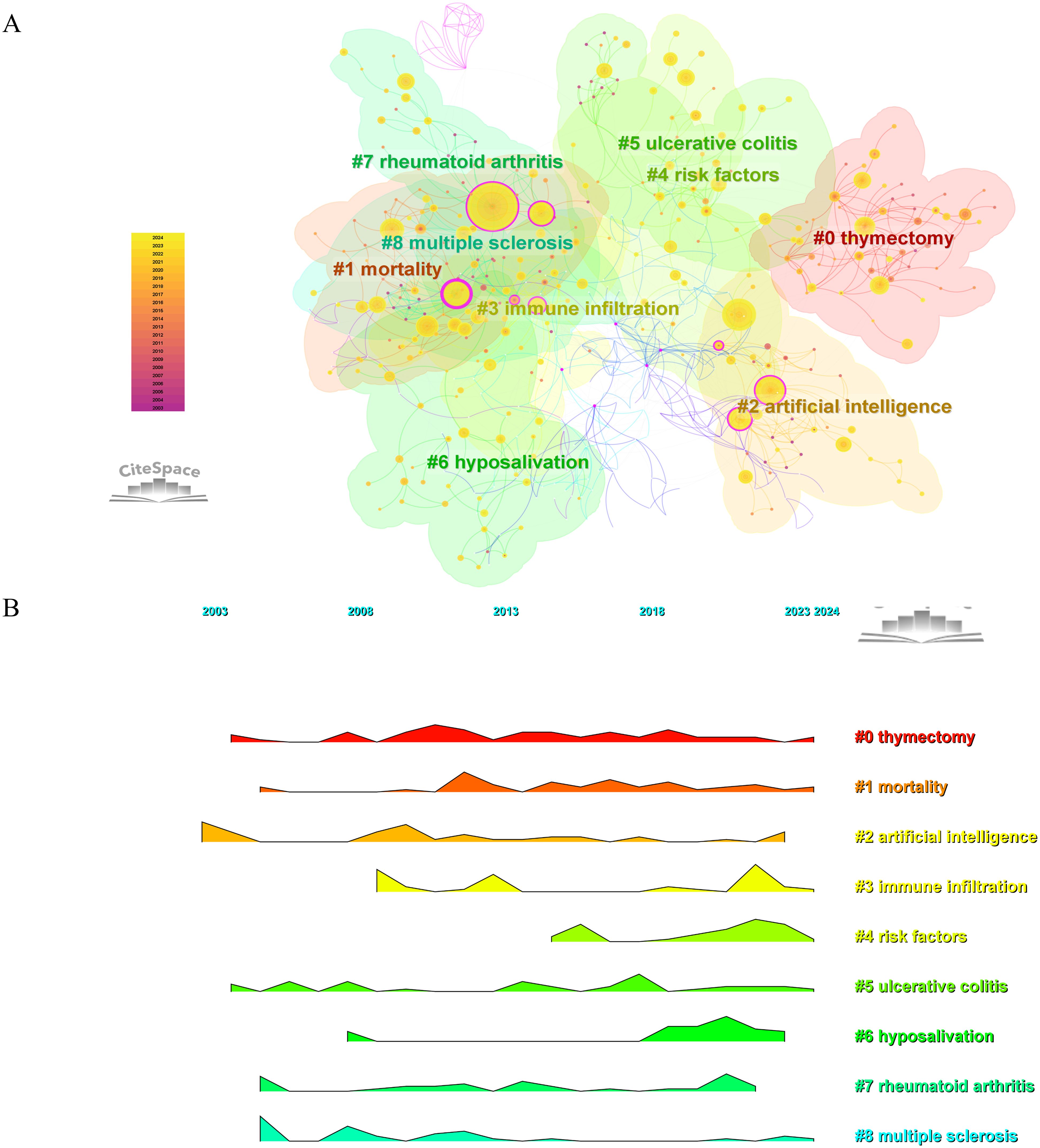
Figure 9. Keywords clustering analysis and development trajectory. (A) Keyword-based cluster analysis. (B) Landscape map analysis of keyword clusters.
4 Discussion
4.1 Global development issues in the research field of AI-AID
In this study, we observe an increasing trend in the number of publications in the field over the past twenty years, with a particularly rapid growth in the past five years. The number of publications surpassed 100 for the first time in 2020, reaching 139, and by 2023 had escalated to 341. In just the first half of 2024, the number had reached 222. This growth trend indicates a continuous rise in research interest in the field, possibly influenced by factors including advancements in related technologies, increased societal attention, and heightened research investments. However, we must also acknowledge that this rapid growth trend may bring about certain challenges. With the increasing number of publications, there could be variations in research quality, necessitating more rigorous peer review mechanisms to ensure the reliability and effectiveness of research.
Both the United States and China have made substantial contributions in this field, however, there is an evident developmental imbalance among countries and regions worldwide. Patients with AID in these two countries benefit from more accurate diagnosis and effective treatment, ultimately improving their quality of life and reducing the suffering and societal burden imposed by these illnesses. Publications and collaborations in the field from African and Central Asian countries are relatively scarce, potentially due to limited healthcare resources and research funding in these regions, leading to disparities in the application of artificial intelligence in medicine. Further analysis supports the notion that countries with fewer publications or citations are predominantly from middle- to low-income or low-income areas.
Although automated, AI-driven medical applications can overcome barriers to healthcare equity in middle- to low-income countries, AI medical models trained in high-income regions may exhibit suboptimal performance when applied in middle- to low-income countries due to the phenomenon of “data set shift,” where differences in patient populations, clinical practices, and healthcare systems lead to discrepancies (48, 49). Hence, it is essential for the future to enhance collaboration with middle- to low-income countries to ensure model generalizability by collecting data from diverse and representative populations across various countries and regions, thereby preventing data set shifting (50).
4.2 Current status of AI in autoimmune diseases
Through the analysis of key terms in the research field, the main applications of AI in autoimmune diseases have been identified. AI is most commonly utilized in diseases such as multiple sclerosis, rheumatoid arthritis, and systemic lupus erythematosus, possibly due to the high prevalence and significant clinical attention these diseases receive. The application scenarios of AI in this field encompass six main areas: patient identification, risk factors and prognosis assessment, diagnosis, classification of disease subtypes, monitoring and decision support, and drug discovery (Figure 10) (5, 51, 52).
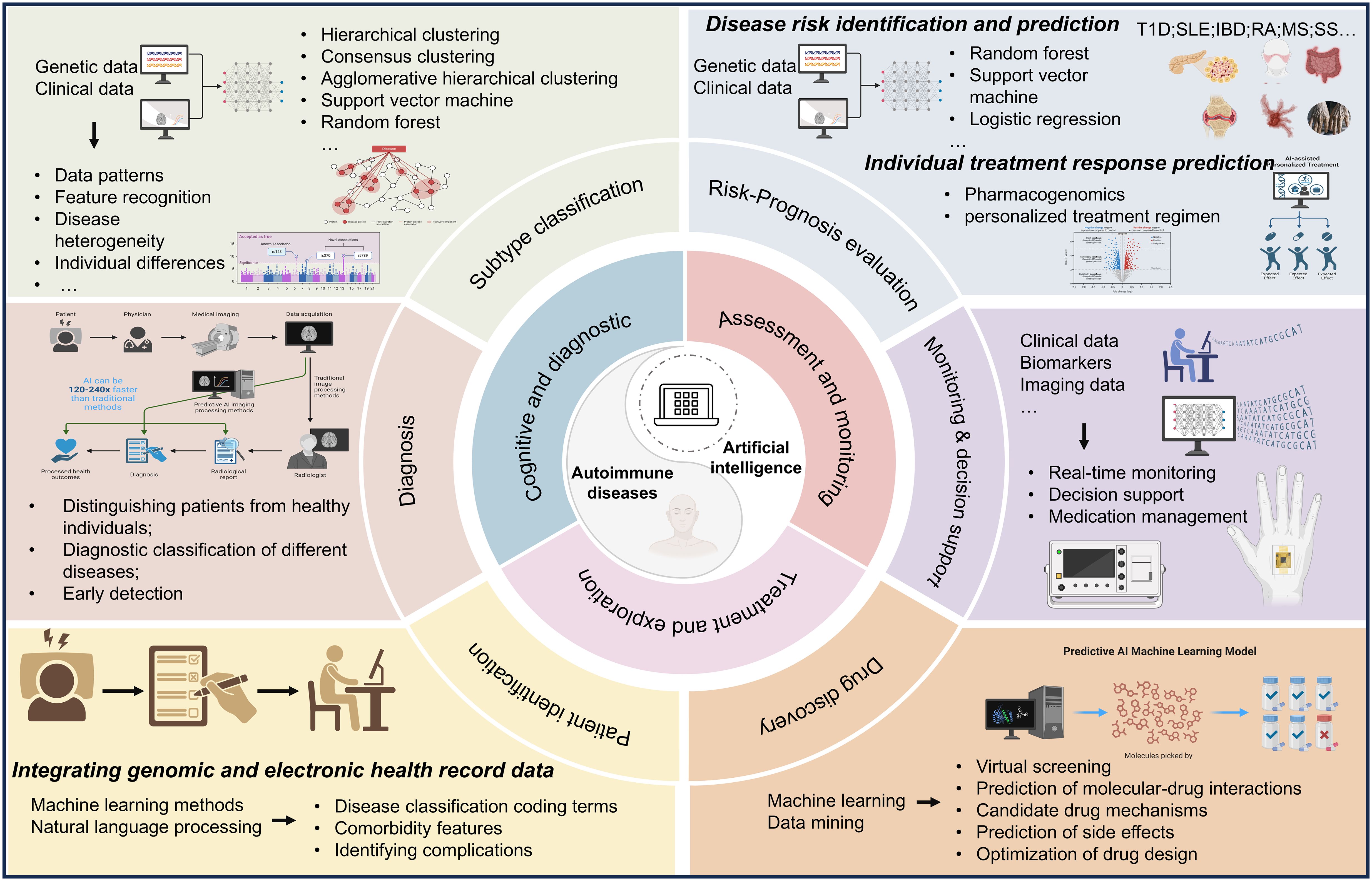
Figure 10. The scheme of major applications of artificial intelligence technology in autoimmune diseases.
4.2.1 Patient identification
Electronic medical records (EMRs) have the capability to integrate complex medical histories, facilitating the recording, supervision, and extraction of clinical data (53–59). Meanwhile, efforts are being made in some studies to integrate genomic and EMR data, advancing the field of precision medicine (60, 61). Machine learning methodologies are utilized for the identification of individuals diagnosed with autoimmune disorders from electronic health records, with natural language processing playing a crucial role in the recognition of associated comorbidities such as celiac disease, osteoarthritis, and rheumatoid arthritis-related complications (musculoskeletal symptoms, infections) (62–66). Furthermore, it is possible to reveal the comorbidity characteristics of autoimmune diseases with other factors, including the co-occurrence of hypertension and autoimmune disorders heightening the risk of Alzheimer’s disease, as well as polycystic ovary syndrome (PCOS) impacting immune system disorders (67, 68). Additionally, enhancements in algorithm efficiency contribute to reducing the elevated error rates stemming from inconsistent terminologies in the International Classification of Diseases coding (69–71).
4.2.2 Risk factors and prognosis assessment
The prediction of autoimmune disease risk and identification of new risk factors involve the utilization of genetic data, clinical data, and other resources (72–75). Common methods include random forests, support vector machines, and logistic regression (76, 77). Analysis of genetic data can reveal disease-associated genetic variations, elucidating the genetic basis of the disease and predicting individual disease risk. Integration and analysis of clinical data can uncover the relationships between clinical characteristics and autoimmune diseases. Feature selection algorithms and similar methods enable the identification of factors closely associated with disease risk from a vast amount of data. These approaches are applied in the prediction of diseases such as inflammatory bowel disease (IBD) (78, 79), type 1 diabetes (T1D) (80–82), rheumatoid arthritis (RA) (83–86), systemic lupus erythematosus (SLE) (87, 88), Sjögren’s syndrome (SS) (76)and multiple sclerosis (MS) (89–91).
In disease progression and prognosis, commonly used methods include support vector machines, random forests, and neural networks combined with clinical data. Research on disease progression and treatment encompasses conditions such as psoriasis, lupus nephritis, rheumatoid arthritis, inflammatory bowel disease, and celiac disease (92–98). Furthermore, machine learning can utilize patient treatment response data to predict the effectiveness of different treatment regimens for individual patients, such as the response to anti-rheumatic drugs, adalimumab, and etanercept in the treatment of rheumatoid arthritis (99, 100). It is worth mentioning that drug prognostic responses in systemic lupus erythematosus, multiple sclerosis, ankylosing spondylitis, inflammatory bowel disease, and psoriasis are gradually being applied (101–105). Genomics is also being increasingly integrated into this research area, exemplified by studies on drug genomic research related to methotrexate treatment response in patients with RA (106).
4.2.3 Diagnosis
AI plays a crucial role in disease diagnosis, encompassing aspects such as distinguishing patients from healthy controls, diagnostic classification of different diseases, and early detection. Some studies focus on distinguishing specific diseases such as RA and SLE from healthy controls (107–110), while others aim to differentiate between diseases with similar symptoms, including myalgic encephalomyelitis and chronic fatigue syndrome, multiple sclerosis, celiac disease, irritable bowel syndrome, and psoriasis (111–113). Additionally, there are research efforts dedicated to the early diagnosis of delayed-onset diseases like MS and RA (114, 115).
4.2.4 Classification of disease subtypes
In the classification of subtypes of autoimmune diseases, machine learning techniques are employed to utilize patient clinical data, genetic data, and other resources to identify potential subtypes by learning patterns and features in the data, thereby understanding disease heterogeneity and individual differences. Common methods include hierarchical clustering, consensus clustering, agglomerative hierarchical clustering, support vector machines, and random forests, among others. This research encompasses subtype classification of diseases such as RA, IBD, MS, SLE, SS, and idiopathic myositis (IM) (116–119).
4.2.5 Monitoring and decision support
Artificial intelligence technology is utilized to analyze patients’ clinical data, biological markers, and imaging data, enabling real-time monitoring and tracking of autoimmune diseases. This assists healthcare professionals in promptly identifying the progression status and changing trends of diseases. Machine learning is applied in the prediction of blood glucose levels, identification of hypoglycemic events, and decision support in diseases such as T1D (120, 121). Digital remote monitoring interventions are employed for disease activity monitoring in patients with inflammatory arthritis (122). Furthermore, AI is utilized for MRI monitoring in MS, treatment compliance in IBD, and drug management (123–125). AI integrated diverse data sources to achieve precise diagnosis and risk prediction, assisted in developing personalized treatment plans, and improved treatment outcomes while utilizing smart devices for real-time patient monitoring, thus providing continuous health management.
4.2.6 Drug discovery
In the field of drug discovery for autoimmune diseases, AI is employed to accelerate and enhance the drug development process using machine learning, data mining, and artificial intelligence technologies. This encompasses virtual screening, prediction of molecular-drug interactions, forecasting candidate drug mechanisms and side effects, as well as drug design optimization. For SLE, some biologics have been approved, and molecular analysis has identified specific molecular features and genetic markers of the disease (126–128). The treatment of RA includes various traditional medications and TNF antagonists, which, through genomic and transcriptomic analyses and machine learning models, guide the prediction of TNF treatment response and the development of new medications (129–132). Personalized drug discovery for other autoimmune diseases is also progressively advancing in relevance (3).
4.3 Challenges and prospects
AI shows potential in the field of autoimmune diseases, but it faces numerous problems and challenges. In terms of models, there is insufficient validation, necessitating enhanced cross-validation and independent testing to improve accuracy and reliability. Complex models are in demand, yet they encounter difficulties in computational power, methods, and data management. Data integration from multiple sources is challenging and requires standardization. Additionally, there is a lack of comprehensive “healthy” immune response models to differentiate between normal and diseased states. In treatment, the testing of combination therapies is hindered by numerous possibilities, requiring improved computational methods to assess their effectiveness and safety, while regulatory acceptance of digital evidence remains uncertain. Autoimmune diseases are complex and heterogeneous, posing difficulties in model construction and precise treatment implementation. Understanding disease mechanisms and developing advanced algorithms are crucial to address these challenges and drive progress in the field. Furthermore, there are significant privacy and security risks in medical health data, making the protection of patient data privacy a critical consideration when utilizing AI for autoimmune disease data processing (3, 5, 133–137). To address these challenges, future research should enhance cross-validation and independent testing, improve computational power, optimize data management and methods, promote data standardization, and develop universal and practical models. Furthermore, researchers must prioritize data privacy protection to ensure legal and compliant usage. Interdisciplinary collaboration should follow ethical guidelines to safeguard patients from potential harm and support the safe and reliable advancement of the field.
AI shows significant potential in the field of autoimmune diseases. The upcoming directions encompass enhanced diagnostic precision and subcategorization achieved by amalgamating diverse datasets and refining deep learning algorithms (138, 139). In the field of personalized therapy, AI is poised to expedite drug discovery processes and investigate combined treatment approaches (140). To facilitate disease surveillance and prognosis evaluation, AI can use wearable technologies and construct predictive models for continuous monitoring and risk assessment (105, 141). Furthermore, fostering interdisciplinary partnerships between medical and engineering sectors is imperative, particularly in the innovation of novel technologies such as digital twins (142). The establishment of extensive databases through data exchange and collaborative research initiatives across institutions will play a pivotal role in advancing autoimmune disease management (5).
4.4 Limitations
It should be noted that there are certain limitations to bibliometric analysis in practical implementation. Due to variations in inclusion criteria and sources among different databases, the publications covered may differ. However, mainstream analysis tools currently struggle to fully eliminate the impact of these differences. Therefore, the choice of using WoSCC as the primary database aims to ensure the credibility and reliability of the data to the greatest extent possible. Additionally, focusing solely on English literature may lead to some omissions, and alterations in institution names could also introduce biases. In summary, we acknowledge these potential issues in conducting bibliometric analysis and have referenced high-quality bibliometric literature in the research process to enhance the accuracy and reliability of the analysis results.
5 Conclusion
This study conducted a comprehensive bibliometric analysis on the application of AI in the field of autoimmune diseases, marking a pioneering endeavor. The analysis focused on publications from the past twenty years, aiming to thoroughly map the developmental trajectory and current status of this field. Through a systematic review of a large body of literature, we accurately identified key publications warranting attention in this field, meticulously examined the dynamic trends of key terms, pinpointed influential groups of authors, and highlighted professional journals and research institutions deserving close attention. Furthermore, this study vividly illustrated the evolution trajectory of research clusters in this field, providing a comprehensive overview of the current state, challenges, and future prospects of AI applications in the fields of autoimmune diseases. The findings serve as crucial reference points and direction indicators for further research and development in this field.
Data availability statement
The raw data supporting the conclusions of this article will be made available by the authors, without undue reservation.
Author contributions
SL: Conceptualization, Writing – original draft, Data curation, Investigation, Project administration, Software. YL: Formal analysis, Data curation, Writing – original draft. ML: Methodology, Writing – original draft. SS: Validation, Writing – original draft. YC: Visualization, Writing – original draft. XS: Visualization, Writing – original draft. CH: Funding acquisition, Supervision, Writing – review & editing, Conceptualization.
Funding
The author(s) declare that financial support was received for the research and/or publication of this article. This research was supported by the Research Funds of Center for Xin’an Medicine and Modernization of Traditional Chinese Medicine of IHM (2023CXMMTCM004), the Research Funds of Center for Xin’an Medicine and Modernization of Traditional Chinese Medicine of IHM (2023CXMMTCM015), the Scientific Research Program of Higher Education Institutions in Anhui Province (2024AH050948), and the Clinical Research Project of Anhui University of Traditional Chinese Medicine (2024YFYLCZX25).
Conflict of interest
The authors declare that the research was conducted in the absence of any commercial or financial relationships that could be construed as a potential conflict of interest.
Generative AI statement
The author(s) declare that no Generative AI was used in the creation of this manuscript.
Publisher’s note
All claims expressed in this article are solely those of the authors and do not necessarily represent those of their affiliated organizations, or those of the publisher, the editors and the reviewers. Any product that may be evaluated in this article, or claim that may be made by its manufacturer, is not guaranteed or endorsed by the publisher.
Supplementary material
The Supplementary Material for this article can be found online at: https://www.frontiersin.org/articles/10.3389/fimmu.2025.1525462/full#supplementary-material
References
1. Caliskan M, Brown CD, Maranville JC. A catalog of GWAS fine-mapping efforts in autoimmune disease. Am J Hum Genet. (2021) 108:549–63. doi: 10.1016/j.ajhg.2021.03.009
2. Gesheva V, Szekeres Z, Mihaylova N, Dimitrova I, Nikolova M, Erdei A, et al. Generation of gene-engineered chimeric DNA molecules for specific therapy of autoimmune diseases. Hum Gene Ther Methods. (2012) 23:357–65. doi: 10.1089/hgtb.2012.051
3. Moingeon P. Artificial intelligence-driven drug development against autoimmune diseases. Trends Pharmacol Sci. (2023) 44:411–24. doi: 10.1016/j.tips.2023.04.005
4. Sadeghi P, Karimi H, Lavafian A, Rashedi R, Samieefar N, Shafiekhani S, et al. Machine learning and artificial intelligence within pediatric autoimmune diseases: applications, challenges, future perspective. Expert Rev Clin Immunol. (2024) 20:1219–36. doi: 10.1080/1744666X.2024.2359019
5. Stafford IS, Kellermann M, Mossotto E, Beattie RM, MacArthur BD, Ennis S. A systematic review of the applications of artificial intelligence and machine learning in autoimmune diseases. NPJ Digit Med. (2020) 3:30. doi: 10.1038/s41746-020-0229-3
6. Desvaux E, Aussy A, Hubert S, Keime-Guibert F, Blesius A, Soret P, et al. Model-based computational precision medicine to develop combination therapies for autoimmune diseases. Expert Rev Clin Immunol. (2022) 18:47–56. doi: 10.1080/1744666X.2022.2012452
7. Katsila T, Konstantinou E, Lavda I, Malakis H, Papantoni I, Skondra L, et al. Pharmacometabolomics-aided pharmacogenomics in autoimmune disease. EBioMed. (2016) 5:40–5. doi: 10.1016/j.ebiom.2016.02.001
8. Laigle L, Chadli L, Moingeon P. Biomarker-driven development of new therapies for autoimmune diseases: current status and future promises. Expert Rev Clin Immunol. (2023) 19:305–14. doi: 10.1080/1744666X.2023.2172404
9. Tong Y, Cheng N, Jiang X, Wang K, Wang F, Lin X, et al. The trends and hotspots in premature ovarian insufficiency therapy from 2000 to 2022. Int J Environ Res Public Health. (2022) 19:11728. doi: 10.3390/ijerph191811728
10. Zhang Q, Li S, Liu J, Chen J. Global trends in nursing-related research on COVID-19: A bibliometric analysis. Front Public Health. (2022) 10:933555. doi: 10.3389/fpubh.2022.933555
11. Liu Y, Huang H, Zhou H, Yuan Y, Shi X. The evolution and future trends of stromal vascular fraction: A bibliometric analysis. Tissue Eng Part C Methods. (2024) 30:143–58. doi: 10.1089/ten.TEC.2023.0310
12. Zhang XL, Zheng Y, Xia ML, Wu YN, Liu XJ, Xie SK, et al. Knowledge domain and emerging trends in vinegar research: A bibliometric review of the literature from WoSCC. Foods. (2020) 9:166. doi: 10.3390/foods9020166
13. Tao G, Yang S, Xu J, Wang L, Yang B. Global research trends and hotspots of artificial intelligence research in spinal cord neural injury and restoration-a bibliometrics and visualization analysis. Front Neurol. (2024) 15:1361235. doi: 10.3389/fneur.2024.1361235
14. Liu J, Wang Y, Zhang Q, Wei J, Zhou H. Scientometric analysis of public health emergencies: 1994-2020. Int J Environ Res Public Health. (2022) 19:640. doi: 10.3390/ijerph19020640
15. Liu YX, Zhu C, Wu ZX, Lu LJ, Yu YT. A bibliometric analysis of the application of artificial intelligence to advance individualized diagnosis and treatment of critical illness. Ann Transl Med. (2022) 10:854. doi: 10.21037/atm-22-913
16. Zhang XM, Zhang X, Luo X, Guo HT, Zhang LQ, Guo JW. Knowledge mapping visualization analysis of the military health and medicine papers published in the web of science over the past 10 years. Mil Med Res. (2017) 4:23. doi: 10.1186/s40779-017-0131-8
17. Yao L, Hui L, Yang Z, Chen X, Xiao A. Freshwater microplastics pollution: Detecting and visualizing emerging trends based on Citespace II. Chemosphere. (2020) 245:125627. doi: 10.1016/j.chemosphere.2019.125627
18. Martínez-Martínez C, Esteve-Claramunt F, Prieto-Callejero B, Ramos-Pichardo JD. Stigma towards Mental Disorders among Nursing Students and Professionals: A Bibliometric Analysis. Int J Environ Res Public Health. (2022) 19:1839. doi: 10.3390/ijerph19031839
19. Peng C, Kuang L, Zhao J, Ross AE, Wang Z, Ciolino JB. Bibliometric and visualized analysis of ocular drug delivery from 2001 to 2020. J Control Release. (2022) 345:625–45. doi: 10.1016/j.jconrel.2022.03.031
20. Sweileh WM. Research trends on human trafficking: a bibliometric analysis using Scopus database. Global Health. (2018) 14:106. doi: 10.1186/s12992-018-0427-9
21. Zou M, Zhang W, Xu Y, Zhu Y. Relationship between COPD and GERD: A bibliometrics analysis. Int J Chron Obstruct Pulmon Dis. (2022) 17:3045–59. doi: 10.2147/COPD.S391878
22. Rea F, Marulli G, Bortolotti L, Feltracco P, Zuin A, Sartori F. Experience with the “da Vinci” robotic system for thymectomy in patients with myasthenia gravis: report of 33 cases. Ann Thorac Surg. (2006) 81:455–9. doi: 10.1016/j.athoracsur.2005.08.030
23. Guan Y, Zhang H, Quang D, Wang Z, Parker S, Pappas DA, et al. Machine learning to predict anti-tumor necrosis factor drug responses of rheumatoid arthritis patients by integrating clinical and genetic markers. Arthritis Rheumatol. (2019) 71:1987–96. doi: 10.1002/art.41056
24. Rückert JC, Swierzy M, Ismail M. Comparison of robotic and nonrobotic thoracoscopic thymectomy: a cohort study. J Thorac Cardiovasc Surg. (2011) 141:673–7. doi: 10.1016/j.jtcvs.2010.11.042
25. Forbes JD, Chen CY, Knox NC, Marrie RA, El-Gabalawy H, de Kievit T, et al. A comparative study of the gut microbiota in immune-mediated inflammatory diseases-does a common dysbiosis exist. Microbiome. (2018) 6:221. doi: 10.1186/s40168-018-0603-4
26. Ritchie MD, Denny JC, Crawford DC, Ramirez AH, Weiner JB, Pulley JM, et al. Robust replication of genotype-phenotype associations across multiple diseases in an electronic medical record. Am J Hum Genet. (2010) 86:560–72. doi: 10.1016/j.ajhg.2010.03.003
27. Ford E, Carroll JA, Smith HE, Scott D, Cassell JA. Extracting information from the text of electronic medical records to improve case detection: a systematic review. J Am Med Inform Assoc. (2016) 23:1007–15. doi: 10.1093/jamia/ocv180
28. Ritchie ME, Phipson B, Wu D, Hu Y, Law CW, Shi W, et al. limma powers differential expression analyses for RNA-sequencing and microarray studies. Nucleic Acids Res. (2015) 43:e47. doi: 10.1093/nar/gkv007
29. Goldstein SD, Yang SC. Assessment of robotic thymectomy using the Myasthenia Gravis Foundation of America Guidelines. Ann Thorac Surg. (2010) 89:1080–5; discussion 1085-6. doi: 10.1016/j.athoracsur.2010.01.038
30. Marulli G, Schiavon M, Perissinotto E, Bugana A, Di Chiara F, Rebusso A, et al. Surgical and neurologic outcomes after robotic thymectomy in 100 consecutive patients with myasthenia gravis. J Thorac Cardiovasc Surg. (2013) 145:730–5; discussion 735-6. doi: 10.1016/j.jtcvs.2012.12.031
31. Carroll RJ, Thompson WK, Eyler AE, Mandelin AM, Cai T, Zink RM, et al. Portability of an algorithm to identify rheumatoid arthritis in electronic health records. J Am Med Inform Assoc. (2012) 19:e162–9. doi: 10.1136/amiajnl-2011-000583
32. Liao KP, Cai T, Gainer V, Goryachev S, Zeng-treitler Q, Raychaudhuri S, et al. Electronic medical records for discovery research in rheumatoid arthritis. Arthritis Care Res (Hoboken). (2010) 62:1120–7. doi: 10.1002/acr.20184
33. Nanni L, Lumini A, Santos FLCD, Paci M, Hyttinen J. Ensembles of dense and dense sampling descriptors for the HEp-2 cells classification problem. Pattern Recog Lett. (2016) 82:28–35. doi: 10.1016/j.patrec.2016.01.026
34. Bolyen E, Rideout JR, Dillon MR, Bokulich NA, Abnet CC, Al-Ghalith GA, et al. Reproducible, interactive, scalable and extensible microbiome data science using QIIME 2. Nat Biotechnol. (2019) 37:852–7. doi: 10.1038/s41587-019-0209-9
35. Vita R, Overton JA, Greenbaum JA, Ponomarenko J, Clark JD, Cantrell JR, et al. The immune epitope database (IEDB) 3.0. Nucleic Acids Res. (2015) 43:D405–12. doi: 10.1093/nar/gku938
36. Zhang X, Zhang D, Jia H, Feng Q, Wang D, Liang D, et al. The oral and gut microbiomes are perturbed in rheumatoid arthritis and partly normalized after treatment. Nat Med. (2015) 21:895–905. doi: 10.1038/nm.3914
37. O’Sullivan KE, Kreaden US, Hebert AE, Eaton D, Redmond KC. A systematic review of robotic versus open and video assisted thoracoscopic surgery (VATS) approaches for thymectomy. Ann Cardiothorac Surg. (2019) 8:174–93. doi: 10.21037/acs.2019.02.04
38. Jamthikar AD, Gupta D, Puvvula A, Johri AM, Khanna NN, Saba L, et al. Cardiovascular risk assessment in patients with rheumatoid arthritis using carotid ultrasound B-mode imaging. Rheumatol Int. (2020) 40:1921–39. doi: 10.1007/s00296-020-04691-5
39. Danieli MG, Brunetto S, Gammeri L, Palmeri D, Claudi I, Shoenfeld Y, et al. Machine learning application in autoimmune diseases: State of art and future prospectives. Autoimmun Rev. (2024) 23:103496. doi: 10.1016/j.autrev.2023.103496
40. Norgeot B, Glicksberg BS, Trupin L, Lituiev D, GianFrancesco M, Oskotsky B, et al. Assessment of a deep learning model based on electronic health record data to forecast clinical outcomes in patients with rheumatoid arthritis. JAMA Netw Open. (2019) 2:e190606. doi: 10.1001/jamanetworkopen.2019.0606
41. Jang S, Kwon EJ, Lee JJ. Rheumatoid arthritis: pathogenic roles of diverse immune cells. Int J Mol Sci. (2022) 23:905. doi: 10.3390/ijms23020905
42. Ayoub I, Wolf BJ, Geng L, Song H, Khatiwada A, Tsao BP, et al. Prediction models of treatment response in lupus nephritis. Kidney Int. (2022) 101:379–89. doi: 10.1016/j.kint.2021.11.014
43. Chen Y, Huang S, Chen T, Liang D, Yang J, Zeng C, et al. Machine learning for prediction and risk stratification of lupus nephritis renal flare. Am J Nephrol. (2021) 52:152–60. doi: 10.1159/000513566
44. Jorge AM, Smith D, Wu Z, Chowdhury T, Costenbader K, Zhang Y, et al. Exploration of machine learning methods to predict systemic lupus erythematosus hospitalizations. Lupus. (2022) 31:1296–305. doi: 10.1177/09612033221114805
45. Aletaha D, Smolen JS. Diagnosis and management of rheumatoid arthritis: A review. JAMA. (2018) 320:1360–72. doi: 10.1001/jama.2018.13103
46. Smolen JS, Landewé R, Bijlsma J, Burmester GR, Dougados M, Kerschbaumer A, et al. EULAR recommendations for the management of rheumatoid arthritis with synthetic and biological disease-modifying antirheumatic drugs: 2019 update. Ann Rheum Dis. (2020) 79:685–99. doi: 10.1136/annrheumdis-2019-216655
47. Yang K, Hu Y, Qi H. Digital health literacy: bibliometric analysis. J Med Internet Res. (2022) 24:e35816. doi: 10.2196/35816
48. Ciecierski-Holmes T, Singh R, Axt M, Brenner S, Barteit S. Artificial intelligence for strengthening healthcare systems in low- and middle-income countries: a systematic scoping review. NPJ Digit Med. (2022) 5:162. doi: 10.1038/s41746-022-00700-y
49. Rajpurkar P, Lungren MP. The current and future state of AI interpretation of medical images. N Engl J Med. (2023) 388:1981–90. doi: 10.1056/NEJMra2301725
50. Ueda D, Matsumoto T, Ehara S, Yamamoto A, Walston SL, Ito A, et al. Artificial intelligence-based model to classify cardiac functions from chest radiographs: a multi-institutional, retrospective model development and validation study. Lancet Digit Health. (2023) 5:e525–525e533. doi: 10.1016/S2589-7500(23)00107-3
51. Collorone S, Coll L, Lorenzi M, Lladó X, Sastre-Garriga J, Tintoré M, et al. Artificial intelligence applied to MRI data to tackle key challenges in multiple sclerosis. Mult Scler. (2024) 30:767–84. doi: 10.1177/13524585241249422
52. Momtazmanesh S, Nowroozi A, Rezaei N. Artificial intelligence in rheumatoid arthritis: current status and future perspectives: A state-of-the-art review. Rheumatol Ther. (2022) 9:1249–304. doi: 10.1007/s40744-022-00475-4
53. Chen W, Huang Y, Boyle B, Lin S. The utility of including pathology reports in improving the computational identification of patients. J Pathol Inform. (2016) 7:46. doi: 10.4103/2153-3539.194838
54. Chen Y, Carroll RJ, Hinz ER, Shah A, Eyler AE, Denny JC, et al. Applying active learning to high-throughput phenotyping algorithms for electronic health records data. J Am Med Inform Assoc. (2013) 20:e253–9. doi: 10.1136/amiajnl-2013-001945
55. Lin C, Karlson EW, Dligach D, Ramirez MP, Miller TA, Mo H, et al. Automatic identification of methotrexate-induced liver toxicity in patients with rheumatoid arthritis from the electronic medical record. J Am Med Inform Assoc. (2015) 22:e151–61. doi: 10.1136/amiajnl-2014-002642
56. Ludvigsson JF, Pathak J, Murphy S, Durski M, Kirsch PS, Chute CG, et al. Use of computerized algorithm to identify individuals in need of testing for celiac disease. J Am Med Inform Assoc. (2013) 20:e306–10. doi: 10.1136/amiajnl-2013-001924
57. Murray SG, Avati A, Schmajuk G, Yazdany J. Automated and flexible identification of complex disease: building a model for systemic lupus erythematosus using noisy labeling. J Am Med Inform Assoc. (2019) 26:61–5. doi: 10.1093/jamia/ocy154
58. Turner CA, Jacobs AD, Marques CK, Oates JC, Kamen DL, Anderson PE, et al. Word2Vec inversion and traditional text classifiers for phenotyping lupus. BMC Med Inform Decis Mak. (2017) 17:126. doi: 10.1186/s12911-017-0518-1
59. Zhou SM, Fernandez-Gutierrez F, Kennedy J, Cooksey R, Atkinson M, Denaxas S, et al. Defining disease phenotypes in primary care electronic health records by a machine learning approach: A case study in identifying rheumatoid arthritis. PloS One. (2016) 11:e0154515. doi: 10.1371/journal.pone.0154515
60. Khan A, Shang N, Petukhova L, Zhang J, Shen Y, Hebbring SJ, et al. Medical records-based genetic studies of the complement system. J Am Soc Nephrol. (2021) 32:2031–47. doi: 10.1681/ASN.2020091371
61. Ryu B, Shin SY, Baek RM, Kim JW, Heo E, Kang I, et al. Clinical genomic sequencing reports in electronic health record systems based on international standards: implementation study. J Med Internet Res. (2020) 22:e15040. doi: 10.2196/15040
62. Chaganti S, Welty VF, Taylor W, Albert K, Failla MD, Cascio C, et al. Discovering novel disease comorbidities using electronic medical records. PloS One. (2019) 14:e0225495. doi: 10.1371/journal.pone.0225495
63. Chen W, Wei K, Zhao W, Zhou X. Estimation of key comorbidities for osteoarthritis progression based on the EMR-claims dataset. IEEE Access. (2019) 7:2431–42. doi: 10.1109/ACCESS.2019.2919998
64. Escudié JB, Rance B, Malamut G, Khater S, Burgun A, Cellier C, et al. A novel data-driven workflow combining literature and electronic health records to estimate comorbidities burden for a specific disease: a case study on autoimmune comorbidities in patients with celiac disease. BMC Med Inform Decis Mak. (2017) 17:140. doi: 10.1186/s12911-017-0537-y
65. Kamps A, Runhaar J, de Ridder M, de Wilde M, van der Lei J, Zhang W, et al. Comorbidity in incident osteoarthritis cases and matched controls using electronic health record data. Arthritis Res Ther. (2023) 25:114. doi: 10.1186/s13075-023-03086-8
66. Widdifield J, Ivers NM, Bernatsky S, Jaakkimainen L, Bombardier C, Thorne JC, et al. Primary care screening and comorbidity management in rheumatoid arthritis in Ontario, Canada. Arthritis Care Res (Hoboken). (2017) 69:1495–503. doi: 10.1002/acr.23178
67. Alessa M, Alsugheir S, Ahmed NAAAAE. Prevalence and predictors of seizure in patients with Alzheimer’s disease at a tertiary care center in Riyadh, Saudi Arabia. Trop J Pharm Res. (2021) 20:2381–6. doi: 10.4314/tjpr.v20i11.21
68. Sharmeen S, Nomani H, Taub E, Carlson H, Yao Q. Polycystic ovary syndrome: epidemiologic assessment of prevalence of systemic rheumatic and autoimmune diseases. Clin Rheumatol. (2021) 40:4837–43. doi: 10.1007/s10067-021-05850-0
69. Chamberlain AM, Roger VL, Noseworthy PA, Chen LY, Weston SA, Jiang R, et al. Identification of incident atrial fibrillation from electronic medical records. J Am Heart Assoc. (2022) 11:e023237. doi: 10.1161/JAHA.121.023237
70. Kapoor T, Mahadeshwar P, Hui-Yuen J, Quinnies K, Tatonetti N, Gartshteyn Y, et al. Prevalence of progressive multifocal leukoencephalopathy (PML) in adults and children with systemic lupus erythematosus. Lupus Sci Med. (2020) 7:e000388. doi: 10.1136/lupus-2020-000388
71. Kwa MC, Ardalan K, Laumann AE, Nardone B, West DP, Silverberg JI. Validation of international classification of diseases codes for the epidemiologic study of dermatomyositis. Arthritis Care Res (Hoboken). (2017) 69:753–7. doi: 10.1002/acr.23010
72. Chin CY, Hsieh SY, Tseng VS. eDRAM: Effective early disease risk assessment with matrix factorization on a large-scale medical database: A case study on rheumatoid arthritis. PloS One. (2018) 13:e0207579. doi: 10.1371/journal.pone.0207579
73. Daneshjou R, Wang Y, Bromberg Y, Bovo S, Martelli PL, Babbi G, et al. Working toward precision medicine: Predicting phenotypes from exomes in the Critical Assessment of Genome Interpretation (CAGI) challenges. Hum Mutat. (2017) 38:1182–92. doi: 10.1002/humu.23280
74. Liu C, Ackerman HH, Carulli JP. A genome-wide screen of gene-gene interactions for rheumatoid arthritis susceptibility. Hum Genet. (2011) 129:473–85. doi: 10.1007/s00439-010-0943-z
75. Wei Z, Wang W, Bradfield J, Li J, Cardinale C, Frackelton E, et al. Large sample size, wide variant spectrum, and advanced machine-learning technique boost risk prediction for inflammatory bowel disease. Am J Hum Genet. (2013) 92:1008–12. doi: 10.1016/j.ajhg.2013.05.002
76. Noguchi K, Saito I, Namiki T, Yoshimura Y, Nakaguchi T. Reliability of non-contact tongue diagnosis for Sjögren’s syndrome using machine learning method. Sci Rep. (2023) 13:1334. doi: 10.1038/s41598-023-27764-4
77. Volkova A, Ruggles KV. Predictive metagenomic analysis of autoimmune disease identifies robust autoimmunity and disease specific microbial signatures. Front Microbiol. (2021) 12:621310. doi: 10.3389/fmicb.2021.621310
78. Isakov O, Dotan I, Ben-Shachar S. Machine learning-based gene prioritization identifies novel candidate risk genes for inflammatory bowel disease. Inflammation Bowel Dis. (2017) 23:1516–23. doi: 10.1097/MIB.0000000000001222
79. Shajari E, Gagné D, Malick M, Roy P, Noël JF, Gagnon H, et al. Application of SWATH mass spectrometry and machine learning in the diagnosis of inflammatory bowel disease based on the stool proteome. Biomedicines. (2024) 12:333. doi: 10.3390/biomedicines12020333
80. Alazwari A, Abdollahian M, Tafakori L, Johnstone A, Alshumrani RA, Alhelal MT, et al. Predicting age at onset of type 1 diabetes in children using regression, artificial neural network and Random Forest: A case study in Saudi Arabia. PloS One. (2022) 17:e0264118. doi: 10.1371/journal.pone.0264118
81. Nguyen C, Varney MD, Harrison LC, Morahan G. Definition of high-risk type 1 diabetes HLA-DR and HLA-DQ types using only three single nucleotide polymorphisms. Diabetes. (2013) 62:2135–40. doi: 10.2337/db12-1398
82. Wei Z, Wang K, Qu HQ, Zhang H, Bradfield J, Kim C, et al. From disease association to risk assessment: an optimistic view from genome-wide association studies on type 1 diabetes. PloS Genet. (2009) 5:e1000678. doi: 10.1371/journal.pgen.1000678
83. Briggs FB, Ramsay PP, Madden E, Norris JM, Holers VM, Mikuls TR, et al. Supervised machine learning and logistic regression identifies novel epistatic risk factors with PTPN22 for rheumatoid arthritis. Genes Immun. (2010) 11:199–208. doi: 10.1038/gene.2009.110
84. González-Recio O, de Maturana EL, Vega AT, Engelman CD, Broman KW. Detecting single-nucleotide polymorphism by single-nucleotide polymorphism interactions in rheumatoid arthritis using a two-step approach with machine learning and a Bayesian threshold least absolute shrinkage and selection operator (LASSO) model. BMC Proc. (2009) 3 Suppl 7:S63. doi: 10.1186/1753-6561-3-s7-s63
85. Maksabedian Hernandez EJ, Tingzon I, Ampil L, Tiu J. Identifying chronic disease patients using predictive algorithms in pharmacy administrative claims: an application in rheumatoid arthritis. J Med Econ. (2021) 24:1272–9. doi: 10.1080/13696998.2021.1999132
86. Negi S, Juyal G, Senapati S, Prasad P, Gupta A, Singh S, et al. A genome-wide association study reveals ARL15, a novel non-HLA susceptibility gene for rheumatoid arthritis in North Indians. Arthritis Rheumatol. (2013) 65:3026–35. doi: 10.1002/art.38110
87. Davis NA, Lareau CA, White BC, Pandey A, Wiley G, Montgomery CG, et al. Encore: Genetic Association Interaction Network centrality pipeline and application to SLE exome data. Genet Epidemiol. (2013) 37:614–21. doi: 10.1002/gepi.21739
88. Li H, Zhou L, Zhou W, Zhang X, Shang J, Feng X, et al. Decoding the mitochondrial connection: development and validation of biomarkers for classifying and treating systemic lupus erythematosus through bioinformatics and machine learning. BMC Rheumatol. (2023) 7:44. doi: 10.1186/s41927-023-00369-0
89. Barile B, Ashtari P, Stamile C, Marzullo A, Maes F, Durand-Dubief F, et al. Classification of multiple sclerosis clinical profiles using machine learning and grey matter connectome. Front Robot AI. (2022) 9:926255. doi: 10.3389/frobt.2022.926255
90. Mowry EM, Hedström AK, GianFrancesco MA, Shao X, Schaefer CA, Shen L, et al. Incorporating machine learning approaches to assess putative environmental risk factors for multiple sclerosis. Mult Scler Relat Disord. (2018) 24:135–41. doi: 10.1016/j.msard.2018.06.009
91. Peng Y, Zheng Y, Tan Z, Liu J, Xiang Y, Liu H, et al. Prediction of unenhanced lesion evolution in multiple sclerosis using radiomics-based models: a machine learning approach. Mult Scler Relat Disord. (2021) 53:102989. doi: 10.1016/j.msard.2021.102989
92. Amirkhani A, Mosavi MR, Mohammadi K, Papageorgiou EI. A novel hybrid method based on fuzzy cognitive maps and fuzzy clustering algorithms for grading celiac disease. Neural Computing Appl. (2016) 30:1573–88. doi: 10.1007/s00521-016-2765-y
93. George Y, Aldeen M, Garnavi R. Psoriasis image representation using patch-based dictionary learning for erythema severity scoring. Comput Med Imaging Graph. (2018) 66:44–55. doi: 10.1016/j.compmedimag.2018.02.004
94. Lewis MJ. Predicting best treatment in rheumatoid arthritis. Semin Arthritis Rheumatol. (2024) 64S:152329. doi: 10.1016/j.semarthrit.2023.152329
95. Lin C, Karlson EW, Canhao H, Miller TA, Dligach D, Chen PJ, et al. Automatic prediction of rheumatoid arthritis disease activity from the electronic medical records. PloS One. (2013) 8:e69932. doi: 10.1371/journal.pone.0069932
96. Niehaus KE, Uhlig HH, Clifton DA. Phenotypic characterisation of Crohn’s disease severity. Annu Int Conf IEEE Eng Med Biol Soc. (2015) 2015:7023–6. doi: 10.1109/EMBC.2015.7320009
97. Raina A, Hennessy R, Rains M, Allred J, Hirshburg JM, Diven DG, et al. Objective measurement of erythema in psoriasis using digital color photography with color calibration. Skin Res Technol. (2016) 22:375–80. doi: 10.1111/srt.12276
98. Wang X, Fu S, Yu J, Ma F, Zhang L, Wang J, et al. Renal interferon-inducible protein 16 expression is associated with disease activity and prognosis in lupus nephritis. Arthritis Res Ther. (2023) 25:112. doi: 10.1186/s13075-023-03094-8
99. Salehi F, Lopera Gonzalez LI, Bayat S, Kleyer A, Zanca D, Brost A, et al. Machine learning prediction of treatment response to biological disease-modifying antirheumatic drugs in rheumatoid arthritis. J Clin Med. (2024) 13:3890. doi: 10.3390/jcm13133890
100. Tao W, Concepcion AN, Vianen M, Marijnissen A, Lafeber F, Radstake T, et al. Multiomics and machine learning accurately predict clinical response to adalimumab and etanercept therapy in patients with rheumatoid arthritis. Arthritis Rheumatol. (2021) 73:212–22. doi: 10.1002/art.41516
101. Ferrè L, Clarelli F, Pignolet B, Mascia E, Frasca M, Santoro S, et al. Combining clinical and genetic data to predict response to fingolimod treatment in relapsing remitting multiple sclerosis patients: A precision medicine approach. J Pers Med. (2023) 13:122. doi: 10.3390/jpm13010122
102. Lee S, Kang S, Eun Y, Won HH, Kim H, Lee J, et al. Machine learning-based prediction model for responses of bDMARDs in patients with rheumatoid arthritis and ankylosing spondylitis. Arthritis Res Ther. (2021) 23:254. doi: 10.1186/s13075-021-02635-3
103. Mease P, Husni ME, Kafka S, Chakravarty SD, Harrison DD, Lo KH, et al. Inhibition of radiographic progression across levels of composite index-defined disease activity in patients with active psoriatic arthritis treated with intravenous golimumab: results from a phase-3, double-blind, placebo-controlled trial. Arthritis Res Ther. (2020) 22:43. doi: 10.1186/s13075-020-2126-1
104. Nguyen NH, Picetti D, Dulai PS, Jairath V, Sandborn WJ, Ohno-MaChado L, et al. Machine learning-based prediction models for diagnosis and prognosis in inflammatory bowel diseases: A systematic review. J Crohns Colitis. (2022) 16:398–413. doi: 10.1093/ecco-jcc/jjab155
105. Toro-Domínguez D, Martorell-Marugán J, Martinez-Bueno M, López-Domínguez R, Carnero-Montoro E, Barturen G, et al. Scoring personalized molecular portraits identify Systemic Lupus Erythematosus subtypes and predict individualized drug responses, symptomatology and disease progression. Brief Bioinform. (2022) 23:bbac332. doi: 10.1093/bib/bbac332
106. Myasoedova E, Athreya AP, Crowson CS, Davis JM, Warrington KJ, Walchak RC, et al. Towards individualized prediction of response to methotrexate in early rheumatoid arthritis: a pharmacogenomics-driven machine learning approach. Arthritis Care Res (Hoboken). (2022) 74:879–88. doi: 10.1002/acr.24834
107. Alaqtash M, Sarkodie-Gyan T, Yu H, Fuentes O, Brower R, Abdelgawad A. Automatic classification of pathological gait patterns using ground reaction forces and machine learning algorithms. Annu Int Conf IEEE Eng Med Biol Soc. (2011) 2011:453–7. doi: 10.1109/IEMBS.2011.6090063
108. de Seny D, Fillet M, Meuwis MA, Geurts P, Lutteri L, Ribbens C, et al. Discovery of new rheumatoid arthritis biomarkers using the surface-enhanced laser desorption/ionization time-of-flight mass spectrometry ProteinChip approach. Arthritis Rheumatol. (2005) 52:3801–12. doi: 10.1002/art.21607
109. Liu C, Pan C, Shen J, Wang H, Yong L. MALDI-TOF MS combined with magnetic beads for detecting serum protein biomarkers and establishment of boosting decision tree model for diagnosis of colorectal cancer. Int J Med Sci. (2011) 8:39–47. doi: 10.7150/ijms.8.39
110. Niu Q, Huang Z, Shi Y, Wang L, Pan X, Hu C. Specific serum protein biomarkers of rheumatoid arthritis detected by MALDI-TOF-MS combined with magnetic beads. Int Immunol. (2010) 22:611–8. doi: 10.1093/intimm/dxq043
111. Arasaradnam RP, Westenbrink E, McFarlane MJ, Harbord R, Chambers S, O’Connell N, et al. Differentiating coeliac disease from irritable bowel syndrome by urinary volatile organic compound analysis–a pilot study. PloS One. (2014) 9:e107312. doi: 10.1371/journal.pone.0107312
112. Cowen EW, Liu CW, Steinberg SM, Kang S, Vonderheid EC, Kwak HS, et al. Differentiation of tumour-stage mycosis fungoides, psoriasis vulgaris and normal controls in a pilot study using serum proteomic analysis. Br J Dermatol. (2007) 157:946–53. doi: 10.1111/j.1365-2133.2007.08185.x
113. Ohanian D, Brown A, Sunnquist M, Furst J, Nicholson L, Klebek L, et al. Identifying key symptoms differentiating myalgic encephalomyelitis and chronic fatigue syndrome from multiple sclerosis. Neurol (ECronicon). (2016) 4:41–5.
114. Liu J, Chen N. A 9 mRNAs-based diagnostic signature for rheumatoid arthritis by integrating bioinformatic analysis and machine-learning. J Orthop Surg Res. (2021) 16:44. doi: 10.1186/s13018-020-02180-w
115. Saccà V, Sarica A, Novellino F, Barone S, Tallarico T, Filippelli E, et al. Evaluation of machine learning algorithms performance for the prediction of early multiple sclerosis from resting-state FMRI connectivity data. Brain Imaging Behav. (2019) 13:1103–14. doi: 10.1007/s11682-018-9926-9
116. Lopez C, Tucker S, Salameh T, Tucker C. An unsupervised machine learning method for discovering patient clusters based on genetic signatures. J BioMed Inform. (2018) 85:30–9. doi: 10.1016/j.jbi.2018.07.004
117. Mossotto E, Ashton JJ, Coelho T, Beattie RM, MacArthur BD, Ennis S. Classification of paediatric inflammatory bowel disease using machine learning. Sci Rep. (2017) 7:2427. doi: 10.1038/s41598-017-02606-2
118. Orange DE, Agius P, DiCarlo EF, Robine N, Geiger H, Szymonifka J, et al. Identification of three rheumatoid arthritis disease subtypes by machine learning integration of synovial histologic features and RNA sequencing data. Arthritis Rheumatol. (2018) 70:690–701. doi: 10.1002/art.40428
119. Wang Y, Wei W, Ouyang R, Chen R, Wang T, Yuan X, et al. Novel multiclass classification machine learning approach for the early-stage classification of systemic autoimmune rheumatic diseases. Lupus Sci Med. (2024) 11:e001125. doi: 10.1136/lupus-2023-001125
120. Georga EI, Protopappas VC, Ardigò D, Polyzos D, Fotiadis DI. A glucose model based on support vector regression for the prediction of hypoglycemic events under free-living conditions. Diabetes Technol Ther. (2013) 15:634–43. doi: 10.1089/dia.2012.0285
121. Georga EI, Protopappas VC, Polyzos D, Fotiadis DI. Evaluation of short-term predictors of glucose concentration in type 1 diabetes combining feature ranking with regression models. Med Biol Eng Comput. (2015) 53:1305–18. doi: 10.1007/s11517-015-1263-1
122. Arumalla N, Chan C, Gibson M, Man YL, Adas MA, Norton S, et al. The clinical impact of electronic patient-reported outcome measures in the remote monitoring of inflammatory arthritis: A systematic review and meta-analysis. Arthritis Rheumatol. (2023) 75:1892–903. doi: 10.1002/art.42559
123. Barnett M, Wang D, Beadnall H, Bischof A, Brunacci D, Butzkueven H, et al. A real-world clinical validation for AI-based MRI monitoring in multiple sclerosis. NPJ Digit Med. (2023) 6:196. doi: 10.1038/s41746-023-00940-6
124. Majidova K, Handfield J, Kafi K, Martin RD, Kubinski R. Role of digital health and artificial intelligence in inflammatory bowel disease: A scoping review. Genes (Basel). (2021) 12:1465. doi: 10.3390/genes12101465
125. McGinnis RS, Mahadevan N, Moon Y, Seagers K, Sheth N, Wright JA Jr, et al. A machine learning approach for gait speed estimation using skin-mounted wearable sensors: From healthy controls to individuals with multiple sclerosis. PloS One. (2017) 12:e0178366. doi: 10.1371/journal.pone.0178366
126. Guthridge JM, Lu R, Tran LT, Arriens C, Aberle T, Kamp S, et al. Adults with systemic lupus exhibit distinct molecular phenotypes in a cross-sectional study. EClinicalMed. (2020) 20:100291. doi: 10.1016/j.eclinm.2020.100291
127. Smith MA, Chiang CC, Zerrouki K, Rahman S, White WI, Streicher K, et al. Using the circulating proteome to assess type I interferon activity in systemic lupus erythematosus. Sci Rep. (2020) 10:4462. doi: 10.1038/s41598-020-60563-9
128. Yaung KN, Yeo JG, Kumar P, Wasser M, Chew M, Ravelli A, et al. Artificial intelligence and high-dimensional technologies in the theragnosis of systemic lupus erythematosus. Lancet Rheumatol. (2023) 5:e151–151e165. doi: 10.1016/S2665-9913(23)00010-3
129. Aterido A, Cañete JD, Tornero J, Blanco F, Fernández-Gutierrez B, Pérez C, et al. A combined transcriptomic and genomic analysis identifies a gene signature associated with the response to anti-TNF therapy in rheumatoid arthritis. Front Immunol. (2019) 10:1459. doi: 10.3389/fimmu.2019.01459
130. Robert M, Miossec P. IL-17 in rheumatoid arthritis and precision medicine: from synovitis expression to circulating bioactive levels. Front Med (Lausanne). (2018) 5:364. doi: 10.3389/fmed.2018.00364
131. Yoosuf N, Maciejewski M, Ziemek D, Jelinsky SA, Folkersen L, Müller M, et al. Early prediction of clinical response to anti-TNF treatment using multi-omics and machine learning in rheumatoid arthritis. Rheumatol (Oxford). (2022) 61:1680–9. doi: 10.1093/rheumatology/keab521
132. Zhao J, Guo S, Schrodi SJ, He D. Molecular and cellular heterogeneity in rheumatoid arthritis: mechanisms and clinical implications. Front Immunol. (2021) 12:790122. doi: 10.3389/fimmu.2021.790122
133. Fasano S, Milone A, Nicoletti GF, Isenberg DA, Ciccia F. Precision medicine in systemic lupus erythematosus. Nat Rev Rheumatol. (2023) 19:331–42. doi: 10.1038/s41584-023-00948-y
134. Guthridge JM, Wagner CA, James JA. The promise of precision medicine in rheumatology. Nat Med. (2022) 28:1363–71. doi: 10.1038/s41591-022-01880-6
135. Kelly CJ, Karthikesalingam A, Suleyman M, Corrado G, King D. Key challenges for delivering clinical impact with artificial intelligence. BMC Med. (2019) 17:195. doi: 10.1186/s12916-019-1426-2
136. Obermeyer Z, Emanuel EJ. Predicting the future - big data, machine learning, and clinical medicine. N Engl J Med. (2016) 375:1216–9. doi: 10.1056/NEJMp1606181
137. Toro-Domínguez D, Alarcón-Riquelme ME. Precision medicine in autoimmune diseases: fact or fiction. Rheumatol (Oxford). (2021) 60:3977–85. doi: 10.1093/rheumatology/keab448
138. Anchang CG, Xu C, Raimondo MG, Atreya R, Maier A, Schett G, et al. The potential of OMICs technologies for the treatment of immune-mediated inflammatory diseases. Int J Mol Sci. (2021) 22:7506. doi: 10.3390/ijms22147506
139. Martorell-Marugán J, López-Domínguez R, García-Moreno A, Toro-Domínguez D, Villatoro-García JA, Barturen G, et al. A comprehensive database for integrated analysis of omics data in autoimmune diseases. BMC Bioinf. (2021) 22:343. doi: 10.1186/s12859-021-04268-4
140. Vamathevan J, Clark D, Czodrowski P, Dunham I, Ferran E, Lee G, et al. Applications of machine learning in drug discovery and development. Nat Rev Drug Discovery. (2019) 18:463–77. doi: 10.1038/s41573-019-0024-5
141. Burmester GR. Rheumatology 4.0: big data, wearables and diagnosis by computer. Ann Rheum Dis. (2018) 77:963–5. doi: 10.1136/annrheumdis-2017-212888
Keywords: artificial intelligence, autoimmune diseases, bibliometric exploration, forefront, content analysis
Citation: Liu S, Liu Y, Li M, Shang S, Cao Y, Shen X and Huang C (2025) Artificial intelligence in autoimmune diseases: a bibliometric exploration of the past two decades. Front. Immunol. 16:1525462. doi: 10.3389/fimmu.2025.1525462
Received: 09 November 2024; Accepted: 27 March 2025;
Published: 22 April 2025.
Edited by:
Andrew W. Taylor, Boston University, United StatesReviewed by:
Irami Araújo Filho, Federal University of Rio Grande do Norte, BrazilZhao-wei Gao, Tangdu Hospital, China
Copyright © 2025 Liu, Liu, Li, Shang, Cao, Shen and Huang. This is an open-access article distributed under the terms of the Creative Commons Attribution License (CC BY). The use, distribution or reproduction in other forums is permitted, provided the original author(s) and the copyright owner(s) are credited and that the original publication in this journal is cited, in accordance with accepted academic practice. No use, distribution or reproduction is permitted which does not comply with these terms.
*Correspondence: Chuanbing Huang, Y2h1YW5iaW5oQDE2My5jb20=