- School of Health Economics and Management, Nanjing University of Chinese Medicine, Nanjing, China
Objective: The aim of the study was to explore the factors influencing the availability of medications for children, and establish a machine learning model to provide an empirical basis for the subsequent formulation and improvement of relevant policies.
Methods: Design: Cross-sectional survey. Setting: 12 provinces, China. Medical doctors from 25 public hospitals were enrolled. All data were randomly divided into a training set and a validation set at a ratio of 7:3. Three prediction models, namely random forest (RF), logistic regression (LR), and extreme gradient boosting (XGBoost), were developed and compared. The receiver operating characteristic curve (ROC) and the associated area under the curve (AUC) were used to evaluate the three models. A nomogram and clinical impact curve (CIC) for availability of medication were developed.
Results: Fifteen of 29 factors in the database that were most likely to be selected were considered to establish the prediction model. The XGBoost model (AUC = 0.915) demonstrated better performance than the RF model (AUC = 0.902) and the LR model (AUC = 0.890). According to the Shapley additive explanation values, the five factors that most significantly affected the availability of medications for children in the XGboost model were as follows: the relatively small number of specialized dosage forms for children; unaffordable medications for children; public education on the accessibility and safety of medication for children; uneven distribution of medical resources, leading to insufficient access to medication for children; and years of service as a doctor. The CIC was used to assess the practical applicability of the factor prediction nomogram.
Conclusions: The XGBoost model can be used to establish a prediction model to screen the factors associated with the availability of medications for children. The most important contributing factors to the models were the following: the relatively small number of specialized dosage forms for children; unaffordable medications for children; public education on the accessibility and safety of medication for children; uneven distribution of medical resources, leading to insufficient access to medication for children; and years of service as a doctor.
Introduction
Medications for children are important for ensuring the level of medical care for children. In the present situation, the availability of medications for children poses a challenge in developing countries (1–3). China is the largest developing country in the world. According to the results of the seventh national population census in China in November 2020, the number of children aged 0–14 years was approximately 250 million, accounting for 17.95% of the total population of China. In the context of an increasing demand for medications, China is also facing a shortage of medication supply for children and a lack of suitable formulations and medication information for children (4).
The availability of medications for children seriously affects the treatment of diseases. Improving the availability of essential medications for children and further promotion of rational medication are the foundations of China's basic medical system. It is also the core function of the national medication policy. Some authors have studied the accessibility of essential medications for children in China (5, 6). However, to the best of our knowledge, there have been no reports on the influencing factors, and a machine learning model to predict medication availability for children has not yet been developed.
In the present study, we surveyed medical doctors with the aim of analyzing the current situation of the availability of medications for children, exploring the factors influencing medication availability for children, and establishing a machine learning model to provide empirical basis for the subsequent formulation and improvement of relevant policies.
Methods
A cross-sectional study and survey were conducted in China from July 2023 to September 2023. We surveyed medical doctors from the health care system in the region, including tertiary hospitals (comprehensive tertiary first-class hospitals and tertiary children's hospitals) and secondary hospitals.
The survey database included the following factors: type of doctor; hospital level; years of service as a doctor; lack of clear medication guidance and difficulty in diagnosis and treatment; relatively small number of specialized dosage forms for children; public education on the accessibility and safety of medications for children; unaffordable medications for children; lack of drug information (lack of information, leading to unreasonable use of medication); training and education of medical staff on improving the safety of medications for children(Regular training on rational medication for medical staff); establishing a multidisciplinary approach to pediatric medication(Some diseases require multidisciplinary discussions before medication can be administered); parents or patients lacking necessary knowledge about children's medications; insufficient number of pediatricians in the local area; uneven distribution of medical resources, leading to insufficient access to medication for children; unified and standardized standards for pediatric medication; improvement in promoting accessibility to medications for children by the government; establishing a database of rational medication for children; strengthening the selection and allocation management of medications for children; pharmacists providing pediatric pharmacy guidance; registration and regulatory's impact on the accessibility of medications for children; high costs of children's medication research and development; high technical barriers for research; long cycle from research and development to clinical use; difficulty recruiting children for clinical trials; strict safety requirements for children's medications; lower market reward; limited applicable group; higher raw and auxiliary materials; higher taste requirements; and short medication cycle.
Model development and statistical analysis
In the present study, all data were randomly divided into a training set and a validation set at a ratio of 3:1. The following supervised machine learning methods were used to develop the predictive models, logistic regression (LR), random forest (RF) and eXtreme Gradient Boosting (XGboost). Parameters were adjusted on the training set. During the training process, RF, XGBoost and LR models were initialized separately; then a batch gradient descent algorithm was applied to iteratively update these parameters until convergence was achieved. The receiver operating characteristic curve (ROC) and the associated area under the curve (AUC) were used to evaluate the three models. A nomogram and a clinical impact curve (CIC) for the availability of medications were developed by selecting the 15 most significant predictors from the best model in order to assess the applicability and utility net advantages of the model with the highest diagnostic value.
Statistical analysis was performed with Python (version 3.9) and R software. Non-normally distributed numerical variables were expressed as median and assessed using the Mann–Whitney U test. Frequency and percentage were used to present categorical variables, and Pearson's chi-square test was used for intergroup comparison. P < 0.05 was considered significant.
Results
General information
A total of 154 doctors (123 pediatricians, 17 pediatric surgeons, and 14 from other departments) from 12 provinces in China were enrolled in the survey. Data for 15 out of the 29 variables in the database of this cross-section survey were used in the final study. Table 1 summarizes the general findings of the survey. Among the 154 doctors, 31 were from Class A tertiary hospitals, 119 were from Third Class A children's hospitals, and 4 were from secondary hospitals.
Prediction model
Only 15 out of the 29 factors in the database that were most likely to be selected were considered to establish the prediction model. We showed that the XGBoost model (AUC = 0.915) demonstrated better performance than the RF model (AUC = 0.902) and the LR model (AUC = 0.890) (Figure 1 and Table 2). In addition, the XGBoost model showed higher accuracy (0.883) and specificity (0.866) than the LR model (accuracy, 0.828; specificity, 0.825) and the RF model (accuracy, 0.852; specificity, 0.843) in the validation set. Figures 2A–C depicts the relative values of each feature in different models.
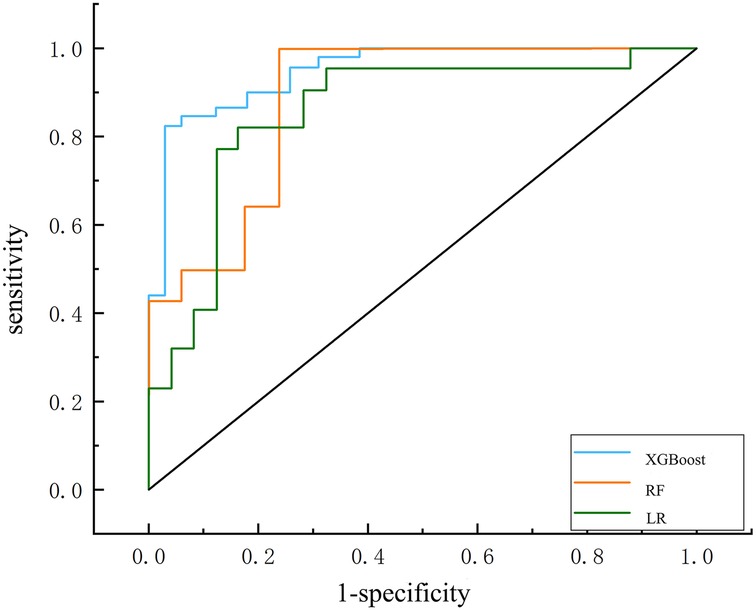
Figure 1 Receiver operating characteristic (ROC) curves of the machine learning classifier in the validation datasets; LR classifier (AUC = 0.890), RF classifier (AUC = 0.902); XGBoost model (AUC = 0.915).
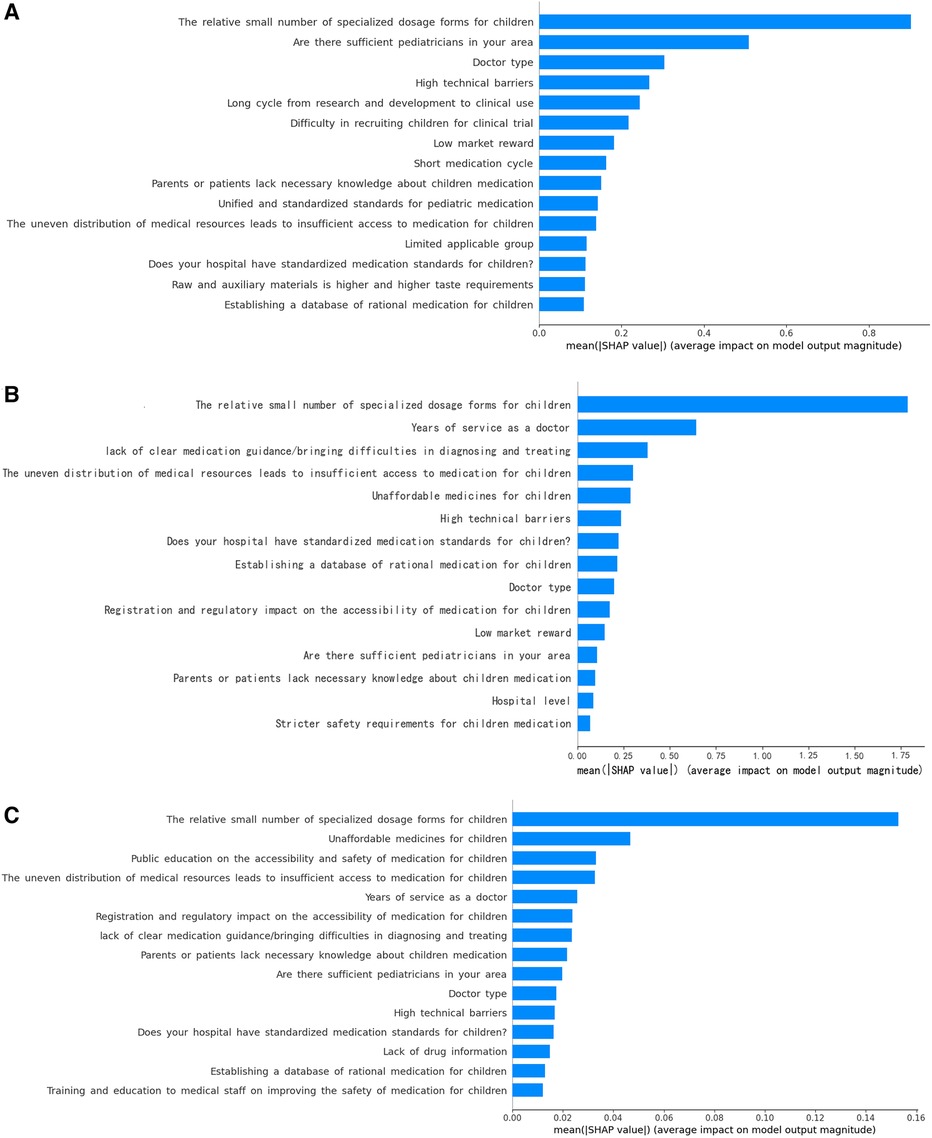
Figure 2 Importance matrix plot of the machine learning models. (A) LR model; (B) RF model; (C) XGBoost model.
Because the XGboost model showed higher accuracy and specificity than the RF and LR models, it was used to establish the risk nomogram. According to the Shapley additive explanation (SHAP) values, the following factors most significantly affected the availability of medications for children in the XGboost model: the relatively small number of specialized dosage forms for children; unaffordable medications for children; public education on the accessibility and safety of medication for children; uneven distribution of medical resources, leading to insufficient access to medication for children; and years of service as a doctor (Figures 3A–C). The 15 most significant factors were integrated to aid in the visualization of the XGboost prediction model. The results indicated that the XGboost model demonstrated reliable prediction of the factors influencing the availability of medications for children in China. Furthermore, we used CIC to assess the practical applicability of the factor prediction nomogram (Figure 4). The results of the CIC analysis indicated that that the nomogram had a superior overall net benefit within the large and practical ranges of threshold probabilities, indicating that the XGboost model has substantial predictive power in practice (Figure 5).
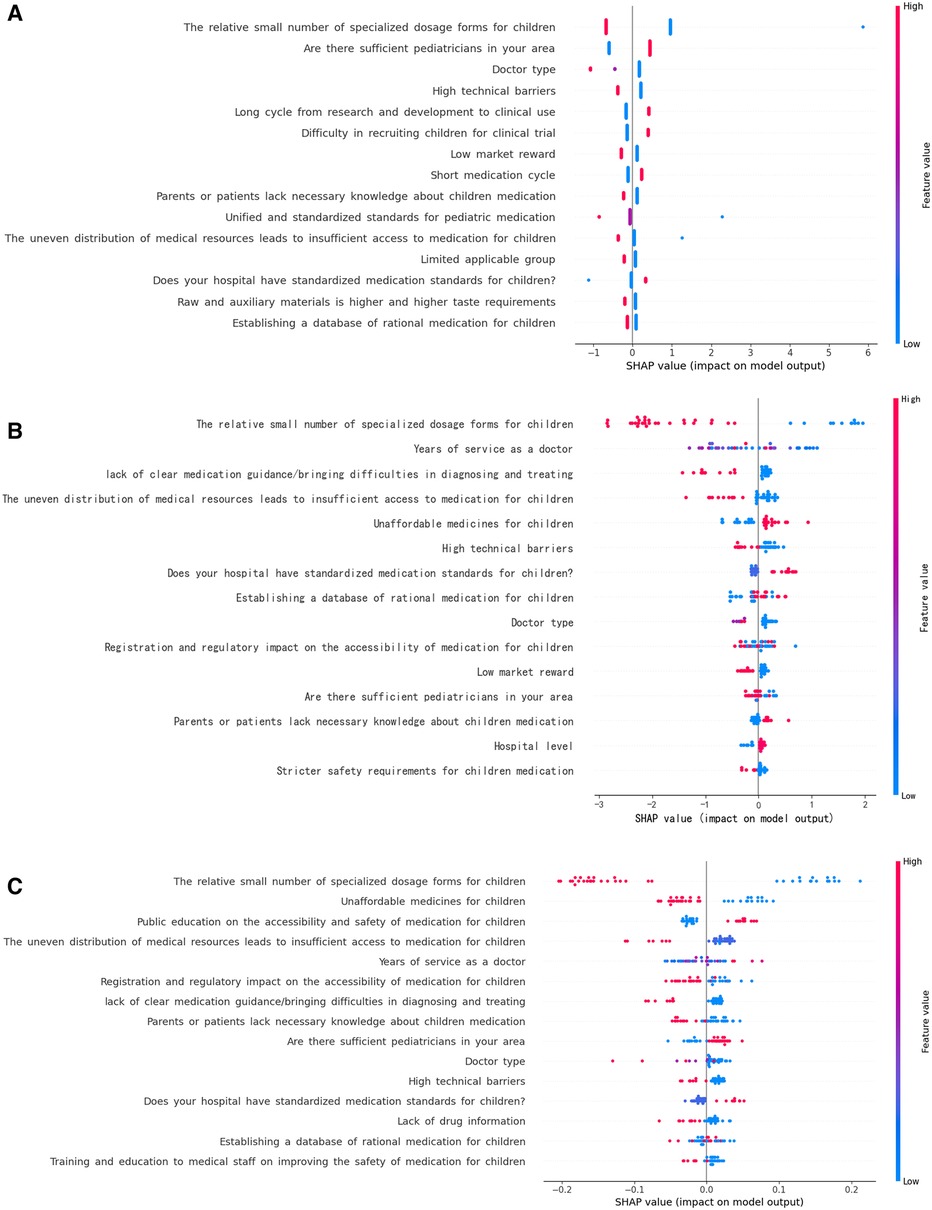
Figure 3 Shapley additive explanations (SHAP) framework for the features in the three machine learning models. (A) LR model; (B) RF model; (C) XGBoost model.
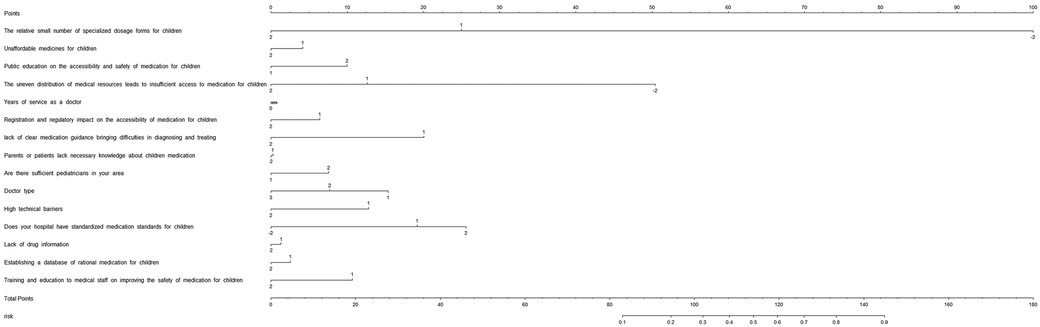
Figure 4 Nomogram to estimate risk factors for the availability of medications. The value of each variable was scored on a point scale from 0 to 100, after which the scores for each variable were added together. That sum was located on the total points axis, which enabled US to predict the probability of the availability of medications for children.
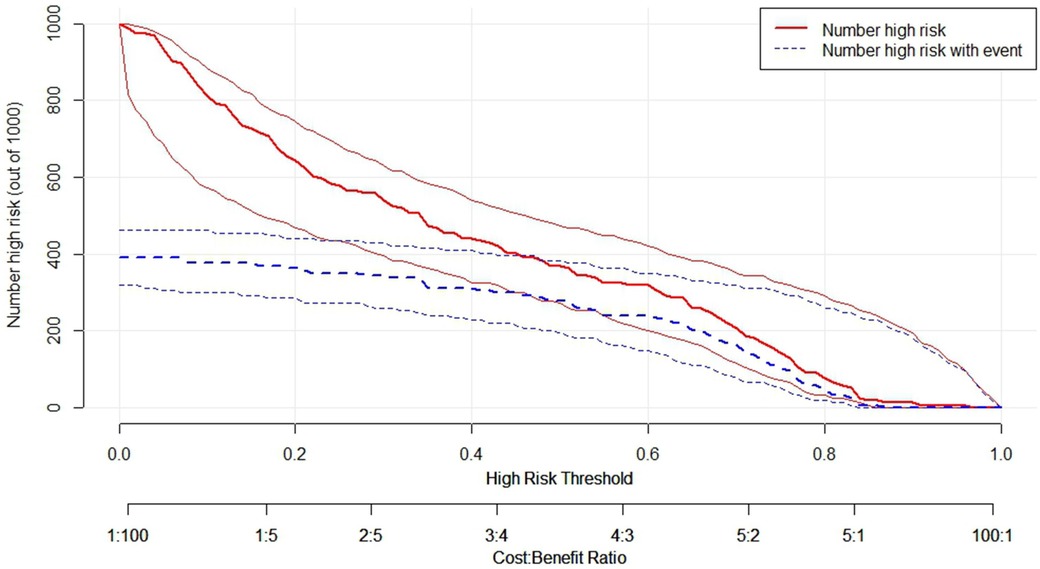
Figure 5 Clinical impact curve (CIC) of XGBoost model, the solid lines (number of high-risk) indicated the number of availability of medications for children who were classified as positive (high risk) by the model at each threshold probability; the dotted lines (number of high-risk events with outcome) was the number of true positives at each threshold probability; CIC visually indicated that nomogram conferred high practical net benefit and confirmed the practical value of the XGBoost model.
Discussion
General findings in the availability and affordability of medications for children
The present study focused on the availability of medications for children. A total of 154 doctors from 12 provinces in China were enrolled in this cross-section survey. The results showed that many factors affected the availability of medications for children. Some factors emphasized the patients’ perspective, such as public education on the accessibility and safety of medications for children; unaffordable medications for children; and parents or patients lacking necessary knowledge about children’s medication. Some factors emphasized the doctors’ perspective, such as years of service; lack of clear medication guidance and difficulties in diagnosis and treatment; shortage of pediatricians; doctor type; and training and education of medical staff on improving the safety of medications for children. Some factors were related to the shortage of resources, and these included the relatively small number of specialized dosage forms for children; registration and regulator's impact on the accessibility of medications for children; the uneven distribution of medical resources, leading to insufficient access to medications for children; high technical barriers; standardized medication standards for children in hospital; and establishing a database of rational medication for children.
Factors associated with the availability of medications for children
Machine learning tools have been applied in healthcare decision-making (7, 8). In the present study, we used machine learning to establish a model for detecting the factors associated with the availability of medications for children. We used three methods to establish the prediction models, namely RF, LR, and XGBoost. Among the three models, the XGBoost model performed better than the RF and LR models, showing higher accuracy and specificity. The advantage of XGBoost is the presence of random seeds, which improves the model by repeating operations even if the parameters remain unchanged, can efficiently and flexibly handle missing data, and may assemble weak prediction models to generate accurate predictions. Therefore, XGBoost performs better in terms of calculation speed (9). Therefore, we used the XGboost model for further analysis. To the best of our knowledge, these models were the first attempt to establish mathematical models to predict the availability of medications for children.
Access to essential medications for children is challenging, especially in developing countries (1–3). There have been some studies on the availability of essential medications for children using the standardized WHO/HAI methodology (5, 6, 10). Sun et al. reported that the availability of essential medications for children was low in public and private sectors. Specifically, the mean availability of existing generic medications and their original products was less than 40% in both public and private sectors. According to Chen et al., the average availability of essential medications for children in China is 1.6%–46.5% (5). These authors have focused on essential medications from China, and showed that the availability of essential medications in China is a challenge. Thus, we conducted a survey from the doctor's view of point to further explore the potential factors influencing the availability of medication for children.
In the present study, 15 factors that are most likely to be considered in practice were selected to develop the model. According to the XGboost model, the following five factors most significantly affected the availability of medications for children: the relatively small number of specialized dosage forms for children; unaffordable medications for children; public education on the accessibility and safety of medication for children; uneven distribution of medical resources, leading to insufficient access to medication for children; and years of service as a doctor.
In the present study, the relatively small number of specialized dosage forms for children was a key factor affecting the availability of medications for children. As reported by Wang, the list of essential medications for children is still unavailable, and lack of access to pediatric essential medications has been causing growing concerns. Strengths and dosage forms suitable for children, such as oral solutions, are still limited and in short supply in the market (11). Acceptability of medication is crucial for all patients. This is particularly true for children, who have different sensory perception, i.e., taste and texture for oral dosage forms, or pain perception for parenteral forms, which may underlie the refusal of therapy (12–14). Another factor affecting the availability of medications for children is related to the parents. There is limited public education on safety and effectiveness of medications for children. Thus, it is necessary to enhance safety education of the public about medication for children. The issue of unaffordable medication for children is common in developing countries, and China is the largest developing country in the world. The high prices of certain essential medications are still a challenge in some developing areas in China. This point has been reported previously (13). There is uneven distribution of medical resources, leading to insufficient access to medication for children in some aeras in China. There is a shortage of pediatricians in some areas because postgraduates are reluctant to become pediatricians and many pediatricians prefer working in developed regions and large medical institutions. We found that years of service as a doctor also affected the availability of medications for children. From the SHAP values, working experience as a doctor was negatively correlated with the availability of medications for children. Our data imply a vital potential of these measures for further training and establishing of a database of rational medication for children. We also need standardized medication standards for children to improve the availability of medications for children.
Previous studies have indicated that the shortage dose for children, high price, and uneven distribution of medical resources are the main causes of the inaccessibility of medications for children in China (5, 15, 16). All these studies are consistent with the prediction results of the present study. This suggests the feasibility of the prediction models for determining the factors associated with availability of medication for children. The advantage of the present study is that machine learning model was developed and used to screen the factors affecting the inaccessibility of medications for children in China. And nomogram was used to provide the sum of the scores to national policy makers and pharmaceutical management departments for estimating the factors associated with availability of medications for children. CIC visually indicated that nomogram conferred high practical net benefit and confirmed the practical value of the XGBoost model, and thus could help improve the availability of these medications.
Strengths and limitations
We herein executed a cross-sectional survey with the aim of analyzing the current situation of the availability of medications for children, exploring the factors influencing medication availability for children, and establishing a machine learning model to provide empirical basis for the subsequent formulation and improvement of relevant policies.
The present study had some limitations. First, it included a relatively small number of medical doctors. Second, the cross-section survey only evaluated the doctors’ perspective, which may have introduced selection bias to the prediction models. Namely, the availability of medications for children also depends on production enterprises, hospitals, social security, and drug supervision, et al. Future cross-section surveys should include production enterprises, hospitals, social security, and drug supervision, more large samples survey from different field and more machine learning algorithms so as to more compressively evaluate the availability of medications for children.
Conclusion
In the present study, we used machine learning to establish three prediction models to screen the factors associated with the availability of medications for children. Also, the visualization of the prediction model and CIC were used to assess the practical applicability of the factor prediction nomogram. The XGBoost model showed the highest sensitivity and specificity. The five factors that most significantly affected the availability of medication for children were as follows: the relatively small number of specialized dosage forms for children; unaffordable medications for children; public education on the accessibility and safety of medication for children; the uneven distribution of medical resources, leading to insufficient access to medication for children; and years of service as a doctor. This is the first report on the use of machine learning to establish prediction models to screen the factors associated with the availability of medications for children.
Data availability statement
The raw data supporting the conclusions of this article will be made available by the authors, without undue reservation.
Ethics statement
This study was approved by the Institutional Review Board of Nanjing University of Chinese Medicine.
Author contributions
J-yG: Conceptualization, Data curation, Investigation, Methodology, Project administration, Supervision, Validation, Writing – original draft, Writing – review & editing.
Funding
The author(s) declare that no financial support was received for the research, authorship, and/or publication of this article.
Conflict of interest
The authors declare that the research was conducted in the absence of any commercial or financial relationships that could be construed as a potential conflict of interest.
Publisher's note
All claims expressed in this article are solely those of the authors and do not necessarily represent those of their affiliated organizations, or those of the publisher, the editors and the reviewers. Any product that may be evaluated in this article, or claim that may be made by its manufacturer, is not guaranteed or endorsed by the publisher.
References
1. Abrha S, Tadesse E, Atey MT, Molla F, Melkam W, Masresha B, et al. Availability and affordability of priority life-saving medicines for under-five children in health facilities of Tigray region, Northern Ethiopia. BMC Pregnancy Childbirth. (2018) 18(1):464. doi: 10.1186/s12884-018-2109-2
2. Sado E, Sufa A. Availability and affordability of essential medicines for children in the western part of Ethiopia: implication for access. BMC Pediatr. (2016) 16:40. doi: 10.1186/s12887-016-0572-3
3. Tadesse T, Abuye H, Tilahun G. Availability and affordability of children essential medicines in health facilities of southern nations, nationalities, and people region, Ethiopia: key determinants for access. BMC Public Health. (2021) 21:714. doi: 10.1186/s12889-021-10745-5
4. Dai Y, Li ZP, Xu H, Zhu L, Zhu YQ, Cheng H, et al. A multicenter survey of the accessibility of essential medicines for children in China. Zhonghua Er Ke Za Zhi. (2020) 58(4):301–7. doi: 10.3760/cma.j.cn112140-20190820-00527
5. Sun XL, Wei J, Yao Y, Chen Q, You D, Xu X, et al. Availability, prices and affordability of essential medicines for children: a cross-sectional survey in Jiangsu province, China. BMJ Open. (2018) 8(10):e023646. doi: 10.1186/s12887-016-0572-3
6. Chen Z, Li S, Choonara I, Zou K, Zeng L, Huang L, et al. Access to medicines for children in China. BMJ Paediatr Open. (2022) 6(1):e001635. doi: 10.1136/bmjpo-2022-001635
7. Jayatilake SMDAC, Ganegoda GU. Involvement of machine learning tools in healthcare decision making. J Healthc Eng. (2021) 2021:6679512. doi: 10.1155/2021/6679512
8. Athey S. Beyond prediction: using big data for policy problems. Science. (2017) 355(6324):483–5. doi: 10.1126/science.aal4321
9. Yuan KC, Tsai LW, Lee KH, Cheng YW, Hsu SC, Lo YS, et al. The development an artificial intelligence algorithm for early sepsis diagnosis in the intensive care unit. Int J Med Inform. (2020) 141:104176. doi: 10.1016/j.ijmedinf.2020.104176
10. Khuluza F, Heide L. Availability and affordability of antimalarial and antibiotic medicines in Malawi. PLoS One. (2017) 12:e0175399. doi: 10.1371/journal.pone.0175399
11. Wang X, Fang Y, Yang S, Jiang M, Yan K, Wu L, et al. Access to paediatric essential medicines: a survey of prices, availability, affordability and price components in Shaanxi province, China. PLoS One. (2014) 9:e90365. doi: 10.1371/journal.pone.0090365
12. Hofmanová JK, Mason J, Batchelor HK. Sensory aspects of acceptability of bitter-flavoured 7.5mm film-coated tablets in adults, preschool and school children. Int J Pharm. (2020) 585:119511. doi: 10.1016/j.ijpharm.2020.119511
13. Walsh J, Schaufelberger D, Iurian S, Klein S, Batchelor H, Turner R, et al. Path towards efficient paediatric formulation development based on partnering with clinical pharmacologists and clinicians, a conect4children expert group white paper. Br J Clin Pharmacol. (2022) 88(12):5034–51. doi: 10.1111/bcp.14989
14. Orubu ESF, Duncan J, Tuleu C, Turner MA, Nunnet A. WHO essential medicines for children 2011–2019: age-appropriateness of enteral formulations. Arch Dis Child. (2022) 107(4):317–22. doi: 10.1136/archdischild-2021-321831
15. Chen Z, Li SY, Zou K, Li H, Zeng L, Lu X, et al. Accessibility of essential anticancer medicines for children in the Sichuan province of China. Front Public Health. (2022) 4(10):980969. doi: 10.3389/fpubh.2022.980969
Keywords: medications, availability, pediatric, machine learning, XGBoost
Citation: Guo J-y (2024) Machine learning for screening and predicting the availability of medications for children: a cross-sectional survey study. Front. Pediatr. 12:1341199. doi: 10.3389/fped.2024.1341199
Received: 20 November 2023; Accepted: 11 June 2024;
Published: 18 June 2024.
Edited by:
Lynn Kemp, Western Sydney University, AustraliaReviewed by:
Zhiyong Zhou, Suzhou Institute of Biomedical Engineering and Technology, Chinese Academy of Sciences (CAS), ChinaFengjun Zhao, Northwest University, China
© 2024 Guo. This is an open-access article distributed under the terms of the Creative Commons Attribution License (CC BY). The use, distribution or reproduction in other forums is permitted, provided the original author(s) and the copyright owner(s) are credited and that the original publication in this journal is cited, in accordance with accepted academic practice. No use, distribution or reproduction is permitted which does not comply with these terms.
*Correspondence: Jing-yan Guo, Z3VvamluZ3lhbnN1emhvdUAxNjMuY29t