- 1Department of Pharmacy, Iqra University, Islamabad, Pakistan
- 2Department of Pharmaceutics, Riphah Institute of Pharmaceutical Sciences, Riphah International University, Islamabad, Pakistan
- 3Department of Medical Oncology, Jiangsu Cancer Hospital and Jiangsu Institute of Cancer Research and the Affiliated Cancer Hospital of Nanjing Medical University, Nanjing, China
- 4Department of Clinical Pharmacy, School of Basic Medicine and Clinical Pharmacy, China Pharmaceutical University, Nanjing, China
Background: Despite the high prevalence of lung cancer, with a five-year survival rate of only 23%, the underlying molecular mechanisms of non-small cell lung cancer (NSCLC) remain unknown. There is a great need to identify reliable candidate biomarker genes for early diagnosis and targeted therapeutic strategies to prevent cancer progression.
Methods: In this study, four datasets obtained from the Gene Expression Omnibus were evaluated for NSCLC- associated differentially expressed genes (DEGs) using bioinformatics analysis. About 10 common significant DEGs were shortlisted based on their p-value and FDR (DOCK4, ID2, SASH1, NPR1, GJA4, TBX2, CD24, HBEGF, GATA3, and DDR1). The expression of significant genes was validated using experimental data obtained from TCGA and the Human Protein Atlas database. The human proteomic data for post- translational modifications was used to interpret the mutations in these genes.
Results: Validation of DEGs revealed a significant difference in the expression of hub genes in normal and tumor tissues. Mutation analysis revealed 22.69%, 48.95%, and 47.21% sequence predicted disordered regions of DOCK4, GJA4, and HBEGF, respectively. The gene-gene and drug-gene network analysis revealed important interactions between genes and chemicals suggesting they could act as probable drug targets. The system-level network showed important interactions between these genes, and the drug interaction network showed that these genes are affected by several types of chemicals that could serve as potential drug targets.
Conclusions: The study demonstrates the importance of systemic genetics in identifying potential drug- targeted therapies for NSCLC. The integrative system- level approach should contribute to a better understanding of disease etiology and may accelerate drug discovery for many cancer types.
Introduction
The increasing incidence of lung cancer has made it the leading cause of cancer death among all human carcinomas. Globally, more than one million people die from lung cancer every year. Lung cancer (LC) consists of two main subtypes, non-small-cell lung cancer (NSCLC) and small-cell lung cancer (SCLC). The chief pathological form of lung cancer is NSCLC, accounting for 80-85% of cases. It includes adenocarcinoma (LUAC) and squamous cell carcinoma (LUSC) (1). With the remarkable advancement of medical technology in recent years, there is still an overall 5-year survival rate of 10-15% with no positive prognosis for lung cancer (2). The reason may be partly due to the problems encountered in the early diagnosis of the disease, in addition to the ineffective pharmacological targets for NSCLC patients (3). Mostly, the diagnosis of NSCLC is made when the disease has reached a progressive stage (2, 4). Some of the important risk factors that have shown an association with NSCLC are tobacco and air pollution, occupational hazards, and dietary and genetic factors that also contribute to its occurrence (5, 6). Over the past two decades, the treatment options for NSCLC have advanced significantly, requiring the need for alternatives to conventional treatment approaches, for example, molecularly targeted therapies and immunotherapies (3, 6).
The c omputational biology and systems biology approaches have greatly facilitated the drug discovery process, which has substantially minimized the cost of drug development. Several drug targets have been identified in our previous studies for breast cancer (7–9), colorectal cancer, methicillin- resistant Staphylococcus aureus (10–12), type 2 diabetes mellitus (13), and hTERT inhibitors (14). There is a great role for genes in the diagnosis, treatment, and prognosis of NSCLC when compared to histological classification. One of the most powerful and reliable techniques to quantify the expression of all genes is RNA microarray analysis (7, 10, 13–15). G ene expression profiling in NSCLC has been extensively done using RNA microarrays; nevertheless, not all genes have been fully explored. D ifferential expression analysis has been widely used as a bioinformatics tool in oncology research in recent years. The differentially expressed genes (DEGs) can be used to explore major diagnoses and identify effective therapeutic approaches for NSCLC, which play a crucial role in the management of cancer occurrence and progression (16). One of the bases for identifying novel targets and the molecular mechanism of NSCLC is to understand the interactions among the identified DEGs, their important signaling pathways, and the proteins through which they interact and cross- talk. Therefore, exploring the existing database and validating the effective targets is beneficial for the early diagnosis and therapeutic approach of NSCLC.
In this study, four microarray gene datasets (GSE1987, GSE17073, GSE 54495, and GSE118370) from the Gene Expression Omnibus (GEO) were accessed and analyzed. DEGs were analyzed between NSCLC tissue and normal lung tissue. Moreover, gene ontology, enrichment, and protein-protein interaction network analysis were performed to clarify the molecular mechanisms of the development and progression of NSCLC. Microarray technology assists in identifying unusual alterations in genome expression analysis. The identified DEGs may have the potential for future targeted therapy, providing better gene selection and serving as candidate biomarkers for NSCLC. This study also identified the genetic variants of NSCLC and their causes, which may help modify therapeutic strategies.
Materials and methods
Processing of microarray datasets
The Gene Expression Omnibus database (GEO) is a public functional database for high- throughput screening of gene expression data, microarray data, and gene chips. In this study, we recovered genome expression datasets from GEO (Affymetrix Human Genome U133A Plus 2.0 Array, Affymetrix Human Genome U95A Array, and Affymetrix Human Genome U133 Plus 2.0 Array) [GSE1987, GSE17073, GSE 54495, GSE118370]. The GSE1987, GSE17073, GSE 54495, and GSE118370 datasets contain 28, two, 17, and six NSCLC tissue samples and 9, 10, 13, and six non-cancer tissue samples, respectively (Table 1). The software tools used in this study are listed in Supplementary Table 1.
Raw data preprocessing, screening, and integration of DEGs
The differentially expressed genes were analyzed using GEO2R (http://www.ncbi.nlm.gov/geo2r), an interactive network tool for screening between NSCLC and non-cancer samples. The tool helps to compare and analyze two or more datasets under experimental conditions. The significant DEGs were detected using the adjusted p-values and the Benjamini- Hochberg false discovery rate. The probe sets with no gene names or genes with multiple probe sets were removed from the study. The upregulated genes were identified using cut- off values of P<0.05 and logFC >1, while the downregulated genes were identified with logFC <1.
Disease gene curation of DEGs
The c uration of significant DEGs was performed with the help of the Comparative Toxicogenomics Database (CTD), the Online Mendelian Inheritance in Man (OMIM), PubMed, and MeSH databases in order to curate their role in NSCLC. The DAVID database was used to retrieve the gene symbol, name, and UniProt ID of the identified DEGs.
Gene enrichment analysis
To identify the functional annotation and gene ontology of the shortlisted DEGs, the DAVID tool was used for functional enrichment analysis for their p- and FDR values. The biological function, transcription factors, and clinical phenotypes of NSCLC-related DEGs were analyzed using the FunRich tool.
Mutation analysis
The genotype-phenotype association helps in decoding the genetic variations, which aids in understanding the mutations arising from cancer and the inherited disease- related processes. Several single nucleotide variants (SNVs) are part of the human genome and are involved in the progression of the disease. The missense SNVs present at the PTM (post-translation modification) protein sites are associated with disease progression due to the substitution of approximately 21% of amino acids. This chemical modification of the amino acid ultimately alters the function of the protein (17). The online web tool ActiveDriverDB database was used to analyze the mutations associated with differentially expressed genes (17). The overall visual summary of the position, frequency, and functional significance of the mutations in the DEGs was provided by the needle plot mutation analysis. We observed the predicted disordered region and the PTM sites for all mutations in the protein sequence. The position of the gene sequence was observed by placing the pin along the protein. The figure legends explain the related mutational effects and PTM sites.
Protein-protein interaction
The protein-protein network analysis was performed to study the interaction of each protein with one or more genes associated with its molecular functions (18). The network reveals the altered activity of these genes in normal or pathological conditions. The potential NSCLC- related gene signatures associated with other genes whose dysfunction results in the disease state were identified through this network. The STRING database was used to analyze the protein-protein interactions of the cDNA dataset DEGs (19). The high confidence score interaction was used in this network analysis, having a score of 0.9-1. The target genes identified by this network were then studied for their role in NSCLC using Cancer GeneticsWeb, the National Cancer Institute, and the OMIM databases. Cytoscape (version 3.9.1) (20) was used to visualize the molecular network and Network Analyzer was used to calculate the topological properties of the networks. The nodes in the network categorized the degree of annotation between genes and diseases.
Identification and validation of shortlisted DEGs
T o validate the expression pattern of identified DEGs in normal and tumor tissues, the GEPIA database was utilized (21). GEPIA (Gene Expression Profiling Interactive Analysis) is helpful in differential expression analysis, patient survival analysis, correlation and profiling plotting, and several other key interactive and customizable features. G ene expression plots in GEPIA are based on TCGA clinical annotations. The Human Protein Atlas database was used to validate the translational levels of the identified oncogenes. V alidation was performed using immunohistopathologic sections between the normal and OSCC samples (https://www.proteinatlas.org/).
Toxicogenomic analysis
The comparative toxicogenomic database (CTD) was used to perform the toxicogenomic analysis. The CTD helps in retrieving the exposome data, which explores the chemical-genome to phenome association. The analysis investigates the mechanism of functional pathway signaling toward the progression of the disease. The chemical-gene and disease interactions are inferred, revealing the particular expression of the gene and its association with disease (22).
miRNA prediction analysis
microRNAs (miRNAs) are small non- coding RNAs that act as post-translational regulators that influence the genes involved in biological signaling pathways. In order to study the gene etiology, the expression and functional role of miRNA play a significant role (23). The disease- specific functional and molecular annotation of DEGs can be investigated by predicting the miRNA target (24). The miRDB online database was used to predict the NSCLC- related DEG miRNA targets for functional target prediction. The prediction data includes several important descriptions, such as 3′-UTR region length, miRNA-candidate target pairs along with target prediction scores, 3′-UTR sequences, miRNA seed binding sites, miRNA target sequences, etc. A score >80 was considered reliable, and the miRNA target was ranked (25, 26). The miRNA targets were analyzed for their site of expression and the biological pathways in which they are commonly used, with the help of the FunRich tool.
Drug-gene network
The drug -gene network analysis was performed to correlate the shortlisted DEGs with the chemicals/drugs that affect the activity of these genes. The CTD database was used to screen the chemical and disease relationships with default parameters. A direct link between the DEGs and anticancer drugs was developed. The FDA approval status of the identified drugs was verified with the Drug Bank database.
Results
Differential expression analysis and identification of DEGs
The four datasets GSE17073, GSE1987, GSE54495, and GSE118370 were used to screen and identify the DEGs after obtaining the microarray normalization results. The dataset GSE17073 contained 30 significant DEGs, GSE1987 contained 508 significant DEGs, GSE54459 contained five significant DEGs, and GSE118370 contained 707 significant DEGs. The volcano plot and the mean difference plot of DEGs from different datasets revealed the significant genes that were upregulated and downregulated. The blue color shows the down regulated genes, while the red indicates the upregulated genes (Figure 1). In GSE1987, GSE17073, GSE54495, and GSE118370, 403, zero, one, and 354 genes are upregulated, respectively, while 405, 30, four, and 353 DEGs are downregulated. In the Venn diagram, the overlap between the datasets was observed, which showed an overlap of 10 significant DEGs with a p-value of <0.05 and logFC <1 for downregulated genes and logFC >1 for upregulated genes (Figure 2). Table 2 shows the expression profiles of the microarray datasets.
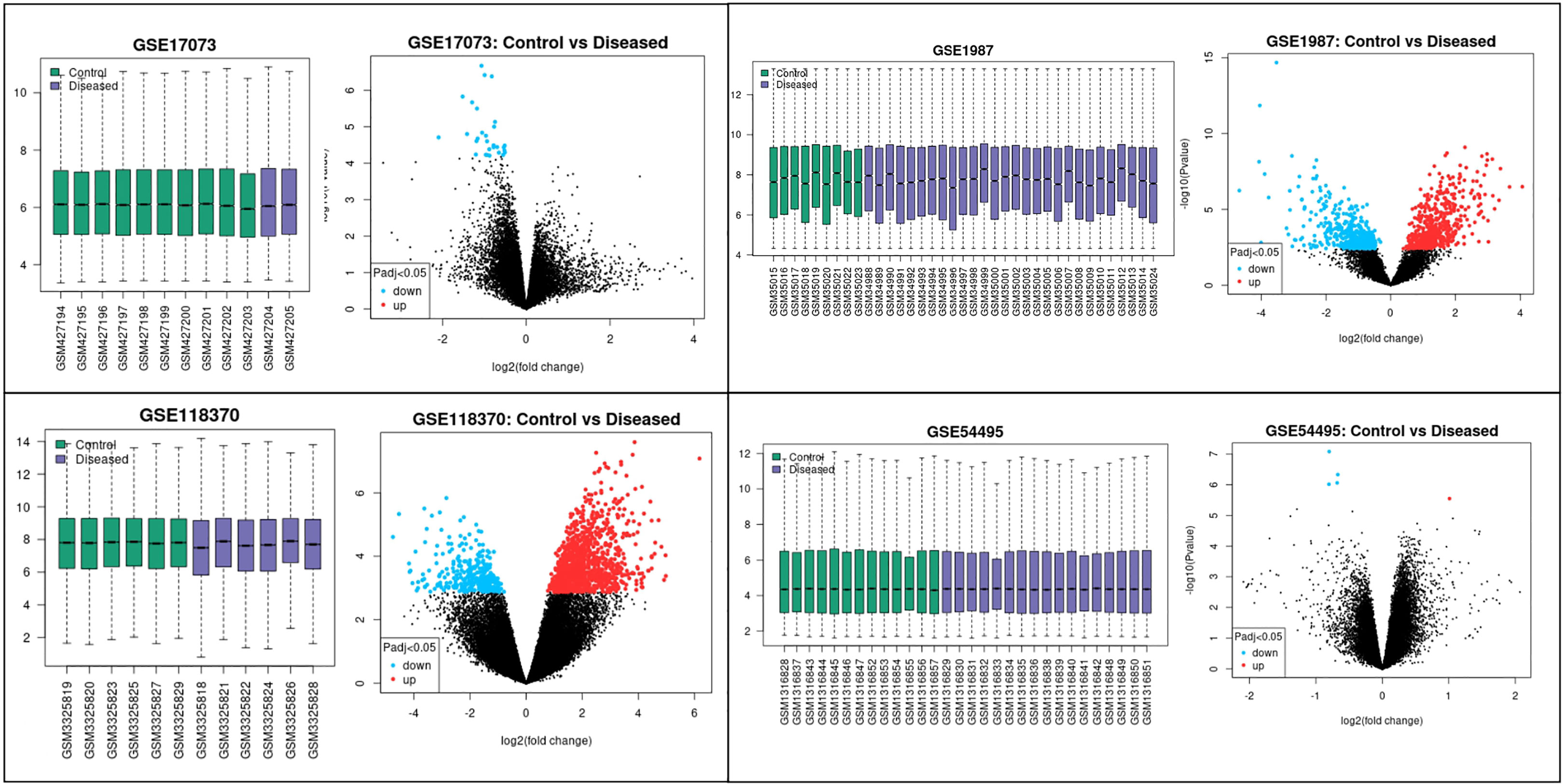
Figure 1 Data accessed from GEO were analyzed in GEO2R. The box plot generated by R represents the normalization of the data obtained after the log transformation. The volcano plot visualizes the DEGs by displaying the statistical significance –log10 p- value versus the log2 fold change. The significantly upregulated and downregulated differentially expressed genes in NSCLC are the highlighted genes in the four datasets. The blue color represents the downregulated genes, while the red color represents the upregulated genes in NSCLC.
Curation of DEGs
From four datasets, we found 10 common DEGs whose gene symbols and biological annotations were retrieved using the David tool. D isease-gene curation was performed by text mining with the help of PubMed, PMC, PMIM, and MeSH (Supplementary Table 1). It was observed that the DEGs DOCK4, ID2, GATA3, SASH1, GJA4, TBX2, HBEF, NPR1, CD24, and DDR1 were the most curated terms in the databases. The mapping of these genes by cancer genetics was performed to further analyze their role in carcinogenesis (Figure 3).
Enrichment analysis of DEGs
The enrichment analysis of DEGs showed that these genes were significantly linked to the regulation of epithelial cell differentiation, ear development, mammary gland alveolus development, regulation of cardiac contraction, cellular senescence, negative regulation of transcription, DNA-templated synthesis, positive regulation of endothelial cell migration, positive regulation of smooth muscle cell proliferation, response to estrogen, and cell chemotaxis (Table 3). The clinical phenotypes associated with the GATA3 gene were n ephrosis and renal agenesis. Vaginal agenesis, septate vagina, uterine agenesis, and uterus didelphys are rarely associated with this gene (Figure 4). The transcriptome analysis revealed expressive transcription factors encoded by these genes, such as LMO2, ELF2, TBX5, PPARG, and FOXC1 (Figure 4).
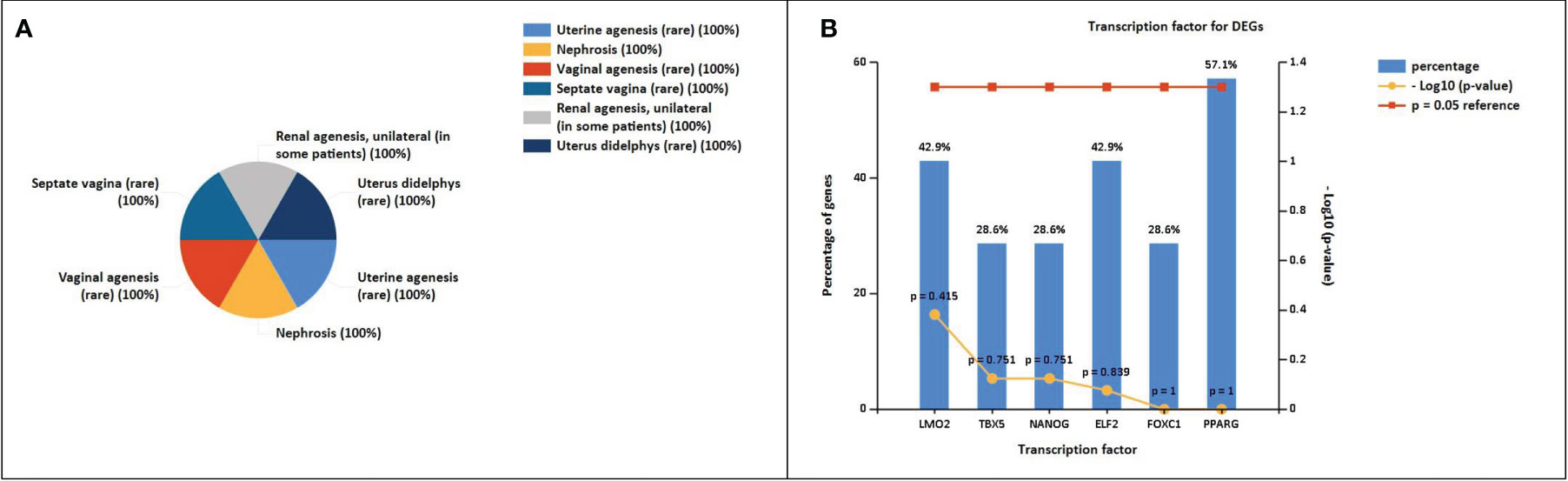
Figure 4 (A) Clinical phenotypes associated with NSCLC-related DEGs using the FunRich tool. (B) Transcription factors identified for NSCLC DEGs using the FunRich tool (p<0.05).
Mutational analysis
Dock4 consists of 30 post-translational modification (PTM) sites having 305 recurrent cancer mutations on the 7- chromosome number negative strand encoding 1966 protein residues with 22.69% predicted disordered regions. The mutation visualization plot shows the DOCK4 isoform mutation at position 378 with the reference amino acid residue V compared to the mutated amino acid residue L, which is enriched for phosphorylation-type mutations, showing the distal mutation PTM impact with the affected site. At position 1770, the L amino acid residue that was enriched with the phosphorylation-type mutation showed proximal mutation. The PTM impact with affected sites were 1769S. SASH1 showed a 68.48% predicted disordered region with 239 mutations observed on chromosome number 6 on the positive strand. 247 protein residues were encoded and 63 PTM sites were observed. At positions 370 and 421, the reference G and R amino acid residues were compared with W and C mutated amino acid residues showing distal PTM mutation impact with affected sites 374S and 417S, respectively. The affected sites at positions 925, 1231, and 1008 have P, L, and S residue sites enriched with a phosphorylation-type mutation affecting PTMs showing a network-rewiring motif gain mutation with mutated amino acid residue R, respectively. A proximal PTM impact at the 837S and 839S affected sites at position 841 was observed with reference amino acid residue D compared to the mutated Y amino acid residue. Similarly, the mutational analysis of GJA4 showed 48.95% of the sequence predicted for disease pathophysiology. A total of 64 mutations were found on the positive strand of chromosome number 1 for GJA4. The number of PTM sites was one, with 333 amino acid residues. In protein HBEGF, 21 mutations were observed on the negative strand of chromosome number 5 encoding 208 protein residues with 47.12% predicted disordered regions (Figure 5).
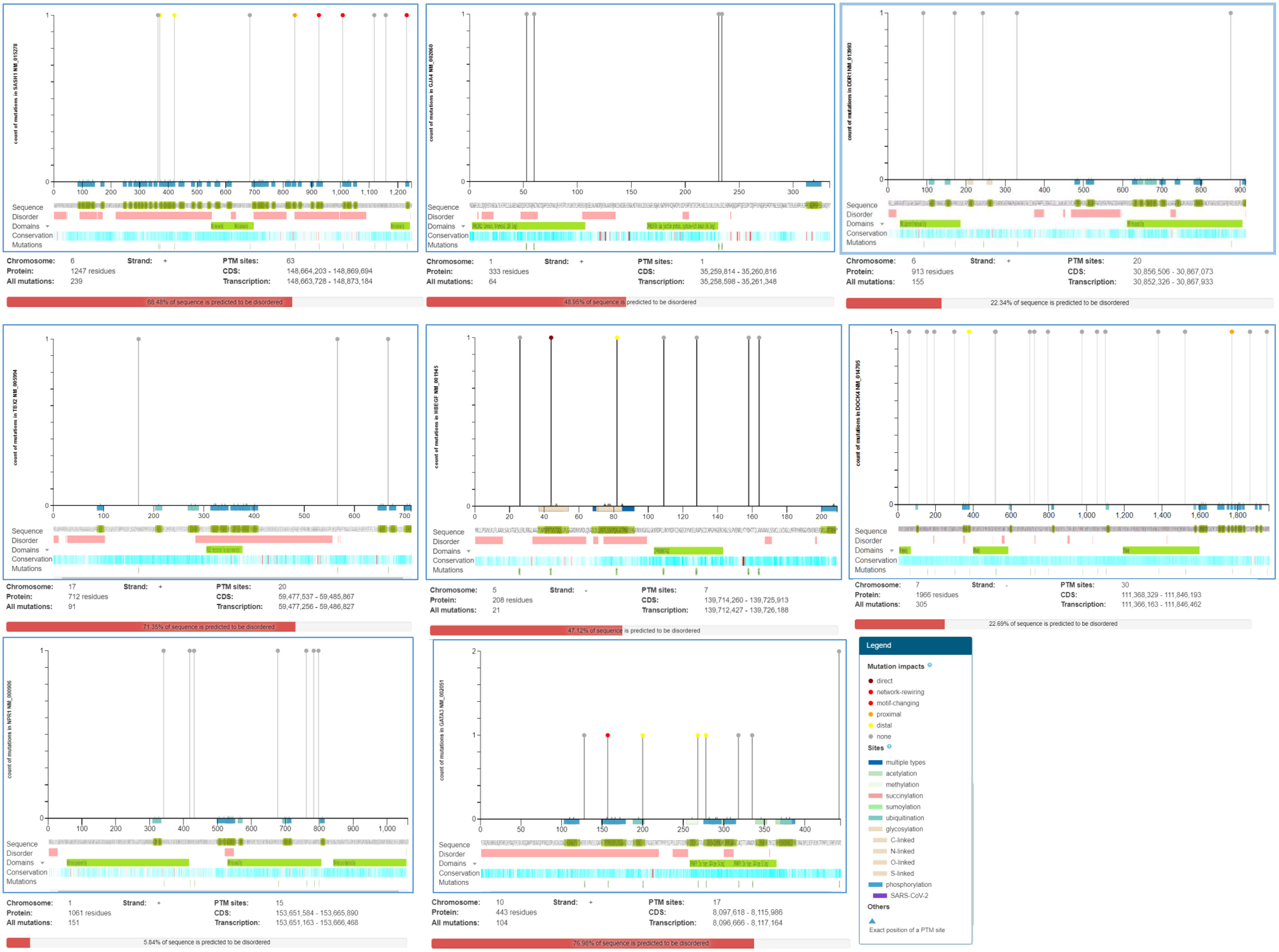
Figure 5 ActiveDriverDB database showing mutations impacting post-translational modification (PTM) sites in proteins. Needle plots indicate PTM site mutations in the identified DEGs. The x-axis indicates the position of the amino acid sequence, while the y-axis shows the number of mutation s. The shading of the x-axis reveals the type of PTM associated with the mutation site.
The mutational analysis of NPR1 showed 15 PTM-affected sites with 151 mutations on the positive strand of chromosome number 1 encoding 1061 protein residues, representing 5.84% of predicted disordered regions. GATA3 showed 76.98% disordered regions with 104 mutations on the positive strand of chromosome number 10 encoding 443 protein residues. In position 157, reference amino acid P showed network rewiring motif loss PTM impacts at affected sites 156S and 162S compared with mutated amino acid T. In positions 278, 268, and 200, reference amino acids G, A, and H and mutated amino acids S, E, and Q showed distal PTM impact enriched with phosphorylation, methylation, and ubiquitination-type mutations, respectively. Similarly, the number of PTM sites for DDR1 was 20, with 913 protein residues having 22.34% of the predicted sequence for the disordered region. A total of 155 mutations were found on the positive strand of chromosome number 6 for DDR1 (Figure 5). Eight isoforms of DDR1 were found (Supplementary Table 2).
Protein -protein network analysis
The STRING database was used to retrieve the related nodes and edges of all NSCLC- associated DEGs (Figure 6). The PPI enrichment p-value was 0.012 with 15 nodes and 17 edges. Figure 6B shows the upregulated and downregulated oncogenes and their associated genes, allowing us to evaluate their biological functions.
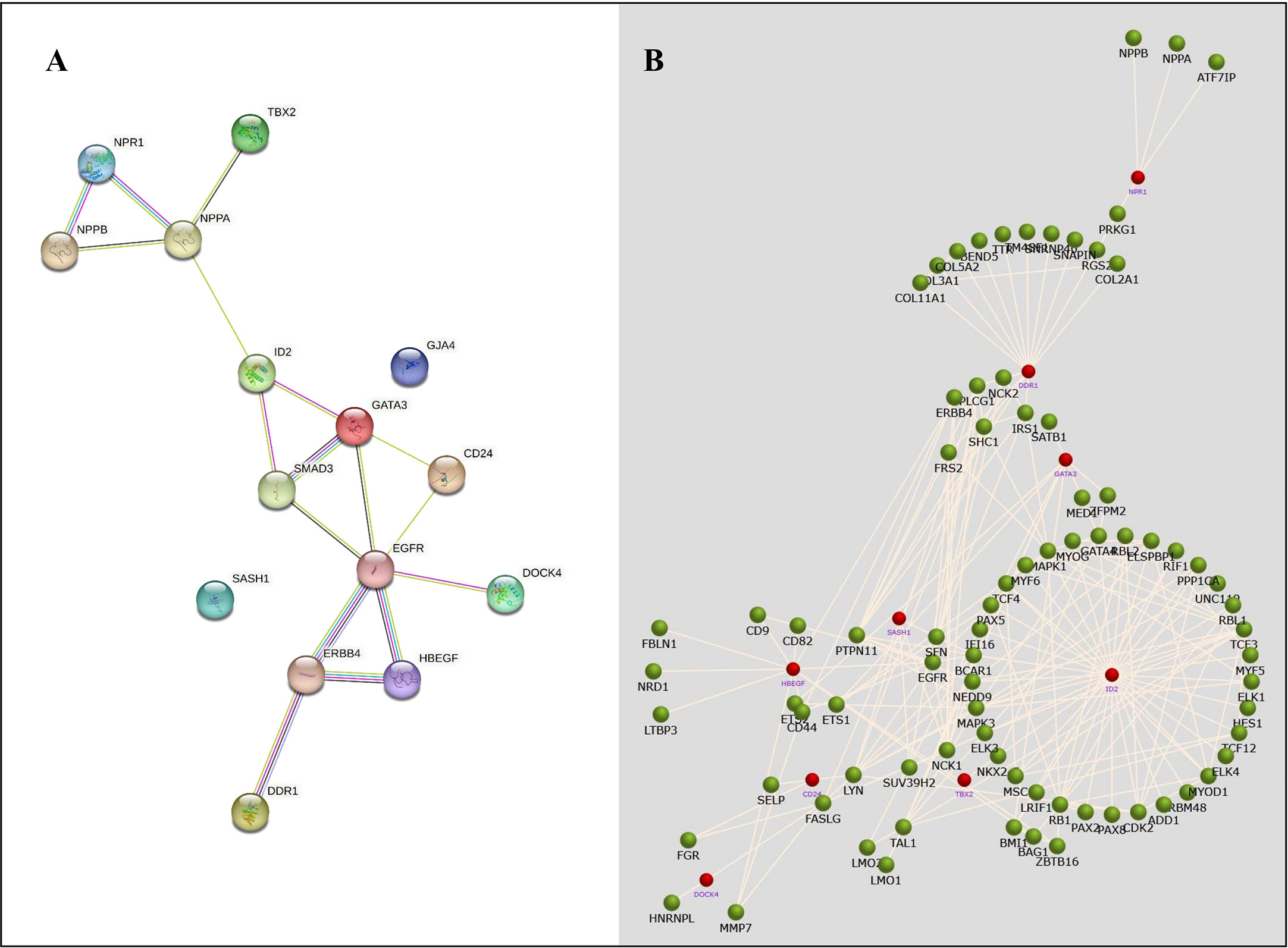
Figure 6 The protein-protein network analysis for identified NSCLC DEGs. (A) A network obtained from the STRING database shows a confidence score of 0.9. (B) A network obtained from the FunRich tool shows the association of several genes with NSCLC-related genes.
Validation of shortlisted DEGs
The Cancer Genome Atlas (TCGA) and the GEPIA databases were employed to further validate our findings. The GEPIA NSCLC data showed that the expressions of these identified DEGs were significantly different between the normal and tumor tissues (Figure 7). The trend was the same as observed in our data, which is consistent with the GEO analysis. Moreover, the Human Protein Atlas database, which showed deregulation of the expression of these seven genes, was used to obtain their immunohistochemistry staining data (Figure 8). E xpression of the hub genes HBEGF, GJA4, DDR1, CD24, TBX2, GATA3, and SASH1 did not appear to be prognostic in lung cancer. No pathological data were found for DOCK4, NPR1, or ID2 genes.
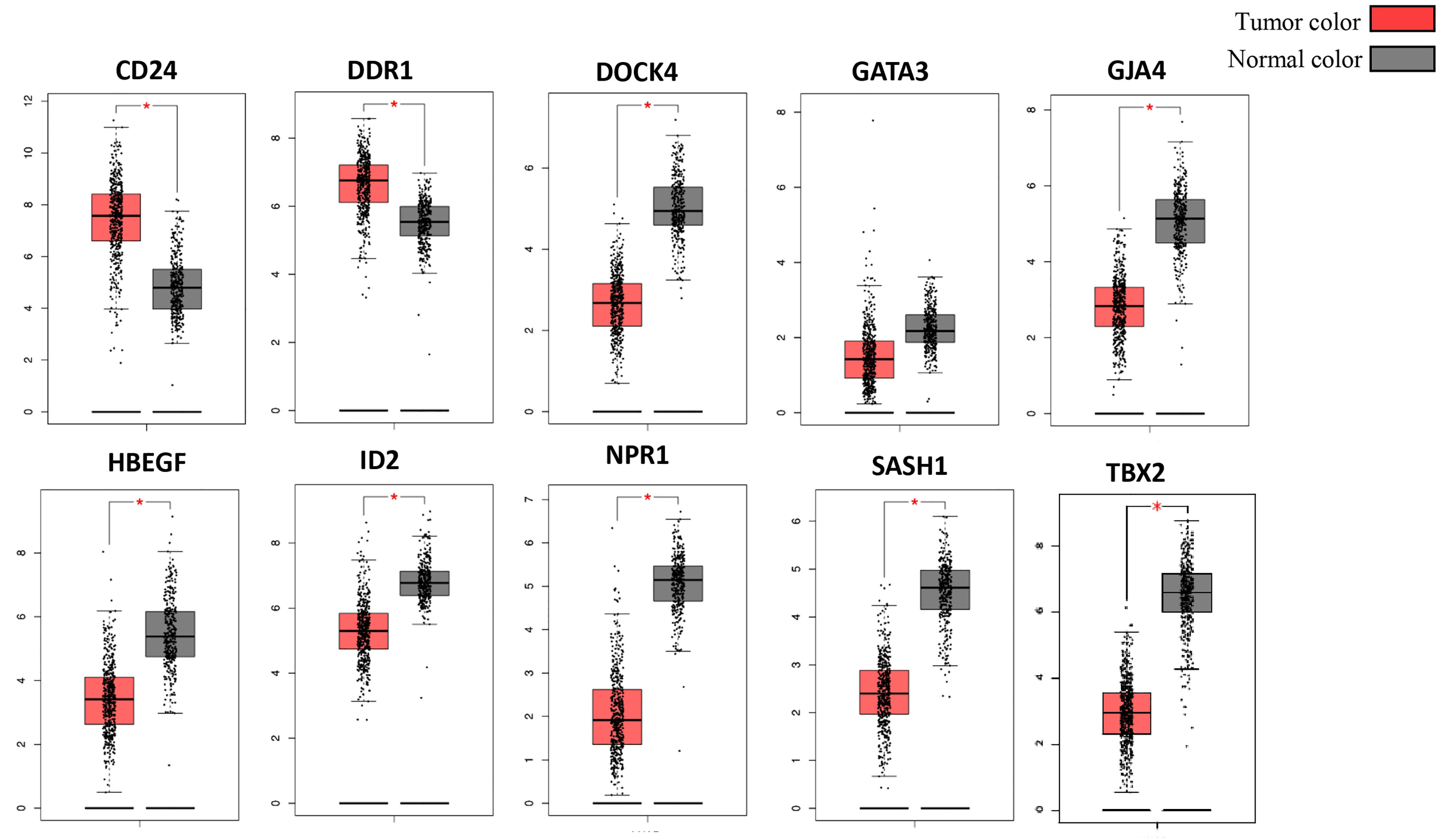
Figure 7 Validation of identified DEGs in the Cancer Genome Atlas (TCGA) database. The box plot shows the expression of genes in mRNA using data from the TCGA database in GEPIA. The method for differential analysis is one-way ANOVA, using disease state. The data was consistent with our study, and their p-values <0.05. The * indicates the difference in gene expression between normal and diseased tissues.
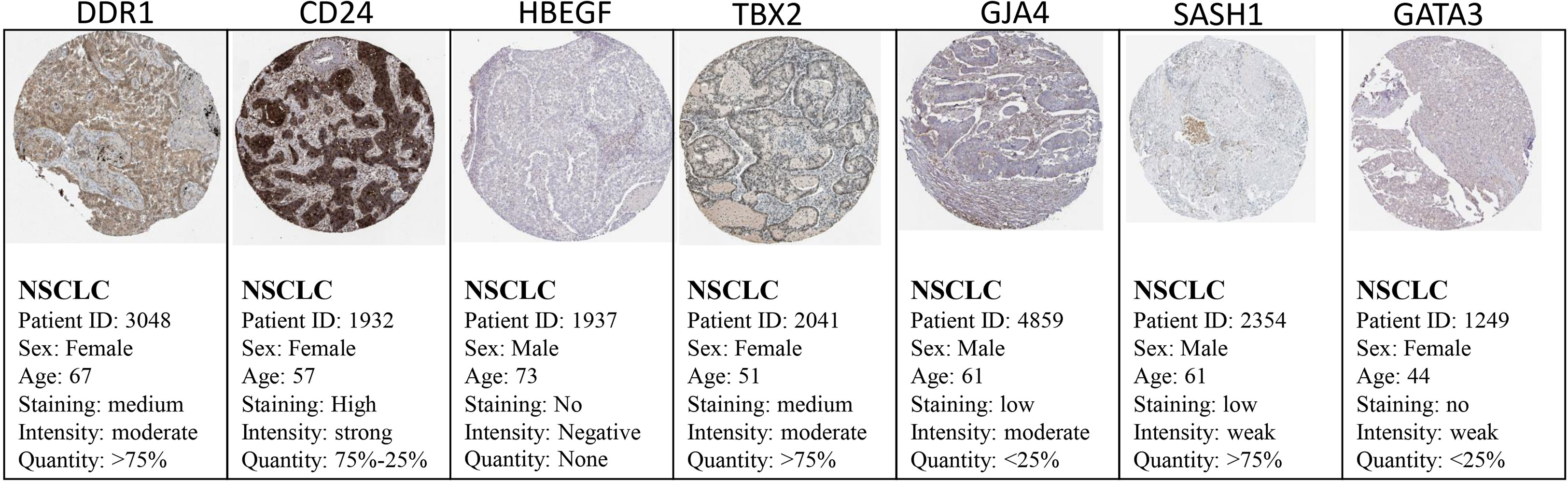
Figure 8 Validation of the identified DEGs at the translational level using the Human Protein Atlas database. The seed genes showed expression in the tissues of NSCLC patients.
Toxicogenomic analysis
The chemical-genotype-phenotype exposome data that may lead to disease progression were explored with the help of toxicogenomic analysis. The effect of different environmental chemicals on the activity and expression of NSCLC- associated DEGs was curated. The data revealed the effects of several chemicals on the increase or decrease of the expression of these NSCLC DEGs on gene activity at different levels (Figure 9). It was also revealed that the co-treatment expression leading to disease occurrence and the same chemical exposure showed different reactivity for different genes. For example, arsenic trioxide decreases the expression of GJA4, but it affects the binding of GATA3. The details of the effect of these chemicals on NSCLC genes are shown in Table 4.
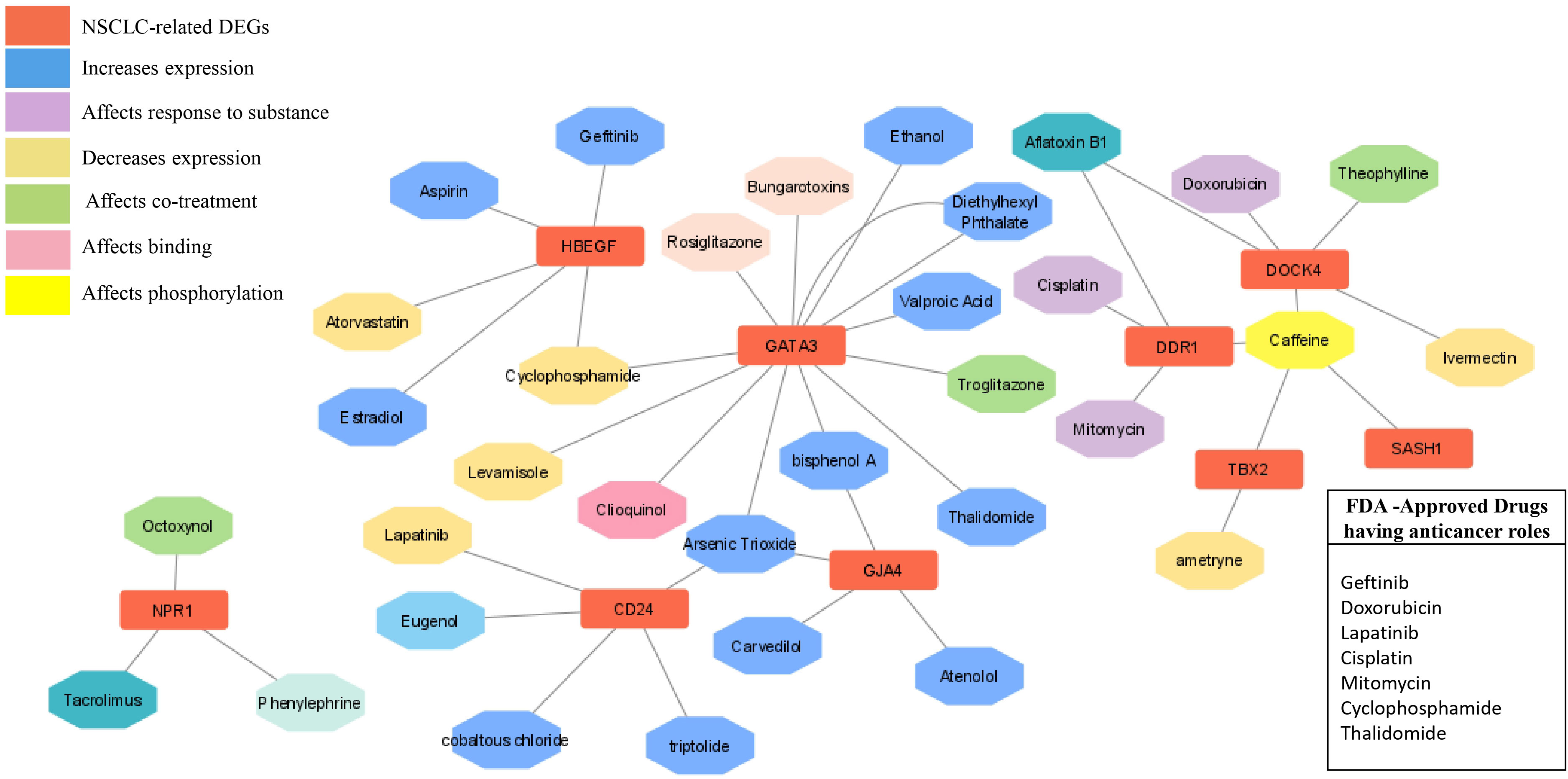
Figure 9 Drug- gene network analysis. The network shows the interaction of important drugs with the identified differentially expressed genes in NSCLC. The red color represents the NSCLC -related DEGs, and their interaction types, such as increased expression after responding to a substance, decreased expression, co-treatment, binding, and phosphorylation are represented by different colors.
miRNA target prediction
The miRNA targets were predicted with the help of the miRDB database based on the algorithms. The miRNA targets such as hsa-miR-302c-5p, hsa-miR-4531, hsa-miR-11181-5p, hsa-miR-4476, hsa-miR-338-5p, hsa-miR-194-5p, hsa-miR-4279, hsa-miR-4742-3p, hsa-miR-4530, and hsa-miR-199a-5p were predicted for DOCK4, SASH1, ID2, GJA4, TBX2, HBEGF, NPR1, GATA3, and CD24, respectively. The onset and progression of the disease may be caused by the deregulation of these genes. Table 5 shows the predicted scores, the total number of miRNA hits, and the seed location of the DEGs. The functional enrichment analysis of these miRNA targets was performed using the FunRich tool. The analysis revealed some important biological pathways associated with these targets, such as the PDGF receptor signaling network, the ErbB receptor signaling network, the glypican signaling pathway, the TRAIL signaling pathway, and the plasma membrane estrogen receptor signaling pathway (Figure 10). The sites of expression for these miRNA targets analyzed were brain (51.1%), placenta (68.6%), ovary (56.2%), kidney (65.1%), heart (38.5%), skeletal muscles (58.5%), and lung (62.9%), with p<0.001.
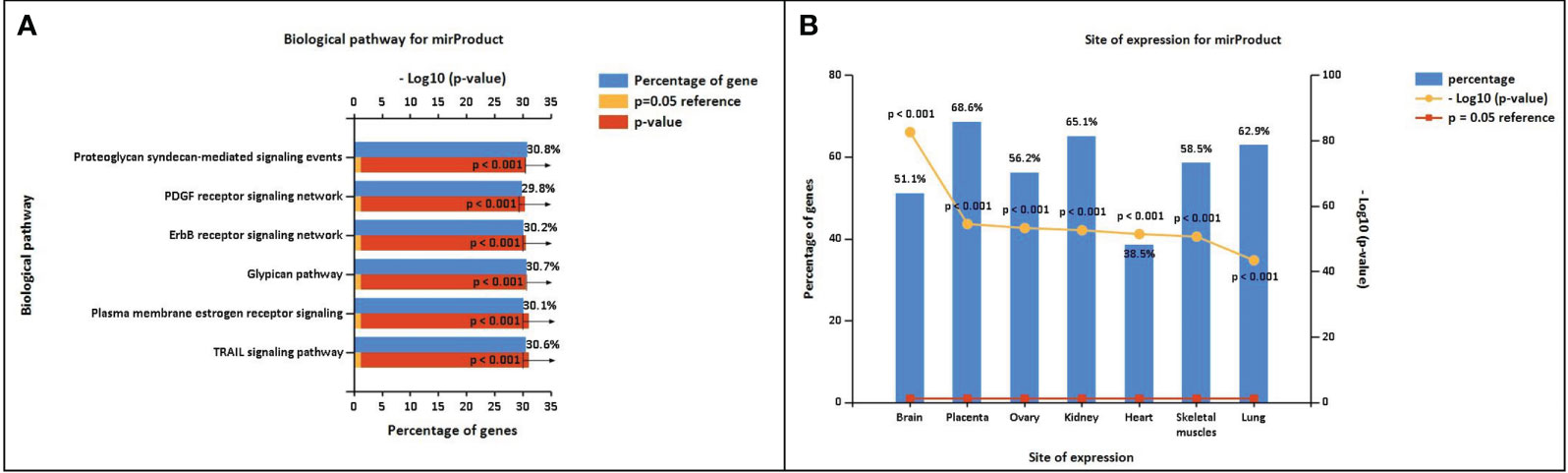
Figure 10 (A) Biological pathway associated with the miRNA predicted targets. (B) Site of expression of mirProduct (p<0.05) showing significant expression in the lungs.
Drug-gene network analysis
In order to explore the available treatment options for NSCLC, a toxicogenomic analysis was performed. The anti-cancer drugs gefitinib, doxorubicin, lapatinib, cisplatin, mitomycin, cyclophosphamide, and thalidomide showed interactions with the hub genes using the CTD database. Several other FDA- approved drugs also showed interactions with the seed genes, such as theophylline, rosiglitazone, aspirin, estradiol, phenylephrine, atorvastatin, and valproic acid. These drugs may serve as novel targets for these genes in the treatment of NSCLC (Figure 9).
Discussion
The study is based on the evaluation of the genetic expression of identified NSCLC DEGs and the understanding of the functional enrichment of their genetic variants. The differential expression analysis revealed 10 significant NSCLC- associated genes (DOCK4, SASH1, ID2, GJA4, TBX2, HBEGF, NPR1, GATA3, and CD24) and were considered the seed genes. The differential expression of the cDNA datasets between cases and controls was explored at the cellular level in lung tissues, and a possible association of these genes was observed in NSCLC cancer. Microarray studies can help in acquiring further information regarding the mechanisms of human genetic disorders.
The protein-protein network analysis revealed that these hub genes have an important association with the disease. These genes showed interaction with several important genes that have a role in NSCLC development, such as, EGFR (27), ERBB4 (28–30), SMAD3 (31–33), and NPPA (34), showing potential cross-talk between these genes in the progression of NSCLC. The hub genes showed important roles in TGF-beta, Rap1, ErbB, and GnRH signaling pathways and hematopoietic cell lineages. T ranscriptome analysis revealed expressive transcription factors encoded by these genes, such as LMO2, ELF2, TBX5, PPARG, and FOXC1. The dysregulation of miRNAs in these genes leads to disease progression and onset, as they are involved in the regulation of post-transcriptional and translational events (35, 36). Therefore, target prediction of miRNAs to aid in functional annotation is crucial (37, 38).
The expression profiling was validated using different databases, such as GEPIA and the Human Protein Atlas, which confirmed the expression of these genes in the diseased state by experimental analysis. The box plot obtained from GEPIA showed an obvious difference in the expression of these genes in the control and disease states. The large-scale characterization of human genomes has become possible through DNA sequencing studies, with different types of genetic variants, such as single nucleotide variants (SNVs) and copy number variations being revealed using this approach. The major challenge in current biomedical research is to determine the association of genotype with phenotypic characteristics, the molecular mechanisms underlying a disease state, the mutations underlying a cancerous state, or any disease variants (39, 40). Several projects are now available that have a large catalog of genetic variants, such as the Cancer Genome Atlas (TCGA), the International Cancer Genome Consortium (ICGC), and others that provide information about thousands of individual and tumor genomes. Post -translational modification (PTM) involves molecular switches of more than 400 amino acid chemical modifications that expand the functional repositories of proteins (31, 41). Almost 400,000 human protein sites are experimentally determined to act as PTM sites, which include phosphorylation, acetylation, ubiquitination, and methylation (42, 43). These PTM sites are helpful in personalized therapies for cancer and are good drug target sites as they aid in the interpretation of genetic variants, the association of genotype and phenotype, and the underlying molecular mechanisms of disease (44, 45). Our study revealed the PTM sites of the hub genes that might be involved in the progression of NSCLC. Moreover, the immunohistochemistry data also showed the expression of these genes in the pathological condition of lung cancer. SASH1 (SAM and SH3 domain-containing protein 1) has a major role in cellular processes such as apoptosis and cellular proliferation. It acts as a tumor suppressor protein. D ifferential expression analysis revealed that SASH1 is downregulated in NSCLC, which may be a factor leading to cancer progression. Burgess et al. (46) studied the association of low SASH1 mRNA expression with poor survival in adenocarcinoma. Their results showed that the compounds that increase the expression level of SASH1 could be used as a novel approach to treating NSCLC, which warrants further studies (46, 47). The expression of HBEGF and its role in lung cancer have been studied by several scientists. HBEGF (heparin-binding EGF-like growth factor) belongs to the EGF family of growth factors and acts as an EGFR ligand. It is more potent than EGF in inducing cellular proliferation and migration. HBEGF has been shown to be upregulated in several cancers, including lung cancer. The gene generates signals for differentiation, migration, proliferation, and cell survival by binding to and over- activating the EGFR pathway (38, 48, 49). Our analysis also revealed overexpression of this gene in NSCLC, which could serve as a potential therapeutic target for NSCLC. GJA4 is a gap junction (GJ) protein also known as Cx37. GJs are involved in intracellular communication through junctions and have an important role in homeostasis. The disruption of GJs results in pathological states, most commonly carcinogenesis (50, 51). The transmembrane proteins, connexins, form the gap junctions. Cxs can serve as tumor suppressors or tumor promoters depending on the stage and type of cancer (52).
DOCK4 belongs to the DOCK180 family, which has diverse cell- specific functions and plays an important role in the metastasis of various tumors such as breast cancer, melanoma, and glioblastoma. The proteins function by engaging in various protein-protein interactions. Yu et al. (53) investigated the role of DOCK4 in the prometastatic effects of TGF-b in lung adenocarcinoma. Their study revealed that TGF-b induced rapid expression of DOCK4 in a Smad-dependent manner. The induction of DOCK4 proved crucial in TGF-B-driven lung adenocarcinoma metastasis (53). TBX2 (T-box) plays a pivotal role in embryonic development and the control of cell cycle progression and carcinogenesis. It has also been implicated in several cancers, including melanoma, pancreatic cancer, and breast cancer. Although studies have shown the role of TBX2 as a tumor suppressor gene (54, 55), there is some evidence correlating the overexpression of TBX2 in NSCLC. TBX2 upregulation was found to be upregulated in NSCLC, making it an important prognostic marker in NSCLC (56). The DDR gene belongs to a novel class of receptor tyrosine kinases and has a potential role in cancer invasion. Evidence suggests that upregulation of DDR1 in NSCLC contributes to progression and poor prognosis, resulting in increased invasiveness (57, 58). CD24, a ligand for P-selectin, has been shown to contribute to the metastatic capacity of CD24- expressing cells (59). Kristiansen et al. demonstrated the expression of CD24 in NSCLC is an independent prognostic tumor marker, underscoring its importance in the metastatic progression of cancer (60). Several pieces of evidence have supported the role of hub genes in the progression and development of NSCLC. The study provides a better understanding of the genetic variations of the genes involved in cancer progression, in addition to their interaction with other proteins in the development of this disease.
D rug-gene network analysis has proven to be essential not only for understanding disease pathophysiology but also for the identification of new drug targets in drug design. The network has identified several potential candidate drugs that have shown associations with these genes.
Conclusions
The study aids in sorting out disease-specific genetic variants from cDNA datasets using a network-based system- level approach. Several complex phenotypic mechanisms, such as cellular replication, apoptosis, mitotic division, and protein signaling, could be understood using this comprehensive and effective method. We have found significant genes (SASH1, TBX2, HBEGF, etc.) linked to NSCLC cancer that can serve as potential drug targets. Potential interactions of these genes with other essential genes leading to cell cycle progression and apoptosis, causing carcinogenesis, have been found. These results can unravel the possible mechanisms of NSCLC cancer progression and occurrence.
Data availability statement
The datasets presented in this study can be found in online repositories. The names of the repository/repositories and accession number(s) can be found in the article/Supplementary Material.
Author contributions
RA organized the database, RA and UI helped in manuscript writing and editing. AM and MS contributed to conception and design of study. All authors contributed to manuscript revision, read, and approved the submitted version.
Conflict of interest
The authors declare that the research was conducted in the absence of any commercial or financial relationships that could be construed as a potential conflict of interest.
Publisher’s note
All claims expressed in this article are solely those of the authors and do not necessarily represent those of their affiliated organizations, or those of the publisher, the editors and the reviewers. Any product that may be evaluated in this article, or claim that may be made by its manufacturer, is not guaranteed or endorsed by the publisher.
Supplementary material
The Supplementary Material for this article can be found online at: https://www.frontiersin.org/articles/10.3389/fonc.2023.1206768/full#supplementary-material
References
1. Miller KD, Nogueira L, Mariotto AB, Rowland JH, Yabroff KR, Alfano CM, et al. Cancer treatment and survivorship statistics, 2019. CA: Cancer J Clin (2019) 69(5):363–85. doi: 10.3322/caac.21565
2. Heigener DF, Reck M. Giant steps and stumbling blocks. Nat Rev Clin Oncol (2018) 15(2):71–2. doi: 10.1038/nrclinonc.2017.178
3. Altaf R, Jadoon SS, Muhammad SA, Ilyas U, Duan Y. Recent advances in immune checkpoint inhibitors for non-small lung cancer treatment. Front Oncol (2022) 12:1014156. doi: 10.3389/fonc.2022.1014156
4. Fathi Z, Syn NL, Zhou J-G, Roudi R. Molecular epidemiology of lung cancer in Iran: implications for drug development and cancer prevention. J Hum Genet (2018) 63(7):783–94. doi: 10.1038/s10038-018-0450-y
5. Wu L, Zhong Y, Yu X, Wu D, Xu P, Lv L, et al. Selective poly adenylation predicts the efficacy of immunotherapy in patients with lung adenocarcinoma by multiple omics research. Anti-Cancer Drugs (2022) 33(9):943–59. doi: 10.1097/CAD.0000000000001319
6. Herbst RS, Morgensztern D, Boshoff C. The biology and management of non-small cell lung cancer. Nature (2018) 553(7689):446–54. doi: 10.1038/nature25183
7. Altaf R, Nadeem H, Babar MM, Ilyas U, Muhammad SA. Genome-scale meta-analysis of breast cancer datasets identifies promising targets for drug development. J Biol Res (2021) 28(1):5. doi: 10.1186/s40709-021-00136-7
8. Altaf R, Nadeem H, Iqbal MN, Ilyas U, Ashraf Z, Imran M, et al. Synthesis, biological evaluation, 2D-QSAR, and molecular simulation studies of dihydropyrimidinone derivatives as alkaline phosphatase inhibitors. ACS omega. (2022) 7(8):7139–54. doi: 10.1021/acsomega.1c06833
9. Altaf R, Nadeem H, Ilyas U, Iqbal J, Paracha RZ, Zafar H, et al. Cytotoxic evaluation, molecular docking, and 2D-QSAR studies of dihydropyrimidinone derivatives as potential anticancer agents. J Oncol (2022) 2022:7715689. doi: 10.1155/2022/7715689
10. Ilyas U, Zaman SU, Altaf R, Nadeem H, Muhammad SA. Genome wide meta-analysis of cDNA datasets reveals new target gene signatures of colorectal cancer based on systems biology approach. J Biol Res (2020) 27:8. doi: 10.1186/s40709-020-00118-1
11. Ilyas U, Naz S, Altaf R, Nadeem H, Muhammad SA, Faheem M, et al. Design, synthesis and biological evaluations of 2-aminothiazole scaffold containing amino acid moieties as anti-cancer agents. Pakistan J Pharm Sci (2021) 34:1509–17.
12. Ilyas U, Altaf R, Aun Muhammad S, Qadir MI, Nadeem H, Ahmed S. Computational drug designing of newly synthesized triazoles against potential targets of methicillin resistant staphylococcus aureus. Pakistan J Pharm Sci (2017) 30(6):2271–9.
13. Huang T, Nazir B, Altaf R, Zang B, Zafar H, Paiva-Santos AC, et al. A meta-analysis of genome-wide gene expression differences identifies promising targets for type 2 diabetes mellitus. Front endocrinology. (2022) 13:985857. doi: 10.3389/fendo.2022.985857
14. Afzaal H, Altaf R, Ilyas U, Zaman SU, Hamdani SDA, Khan S, et al. Virtual screening and drug repositioning of FDA-approved drugs from the ZINC database to identify the potential hTERT inhibitors. Front Pharmacol (2022) 13. doi: 10.3389/fphar.2022.1048691
15. Rao MS, Van Vleet TR, Ciurlionis R, Buck WR, Mittelstadt SW, Blomme EA, et al. Comparison of RNA-seq and microarray gene expression platforms for the toxicogenomic evaluation of liver from short-term rat toxicity studies. Front Genet (2019) 9:636. doi: 10.3389/fgene.2018.00636
16. Yang X, Zhu S, Li L, Zhang L, Xian S, Wang Y, et al. Identification of differentially expressed genes and signaling pathways in ovarian cancer by integrated bioinformatics analysis. OncoTargets Ther (2018) 11:1457–74. doi: 10.2147/OTT.S152238
17. Krassowski M, Paczkowska M, Cullion K, Huang T, Dzneladze I, Ouellette BFF, et al. ActiveDriverDB: human disease mutations and genome variation in post-translational modification sites of proteins. Nucleic Acids Res (2018) 46(D1):D901–D10. doi: 10.1093/nar/gkx973
18. Rachlin J, Cohen DD, Cantor C, Kasif S. Biological context networks: a mosaic view of the interactome. Mol Syst Biol (2006) 2(1):66. doi: 10.1038/msb4100103
19. Mering Cv, Huynen M, Jaeggi D, Schmidt S, Bork P, Snel B. STRING: a database of predicted functional associations between proteins. Nucleic Acids Res (2003) 31(1):258–61. doi: 10.1093/nar/gkg034
20. Cline MS, Smoot M, Cerami E, Kuchinsky A, Landys N, Workman C, et al. Integration of biological networks and gene expression data using cytoscape. Nat Protoc (2007) 2(10):2366–82. doi: 10.1038/nprot.2007.324
21. Tang Z, Li C, Kang B, Gao G, Li C, Zhang Z. GEPIA: a web server for cancer and normal gene expression profiling and interactive analyses. Nucleic Acids Res (2017) 45(W1):W98–W102. doi: 10.1093/nar/gkx247
22. Davis AP, Grondin CJ, Johnson RJ, Sciaky D, McMorran R, Wiegers J, et al. The comparative toxicogenomics database: update 2019. Nucleic Acids Res (2019) 47(D1):D948–D54. doi: 10.1093/nar/gky868
23. Alshalalfa M, Alhajj R. Using context-specific effect of miRNAs to identify functional associations between miRNAs and gene signatures. BMC Bioinf (2013) 14(12):1–13. doi: 10.1186/1471-2105-14-S12-S1
24. Ambros V. The functions of animal microRNAs. Nature (2004) 431(7006):350–5. doi: 10.1038/nature02871
25. Chen Y, Wang X. miRDB: an online database for prediction of functional microRNA targets. Nucleic Acids Res (2020) 48(D1):D127–D31. doi: 10.1093/nar/gkz757
26. Liu W, Wang X. Prediction of functional microRNA targets by integrative modeling of microRNA binding and target expression data. Genome Biol (2019) 20:1–10. doi: 10.1186/s13059-019-1629-z
27. Bethune G, Bethune D, Ridgway N, Xu Z. Epidermal growth factor receptor (EGFR) in lung cancer: an overview and update. J Thorac Dis (2010) 2(1):48.
28. Kurppa KJ, Denessiouk K, Johnson MS, Elenius K. Activating ERBB4 mutations in non-small cell lung cancer. Oncogene (2016) 35(10):1283–91. doi: 10.1038/onc.2015.185
29. Hu X, Xu H, Xue Q, Wen R, Jiao W, Tian K. The role of ERBB4 mutations in the prognosis of advanced non-small cell lung cancer treated with immune checkpoint inhibitors. Mol Med (2021) 27(1):126. doi: 10.1186/s10020-021-00387-z
30. Starr A, Greif J, Vexler A, Ashkenazy-Voghera M, Gladesh V, Rubin C, et al. ErbB4 increases the proliferation potential of human lung cancer cells and its blockage can be used as a target for anti-cancer therapy. Int J cancer. (2006) 119(2):269–74. doi: 10.1002/ijc.21818
31. Marwitz S, Ballesteros-Merino C, Jensen SM, Reck M, Kugler C, Perner S, et al. Phosphorylation of SMAD3 in immune cells predicts survival of patients with early stage non-small cell lung cancer. J Immunotherapy Cancer (2021) 9(2):e001469. doi: 10.1136/jitc-2020-001469
32. Qian Z, Zhang Q, Hu Y, Zhang T, Li J, Liu Z, et al. Investigating the mechanism by which SMAD3 induces PAX6 transcription to promote the development of non-small cell lung cancer. Respir Res (2018) 19:1–11. doi: 10.1186/s12931-018-0948-z
33. Chung JY-F, Tang PC-T, Chan MK-K, Xue VW, Huang X-R, Ng CS-H, et al. Smad3 is essential for polarization of tumor-associated neutrophils in non-small cell lung carcinoma. Nat Commun (2023) 14(1):1794. doi: 10.1038/s41467-023-37515-8
34. Lu D, Luo P, Zhang J, Ye Y, Wang Q, Li M, et al. Patient−derived tumor xenografts of lung squamous cell carcinoma alter long non−coding RNA profile but not responsiveness to cisplatin. Oncol Letters. (2018) 15(6):8589–603. doi: 10.3892/ol.2018.8401
35. Lim LP, Lau NC, Garrett-Engele P, Grimson A, Schelter JM, Castle J, et al. Microarray analysis shows that some microRNAs downregulate large numbers of target mRNAs. Nature (2005) 433(7027):769–73. doi: 10.1038/nature03315
36. Baek D, Villén J, Shin C, Camargo FD, Gygi SP, Bartel DP. The impact of microRNAs on protein output. Nature (2008) 455(7209):64–71. doi: 10.1038/nature07242
37. Wong N, Wang X. miRDB: an online resource for microRNA target prediction and functional annotations. Nucleic Acids Res (2015) 43(D1):D146–D52. doi: 10.1093/nar/gku1104
38. Wang L, Lu Y-F, Wang C-S, Xie Y-X, Zhao Y-Q, Qian Y-C, et al. HB-EGF activates the EGFR/HIF-1α pathway to induce proliferation of arsenic-transformed cells and tumor growth. Front Oncol (2020) 10:1019. doi: 10.3389/fonc.2020.01019
39. Gonzalez-Perez A, Mustonen V, Reva B, Ritchie G, Creixell P, Karchin R, et al. International cancer genome consortium mutation p, consequences subgroup of the bioinformatics analyses working G: computational approaches to identify functional genetic variants in cancer genomes. Nat Methods (2013) 10:723–9. doi: 10.1038/nmeth.2562
40. MacArthur D, Manolio T, Dimmock D, Rehm H, Shendure J, Abecasis G, et al. Guidelines for investigating causality of sequence variants in human disease. Nature (2014) 508(7497):469–76. doi: 10.1038/nature13127
41. Mann M, Jensen ON. Proteomic analysis of post-translational modifications. Nat Biotechnol (2003) 21(3):255–61. doi: 10.1038/nbt0303-255
42. Hornbeck PV, Kornhauser JM, Tkachev S, Zhang B, Skrzypek E, Murray B, et al. PhosphoSitePlus: a comprehensive resource for investigating the structure and function of experimentally determined post-translational modifications in man and mouse. Nucleic Acids Res (2012) 40(D1):D261–D70. doi: 10.1093/nar/gkr1122
43. Prasad K, Goel R, Kandasamy K, Keerthikumar S, Kumar S, Mathivanan S, et al. Human protein reference database. Nucleic Acids Res (2009) 37:767–72. doi: 10.1007/978-1-60761-232-2_6
44. Hoeller D, Dikic I. Targeting the ubiquitin system in cancer therapy. Nature (2009) 458(7237):438–44. doi: 10.1038/nature07960
45. Jones PA, Issa J-PJ, Baylin S. Targeting the cancer epigenome for therapy. Nat Rev Genet (2016) 17(10):630–41. doi: 10.1038/nrg.2016.93
46. Burgess JT, Bolderson E, Adams MN, Duijf PHG, Zhang S-D, Gray SG, et al. SASH1 is a prognostic indicator and potential therapeutic target in non-small cell lung cancer. Sci Rep (2020) 10(1):18605. doi: 10.1038/s41598-020-75625-1
47. Chen E-g, Chen Y, Dong L-l, Zhang J-s. Effects of SASH1 on lung cancer cell proliferation, apoptosis, and invasion in vitro. Tumor Biol (2012) 33:1393–401. doi: 10.1007/s13277-012-0387-2
48. Yotsumoto F, Fukagawa S, Miyata K, Nam SO, Katsuda T, Miyahara D, et al. HB-EGF is a promising therapeutic target for lung cancer with secondary mutation of EGFRT790M. Anticancer Res (2017) 37(7):3825–31. doi: 10.21873/anticanres.11761
49. Van Hiep N, Sun W-L, Feng P-H, Lin C-W, Chen K-Y, Luo C-S, et al. Heparin binding epidermal growth factor–like growth factor is a prognostic marker correlated with levels of macrophages infiltrated in lung adenocarcinoma. Front Oncol (2022) 12. doi: 10.3389/fonc.2022.963896
50. Aasen T, Mesnil M, Naus CC, Lampe PD, Laird DW. Gap junctions and cancer: communicating for 50 years. Nat Rev Cancer. (2016) 16(12):775–88. doi: 10.1038/nrc.2016.105
51. Siebert AP, Ma Z, Grevet JD, Demuro A, Parker I, Foskett JK. Structural and functional similarities of calcium homeostasis modulator 1 (CALHM1) ion channel with connexins, pannexins, and innexins. J Biol Chem (2013) 288(9):6140–53. doi: 10.1074/jbc.M112.409789
52. Luo K-J, Chen C-X, Yang J-P, Huang Y-C, Cardenas ER, Jiang JX. Connexins in lung cancer and brain metastasis. Front Oncol (2020) 10:599383. doi: 10.3389/fonc.2020.599383
53. Yu J-R, Tai Y, Jin Y, Hammell MC, Wilkinson JE, Roe J-S, et al. TGF-β/Smad signaling through DOCK4 facilitates lung adenocarcinoma metastasis. Genes Dev (2015) 29(3):250–61. doi: 10.1101/gad.248963.114
54. Khalil AA, Sivakumar S, San Lucas FA, McDowell T, Lang W, Tabata K, et al. TBX2 subfamily suppression in lung cancer pathogenesis: a high-potential marker for early detection. Oncotarget (2017) 8(40):68230. doi: 10.18632/oncotarget.19938
55. Nehme E, Rahal Z, Sinjab A, Khalil A, Chami H, Nemer G, et al. Epigenetic suppression of the T-box subfamily 2 (TBX2) in human non-small cell lung cancer. Int J Mol Sci (2019) 20(5):1159. doi: 10.3390/ijms20051159
56. Hu B, Mu H-P, Zhang Y-Q, Su C-Y, Song J-T, Meng C, et al. Prognostic significance of TBX2 expression in non-small cell lung cancer. J Mol Histol. (2014) 45(4):421–6. doi: 10.1007/s10735-014-9569-0
57. Yang SH, Baek HA, Lee HJ, Park HS, Jang KY, Kang MJ, et al. Discoidin domain receptor 1 is associated with poor prognosis of non-small cell lung carcinomas. Oncol Rep (2010) 24(2):311–9. doi: 10.3892/or_00000861
58. Miao L, Zhu S, Wang Y, Li Y, Ding J, Dai J, et al. Discoidin domain receptor 1 is associated with poor prognosis of non-small cell lung cancer and promotes cell invasion via epithelial-to-mesenchymal transition. Med Oncol (2013) 30:1–9. doi: 10.1007/s12032-013-0626-4
59. Lee HJ, Choe G, Jheon S, Sung S-W, Lee C-T, Chung J-H. CD24, a novel cancer biomarker, predicting disease-free survival of non-small cell lung carcinomas: a retrospective study of prognostic factor analysis from the viewpoint of forthcoming (seventh) new TNM classification. J Thorac Oncol (2010) 5(5):649–57. doi: 10.1097/JTO.0b013e3181d5e554
Keywords: NSCLC, mutational analysis, differentially expressed genes, microarray, bioinformatics
Citation: Altaf R, Ilyas U, Ma A and Shi M (2023) Identification and validation of differentially expressed genes for targeted therapy in NSCLC using integrated bioinformatics analysis. Front. Oncol. 13:1206768. doi: 10.3389/fonc.2023.1206768
Received: 16 April 2023; Accepted: 15 May 2023;
Published: 31 May 2023.
Edited by:
Liyun Shi, Nanjing University of Chinese Medicine, ChinaReviewed by:
Muhammad Tariq Khan, Capital University of Science & Technology, PakistanOmer Farooq, Shifa Tameer-e-Millat University, Pakistan
Anjali Chauhan, University of Florida, United States
Copyright © 2023 Altaf, Ilyas, Ma and Shi. This is an open-access article distributed under the terms of the Creative Commons Attribution License (CC BY). The use, distribution or reproduction in other forums is permitted, provided the original author(s) and the copyright owner(s) are credited and that the original publication in this journal is cited, in accordance with accepted academic practice. No use, distribution or reproduction is permitted which does not comply with these terms.
*Correspondence: Meiqi Shi, c2hpbWVpcWkxOTYzQDE2My5jb20=