- 1Department of Medicine, Saint Vincent Hospital, Worcester, MA, United States
- 2Department of Internal Medicine, Division of Medical Oncology at the Arthur G. James Cancer Hospital and Richard J. Solove Research Institute, The Ohio State University Comprehensive Cancer Center, Columbus, OH, United States
Pancreatic Ductal Adenocarcinoma (PDAC) accounts for a significant burden of global cancer deaths worldwide. The dismal outcomes associated with PDAC can be overcome by detecting the disease early and developing tools that predict response to treatment, allowing the selection of the most optimal treatment. Over the last couple of years, significant progress has been made in the development of novel biomarkers that aid in diagnosis, prognosis, treatment selection, and monitoring response. Blood-based biomarkers offer an alternative to tissue-based diagnosis and offer immense potential in managing PDAC. In this review, we have discussed the advances in blood-based biomarkers in PDAC, such as DNA (mutations and methylations), RNA, protein biomarkers and circulating tumor cells (CTC) over the last decade and also elucidated all aspects of practical implementation of these biomarkers in clinical practice. We have also discussed implementing multiomics utilizing more than one biomarker and targeted therapies that have been developed using these biomarkers.
Introduction
Pancreatic ductal adenocarcinoma (PDAC) accounts for around 3% of all cancers in the United States and about 7% of all cancer deaths estimated in 2023 (1). The last few years have seen a significant increase in the global burden of PDAC diagnosis. The American Cancer Society projects that in 2025, approximately 67,440 patients will be diagnosed with PDAC, and an estimated 51,980 will succumb to the disease (2). Males are reported to have a higher prevalence of PDAC compared to females. While smoking rates have declined over recent decades, the rising incidence of diabetes is hypothesized to be a key driver of the increasing PDAC cases. Biomarkers such as fasting blood glucose and insulin resistance have shown significant associations with PDAC, and patients with the disease frequently present with elevated HbA1c levels (3). In common clinical practice, PDAC is classified into resectable, unresectable and borderline resectable to determine the possibility of surgical intervention and the need for neoadjuvant therapy (4, 5). A large proportion of patients have unresectable disease at the time of diagnosis (6). At the time of initial presentation, 50% patients are found to have metastatic PDAC, with 30-35% being unresectable and only 10-15% being amenable to surgery (3). There may also be the presence of pancreatic intraepithelial neoplasms (PanIN) which may progress to high-grade dysplasia and PDAC (3).
Early-stage PDAC is clinically silent while patients with advanced disease have nonspecific signs and symptoms in the form of anorexia, weight loss, abdominal pain, jaundice, acholic stools, and dark urine which are the manifestations of biliary tract obstruction. The most commonly used diagnostic tools include tri-phasic pancreatic protocol computed tomography (CT), magnetic resonance imaging (MRI), and endoscopic ultrasound-guided fine needle aspiration for cytological diagnosis (7). Serum carbohydrate antigen (CA 19-9) has been used to aid diagnosis in symptomatic patients and to predict recurrence after resection but its success as a screening tool has been underwhelming. There has been no effective strategy so far to screen and detect PDAC in early stages.
The management for PDAC currently depends on conventional polychemotherapies with poor outcomes and targeted therapy are seldom used (8). The dismal outcomes associated with PDAC necessitate the urgent need to develop tools that can identify cancer early (9). There is also an urgent need for tools to predict prognosis and response to treatment. There has been a huge push in the last couple of years towards the development of novel serum biomarkers that can aid in diagnosis, prognosis and assist in tailoring treatment and monitoring response post-treatment.
Based on current practice, tissue biopsies are the current method to assess molecular information about the tumor and essential for diagnosis, screening, and mutation expression. Tissue biopsies come with its own inherent set of disadvantages: the requirement of invasive surgery, which poses risks for the patient (10). Besides, certain tumors are difficult to access on account of challenging anatomical locations, including in PDAC. There is also an associated risk of augmenting the risk of metastatic seeding (11). There can also be variations in the amount of tissue extracted and results obtained due to the tumor heterogeneity, inter-operator variation, and evolving nature of the tumor. Lastly, the costs involved and the requirement of an operation theatre can be an inhibiting factor that is a hindrance to tumor characterization (12). Tumor monitoring is required at different times of the disease course to monitor treatment, and it is not feasible to have repeat biopsies every time (13). Monitoring using radiology while providing a good physical estimation hardly provides any evidence of molecular/pathological features that are vital to understanding the prognosis and treatment (14). Liquid biopsy and blood-based biomarkers are based on the principle that during apoptosis and necrosis, a few of these biomarkers are released into circulation, and their identification and capture provide an accurate molecular and pathological characterization of the tumor (15). The non-invasive nature of this process is appealing both to the patients and the clinician to avoid potential complications and morbidity associated with invasive biopsy measures and allow for dynamic monitoring of the tumor at different time points (16). Studies have been conducted in several malignant tumors with utility such as prostate, colorectal, lung, and breast with a great amount of research also having been conducted on PDAC (17–20).
While samples for liquid biopsy can be derived from both plasma and serum, plasma samples are often preferable to serum samples for analysis in clinical applications. This stems from the fact that while serum may have higher amounts of circulating free DNA, RNA and proteins, it is confounded by the presence of DNA, RNA and proteins released during the lysis of circulating white blood cells, including neutrophils, that reduce the relative proportion of tumor DNA, RNA and protein (21). It is also a prerequisite to collect serum at room temperature for clotting, which further increases the risk of cell lyses and degradation of DNA, RNA and protein (22). Most studies, however, are based on serum samples in spite of the advantages of plasma in this regard. This is due to the convenience of serum use in clinical laboratories or because of limitations in sample availability for retrospective studies (22).
In our 2022 publication, we explored the potential of cell-free DNA (cfDNA) testing (methylation and mutation) in clinical settings (23). Despite its promising results in retrospective and limited prospective studies, no new clinical applications have emerged since then. In the sections that follow, we discuss other important blood-based biomarkers such as RNA, proteins, circulating tumor cells (CTC) and multiomic tests in addition to cfDNA. We have focused on the recent advances in blood-based biomarkers such as DNA (mutations and methylations), CTC, RNA, and protein, and all aspects of practical implementation of these biomarkers in clinical practice.
Cell-free DNA
Cell-free DNA (cfDNA) encompasses normal cell DNA, tumor cell DNA, and exosomal DNA (24). As cancer cells undergo apoptosis or necrosis, genetic material is aberrantly released into the bloodstream as either free nucleic acid or exosomes (24). It has been hypothesized that cfDNA can potentially be used to diagnose and stage PDAC, and research is also being conducted to investigate the role of cfDNA as a potential predictor of treatment response. Multiple studies have demonstrated over the last couple of years the presence of high levels of cfDNA in blood of patients with invasive tumors including pancreas, colon, and melanoma (25–27). cfDNA in PDAC was first reported by Shapiro et al. in 1983 when he found that cfDNA was significantly elevated in PDAC compared to healthy controls (28). He hypothesized its utility as a potential diagnostic and prognostic biomarker. In the past decade, we have made huge strides in implementing cfDNA into clinical practice. This has been supported by multiple studies demonstrating the high degree of correlation that exists between tumor DNA and cfDNA (29).
Numerous techniques are available to detect cfDNA of tumor cells. The most popularly used techniques include droplet digital PCR (ddPCR), Whole Genome Sequencing (WGS) and Whole Exome Sequencing (WES) (30). Other techniques that have also been used in studies include Beads, Emulsion, Amplification, and magnetics (BEAMing), Cancer Personalized Profiling by Deep Sequencing (CAPP-Seq), Tagged-amplicon deep sequencing (Tam-Seq) (30). ddPCR identifies rare mutations and copy number variations. The major limitation in ddPCR, in spite of its high sensitivity, is that it is only able to detect specific genomic sequences in the sample (31). BEAMing, while combining PCR with flow cytometry, also suffers from similar limitations (32). CAPP-Seq uses large genomic libraries in tandem with individual patient sample sequences to identify cfDNA alterations. It compares well-known tumor alterations with DNA oligonucleotides to identify patient-specific alterations (33). While there is an advantage in the identification of insertions/deletions, single nucleotide variants, rearrangements, and copy variants, it often struggles to identify fusions, which is not a problem with ddPCR, WES, or WGS. Tam-Seq employs primers to tag and identify the desired genomic sequence but requires that the sequence be characterized to be included in the analysis (34). WGS and WES analyses all the potential tumor mutations present in the entire genome allowing for comprehensive analysis and characterization. The extensive coverage comes with its own downside related to the increased cost, error rate and sensitivity (35).
In our previous review (23), we have elucidated how cfDNA somatic mutations can either be divided into specific mutations or nonspecific total mutated cfDNA. Numerous studies in the past have implicated KRAS mutations as a poor prognostic biomarker in PDAC. It has been associated with advanced and metastatic diseases and many studies have evaluated and found the presence of mutated KRAS and its quantification as important factors in determining the prognosis of PDAC (36). Ankeny et al. (37) conducted one of the earlier studies to clinically explore the utility of mutated KRAS in circulating tumour DNA (ctDNA) and its implication in PDAC. Using techniques of immunocytochemistry and chip mounting, they counted the number of CTC in PDAC and healthy controls. 78% patients in the PDAC group were found to have CTC in peripheral venous blood compared to 3.6% in the non-adenocarcinoma group. There was also a statistically significant difference that existed between stage 4 and other PDAC. Overall CTC had an Area Under Curve (AUC)=0.885 for discriminating locoregional disease versus metastatic disease. Other frequently detected mutations in cfDNA of patients with PDAC besides KRAS include TP53 and CDKN2A, with ATM, PIK3CA, PTEN, TERT, NF1, JAK2, and GNAS mutations also seen (29, 38). In a previous paper from 2022, we have described in detail about the cfDNA genomic and epigenetic biomarkers that have been studied to aid in the diagnosis of PDAC and its role in determining the stage and prognosis in this population (23). We will look at the most recent advances (last 3 years) in the field in this section (Table 1).
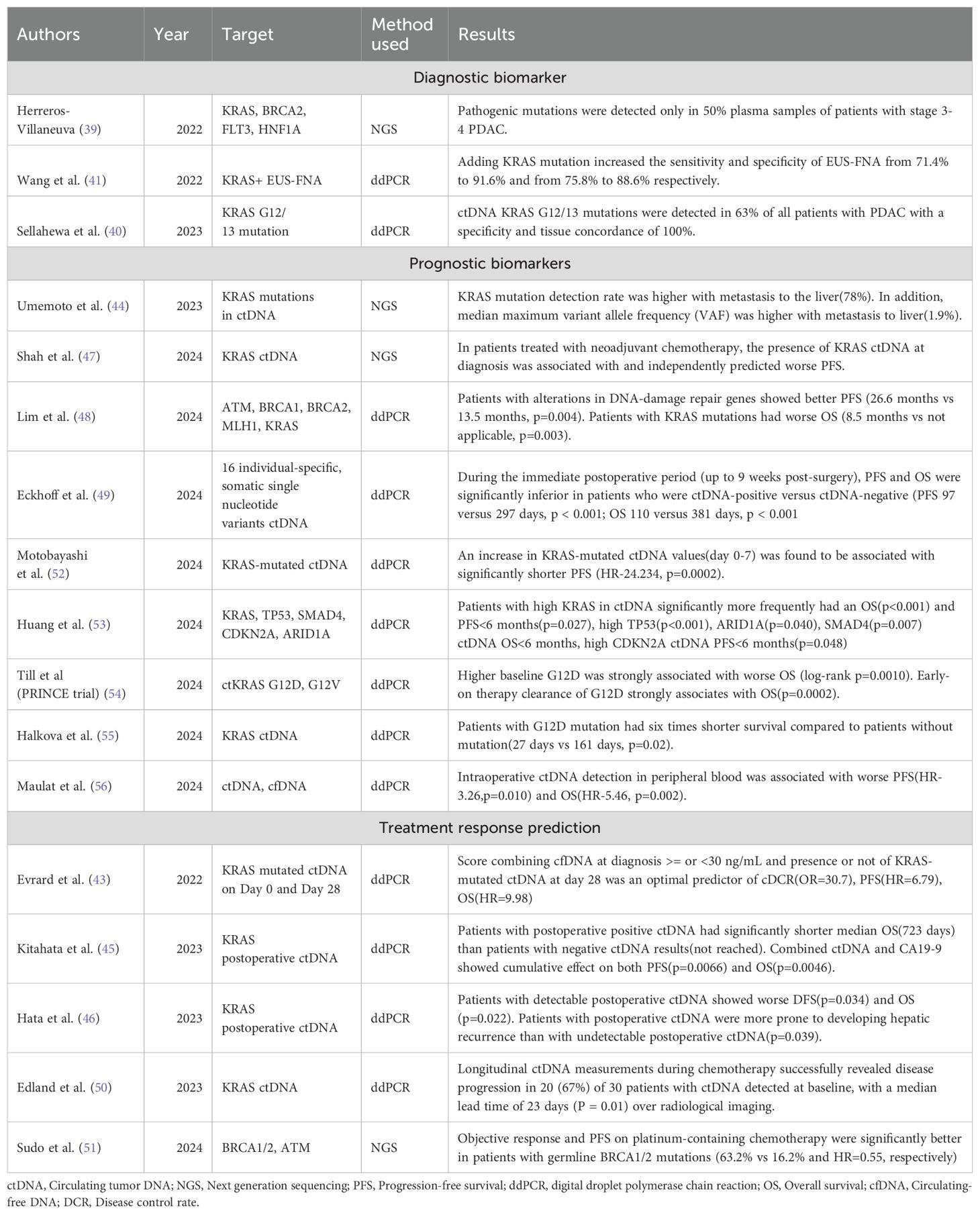
Table 1. Latest developments in prognosis, and treatment response prediction of PDAC using DNA biomarkers.
Numerous studies have been conducted in the last 3 years utilizing cfDNA in diagnosing PDAC. Herreros-Villaneuva (39) performed next-generation sequencing (NGS) on the plasma samples of 27 patients with PDAC between 2016 and 2020 in Spain using a commercial panel of 65 genes to detect cfDNA but found that pathogenic mutations were detected only in 50% of samples from patients with stage 3-4 PDAC, highlighting the need for further work in this area. Sellahewa et al. (40) utilized ddPCR in 81 patients with PDAC and 30 patients with benign pancreatic disease to analyze for KRAS G12/G13 ctDNA mutation and found that KRAS G12/G13 mutations were detected in 63% of all patients with PDAC and in 76% of patients who also had the same mutation detected in primary. The specificity and tissue concordance were 100%. KRAS has also been used in conjunction with endoscopic ultrasound-guided fine-needle aspiration (EUS-FNA) by Wang et al. (41) who found that the addition of the ctDNA biomarker increased the sensitivity and accuracy of EUS-FNA from 71.4% to 91.6% and 75.8% to 88.6% respectively. This probably represents a more accurate role of ctDNA in PDAC diagnosis, where it can serve in conjunction with other invasive diagnostic techniques that otherwise perform poorly by themselves. Pol et al. (42) took a unique approach, collecting samples from 203 healthy and 664 cancer plasma detection encompassing 12 cancer types and employed whole genome sequencing to analyze the plasma mitochondrial DNA fraction. They found that the mitochondrial DNA fraction was elevated in 5 cancers-cholangiocarcinoma, colorectal, liver, pancreatic, and prostate cancer, in comparison to healthy individuals. This elevation in mitochondrial DNA was independent of the remaining cfDNA fraction. A predictive model integrating mitochondrial DNA and copy number analysis increased the AUC from 0.73 when using copy number alterations alone to 0.81.
An interesting study that was conducted post-2022 was the KRASCIPANC study by Evrard et al. (43) who collected serum samples from patients with unresectable PDAC on day 1 and before each cycle of chemotherapy at different time points until the progression of the disease. They developed an interesting risk stratification tool that helped better understand the prognosis by taking into account the ctDNA of mutated KRAS at day 0 and day 28 to classify PDAC into best, mid, and worst subcategories with disease control rates of 88%, 53%, and 20% respectively. This essentially described a study that serves a dual purpose of providing an understanding of the prognosis and treatment response. Another important study was the Gozila study by Umemoto et al. in Japan (44). Stratifying KRAS mutation rates by sites of metastasis, they found that detection of KRAS mutations in ctDNA was significantly higher in patients with metastasis to liver (75%) compared to lymph nodes (60%) and lungs (46%). The median maximum variable allele frequency (VAF) was also significantly higher with liver metastasis (1.8%) than other sites. Another notable study conducted by Kitahata et al. (45) found a strong correlation between the presence of postoperative ctDNA and overall survival (positive ctDNA with an OS of 723 days versus not reached for the absence of ctDNA). Findings of Hata et al. (46) were in agreement and they found that detection of postoperative ctDNA was associated with worse overall survival (OS) and progression-free survival (PFS). However, contrary to other studies, they concluded that preoperative ctDNA did not affect long-term outcomes. Shah et al. (47) investigated the utility of ctDNA in localized PDAC to guide clinical decisions. Using a 105 NGS gene panel to investigate patients with localized PDAC, they found that the presence of baseline ctDNA was associated with a worse CA19-9 response than those in which baseline ctDNA was not detected, thereby providing an alternative biomarker of treatment response to neoadjuvant chemotherapy (NAC). Lim et al. (48) investigated the prognostic value of DNA damage repair genes (ATM, BRCA1, BRCA2, MLH1) and found the presence of mutations in DNA damage repair genes were associated with a significantly better PFS compared to those without mutations in these genes (PFS 26.6 versus 13.5 months). A hopeful prospect for the future is the possibility of using individualised ctDNA panels for assessing the prognosis and treatment response as assessed by Eckhoff et al. (49) who tracked individual specific 16 single nucleotide variants for ctDNA detection and described that its presence was associated with worse RFS and OS. ctDNA represents a growing field in PDAC to predict the prognosis and treatment response. One study by Edland et al. (50) has also investigated how longitudinal ctDNA monitoring revealed disease progression in 67% patients with a median lead time of 23 days over radiological imaging. Other possible mutations such as germline BRCA1/2 mutations may demonstrate a better response to platinum containing chemotherapy and may prove a vital role in decision making (51).
The sensitivity of cfDNA also depends on the number of mutations and alterations identified, which can pose unique challenges. Detecting a single mutation in thousands of circulating cfDNA can significantly affect the ability to identify cancer. In contrast, the detection of a larger number of alterations in the genome increases the sensitivity by increasing the probability. This has also been demonstrated in Monte Carlo simulations that have shown that increasing the number of abnormalities from a few to hundreds can improve their detection (57). This forms the basis for fragmentomics, a novel approach developed over the last few years that is based on low coverage of WGS of isolated cfDNA. One of the first instances was described by Cristiano et al. (58) in 2019, who developed a novel technique called as DELFI (DNA evaluation of fragments for early interpretation) to analyze genome-wide differences in fragmentation patterns. Analyzing the fragmentation profiles of 236 patients with breast, colorectal, lung, ovarian, pancreatic, gastric, or bile duct cancer using machine learning, they found that incorporating genome-wide fragmentation features had sensitivities of detection ranging from 57% to >99% among the different cancers with a specificity of 98% and an overall AUC of 0.94 (58). Three primary surrogates can be measured in fragmentomics: the fragment size, the end motif, and the nucleosome imprint. Numerous techniques have been described that assist in the implementation of fragmentomics: calculation of tumor fraction by enriching short fragments, motif diversity score (MDS), orientation-aware cfDNA fragmentation (OCF), windowed protection score (WPS), DNA evaluation of fragments for early interception (DELFI), fragmentation evaluation of epigenetics from cfDNA (FREE-C), Epigenetic expression interference from cell-free DNA-sequencing (EPIC-seq) (59). The ideal choice of sample for fragmentomics analysis was determined to be plasma by Lee et al. (60), who found that while large fragments were increased in serum, the KRAS-mutated fraction in serum was significantly lower than that of plasma. One significant challenge in the implementation of large-scale fragmentomics is that the small amount of cfDNA makes library construction difficult, and the limited screening accuracy in different states limits its utility in cancer screening (59).
There have been numerous studies done specific to PDAC in fragmentomics. Liu et al. (61) utilized hybrid-capture-based cfDNA sequencing to analyze cfDNA fragments in PDAC and found that increasing the threshold of fragments decreases the ability to detect KRAS mutations in plasma, and the ability to recover ultra-short fragments increased the performance to detect KRAS mutations. This was followed up by Zvereva (62), who studied the plasma DNA samples of 40 patients with PDAC already known to carry KRAS mutation at codon 12 and screened cfDNA using a 4-size amplicon strategy to determine if their size would change detection rates, in turn giving an idea of malignant vs non-malignant cfDNA fragment sizes. They found that higher KRAS amplicon size (167bp and 218bp) was significantly associated with lower detectable cfDNA mutant allelic fractions (p<0.0001). One of the latest studies was performed by Shi et al. (63) who utilized fragmentomic features of pancreatobiliary cancers and combined them with CA19-9 to create a stacked model that was able to distinguish the respective cancers with an AUC of 0.978 in the training cohort and 0.941 in the validation cohort and performed fairly well even with low-coverage sequencing depth (AUC=0.905). Integrating with CA 19-9 enhanced the performance of the stacked model even further, achieving an AUC as high as 0.995.
Incorporating ctDNA into clinical practice with PDAC in supplementation with radiological imaging and its translation into better outcomes represents an exciting research proposition that needs further investigation.
DNA methylation markers
DNA methylation is known to affect a variety of processes in cells, including imprinting, transcriptional regulation and integrity of developmental processes (64). It mediates its influence via its effects on promoters and enhancers (noncoding DNA elements), which play an important role in gene regulation. In a normal cell, it maintains genomic integrity by methylating the 5’ position of the cytosine ring through a family of enzymes called as DNA methyltransferases (DNMT). This occurs in regions of repetitive genomic regions, including satellite DNA, long interspersed transposable elements (LINEs) and short interspersed transposable elements (SINEs). The principle of inhibiting gene expression is secondary to two mechanisms-direct inhibition by inhibition of specific transcription factors or indirectly through recruitment of methyl-CpG-binding domain proteins (65). DNA methylation can contribute to cancer in three ways: first, by hypomethylation of the cancer genome disinhibiting tumorigenesis, second by focal hypermethylation at tumour suppressor regions, and third, by direct mutagenesis through deamination, UV irradiation or exposure to carcinogens (66).
There are three main techniques for identification of differentially-methylated regions- bisulfite conversion-based methods, restriction enzyme-based approaches and affinity enrichment-based assays (67). Bisulfite-based methods are utilized the most largely due to its economical nature and rapid results. On the other hand, restriction-based enzyme approaches, while being more accurate, are limited due to the higher costs involved in their implementation. The third method of using an enrichment-based assay is easy with good sensitivity and specificity. However, one limitation of this approach is enrichment of sequences with higher number of CpG is more frequent than CpG poor fragments leading to its underrepresentation (67).
DNA methylation profiles of malignant conditions can be undifferentiable from non-malignant conditions if they are derived from the same tissue. One of the first studies utilizing the methylation signature in cfDNA was performed by Leman-Werman (68), who were able to interrogate methylome databases to identify cell/tissue-specific methylation signatures of diverse conditions, including multiple sclerosis, type 1 diabetes and PDAC or chronic pancreatitis (CP). Li et al. (69) utilized the hydroxymethylation profiles of colorectal, gastric, liver, thyroid, and pancreatic cancer and compared them with their adjacent tissues to find definitive signatures that were characteristic of the cancer types. In the last few years, there has been significant progress in the development of biomarkers identifying DNA methylation aberrations that assist in the diagnosis and prognosis of PDAC. Table 2 below highlights the latest advances in methylated DNA biomarkers that have occurred over the last decade.
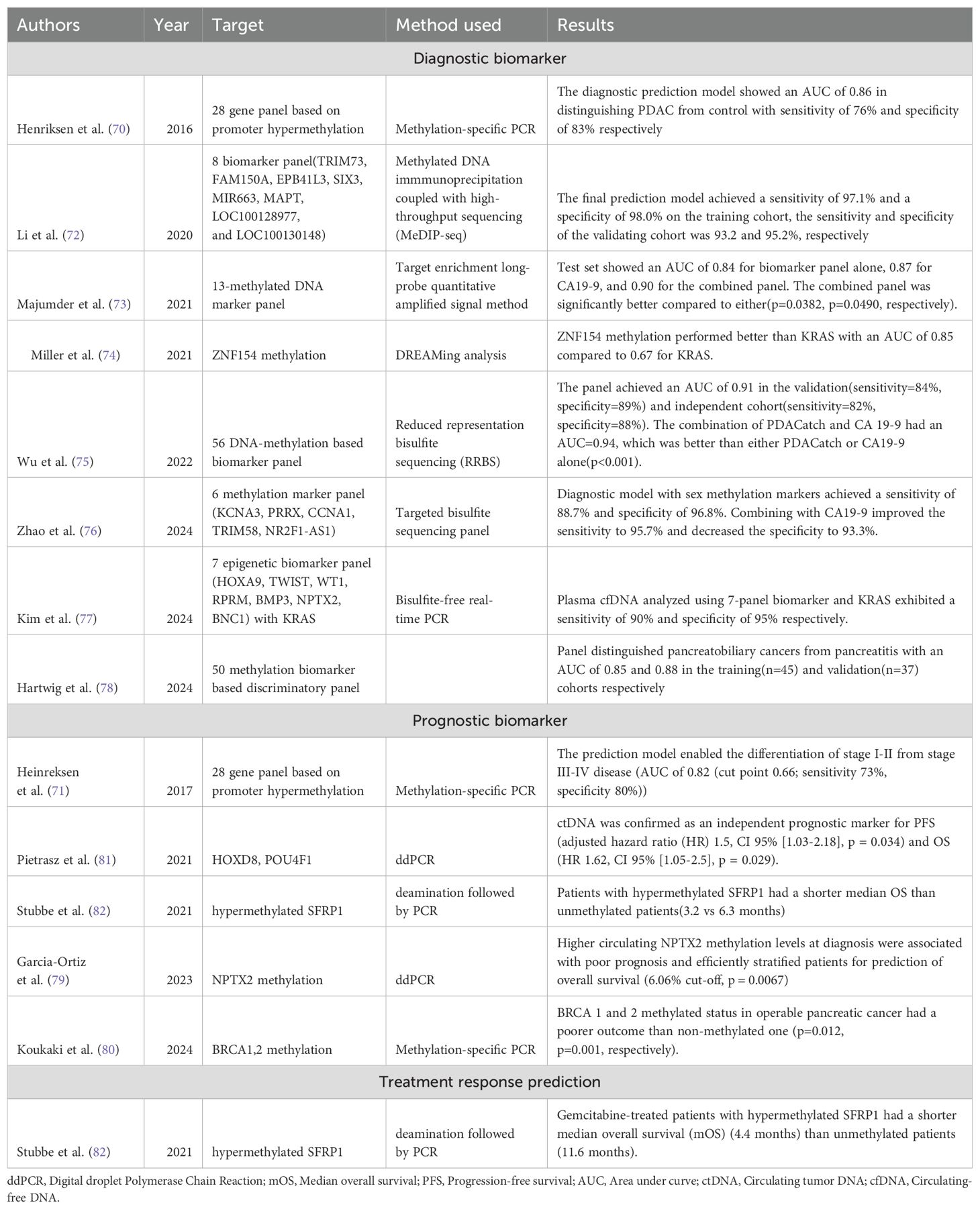
Table 2. Table showing the latest developments in diagnosis, prognosis, and treatment response prediction of PDAC using DNA methylation biomarkers.
A plethora of studies have been conducted in the last ten years that have furthered our understanding in the role of these DNA methylation biomarkers in the diagnosis of PDAC. One of the earlier studies assessing the role of DNA methylation in the diagnosis of PDAC was conducted by Henriksen et al. (70) in 2016 who employed a 28-gene panel based on promoter hypermethylation in PDAC, CP, and acute pancreatitis patients and created a diagnostic prediction model (age >65, BMP3, RASSF1A, BNC1, MESTv2, TFPI2, APC, SFRP1 and SFRP2) that had an area under the curve of 0.86 with a sensitivity of 76% and specificity of 83% respectively. The authors of that study also studied the 28 gene panel to create a prediction model (SEPT9v2, SST, ALX4, CDKN2B, HIC1, MLH1, NEUROG1, and BNC1) that enabled prognostication by differentiating stage 4 from stage 1-3 disease with an AUC of 0.87 and sensitivity, specificity of 74% and 87% respectively (71). Li et al. (72) performed a genome-wide cfDNA methylation profiling study utilizing immunoprecipitation with high-throughput sequencing and found a total of eight biomarkers that achieved a sensitivity and specificity of 97.1% and 98.0%, respectively, in the training cohort and 93.2% and 95.2% in the validation cohort. Majumder et al. (73) identified a panel of 13 sets of methylated biomarkers that were individually comparable to CA 19-9 and, when combined, performed superiorly compared to either with an AUC of 0.90 (p<0.05). Miller (74) assessed the utility of hypermethylation of the CpG island of ZNF154 as a diagnostic biomarker for multiple cancers, including colon, liver, ovarian, and pancreatic cancer patients. They collected 2711 peripheral blood samples and found that ZNF154 cfDNA methylation outperformed KRAS mutation with an AUC of 0.85 compared to 0.67 for KRAS and were particularly helpful in the diagnosis of early-stage disease (AUC=0.87). Wu et al. (75) developed a targeted methylation sequencing assay called PDACatch utilizing 546 plasma samples from 232 PDAC, 323 healthy controls and 25 patients with CP. Using a 56-marker classifier, they found that this panel differentiated PDAC from healthy controls (HC) and CP with an AUC of 0.91. Importantly, it detected CA19-9 negative PDAC at sensitivities of 75% and 100% in the validation and independent tests, respectively. Utilizing a combination of PDACatch and CA19-9 outperformed either with an AUC of 0.94 (p<0.001). Recently, Zhao et al. (76) identified a six-methylation marker based on liquid biopsy utilizing samples from 262 patients with PDAC and 212 HC and found that the panel achieved high sensitivity and specificity with 88.7% sensitivity for all cases of PDAC and 78% for stage 1 patients. The panel also had a high specificity of 96.8%. Combining the panel with CA19-9 improved the performance further, with a sensitivity of 95.7% and 95.5% for overall cases and stage 1 patients and a specificity of 96.8%. Kim et al. (77) assessed a 7-panel epigenetic biomarker panel developed from 46 patients with PDAC who underwent surgical resection in combination with KRAS and found that the panel showed a sensitivity of 90% and 95% specificity with an overall 93.3% accuracy in discriminating PDAC, which was comparable to 90% showed with CA19-9 and CEA. Hartwig et al. (78) utilized NGS followed by enrichment using methyl-binding domains to compare 25 cases each of PDAC, pancreatitis, controls and seven cases of IPMN and identified a 50 biomarker-based discriminatory panel that showed an AUC of 0.85 and 0.88, respectively, for the training and validation cohort and was also able to distinguish high grade from low-grade IPMN.
Epigenetic biomarkers have also shown great utility in assessing the prognosis of patients with PDAC. Garcia-Ortiz et al. (79) analyzed plasma samples from 44 patients with metastatic PDAC and found that NPTX2 methylation levels at diagnosis were associated with poor prognosis and a cut-off of 6.06% were significant in stratifying patients(p=0.0067) into low-risk (OS-410 days) versus high-risk (OS-187 days). Koukaki et al. (80) tested the applicability of BRCA1 and 2 methylation status in cfDNA as a prognostic biomarker in 55 patients with operable and 50 patients with metastatic pancreatic cancer. They found that in patients with operable pancreatic cancer, a methylated BRCA1 and 2 promoter status conferred a poorer outcome than a non-methylated one (p=0.012, p=0.001, respectively). One of the most important studies in this regard was done by Pietrasz et al. (81) when they prospectively collected 372 plasma samples based on 354 patients targeting two methylated markers (HOXD8 and POU4F1) and found that ctDNA positive for them was an independent prognostic biomarker for PFS (adjusted HR=1.5(1.03-2.18)) and OS(HR=1.62(1.05-2.5)).
While a number of studies have studied potentially useful diagnostic and prognostic biomarkers in DNA methylation for PDAC, a biomarker that can assist with treatment response prediction remains elusive. One such study was performed by Stubbe et al. (82) who exploited secreted frizzled-related protein 1 (SFRP1) hypermethylation as an independent prognostic biomarker for survival and gemcitabine effectiveness in patients with stage 4 PDAC. In a cohort of 40 patients, Stubbe found that hypermethylated SFRP1 was associated with a shorted median OS compared to unmethylated patients (3.2 vs 6.3 months, adjusted HR-3.53(1.85-6.74)). Furthermore, they found that gemcitabine-treated patients with hypermethylated SFRP1 had a shorter median OS of 4.4 months vs 11.6 months in unmethylated patients, thereby predicting response to gemcitabine (HR-3.48(1.39-8.70)). DNA methylation biomarkers represent an interesting prospect that holds great relevance and may become an important part of clinical practice in the following years.
Circulating tumor cells
CTCs are tumor cells that have separated from the primary tumor and are carried by the bloodstream or the lymphatic system (83). They were first described by Ashworth who had noticed that some cells in the blood of metastatic cancer resembled tumor cells in the primary tumors (84). Although CTCs derive their origin from primary tumours, they behave differently in some ways. The most important feature that distinguishes them from the primary tumour is the epithelial-mesenchymal transformation (EMT) properties that facilitate them to break from the primary tumour, intravasate into the bloodstream, disseminate in clusters of CTC that increase their ability to metastasize and demonstrate stem-cell-like properties (85). Studies have shown the existence of CTC even in the early stages of tumor evolution (86). Due to the EMT property of these cells, it is essential to detect tumor cells not only with epithelial markers available at the origin but also to include mesenchymal markers that improve the sensitivity of their assays. CTC monitoring essentially acts as a monitor for genomic instability and provides additional information with regard to tumor resistance and metastases, serving as a tool for treatment response and precision medicine (83). Molecular and pathological concordance of CTC with the primary tumor has been a topic of debate, with numerous studies showing mixed results.
Traditionally, CTCs were analyzed using FISH and flow cytometry (87). With the advances in molecular biology over the last decade and the advent of single-cell sequencing, it has become possible to conduct extensive genomic, transcriptomic, proteomic and DNA methylation analysis that has broadened our horizon and expanded the utility of CTC. Essentially, CTC technologies can be based on three principles-1) capture and enrichment, 2) detection and identification, and 3) release (88). Capture and enrichment involves interaction between CTC and materials either through physical interactions or antigen-antibody interactions. The second method involves the utilization of ultrasensitive techniques for small quantities of CTC through fluorescence microscopy, spectrophotometry, flow cytometry, electrical impedance, and Raman scattering (88). Released CTC finds utility in genomic, transcriptomic, proteomic analysis and CTC culture. Capture and enrichment based on physical properties often suffer from severe shortcomings, including being inefficient, impure, and lacking specificity. The only advantage is the decreased cost (89). Antigen-antibody-based capture and enrichment utilize EpCAM and vimentin to enrich CTC and negatively enrich CD45 to remove leucocytes (90). This technique has been widely utilized with the CellSearch system based on a similar principle (91). The problem with this system is that CTC are heterogenous with variable EpCAM expression that might lead to inaccurate results (92). New technologies for CTC analysis include nanotechnology-based techniques and microfluidic-based techniques (93, 94). These two techniques transcend the limitations posed by the earlier two techniques but have their own shortcomings. Microfluidic-based cell sorting has not been widely adopted because of the long set-up time, high initial cost, bulky instrumentation, and limited ability to perform single-cell molecular analysis (93). Nanotechnology-based techniques are cost-effective and simple. However, nanoparticle probes’ aggregation and binding can affect the results’ reliability and reproducibility (94).
Numerous studies have explored the role of CTC in PDAC diagnosis, prognosis and treatment prediction. Table 3 highlighting the studies assessing the diagnostic, prognostic and treatment response prediction properties of CTC has been given below
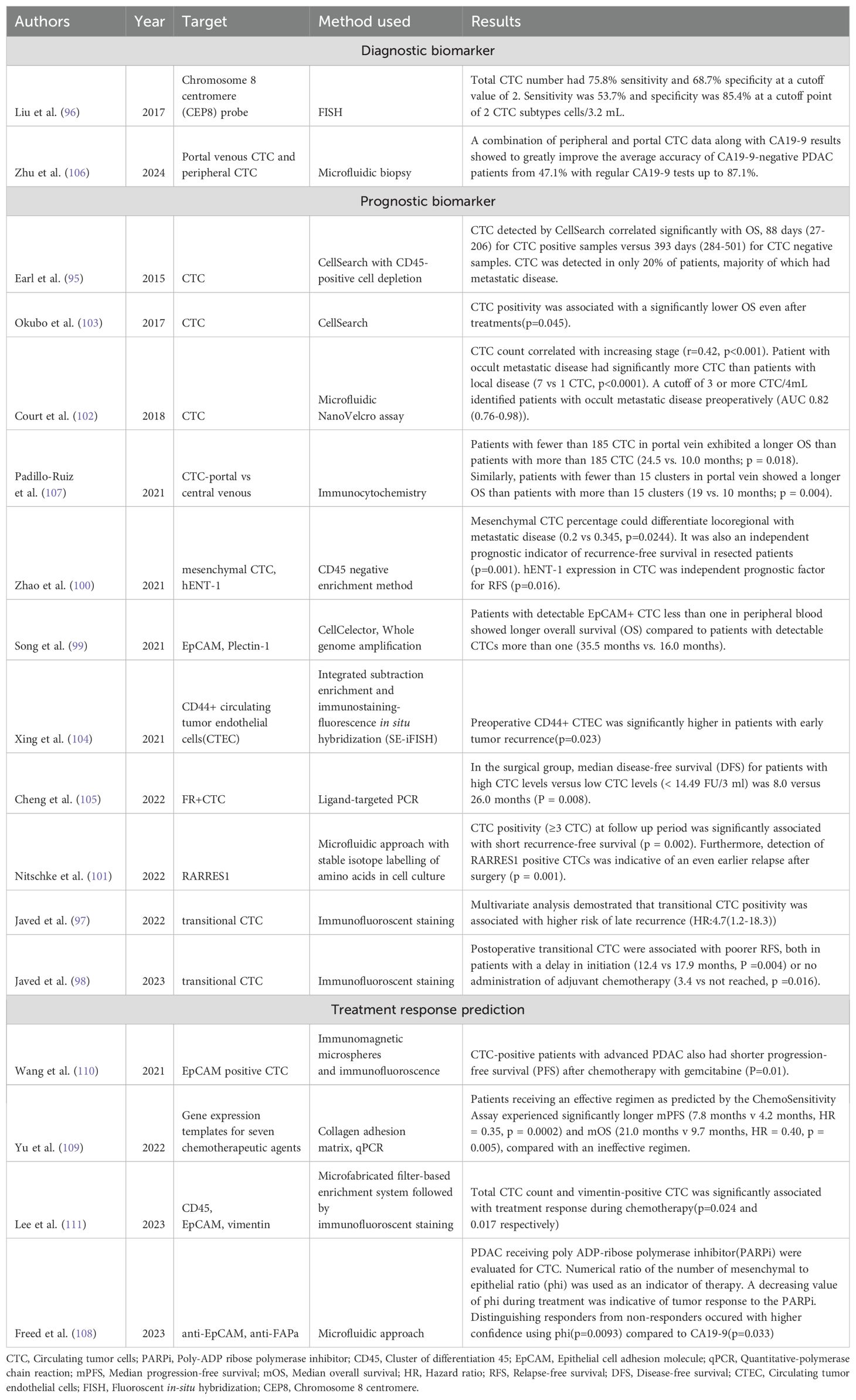
Table 3. Table showing the latest developments in diagnosis, prognosis, and treatment response prediction of PDAC using CTC biomarkers.
Earl et al. (95) simultaneously explored the role of CTC and KRAS mutant cfDNA in blood and found that the presence of CTC correlated significantly with OS, 88 days for CTC positive versus 393 days for CTC negative, although they were only detected in 20% of patients. Liu et al. (96) employed FISH to assess the subtype of CTC with chromosome 8 centromere probe in 143 blood samples from PDAC patients and healthy controls and found that both the subtype and total CTC were significantly increased in PDAC patients compared to healthy controls. Total CTC number had 75.8% sensitivity and 68.7% specificity at a cutoff value of 2 cells. 2 CTC subtypes as a cutoff showed a sensitivity of 53.7% and specificity of 85.4%. Javed et al. (97) used transitional CTC to assess recurrence risk one year postoperatively and found that positive transitional CTC was associated with a higher rate of late recurrence (HR=4.7(1.2-18.3)). One-year CTC positivity was associated with a higher rate of recurrence during the second year (OR=13.1(1.6-1953.4)). The same group later also studied the use of transitional CTC to study patients who had a delay in adjuvant therapy by greater than 8 weeks and found that the presence of transitional CTC was associated with a poorer relapse-free survival both in patients with a delay in initiation(p=0.004) as well as no receipt of adjuvant chemotherapy(p=0.016) (98). Song et al. (99) found that postoperative patients with detectable EpCAM-positive CTC of less than one in peripheral blood showed a longer overall survival (35.5 months vs 16.0 months). Zhao et al. (100) differentiated CTC into subtypes based on mesenchymal and human equilibrate nucleoside transporter-1(hENT-1) and found that mesenchymal CTC was detected in 81% of patients and it could differentiate locoregional disease from metastatic disease based on a percentage (p=0.0244). It was also an independent prognostic indicator of recurrence-free survival in resected patients(p=0.001). Nitschke et al. (101) employed RARRES1 expression in CTC using the technique of stable isotope labelling of amino acids in cell culture (SILAC) and concluded that CTC detection on follow-up was significantly associated with short recurrence-free survival(p=0.002), and presence of RARRES1 expression was indicative of an even earlier relapse after surgery(p=0.001). CTC used preoperatively has also been found to have significant implications on prognosis. Court et al. (102) preoperatively assessed CTC in 100 PDAC patients and found that a cut-off of 3 or more CTC in 4 mL correctly identified occult metastatic disease preoperatively (AUC-0.82(0.76-0.98)). Okubo et al. (103) assessed the use of CTC in 65 patients with advanced PDAC (borderline/unresectable) and found that the overall survival rate was significantly lower in patients with positive CTC (p=0.045). This confidence was accentuated further in borderline patients with CTC positivity(p=0.0051). It also served as an independent prognostic factor. Preoperative CD44+ circulating tumor endothelial cells (CTEC) have also been found to be significantly associated with early tumor recurrence (DFS<6 months, p=0.023), as demonstrated by Xing et al. (104). Similarly, folate receptor-positive CTCs have been studied by Cheng et al. (105) who found that there was a significantly decreased median disease-free survival (DFS) for patients with high CTC levels (8 vs 26 months, p=0.008). An area of great interest recently has been the isolation of CTC from portal venous samples, considering the greater proportion of CTC detected closer to the source. Zhu et al. (106) combined peripheral and portal venous CTC with CA 19-9 and found that this combined biomarker panel could improve the accuracy of CA19-9 negative PDAC from 47.1% to 87.1%. Furthermore, portal venous blood samples were found to have twice the number of CTC as peripheral blood (21.4 cells vs 10.9 cells per 5 mL). Padillo-Ruiz et al. (107) also explored this concept by comparing CTC in portal venous blood with a central venous catheter (CVC) and found that while there was no significant correlation observed in CVC analyses, there was a significantly increased OS in patients with less than 185 CTC (24.5 vs 10.0 months, p=0.018) for a sample drawn from the portal vein.
Further studies are required to explore the role of CTC in treatment response prediction in PDAC. One study was conducted by Freed et al. (108) when they assessed the response of PDAC patients on Poly ADP-ribose polymerase inhibitor (PARPi) therapy using a mesenchymal-epithelial CTC ratio(phi). They found that phi(p=0.0093) could differentiate responders and non-responders with higher confidence than CA 19-9(p=0.033). Further, for CA 19-9 non-producers, phi correctly predicted the outcome in 72% of PDAC patients. Yu et al. (109) utilized a chemosensitivity assay to determine an effective regimen based on FOLFOX and gemcitabine-paclitaxel regimen and found that the assay was able to predict an effective regimen with significantly longer PFS (7.8 months vs 4.2 months, HR=0.35, p=0.0002) and overall survival (21 months vs 9.7 months, HR=0.40, p=0.005). Gemcitabine resistance was also studied by Wang et al. (110), who found that CTC-positive patients with advanced PDAC had a shorter PFS after chemotherapy with gemcitabine, which was indicative of treatment resistance(p=0.01). Lee et al. (111) also studied the treatment response prediction ability of CTC and found that CTC count and subtype CTC vimentin positive significantly correlated with treatment response during chemotherapy (p=0.024, p=0.017). Research on CTC has been remarkable and shows great promise in clinical application of PDAC.
RNA biomarkers
The first instance of circulating free nucleic acids dates back to 1970s when they were isolated from the blood of cancer patients (112). It was initially hypothesized that these circulating free RNAs came from cancer cells. Later, as more research was done the origins of the circulating RNAs were attributed to necrosis and apoptosis of cancer cells and also thought to be actively secreted by the tumors in extracellular vesicles (113). During apoptosis, DNA, RNA, protein, and cellular organelles are packaged in the form of apoptotic bodies and released into circulation. Apoptotic bodies are a component of extracellular membrane vesicles (EMV), which play an important role in phagocytosis and the horizontal transfer of genes (114, 115). The other two parts of EMV include exosomes and microvesicles. Exosomes represent the smallest EMV actively secreted, while microvesicles are produced as a budding of the plasma membrane. Both of them play an important role in intracellular communication (114, 115). Kopreski et al. detected the first extracellular human mRNA in the blood of melanoma patients (116). Following this, multiple other studies found other forms of RNA in the serum or blood (117). RNA are relatively unstable molecules theoretically susceptible to degradation by the abundant ribonucleases in the blood. This is overcome by packaging RNA along with lipids and proteins in the form of vesicles to transport them outside the cell and protect it from outside degradation. The free RNA released into the circulation can be divided into coding RNA and non-coding RNA. Coding RNAs include messenger RNA (mRNA). mRNA are the protein-coding regions that contain the information produced from DNA transcription, which then undergo multiple processes, including 5 methyl capping, splicing, and 3 polyadenylation to produce refined mRNA finally. Analyzing cell-free circulating mRNA can prove an important cancer biomarker tool. Analysis of circulating mRNA provides the benefit of analysis of alternate and splicing variants through which epigenetic transcriptional regulation can be revealed. Human telomerase reverse transcriptase (hTERT) mRNA has been studied in multiple cancers. Telomerase activity and length correlates with cellular senescence (118). hTERT mRNA is upregulated in multiple cancers, including breast cancer, hepatocellular cancer, gastric cancer, and prostate cancer (118). Another notable mRNA that has been extensively studied includes epidermal growth, receptor (EGFR) which is overexpressed in the peripheral blood of patients with pancreatic, non-small cell lung, colon cancer and can be detected using RT-PCR (119, 120). Unfortunately, mRNA molecules typically tend to be between 300 and 4000 base pair in size and due to the long size along with the presence of high RNA activity in the blood, these mRNA molecules are subject to fregmentation, causing significant challenges in identifying biomarkers that have clinical utility. As a result, no reliable mRNA biomarkers have been found to date (121). On the other hand, noncoding RNA (ncRNA) has caused significant excitement in biomarker development. This includes transfer RNA(tRNA), ribosomal RNA(rRNA), micro RNA(miRNA), small interfering RNA(siRNA), piwi-interacting RNA(piRNA), small nuclear RNA(snRNA), small nucleolar RNA(snoRNA), YRNA, circular RNA, pseudogene RNA, and telomerase RNA (122). These ncRNAs vary based on size and function. The size ranges between 18-200 nucleotides long. tRNA and rRNA constitute the housekeeping non-coding RNA (ncRNA). On the other hand, regulatory ncRNA includes miRNA, snRNA and piRNA. The small ncRNA is involved in gene regulation, RNA interference, and spliceosome modification (123). Among these short ncRNA, miRNA have been the most extensively studied for biomarker development for different diseases. These miRNAs are around 20-25 nucleotide long oligonucleotides and have notably been associated with post-transcriptional silencing and expression of genes associated with mRNA (122). They also play an important role in cross-communication between cancer and dendritic cells (124). Besides these functions, they are vital in cell growth, maturation, prognosis, and proliferation, and their dysregulation can cause increased expression of oncogenes present downstream and facilitate cancer development (125). Multiple miRNA targets have also been found to predict the prognosis of malignancy (126).
Another extensively studied RNA includes long noncoding RNA (lnc RNA), which includes circular RNA, pseudogene RNA, and telomerase RNA, ranging from size 400 bp to 4kbp in length. These lncRNA are derived from exosomes and play an important role in metastasis, therapy resistance, angiogenesis, and tumor growth. They have also been known to modify tumor microenvironment, which facilitates cancer development and progression (127). An extensively studied field in lncRNAs include circular RNAs (circRNA). The circRNAs are produced by back splicing, unlike the usual linear RNA slicing process (128). They are considered as co-transcriptional products. Earlier, it was thought that the circRNAs have no role in eukaryotic gene expression. In the last couple of years, the role of circRNAs has been explained in greater detail. Major circRNA biogenesis is mediated by epithelial-mesenchymal transition (EMT) (129). It has also been found to act as miRNA and as latent competitive endogenous RNA molecules that compete with miRNA binding sites (130). circRNAs also consist of both intronic and exonic circRNA. Intronic circRNA can affect inheritance and epigenetics in the cytoplasm. Exonic circRNA can interact with miRNA and mediate miRNA function (129).
The most commonly employed methods for detecting circulating miRNA include quantitative Real-Time PCR (qRT-PCR), hybridization-based technology (Microarray), and high-throughput sequencing (NGS). Out of these three, qRT-PCR is the most widely adopted, largely related to its simple nature, allowing easy use and cost-efficient nature (131). Microarray and NGS, on the other hand, allow a large number of parallel analyses and are useful for genome-wide circulating miRNA profiling and high-throughput detection of circulating miRNA in body fluids. Microarrays are also quite flexible and can be tailored according to needs with a relatively straightforward concept and cheaper cost than NGS (132). The limitations with microarray are the large number of RNA samples needed and the technical variations in additional experimental steps that can affect external validity. As such, it tends to have a lower specificity than qRT-PCR and NGS. NGS is the most promising tool for high-throughput analysis. While qRT-PCR and microarray can only profile known miRNA, NGS quantifies a variety of small RNAs (including unknown ones) with a wide array (133). Deep sequencing facilitates the identification of mutations in miRNA. The high cost and the need for extensive computational infrastructure and bioinformatics support limit its universal adoption (134).
Most of the study surrounding RNA biomarkers in PDAC has been focused on miRNA and lnc RNA. Table 4 below highlights all the developments in the field of RNA biomarkers in the past few years. One of the largest studies attempted to study the diagnostic value of circulating miRNA in PDAC. It looked at 409 patients with PDAC, 25 patients with chronic pancreatitis and 312 healthy participants. A diagnostic index was developed based on miR–145, 150, 223, and 636. The sensitivity and specificity of the diagnostic miRNA panel combined with CA 19–9 was compared with CA 19–9 alone and found to have significantly increased AUC when compared to CA 19–9 alone (AUC 0.93 vs 0.89) (135). Since then, numerous studies have been conducted over the last decade to find out more potential RNA diagnostic and prognostic biomarkers in PDAC. Kim et al. (136) studied 55 patient samples and analyzed genome-wide expression of serum miRNA in PDAC and biliary tract cancer to identify novel biomarker candidates using sequencing techniques. Three of the highest performing miRNA (miR-744-5p, 409–3p, 128–3p) could distinguish cancer patients from controls with a diagnostic accuracy of 92.7%. An earlier study using miRNA and PDAC was done by Abue et al. (137), who checked the expression of miR-483-3p, and 21 in PDAC. They found that the mean plasma miR-483-3p and 21 were significantly higher in PDAC compared to IPMN and healthy controls. They also found that the presence of miR-21 was associated with lymph node and liver metastasis, suggesting advanced disease. This paved the way for future studies using miRNA biomarkers in PDAC clinical investigations.
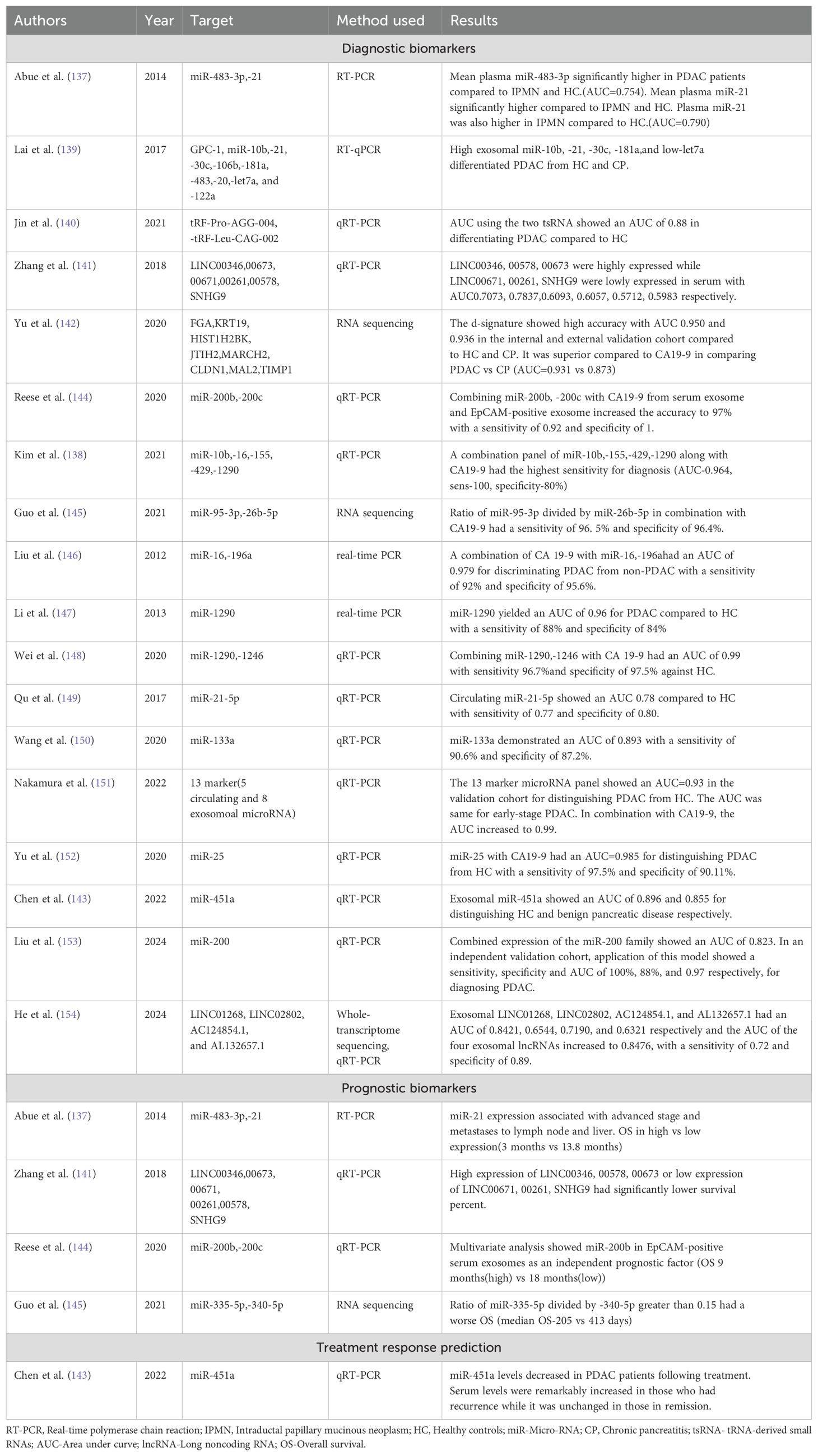
Table 4. Table showing the latest developments in diagnosis, prognosis, and treatment response prediction of PDAC using RNA biomarkers.
Another study conducted by Kim et al. (138) captured extracellular vesicles using magnetic beads and identified 5 miRNAs, including miR–10b, 16, 155, 429, and 1290 markedly elevated in PDAC. When combined with CA 19–9, the diagnostic panel reported a sensitivity of 100%, specificity of 80% and an AUC of 0.964. The biggest challenge in PDAC has been the ability to differentiate chronic pancreatitis from PDAC. Lai et al. (139) used miRNA signature and exosomal glypican to find a panel which can distinguish chronic pancreatitis from PDAC. They found that exosomal miRNA (high exosomal miR-10b, -21, -30c, -181a, low miR-let7a) was superior to exosomal glypican and CA19-9 in differentiating PDAC from chronic pancreatitis. Jin et al. (140) described another interesting study in which they did small RNA sequencing of 30 patients with PDAC and 30 healthy controls and found that more variations were detected among tRNA-derived small RNA (tsRNA) than miRNA. They used this finding to validate findings in 24 separated PDAC patients and healthy controls using the most significantly variable tsRNA and found two potential candidate tsRNA(tRF-Pro-AGG-004, tRF-Leu-CAG-002) with an AUC=0.88 in differentiating PDAC from healthy controls.
A substantial effort has also been made to explore the role of lncRNA in PDAC. Zhang et al. (141) studied the expression levels of six lncRNA in serum and tissues of patients with PDAC (LINC00346, LINC00578, LINC00673, LINC00671, LINC00261, and SNHG9). LINC00346, LINC00578, and LINC00673 were highly expressed, whereas LINC00671, LINC00261, and SNHG9 were expressed at lower levels when compared with healthy controls. Their expression levels also correlated with the clinical stage of the disease. Besides, those with higher and lower expression levels of the corresponding lncRNA also had a significantly lower OS. Yu et al. (142) explored another avenue when they conducted a case-control study with 501 participants (284 with PDAC, 100 with chronic pancreatitis (CP), and 117 healthy subjects). They performed an EV long RNA sequencing (evLR) and utilized a machine learning algorithm to develop a diagnostic signature of eight unique evLR(FGA, KRT19, HIST1H2BK, ITIH2, MARCH2, CLDN1, MAL2 and TIMP1). This panel of evLR showed a high diagnostic accuracy of distinguishing PDAC from healthy controls with an AUC of 0.960, 0.950 and 0.936 in the training, internal validation and external validation cohort, respectively. The signature also performed well in distinguishing early (stage 1 and 2) PDAC with an AUC of 0.949. When compared with CA19-9 in distinguishing PDAC from CP, the signature was significantly superior (AUC= 0.931 vs 0.873, p=0.028).
Research in this area needs to focus more on utilizing RNA biomarkers for treatment response prediction. Chen et al. (143) utilized serum miR-451 as a tool for treatment response. They found that miR-451 decreased to nearly undetectable levels after resection and treatment. After treatment, miR-451 also proved valuable as a surveillance tool to detect recurrence. A rebound increase in miR-451 during the surveillance period highly correlated with recurrence, while levels of patients in remission did not change considerably. miR-451 could also be used as a diagnostic biomarker and showed an AUC of 0.896 and 0.855 in distinguishing PDAC from healthy controls and benign pancreatic diseases, respectively. RNA biomarkers can be a game-changer in the management of PDAC. The challenges hindering the translation of miRNA and lncRNA into clinical practice include primarily the associated cost and technical expertise. Future research needs to focus on more biomarkers to guide treatment decisions and find ways to implement them in day-to-day clinical practice and lowering the associated cost.
Protein biomarkers
Proteins dynamically interact with each other having numerous intermolecular interactions and undergoing numerous posttranslational modifications. Their roles in modulation of molecular processes and pathways makes protein biomarkers relevant in tumorigenesis and progression (155). Liquid biopsy for proteins involves numerous technologies: Mass spectrometry (MS), antibody/antigen arrays, aptamer-based assays, proximity extension assays (PEA) and reverse phase protein arrays (RPPA) (156). This is in addition to traditional techniques such as ELISA, chemiluminescence and radioimmunoassay, which are cheaper and usually have a single target (157). MS-based proteomics is widely used these days in conjugation with liquid chromatography (LC) for liquid biopsy screening (158).MS technology allows large-scale untargeted proteomic and targeted analysis rapidly. Several studies have focused on protein profiling using the matrix-assisted laser desorption/ionisation time-of-flight (MALDI-TOF) and surface-enhanced laser desorption/ionisation time-of-flight (SELDI-TOF) (159, 160). MS, however, requires significant optimization and expertise and is often time-consuming. They may also be affected by abundant proteins, and many strategies have been implemented to deplete them, including ultrafiltration, solid phase and organic solvent extraction, and serum or plasma fractionation (161). While traditional ELISA is a singleplex assay, many contemporary multiplex methods have been developed that measure many target proteins over a wide range without the need for any depletion. These assays are dependent on specific antibodies or modified aptamers (162). Affinity-based protein profiling assays depend on antibodies or aptamers that recognize specific epitopes, without cross-reactivity to other proteins (162). There have been relatively few studies examining the performance of these assays, and it remains critical for proteomics-related liquid biopsy. While excellent as an exploratory tool, the scalability, inter-assay variation and costs of antibody/antigen arrays are often the rate-limiting steps in designing a study pipeline. Aptamers are short single-stranded DNA, RNA, or peptides that, upon folding into specific tertiary structures, bind to cognate protein targets in native states with high affinity and specificity (163). Aptamers have a higher affinity and specificity than antibodies, and can be readily synthesized and selected in vitro with low variation, allowing a cost-efficient way to make it scalable (163). It is a topic of great scope although aptamers available to the research communities are still limited compared to antibodies. In PEA multiple antibody pairs for proteins of interest are pooled and labelled with complementary DNA oligo sequences to allow high-fidelity hybridization (164). This happens only when true antibody pairs are in proximity to target proteins. The dsDNA is then PCR-amplified, allowing measurement of relative concentration of target proteins. PEA has outperformed LC-MS methods, presenting wider dynamic ranges with high accuracy and reproducibility (164, 165). The limitation is the tedious library preparation and NGS requirement which can contribute to biases and intra-inter-experimental variations when high sample size throughput is in place (164). Another technique that has captured attention is RPPA. In RPPA, fully denatured protein lysates are immobilized onto solid substrates, and this process can be repeated to allow any number of targets to be interrogated (166–168). RPPA allows robust parallel large sample profiling ranging from hundreds to a few thousand samples. RPPA is especially useful in blood cancer and other liquid biopsies due to its ability to track intra-cellular proteins (166). Limitations with RPPA involve sophisticated experimental workflow, prolonged experimental process (with more proteomics), slow turnaround time, and the validation of RPPA-usable antibodies
The quantitative measurement of proteome poses a greater challenge than assessing the genome. Around one million different proteins are present in the human body, through various combinations of epigenetic regulations, differential RNA splicing and post-translational modifications. In contrast, there are only 22,000-25,000 coding genes within the human genome (169). Proteins are in a constant state of flux, with rapid changes in abundance and dynamically modifying as a response to all kinds of environmental stimuli. The genome, in contrast, is relatively stable with slow rate of change.
Historically, there has been a great deal of emphasis on the utility of protein biomarkers for diagnosing and treating PDAC. Table 5 below highlights all the major developments in the field of protein biomarkers in the past few years.The most well-known serological biomarker in PDAC is carbohydrate antigen 19-9(CA19-9). CA19-9 has only been reported to be elevated in 80% of all patients with PDAC. It has also traditionally been utilized to monitor disease progress or treatment responsiveness. There can also be false positives with benign conditions such as chronic pancreatitis, biliary obstruction and cholangitis (170, 171). A meta-analysis conducted in 2018 to assess the diagnostic value of CA19-9 in PDAC had concluded the sensitivity of CA19-9 as 0.80 in the diagnosis of PDAC and a specificity of 0.75 with an area under the curve (AUC) of 0.84 (172). Another important biomarker discovered in the last decade is CA242. A meta-analysis comprising 21 studies and 3497 participants was conducted in 2015 to check the utility of cancer-antigen 242(CA242) in conjunction with CA19-9 and CEA in diagnosing PDAC. A sensitivity as high as 90% was achieved by combining CA19-9 with CA242 (173). Another study utilized a biomarker panel of CA19-9, serum periostin and CA242, which was able to distinguish early stage PDAC from controls with an AUC of 0.98 and benign conditions with an AUC of 0.90 (174).
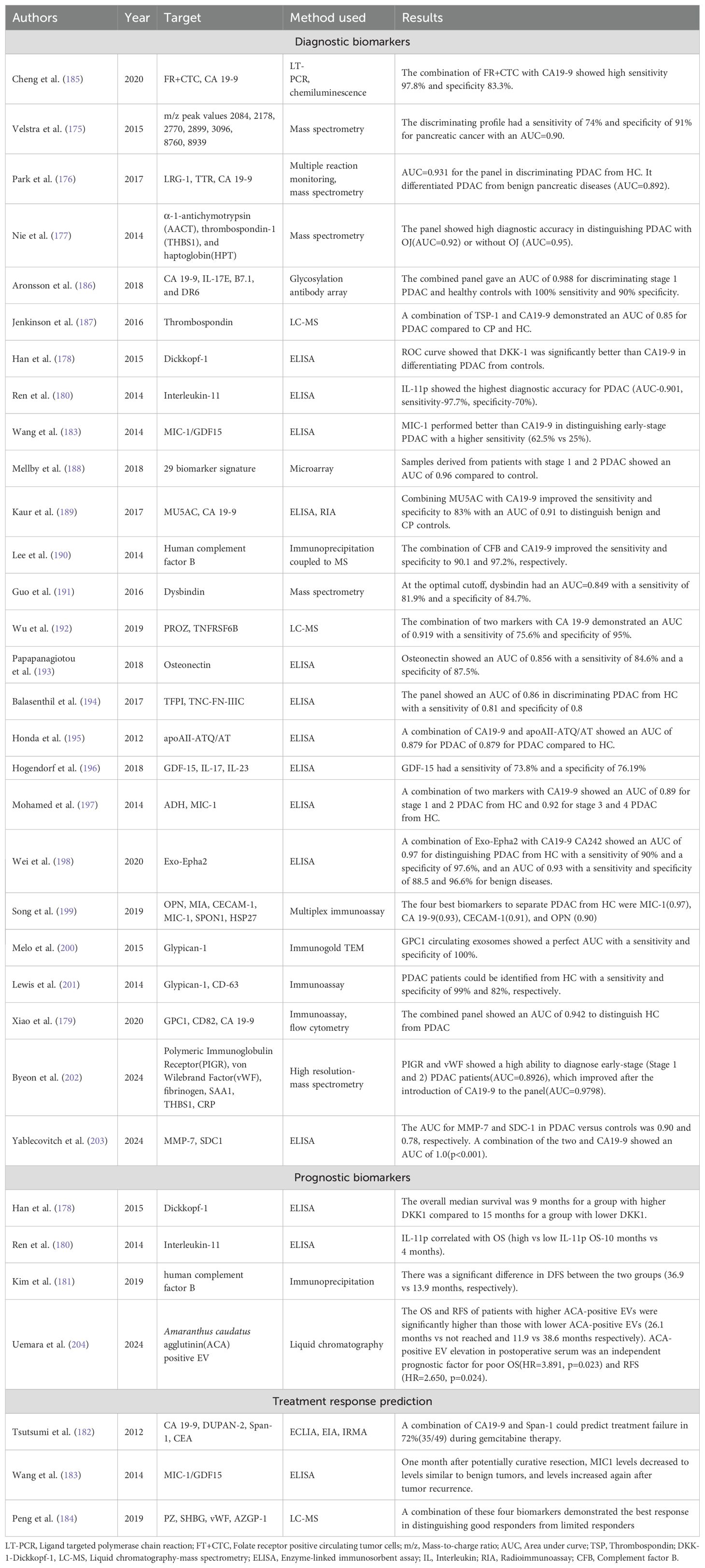
Table 5. Table showing the latest developments in diagnosis, prognosis, and treatment response prediction of PDAC using protein biomarkers.
Velstra et al. (175) performed one of the earliest biomarker analyses utilizing mass spectrometry when they utilized mass spectrometry peaks to identify a unique biomarker signature that could be utilized in PDAC. The discriminating profile demonstrated a sensitivity of 74% and a specificity of 91%in distinguishing PDAC from controls with an AUC of 0.90. Another exciting study utilizing mass spectrometry was done by Park et al. (176)when they identified a unique panel of protein biomarkers comprising leucine-rich alpha-2 glycoprotein (LRG1), transthyretin (TTR), and CA19-9 from a potential 1000 biomarkers from 134 clinical samples using mass spectrometry. The triple biomarker panel outperformed CA19-9 with an AUC of 0.931 compared to 0.826 for CA19-9 in diagnosing PDAC compared to controls. The superiority was even more prominent in differentiating PDAC from benign pancreatic disease and other cancers. Nie et al. (177) studied a combination of alpha-1-anti chymotrypsin, thrombospondin-1, and haptoglobin, which outperformed CA19-9 in distinguishing PDAC from normal controls, diabetes, cyst, and chronic pancreatitis. A combination of these markers was capable of distinguishing PDAC from other conditions with (AUC=0.92) or without obstructive jaundice (AUC=0.95). Dickkopf-1 (DKK1) was studied by Han et al. (178) as an alternative to CA 19-9 when they obtained serological levels of both the markers using ELISA and followed up the patient for two years to find that DKK1 was significantly better in distinguishing patients with PDAC from controls (AUC=0.919 vs 0.853). The study also found DKK1 as a useful prognostic biomarker, with the OS being 9 months for samples with higher DKK1 levels compared to 15 months for patients with lower DKK1 levels. Xiao et al. (179) have also explored the use of flow cytometry and immunoassay in the diagnosis of PDAC using a combined panel comprising glypican-1, CD82, and serum CA19-9. The combined panel effectively distinguished PDAC from healthy population (AUC-0.942). Ren et al. (180) have also explored the use of interleukin-11 as both a potential diagnostic and prognostic biomarker. On comparing the serum levels of IL-11 in 44 patients with PDAC with healthy controls, median baseline levels of IL-11 levels of patients with PDAC were significantly higher than those of healthy controls (p<0.001). IL-11 as a biomarker showed a sensitivity and specificity of 97.7% and 70.0% respectively with an AUC of 0.901 in distinguishing PDAC from healthy controls. Patients with distant metastases were found to have lower median levels of IL-11 and demonstrated a correlation with overall survival (lower than median IL-11 had 4 months compared to 10 months for those with higher median IL-11 levels). Another prognostic biomarker which has been studied in the past includes complement factor B(CFB). Stratifying patients on the cutoff value for CFB, Kim et al. (181) found that there was a significant difference in the DFS and OS for patients with low versus high CFB(DFS- 36.9 vs 13.9 months, p:0.007; OS-49.7 vs 29.0 months, p:0.048).
Numerous studies have also explored the use of protein biomarkers as a treatment response and prediction tool. One of the earliest studies to explore this was by Tsutsumi et al. (182), who explored the utility of tumor markers such as CEA, CA19-9, duke pancreatic mono-clonal antigen type 2(DUPAN-2) and s-pancreas antigen-1 (Span-1) to determine if it can assist in earlier confirmation of treatment failure. Their study yielded that a combination of CA 19-9 and Span-1 could facilitate a more accurate determination of treatment failure than CA 19-9, finding a failure in 72% of patients (p=0.004). Wang et al. (183) utilized macrophage inhibitory cytokine 1(MIC-1) as a treatment response monitoring tool and found that MIC-1 levels significantly decreased a month after curative resection in PDAC, and the levels rebounded whenever there was a tumour relapse that had occurred. As a diagnostic biomarker as well, MIC-1 performed better than CA19-9 showing a higher sensitivity (65.8% vs 53.3%). It was also more effective in distinguishing early-stage PDAC from normal serum compared to CA19-9(62.5% vs 25%). Peng et al. (184) utilized MS to identify a unique biomarker panel of PDAC patients with good response versus poor responders and found that a composite biomarker panel of PZ, SHBG, vWF, and CA 19-9 could segregate good responders from limited responders effectively.
While protein biomarkers still remain one of the most widely studied topics in PDAC, the approach suffers from a few shortcomings. Irrelevant clinical questions and underpowered designs falling short of statistical significance are major issues hindering protein biomarkers’ development. Other important factors that prevent the implementation of protein biomarkers involve the technology standards expected and needed for biomarker discovery and the lack of reproducibility in real-world settings. Despite all the above challenges, proteins represent an interesting avenue with vast potential that can be tapped into. CA19-9 represents a great success story in terms of PDAC, and it is hoped that more such biomarkers can be developed that find implementation and use in daily practice.
Blood-based multiomic biomarkers
The shortcomings in each set of biomarkers have spurred an interest in utilizing a combination of DNA, RNA and protein biomarkers to determine the diagnosis, prognosis and treatment response in PDAC. An interesting study was conducted by Cohen et al. (205)utilizing a combination of ctDNA (KRAS mutation), CEA, CA19-9, hepatocyte growth factor (HGF), osteopontin (OPN) through polymerase chain reaction and immunoassay and found that the combination biomarker panel increased the sensitivity to 64% and the specificity to 99.5%. Another groundbreaking study exploring the use of miRNA and protein biomarker panel was conducted by Madhavan et al. (206), who utilized a combination of flow cytometry and qRT-PCR to optimise a panel of miRNA and protein biomarkers that could detect PDAC with a sensitivity of 100% and specificity of 80%. One of the latest studies in this approach was conducted by Chen et al. (207) when they used PCR and chemiluminescence to use a combination of circulating tumour cells and CA19-9 as a diagnostic panel for PDAC and found that the combination demonstrated high sensitivity and specificity of 97.8% and 83.3% respectively.
Utilizing a combination approach while improving the detection rates and sensitivity sometimes adds the shortcomings of technical expertise and overhead expenses which come along with the addition of techniques. This can at present be only practiced in higher centers which are equipped with facilities and money to bear these expenses. Table 6 shows a summary of combination strategies utilizing DNA, RNA, protein biomarker studied in the management of PDAC.
Therapeutic applications and targeted therapy
Advanced technologies such as NGS have also facilitated the development of many targeted therapies based on a specific cell receptor/target/mutation. PDAC, based on the mutation status detected in NGS, can be divided into four subgroups: stable, locally rearranged, scattered, and unstable (208). Combining genomic and transcriptomic data with the relevant proteomic status allows a much more pragmatic classification of PDAC with therapeutic targets. Numerous oncogenes have been explored and evaluated for potential targets. The most commonly mutated oncogene in PDAC is KRAS. Ongoing phase 1 and 2 clinical trials are assessing the utility of MRTX849, a small molecule that selectively modifies mutant cysteine in KRAS G12C (209). First-generation EGFR inhibitors tried for this purpose, such as erlotinib and gefitinib, failed to show any substantial benefit (median HR=0.94, 95% confidence interval 0.76-1.15, p=0.26) (210). The lack of response was thought to be secondary to the resistance caused by the non-EGFR members of the ERBB family. To overcome this issue, irreversible tyrosine kinase inhibitors such as afatinib and neratinib were developed. Ongoing clinical trials (NCT02451553) assess afatinib’s efficacy in PDAC (211). Another EGFR inhibitor, nimotuzumab, was evaluated in locally advanced or metastatic PDAC in a phase 2 trial where the median OS was significantly improved with its use (OS=8.6 vs 6.0 months, HR=0.69, p=0.03) (212). Efforts have also been made to combine EGFR inhibitors with other pharmaceuticals to bypass the equivocal benefit seen with the use of a single agent. A phase 2 trial (NCT01222689) utilizing the combination of erlotinib plus selumetinib in metastatic PDAC showed modest antitumor activity with a median OS of 7.3 months (213).
Proteins downstream of KRAS, including RAF/PI3K/AKT/mTOR, have also been studied as potential targets of interest. MEK inhibitors, including selumetinib and trametinib, were tried in advanced PDAC but failed to yield significant results (selumetinib HR=1.03,p=0.92; trametinib HR=0.98, p=0.453) (214, 215). BKM120, a PI3K inhibitor combined with selumetinib, has shown promising results in murine models but is still nascent and requires further investigations (216).
The results have been slightly better when looking at targets beyond KRAS. A subsection of patients with KRAS harbour other mutations, such as NTRK and NRG1 (125). TRK inhibitors have recently entered the foray and include drugs including larocectinib and entrectinib found to have efficacy in PDAC harbouring these mutations (larocectinib response rate was 79%, and the entrectinib response rate was 57%) (217, 218).
TP53 is one of the most commonly inactivated tumor suppressors in PDAC, with the incidence found to be around 70% in individuals with PDAC (127). COTI-2, a TP53 reactivator drug, is currently being studied (NCT02433626) to evaluate its efficacy and utility in improving the prognosis of patients with TP53 mutant PDAC (219). Another potential target currently being studied involves CDK4/6. Inspired by the success of ribociclib and palbociclib in metastatic breast cancer and liposarcoma, they were tested in murine models of PDAC, where they demonstrated great promise, and they are currently also being evaluated in clinical trials (NCT02501902) (220). Another success story that derives its roots from breast cancer includes PARP inhibitors such as olaparib. A recent prospective phase 3 trial (NCT02184195) performed to evaluate the efficacy of olaparib in patients with mutant BRCA metastatic PDAC found that the PFS was increased significantly in the olaparib group (PFS 7.4 months versus 3.8 months, HR=0.53, p=0.004) (221).
Targeted therapy adds to the arsenal of options available at our disposal for treatment of PDAC. The success in targeted therapy especially in lung cancers and the rapid progression in techniques of liquid biopsy creates optimism with respect to improved treatment outcomes with targeted therapy in PDAC.
Future directions and conclusion
While numerous advances have been made in terms of biomarkers facilitating the diagnosis and prognosis of PDAC, few biomarkers have been explored which allow treatment response prediction. More effort needs to be focused on this area since they fit much more seamlessly into clinical practice and allow a greater understanding and clarity about the natural course of the disease. It also allows the oncologist to be prepared for failure and think about other options for treatment and allows patients to be better prepared about the consequences of the cancer. Utilizing a combination of genomics, transcriptomics and proteomics allows a greater sensitivity and certainty and gives greater discriminatory power to distinguish benign pancreatic diseases from PDAC. Efforts should be made to decrease the expenses associated with it so that the economic costs can be accommodated within the total treatment cost that is entailed with the diagnosis of PDAC. Biomarker discovery in PDAC holds great promise and utilizing it with imaging evidence and clinical suspicion may hold the solution to the conundrum of early diagnosis of PDAC allowing complete curative treatment of the disease. Figure 1 demonstrates an illustration highlighting the latest developments in DNA, RNA, and protein biomarkers.
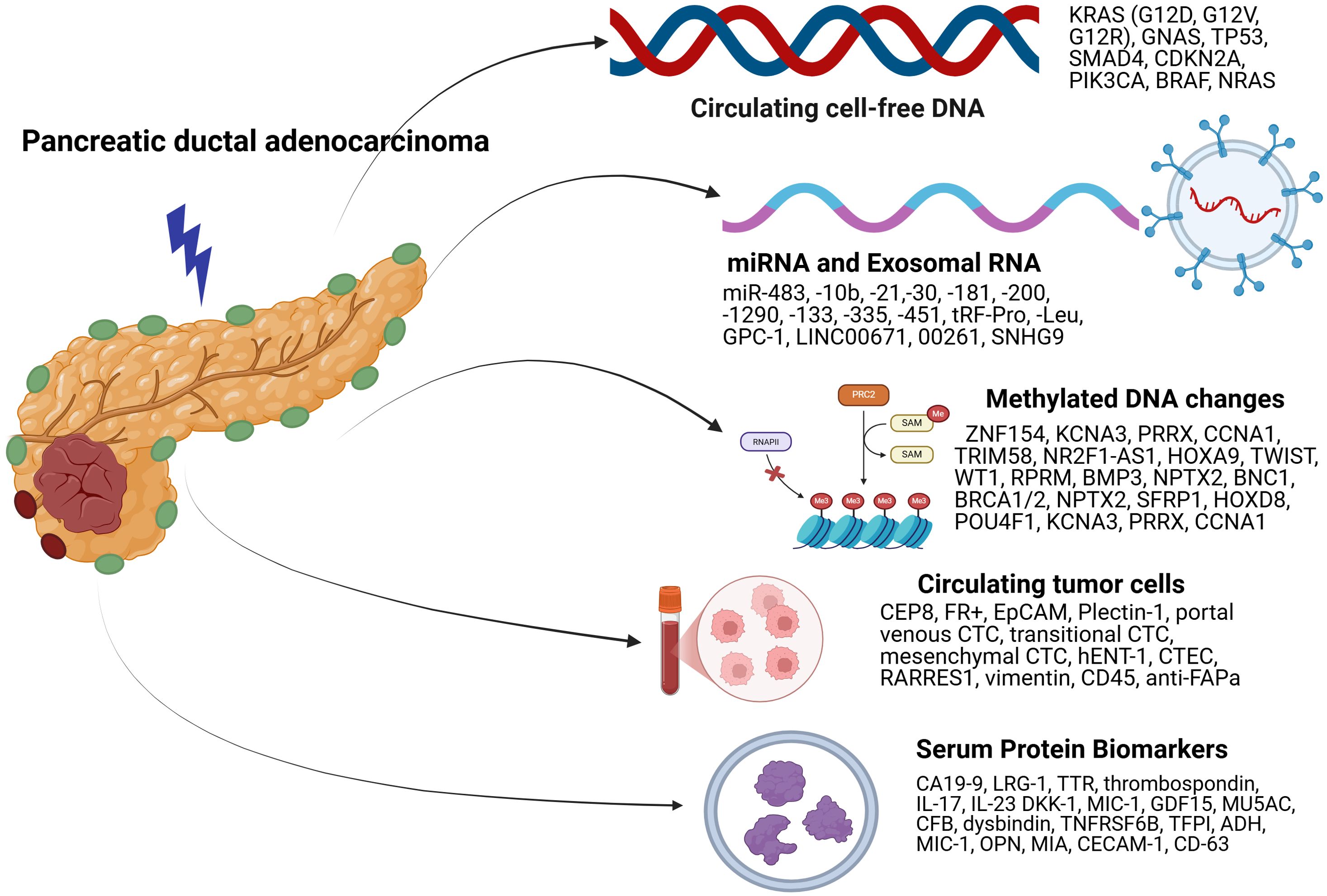
Figure 1. Illustration summarizing latest developments in serum DNA, RNA, CTC, DNA methylation, and protein biomarkers.
Author contributions
RJ: Writing – original draft, Writing – review & editing. AM: Conceptualization, Supervision, Writing – review & editing.
Funding
The author(s) declare that no financial support was received for the research and/or publication of this article.
Conflict of interest
The authors declare that the research was conducted in the absence of any commercial or financial relationships that could be construed as a potential conflict of interest.
Generative AI statement
The author(s) declare that no Generative AI was used in the creation of this manuscript.
Publisher’s note
All claims expressed in this article are solely those of the authors and do not necessarily represent those of their affiliated organizations, or those of the publisher, the editors and the reviewers. Any product that may be evaluated in this article, or claim that may be made by its manufacturer, is not guaranteed or endorsed by the publisher.
Abbreviations
PDAC, Pancreatic ductal adenocarcinoma; PaIN, Pancreatic intraepithelial neoplasms; DNA, Deoxyribonucleic acid; RNA, Ribonucleic acid cfDNA, Cell-free DNA; ctDNA, Circulating tumor DNA; CTC, Circulating tumor cells; AUC, Area Under Curve; NGS, Next Generation Sequencing; ddPCR, Digital Droplet Polymerase Chain Reaction; VAF, Variant Allele Frequency; FS, Progression-free Survival; OS, Overall Survival; cDCR, Complete Disease Control Rate; NAC, Neoadjuvant chemotherapy; EMV, Extracellular membrane vesicles; mRNA, Messenger RNA; tRNA, transfer RNA; hTERT, Human Telomerase Reverse Transcriptase; EGFR, Epidermal Growth Factor Receptor; ncRNA, Noncoding RNA; miRNA, Micro RNA; snRNA, Small Nuclear RNA; piRNA, Piwi-interacting RNA; lnc RNA, Long noncoding RNA; circRNA, Circular RNA; evLR, EV long RNA sequencing; CP, Chronic Pancreatitis; HC, Healthy Controls; RT-PCR-Real-time Polymerase Chain Reaction; LC-MS, Liquid Chromatography-Mass Spectrometry; ELISA, Enzyme-linked Immunosorbent Assay.
References
1. Siegel RL, Giaquinto AN, Jemal A. Cancer statistics, 2024. CA: A Cancer J Clin. (2024) 74:12–49. Available online at: https://www.cancer.org/research/cancer-facts-statistics/all-cancer-facts-figures/2024-cancer-facts-figures.html (Accessed March 11, 2025).
2. Facts about pancreatic cancer. American Cancer Society. (2025). Available online at: https://www.cancer.org/cancer/types/pancreatic-cancer/about/key-statistics.html (Accessed March 11, 2025).
3. Rawla P, Sunkara T, Gaduputi V. Epidemiology of pancreatic cancer: global trends, etiology and risk factors. World J Oncol. (2019) 10(1):10–27. (Accessed March 11,2025). doi: 10.14740/wjon1166
4. Amin MB, Greene FL, Edge SB, Compton CC, Gershenwald JE, Brookland RK, et al. The Eighth Edition AJCC Cancer Staging Manual: Continuing to build a bridge from a population-based to a more “personalized” approach to cancer staging. CA: A Cancer J Clin. (2017) 67(2):93–9. doi: 10.3322/caac.21388
5. Isaji S, Mizuno S, Windsor JA, Bassi C, Fernández-del Castillo C, Hackert T, et al. International consensus on definition and criteria of borderline resectab le pancreatic ductal adenocarcinoma 2017. Pancreatology. (2018) 18(1):2–11. (Accessed March 11, 2025). doi: 10.1016/j.pan.2017.11.011
6. Sens MA, Zhou XD, Weiland T, Cooley AM. Unexpected neoplasia in autopsies: potential implications for tissue and organ safety. Arch Pathol Lab Med. (2009) 133(12):1923–31. Available online at (Accessed March 11, 2025). doi: 10.5858/133.12.1923
7. Cruz LA, Young AP, Mack T, Ruffin I. Diagnosis and management of pancreatic cancer. Am Family Physician. (2014) 89:626–32. Available online at: https://www.aafp.org/pubs/afp/issues/2014/0415/p626.html (Accessed March 11, 2025).
8. Peschke K, Jakubowsky H, Schäfer A, Maurer C, Lange S, Orben F, et al. dentification of treatment-induced vulnerabilities in pancreatic cancer patients using functional model systems.EMBO Mol Med. (2022) 14(4). (Accessed March 11, 2025). doi: 10.15252/emmm.202114876
9. Søreide K, Ismail W, Roalsø M, Ghotbi J, Zaharia C. Early diagnosis of pancreatic cancer: clinical premonitions, timely precursor detection and increased curative-intent surgery. Cancer Control. (2023) 30. (Accessed March 11,2025). doi: 10.1177/10732748231154711
10. Marrugo-Ramírez J, Mir M, Samitier J. Blood-based cancer biomarkers in liquid biopsy: A promising non-invasive alternative to tissue biopsy. Int J Mol Sci. (2018) 19(10). (Accessed March 11. 2025). doi: 10.3390/ijms19102877
11. Robertson EG, Baxter G. Tumour seeding following percutaneous needle biopsy: the real story. Clin Radiol. (2011) 66(11):1007–14. (Accessed March 11, 2025). doi: 10.1016/j.crad.2011.05.012
12. Crowley E, di Nicolantonio F, Loupakis F, Bardelli A. Liquid biopsy: monitoring cancer-genetics in the blood. Nat Rev Clin Oncol. (2013) 10(8):472–84. (Accessed March 11, 2025). doi: 10.1038/nrclinonc.2013.110
13. Diehl F, Schmidt K, Choti MA, Romans K, Goodman S, Li M, et al. Circulating mutant DNA to assess tumor dynamics. Nat Med. (2008) 14(9):985–90. (Accessed March 11, 2025). doi: 10.1038/nm.1789
14. Batool SM, Yekula A, Khanna P, Hsia T, Gamblin AS, Ekanayake E, et al. The Liquid Biopsy Consortium: Challenges and opportunities for early cancer detection and monitoring. Cell Rep Med. (2023) 4(10):101198. doi: 10.1016/j.xcrm.2023.101198
15. Tadimety A, Closson A, Li C, Yi S, Shen T, Zhang JXJ. Advances in liquid biopsy on-chip for cancer management: Technologies, biomarkers, and clinical analysis. Crit Rev Clin Lab Sci. (2018) 55(3):140–62. (Accessed March 11, 2025). doi: 10.1080/10408363.2018.1425976
16. Chen X, Ba Y, Ma L, Cai X, Yin Y, Wang K, et al. Characterization of microRNAs in serum: a novel class of biomarkers for diagnosis of cancer and other diseases. Cell Res. (2008) 18(10):997–1006. (Accessed March 11, 2025) 2008. doi: 10.1038/cr.2008.282
17. Wroclawski ML, Serpa-Neto A, Fonseca FLA, Castro-Neves-Neto O, Pompeo ASFL, MaChado MT, et al. Cell-free plasma DNA as biochemical biomarker for the diagnosis and follow-up of prostate cancer patients. Tumour Biol. (2013) 34(5):2921–7. Available online at (Accessed March 11, 2025). doi: 10.1007/s13277-013-0854-4
18. Schwarzenbach H, Stoehlmacher J, Pantel K, Goekkurt E. Detection and monitoring of cell-free DNA in blood of patients with colorectal cancer. Ann NY Acad Sci. (2008) 1137:190–6. (Accessed March 11, 2025). doi: 10.1196/annals.1448.025
19. Analysis of Circulating Tumor DNA in Plasma at Diagnosis and during Follow-Up of Lung Cancer Patients1, in: Cancer Research. American Association for Cancer Research. Available online at: https://aacrjournals.org/cancerres/article/61/12/4675/507505/Analysis-of-Circulating-Tumor-DNA-in-Plasma-at (Accessed March 11, 2025).
20. Cristofanilli M, Budd GT, Ellis MJ, Stopeck A, Matera J, Miller MC, et al. Circulating tumor cells, disease progression, and survival in metastatic breast cancer. N Engl J Med. (2004) 351(8):781–91. (Accessed March 11, 2025). doi: 10.1056/NEJMoa040766
21. Hagn G, Meier-Menches SM, Plessl-Walder G, Mitra G, Mohr T, Preindl K, et al. Plasma instead of serum avoids critical confounding of clinical metabolomics studies by platelets. J Proteome Res. (2024) 23(8):3064–75. (Accessed March 11, 2025). doi: 10.1021/acs.jproteome.3c00761
22. Pittella-Silva F, Chin YM, Chan HT, Nagayama S, Miyauchi E, Low SK, et al. Plasma or serum: which is preferable for mutation detection in liquid biopsy? Clin Chem. (2020) 66(7):946–57. (Accessed March 11, 2025). doi: 10.1093/clinchem/hvaa103
23. Sheel A, Addison S, Nuguru SP, Manne A. Is cell-free DNA testing in pancreatic ductal adenocarcinoma ready for prime time? Cancers. (2022) 14(14). (Accessed March 11, 2025). doi: 10.3390/cancers14143453
24. Sorbini M, Carradori T, Togliatto GM, Vaisitti T, Deaglio S. Technical advances in circulating cell-free DNA detection and analysis for personalized medicine in patients’ Care. Biomolecules. (2024) 14(4). (Accessed March 11, 2025). doi: 10.3390/biom14040498
25. Chung DC, Gray DM, Singh H, Issaka RB, Raymond VM, Eagle C, et al. A cell-free DNA blood-based test for colorectal cancer screening. N Engl J Med. (2024) 390(11):973–83. (Accessed March 11, 2025). doi: 10.1056/NEJMoa2304714
26. Váraljai R, Elouali S, Lueong SS, Wistuba-Hamprecht K, Seremet T, Siveke JT, et al. The predictive and prognostic significance of cell-free DNA concentration in melanoma. J Eur Acad Dermatol Venereol. (2021) 35(2):387–95. (Accessed March 11, 2025). doi: 10.1111/jdv.16766
27. Bunduc S, Gede N, Váncsa S, Lillik V, Kiss S, Dembrovszky F, et al. Prognostic role of cell-free DNA biomarkers in pancreatic adenocarcinoma: A systematic review and meta-analysis. Crit Rev Oncol Hematol. (2022) 169. (Accessed March 11, 2025). doi: 10.1016/j.critrevonc.2021.103548
28. Shapiro B, Chakrabarty M, Cohn EM, Leon SA. Determination of circulating DNA levels in patients with benign or malignant gastrointestinal disease. Cancer. (1983) 51:2116–20. doi: 10.1002/1097-0142(19830601)51:11<2116::aid-cncr2820511127>3.0.co;2-s
29. George B, Haberberger J, Ferguson NL, Gjoerup O, McGregor K, Hendifar AE, et al. Correlation between comprehensive genomic profiling (CGP) utilizing tissue-based testing (T-CGP) and cell-free DNA (cfDNA) in patients (pts) with pancreatic ductal adenocarcinoma (PDAC). J Clin Oncol. (2021) 39(3_suppl):422–422. (Accessed March 11, 2025). doi: 10.1200/JCO.2021.39.3_suppl.422
30. Chung J, Xiao S, Gao Y, Soung YH. Recent technologies towards diagnostic and therapeutic applications of circulating nucleic acids in colorectal cancers. Int J Mol Sci. (2024) 25(16). (Accessed March 11, 2025). doi: 10.3390/ijms25168703
31. Zhang B, Xu CW, Shao Y, Wang HT, Wu YF, Song YY, et al. Comparison of droplet digital PCR and conventional quantitative PCR for measuring EGFR gene mutation. Exp Ther Med. (2015) 9(4):1383. (Accessed March 11, 2025). doi: 10.3892/etm.2015.2221
32. Garciá-Foncillas J, Alba E, Aranda E, Diáz-Rubio E, López-López R, Tabernero J, et al. Incorporating BEAMing technology as a liquid biopsy into clinical practice for the management of colorectal cancer patients: an expert taskforce review. Ann Oncol. (2017) 28(12):2943–9. (Accessed March 11, 2025). doi: 10.1093/annonc/mdx501
33. Newman AM, v. BS, To J, JF W, Eclov NCW, LA M, et al. An ultrasensitive method for quantitating circulating tumor DNA with broad patient coverage. Nat Med. (2014) 20(5):548–54. (Accessed March 11, 2025). doi: 10.1038/nm.3519
34. Forshew T, Murtaza M, Parkinson C, Gale D, Tsui DWY, Kaper F, et al. Noninvasive identification and monitoring of cancer mutations by targeted deep sequencing of plasma DNA. Science Transl Med. (2012) 4(136). (Accessed March 11, 2025). doi: 10.1126/scitranslmed.3003726
35. Imperial R, Nazer M, Ahmed Z, Kam AE, Pluard TJ, Bahaj W, et al. Matched whole-genome sequencing (WGS) and whole-exome sequencing (WES) of tumor tissue with circulating tumor DNA (ctDNA) analysis: complementary modalities in clinical practice. Cancers. (2019) 11(9). (Accessed March 11, 2025). doi: 10.3390/cancers11091399
36. Milin-Lazovic J, Madzarevic P, Rajovic N, Djordjevic V, Milic N, Pavlovic S, et al. Meta-analysis of circulating cell-free dna’s role in the prognosis of pancreatic cancer. Cancers. (2021) 13(14):3378. doi: 10.3390/cancers13143378
37. Ankeny JS, Court CM, Hou S, Li Q, Song M, Wu D, et al. Circulating tumour cells as a biomarker for diagnosis and staging in pancreatic cancer. (2016). Br J Cancer. (2016) 114(12):1367–75. doi: 10.1038/bjc.2016.121
38. Serial cell-free DNA. (cfDNA) sampling in advanced pancreatic ductal adenocarcinoma (PDAC) patients may predict therapeutic outcome. ASCO. Available online at: https://www.asco.org/posters/POSTER323325 (Accessed March 11, 2025).
39. Herreros-Villanueva M, Bujanda L, Ruiz-Rebollo L, Torremocha R, Ramos R, Martín R, et al. Circulating tumor DNA tracking in patients with pancreatic cancer using next-generation sequencing. Gastroenterol Hepatol. (2022) 45(8):637–44. (Accessed March 11, 2025). doi: 10.1016/j.gastrohep.2021.12.011
40. Sellahewa R, Moghaddam SM, Lundy J, Jenkins BJ, Croagh D. Circulating tumor DNA is an accurate diagnostic tool and strong prognostic marker in pancreatic cancer. Pancreas. (2023) 52(3):E188–95. doi: 10.1097/MPA.0000000000002239
41. Wang R, Zhao Y, Wang Y, Zhao Z, Chen Q, Duan Y, et al. Diagnostic and prognostic values of KRAS mutations on EUS-FNA specimens and circulating tumor DNA in patients with pancreatic cancer. Clin Transl Gastroenterol. (2022) 13(5). (Accessed March 11, 2025). doi: 10.14309/ctg.0000000000000487
42. van der Pol Y, Moldovan N, Ramaker J, Bootsma S, Lenos KJ, Vermeulen L, et al. The landscape of cell-free mitochondrial DNA in liquid biopsy for cancer detection. Genome Biol. (2023) 24(1). (Accessed March 11, 2025). doi: 10.1186/s13059-023-03074-w
43. Evrard C, Ingrand P, Rochelle T, Martel M, Tachon G, Flores N, et al. Circulating tumor DNA in unresectab le pancreatic cancer is a strong predictor of first-line treatment efficacy: The KRASCIPANC prospective study. Dig Liver Dis. (2023) 55(11):1562–72. (Accessed March 11, 2025). doi: 10.1016/j.dld.2023.03.011
44. Umemoto K, Sunakawa Y, Ueno M, Furukawa M, Mizuno N, Sudo K, et al. Clinical significance of circulating-tumour DNA analysis by metastatic sites in pancreatic cancer. Br J Cancer. (2023) 128(8):1603–8. (Accessed March 11, 2025). doi: 10.1038/s41416-023-02189-y
45. Kitahata Y, Kawai M, Hirono S, Miyazawa M, Motobayashi H, et al. Circulating tumor DNA as a potential prognostic marker in patients with borderline-resectab le pancreatic cancer undergoing neoadjuvant chemotherapy followed by pancreatectomy. Ann Surg Oncol. (2022) 29(3):1596–605. (Accessed March 11, 2025). doi: 10.1245/s10434-021-10985-0
46. Hata T, Mizuma M, Motoi F, Ohtsuka H, Nakagawa K, Morikawa T, et al. Prognostic impact of postoperative circulating tumor DNA as a molecular minimal residual disease marker in patients with pancreatic cancer undergoing surgical resection. J Hepatobiliary Pancreat Sci. (2023) 30(6):815–24. (Accessed March 11, 2025). doi: 10.1002/jhbp.1282
47. Shah D, Wells A, Cox M, Dawravoo K, Abad J, D’Souza A, et al. Prospective Evaluation of Circulating Tumor DNA using Next Generation Sequencing as a Biomarker during Neoadjuvant Chemotherapy in Localized Pancreatic Cancer. Ann Surg. (2024). (Accessed March 11, 2025). Available from: https://pubmed.ncbi.nlm.nih.gov/38258582/. doi: 10.1097/SLA.0000000000006209
48. Lim DH, Yoon H, Kim KP, Ryoo BY, Lee SS, Park DH, et al. Analysis of plasma circulating tumor DNA in borderline resectab le pancreatic cancer treated with neoadjuvant modified FOLFIRINOX: clinical relevance of DNA damage repair gene alteration detection. Cancer Res Treat. (2023) 55(4):1313–20. (Accessed March 11, 2025). doi: 10.4143/crt.2023.452
49. Eckhoff AM, Kanu E, Fletcher A, Bao M, Aushev VN, Spickard E, et al. Initial report: personalized circulating tumor DNA and survival in patients with resectab le pancreatic cancer. Ann Surg Oncol. (2024) 31(3):1444–6. (Accessed March 11, 2025). doi: 10.1245/s10434-023-14751-2
50. Edland KH, Tjensvoll K, Oltedal S, Dalen I, Lapin M, Garresori H, et al. Monitoring of circulating tumour DNA in advanced pancreatic ductal adenocarcinoma predicts clinical outcome and reveals disease progression earlier than radiological imaging. Mol Oncol. (2023) 17(9):1857–70. (Accessed March 11, 2025). doi: 10.1002/1878-0261.13472
51. Sudo K, Nakamura Y, Ueno M, Furukawa M, Mizuno N, Kawamoto Y, et al. Clinical utility of BRCA and ATM mutation status in circulating tumour DNA for treatment selection in advanced pancreatic cancer. Br J Cancer. (2024) 131(7). (Accessed March 11, 2025). doi: 10.1038/s41416-024-02834-0
52. Motobayashi H, Kitahata Y, ichi OK, Miyazawa M, Ueno M, Hayami S, et al. Short-term serial circulating tumor DNA assessment predicts therapeutic efficacy for patients with advanced pancreatic cancer. J Cancer Res Clin Oncol. (2024) 150(2). (Accessed March 11, 2025). doi: 10.1007/s00432-023-05594-1
53. Huang L, Lv Y, Guan S, Yan H, Han L, Wang Z, et al. High somatic mutations in circulating tumor DNA predict response of metastatic pancreatic ductal adenocarcinoma to first-line nab-paclitaxel plus S-1: prospective study. J Transl Med. (2024) 22(1). (Accessed March 11, 2025). doi: 10.1186/s12967-024-04989-z
54. Till JE, McDaniel L, Pfeiffer SM, Maurer DM, Yu J, Spencer C, et al. Circulating KRAS variant-specific shedding and association with survival in patients with metastatic pancreatic ductal adenocarcinoma (mPDAC) receiving chemoimmunotherapy. J Clin Oncol. (2022) 40(16_suppl):2548–2548. doi: 10.1200/JCO.2022.40.16_suppl.2548
55. Hálková T, Bunganič B, Traboulsi E, Minárik M, Zavoral M, Benešová L. Prognostic role of specific KRAS mutations detected in aspiration and liquid biopsies from patients with pancreatic cancer. Genes. (2024) 15(10). (Accessed March 11, 2025). doi: 10.3390/genes15101302
56. Maulat C, Canivet C, Cabarrou B, Pradines A, Selves J, Casanova A, et al. Prognostic impact of circulating tumor DNA detection in portal and peripheral blood in resected pancreatic ductal adenocarcinoma patients. Sci Rep. (2024) 14(1):1–14. doi: 10.1038/s41598-024-76903-y
57. Shen SY, Singhania R, Fehringer G, Chakravarthy A, Roehrl MHA, Chadwick D, et al. Sensitive tumour detection and classification using plasma cell-free DNA methylomes. Nature. (2018) 563(7732):579–83. doi: 10.1038/s41586-018-0703-0
58. Cristiano S, Leal A, Phallen J, Fiksel J, Adleff V, Bruhm DC, et al. Genome-wide cell-free DNA fragmentation in patients with cancer. Nature. (2019) 570(7761):385. doi: 10.1038/s41586-019-1272-6
59. Qi T, Pan M, Shi H, Wang L, Bai Y, Ge Q. Cell-free DNA fragmentomics: the novel promising biomarker (2023). (Accessed March 11, 2025). doi: 10.3390/ijms24021503
60. Lee JS, Kim M, Seong MW, Kim HS, Lee YK, Kang HJ. Plasma vs. serum in circulating tumor DNA measurement: characterization by DNA fragment sizing and digital droplet polymerase chain reaction. Clin Chem Lab Med. (2020) 58(4):527–32. doi: 10.1515/cclm-2019-0896
61. Liu X, Liu L, Ji Y, Li C, Wei T, Yang X, et al. Enrichment of short mutant cell-free DNA fragments enhanced detection of pancreatic cancer. EBioMedicine. (2019) 41:345–56. (Accessed March 11, 2025). doi: 10.1016/j.ebiom.2019.02.010
62. Zvereva M, Roberti G, Durand G, Voegele C, Nguyen MD, Delhomme TM, et al. Circulating tumour-derived KRAS mutations in pancreatic cancer cases are predominantly carried by very short fragments of cell-free DNA. EBioMedicine. (2020) 55. (Accessed March 11, 2025). doi: 10.1016/j.ebiom.2019.09.042
63. Shi X, Guo S, Duan Q, Zhang W, Gao S, Jing W, et al. Detection and characterization of pancreatic and biliary tract cancers using cell-free DNA fragmentomics. J Exp Clin Cancer Res. (2024) 43(1). (Accessed March 11, 2025). doi: 10.1186/s13046-024-03067-y
64. Grady WM, Yu M, Markowitz SD. Epigenetic alterations in the gastrointestinal tract: Current and emerging use for biomarkers of cancer. Gastroenterology. (2020) 160(3):690. (Accessed March 11, 2025). doi: 10.1053/j.gastro.2020.09.058
65. Brancaccio M, Natale F, Falco G, Angrisano T. Cell-free DNA methylation: the new frontiers of pancreatic cancer biomarkers’ Discovery. Genes. (2020) 11:14. doi: 10.3390/genes11010014
66. Baylin SB, Jones PA. Epigenetic Determinants of Cancer. Cold Spring Harb Perspect Biol. (2016) 8(9):a019505. doi: 10.1101/cshperspect.a019505
67. Huang J, Wang L. Cell-free DNA methylation profiling analysis-technologies and bioinformatics. Cancers (Basel). (2019) 11(11):1741. doi: 10.3390/cancers11111741
68. Lehmann-Werman R, Neiman D, Zemmour H, Moss J, Magenheim J, Vaknin-Dembinsky A, et al. Identification of tissue-specific cell death using methylation patterns of circulating DNA. Proc Natl Acad Sci USA. (2016) 113(13):E1826–34. (Accessed March 11, 2025). doi: 10.1073/pnas.1519286113
69. Li W, Zhang X, Lu X, You L, Song Y, Luo Z, et al. 5-Hydroxymethylcytosine signatures in circulating cell-free DNA as diagnostic biomarkers for human cancers. Cell Res. (2017) 27(10):1243–57. doi: 10.1038/cr.2017.121
70. Henriksen SD, Madsen PH, Larsen AC, Johansen MB, Drewes AM, Pedersen IS, et al. Cell-free DNA promoter hypermethylation in plasma as a diagnostic marker for pancreatic adenocarcinoma. Clin Epigenetics. (2016) 8(1). (Accessed March 11, 2025). doi: 10.1186/s13148-016-0286-2
71. Henriksen SD, Madsen PH, Larsen AC, Johansen MB, Pedersen IS, Krarup H, et al. Cell-free DNA promoter hypermethylation in plasma as a predictive marker for survival of patients with pancreatic adenocarcinoma. Oncotarget. (2017) 8:93942–56. doi: 10.18632/oncotarget.21397
72. Li S, Wang L, Zhao Q, Wang Z, Lu S, Kang Y, et al. Genome-wide analysis of cell-free DNA methylation profiling for the early diagnosis of pancreatic cancer. Front Genet. (2020) 11. (Accessed March 11, 2025). doi: 10.3389/fgene.2020.596078
73. Majumder S, Taylor WR, Foote PH, Berger CK, Wu CW, Mahoney DW, et al. High detection rates of pancreatic cancer across stages by plasma assay of novel methylated DNA markers and CA19-9. Clin Cancer Res. (2021) 27(9):2523–32. (Accessed March 11, 2025). doi: 10.1158/1078-0432.CCR-20-0235
74. Miller BF, Petrykowska HM, Elnitski L. Assessing ZNF154 methylation in patient plasma as a multicancer marker in liquid biopsies from colon, liver, ovarian and pancreatic cancer patients. Sci Rep. (2021) 11(1). (Accessed March 11, 2025). doi: 10.1038/s41598-020-80345-7
75. Wu H, Guo S, Liu X, Li Y, Su Z, He Q, et al. Noninvasive detection of pancreatic ductal adenocarcinoma using the methylation signature of circulating tumour DNA. BMC Med. (2022) 20(1). (Accessed March 11, 2025). doi: 10.1186/s12916-022-02647-z
76. Zhao G, Jiang R, Shi Y, Gao S, Wang D, Li Z, et al. Circulating cell-free DNA methylation-based multi-omics analysis allows early diagnosis of pancreatic ductal adenocarcinoma. Mol Oncol. (2024) 18(11). (Accessed March 11, 2025). doi: 10.1002/1878-0261.13643
77. Kim H, Chu J, Do IG, Lee YP, Kim HK, Yang Y, et al. Novel diagnostic biomarkers for pancreatic cancer: assessing methylation status with epigenetic-specific peptide nucleic acid and KRAS mutation in cell-free DNA. Front Oncol. (2024) 14. (Accessed March 11, 2025). doi: 10.3389/fonc.2024.1395473
78. Hartwig C, Müller J, Klett H, Kouhestani D, Mittelstädt A, Anthuber A, et al. iscrimination of pancreato-biliary cancer and pancreatitis patients by non-invasive liquid biopsy. Mol Cancer. (2024) 23(1). (Accessed March 11, 2025). doi: 10.1186/s12943-024-01943-x
79. García-Ortiz MV, Cano-Ramírez P, Toledano-Fonseca M, Cano MT, Inga-Saavedra E, Rodríguez-Alonso RM, et al. Circulating NPTX2 methylation as a non-invasive biomarker for prognosis and monitoring of metastatic pancreatic cancer. Clin Epigenetics. (2023) 15(1). (Accessed March 11, 2025). doi: 10.1186/s13148-023-01535-4
80. Koukaki T, Balgkouranidou I, Biziota E, Karayiannakis A, Bolanaki H, Karamitrousis E, et al. Prognostic significance of BRCA1 and BRCA2 methylation status in circulating cell-free DNA of Pancreatic Cancer patients. J Cancer. (2024) 15(9):2573–9. (Accessed March 11, 2025). doi: 10.7150/jca.93184
81. Pietrasz D, Wang-Renault S, Taieb J, Dahan L, Postel M, Durand-Labrunie J, et al. Prognostic value of circulating tumour DNA in metastatic pancreatic cancer patients: post-hoc analyses of two clinical trials. Br J Cancer. (2022) 126(3). (Accessed March 11, 2025). doi: 10.1038/s41416-021-01624-2
82. Stubbe BE, Henriksen SD, Madsen PH, Larsen AC, Krarup HB, Pedersen IS, et al. Validation of SFRP1 promoter hypermethylation in plasma as a prognostic marker for survival and gemcitabine effectiveness in patients with stage IV pancreatic adenocarcinoma. Cancers. (2021) 13(22). (Accessed March 11, 2025). doi: 10.3390/cancers13225717
83. Lin D, Shen L, Luo M, Zhang K, Li J, Yang Q, et al. Circulating tumor cells: biology and clinical significance. Signal Transduct Target Ther. (2021) 6(1):1–24. doi: 10.1038/s41392-021-00817-8
84. Ashworth TR. A case of cancer in which cells similar to those in the tumors were seen in the blood after death. Australas Med J. (1869) 14:146–9.
85. Pantel K, Speicher MR. The biology of circulating tumor cells. Oncogene. (2016) 35(10):1216–24. doi: 10.1038/onc.2015.192
86. Bettegowda C, Sausen M, Leary RJ, Kinde I, Wang Y, Agrawal N, et al. Detection of circulating tumor DNA in early- and late-stage human Malignancies. Sci Transl Med. (2014) 6(224). doi: 10.1126/scitranslmed.3007094
87. Mayer JA, Pham T, Wong KL, Scoggin J, v. SE, Clarin T, et al. FISH-based determination of HER2 status in circulating tumor cells isolated with the microfluidic CEE™ platform. Cancer Genet. (2011) 204:589–95. doi: 10.1016/j.cancergen.2011.10.011
88. Ju S, Chen C, Zhang J, Xu L, Zhang X, Li Z, et al. Detection of circulating tumor cells: opportunities and challenges. Biomark Res. (2022) 10(1):1–25. (Accessed March 11, 2025). doi: 10.1186/s40364-022-00403-2
89. Vona G, Sabile A, Louha M, Sitruk V, Romana S, Schutze K, et al. Isolation by size of epithelial tumor cells: A new method for the immunomorphological and molecular characterization of circulating tumor cells. Am J Pathol. (2000) 156(1):57–63. doi: 10.1016/S0002-9440(10)64706-2
90. Sajay BNG, Chang CP, Ahmad H, Khuntontong P, Wong CC, Wang Z, et al. Microfluidic platform for negative enrichment of circulating tumor cells. Biomed Microdevices. (2014) 16(4):537–48. (Accessed March 11, 2025). doi: 10.1007/s10544-014-9856-2
91. Wang C, Mu Z, Ye Z, Zhang Z, Abu-Khalaf MM, Silver DP, et al. Prognostic value of HER2 status on circulating tumor cells in advanced-stage breast cancer patients with HER2-negative tumors. Breast Cancer Res Treat. (2020) 181(3):679–89. (Accessed March 11, 2025). doi: 10.1007/s10549-020-05662-x
92. Allard WJ, Matera J, Miller MC, Repollet M, Connelly MC, Rao C, et al. Tumor Cells Circulate in the Peripheral Blood of All Major Carcinomas but not in Healthy Subjects or Patients With Nonmalignant Diseases. Clin Cancer Res. (2004) 10(20):6897–904. (Accessed March 11, 2025). doi: 10.1158/1078-0432.CCR-04-0378
93. Nasiri R, Shamloo A, Ahadian S, Amirifar L, Akbari J, Goudie MJ, et al. Microfluidic-based approaches in targeted cell/particle separation based on physical properties: fundamentals and applications. Small. (2020) 16(29):e2000171. doi: 10.1002/smll.202000171
94. Zhang Y, Li M, Gao X, Chen Y, Liu T. Nanotechnology in cancer diagnosis: Progress, challenges and opportunities. J Hematol Oncol. (2019) 12(1):1–13. (Accessed March 11, 2025). doi: 10.1186/s13045-019-0833-3
95. Earl J, Garcia-Nieto S, Martinez-Avila JC, Montans J, Sanjuanbenito A, Rodríguez-Garrote M, et al. Circulating tumor cells (Ctc) and kras mutant circulating free Dna (cfdna) detection in peripheral blood as biomarkers in patients diagnosed with exocrine pancreatic cancer. BMC Cancer. (2015) 15(1). (Accessed March 11, 2025). doi: 10.1186/s12885-015-1779-7
96. Liu H, Sun B, Wang S, Liu C, Lu Y, Li D, et al. Circulating tumor cells as a biomarker in pancreatic ductal adenocarcinoma. Cell Physiol Biochem. (2017) 42(1):373–82. (Accessed March 11, 2025). doi: 10.1159/000477481
97. Javed AA, Ding D, Hasanain A, van Oosten F, Yu J, Cameron JL, et al. Persistent circulating tumor cells at 1 year after oncologic resection predict late recurrence in pancreatic cancer. Ann Surg. (2023) 277(6):859–65. (Accessed March 11, 2025). doi: 10.1097/SLA.0000000000005708
98. Javed AA, Floortje Van Oosten A, Habib JR, Hasanain A, Kinny-Köster B, Gemenetzis G, et al. A delay in adjuvant therapy is associated with worse prognosis only in patients with transitional circulating tumor cells after resection of pancreatic ductal adenocarcinoma. Ann Surg. (2023) 277(6):866–72. (Accessed March 11, 2025). doi: 10.1097/SLA.0000000000005710
99. Song BG, Kwon W, Kim H, Lee EM, Han YM, Kim H, et al. Detection of circulating tumor cells in resectab le pancreatic ductal adenocarcinoma: A prospective evaluation as a prognostic marker. Front Oncol. (2021) 10. (Accessed March 11, 2025). doi: 10.3389/fonc.2020.616440
100. Zhao X, Ma Y, Dong X, Zhang Z, Tian X, Zhao X, et al. Molecular characterization of circulating tumor cells in pancreatic ductal adenocarcinoma: potential diagnostic and prognostic significance in clinical practice. Hepatobiliary Surg Nutr. (2021) 10(6):796–810. (Accessed March 11, 2025). doi: 10.21037/hbsn-20-383
101. Nitschke C, Markmann B, Tölle M, Kropidlowski J, Belloum Y, Goetz MR, et al. Characterization of RARRES1 expression on circulating tumor cells as unfavorable prognostic marker in resected pancreatic ductal adenocarcinoma patients. Cancers. (2022) 14(18). (Accessed March 11, 2025). doi: 10.3390/cancers14184405
102. Court CM, Ankeny JS, Sho S, Winograd P, Hou S, Song M, et al. Circulating tumor cells predict occult metastatic disease and prognosis in pancreatic cancer. Ann Surg Oncol. (2018) 25(4):1000–8. (Accessed March 11, 2025). doi: 10.1245/s10434-017-6290-8
103. Okubo K, Uenosono Y, Arigami T, Mataki Y, Matsushita D, Yanagita S, et al. Clinical impact of circulating tumor cells and therapy response in pancreatic cancer. Eur J Surg Oncol. (2017) 43(6):1050–5. (Accessed March 11, 2025). doi: 10.1016/j.ejso.2017.01.241
104. Xing C, Li Y, Ding C, Wang S, Zhang H, Chen L, et al. CD44+ Circulating tumor endothelial cells indicate poor prognosis in pancreatic ductal adenocarcinoma after radical surgery: A pilot study. Cancer Manag Res. (2021) 13:4417–31. (Accessed March 11, 2025). doi: 10.2147/CMAR.S309115
105. Cheng H, Yang J, Fu X, Mao L, Chu X, Lu C, et al. Folate receptor-positive circulating tumor cells predict survival and recurrence patterns in patients undergoing resection for pancreatic cancer. Front Oncol. (2022) 12. (Accessed March 11, 2025). doi: 10.3389/fonc.2022.1012609
106. Zhu Z, Zhang Y, Zhang W, Tang D, Zhang S, Wang L, et al. High-throughput enrichment of portal venous circulating tumor cells for highly sensitive diagnosis of CA19-9-negative pancreatic cancer patients using inertial microfluidics. Biosens Bioelectron. (2024) 259. (Accessed March 11, 2025). doi: 10.1016/j.bios.2024.116411
107. Padillo-Ruiz J, Suarez G, Pereira S, Calero-Castro FJ, Tinoco J, Marin L, et al. Circulating tumor cells enumeration from the portal vein for risk stratification in early pancreatic cancer patients. Cancers. (2021) 13(24). (Accessed March 11, 2025). doi: 10.3390/cancers13246153
108. Freed IM, Kasi A, Fateru O, Hu M, Gonzalez P, Weatherington N, et al. Circulating tumor cell subpopulations predict treatment outcome in pancreatic ductal adenocarcinoma (PDAC) patients. Cells. (2023) 12(18). (Accessed March 11, 2025). doi: 10.3390/cells12182266
109. Yu KH, Park J, Mittal A, Abou-Alfa GK, Dika I, AS E, et al. Circulating tumor and invasive cell expression profiling predicts effective therapy in pancreatic cancer. Cancer. (2022) 128(15):2958–66. (Accessed March 11, 2025). doi: 10.1002/cncr.34269
110. Wang X, Hu L, Yang X, Chen F, Xu H, Yu H, et al. Clinical prognostic value of circulating tumor cells in the treatment of pancreatic cancer with gemcitabine chemotherapy. Exp Ther Med. (2021) 22(4). (Accessed March 11, 2025). doi: 10.3892/etm.2021.10574
111. Lee HS, Jung EH, Shin H, Park CS, Park SB, Jung DE, et al. Phenotypic characteristics of circulating tumor cells and predictive impact for efficacy of chemotherapy in patients with pancreatic cancer: a prospective study. Front Oncol. (2023) 13. (Accessed March 11, 2025). doi: 10.3389/fonc.2023.1206565
112. Leon SA, Shapiro B, Sklaroff DM, Yaros MJ. Free DNA in the serum of cancer patients and the effect of therapy. Cancer Res. (1977) 37:646–50.
113. Yuan A, Farber EL, Rapoport AL, Tejada D, Deniskin R, Akhmedov NB, et al. Transfer of microRNAs by embryonic stem cell microvesicles. PLoS One. (2009) 4(3). (Accessed March 11, 2025). doi: 10.1371/journal.pone.0004722
114. Lázaro-Ibáñez E, Sanz-Garcia A, Visakorpi T, Escobedo-Lucea C, Siljander P, Ayuso-Sacido Á, et al. Different gDNA content in the subpopulations of prostate cancer extracellular vesicles: apoptotic bodies, microvesicles, and exosomes. Prostate. (2014) 74(14):1379–90. (Accessed March 11, 2025). doi: 10.1002/pros.22853
115. Yamamoto S, Azuma E, Muramatsu M, Hamashima T, Ishii Y, Sasahara M. Significance of extracellular vesicles: pathobiological roles in disease. Cell Struct Funct. (2016) 41(2):137–43. (Accessed March 11, 2025). doi: 10.1247/csf.16014
116. Detection of tumor messenger RNA in the serum of patients with Malignant melanoma, in: Clinical cancer research. American Association for Cancer Research. Available online at: https://aacrjournals.org/clincancerres/article/5/8/1961/287789/Detection-of-Tumor-Messenger-RNA-in-the-Serum-of (Accessed March 11, 2025).
117. Taylor DD, Gercel-Taylor C. MicroRNA signatures of tumor-derived exosomes as diagnostic biomarkers of ovarian cancer. Gynecol Oncol. (2008) 110(1):13–21. doi: 10.1016/j.ygyno.2008.04.033
118. Lawrie CH, Gal S, Dunlop HM, Pushkaran B, Liggins AP, Pulford K, et al. Detection of elevated levels of tumour-associated microRNAs in serum of patients with diffuse large B-cell lymphoma. Br J Haematol. (2008) 141(5):672–5. (Accessed March 11, 2025). doi: 10.1111/j.1365-2141.2008.07077.x
119. Telomerase RNA as a detection marker in the serum of breast cancer patients1, in: Clinical cancer research. American Association for Cancer Research. Available online at: https://aacrjournals.org/clincancerres/article/6/10/3823/287914/Telomerase-RNA-as-a-Detection-Marker-in-the-Serum (Accessed March 11, 2025).
120. Kirkpatrick KL, Clark G, Ghilchick M, Newbold RF, Mokbel K. hTERT mRNA expression correlates with telomerase activity in human breast cancer. Eur J Surg Oncol. (2003) 29(4):321–6. (Accessed March 11, 2025). doi: 10.1053/ejso.2002.1374
121. Larson MH, Pan W, Kim HJ, Mauntz RE, Stuart SM, Pimentel M, et al. comprehensive characterization of the cell-free transcriptome reveals tissue- and subtype-specific biomarkers for cancer detection. Nat Commun. (2021) 12(1). (Accessed March 11, 2025). doi: 10.1038/s41467-021-22444-1
122. Chaddha M, Rai H, Gupta R, Thakral D. Integrated analysis of circulating cell free nucleic acids for cancer genotyping and immune phenotyping of tumor microenvironment. Front Genet. (2023) 14:1138625. doi: 10.3389/fgene.2023.1138625
123. Dozmorov MG, Giles CB, Koelsch KA, Wren JD. Systematic classification of non-coding RNAs by epigenomic similarity. BMC Bioinformatics. (2013) 14(SUPPL.14):1–12. (Accessed March 11, 2025). doi: 10.1186/1471-2105-14-S14-S2
124. Wang J, Yue BL, Huang YZ, Lan XY, Liu WJ, Chen H. Exosomal RNAs: novel potential biomarkers for diseases-A review. Int J Mol Sci. (2022) 23(5). (Accessed March 11, 2025). doi: 10.3390/ijms23052461
125. Cheung KWE, Choi S yu R, Lee LTC, Lee NLE, Tsang HF, Cheng YT, et al. The potential of circulating cell free RNA as a biomarker in cancer. Expert Rev Mol Diagn. (2019) 19(7):579–90. (Accessed March 11, 2025). doi: 10.1080/14737159.2019.1633307
126. Weber JA, Baxter DH, Zhang S, Huang DY, Huang KH, Lee MJ, et al. The microRNA spectrum in 12 body fluids. Clin Chem. (2010) 56(11):1733–41. Available online at (Accessed March 11, 2025). doi: 10.1373/clinchem.2010.147405
127. Roshani M, Baniebrahimi G, Mousavi M, Zare N, Sadeghi R, Salarinia R, et al. Exosomal long non-coding RNAs: novel molecules in gastrointestinal cancers’ progression and diagnosis. Front Oncol. (2022) 12:1014949. doi: 10.3389/fonc.2022.1014949
128. Zhang XO, bin WH, Zhang Y, Lu X, LL C, Yang L. Complementary sequence-mediated exon circularization. Cell. (2014) 159(1):134–47. (Accessed March 11, 2025). doi: 10.1016/j.cell.2014.09.001
129. Zhao ZJ, Shen J. Circular RNA participates in the carcinogenesis and the Malignant behavior of cancer. RNA Biol. (2015) 14(5):514. doi: 10.1080/15476286.2015.1122162
130. Taulli R, Loretelli C, Pandolfi PP. From pseudo-ceRNAs to circ-ceRNAs: a tale of cross-talk and competition. Nat Struct Mol Biol. (2013) 20(5):541–3. (Accessed March 11, 2025). doi: 10.1038/nsmb.2580
131. Moldovan L, Batte KE, Trgovcich J, Wisler J, Marsh CB, Piper M. Methodological challenges in utilizing miRNAs as circulating biomarkers. J Cell Mol Med. (2014) 18(3):371–90. (Accessed March 12, 2025). doi: 10.1111/jcmm.12236
132. Liu CG, Calin GA, Volinia S, Croce CM. MicroRNA expression profiling using microarrays. Nat Protoc. (2008) 3(4):563–78. (Accessed March 12, 2025). doi: 10.1038/nprot.2008.14
133. Pritchard CC, Cheng HH, Tewari M. MicroRNA profiling: approaches and considerations. Nat Rev Genet. (2012) 13(5):358–69. (Accessed March 12, 2025). doi: 10.1038/nrg3198
134. Chen M, Zhao H. Next-generation sequencing in liquid biopsy: cancer screening and early detection. Hum Genomics. (2019) 13(1):34. (Accessed March 12, 2025). doi: 10.1186/s40246-019-0220-8
135. Schultz NA, Dehlendorff C, v. JB, JK B, KR N, SE B, et al. MicroRNA biomarkers in whole blood for detection of pancreatic cancer. JAMA. (2014) 311(4):392–404. (Accessed March 12, 2025). doi: 10.1001/jama.2013.284664
136. Kim K, Yoo D, Lee HS, Lee KJ, Park SB, Kim C, et al. Identification of potential biomarkers for diagnosis of pancreatic and biliary tract cancers by sequencing of serum microRNAs. BMC Med Genomics. (2019) 12(1):1–11. (Accessed March 12, 2025). doi: 10.1186/s12920-019-0521-8
137. Abue M, Yokoyama M, Shibuya R, Tamai K, Yamaguchi K, Sato I, et al. Circulating miR-483-3p and miR-21 is highly expressed in plasma of pancreatic cancer. Int J Oncol. (2014) 46(2):539. doi: 10.3892/ijo.2014.2743
138. Kim MW, Koh H, Kim JY, Lee S, Lee H, Kim Y, et al. Tumor-specific miRNA signatures in combination with CA19-9 for liquid biopsy-based detection of PDAC. Int J Mol Sci. (2021) 22(24). (Accessed March 12, 2025). doi: 10.3390/ijms222413621
139. Lai X, Wang M, McElyea SD, Sherman S, House M, Korc M. microRNA signature in circulating exosomes is superior to exosomal glypican-1 levels for diagnosing pancreatic cancer. Cancer Lett. (2017) 393:86–93. (Accessed March 12, 2025). doi: 10.1016/j.canlet.2017.02.019
140. Jin F, Yang L, Wang W, Yuan N, Zhan S, Yang P, et al. A novel class of tsRNA signatures as biomarkers for diagnosis and prognosis of pancreatic cancer. Mol Cancer. (2021) 20(1):1–8. (Accessed March 12, 2025). doi: 10.1186/s12943-021-01389-5
141. Zhang B, Li C, Sun Z. Long non-coding RNA LINC00346, LINC00578, LINC00673, LINC00671, LINC00261, and SNHG9 are novel prognostic markers for pancreatic cancer. Am J Transl Res. (2018) 10(8):2648. Available online at: https://pmc.ncbi.nlm.nih.gov/articles/PMC6129514/ (Accessed March 12, 2025).
142. Yu S, Li Y, Liao Z, Wang Z, Wang Z, Li Y, et al. Plasma extracellular vesicle long RNA profiling identifies a diagnostic signature for the detection of pancreatic ductal adenocarcinoma. Gut. (2020) 69(3):540–50. (Accessed March 12, 2025). doi: 10.1136/gutjnl-2019-318860
143. Chen J, Yao D, Chen W, Li Z, Guo Y, Zhu F, et al. Serum exosomal miR-451a acts as a candidate marker for pancreatic cancer. Int J Biol Markers. (2022) 37(1):74–80. (Accessed March 12, 2025). doi: 10.1177/17246008211070018
144. Reese M, Flammang I, Yang Z, Dhayat SA. Potential of exosomal microRNA-200b as liquid biopsy marker in pancreatic ductal adenocarcinoma. Cancers. (2020) 12(1):197. doi: 10.3390/cancers12010197
145. Guo S, Qin H, Liu K, Wang H, Bai S, Liu S, et al. Blood small extracellular vesicles derived miRNAs to differentiate pancreatic ductal adenocarcinoma from chronic pancreatitis. Clin Transl Med. (2021) 11(9):e520. doi: 10.1002/ctm2.520
146. Liu J, Gao J, Du Y, Li Z, Ren Y, Gu J, et al. Combination of plasma microRNAs with serum CA19-9 for early detection of pancreatic cancer. Int J Cancer. (2012) 131(3):683–91. (Accessed March 12, 2025). doi: 10.1002/ijc.26422
147. Li A, Yu J, Kim H, Wolfgang CL, Canto MI, Hruban RH, et al. MicroRNA array analysis finds elevated serum miR-1290 accurately distinguishes patients with low-stage pancreatic cancer from healthy and disease controls. Clin Cancer Res. (2013) 19(13):3600–10. (Accessed March 12, 2025). doi: 10.1158/1078-0432.CCR-12-3092
148. Wei J, Yang L, Wu YN, Xu J. Serum miR-1290 and miR-1246 as Potential Diagnostic Biomarkers of Human Pancreatic Cancer. J Cancer. (2020) 11(6):1325. doi: 10.7150/jca.38048
149. Qu K, Zhang X, Lin T, Liu T, Wang Z, Liu S, et al. Circulating miRNA-21-5p as a diagnostic biomarker for pancreatic cancer: evidence from comprehensive miRNA expression profiling analysis and clinical validation. Sci Rep. (2017) 7(1):1692. doi: 10.2147/OTT.S207963
150. Wang Z. Diagnostic performance for declined microRNA-133a in pancreatic cancer. J Cell Biochem. (2020) 121(8 9):3882–6. (Accessed March 12, 2025). doi: 10.1002/jcb.29547
151. Nakamura K, Zhu Z, Roy S, Jun E, Han H, Munoz RM, et al. An exosome-based transcriptomic signature for noninvasive, early detection of patients with pancreatic ductal adenocarcinoma: A multicenter cohort study. Gastroenterology. (2022) 163(5):1252–1266.e2. Available online at (Accessed March 12, 2025). doi: 10.1053/j.gastro.2022.06.090
152. Yu Y, Tong Y, Zhong A, Wang Y, Lu R, Guo L. Identification of Serum microRNA-25 as a novel biomarker for pancreatic cancer. Medicine (Baltimore). (2020) 99(52):e23863. (Accessed March 12, 2025). doi: 10.1097/MD.0000000000023863
153. Liu DSK, Puik JR, Patel BY, Venø MT, Vahabi M, Prado MM, et al. Unlocking the diagnostic power of plasma extracellular vesicle miR-200 family in pancreatic ductal adenocarcinoma. J Exp Clin Cancer Res. (2024) 43(1):1–17. (Accessed March 12, 2025). doi: 10.1186/s13046-024-03090-z
154. He X, Chen L, Di Y, Li W, Zhang X, Bai Z, et al. Plasma-derived exosomal long noncoding RNAs of pancreatic cancer patients as novel blood-based biomarkers of disease. BMC Cancer. (2024) 24(1). (Accessed March 12, 2025). doi: 10.1186/s12885-024-12755-z
155. Blattmann P, Aebersold R. The advent of mass spectrometry-based proteomics in systems biology research. Encyclopedia Cell Biol. (2016) 4:166–76. doi: 10.1016/B978-0-12-394447-4.40030-1
156. Ding Z, Wang N, Ji N, Chen ZS. Proteomics technologies for cancer liquid biopsies. Mol Cancer. (2022) 21(1):1–11. (Accessed March 12, 2025). doi: 10.1186/s12943-022-01526-8
157. Landegren U, Hammond M. Cancer diagnostics based on plasma protein biomarkers: hard times but great expectations. Mol Oncol. (2021) 15(6):1715–26. (Accessed March 12, 2025). doi: 10.1002/1878-0261.12809
158. Hu S, Loo JA, Wong DT. Human body fluid proteome analysis. Proteomics. (2006) 6(23):6326–53. (Accessed March 12, 2025). doi: 10.1002/pmic.200600284
159. Meuleman W, Engwegen JYMN, Gast MCW, Beijnen JH, Reinders MJT, Wessels LFA. Comparison of normalisation methods for surface-enhanced laser desorption and ionisation (SELDI) time-of-flight (TOF) mass spectrometry data. BMC Bioinformatics. (2008) 9(1):1–11. (Accessed March 12, 2025). doi: 10.1186/1471-2105-9-88
160. Giacomelli C, Bazzichi L, Giusti L, Ciregia F, Baldini C, da Valle Y, et al. MALDI-TOF and SELDI-TOF analysis: “tandem” techniques to identify potential biomarker in fibromyalgia. Reumatismo. (2011) 63(3):165–70. Available online at: https://www.reumatismo.org/reuma/article/view/534 (Accessed March 12, 2025).
161. Zhang G, Ueberheide BM, Waldemarson S, Myung S, Molloy K, Eriksson J, et al. Protein quantitation using mass spectrometry. Methods Mol Biol. (2010) 673:211. (Accessed March 12, 2025). doi: 10.1007/978-1-60761-842-3_13
162. Gold L, Ayers D, Bertino J, Bock C, Bock A, Brody EN, et al. Aptamer-based multiplexed proteomic technology for biomarker discovery. PLoS One. (2010) 5(12):e15004. doi: 10.1371/journal.pone.0015004
163. Ostroff RM, Bigbee WL, Franklin W, Gold L, Mehan M, Miller YE, et al. Unlocking biomarker discovery: large scale application of aptamer proteomic technology for early detection of lung cancer. PLoS One. (2010) 5(12):e15003. (Accessed March 12, 2025). doi: 10.1371/journal.pone.0015003
164. Assarsson E, Lundberg M, Holmquist G, Björkesten J, Thorsen SB, Ekman D, et al. Homogenous 96-plex PEA immunoassay exhibiting high sensitivity, specificity, and excellent scalability. PLoS One. (2014) 9(4):e95192. (Accessed March 12, 2025). doi: 10.1371/journal.pone.0095192
165. Bhardwaj M, Gies A, Weigl K, Tikk K, Benner A, Schrotz-King P, et al. Evaluation and validation of plasma proteins using two different protein detection methods for early detection of colorectal cancer. Cancers. (2019) 11(10):1426. doi: 10.3390/cancers11101426
166. Petricoin E, Wulfkuhle J, Howard M, Pierobon M, Espina V, Luchini A, et al. RPPA: origins, transition to a validated clinical research tool, and next generations of the technology. Adv Exp Med Biol. (2019) 1188:1–19. (Accessed March 12, 2025). doi: 10.1007/978-981-32-9755-5_1
167. Akbani R, Becker KF, Carragher N, Goldstein T, de Koning L, Korf U, et al. Realizing the promise of reverse phase protein arrays for clinical, translational, and basic research: A workshop report the RPPA (Reverse Phase Protein Array) Society. Mol Cell Proteomics. (2014) 13(7):1625–43. doi: 10.1074/mcp.O113.034918
168. Tibes R, Qiu YH, Lu Y, Hennessy B, Andreeff M, Mills GB, et al. Reverse phase protein array: validation of a novel proteomic technology and utility for analysis of primary leukemia specimens and hematopoietic stem cells. Mol Cancer Ther. (2006) 5(10):2512–21. (Accessed March 12, 2025). doi: 10.1158/1535-7163.MCT-06-0334
169. Amaral P, Carbonell-Sala S, de la Vega FM, Faial T, Frankish A, Gingeras T, et al. The status of the human gene catalogue. Nature. (2023) 622(7981):41–7. (Accessed March 12, 2025). doi: 10.1038/s41586-023-06490-x
170. Ballehaninna UK, Chamberlain RS. The clinical utility of serum CA 19-9 in the diagnosis, prognosis and management of pancreatic adenocarcinoma: An evidence based appraisal. J Gastrointest Oncol. (2012) 3(2):105–19. doi: 10.3978/j.issn.2078-6891.2011.021
171. Kriz D, Ansari D, Andersson R. Potential biomarkers for early detection of pancreatic ductal adenocarcinoma. Clin Transl Oncol. (2020) 22(12):2170. doi: 10.1007/s12094-020-02372-0
172. Xing H, Wang J, Wang Y, Tong M, Hu H, Huang C, et al. Diagnostic value of CA 19-9 and carcinoembryonic antigen for pancreatic cancer: A meta-analysis. Gastroenterol Res Pract. (2018) 2018:8704751. (Accessed March 12, 2025). doi: 10.1155/2018/8704751
173. Zhang Y, Yang J, Li H, Wu Y, Zhang H, Chen W. Tumor markers CA19-9, CA242 and CEA in the diagnosis of pancreatic cancer: a meta-analysis. Int J Clin Exp Med. (2015) 8(7):11683. (Accessed March 12, 2025).
174. Dong D, Jia L, Zhang L, Ma N, Zhang A, Zhou Y, et al. Periostin and CA242 as potential diagnostic serum biomarkers complementing CA19.9 in detecting pancreatic cancer. Cancer Sci. (2018) 109(9):2841–51. (Accessed March 12, 2025). doi: 10.1111/cas.13712
175. Velstra B, Vonk MA, Bonsing BA, Mertens BJ, Nicolardi S, Huijbers A, et al. Serum peptide signatures for pancreatic cancer based on mass spectrometry: a comparison to CA19-9 levels and routine imaging techniques. J Cancer Res Clin Oncol. (2015) 141(3):531–41. (Accessed March 12, 2025). doi: 10.1007/s00432-014-1812-2
176. Park J, Choi Y, Namkung J, Yi SG, Kim H, Yu J, et al. Diagnostic performance enhancement of pancreatic cancer using proteomic multimarker panel. Oncotarget. (2017) 8(54):93117–30. (Accessed March 12, 2025). doi: 10.18632/oncotarget.21861
177. Nie S, Lo A, Wu J, Zhu J, Tan Z, Simeone DM, et al. Glycoprotein biomarker panel for pancreatic cancer discovered by quantitative proteomics analysis. J Proteome Res. (2014) 13(4):1873–84. (Accessed March 12, 2025). doi: 10.1021/pr400967x
178. Han Sx, Zhou X, Sui X, He Cc, Cai Mj, Ma J, et al. Serum dickkopf-1 is a novel serological biomarker for the diagnosis and prognosis of pancreatic cancer. Oncotarget. (2015) 6(23):19907–17. (Accessed March 12, 2025). doi: 10.18632/oncotarget.4529
179. Xiao D, Dong Z, Zhen L, Xia G, Huang X, Wang T, et al. Combined exosomal GPC1, CD82, and serum CA19-9 as multiplex targets: A specific, sensitive, and reproducible detection panel for the diagnosis of pancreatic cancer. Mol Cancer Res: MCR. (2020) 18(2):1300–10. doi: 10.1158/1541-7786.MCR-19-0588
180. Ren C, Chen Y, Han C, Fu D, Chen H. Plasma interleukin-11 (IL-11) levels have diagnostic and prognostic roles in patients with pancreatic cancer. Tumour Biology: J Int Soc Oncodevelopmental Biol Med. (2014) 35(11):11467–72. doi: 10.1007/s13277-014-2459-y
181. Kim SH, Lee MJ, Hwang HK, Lee SH, Kim H, Paik YK, et al. Prognostic potential of the preoperative plasma complement factor B in resected pancreatic cancer: A pilot study. Cancer Biomarkers. (2019) 24(3):335–42. doi: 10.3233/CBM-181847
182. Tsutsumi K, Kawamoto H, Hirao K, Sakakihara I, Yamamoto N, Noma Y, et al. Monitoring of CA19-9 and SPan-1 can facilitate the earlier confirmation of progressing pancreatic cancer during chemotherapy. Pancreatology. (2012) 12(5):409–16. doi: 10.1016/j.pan.2012.07.009
183. Wang X, Li Y, Tian H, Qi J, Li M, Fu C, et al. Macrophage inhibitory cytokine 1 (MIC-1/GDF15) as a novel diagnostic serum biomarker in pancreatic ductal adenocarcinoma. BMC Cancer. (2014) 14:578. doi: 10.1186/1471-2407-14-578
184. Peng H, Chen R, Brentnall TA, Eng JK, Picozzi VJ, Pan S. Predictive proteomic signatures for response of pancreatic cancer patients receiving chemotherapy. Clin Proteomics. (2019) 16:31. doi: 10.1186/s12014-019-9251-3
185. Cheng H, He W, Yang J, Ye Q, Cheng L, Pan Y, et al. Ligand-targeted polymerase chain reaction for the detection of folate receptor-positive circulating tumour cells as a potential diagnostic biomarker for pancreatic cancer. Cell Proliferation. (2020) 53(9):e12880. doi: 10.1111/cpr.12880
186. Aronsson L, Andersson R, Bauden M, Andersson B, Bygott T, Ansari D. High-density and targeted glycoproteomic profiling of serum proteins in pancreatic cancer and intraductal papillary mucinous neoplasm. Scand J Gastroenterol. (2018) 53(12):1597–603. doi: 10.1080/00365521.2018.1532020
187. Jenkinson C, Elliott VL, Evans A, Oldfield L, Jenkins RE, O’brien DP, et al. Decreased serum thrombospondin-1 levels in pancreatic cancer patients up to 24 months prior to clinical diagnosis: Association with diabetes mellitus. Clin Cancer Res. (2016) 22(7):1734–43. doi: 10.1158/1078-0432.CCR-15-0879
188. Mellby LD, Nyberg AP, Johansen JS, Wingren C, Nordestgaard BG, Bojesen SE, et al. Serum biomarker signature-based liquid biopsy for diagnosis of early-stage pancreatic cancer. J Clin Oncol. (2018) 36(28):2887–94. doi: 10.1200/JCO.2017.77.6658
189. Kaur S, Smith LM, Patel A, Menning M, Watley DC, Malik SS, et al. A combination of MUC5AC and CA19-9 improves the diagnosis of pancreatic cancer: A multicenter study. Am J Gastroenterol. (2017) 112(1):172–83. doi: 10.1038/ajg.2016.482
190. Lee MJ, Na K, Jeong SK, Lim JS, Kim SA, Lee MJ, et al. Identification of human complement factor B as a novel biomarker candidate for pancreatic ductal adenocarcinoma. J Proteome Res. (2014) 13(11):4878–88. doi: 10.1021/pr5002719
191. Guo X, Lv X, Fang C, Lv X, Wang F, Wang D, et al. Dysbindin as a novel biomarker for pancreatic ductal adenocarcinoma identified by proteomic profiling. Int J Cancer. (2016) 139(8):1821–9. doi: 10.1002/ijc.v139.8
192. Wu X, Zhang ZX, Chen XY, Xu YL, Yin N, Yang J, et al. A panel of three biomarkers identified by iTRAQ for the early diagnosis of pancreatic cancer. Proteomics - Clin Appl. (2019) 13(5):e1800195. doi: 10.1002/prca.201800195
193. Papapanagiotou A, Sgourakis G, Karkoulias K, Raptis D, Parkin E, Brotzakis P, et al. Osteonectin as a screening marker for pancreatic cancer: A prospective study. J Int Med Res. (2018) 46(7):2769–79. doi: 10.1177/0300060518772413
194. Balasenthil S, Huang Y, Liu S, Marsh T, Chen J, Stass SA, et al. A plasma biomarker panel to identify surgically resectab le early-stage pancreatic cancer. J Natl Cancer Institute. (2017) 109(8):djw341. doi: 10.1093/jnci/djw341
195. Honda K, Okusaka T, Felix K, Nakamori S, Sata N, Nagai H, et al. Altered plasma apolipoprotein modifications in patients with pancreatic cancer: protein characterization and multi-institutional validation. PloS One. (2012) 7(10):e46908. doi: 10.1371/journal.pone.0046908
196. Hogendorf P, Durczynski A, Skulimowski A, Kumor A, Poznanska G, Strzelczyk J. Growth differentiation factor (GDF-15) concentration combined with Ca125 levels in serum is superior to commonly used cancer biomarkers in differentiation of pancreatic mass. Cancer Biomarkers. (2018) 21(3):505–11. doi: 10.3233/CBM-170203
197. Mohamed AA, Soliman H, Ismail M, Ziada D, Farid TM, Aref AM, et al. Evaluation of circulating ADH and MIC-1 as diagnostic markers in Egyptian patients with pancreatic cancer. Pancreatology. (2015) 15(1):34–9. doi: 10.1016/j.pan.2014.10.008
198. Wei Q, Zhang J, Li Z, Wei L, Ren L. Serum exo-ephA2 as a potential diagnostic biomarker for pancreatic cancer. Pancreas. (2020) 49(9):1213–9. doi: 10.1097/MPA.0000000000001660
199. Song J, Sokoll LJ, Pasay JJ, Rubin AL, Li H, Bach DM, et al. Identification of serum biomarker panels for the early detection of pancreatic cancer. Cancer Epidemiol Biomarkers Prev. (2019) 28(1):174–82. doi: 10.1158/1055-9965.EPI-18-0483
200. Melo SA, Luecke LB, Kahlert C, Fernandez AF, Gammon ST, Kaye J, et al. Glypican-1 identifies cancer exosomes and detects early pancreatic cancer. Nature. (2015) 523(7559):177–82. doi: 10.1038/nature14581
201. Lewis JM, Vyas AD, Qiu Y, Messer KS, White R, Heller MJ. Integrated analysis of exosomal protein biomarkers on alternating current electrokinetic chips enables rapid detection of pancreatic cancer in patient blood. ACS Nano. (2018) 12(4):3311–20. doi: 10.1021/acsnano.7b08199
202. Byeon S, McKay MJ, Molloy MP, Gill AJ, Samra JS, Mittal A, et al. Novel serum protein biomarker panel for early diagnosis of pancreatic cancer. Int J Cancer. (2024) 155:365–71. doi: 10.1002/ijc.v155.2
203. Yablecovitch D, Nadler M, Ben-Horin S, Picard O, Yavzori M, Fudim E, et al. Serum matrix metalloproteinase-7, Syndecan-1, and CA 19-9 as a biomarker panel for diagnosis of pancreatic ductal adenocarcinoma. Cancer Med. (2024) 13(17):e70144. doi: 10.1002/cam4.v13.17
204. Uemura S, Kabe Y, Kitago M, Matsuda S, Abe Y, Hasegawa Y, et al. Prognosis prediction of PDAC via detection of O -glycan altered extracellular vesicles in perioperative sera. Cancer Science. (2024) 115:3718–28. doi: 10.1111/cas.v115.11
205. Cohen JD, Javed AA, Thoburn C, Wong F, Tie J, Gibbs P, et al. Combined circulating tumor DNA and protein biomarker-based liquid biopsy for the earlier detection of pancreatic cancers. Proc Natl Acad Sci. (2017) 114:10202–7. doi: 10.1073/pnas.1704961114
206. Madhavan B, Yue S, Galli U, Rana S, Gross W, Müller M, et al. Combined evaluation of a panel of protein and miRNA serum-exosome biomarkers for pancreatic cancer diagnosis increases sensitivity and specificity. Int J Cancer. (2015) 136:2616–27. doi: 10.1002/ijc.v136.11
207. Chen J, Wang H, Zhou L, Liu Z, Tan X. A combination of circulating tumor cells and CA199 improves the diagnosis of pancreatic cancer. J Clin Lab Analysis. (2022) 36(5):e24341. doi: 10.1002/jcla.24341
208. Waddell N, Pajic M, Patch AM, Chang DK, Kassahn KS, Bailey P, et al. Whole genomes redefine the mutational landscape of pancreatic cancer. Nature. (2015) 518(7540):495–501. doi: 10.1038/nature14169
209. Christensen JG, Olson P, Briere T, Wiel C, Bergo MO. Targeting Krasg12c-mutant cancer with a mutation-specific inhibitor. J Internal Med. (2020) 288(2):183–91. doi: 10.1111/joim.v288.2
210. Sinn M, Bahra M, Liersch T, Gellert K, Messmann H, Bechstein W, et al. CONKO-005: Adjuvant chemotherapy with gemcitabine plus erlotinib versus gemcitabine alone in patients after r0 resection of pancreatic cancer: A multicenter randomized phase III trial. J Clin Oncol. (2017) 35(29):3330–7. doi: 10.1200/JCO.2017.72.6463
211. Moll HP, Pranz K, Musteanu M, Grabner B, Hruschka N, Mohrherr J, et al. Afatinib restrains K-RAS-driven lung tumorigenesis. Sci Trans Med. (2018) 10(446):eaao2301. doi: 10.1126/scitranslmed.aao2301
212. Schultheis B, Reuter D, Ebert MP, Siveke J, Kerkhoff A, Berdel WE, et al. Gemcitabine combined with the monoclonal antibody nimotuzumab is an active first-line regimen in KRAS wildtype patients with locally advanced or metastatic pancreatic cancer: A multicenter, randomized phase IIb study. Ann Oncol. (2017) 28(10):2429–35. doi: 10.1093/annonc/mdx343c/mdx343
213. Ko AH, Bekaii-Saab T, van Ziffle J, Mirzoeva OM, Joseph NM, Talasaz AA, et al. A multicenter, open-label phase II clinical trial of combined MEK plus EGFR inhibition for chemotherapy-refractory advanced pancreatic adenocarcinoma. Clin Cancer Res. (2016) 22(1):61–8. doi: 10.1158/1078-0432.CCR-15-0979
214. Bodoky G, Timcheva C, Spigel DR, la Stella PJ, Ciuleanu TE, Pover G, et al. A phase II open-label randomized study to assess the efficacy and safety of selumetinib (AZD6244 [ARRY-142886]) versus capecitabine in patients with advanced or metastatic pancreatic cancer who have failed first-line gemcitabine therapy. Investigational New Drugs. (2012) 30(3):1216–23. doi: 10.1007/s10637-011-9687-4
215. Infante JR, Somer BG, Park JO, Li CP, Scheulen ME, Kasubhai SM, et al. A randomised, double-blind, placebo-controlled trial of trametinib, an oral MEK inhibitor, in combination with gemcitabine for patients with untreated metastatic adenocarcinoma of the pancreas. Eur J Cancer. (2014) 50(12):2072–81. doi: 10.1016/j.ejca.2014.04.024
216. Alagesan B, Contino G, Guimaraes AR, Corcoran RB, Deshpande V, R.wojtkiewicz G, et al. Combined MEK and PI3K inhibition in a mouse model of pancreatic cancer. Clin Cancer Res. (2015) 21(2):396–404. doi: 10.1158/1078-0432.CCR-14-1591
217. Doebele RC, Drilon A, Paz-Ares L, Siena S, Shaw AT, Farago AF, et al. Entrectinib in patients with advanced or metastatic NTRK fusion-positive solid tumours: integrated analysis of three phase 1–2 trials. Lancet Oncol. (2020) 21(2):271–82. doi: 10.1016/S1470-2045(19)30691-6
218. Hong DS, DuBois SG, Kummar S, Farago AF, Albert CM, Rohrberg KS, et al. Larotrectinib in patients with TRK fusion-positive solid tumours: a pooled analysis of three phase 1/2 clinical trials. Lancet Oncol. (2020) 21(4):531–40. doi: 10.1016/S1470-2045(19)30856-3
219. Lindemann A, Patel AA, Silver NL, Tang L, Liu Z, Wang L, et al. COTI-2, a novel thiosemicarbazone derivative, exhibits antitumor activity in HNSCC through p53-dependent and -independent mechanisms. Clin Cancer Res. (2019) 25(18):5650–62. doi: 10.1158/1078-0432.CCR-19-0096
220. Chou A, Froio D, Nagrial AM, Parkin A, Murphy KJ, Chin VT, et al. Tailored first-line and second-line CDK4-targeting treatment combinations in mouse models of pancreatic cancer. Gut. (2018) 67(12):2142–55. doi: 10.1136/gutjnl-2017-315144
Keywords: pancreatic ductal adenocarcinoma, biomarkers, blood, DNA, RNA, protein
Citation: Juthani R and Manne A (2025) Blood-based biomarkers in pancreatic ductal adenocarcinoma: developments over the last decade and what holds for the future- a review. Front. Oncol. 15:1555963. doi: 10.3389/fonc.2025.1555963
Received: 06 January 2025; Accepted: 24 March 2025;
Published: 22 April 2025.
Edited by:
Jessica Dal Col, University of Salerno, ItalyReviewed by:
Richard T. Waldron, Cedars Sinai Medical Center, United StatesGuodong Zhao, Zhejiang University of Technology, China
Copyright © 2025 Juthani and Manne. This is an open-access article distributed under the terms of the Creative Commons Attribution License (CC BY). The use, distribution or reproduction in other forums is permitted, provided the original author(s) and the copyright owner(s) are credited and that the original publication in this journal is cited, in accordance with accepted academic practice. No use, distribution or reproduction is permitted which does not comply with these terms.
*Correspondence: Ronit Juthani, cm9uaXRqdXRoYW5pMjNAZ21haWwuY29t