- 1Dalian Medical University, Dalian, China
- 2The Dandong Central Hospital, Dandong, China
- 3The Second Hospital of Dalian Medical University, Dalian, China
- 4Medical College of Wuhan University of Science and Technology, Wuhan, China
Background: Cancer is a leading cause of death, especially among women, with cancers like breast, ovarian, and cervical cancer presenting unique diagnostic and treatment challenges. Systemic inflammation plays a significant role in cancer progression, affecting both tumor development and therapeutic outcomes. Despite the established link between inflammation and cancer, comprehensive studies on the prognostic value of the Aggregate Index of Systemic Inflammation (AISI) in female cancer patients are lacking. This study explores the association between AISI and mortality outcomes, including all-cause and cardiovascular mortality, in female cancer patients.
Methods: This study analyzes data from the NHANES database and Dandong Central Hospital. Kaplan-Meier survival curves and multivariable Cox proportional hazards regression analyses were used to assess the relationship between AISI and all-cause and cardiovascular mortality. Restricted cubic spline plots and subgroup analyses were applied to explore potential interactions.
Results: Elevated AISI levels were strongly associated with increased all-cause and cardiovascular mortality. Patients in the highest AISI quartile demonstrated significantly higher mortality risks compared to those in the lowest quartile. ROC curve analysis indicated superior predictive performance of AISI over SII. Restricted cubic spline plots revealed a linear relationship, with mortality risk notably increasing when AISI levels were elevated.
Conclusion: AISI is a robust predictor of all-cause and cardiovascular mortality in female cancer patients. Its ease of measurement and strong prognostic value make it a valuable tool for risk assessment and management in this population.
Introduction
In 2022, nearly 20 million new cancer cases were reported, alongside 9.7 million cancer-related deaths. It is estimated that approximately one in five individuals, regardless of gender, will develop cancer in their lifetime, while roughly one in nine men and one in twelve women are expected to succumb to the disease (1). A recent study highlighted the disproportionate cancer mortality among women, revealing that approximately one million children lost their mothers to cancer in 2020. Nearly half of these maternal deaths were attributed to breast or cervical cancer (2). Among female patients, certain cancers, such as breast, ovarian, and cervical cancers, have higher rates of occurrence. These types of cancer are closely linked to women’s physiological characteristics and hormonal environments, which makes their diagnosis and treatment more complex (3). Therefore, improving cancer treatment strategies to better meet the needs of female patients can reduce the risk of complications and enhance long-term survival outcomes (4, 5).
In recent years, growing evidence has shown that systemic inflammatory responses play a critical role in the onset and progression of cancer (6, 7). Inflammation is involved in various stages of tumor development, including initiation, promotion, malignant transformation, invasion, and metastasis. Furthermore, inflammation has a significant impact on immune surveillance and therapeutic responses (8). AISI has been widely used to explore the link between inflammation and various diseases (9–11). As a systemic inflammation indicator, AISI has emerged as a practical and valuable tool. This biomarker is characterized by ease of collection, rapid results, low cost, and high efficiency and reliability, making it accessible through routine blood tests, offering notable advantages for clinical application. Additionally, the Systemic Immune-Inflammation Index (SII), another immunoinflammatory marker, has been used to predict and evaluate the prognosis of various solid tumors, including gastric cancer, small-cell lung cancer, and ovarian cancer (12–15). However, while AISI has been applied in studies of idiopathic pulmonary fibrosis (IPF), COVID-19, hypertension, and certain cancers (16–19), research on its prognostic value in female cancer patients remains limited.
Against this background, the present study aims to investigate the potential association between AISI, a blood-based inflammatory biomarker, and mortality outcomes (including all-cause mortality and cardiovascular events) in female cancer patients. Additionally, the study seeks to evaluate the comparative advantages of AISI and SII in prognostic assessments, providing clinicians with a novel, convenient, and reliable tool for risk assessment and management strategies for female cancer patients.
The National Health and Nutrition Examination Survey (NHANES) is a nationally representative cross-sectional survey aimed at assessing the health and nutritional status of the U.S. population. Utilizing a large, nationally representative dataset from the NHANES database, this study examines the association between the novel inflammatory biomarker AISI and all-cause as well as cardiovascular mortality in female cancer patients.
Methods
Data source and study population
This study utilized two datasets: one derived from the NHANES database and the other from inpatient data of Dandong Central Hospital. Sample 1 comprises participants from the NHANES database, covering the period from 1999 to 2023. NHANES is organized and administered by the National Center for Health Statistics (NCHS), utilizing a nationally representative, stratified, multistage probability sampling method (20). The database is managed and maintained by NCHS, with all participants providing written informed consent, and the study received approval from the NCHS Institutional Review Board (IRB). Additional information is available at: http://www.cdc.gov/nchs/nhanes/irba98.htm. Given that NHANES is a publicly available dataset containing anonymous data, this study did not require further ethical approval or informed consent. The study adhered to ethical guidelines for the protection of human subjects’ safety and privacy as outlined by relevant authorities and data administrators. The study cohort comprised adult female participants diagnosed with cancer, along with a general population sample. The exclusion criteria were as follows: 1) All male participants and female individuals under the age of 18; 2) participants with missing hematologic laboratory test data on neutrophils (NEU), platelets (PLT), monocytes (MON), or lymphocytes (LYM); 3) individuals lacking covariate data; 4) individuals with incomplete or undisclosed mortality data; and 5) participants without available sample weight data. In total, 2,387 participants were included in the analysis. The participant selection process is depicted in Figure 1.
Sample 2 includes electronic medical record data from female patients diagnosed with cancer at our hospital between January 2022 and December 2023. The dataset records baseline characteristics and laboratory test results at admission. Blood samples were collected, processed, and analyzed following standardized international biochemical laboratory protocols. This study was conducted in accordance with the ethical guidelines outlined in the 1964 Declaration of Helsinki and received approval from the IRB; therefore, written informed consent was not required. The inclusion criteria were as follows: (1) absence of a pathological report confirming cancer diagnosis; (2) incomplete laboratory test or electronic medical record data; (3) presence of underlying diseases that directly affect four key hematological parameters, such as infections, liver cirrhosis, exogenous albumin supplementation, or leukemia; (4) lack of complete follow-up records. Ultimately, 116 participants were included, with the detailed screening process illustrated in Supplementary Figure 1.
Cancer status and mortality
The diagnosis of cancer or malignant neoplasm was based on responses to the following questions from the NHANES questionnaire (MCQ220, MCQ230A, MCQ230B, MCQ230C): 1) Has a doctor or other healthcare professional ever told you that you had cancer or a malignant tumor? 2) If yes, what type of cancer was it?
NHANES data were linked with mortality data from the National Death Index (NDI) through December 31, 2019, which is publicly available. This linkage facilitated the determination of mortality outcomes in the study population. To ensure accurate data matching, a probabilistic matching algorithm was applied. Causes of death were classified according to the International Classification of Diseases, 10th Revision (ICD-10) (21). With the NCHS classifying heart diseases (054–064) and all other causes (010) for our study (21).
AISI definition
AISI was derived using Beckman Coulter’s counting and classification method, based on parameters from a complete blood count (CBC). This method involves automated sample handling, including dilution and mixing, with hemoglobin measurements conducted via a single-beam photometer. White blood cell (WBC) classification was performed using VCS technology. CBC analysis of blood samples was conducted at the NHANES mobile examination center (MEC) using the Beckman Coulter DxH 800 device, which also provided blood cell distribution data. According to previous studies, the AISI formula is calculated as (NEU * PLT * MONO)/LYM (22). Additionally, due to the left-skewed distribution of inflammatory markers observed in regression analysis, a logarithmic (ln) transformation of the AISI was applied.
Covariates
This study identified independent risk factors associated with female cancer and incorporated them as covariates in the analysis. These covariates were selected based on prior research to minimize potential confounding bias. Specifically, the included covariates were age, race, education level, income-to-poverty ratio, body mass index (BMI), smoking status, alcohol consumption, diabetes, and hypertension. Demographic information, including age, race, education level, and income-to-poverty ratio, was collected by trained interviewers using the computer-assisted personal interview (CAPI) system. Anthropometric measurements were obtained by trained health technicians at the NHANES MEC, and BMI was calculated as weight (kg) divided by height (m) squared.
Smoking status was categorized based on participants’ responses to two survey questions: “Have you ever smoked 100 or more cigarettes?” and “Do you currently smoke?” Participants were classified into three groups: never smokers, former smokers, and current smokers. Never smokers were defined as individuals who had never smoked 100 or more cigarettes and were not currently smoking. Current smokers were those who had smoked 100 or more cigarettes and were still smoking at the time of the survey, while former smokers were those who had smoked 100 or more cigarettes in the past but had since quit. Alcohol consumption was categorized according to self-reported drinking frequency into four groups: heavy drinkers, moderate drinkers, light drinkers, and non-drinkers. Heavy drinkers were defined as individuals consuming four or more alcoholic beverages per day, moderate drinkers as those consuming up to three drinks per day, light drinkers as those who had consumed alcohol fewer than 12 times in the past year, and non-drinkers as those who reported never consuming alcohol.
Diabetes and hypertension diagnoses were determined using a combination of questionnaire responses and laboratory data to enhance diagnostic accuracy. The diabetes-related questionnaire included questions such as: “Has a doctor ever diagnosed you with diabetes?” “Do you take insulin?” and “Do you take oral hypoglycemic medications?” Laboratory diagnostic criteria for diabetes were defined as fasting blood glucose levels ≥7.0 mmol/L, HbA1c ≥6.5%, or oral glucose tolerance test (OGTT) blood glucose levels ≥11.1 mmol/L. Similarly, hypertension was diagnosed based on multiple blood pressure measurements ≥130/80 mmHg or a self-reported physician diagnosis.
Statistical analysis
To ensure the sample’s national representativeness, MEC weights from the NHANES sampling design were applied following the NHANES weighting guidelines. Continuous variables were reported as weighted means with their corresponding standard errors, while categorical variables were expressed as frequencies and weighted proportions. The chi-square test was employed to evaluate differences in categorical variables, and the Kruskal-Wallis test was utilized to compare continuous variables across AISI quartiles (Q1–Q4).
Multivariable Cox proportional hazards regression analysis was conducted to investigate the relationship between AISI and mortality, with adjustments made for demographic characteristics (Model 2) and all covariates (Model 3). Hazard ratios (HRs) and 95% confidence intervals (CIs) were calculated to quantify the strength of these associations. Kaplan-Meier survival curves were constructed to visualize survival probabilities across different AISI quartiles, and statistical significance of the differences was assessed.
Additionally, we generated the receiver operating characteristic (ROC) curves for AISI and SII in relation to both all-cause and cardiovascular mortality rates to assess their predictive performance in female cancer patients. To further explore these relationships, we employed restricted cubic spline methods to visually demonstrate the potential linear association between AISI and both all-cause and cardiovascular mortality rates. For a more in-depth analysis, we conducted threshold analysis to examine the association between AISI and mortality rates. Subgroup analysis was performed to assess the potential impact of other variables on these relationships, thereby validating the robustness of our findings. Finally, we implemented mediation analysis to investigate the mediating effect of AISI on the relationship between cancer and both all-cause and cardiovascular mortality in female patients.
All statistical tests were two-sided, with a p-value of less than 0.05 considered indicative of statistical significance. Data analysis was performed using IBM SPSS Statistics version 25.0 and R version 4.3.1.
Results
Study population and baseline characteristics
Between 1999 and 2023, a total of 142,310 participants from the NHANES database were included in this study. After excluding individuals who did not meet the study criteria or had incomplete data, the final cohort comprised 2,387 female cancer patients and 25,109 individuals from the general population (Figure 1). The mean age of participants was 62 ± 15 years. Baseline characteristics are summarized in Table 1. Significant differences were observed across groups in terms of age, race, education level, hypertension, and diabetes according to the quartiles of AISI (p < 0.05). Supplementary Table 1 presents the clinical characteristics of the patients in Sample 2. The study population is predominantly concentrated around the age of 60.
Mortality outcomes
During the 20-year follow-up period, Kaplan-Meier survival curves indicated 678 cases of all-cause mortality and 136 cases of cardiovascular mortality. Higher quartiles of AISI were associated with reduced survival rates, highlighting an independent association between elevated AISI levels and increased all-cause and cardiovascular mortality among adult female cancer patients in the United States (Figure 2).
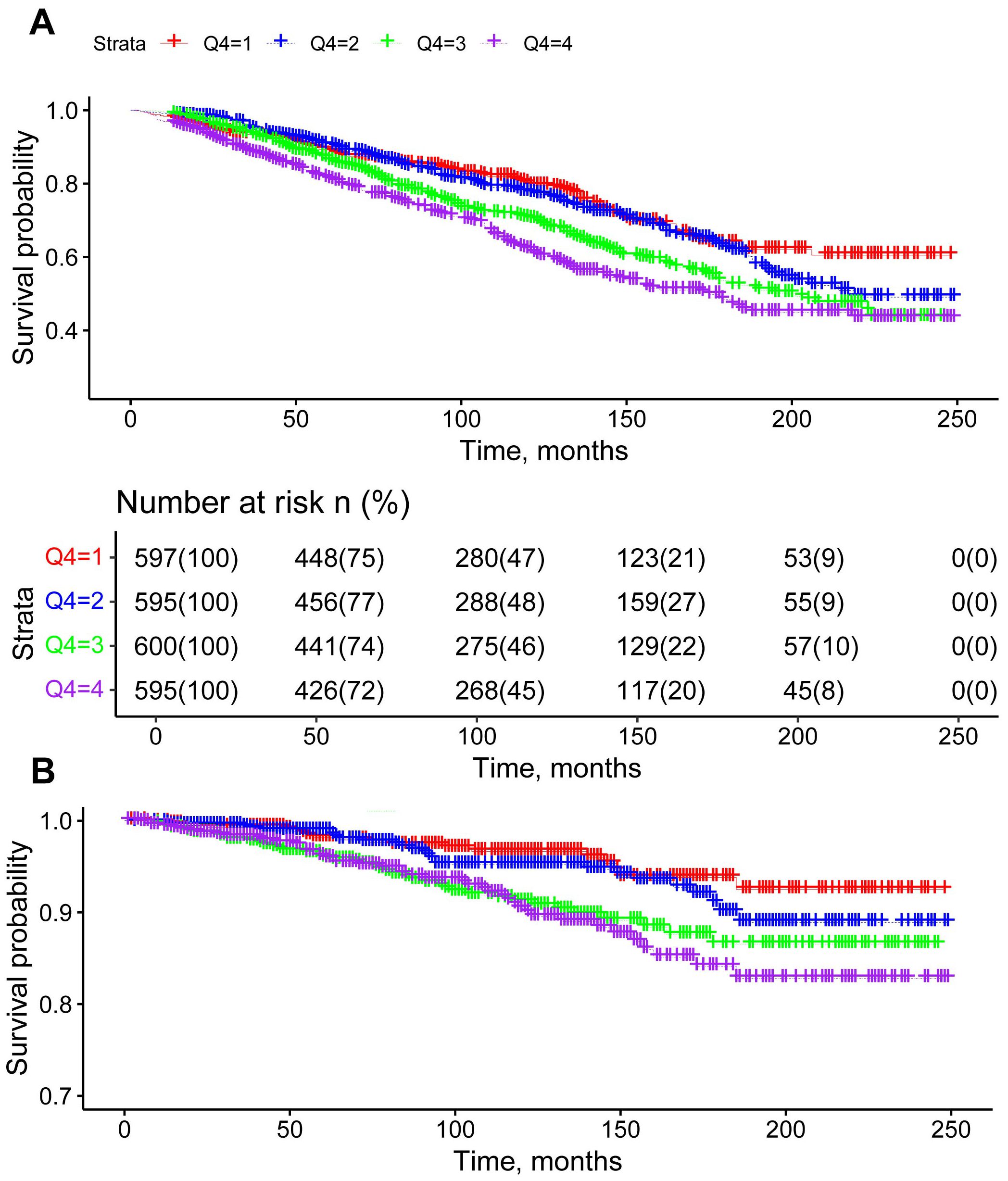
Figure 2. Kaplan-Meier survival curves for all-cause mortality (A) and cardiovascular mortality (B) stratified by AISI quartiles, adjusted for age, race, education, poverty-to-income ratio, hypertension, diabetes, BMI, alcohol use, and smoking.
Comparison of predictive performance: AISI vs. SII
Furthermore, ROC curves were employed to compare the predictive performance of SII and AISI in female cancer patients. As shown in Figure 3, AISI demonstrated superior predictive ability for both all-cause and cardiovascular mortality. Specifically, the area under the curve (AUC) for AISI predicting all-cause mortality was 0.5918, while the AUC for SII was slightly lower at 0.5693. Similarly, for cardiovascular mortality, AISI showed a higher AUC of 0.6030 compared to SII’s AUC of 0.5847. In addition, we calculated the Net Reclassification Improvement (NRI) and Integrated Discrimination Improvement (IDI) to further evaluate the improvement in classification and risk stratification with AISI compared to SII. As shown in Supplementary Table 2, the NRI and IDI analyses confirmed that AISI provided a significantly better reclassification of patients’ mortality risks, particularly in identifying high-risk individuals. The Harrell’s Concordance Index also indicated a higher concordance between AISI’s predictions and actual mortality events, further supporting its superior predictive ability.
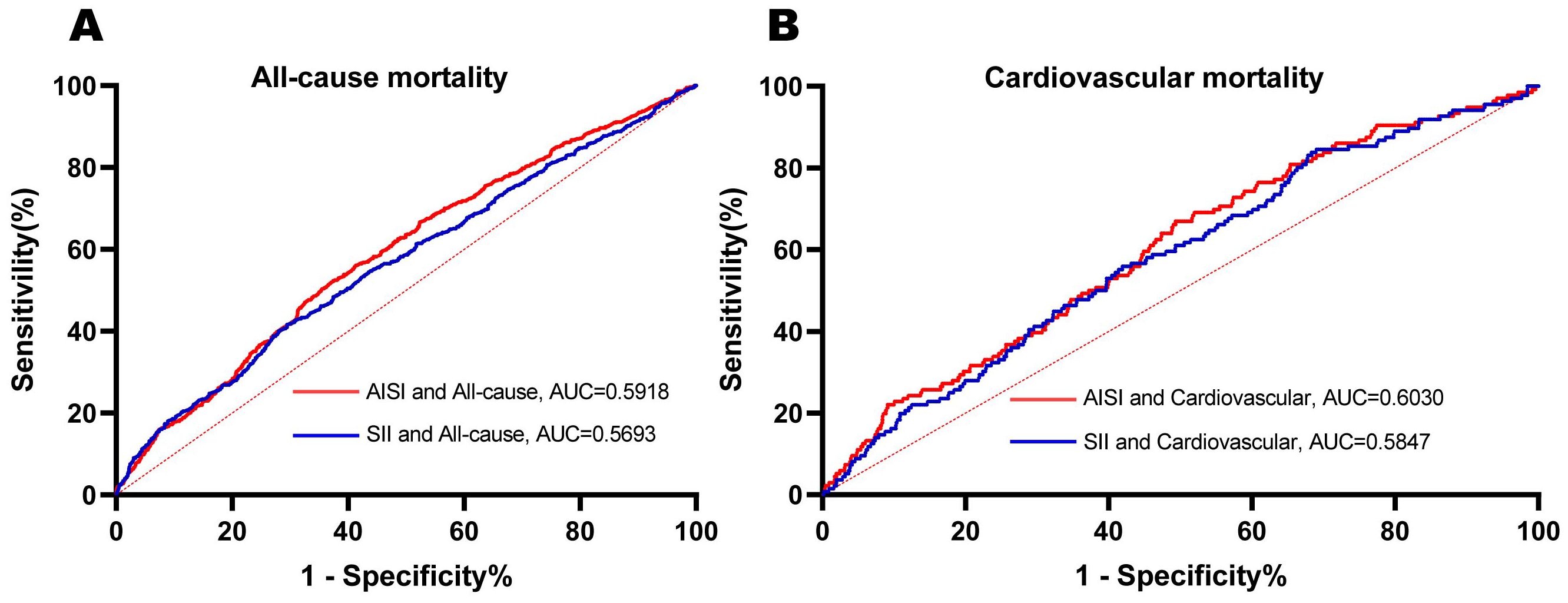
Figure 3. ROC curves were utilized to assess the differences in predictive abilities of SII and AISI for all-cause (A) and cardiovascular (B) mortality in female cancer patients. ROC receiver operating characteristic curve, AUC area under the curve.
Regression analysis of AISI and mortality risk
Proportional hazards regression analysis identified a positive correlation between AISI levels and mortality. This association remained robust after adjusting for various covariates across Models 2 and 3. Specifically, each one-unit increase in AISI was associated with a 35% increase in the risk of all-cause mortality (95% CI: 1.20–1.51) and a 48% increase in the risk of cardiovascular mortality (95% CI: 1.14–1.94). Additionally, the data from Sample 2 yielded similar findings. Cox regression analysis was performed on the Sample 2 population, and the results further demonstrated a positive association between AISI and all-cause mortality in female cancer patients (1.57 [1.12, 2.21]). For detailed results, please refer to Supplementary Table 3. Compared to patients in the lowest AISI quartile (Q1), those in the highest quartile (Q4) exhibited significantly higher risks for both all-cause and cardiovascular mortality. Furthermore, patients in the third AISI quartile (Q3) demonstrated an elevated risk of cardiovascular mortality relative to those in Q1. These findings underscore a strong association between higher AISI quartiles and increased risks of all-cause and cardiovascular mortality. AISI may serve as a valuable indicator for assessing patient mortality risk, particularly for cardiovascular events. The independent positive correlation between AISI, analyzed as both a continuous and categorical variable, and mortality is further supported by detailed effect sizes presented in Table 2.
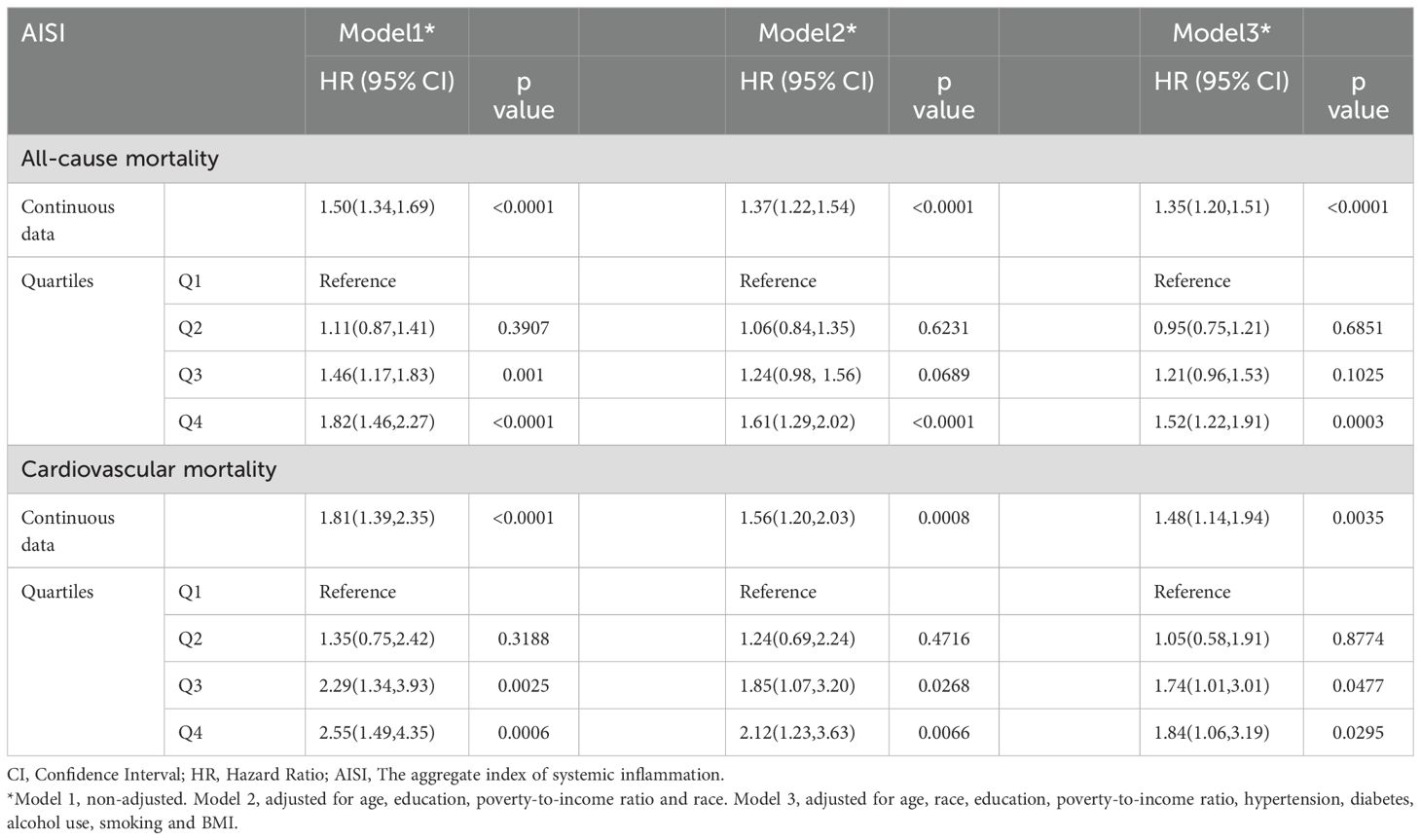
Table 2. HRs (95% CIs) for all-cause and cardiovascular mortality according to different types of AISI data in cancer patients from NHANES (1999–2023) among U.S. Adult females.
Linear relationship between AISI and mortality
Restricted cubic spline plots revealed a clear linear relationship between AISI and both all-cause and cardiovascular mortality (linear P < 0.05, Figure 4). This analysis was adjusted for all covariates. Notably, when AISI reached a value of 5.61, the hazard ratio (HR) for both all-cause and cardiovascular mortality was 1, indicating a threshold at which the risk of mortality transitions from low to high. AISI levels exceeding this threshold were significantly associated with a poorer prognosis among female cancer patients. We also applied RCS curves to the Sample 2 population to visualize the results and determined that an AISI level of 5.6 resulted in an AISI risk ratio equal to 1. (See Supplementary Figure 2 for details).
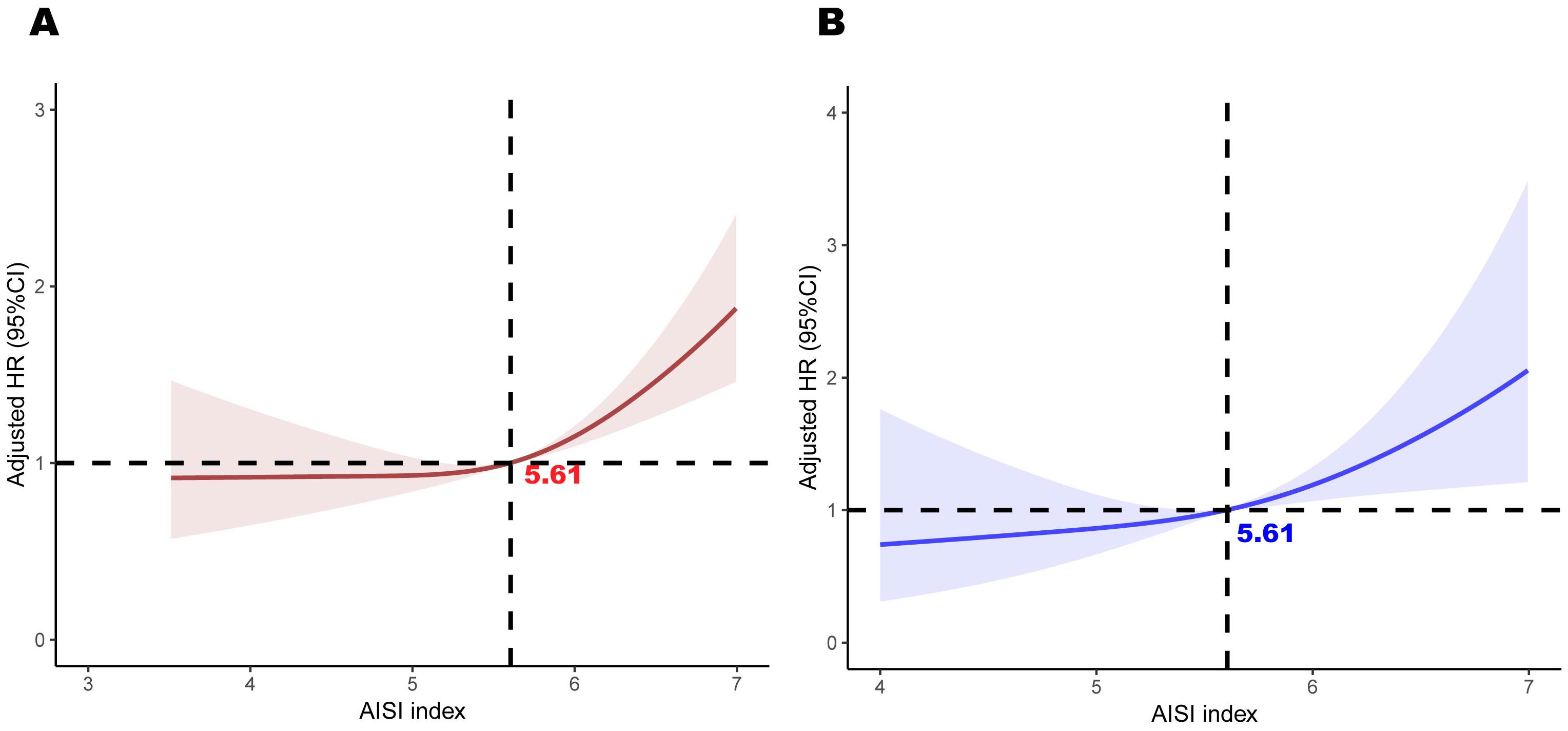
Figure 4. Association between AISI and all-cause mortality (A) and cardiovascular mortality (B) in participants with female cancer, adjusted for age, race, education, poverty-to-income ratio, hypertension, diabetes, BMI, alcohol use, and smoking. The shaded areas represent the 95% CI.
Subgroup analysis
Subgroup analyses were conducted to evaluate the influence of other covariates on the association between AISI and mortality (Figure 5). Hazard ratio (HR) of 1 based on Cox proportional hazards regression analysis was used as the reference point for the threshold. By categorizing AISI into low- and high-risk groups based on the threshold associated with the lowest risk, the analysis revealed that female cancer patients with elevated AISI levels (AISI > 5.61) exhibited a significantly higher risk of all-cause mortality compared to those with lower AISI levels (HR = 1.58, 95% CI: 1.26–1.99). These findings suggest that elevated AISI levels serve as a strong predictor of increased mortality risk.
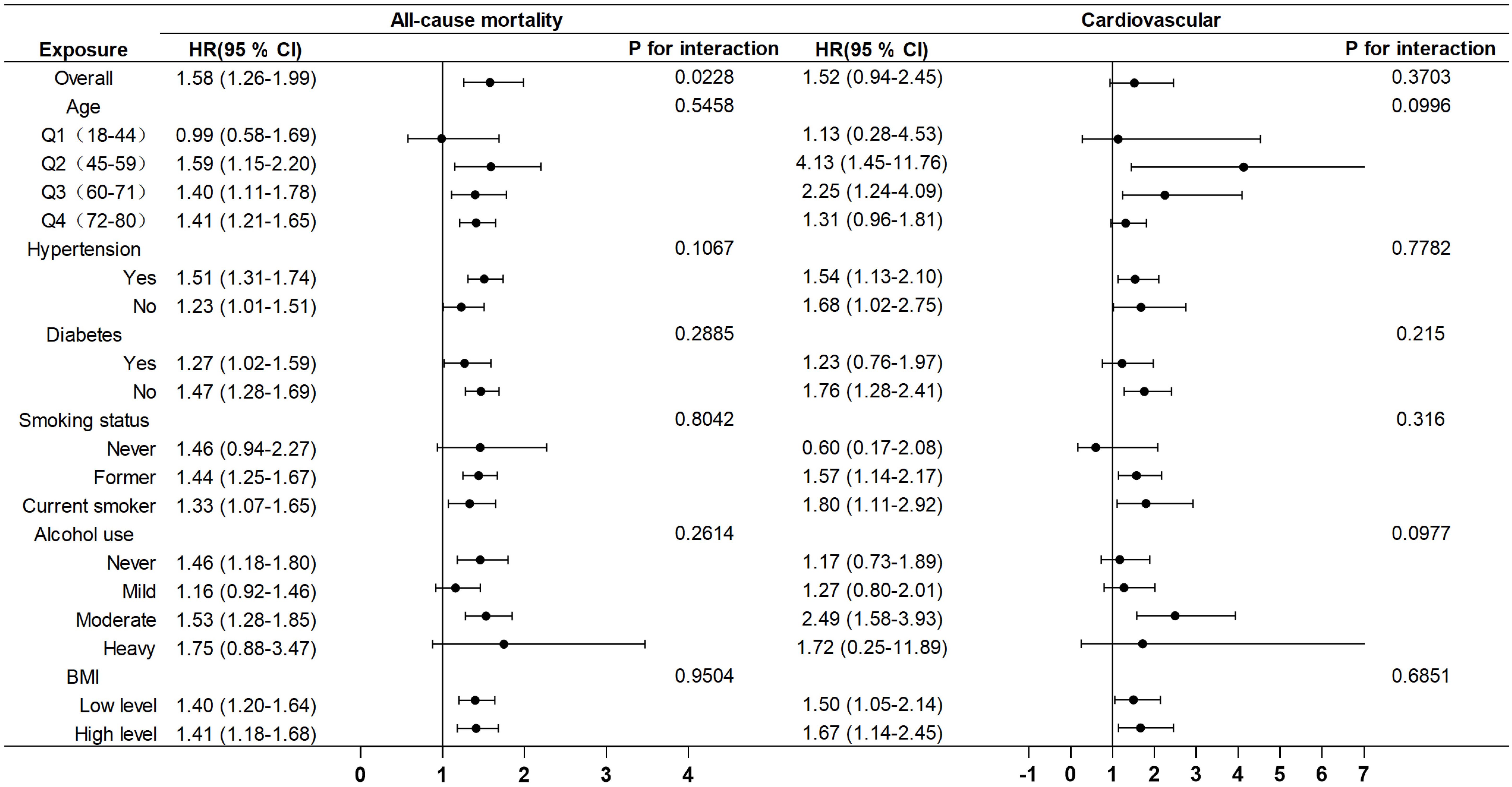
Figure 5. Subgroup analysis of the associations between AISI and all-cause and cardiovascular mortality, adjusted for age, race, education, poverty-to-income ratio, hypertension, diabetes, BMI, alcohol use, and smoking.
Mediation analysis
Finally, the results of the mediation analysis showed that AISI did not play a mediating role in the effect of cancer on all-cause mortality and cardiovascular mortality in female patients (Table 3).
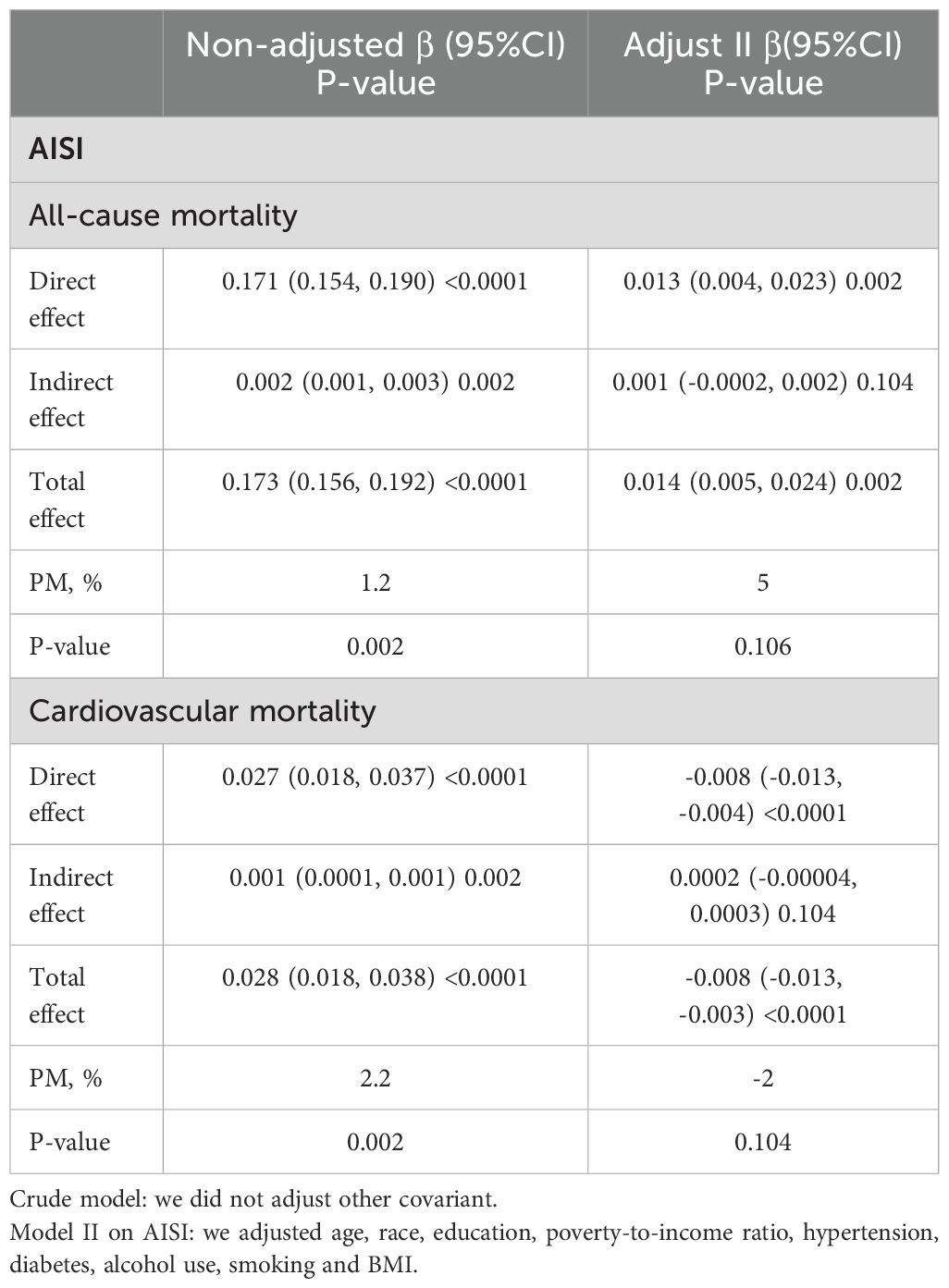
Table 3. Mediation analysis of the association between Female cancer patients and the risk of all cause and cardiovascular mortality mediated by AISI.
Discussion
In this retrospective cohort study, we employed two datasets to investigate the association between AISI and both all-cause and cardiovascular mortality in female cancer patients. The study’s primary finding revealed a robust, positive correlation between AISI—analyzed both as a continuous variable and categorized into quartiles—and mortality outcomes in this population. This relationship persisted even after adjusting for a range of potential confounding factors. Notably, when compared with the previously established SII, AISI exhibited superior predictive accuracy for mortality risk in female cancer patients. Subgroup analyses further underscored that elevated AISI levels were strongly linked to a heightened risk of all-cause mortality. However, in the studied cohort, covariates such as age, hypertension, diabetes, smoking, alcohol consumption, and body mass index did not significantly influence the relationship between AISI and mortality (all P-values > 0.05). Moreover, no significant interactions were observed between AISI and these covariates, indicating that AISI may serve as a robust independent predictor of mortality in this patient population, with minimal confounding from other established risk factors. By addressing a critical research gap, our findings highlight the potential utility of AISI as a valuable prognostic marker for assessing disease severity and clinical outcomes in female cancer patients.
Inflammation significantly contributes to cancer development and progression through multiple biological pathways. Growing evidence underscores a strong association between inflammation and cancer progression, as demonstrated in recent studies (23–26). In the context of female cancers, chronic inflammation and sustained immune activation have been identified as critical factors driving disease progression (27). AISI, a composite biomarker of systemic inflammation, is constructed using key inflammatory components, including neutrophils, platelets, monocytes, and lymphocytes.
Inflammation and immune cells within the tumor microenvironment (TME) play a pivotal role in influencing the growth and progression of cancer cells (28, 29). Among these, neutrophils are the most abundant immune cells in the TME and have a dual role in tumor biology. Elevated neutrophil counts suppress the secretion of tumor necrosis factor-α, which subsequently increases circulating levels of vascular endothelial growth factor (VEGF). The overexpression of VEGF facilitates tumor angiogenesis, thereby accelerating tumor growth and metastasis (30). Moreover, neutrophils secrete various inflammatory mediators, including matrix metalloproteinase-9, neutrophil elastase (NE), and interleukin-8, all of which contribute to tumor proliferation and metastasis (31, 32). Conversely, neutrophils also exhibit antitumor properties by releasing neutrophil extracellular traps (NETs), which mediate tumor cell destruction through components such as histones, NE, and myeloperoxidase (MPO) (33–35). Richardson et al. further demonstrated a correlation between NETs released by activated neutrophils and poor prognosis in patients with colorectal cancer (36).
Neutrophilia is often accompanied by relative lymphocytopenia, highlighting a potential weakening of cell-mediated adaptive immune responses (37). Lymphocytes, as key mediators of immune surveillance and immunoediting, play a critical role in antitumor immunity, with their infiltration into TME serving as a fundamental requirement for effective immune responses against tumors (38). Tumor-infiltrating lymphocytes are strongly associated with improved survival across various cancers, whereas low lymphocyte counts or inadequate infiltration are linked to poorer survival outcomes (39). Specifically, lymphocytopenia has been identified as an independent prognostic factor for both overall survival and progression-free survival in patients with metastatic breast cancer (40). Moreover, interactions between CD8+ cytotoxic T lymphocytes and CD4+ helper T lymphocytes are essential for inducing tumor cell apoptosis via immune-mediated antitumor mechanisms (41). Overall, diminished lymphocyte counts may reflect weak or insufficient tumor-specific immune responses, underscoring the critical importance of lymphocytes in cancer immunology.
Monocytes are widely recognized as protumorigenic cells, serving as major sources of chemokines and cytokines within TME, thereby driving tumor progression and metastasis (42, 43). Tumor-secreted CCL5 and CXCL8 also play roles in recruiting monocytes, neutrophils, and other leukocytes, which can differentiate into TAMs and tumor-associated neutrophils (TANs), both of which can assume pro-tumorigenic roles (44). Furthermore, tumor cells can drive the substantial accumulation of tumor-promoting myeloid immune cells within the tumor microenvironment. For instance, myeloid-derived suppressor cells (MDSCs), tumor-associated macrophages (TAMs), and regulatory T cells have been identified as the predominant tumor-promoting immune cells in the tumor microenvironment (45). Studies, such as those conducted by Bingle et al. (46), have demonstrated that macrophage density is strongly associated with poor clinical prognosis in various solid tumors. Additionally, research has revealed that TAMs promote tumor cell migration, invasion, and angiogenesis while simultaneously suppressing antitumor immune responses, thus facilitating tumor dissemination and metastasis (47, 48). Consistent with these findings, elevated peripheral monocyte levels have been correlated with poor prognoses across multiple cancer types (49–51).
Platelets, beyond their conventional roles in hemostasis and wound healing, are actively involved in all stages of tumor progression. Recent studies highlight the critical role of platelets within TME. Platelets interact with TME components via membrane proteins and secrete cytokines that regulate tumor growth, metastasis, and invasion (52). During hematogenous metastasis, platelets shield tumor cells, enhance their survival, and facilitate immune evasion, thereby promoting ectopic metastasis (53). Additionally, the interaction between tumor cells and platelets triggers platelet activation, elevates platelet counts, and increases the risk of thrombosis in cancer patients (54). Elevated platelet counts have been identified as adverse prognostic indicators across various cancer types (54, 55). Furthermore, Nicholas et al. (56)demonstrated that the heightened cardiovascular risk observed in female cancer patients is influenced by multiple factors, including chronic inflammation, oxidative stress, metabolic dysregulation, clonal hematopoiesis, gut dysbiosis, hormonal alterations, and cellular senescence. These exacerbated immune-inflammatory responses not only accelerate cancer progression but also contribute to irreversible cardiovascular damage, significantly increasing mortality and the incidence of adverse cardiovascular events (57). Thus, mitigating the overactivation of immune-inflammatory pathways may help delay cancer progression and reduce associated cardiovascular risks.
Our study presents several key strengths. First, the cohort analysis is based on 15 cycles of the US NHANES from 1999 to 2023, which utilizes a rich dataset and spans a long survey period, thereby ensuring the robustness and reliability of the results. Second, to further validate the research findings, we utilized clinical data from Dandong Central Hospital as a validation set, thereby enhancing the external validity of the results and improving the generalizability and applicability of the conclusions. Third, Numerous studies have demonstrated that conventional inflammatory markers, such as the neutrophil-lymphocyte ratio (NLR), platelet-lymphocyte ratio (PLR), and C-reactive protein (CRP), possess strong prognostic predictive capabilities in cancer patients (58–63). However, AISI is considered to offer a more comprehensive reflection of the systemic inflammatory status compared to traditional markers, particularly excelling in the prediction of poor prognosis. A study involving patients with IPF found that AISI demonstrated the highest prognostic value among various inflammatory markers, significantly outperforming NLR and PLR (19). This advantage is primarily attributed to AISI’s incorporation of monocyte count, which is more sensitive to inflammatory responses, thereby providing a more accurate assessment of the systemic inflammatory state. Finally, our study is the first to systematically investigate the relationship between the inflammation biomarker AISI and both all-cause and cardiovascular mortality in female cancer patients. Our aim is to identify novel prognostic indicators for the elevated mortality risk in this population, providing clinicians with a new tool for identifying high-risk individuals. Not only that, but we employed multiple analytical approaches, including Cox proportional hazards regression, subgroup analysis, and Kaplan-Meier survival curves, all of which further substantiate the effectiveness of AISI as a reliable predictor of mortality risk in female cancer survivors.
Limitations
While our study provides significant insights, it is important to recognize its limitations. First, the use of cross-sectional laboratory data restricts our ability to accurately assess longitudinal changes or responses to interventions. To address this limitation, dynamic monitoring of AISI levels could offer a more comprehensive understanding of temporal fluctuations in immune-inflammatory status. Second, we lacked access to detailed clinical information on cancer patients, including TNM staging and tumor size. Although tumor stratification was performed, and confounding factors were controlled, residual confounding by unmeasured variables remains a possibility. Factors such as treatment regimens and genetic variations, which were not included in the NHANES dataset, may influence the stability of our results. Future clinical cohort studies are necessary to validate our findings and examine the potential impact of these variables. Third, the reliance on household interviews and questionnaires in the NHANES dataset introduces risks of reporting inaccuracies and recall bias. Although rigorous validation processes are employed by agencies like the NCHS, these inherent limitations cannot be completely eliminated.
Conclusions
By investigating the characteristics of the inflammatory biomarkers AISI in female cancer patients, we found that AISI is linearly positively correlated with both all-cause mortality and cardiovascular mortality in this population. AISI is not only a valuable prognostic biomarker, but its simplicity, ease of measurement, and cost-effectiveness also underscore its broad potential for clinical application. High-risk patients, as determined by AISI levels, can benefit from enhanced cardiovascular disease monitoring and intervention, which may ultimately reduce mortality risk. It is recommended that AISI be incorporated into the routine screening process for female cancer patients. Furthermore, when combined with other clinical indicators, AISI can contribute to the development of personalized health management strategies. For instance, if AISI exceeds 5.61, intensified cardiovascular disease interventions should be considered, and cancer treatment regimens may be adjusted accordingly. Therefore, our study highlights the potential clinical value of the inflammation biomarker AISI in predicting the prognosis of female cancer patients.
Data availability statement
Publicly available datasets were analyzed in this study. This data can be found here: http://www.cdc.gov/nchs/nhanes.
Author contributions
YY: Conceptualization, Data curation, Formal Analysis, Funding acquisition, Investigation, Methodology, Project administration, Resources, Software, Supervision, Validation, Visualization, Writing – original draft, Writing – review & editing. ZH: Data curation, Investigation, Writing – original draft. YY: Formal Analysis, Methodology, Validation, Writing – original draft. HW: Visualization, Writing – original draft. WS: Conceptualization, Supervision, Writing – review & editing. NW: Conceptualization, Funding acquisition, Supervision, Writing – review & editing.
Funding
The author(s) declare that financial support was received for the research and/or publication of this article. This research was jointly supported by the “1+X” Research Project of The Second Hospital of Dalian Medical University (YJ2024001202), the “1+X” Clinical Technology Enhancement Project on Ovarian Cancer Ultra Radical Surgery (2022LCJSZD04), and the “Xingliao Talent Plan” Medical Expert Project (YXMJ-QN-17).
Conflict of interest
The authors declare that the research was conducted in the absence of any commercial or financial relationships that could be construed as a potential conflict of interest.
Generative AI statement
The author(s) declare that no Generative AI was used in the creation of this manuscript.
Publisher’s note
All claims expressed in this article are solely those of the authors and do not necessarily represent those of their affiliated organizations, or those of the publisher, the editors and the reviewers. Any product that may be evaluated in this article, or claim that may be made by its manufacturer, is not guaranteed or endorsed by the publisher.
Supplementary material
The Supplementary Material for this article can be found online at: https://www.frontiersin.org/articles/10.3389/fonc.2025.1552341/full#supplementary-material
Supplementary Figure 1 | Selection process for study cohorts.
Supplementary Figure 2 | Association between AISI and all-cause mortality (A) and cardiovascular mortality (B) in participants with female cancer, adjusted for age, hypertension, diabetes, BMI, alcohol use, and smoking. The shaded areas represent the 95% CI.
Supplementary Table 1 | Baseline characteristics of the study cohort.
Supplementary Table 2 | Comparison of prognostic ability for all-cause and Cardiovascular mortality between AISI and SII.
Supplementary Table 3 | HRs (95% CIs) for all-cause and cardiovascular mortality according to different types of AISI data in cancer patients from Dandong Central Hospital (2022–2023) among adult females.
Abbreviations
NHANES, National Health and Nutrition Examination Survey; AISI, Aggregate Index of Systemic Inflammation; BMI, Body Mass Index; NCHS, National Center for Health Statistics; SII, Systemic Immune-Inflammation Index; IPF, idiopathic pulmonary fibrosis; IRB, Institutional Review Board; NEU, neutrophils; PLT, platelets; MON, monocytes; LYM, lymphocytes; NDI, National Death Index; CBC, complete blood count; WBC, white blood cell; MEC, mobile examination center; CAPI, computer-assisted personal interview; ROC, receiver operating characteristic; HR, hazard ratio; TME, tumor microenvironment; VEGF, vascular endothelial growth factor; NE, neutrophil elastase; NETs, neutrophil extracellular traps; MPO, myeloperoxidase; TAMs, tumor-associated macrophages; AUC, the area under the curve; NRI, Net Reclassification Improvement; IDI, Integrated Discrimination Improvement; NLR, neutrophil-lymphocyte ratio; PLR, platelet-lymphocyte ratio; CRP, C-reactive protein
References
1. Bray F, Laversanne M, Sung H, Ferlay J, Siegel RL, Soerjomataram I, et al. Global cancer statistics 2022: GLOBOCAN estimates of incidence and mortality worldwide for 36 cancers in 185 countries. CA: A Cancer J clinicians. (2024) 74:229–63. doi: 10.3322/caac.21834
2. Guida F, Kidman R, Ferlay J, Schüz J, Soerjomataram I, Kithaka B, et al. Global and regional estimates of orphans attributed to maternal cancer mortality in 2020. Nat Med. (2022) 28:2563–72. doi: 10.1038/s41591-022-02109-2
3. Pecorelli S, Favalli G, Zigliani L, Odicino F. Cancer in women. Int J Gynecology Obstetrics. (2003) 82:369–79. doi: 10.1016/S0020-7292(03)00225-X
4. Dong J, Jiang W, Zhang W, Guo T, Yang Y, Jiang X, et al. Exploring the J-shaped relationship between HALP score and mortality in cancer patients: A NHANES 1999-2018 cohort study. Front Oncol. (2024) 14. doi: 10.3389/fonc.2024.1388610
5. Hersch J, Juraskova I, Price M, Mullan B. Psychosocial interventions and quality of life in gynaecological cancer patients: a systematic review. Psycho-Oncology: J Psychological Soc Behav Dimensions Cancer. (2009) 18:795–810. doi: 10.1002/pon.v18:8
6. Shalapour S, Karin M. Immunity, inflammation, and cancer: an eternal fight between good and evil. J Clin Invest. (2015) 125:3347–55. doi: 10.1172/JCI80007
7. Greten FR, Grivennikov SI. Inflammation and cancer: triggers, mechanisms, and consequences. Immunity. (2019) 51:27–41. doi: 10.1016/j.immuni.2019.06.025
8. Zhou X, Du Y, Huang Z, Xu J, Qiu T, Wang J, et al. Prognostic value of PLR in various cancers: a meta-analysis. PloS One. (2014) 9:e101119. doi: 10.1371/journal.pone.0101119
9. Fan W, Wei C, Liu Y, Sun Q, Tian Y, Wang X, et al. The prognostic value of hematologic inflammatory markers in patients with acute coronary syndrome undergoing percutaneous coronary intervention. Clin Appl Thrombosis/Hemostasis. (2022) 28:10760296221146183. doi: 10.1177/10760296221146183
10. Jin N, Huang L, Hong J, Zhao X, Hu J, Wang S, et al. The association between systemic inflammation markers and the prevalence of hypertension. BMC Cardiovasc Disord. (2023) 23:615. doi: 10.1186/s12872-023-03661-6
11. Yin X, Zou J, Yang J. The association between the aggregate index of systemic inflammation and risk of rheumatoid arthritis: retrospective analysis of NHANES 1999–2018. Front Med. (2024) 11:1446160. doi: 10.3389/fmed.2024.1446160
12. He K, Si L, Pan X, Sun L, Wang Y, Lu J, et al. Preoperative systemic immune–inflammation index (SII) as a superior predictor of long-term survival outcome in patients with stage I–II gastric cancer after radical surgery. Front Oncol. (2022) 12:829689. doi: 10.3389/fonc.2022.829689
13. Nie D, Gong H, Mao X, Li Z. Systemic immune-inflammation index predicts prognosis in patients with epithelial ovarian cancer: a retrospective study. Gynecologic Oncol. (2019) 152:259–64. doi: 10.1016/j.ygyno.2018.11.034
14. Yang R, Chang Q, Meng X, Gao N, Wang W. Prognostic value of systemic immune-inflammation index in cancer: a meta-analysis. J Cancer. (2018) 9:3295. doi: 10.7150/jca.25691
15. Zhou Y, Dai M, Zhang Z. Prognostic significance of the systemic immune-inflammation index (SII) in patients with small cell lung cancer: a meta-analysis. Front Oncol. (2022) 12:814727. doi: 10.3389/fonc.2022.814727
16. Hosseninia S, Ghobadi H, Garjani K, Hosseini SAH, Aslani MR. Aggregate index of systemic inflammation (AISI) in admission as a reliable predictor of mortality in COPD patients with COVID-19. BMC Pulmonary Med. (2023) 23:107. doi: 10.1186/s12890-023-02397-5
17. Wang H-K, Wei Q, Yang Y-L, Lu T-Y, Yan Y, Wang F. Clinical usefulness of the lymphocyte-to-monocyte ratio and aggregate index of systemic inflammation in patients with esophageal cancer: a retrospective cohort study. Cancer Cell Int. (2023) 23:13. doi: 10.1186/s12935-023-02856-3
18. Xiu J, Lin X, Chen Q, Yu P, Lu J, Yang Y, et al. The aggregate index of systemic inflammation (AISI): A novel predictor for hypertension. Front Cardiovasc Med. (2023) 10:1163900. doi: 10.3389/fcvm.2023.1163900
19. Zinellu A, Collu C, Nasser M, Paliogiannis P, Mellino S, Zinellu E, et al. The aggregate index of systemic inflammation (AISI): A novel prognostic biomarker in idiopathic pulmonary fibrosis. J Clin Med. (2021) 10:4134. doi: 10.3390/jcm10184134
20. Johnson CL, Dohrmann SM, Burt VL, Mohadjer LK. National health and nutrition examination survey: sample design, 2011-2014. Vital Health Stat. (2014) 2(162):1–33.
21. Brämer GR. International statistical classification of diseases and related health problems. Tenth revision. World Health Stat Q. (1988) 41(1):32–6.
22. Putzu C, Cortinovis DL, Colonese F, Canova S, Carru C, Zinellu A, et al. Blood cell count indexes as predictors of outcomes in advanced non-small-cell lung cancer patients treated with Nivolumab. Cancer Immunology Immunotherapy. (2018) 67:1349–53. doi: 10.1007/s00262-018-2182-4
23. Feliciano EMC, Kroenke CH, Meyerhardt JA, Prado CM, Bradshaw PT, Kwan ML, et al. Association of systemic inflammation and sarcopenia with survival in nonmetastatic colorectal cancer: results from the C SCANS study. JAMA Oncol. (2017) 3:e172319. doi: 10.1001/jamaoncol.2017.2319
24. Grivennikov SI, Greten FR, Karin M. Immunity, inflammation, and cancer. Cell. (2010) 140:883–99. doi: 10.1016/j.cell.2010.01.025
25. Mandaliya H, Jones M, Oldmeadow C, Nordman II. Prognostic biomarkers in stage IV non-small cell lung cancer (NSCLC): neutrophil to lymphocyte ratio (NLR), lymphocyte to monocyte ratio (LMR), platelet to lymphocyte ratio (PLR) and advanced lung cancer inflammation index (ALI). Trans Lung Cancer Res. (2019) 8:886. doi: 10.21037/tlcr.2019.11.16
26. Miyamoto R, Inagawa S, Sano N, Tadano S, Adachi S, Yamamoto M. The neutrophil-to-lymphocyte ratio (NLR) predicts short-term and long-term outcomes in gastric cancer patients. Eur J Surg Oncol. (2018) 44:607–12. doi: 10.1016/j.ejso.2018.02.003
27. Yang M, Zhang Q, Ge Y, Tang M, Hu C, Wang Z, et al. Prognostic roles of inflammation-and nutrition-based indicators for female patients with cancer. J Inflammation Res. (2022) 15:3573–86. doi: 10.2147/JIR.S361300
28. Plitas G, Rudensky AY. Regulatory T cells in cancer. Annu Rev Cancer Biol. (2020) 4:459–77. doi: 10.1146/annurev-cancerbio-030419-033428
29. Rimini M, Casadei-Gardini A, Ravaioli A, Rovesti G, Conti F, Borghi A, et al. Could inflammatory indices and metabolic syndrome predict the risk of cancer development? Analysis from the Bagnacavallo population study. J Clin Med. (2020) 9:1177. doi: 10.3390/jcm9041177
30. Xie J, Guo Z, Zhu Y, Ma M, Jia G. Peripheral blood inflammatory indexes in breast cancer: A review. Medicine. (2023) 102:e36315. doi: 10.1097/MD.0000000000036315
31. Houghton AM, Rzymkiewicz DM, Ji H, Gregory AD, Egea EE, Metz HE, et al. Neutrophil elastase–mediated degradation of IRS-1 accelerates lung tumor growth. Nat Med. (2010) 16:219–23. doi: 10.1038/nm.2084
32. Walz W, Cayabyab FS. Neutrophil infiltration and matrix metalloproteinase-9 in lacunar infarction. Neurochemical Res. (2017) 42:2560–5. doi: 10.1007/s11064-017-2265-1
33. Cedervall J, Hamidi A, Olsson A-K. Platelets, NETs and cancer. Thromb Res. (2018) 164:S148–52. doi: 10.1016/j.thromres.2018.01.049
34. Saffarzadeh M, Juenemann C, Queisser MA, Lochnit G, Barreto G, Galuska SP, et al. Neutrophil extracellular traps directly induce epithelial and endothelial cell death: a predominant role of histones. PloS One. (2012) 7:e32366. doi: 10.1371/journal.pone.0032366
35. Schedel F, Mayer-Hain S, Pappelbaum KI, Metze D, Stock M, Goerge T, et al. Evidence and impact of neutrophil extracellular traps in Malignant melanoma. Pigment Cell melanoma Res. (2020) 33:63–73. doi: 10.1111/pcmr.12818
36. Richardson J, Hendrickse C, Gao-Smith F, Thickett D. Neutrophil extracellular trap production in patients with colorectal cancer in vitro. Int J Inflammation. (2017) 2017:4915062. doi: 10.1155/2017/4915062
37. Zahorec R. Ratio of neutrophil to lymphocyte counts–rapid and simple parameter of systemic inflammation and stress in critically ill. Bratisl Lek Listy. (2001) 102:5–14.
38. Brummel K, Eerkens AL, de Bruyn M, Nijman HW. Tumour-infiltrating lymphocytes: from prognosis to treatment selection. Br J Cancer. (2023) 128:451–8. doi: 10.1038/s41416-022-02119-4
39. Väyrynen J, Tuomisto A, Klintrup K, Mäkelä J, Karttunen T, Mäkinen M. Detailed analysis of inflammatory cell infiltration in colorectal cancer. Br J Cancer. (2013) 109:1839–47. doi: 10.1038/bjc.2013.508
40. De Giorgi U, Mego M, Scarpi E, Giuliano M, Giordano A, Reuben JM, et al. Relationship between lymphocytopenia and circulating tumor cells as prognostic factors for overall survival in metastatic breast cancer. Clin Breast Cancer. (2012) 12:264–9. doi: 10.1016/j.clbc.2012.04.004
41. Li C, Jiang P, Wei S, Xu X, Wang J. Regulatory T cells in tumor microenvironment: new mechanisms, potential therapeutic strategies and future prospects. Mol Cancer. (2020) 19:1–23. doi: 10.1186/s12943-019-1085-0
42. Eruslanov E, Neuberger M, Daurkin I, Perrin GQ, Algood C, Dahm P, et al. Circulating and tumor-infiltrating myeloid cell subsets in patients with bladder cancer. Int J Cancer. (2012) 130:1109–19. doi: 10.1002/ijc.v130.5
43. Landskron G, de la Fuente M, Thuwajit P, Thuwajit C, Hermoso MA. Chronic inflammation and cytokines in the tumor microenvironment. J Immunol Res. (2014) 2014:149185. doi: 10.1155/2014/149185
44. Yang J, Tang S, Saba NF, Shay C, Teng Y. Tumor secretome shapes the immune landscape during cancer progression. J Exp Clin Cancer Res. (2025) 44:47. doi: 10.1186/s13046-025-03302-0
45. Liu Y, Cao X. The origin and function of tumor-associated macrophages. Cell Mol Immunol. (2015) 12:1–4. doi: 10.1038/cmi.2014.83
46. Bingle L, Brown N, Lewis CE. The role of tumour-associated macrophages in tumour progression: implications for new anticancer therapies. J Pathology: A J Pathological Soc Great Britain Ireland. (2002) 196:254–65. doi: 10.1002/path.v196:3
47. Peng L-s, Zhang J-y, Teng Y-s, Zhao Y-l, Wang T-t, Mao F-y, et al. Tumor-associated monocytes/macrophages impair NK-cell function via TGFβ1 in human gastric cancer. Cancer Immunol Res. (2017) 5:248–56. doi: 10.1158/2326-6066.CIR-16-0152
48. Chu X, Tian Y, Lv C. Decoding the spatiotemporal heterogeneity of tumor-associated macrophages. Mol Cancer. (2024) 23:150. doi: 10.1186/s12943-024-02064-1
49. Han L, Jia Y, Song Q, Wang N, Wang J, Bai B, et al. Prognostic significance of preoperative absolute peripheral monocyte count in esophageal squamous cell carcinoma. Dis Esophagus. (2016) 29:740–6. doi: 10.1111/dote.2016.29.issue-7
50. Valero C, Pardo L, López M, García J, Camacho M, Quer M, et al. Pretreatment count of peripheral neutrophils, monocytes, and lymphocytes as independent prognostic factor in patients with head and neck cancer. Head Neck. (2017) 39:219–26. doi: 10.1002/hed.24561
51. Wang Y-Q, Zhu Y-J, Pan J-H, Xu F, Shao X-G, Sha J-J, et al. Peripheral monocyte count: an independent diagnostic and prognostic biomarker for prostate cancer–a large Chinese cohort study. Asian J andrology. (2017) 19:579–85. doi: 10.4103/1008-682X.186185
52. Li S, Lu Z, Wu S, Chu T, Li B, Qi F, et al. The dynamic role of platelets in cancer progression and their therapeutic implications. Nat Rev Cancer. (2024) 24:72–87. doi: 10.1038/s41568-023-00639-6
53. Xie Q, Zhou J, He C, Xu Y, Tao F, Hu M. Unlocking the intricacies: exploring the complex interplay between platelets and ovarian cancer. Crit Rev Oncol Hematol. (2024) 202:104465. doi: 10.1016/j.critrevonc.2024.104465
54. Stone RL, Nick AM, McNeish IA, Balkwill F, Han HD, Bottsford-Miller J, et al. Paraneoplastic thrombocytosis in ovarian cancer. New Engl J Med. (2012) 366:610–8. doi: 10.1056/NEJMoa1110352
55. Bailey SE, Ukoumunne OC, Shephard E, Hamilton W. How useful is thrombocytosis in predicting an underlying cancer in primary care? A systematic review. Family Pract. (2017) 34:4–10. doi: 10.1093/fampra/cmw100
56. Wilcox NS, Amit U, Reibel JB, Berlin E, Howell K, Ky B. Cardiovascular disease and cancer: shared risk factors and mechanisms. Nat Rev Cardiol. (2024) 21(9):617–31. doi: 10.1038/s41569-024-01017-x
57. Willerson JT, Ridker PM. Inflammation as a cardiovascular risk factor. Circulation. (2004) 109:II–2-II-10. doi: 10.1161/01.CIR.0000129535.04194.38
58. Patel DA, Xi J, Luo J, Hassan B, Thomas S, Ma CX, et al. Neutrophil-to-lymphocyte ratio as a predictor of survival in patients with triple-negative breast cancer. Breast Cancer Res Treat. (2019) 174:443–52. doi: 10.1007/s10549-018-05106-7
59. Polterauer S, Grimm C, Tempfer C, Sliutz G, Speiser P, Reinthaller A, et al. C-reactive protein is a prognostic parameter in patients with cervical cancer. Gynecologic Oncol. (2007) 107:114–7. doi: 10.1016/j.ygyno.2007.06.001
60. Schmid M, Schneitter A, Hinterberger S, Seeber J, Reinthaller A, Hefler L. Association of elevated C-reactive protein levels with an impaired prognosis in patients with surgically treated endometrial cancer. Obstetrics Gynecology. (2007) 110:1231–6. doi: 10.1097/01.AOG.0000292085.50987.f2
61. Templeton AJ, McNamara MG, Šeruga B, Vera-Badillo FE, Aneja P, Ocaña A, et al. Prognostic role of neutrophil-to-lymphocyte ratio in solid tumors: a systematic review and meta-analysis. J Natl Cancer Institute. (2014) 106:dju124. doi: 10.1093/jnci/dju124
62. Zhang M, Huang X-z, Song Y-x, Gao P, Sun J-x, Wang Z-n. High platelet-to-lymphocyte ratio predicts poor prognosis and clinicopathological characteristics in patients with breast cancer: a meta-analysis. BioMed Res Int. (2017) 2017:9503025. doi: 10.1155/2017/9503025
Keywords: female cancer, aggregate index of systemic inflammation, AISI, mortality, NHANES
Citation: Yang Y, Hu Z, Ye Y, Wu H, Sun W and Wang N (2025) Association of aggregate index of systemic inflammation with increased all-cause and cardiovascular mortality in female cancer patients. Front. Oncol. 15:1552341. doi: 10.3389/fonc.2025.1552341
Received: 02 January 2025; Accepted: 04 April 2025;
Published: 29 April 2025.
Edited by:
Alireza Sadjadi, Tehran University of Medical Sciences, IranReviewed by:
Shanchun Su, Duke University, United StatesYuhao Xie, St. John’s University, United States
Liangyun Xie, Henan University of Science and Technology, China
Copyright © 2025 Yang, Hu, Ye, Wu, Sun and Wang. This is an open-access article distributed under the terms of the Creative Commons Attribution License (CC BY). The use, distribution or reproduction in other forums is permitted, provided the original author(s) and the copyright owner(s) are credited and that the original publication in this journal is cited, in accordance with accepted academic practice. No use, distribution or reproduction is permitted which does not comply with these terms.
*Correspondence: Ning Wang, bm9uYXdhcmVAc2luYS5jb20=