- 1Department of Chemistry, Islamia College Peshawar, Peshawar, Pakistan
- 2Dr. Panjwani Center for Molecular Medicine and Drug Research, International Center for Chemical and Biological Sciences (PCMD-ICCBS), University of Karachi, Karachi, Pakistan
- 3Department of Clinical Microbiology, Sindh Institute of Urology and Transplantation (SIUT), Karachi, Pakistan
- 4TWC, HEJ-ICCBS, University of Karachi, Karachi, Pakistan
- 5Research Center for Advanced Materials Science (RCAMS), Department of Chemistry, Faculty of Science, King Khalid University, Abha Aseer, Saudi Arabia
- 6Centre for Technological Development in Health (CDTS), Oswaldo Cruz Foundation (Fiocruz), Rio de Janeiro, Brazil
Introduction: The genus Alistipes consists of anaerobic, Gram-negative bacteria with 13 species that colonize the entire gastrointestinal tract and are a serious health concern. They contribute to gut dysbiosis, intestinal inflammation, colorectal cancer, and depression.
Methods: To explore potential therapeutic targets and inhibitors, we filtered the core genome of Alistipes strains through subtractive genomics for non-host homology, gene essentiality, PPI, KEGG pathways, virulence, cellular localization, and druggability. The potential targets were docked against two drug-like libraries (ZINC, n = 11,993) and TCM (n = 36,043). ADMET profiling for best hits and MD simulation for apo/complex structures were performed, followed by physicochemical and pharmacokinetic evaluation and complex stabilities.
Results and Discussion: A set of 39 potential proteins was drastically reduced to only two targets after sequential data mining. The 3D structures of the selected targets (LpxA and KdsB) revealed good druggability scores. The top hits (ZINC85530940, ZINC05161112, ZINC95911713, and ZINC05566415) for both targets showed maximum H-bond interactions. The RMSD and RMSF values exhibited compactness with minimum fluctuation in ligand-bound complexes. The β-factor of ZINC05161112 at 327th residue and 352nd residue exhibited higher thermal instability, consistent with the RMSF results. The globularity of the complexes and apo structures remained consistent, whereas the LpxA complexes exhibited lower solvent-accessible surface area. For the KdsB, the surface area for ZINC5566415 increased significantly, with a steep decrease for ZINC95911713, establishing rather stable protein-ligand complexes. The results highlight the importance of identifying novel inhibitors and therapeutic targets. They are crucial for establishing better treatment regimes for human health and to aid in controlling the pathogenicity of Alistipes species.
1 Introduction
Humans acquire microbiota at birth, and during their life span, their gut is colonized by trillions of microorganisms, primarily bacteria. A broad array of bacterial phyla has been revealed using 16 S rRNA gene sequencing, with Firmicutes and Bacteroidetes being the most abundant (Goodrich et al., 2017). The human gut microbiota is important for immune response regulation, pathogen defense, digestive assistance, neurologic signaling, and vascularization (Lynch and Pedersen, 2016). An imbalance, or dysbiosis, in the gut microbiota has been linked to various disorders, including cardiovascular diseases (Halfvarson et al., 2017), inflammatory bowel disease (Yoshida et al., 2018), cancer (Vivarelli et al., 2019), and neurological disorders (Petra et al., 2015). Researchers have investigated various microbial genera and species within the microbiome to determine their role and identify specific bacterial species that influence disease progression and treatment response.
Among the diverse gut microbial populations, the genus Alistipes has gained attention due to its dual role in gut homeostasis and disease progression. This relatively new genus of bacteria from the Bacteroidetes phylum represents a group of Gram-negative, rod-shaped, anaerobic, and non-spore-forming bacteria. It has 13 species as of April 2020, according to the taxonomy database at the National Center for Biotechnology Information (NCBI) (Basson et al., 2020). While Alistipes species are primarily commensals in the gastrointestinal tract (GIT), their presence has been reported in appendicular, abdominal, perirectal, and brain abscesses, as well as in the bloodstream, urine, and peritoneal fluid, suggesting opportunistic pathogenicity (Shkoporov et al., 2015).
Alistipes species have also been implicated in gut dysbiosis and inflammatory disorders. For example, Alistipes putredinis has been identified in patients with appendicitis and abdominal abscesses, while Alistipes onderdonkii and Alistipes shahii were isolated from appendix tissue and urine samples (Rautio et al., 2003; Song et al., 2006). Several new Alistipes species have been identified in human fecal samples, including Alistipes communis, Alistipes dispar, Alistipes megaguti, and Alistipes provencensis (Bellali et al., 2019). The metabolic adaptability of Alistipes, including unique lipid biosynthesis pathways such as sphingolipid and sulfonolipid production, further distinguishes this genus from other gut microbes (Geiger et al., 2010).
Chronic intestinal inflammation, including inflammatory bowel diseases (IBD), is commonly treated with antibiotics, immunosuppressants, and biologic therapies. Antibiotics like metronidazole, ciprofloxacin, and rifaximin help regulate gut microbiota but face increasing resistance in gut bacteria, including Alistipes, which challenges their long-term effectiveness. Immunosuppressants (azathioprine, methotrexate, corticosteroids) control inflammation but do not address gut dysbiosis, while biologics (infliximab, adalimumab, vedolizumab) target immune pathways without eliminating opportunistic pathogens that may contribute to disease recurrence (Parker et al., 2020; Zhang et al., 2024).
Recent studies have highlighted the antibiotic resistance profiles of Alistipes species, demonstrating resistance to vancomycin, kanamycin, colistin, clindamycin, cefoxitin, and tetracycline, among others. Their metabolic adaptability and resistance mechanisms make them an emerging concern in antimicrobial research. Despite their clinical relevance, limited research has focused on identifying potential drug targets for therapeutic intervention (Rautio et al., 2003; Mishra et al., 2012; Lagier et al., 2012; Pfleiderer et al., 2014; Jousimies-Somer, 2002).
The primary phase in the vaccine, drug, and diagnostic biomarker development process is the identification of targets of interest. For that purpose, an in silico subtractive proteomic approach is commonly used (Wisal et al., 2024; Hassan et al., 2018; Irfan et al., 2023; Basharat et al., 2022; Kalhor et al., 2023; Alqurashi et al., 2024). Many advantages of such approaches include cost-effectiveness, reduced time and labor, reproducibility, and robustness to yield broad-spectrum therapeutic candidates (Barh et al., 2010a; Barh et al., 2010b). This study employs in silico subtractive genomics to identify potential druggable targets in Alistipes species. Using core-genome analysis, essential gene filtering, protein-protein interaction (PPI) analysis, and druggability assessments, we aim to pinpoint novel antibacterial targets. Furthermore, molecular docking, ADMET profiling, and molecular dynamics simulations are utilized to evaluate promising inhibitors, ensuring their stability, pharmacokinetics, and binding affinity. This study provides a computational framework for developing targeted therapies against Alistipes-associated gut dysbiosis while minimizing disruption to the broader gut microbiome.
2 Approaches and methodologies
2.1 Strains selection and identification of core genome
In the present work, 9 Alistipes strains with complete genomes were included for the core-genome analysis. We selected complete genomes to ensure comprehensive core-genome and accurate data analysis. This allows us to capture the full genetic information, ensuring no data is missed and enhancing the reliability of our findings for a thorough investigation of unique, essential, and non-homologous proteins across Alistipes strains. For this study, nine Alistipes strains with complete genomes were randomly chosen, and the DNA, protein, and general genome statistics were acquired from the National Center for Biotechnology Information (NCBI), a public database freely available to the scientific community (https://www.ncbi.nlm.nih.gov/). This database contains manually curated protein sequences under the UniProtKB/Swiss-Prot and automatically annotated protein sequences under the UniProtKB/TrEMBL (Boutet et al., 2007). After the retrieval of complete genomes, the core genome was discovered through the pangenome approach in the PATRIC web server (Wattam et al., 2014). For the identification of the core genome, the PATRIC software provides a robust comparative analysis environment, allowing us to perform detailed comparisons of gene content, sequence variations, and functional annotations. The selection criteria in PATRIC are as follows: one strain is randomly chosen as the reference strain (Alistipes finegoldii), and the remaining strains are compared to it using the modified parameters, from which the core genes (shared by all strains) are filtered. The PATRIC employs the Basic Local Alignment Search Tool algorithm for protein sequences (BLASTp) with the standard scoring matrix BLOSUM62 and the following cutoff values: e = 1 × 10−5, % coverage ≥ 90%, and % identity ≥ 95% (Mahram and Herbordt, 2015; Eddy, 2004).
2.2 Identification of essential and non-homologous genes
The Database of Essential Genes (DEG) server was used to eliminate the redundant proteins (Luo et al., 2021). The identified proteins were compared to Prokaryotes, Eukaryotes, and Archaea, using default settings such as e-value = 0.0001, bit score = 100, and % identity 35. Only proteins that did not show a significant match to any of the Prokaryotes, Eukaryotes, and Archaea protein datasets were considered for inclusion (Luo et al., 2021). For homologous sequences exclusion, pathogen-specific and non-host homolog drug targets were filtered by subjecting the resultant sequences to the BLASTp (http://blast.ncbi.nlm.nih.gov/Blast.cgi) against human proteome (TaxID: 9,606) (e-value: 10-4), a step to avoid any cross-reactivity in a possible host (Kerfeld and Scott, 2011).
2.3 Cellular localization and PPI prediction
The subcellular localization of the unique, essential, and non-homologs was predicted using the CELLO2GO (v2.5) (http://cello.life.nctu.edu.tw/cello2go/) (Yu et al., 2014) and the PSORTb (v3.0.2) (http://www.psortb.org/psortb/) (Yu et al., 2010). The protein sequences were submitted in FASTA format with the organism type set to bacteria and Gram stain set to negative. For bacteria, protein subcellular localization prediction is the accurate tool, and it applies Support Vector Machines (SVMs) that assign a possible localization site to a protein. Furthermore, it assigns the five subcellular locations, i.e., Periplasm, extracellular, cytoplasm, inner membrane, and outer membrane, to Gram-negative bacteria. Parallel to the PSORTb, the CELLO2GO takes the functionality of the SVMs at two levels. The initial classification of a protein subcellular location is performed based on sequence-derived molecular descriptors, followed by a final decision centered on the probability of the subcellular location. Subcellular localization analysis was performed to refine target selection by identifying proteins based on their accessibility and functional relevance. We prioritized cytoplasmic proteins for further analysis, including protein-protein interaction (PPI) mapping and target selection. Cytoplasmic proteins were selected due to their essential roles in metabolic and regulatory pathways, making them viable candidates for small-molecule inhibitors. This approach ensured the selection of high-confidence therapeutic targets that are both functionally significant and pharmacologically accessible (Yu et al., 2004).
Protein-protein interaction (PPI) was performed using the STRING (Search Tool for the Retrieval of Interacting Genes/Proteins) database (v11.5) (https://string-db.org/), applying a minimum interaction confidence score of 0.7 (high confidence) to ensure reliable functional associations (Szklarczyk et al., 2019). STRING is a protein-protein interaction platform that contains both known and anticipated interactions. The interactions arise from computational prediction, information transfer between species, and interactions gathered from other (primary) databases, and they comprise both direct (physical) and indirect (functional) relationships. Only interactions supported by experimental evidence, database annotations, and computational predictions with a combined score above 0.7 were considered for further analysis. Additionally, nodes with fewer than three interactions were excluded to refine the interaction network and focus on biologically significant targets. This filtering approach ensured the selection of highly connected and functionally relevant proteins for downstream analysis (Szklarczyk et al., 2021).
2.4 Drug target prioritization for target selection
Several variables, including molecular function, molecular weight, pathway analysis, cellular localization, and virulence, were taken into consideration when determining prospective drug targets (Agüero et al., 2008). The ProtParam tool2 calculates the molecular weight (MW) (Gasteiger et al., 2005) for targets, and an MW ≤ 100 kDa is regarded as an ideal (Hossain et al., 2016; Mondal et al., 2015). In bacteria, any biological function of proteins is defined in the context of their location and function, where inhibition of such function requires the downregulation of molecular interaction partners due to the promiscuous nature of a target protein, which might impact their activity. On the other hand, for the physiological function of hypothetical and novel proteins, understanding protein function and virulence factors is often critical (Scott et al., 2005).
The potentially druggable proteins brought forth by the genomic screening carried information about their subcellular location and the metabolic pathways involvement, the UniProtKB was used to gather information regarding the function of the protein, catalytic requirements for enzymatic activity, and active isoforms (dependency on cofactors, subunit’s structure, and associated post-translational modifications) (Consortium, 2015). The VFDB tool (Virulence Factor Database) was employed to determine the virulence of target proteins (Chen et al., 2005a), while the MHOLline biological workflow (http://www.mholline2.lncc.br) was employed to group the proteins based on template similarity scores to identify the top drug candidates for 3D modeling (Hassan et al., 2014). All these steps allowed the choice of the current targets for CADD analysis (computer-aided drug design).
2.5 KEGG metabolic pathway analyses
Mining of potential drug targets depends highly on the categorization of proteins involved in pathogen-specific metabolic pathways. The KEGG Automatic Annotation Server (KAAS) was utilized to screen out the essential proteins for metabolic pathway analysis (Moriya et al., 2007). Kyoto Encyclopedia of Gene and Genome (KEGG) pathway database then further maps out proteins involved in host-specific pathways by comparative analysis of host and pathogen metabolic pathways (Kanehisa and Goto, 2002). The output files by the KAAS server comprised information such as the enzyme names, the Enzyme Commission (EC) numbers, alternative pathways, the KEGG Orthology (KO) list assignment, and the metabolic pathways. The obtained dataset symbolizes the non-homologous proteins involved in crucial pathways of A. finegoldii (Kanehisa et al., 2017; Kanehisa et al., 2017). The anticipated pathogen pathways were manually compared to human pathways, unique and common, where unique were and exclusive to the bacterium and, hence, the focus of the current work, where common pathways were those present in both the bacterium and the host.
In addition to the KEGG, the MetaCyc or BioCyc tools also provide comprehensive databases and precise and detailed annotations and have been used in some of our previous works (Shah Hassan et al., 2012; Pereira et al., 2013). However, in this work, we selected the KAAS due to its ability to map the KEGG pathways specific to bacterial species, which aligns with our focus on pathogen-specific pathways. It offers a comprehensive database and precise KO (KEGG Orthology) assignments, integrating data from the KEGG GENES database and providing reliable and detailed annotations. This approach provides a more consistent and comprehensive view compared to other tools like the MetaCyc/BioCyc. Furthermore, the final targets were blasted against the gut microbiota (a manually assembled fasta file containing 52,618 genes) to avoid impact on the gut microbiota using the following parameters: e-value = 10, bit score = 200, and identity = 35%.
2.6 3D structure modeling and energy minimization
The three-dimensional structures of the potential drug targets, LpxA and KdsB (locus IDs: Alfi_0084, Alfi_2459), were not available in the RCSB-PBD database (https://www.rcsb.org). Therefore, we followed the comparative homology modeling to predict the 3D structures using the respective amino acids or nucleotide sequences from the reference genome of A. finegoldii. The selected sequences were then subjected to a BLASTp search against the PDB database for the selection of a suitable template with the best sequence identity and coverage. Templates with sequence identity and query coverage of ≥90% were selected for structure modeling.
To identify the best therapeutic candidates, protein sequences were sorted based on their similarities to the templates using the MHOLline (v2.0) biological workflow (http://www.mholline2.lncc.br) as adapted from Hassan et al. (2014). This workflow performed quality-based sequence sorting, and the highest quality sequences were subjected to the SWISS-MODEL server (Arnold et al., 2006). The 3D structure models for the filtered protein targets were then generated and visualized using the molecular graphics tool PyMOL (https://pymol.org/2/). To check the reliability of the generated model, a validation step is crucial; hence, all models were evaluated using PDBSum (Laskowski, 2001), ERRAT value (Dym et al., 2012), Verify3D (Eisenberg et al., 1997), and ProSA (Wiederstein and Sippl, 2007). All these measurements were used in the selection of the best 3D model.
The selected models (LpxA and KdsB) were subjected to energy minimization to improve their qualities for further use in docking, etc., studies. A powerful visualization tool, UCSF Chimera, was used to analyze the structures (Pettersen et al., 2004) for minimized energy. Gasteiger charges were assigned to protein, and structural constraints were removed by 1,500 rounds of minimization runs (750 steepest descent followed by 750 conjugate gradients) with a step size of 0.02 Å, under ff03. rl force field (Pettersen et al., 2004).
2.7 Druggable and catalytic pocket detection
The filtered targets were subsequently examined for potential binding pockets by calculating the druggable score using the DoGSiteScorer programme (https://proteins.plus). Protein + contains several automated tools, including a pocket detection technique used to assess the druggability of protein cavities. To utilise Protein+, the target of interest must be in 3D format (.pdb). Consequently, structures obtained from SWISS-MODEL alongside MHOLline were employed in this phase. DoGSiteScorer was then applied to evaluate the druggability of these 3D structures, which provides the pocket residues and druggability scores (ranging from 0 to 1). A protein cavity with a score closer to one is considered to be a highly druggable protein cavity.
2.8 Retrieval of ligand libraries, molecular docking, and ADMET profile
To get druggable compounds with a Tanimoto threshold level of 60%, the ZINC database was queried for (n = 11,993) druggable lead molecules (Sterling and Irwin, 2015; Sterling and Irwin, 2015), additionally, compounds retrieved from the TCM database (Traditional Chinese Medicine) (http://ZINC.docking.org/) (n = 36,043) were also screened for top lead compounds selection through the MOE (Molecular Operating Environment) (Vilar et al., 2008). In the next step, partial charges were added, and their energies were reduced using an energy minimization method (default parameters). Following their binding energies, chemicals that had been docked were sorted in ascending order of their binding energies. All best structure conformations with the least amount of energy were selected. A docking study was conducted by using the MOE to transform the 3D structure of the proposed drug targets into receptor molecules for virtual screening. The entire docking procedure concluded with the selection of the best-docked chemical compounds, which were analyzed using the ligand interaction mode of MOE (Molecular Operating Environment) (Chemical Computing Group, Inc., 2013) to understand the interaction that contributed to the binding of the ligands (Vilar et al., 2008).
To find appropriate inhibitors, they must pass Lipinski’s drug-like test while still requiring the least amount of energy (Lipinski, 2000; Barker and Khossravi, 2001; Lipinski et al., 2001). We conducted ADME/Tox analysis on the top-scoring compounds using an ADMET prediction server (http://lmmd.ecust.edu.cn/admetsar2) (Yang et al., 2019) alongside the SwissADME server (http://www.swissadme.ch/) to validate further physicochemical characteristics of selected hits for further skin permeation values (Daina et al., 2017).
2.9 Molecular dynamics (MD) simulation of the predicted targets
Molecular dynamics (MD) simulation was used to study the ligand-receptor interactions over time, providing insights into particle movement and complex stability within the system. MD simulations rely on classical and Newtonian mechanics, including Molecular Mechanics/Quantum Mechanics (MM/QM) methods, among others. Using GROMACS v4.1.5 and the GROMOS 54a7 force field, simulations captured the dynamic behavior of these complexes (Páll et al., 2020; Michaud-Agrawal et al., 2011). A triclinic periodic boundary box with 10 Å extensions surrounded the protein structures, allowing ample space for solvation and minimization.
Using the PyMol program, the ligands were first saved in. mol format before being converted to. pdb format. The MD simulation process involved four stages: minimization (1,500 steps, including steepest descent and conjugate gradient to remove steric clashes), heating to the target temperature, NVT and NPT equilibration for temperature and pressure stabilization, and a 100 ns production run. The RESPA integrator managed motion integration and covalent bonds with hydrogen atoms (Ma et al., 2003). TIP3P water molecules and 0.15 M Na+ and Cl− ions ensured system stability and charge neutrality (Jorgensen et al., 1983).
After the simulation, the root mean square deviation (RMSD), root mean square fluctuation (RMSF), solvent-accessible surface area (SASA), hydrogen bonds (H-bonds), and the radius of gyration (Rg) were calculated to assess stability and conformational behavior. Built-in GROMACS modules and XMgrace generated these outputs, which provide insights into the structural dynamics of the complexes (Michaud-Agrawal et al., 2011).
3 Results and discussion
3.1 Genome retrieval and identification of core genomes
The genome assembly and annotation report of the selected strains were checked using the NCBI (National Center for Biotechnology Information), a database resource that provides access to biomedical and genomic information. The complete genome sequence of A. finegoldii was obtained from the NCBI database and was randomly used as “the reference strain” to ensure the correctness of the results. To implement the subtractive genomics approach, only the complete genomes were selected for all Alistipes strains (Figure 1). The core genome was explored to discover pharmacological targets that were orthologs across all strains. For the most part, only the core genes of organisms, which are defined as the genes that are consistently present in all populations of an organism in all sorts of harsh conditions, were retracted. The core genome was discovered via the PATRIC program, and the overall number of genes discovered in the pangenome was 3,018, with 2,875 of them being non-redundant genes (Figure 2). After genome retrieval, the redundant gene removal was performed using a PATRIC application. This led to the identification of 143 redundant genes, and the remaining set was subjected to further genome subtraction analysis. Genome statistics like genome size, number of proteins, % GC content, bio-project information, and genome assembly data, among others, of all the selected strains are tabulated in Supplementary Table A.
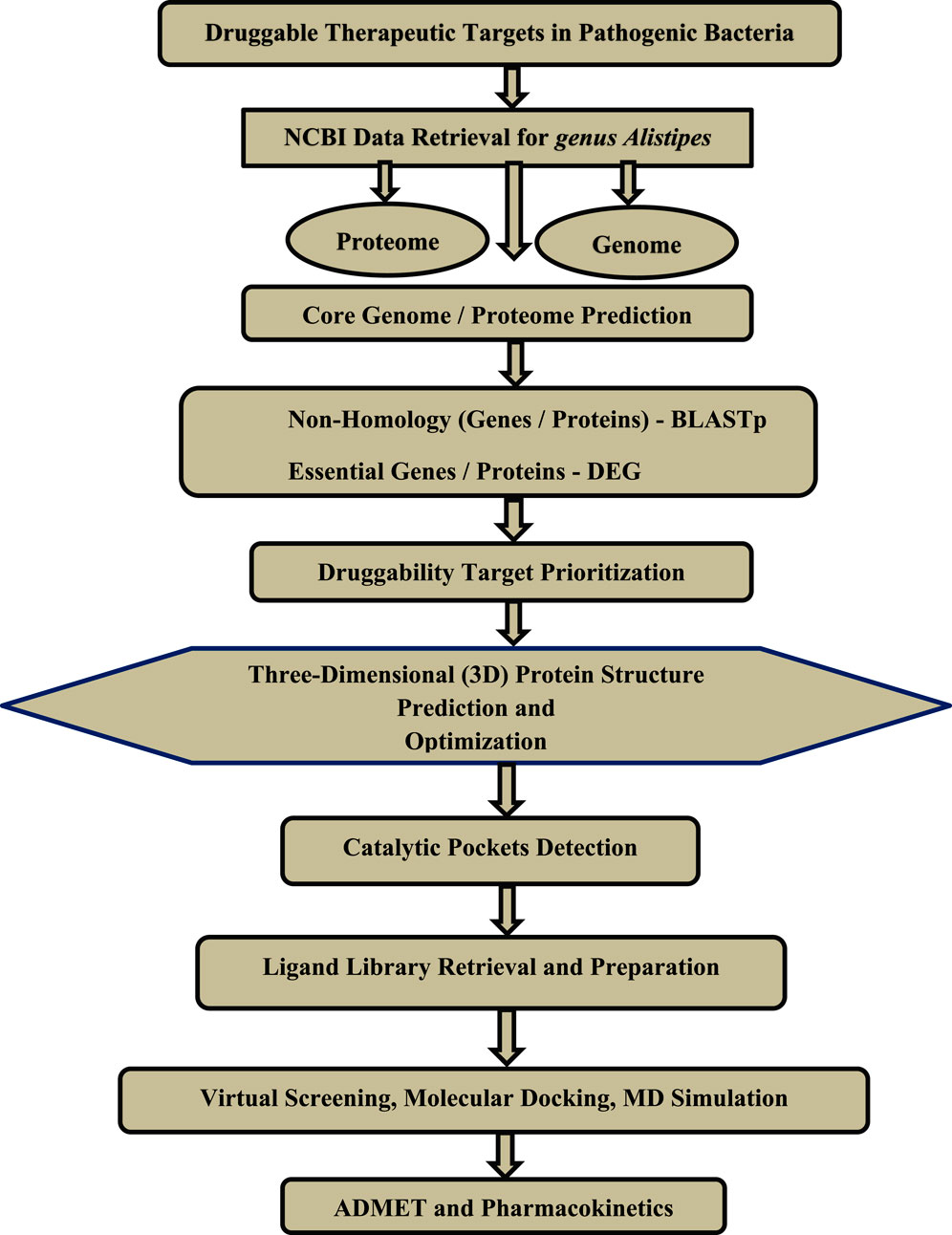
Figure 1. The general workflow of the step-by-step methodology followed in this study is based on subtractive genomics for the identification of druggable therapeutic targets.
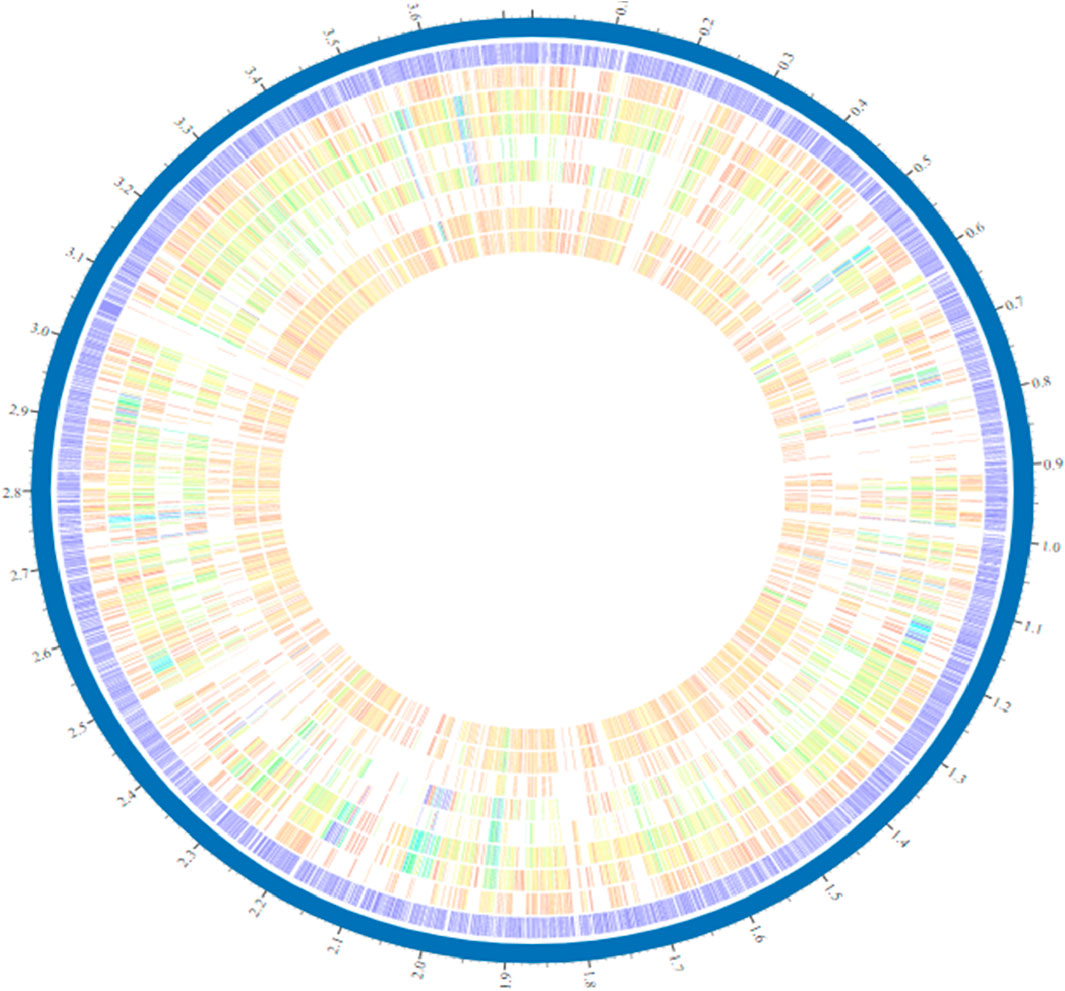
Figure 2. Circular genome representation of Alistipes generated through the PATRIC server. Among strains of the species Alistipes, varying hues and intensities indicate the existence or absence of distinct genes, genic islets, genomic islands, or other genetic elements.
3.2 Identification of non-host homologous and essential proteins
It is necessary to parse the file produced by the NCBI-BLASTp of the A. finegoldii core genome against the human genome. In total, 2,875 core genes were identified, and 2,571 proteins were found to be non-homologous with the human proteome. 344 genes were found to be host-homologous genes and were removed to avoid the resulting side effects. The BLASTp search was performed against prokaryotes, eukaryotes, and archaea for the host non-homologous proteins to determine the DEG essentiality (e-value = 10-4, bit score = 100 and sequence identity = ≥ 30%) (Luo et al., 2014). The results showed that out of the non-homologous set, 490 proteins are essential to Alistipes finegoldi. These steps are important for mining essential targets that are reportedly involved in performing vital cellular functions and avoiding cross-reactivity/binding of the drugs to undesired host protein sites.
3.3 Subcellular localisation and PPI for drug target prioritization
The essential targets were further processed for subcellular localisation prediction to refine the selection of potential drug targets, where 325 were cytoplasmic proteins, 150 were cytoplasmic-membrane proteins, 6 were inner-membrane proteins, and 1 was a periplasmic protein. Cytoplasmic proteins were selected as they are crucial for bacterial growth and metabolism and play essential roles in regulation, making them viable candidates for small-molecule inhibitors. Deciphering the protein-protein interactions network is very important in understanding the role of individual proteins and, thereby, in their classification and prioritisation as targets. After drug target prioritisation, a set of 39 proteins was submitted to the STRING database to identify hub proteins showing multiple interactions. The STRING database determines the interrelation between proteins, which is essential for proper functioning and gives detailed knowledge about proteins involved in single or multiple pathways. In total, nine proteins were shown to have numerous interactions and were thus classified as hub proteins and were considered for KEGG analyses (Figure 3).
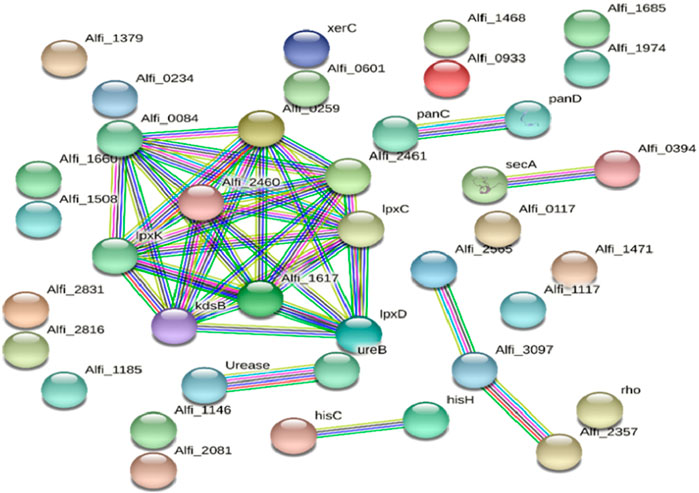
Figure 3. The STRING network of protein-protein interactions of the potential protein targets in Alistipes. The nodes and edges represent the proteins and their interactions, respectively, where the edge color represents the nature of the predicted interaction (experimentally curated or computationally predicted). The nodes are either shown as colored circles (query proteins and first shell of interactors) or white circles (second shell of interactors) and either empty circles (proteins of unknown 3D structure) or filled circles (3D structures are known or predicted). While line color reflects the type of interaction evidence and line width shows the strength of data support, the edges show both functional and physical protein interactions. Of the known interactions, those in purple have been determined experimentally, whereas those in cyan are from carefully selected databases. Gene neighborhood analyses are shown by green in expected interactions, gene fusion events by red, and gene co-occurrence by blue. The remaining relationships are navy blue = protein homology, black = co-expression, and olive = text-mining.
The cytoplasmic proteins were prioritised based on properties like molecular weight, virulence factor, druggability score, and pathway analysis. All the predicted potential proteins were also screened against the virulence factor database (VFDB) (Chen et al., 2005b), which predicted them to be involved in pathogen virulence. The molecular weight of all proteins was less than 100 kDa, according to ProtParam; thereby, these molecules fulfill the Lipinski threshold. Figure 4 displays how the total number of core genes was reduced and allocated during the subtractive genomic approach. Theoretically, the Lipinski’s rule of five applies to oral active drugs, which make up the biggest class of medicinal compounds. A maximum of one violation is permitted. This rule was intended to be a general guideline for the chemist to take care of these factors to avoid any issues rather than to specifically exclude molecules or compounds that violated these principles. Nevertheless, it was still possible to biologically analyse the tested compounds. Lenacapavir, an HIV medication, and hepatitis C virus medications like ledipasvir, velpatasvir, and voxilaprevir are excellent examples. Nonetheless, the rule of five continues to offer medication designers helpful limits. For optimal oral bioavailability, the Veber’s rule restricts the surface area to 140Å and the number of rotatable bonds to 10. The GI absorption and impaired permeability of the chosen compounds of interest across the membrane’s bilayer would generally be affected by any medicine that deviates from both of these guidelines. While more rotatable bonds can improve solubility and drug absorption, they can also decrease permeability (Lipinski, 2000; Lipinski et al., 2001; Caminero Gomes Soares et al., 2023; Hartung et al., 2023).
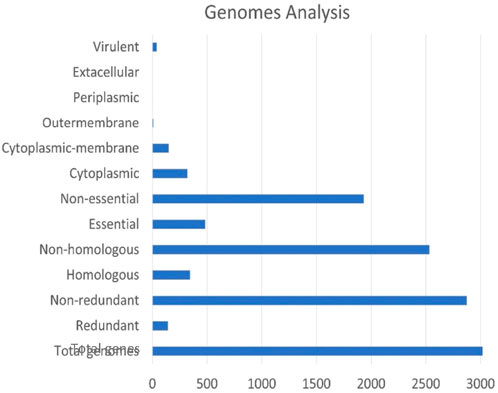
Figure 4. An overview of the screened proteins at the end of each subtractive genomics approach. The X-axis represents the total number of genes in all selected genomes, whereas the Y-axis represents the different steps of the subtractive genomics workflow towards compartmentalisation of genome portions based on their cellular function and localization.
3.4 Metabolic pathway analyses via KEGG
The query nine proteins were submitted to a pathway analysis using the KEGG database (Kanehisa et al., 2017). It is quite evident from the obtained results that most of the targets were engaged in multiple metabolic pathways, such as lipopolysaccharides biosynthesis and fatty acid biosynthesis, among others. In contrast, only one protein was involved in resistance pathways, such as the cationic antimicrobial peptide resistance pathway (CAMP) (Table 1; Figure 5).
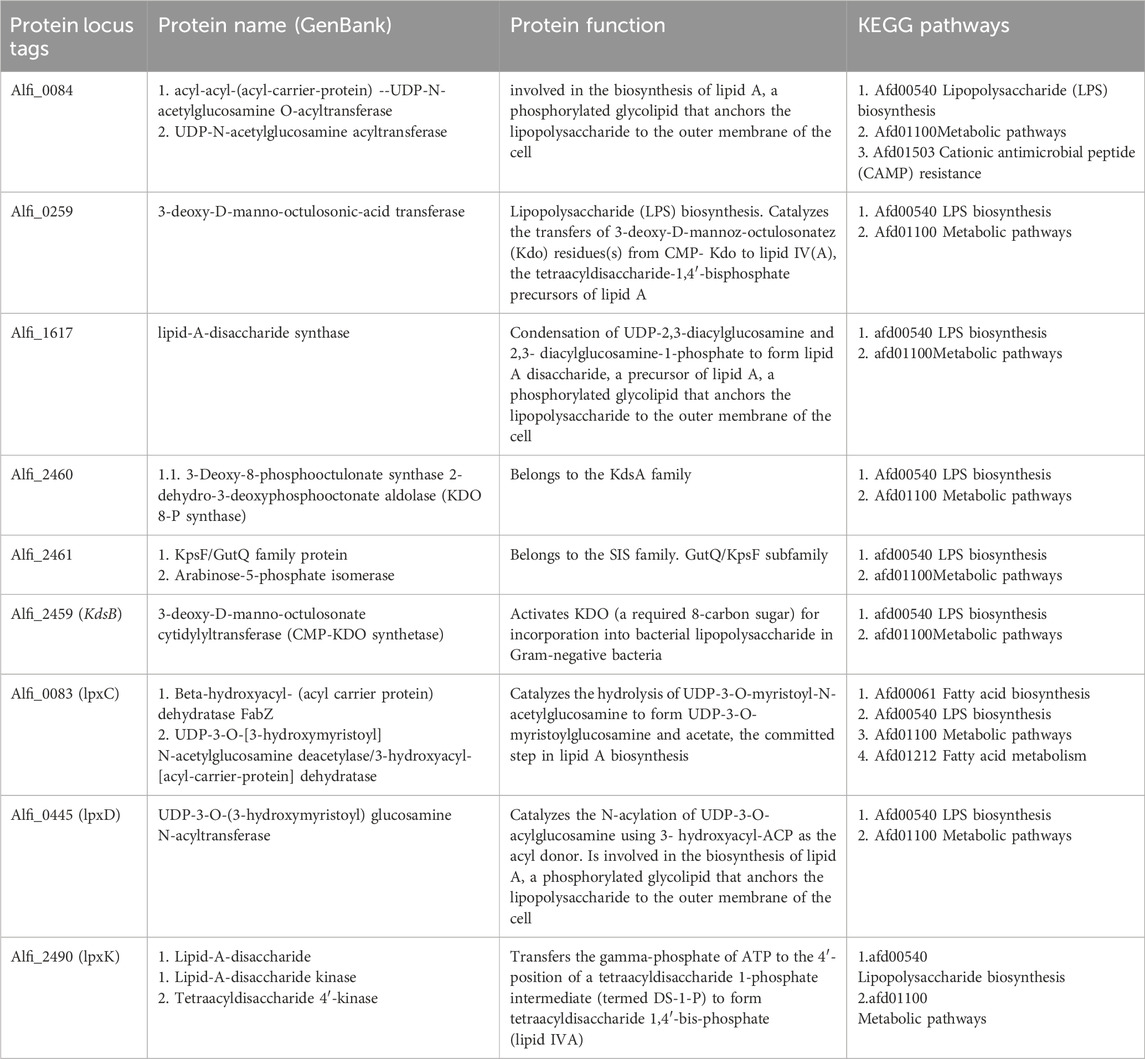
Table 1. Specific drug target prioritization criteria induced and functionally annotated for 9 non-host homologous proteins. Each identifier corresponds to a protein locus tag assigned by NCBI rather than specific protein names, which are given in a separate column.
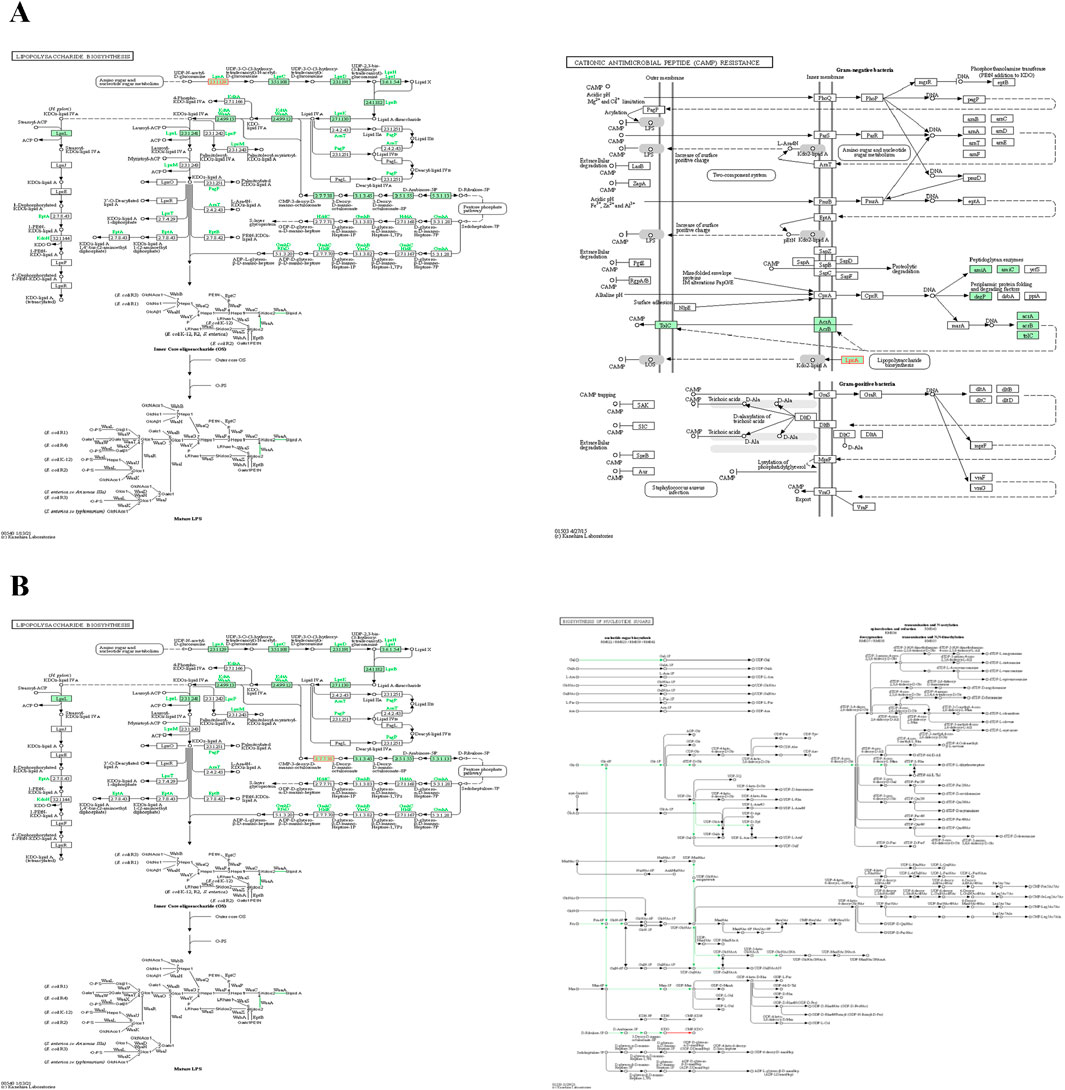
Figure 5. Important pathways of final targets (A) Alfi_0084 and (B) Alfi_2459. For a detailed description, the readers are referred to the online KEGG database for the genus Alistipes. Metabolites are shown by nodes, metabolite class is indicated by shape, and reactions are represented by lines.
3.5 Proteins 3d structure modeling and druggability assessment
From the set of nine proteins, only two cytoplasmic proteins were chosen as prospective therapeutic targets based on the metabolic pathways they are involved in and the % identity with their respective templates (RCSB-PDB, ≥25%). The 3D structure availability of a protein is the starting point for CADD analysis. The structure of identified druggable receptor proteins, i.e., the LpxA and KdsB, were generated through the SWISS-MODEL (Figure 6). The information on the active site residues was retrieved from the respective template structures for each putative target and was useful during the docking step for best inhibitor selection (Table 2). After cross-checking the stereochemical properties, the best 3D model structure was selected. Model 1 from the SWISS-MODEL was subjected to further analysis based on good physicochemical and quality measures. Besides significant coverage, it showed strong stereochemistry with no residue in the disallowed region and the lowest Z score. Energy minimisation was carried out to relax the structure and remove the steric clashes of the side chain. The physicochemical and Ramachandran properties of both models, where maximum residues are present in the most favored regions, are given in Table 3.
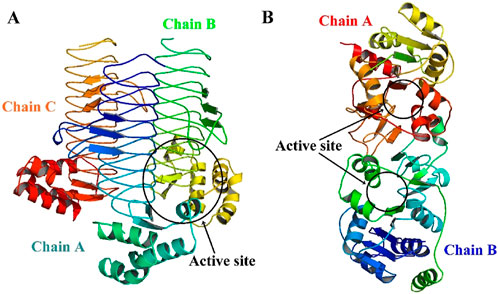
Figure 6. 3D structures of target proteins generated using SWISS-MODEL (A) LpxA (Alfi_0084) and (B) KdsB (Alfi_2459).
The DoGSiteScorer was used to establish a link between the drug-binding ability of the predicted targets based on their binding pocket structure and activity. The DoGSiteScorer does functional characterisation, druggability estimate, and automatically predicts pockets and sub-pockets in a target protein’s 3D structure. A protein that exhibits the highest contact affinity with a drug molecule is said to be highly druggable. On a scale of 0–1, the druggability measurement is scored as follows: ≥0.6 for medium-to-high druggable proteins and ≥0.8 for highly druggable proteins. Even though a protein of interest may have multiple anticipated druggable pockets, docking analyses typically only take into account the highly druggable pockets. The druggable pockets with their corresponding druggability statistics were identified for both LpxA and KdsB (Alfi_0084 and Alfi_2459), and are given in Table 4, only the highly druggable pockets are shown in Figure 7.
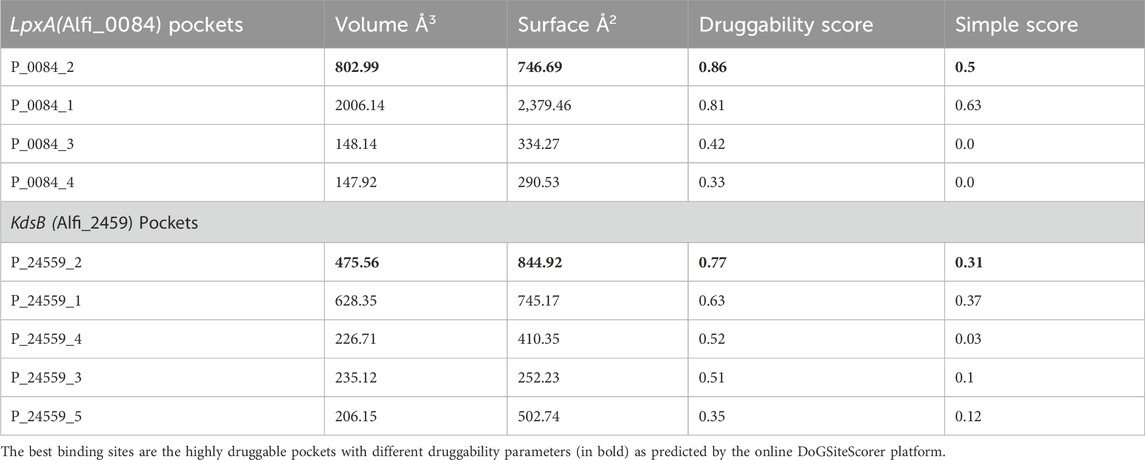
Table 4. DoGSiteScorer pockets and druggability scores for the Alfi_0084 (LpxA) and the Alfi_2459 (KdsB). Only the highly druggable pockets (bold) were considered further as the best binding sites.
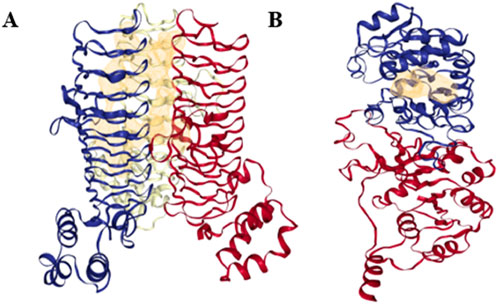
Figure 7. Highly druggable pockets of the final targets (A) LpxA (Alfi_0084), (B) KdsB (Alfi_2459). Only one highly druggable pocket is shown for each target protein in yellow mesh form as predicted by the DoGSiteScorer (druggable pocket ≥0.6, highly druggable pocket ≥0.8).
3.6 Molecular docking and ADMET profiling of top compounds
A total of 11,993 ZINC compounds were screened against two targets using the MOE docking pipeline. Based on known ligands of the template structures and their active site residues reported in the literature, lead compounds were identified for LpxA (Alfi_0084) and KdsB (Alfi_2459). The active site residues from the template structures were cross-validated with the druggable cavities identified using DoGSiteScorer and further analyzed using MOE and PyMOL tools to ensure precise ligand placement.
After uploading and preparing the receptor/protein models, MOE provided multiple cavity selection options, allowing us to confirm that docking was performed in the most biologically relevant and druggable sites. This step ensured that docking occurred within functionally significant binding pockets while also aligning with the druggability predictions. To maximize inhibitor screening, a second compound library from Traditional Chinese Medicine (TCM, n = 36,043) was also evaluated.
The top hits for each receptor were docked, and the five best compounds from each library were further analyzed based on binding affinity, hydrogen bonding interactions, and structural orientation within the receptor’s active sites. The docking poses were carefully examined in MOE to ensure that the best-ranked molecules occupied functionally critical residues, reinforcing the accuracy of the docking process (Table 5).
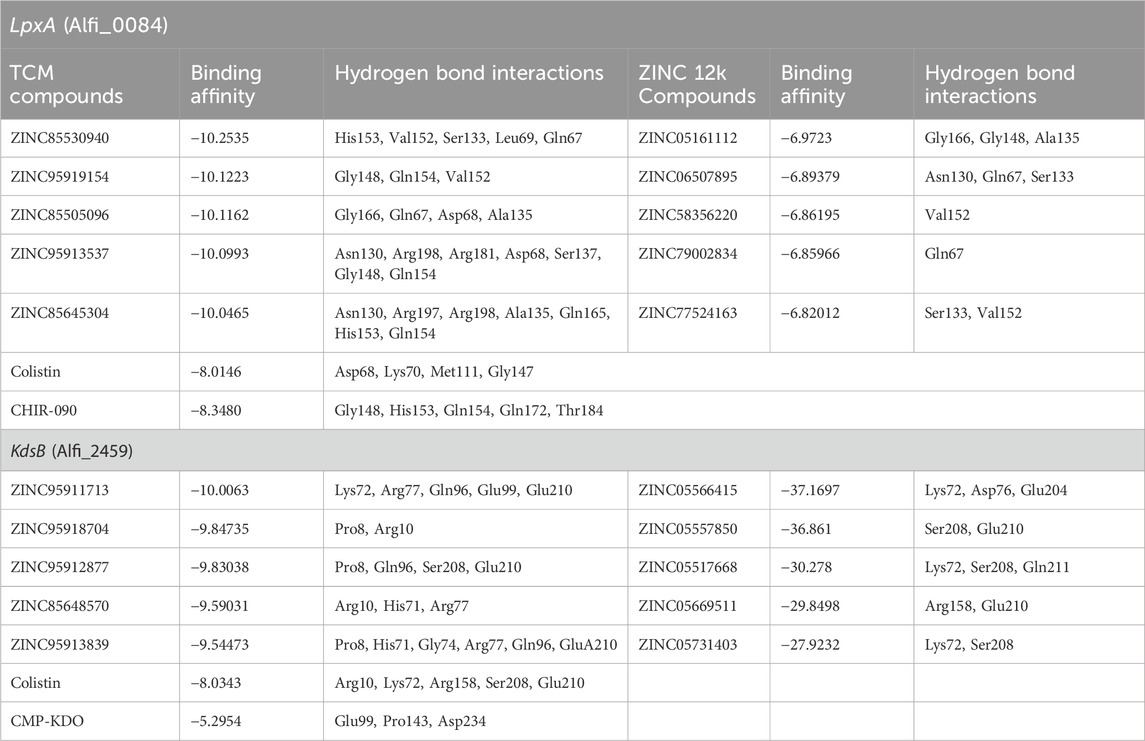
Table 5. Docking results of inhibitors arranged in descending order with corresponding binding affinities within the LpxA (Alfi_0084) and the KdsB (Alfi_2459) binding sites.
For convenience and simplicity, only the LpxA and KdsB terms will be used hereafter for the two identified targets. The ligand confirmation of the five top compounds from each library was performed according to the binding affinities with the receptor targets, the LpxA and the KdsB. The modeled structures of the LpxA and the KdsB which are active in lipopolysaccharide’s metabolism and other critical pathways were docked against five TCM hits with scores −10.2535 kcal/mol, −10.1223 kcal/mol, −10.1162 kcal/mol, −10.0993 kcal/mol and −10.0465 kcal/mol and −10.0063 kcal/mol, −9.84735 kcal/mol, −9.83038 kcal/mol, −9.59031 kcal/mol, −9.54473 kcal/mol, respectively. Amongst the ZINC12k library, the top hits exhibited scores of −6.9723 kcal/mol, −6.89379 kcal/mol, −6.86195 kcal/mol, −6.85966 kcal/mol, −6.82012 kcal/mol, and −37.1697 kcal/mol, −36.861 kcal/mol, −30.278 kcal/mol, −29.8498 kcal/mol, −27.9232 kcal/mol for the LpxA and the KdsB receptors. Notably, the stability of the complexes in terms of the binding energy scores for the ZINC12k componds was observed as the lowest one, ranging between −27 kcal/mol and 37 kcal/mol. (Table 5; Figures 8; Figure 11).
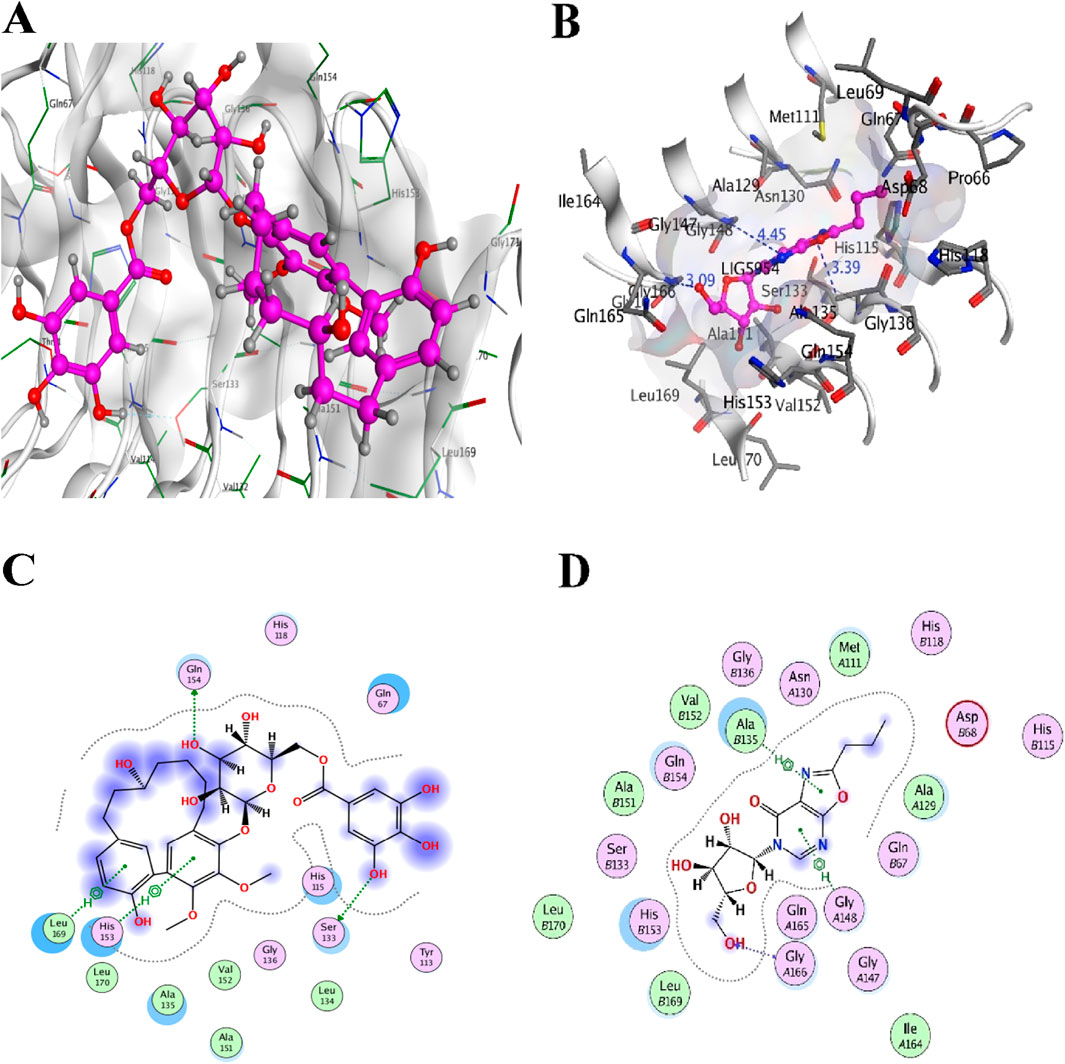
Figure 8. Top two inhibitors, each from TCM and ZINC libraries, respectively, are docked in the druggable cavity of the LpxA, representing hydrogen bond interactions (A, C) as 3D and 2D representation for ZINC85530940 and (B, D) as 3D and 2D representation for ZINC05161112).
Colistin and CHIR-090 are the reported potent inhibitors of the newly identified target LpxA of Alisipes, whereas Colistin again and CMP-KDO are the reported potent inhibitors of KdsB. The 2D/3D structures of these compounds were retrieved from the public databases and were docked as the reference compounds in the druggable cavities of the two target proteins. The number of H-bonds and the interacting residues, the S energy scores of the inhibitor-bound complexes and their 2D and 3D structural information are presented (Table 5; Figures 9; Figure 10). The binding affinities of the top TCM compounds are higher than the reference antibiotics with varying numbers and types of amino acid residues in the druggable cavities of both targets. However, the reference compounds exhibited higher affinities towards the druggable targets than the top ZINC compounds. On the other hand, the docked reference compounds for the KdsB have the lowest binding energy scores than the top compounds of both the TCM and ZINC databases. Again, the nature and number of the residues of the druggable cavities that made Hydrogen bond interactions remained different; in some cases, even higher numbers of interactions were observed for the reference antibiotic inhibitors (Table 5).
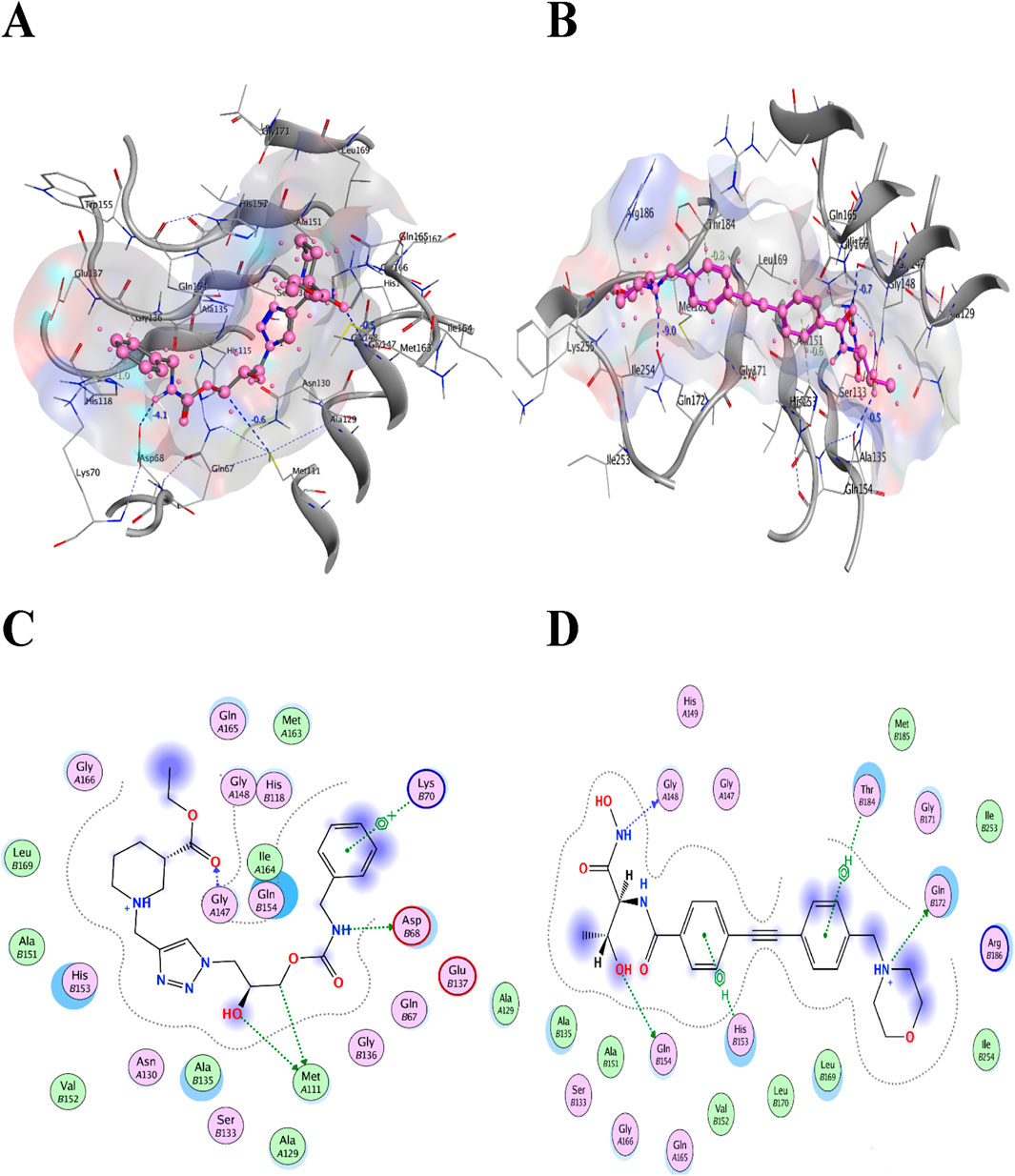
Figure 9. The two commonly used potent antibiotics are docked in the druggable cavity of the LpxA, representing hydrogen bond interactions (A, C) as 3D and 2D representation for Colistin and (B, D) as 3D and 2D representation for CHIR-090) and binding affinities of −8.0146 for Colistin and −8.3480 for CHIR-090, respectively.
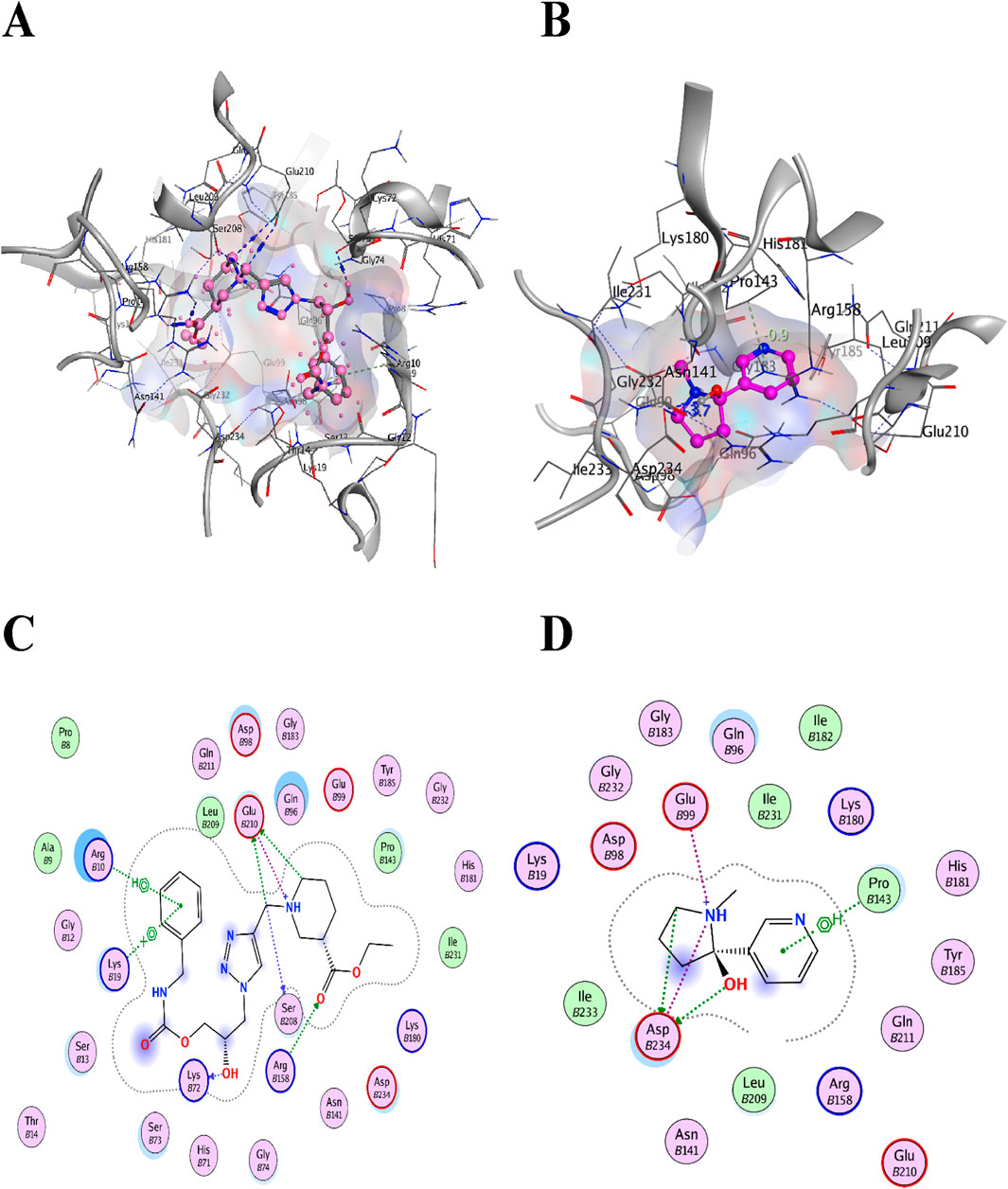
Figure 10. The two commonly used potent antibiotics are docked in the druggable cavity of the KdsB, representing hydrogen bond interactions (A, C) as 3D and 2D representation for Colistin and (B, D) as 3D and 2D representation for CMP-KDO) and binding affinities of −8.0343 for Colistin and −5.2954 for CMP-KDO, respectively.
Further, the physicochemical properties of the top hits were checked, including the Lipinski rule of 5 (LR-5), a prime condition to fulfill the rational drug design, and the bioactivity scores for putative drugs of oral use. The ZINC compounds revealed no violations for the LR-5, i.e., no more than five hydrogen bond donors, no more than ten hydrogen bond acceptors, logP (partition coefficient) not more than five, rotatable bonds less than 10, polar floor location not extra than 140 and a molecular weight less than 500 g/mol. The Log Po/w information are also included in Table 6 for all selected inhibitors, where the consensus Log Po/w value of compound ZINC95911713 is 6.53, which violates the LR-5 somehow, yet it can be observed that the consensus Log Po/w values of other three best hits from ZINC and TCM libraries are much lower than 3.5. However, the TCM compound ZINC85530940 exhibited three violations of the Lipinski rule and one of the Veber rule, even as ZINC95911713 confirmed violations for each rule. Different analyses carried out for the top hit compounds are shown below in Table 6.
Among the top five hits from TCM and ZINC12K libraries, ZINC85530940 and ZINC05161112 for LpxA and ZINC95911713 and ZINC05566415 for KdsB were selected, respectively, based on their least binding energies, the number of hydrogen bonds and an adequate orientation within the active site cavity. The goal of the study was to screen two different compound databases using a similar approach to identify potential inhibitors. The large differences in binding affinity predictions were the top compounds from TCM were the best candidates for LpxA, and the best compounds from ZINC 12 were the best for KdsB. In addition, since there is no reference for a binding affinity threshold, remarkable differences in the binding affinity of these inhibitors were observed. The interaction of inhibitors with their target binding sites could be attributed in terms of binding affinities, i.e., high affinities in inhibitor-receptor complexes result from greater attractive forces between the ligand and its receptor and vice versa.
According to the results, the top hits possess good bioactivity and drug-like properties, as shown in Table 6. The identified best docking pose revealed the most interacting residues in the active sites of the LpxA and the KdsB. The pose view within the druggable pockets showing interactions between the selected compounds and their respective individual targets are shown in Figures 8; Figure 11.
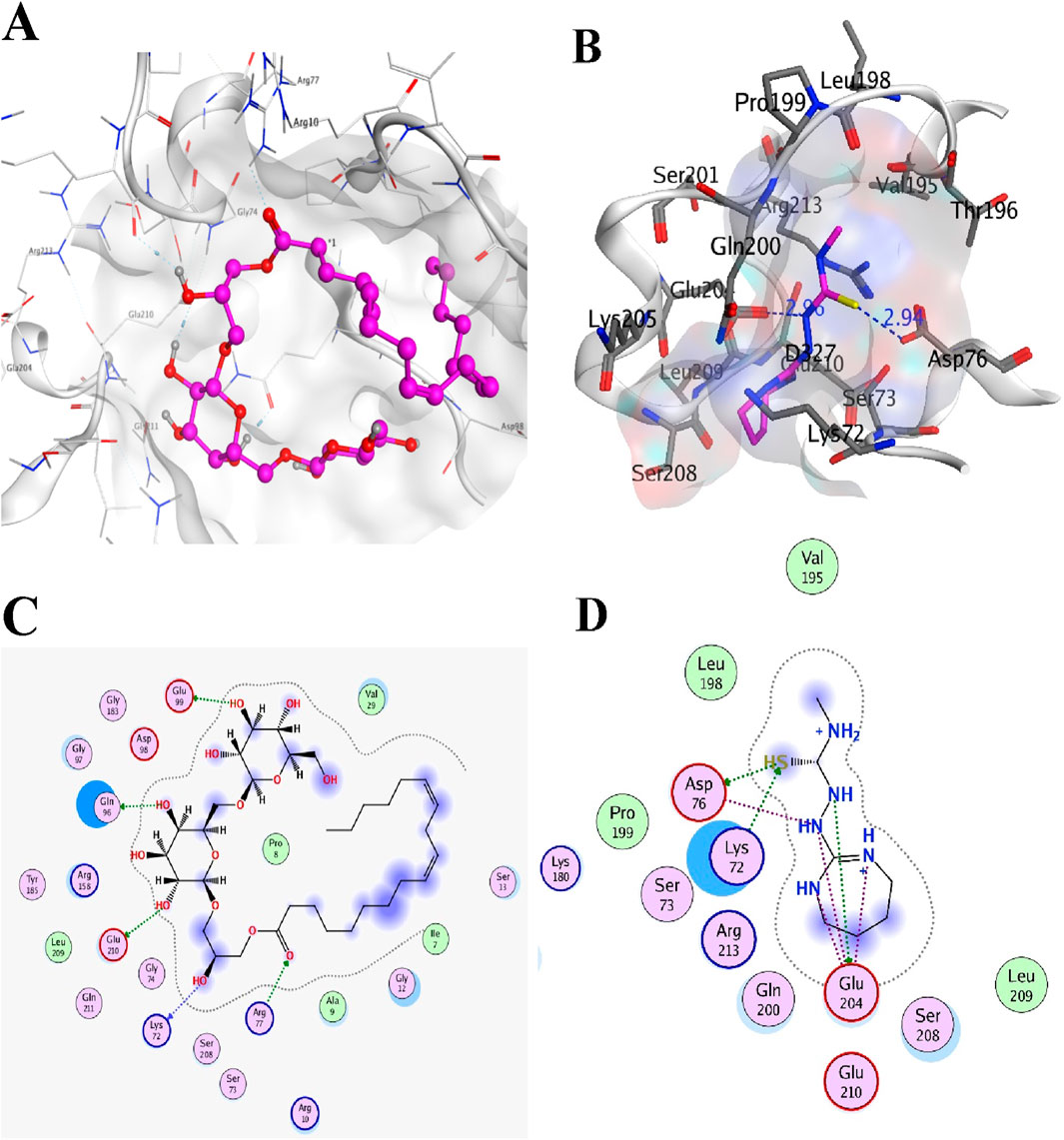
Figure 11. Top two inhibitors, each from TCM and ZINC libraries, respectively, are docked in the druggable cavity of the KdsB, representing hydrogen bond interactions (A, C) as 3D and 2D representation for ZINC95911713 and (B, D) as 3D and 2D representation for ZINC05566415).
3.7 Molecular dynamic simulation
The most fundamental element associated with the function of proteins is their conformational dynamics. Functional information of protein molecules is encrypted in its structure. To unravel its functional variability, a comprehensive understanding of the structure is needed. Here, the MD simulation was performed as per the available computational facilities at the time this study was conducted to explore the conformational aspect of protein-ligand interactions and to evaluate the stability of the homology model and enzyme inhibitor complex. Data analysis like root mean square deviation (RMSD), root mean square fluctuation (RMSF), radius of gyration (Rg) and β-factor values, solvent-accessible surface area (SASA), and Binding energies were used to determine the conformational changes and stability index of secondary structure elements of the simulated complexes.
3.7.1 Root mean square deviation (RMSD)
RMSD explains the backbone analysis and Cα atoms dynamics over some time of docked protein (Figure 12). For both the LpxA and the KdsB, minor fluctuations were observed initially at the start of the simulation, but as the simulation proceeded, stability was observed for the apo and the docked complexes. The average RMSD value for the LpxA-apo was 3.7Å with an SD of 0.4; for ZINC05161112, the RMSD value was 3.67Å with an SD of 0.48, and for ZINC85830940, the value stabilized at 3.49 Å with an SD of 0.58. The average RMSD value for the KdsB was 4.59Å with an SD of 0.56; for ZINC5566415, the value was 4.51Å with an SD of 0.40, while ZINC95911713 exhibited an RMSD of 3.4 Å with an SD of 0.2, exhibiting more stability than any other docked or apoprotein structure. Overall, the pattern of the RMSD graph does not support any major domain shifts within the structural framework of the protein-ligand complex. The placement of ligands was well-complemented within the binding site during the simulation and stabilized the protein significantly Figure 12.
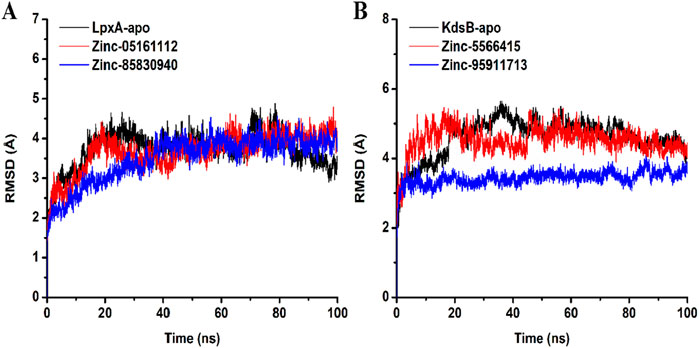
Figure 12. RMSD plot of the LpxA (A) and the KdsB (B) for apo and ligand-bound complex over 100ns simulation run.
3.7.2 Root mean square fluctuations (RMSF)
Structure flexibility and fluctuation of Cα residues over time were observed by the RMSF graph analysis. The RMSF values of the apo LpxA protein calculated were highly in line with the RMSF values obtained for the docked complexes. Some major fluctuations were observed for ZINC05161112 at 327th residue having 4.1Å and 352nd residue exhibiting 2.9 Å that was unique to this docked complex. For ZINC85830940, residue 607th exhibited a 3.2Å RMSF value that was stable for the other docked and apoprotein. The RMSF of the remaining residues depicted comparably more compactness of the ligand-bound protein than the apo LpxA protein. For the KdsB protein, the RMSF values were much lower, depicting more compactness than the apoprotein. The loop region of the ZINC95911713-bound KdsB protein at the N terminal region exhibited more fluctuations. However, overall, the RMSF graph represented much more compactness in the ligand-bound KdsB protein compared to the apoprotein (Figure 13).
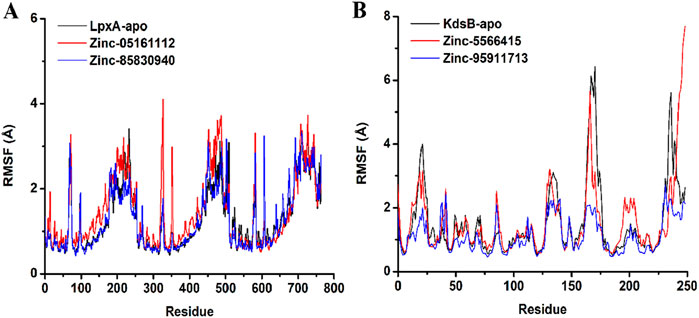
Figure 13. RMSF plot of the LpxA (A) and the KdsB (B) for apo and ligand-bound complex over 100ns simulation run.
3.7.3 β-Factor analysis
β-Factor explains the thermal stability and flexibility of the protein over some time. The quantity of β-factor is measured in RMSF. Its value on the level of localized atomic fluctuation collectively contributes to the global vibrational movement of the protein and its thermal stability. The β-factor value for ZINC05161112 at 327th residue and 352nd residue exhibited higher thermal instability that was exactly in line with the RMSF results. Similarly, for ZINC85830940, residue 607th demonstrated higher thermal instability in that region. Few residues lying between 450th – 460th position for both ZINC compounds also showed a certain degree of thermal instability and thermal flexibility and were cross-checked with the corresponding RMSF observations and were found similar. In contrast, the protein KdsB-apo and the ZINC5566415-bound KdsB exhibited fewer elevated thermal instabilities at around the 170th residue and at the 235th residue. Interestingly, the ZINC95911713-bound KdsB exhibited comparatively global vibrational and thermal stabilities at the aforementioned position as well as at other positions during the whole simulation period. Collectively, the β-factor and the RMSF values are in line, showing the accuracy of the simulation (Figure 14).
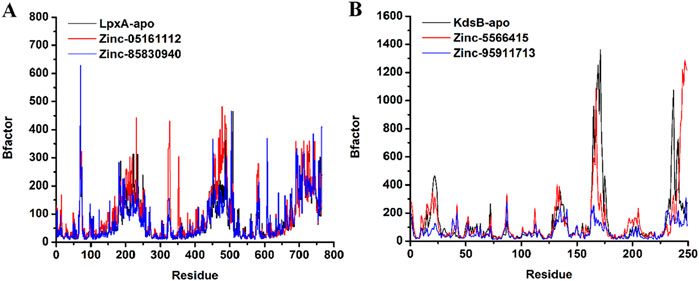
Figure 14. β-Factor plot of the LpxA (A) and the KdsB (B) for apo and ligand-bound complex over 100ns simulation run.
3.7.4 Radius of gyration (Rg)
The radius of gyration was calculated to evaluate the structural compactness as a function of time for apo and protein-ligand complex. The average Rg value for apoprotein was recorded as 28.1Å with an SD of 0.3 while ZINC05161112 bound the LpxA protein exhibited an average Rg value of 28.05 with an SD of 0.3 and ZINC85830940 exhibited an average Rg score of 27.93 with Sd of 0.4. For the KdsB apo protein, the Rg value was recorded as 18.73 with an SD of 0.16, while ZINC5566415 bound KdsB exhibited 18.99 with an SD of 0.16 and ZINC95911713 exhibited 18.77 with an SD of 0.1. These values suggest that the overall globularity of the protein upon ligand binding was neither decreased nor increased but remained consistent with the apoprotein structure (Figure 15).
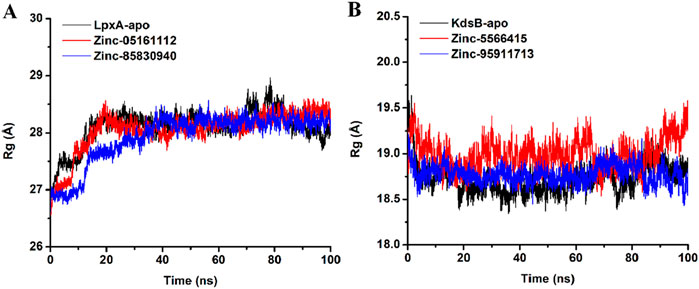
Figure 15. Radius of gyration (Rg) plot of the LpxA (A) and the KdsB (B) for apo and ligand-bound complex over 100ns simulation run.
3.7.5 Evaluation of hydrogen bonds
Hydrogen bond analysis provides an essential understanding of the intramolecular hydrogen bond network of apo and the ligand-bound LpxA and the KdsB proteins. Figure 16 provides deep insights into the Hydrogen bond network of both the apoproteins structures and ligand binding. Careful evaluation of the number of H-bonds revealed that the average number of H-bonds for the apo LpxA protein was 702.9, while upon binding of ZINC05161112, the average number of H-bonds increased to 709.7, and upon binding of ZINC85830940, the average H-bonds decreased to 701.8. The results depict that upon ligand binding, the number of H-bonds significantly varied between both the LpxA proteins. For the KdsB protein, the apo form exhibited 200.6 average H-bonds, while the ligand-bound KdsB protein recorded the average number of H-bonds as 203.10 and 206.48 for ZINC5566415 and ZINC95911713, respectively (Figure 16B). The H-bond number significantly increased upon ligand binding which depicts that the KdsB protein achieved stability and favors the complex form rather than the apo form.
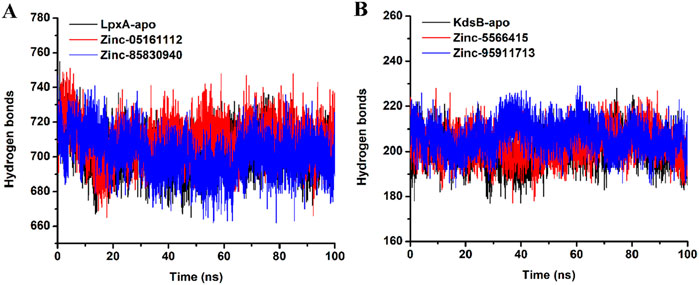
Figure 16. H-bonds plot of the LpxA (A) and the KdsB (B) for apo and ligand-bound complex over 100ns simulation run.
3.7.6 Solvent-Accessible Surface Area
The SASA (Solvent-accessible surface area) parameter determines how much of the protein surface is accessible to the aqueous solvent. The SASA formula can be used to estimate the magnitude of conformational fluctuations that occurred during contact. The plot of SASA values vs time for all complexes is shown in Figure 17. Through molecular dynamic simulations, the average SASA of apo-LpxA is 32,324.7, while for ZINC05161112 bound protein complex was 32,088.23 and ZINC85830940 bound LpxA was recorded as 31,323.2. On the other hand, the apo-KdsB protein exhibited 13,025, while ZINC5566415 and ZINC95911713 exhibited 13,225.8 and 12,315.7, respectively. All of the ligand-bound bound-LpxA complexes exhibited lower SASA values, while for the KdsB, the SASA values for ZINC5566415 showed inconsistent increase at different time intervals while having a steep decrease for ZINC95911713. As a result of our SASA research, we have observed that the LpxA protein-ligand complex is rather more stable than the KdsB complex.
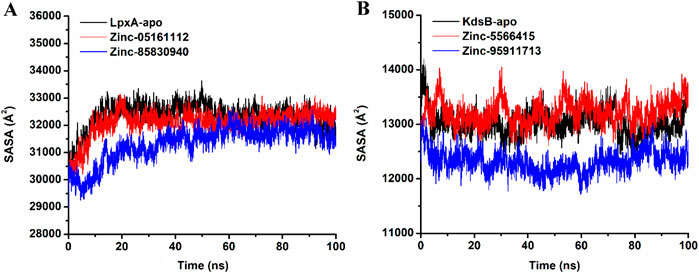
Figure 17. Solvent-accessible surface area (SASA) plot of the LpxA (A) and the KdsB (B) for apo and ligand-bound complex over 100ns simulation run.
LpxA: LpxA (UDP-N-acetylglucosamine O-acyltransferase) is essential for lipid A synthesis, a core component of lipopolysaccharide (LPS) in Gram-negative bacteria, including Alistipes. LPS forms a protective outer layer that enables bacterial evasion of immune responses and triggers strong inflammatory responses by activating Toll-like receptor 4 (TLR4) in host cells, a mechanism associated with chronic intestinal inflammation and gut dysbiosis (Raetz and Whitfield, 2002). By inhibiting LpxA, lipid A biosynthesis can be disrupted, weakening the bacterial cell membrane and enhancing susceptibility to immune clearance (Hornef et al., 2002). Studies have shown that targeting LpxA can significantly reduce bacterial viability and virulence, making it a promising therapeutic target for controlling bacterial infections (Emiola et al., 2015; Wang and Quinn, 2010).
KdsB: KdsB (3-deoxy-D-manno-octulosonate cytidylyltransferase) is another crucial enzyme in LPS biosynthesis (Schmidt et al., 2011). It facilitates the incorporation of Kdo (3-deoxy-D-manno-octulosonic acid) into lipid A, which is vital for bacterial viability. Inhibiting KdsB disrupts LPS integrity, making the bacterial outer membrane more susceptible to immune responses and reducing virulence. Mutations in KdsB are lethal for bacteria, indicating its essential role in maintaining LPS structure and function (Alfahemi, 2020). Research supports the development of small-molecule inhibitors for KdsB, demonstrating its potential as a drug target for antimicrobial therapies (Schmidt et al., 2011; Ahmad et al., 2019).
It is important to note that in the present work, the identified compounds are from ZINC and TCM libraries, which are commercially available and are ready to “prepare on demand”. Furthermore, the ADMET and pharmacokinetic profiling of the selected identified compounds/inhibitors primarily elucidate their safety measures, which renders them “best hits” for futuristic in vitro and other mechanistic analyses. The overall interaction scores, number of H-bonds, and other thermodynamic parameters are good predicted indicators and thus hypothesize the inhibitory effectiveness of all the final hits.
4 Conclusion
The increasing resistance of bacterial pathogens to antibiotics underscores the urgent need for novel drug targets and therapeutic strategies. In this study, we employed a subtractive genomics and pangenome analysis approach to identify potential therapeutic targets in Alistipes, a gut-associated opportunistic pathogen. Through a systematic computational pipeline, LpxA and KdsB were identified as essential, non-host homologous, and druggable proteins, making them promising targets for antibacterial drug development. Further molecular docking and ADMET profiling of these targets against two extensive compound libraries, the ZINC database (n = 11,993) and the Traditional Chinese Medicine (TCM) database (n = 36,043), led to the identification of ZINC05161112, ZINC85530940, ZINC05566415, and ZINC9591171 as potential inhibitors. Molecular dynamics (MD) simulations further confirmed the stability and binding efficacy of these compounds within the active sites of LpxA and KdsB, suggesting their potential as lead drug candidates.
While this study provides a strong computational framework for robust drug discovery that addresses an urgent need for novel antimicrobial strategies, there are certain limitations. The lack of experimental validation remains a key point at this stage, and future studies are required that should focus on in vitro and in vivo assessments to confirm the biological efficacy of the identified inhibitors. Additionally, structural refinement of the lead compounds may enhance their pharmacokinetic properties and therapeutic potential. Further exploration of Alistipes metabolic pathways could also uncover additional druggable targets to complement the inhibition of LpxA and KdsB.
In conclusion, with a certain degree of modifications, this research and other similar approaches lead directly or indirectly to fulfill the objective of identifying novel drug targets in Alistipes and provide a comprehensive computational strategy for structure-based drug discovery (Hassan et al., 2012; 2018; 2023; Pethick et al., 2012b; 2012a; Dorella et al., 2013; Basharat et al., 2022; Afzal et al., 2023; Fatima et al., 2023; Kalhor et al., 2023; Alqurashi et al., 2024). The findings serve as a foundation for future experimental validation and clinical investigations aiming to develop targeted therapeutic interventions against Alistipes-associated infections.
Data availability statement
The original contributions presented in the study are included in the article/supplementary material, further inquiries can be directed to the corresponding author.
Author contributions
SS: Conceptualization, Data curation, Formal Analysis, Investigation, Methodology, Software, Validation, Visualization, Writing – original draft, Writing – review and editing. AW: Conceptualization, Data curation, Formal Analysis, Investigation, Methodology, Software, Validation, Visualization, Writing – original draft, Writing – review and editing. SR: Supervision, Validation, Visualization, Writing – original draft, Writing – review and editing, RA: Investigation, Methodology, Validation, Visualization, Writing – original draft, Writing – review and editing. AU: Supervision, Validation, Visualization, Writing – original draft, Writing – review and editing. F-AK: Supervision, Validation, Visualization, Writing – original draft, Writing - review and editing. MI: Investigation, Methodology, Validation, Visualization, Writing – original draft, Writing – review and editing. MI: Supervision, Validation, Visualization, Writing – original draft, Writing – review and editing, PC: Investigation, Methodology, Software, Validation, Visualization, Writing – original draft, Writing – review and editing. RR: Investigation, Validation, Visualization, Writing – original draft, Writing – review and editing. FS: Supervision, Visualization, Writing – original draft, Writing – review and editing, SH: Conceptualization, Supervision, Writing – original draft, Writing – review and editing.
Funding
The author(s) declare that no financial support was received for the research and/or publication of this article.
Acknowledgments
We would like to acknowledge the mutual research/academic collaboration of authors from different institutions, including JRC Genome Research, ICCBS, University of Karachi, Karachi-Pakistan, Department of Chemistry, Islamia College Peshawar–Pakistan, CDTS_Oswaldo Fiocruz, RJ - CAPES, Brazil and other national/international academic/research organizations. The author/s would like to thank the Deanship of Scientific Research at Shaqra University, Saudi Arabia, for supporting this work. The authors express their appreciation to the Deanship of Scientific Research at King Khalid University, Saudi Arabia, for this work through a research group program under grant number RGP-2/518/46.
Conflict of interest
The authors declare that the research was conducted in the absence of any commercial or financial relationships that could be construed as a potential conflict of interest.
Generative AI statement
The author(s) declare that no Generative AI was used in the creation of this manuscript.
Publisher’s note
All claims expressed in this article are solely those of the authors and do not necessarily represent those of their affiliated organizations, or those of the publisher, the editors and the reviewers. Any product that may be evaluated in this article, or claim that may be made by its manufacturer, is not guaranteed or endorsed by the publisher.
References
Afzal, M., Hassan, S. S., Sohail, S., Camps, I., Khan, Y., Basharat, Z., et al. (2023). Genomic landscape of the emerging XDR Salmonella Typhi for mining druggable targets clpP, hisH, folP and gpmI and screening of novel TCM inhibitors, molecular docking and simulation analyses. BMC Microbiol. 23, 25. doi:10.1186/s12866-023-02756-6
Agüero, F., AL-Lazikani, B., Aslett, M., Berriman, M., Buckner, F. S., Campbell, R. K., et al. (2008). Genomic-scale prioritization of drug targets: the TDR Targets database. Nat. Rev. Drug Discov. 7, 900–907. doi:10.1038/nrd2684
Ahmad, S., Raza, S., Abro, A., Liedl, K. R., and Azam, S. S. (2019). Toward novel inhibitors against KdsB: a highly specific and selective broad-spectrum bacterial enzyme. J. Biomol. Struct. Dyn. 37, 1326–1345. doi:10.1080/07391102.2018.1459318
Alfahemi, H. H. J. (2020). Purification of the KdsB protein of Escherichia coli and studies on the phenotype of a kdsB mutant in a K1 capsule expressing strain. United Kingdom: The University of Manchester.
Alqurashi, A., Ahmad, W., Rahman, Z., Nawab, J., Siddiqui, M. F., Akbar, A., et al. (2024). Identification of a novel drug target in Porphyromonas gingivalis by a computational genome analysis approach. Open Chem. 22, 20240037. doi:10.1515/chem-2024-0037
Arnold, K., Bordoli, L., Kopp, J., and Schwede, T. (2006). The SWISS-MODEL workspace: a web-based environment for protein structure homology modelling. Bioinforma. Oxf. Engl. 22, 195–201. doi:10.1093/bioinformatics/bti770
Barh, D., Misra, A. N., and Anil, K. (2010a). In silico identification of dual ability of N. Gonorrhoeae ddl for developing drug and vaccine against pathogenic Neisseria and other human pathogens. J. Proteomics and Bioinforma. 3.
Barh, D., Misra, A. N., Kumar, A., and Vasco, A. (2010b). A novel strategy of epitope design in Neisseria gonorrhoeae. Bioinformation 5, 77–85. doi:10.6026/97320630005077
Barker, S. A., and Khossravi, D. (2001). Drug delivery strategies for the new Millennium. Drug Discov. Today 6, 75–77. doi:10.1016/s1359-6446(00)01634-2
Basharat, Z., Akhtar, U., Khan, K., Alotaibi, G., Jalal, K., Abbas, M. N., et al. (2022). Differential analysis of Orientia tsutsugamushi genomes for therapeutic target identification and possible intervention through natural product inhibitor screening. Comput. Biol. Med. 141, 105165. doi:10.1016/j.compbiomed.2021.105165
Basson, A. R., Cominelli, F., and Rodriguez-Palacios, A. (2020). Patterns of ‘analytical irreproducibility’in multimodal diseases. bioRxiv.
Bellali, S., Naud, S., Ndongo, S., Lo, C. I., Anani, H., Raoult, D., et al. (2019). Corynebacterium pacaense sp. nov., Alistipes megaguti sp. nov., Alistipes provencensis sp. nov., 3 new bacteria isolated from fresh human stool specimens. New Microbes New Infect. 32, 100593. doi:10.1016/j.nmni.2019.100593
Boutet, E., Lieberherr, D., Tognolli, M., Schneider, M., and Bairoch, A. (2007). UniProtKB/Swiss-Prot. Methods Mol. Biol. Clift. N.J. 406, 89–112. doi:10.1007/978-1-59745-535-0_4
Caminero Gomes Soares, A., Marques Sousa, G. H., Calil, R. L., and Goulart Trossini, G. H. (2023). Absorption matters: a closer look at popular oral bioavailability rules for drug approvals. Mol. Inf. 42, e202300115. doi:10.1002/minf.202300115
Chen, L., Yang, J., Yu, J., Yao, Z., Sun, L., Shen, Y., et al. (2005a). VFDB: a reference database for bacterial virulence factors. Nucleic Acids Res. 33, D325–D328. doi:10.1093/nar/gki008
Chen, L., Yang, J., Yu, J., Yao, Z., Sun, L., Shen, Y., et al. (2005b). VFDB: a reference database for bacterial virulence factors. Nucleic Acids Res. 33, D325–D328. doi:10.1093/nar/gki008
Consortium, U. (2015). UniProt: a hub for protein information. Nucleic acids Res. 43, D204–D212. doi:10.1093/nar/gku989
Daina, A., Michielin, O., and Zoete, V. (2017). SwissADME: a free web tool to evaluate pharmacokinetics, drug-likeness and medicinal chemistry friendliness of small molecules. Sci. Rep. 7, 42717. doi:10.1038/srep42717
Dorella, F. A., Gala-Garcia, A., Pinto, A. C., Sarrouh, B., Antunes, C. A., Ribeiro, D., et al. (2013). Progression Of ‘Omics’ Methodologies For Understanding The Pathogenicity Of Corynebacterium Pseudotuberculosis: The Brazilian Experience. Comput. Struct. Biotechnol. J. 6, e201303013. doi:10.5936/csbj.201303013
Eddy, S. R. (2004). Where did the BLOSUM62 alignment score matrix come from? Nat. Biotechnol. 22, 1035–1036. doi:10.1038/nbt0804-1035
Eisenberg, D., Lüthy, R., and Bowie, J. U. (1997). VERIFY3D: assessment of protein models with three-dimensional profiles. Methods Enzym. 277, 396–404. doi:10.1016/s0076-6879(97)77022-8
Emiola, A., George, J., and Andrews, S. S. (2015). A complete pathway model for lipid A biosynthesis in Escherichia coli. PloS one 10, e0121216. doi:10.1371/journal.pone.0121216
Fatima, S., Akbar, A., Irfan, M., Shafee, M., Ali, A., Ishaq, Z., et al. (2023). Virulence factors and antimicrobial resistance of uropathogenic Escherichia coli EQ101 UPEC isolated from UTI patient in quetta, balochistan, Pakistan. BioMed Res. Int. 2023, 7278070. doi:10.1155/2023/7278070
Gasteiger, E., Hoogland, C., Gattiker, A., Duvaud, S. E., Wilkins, M. R., Appel, R. D., et al. (2005). “Protein identification and analysis tools on the ExPASy server,” in The proteomics protocols handbook. Editor J. M. WALKER (Totowa, NJ: Humana Press).
Geiger, O., González-Silva, N., López-Lara, I. M., and Sohlenkamp, C. (2010). Amino acid-containing membrane lipids in bacteria. Prog. Lipid Res. 49, 46–60. doi:10.1016/j.plipres.2009.08.002
Goodrich, J. K., Davenport, E. R., Clark, A. G., and Ley, R. E. (2017). The relationship between the human genome and microbiome comes into view. Annu. Rev. Genet. 51, 413–433. doi:10.1146/annurev-genet-110711-155532
Halfvarson, J., Brislawn, C. J., Lamendella, R., Vazquez-Baeza, Y., Walters, W. A., Bramer, L. M., et al. (2017). Dynamics of the human gut microbiome in inflammatory bowel disease. Nat. Microbiol. 2, 17004. doi:10.1038/nmicrobiol.2017.4
Hartung, I. V., Huck, B. R., and Crespo, A. (2023). Rules were made to be broken. Nat. Rev. Chem. 7, 3–4. doi:10.1038/s41570-022-00451-0
Hassan, S. S., Guimarães, L. C., Pereira, U. D. P., Islam, A., Ali, A., Bakhtiar, S. M., et al. (2012). Complete genome sequence of Corynebacterium pseudotuberculosis biovar ovis strain P54B96 isolated from antelope in South Africa obtained by rapid next generation sequencing technology. Stand. Genomic Sci. 7, 189–199. doi:10.4056/sigs.3066455
Hassan, S. S., Jamal, S. B., Radusky, L. G., Tiwari, S., Ullah, A., Ali, J., et al. (2018). The druggable pocketome of corynebacterium diphtheriae: a new approach for in silico putative druggable targets. Front. Genet. 9, 44. doi:10.3389/fgene.2018.00044
Hassan, S. S., Shams, R., Camps, I., Basharat, Z., Sohail, S., Khan, Y., et al. (2023). Subtractive sequence analysis aided druggable targets mining in Burkholderia cepacia complex and finding inhibitors through bioinformatics approach. Mol. Divers 27, 2823–2847. doi:10.1007/s11030-022-10584-5
Hassan, S. S., Tiwari, S., Guimarães, L. C., Jamal, S. B., Folador, E., Sharma, N. B., et al. (2014). Proteome scale comparative modeling for conserved drug and vaccine targets identification in Corynebacterium pseudotuberculosis. BMC Genomics 15, S3. doi:10.1186/1471-2164-15-S7-S3
Hornef, M. W., Wick, M. J., Rhen, M., and Normark, S. (2002). Bacterial strategies for overcoming host innate and adaptive immune responses. Nat. Immunol. 3, 1033–1040. doi:10.1038/ni1102-1033
Hossain, M. U., Khan, M., Hashem, A., Islam, M., Morshed, M. N., Keya, C. A., et al. (2016). Finding potential therapeutic targets against Shigella flexneri through proteome exploration. Front. Microbiol. 7, 1817. doi:10.3389/fmicb.2016.01817
Irfan, M., Tariq, M., Basharat, Z., Khan, R. M. A., Jahanzaeb, M., Shakeel, M., et al. (2023). Genomic analysis of Chryseobacterium indologenes and conformational dynamics of the selected DD-peptidase. Res. Microbiol. 174, 103990. doi:10.1016/j.resmic.2022.103990
Jorgensen, W. L., Chandrasekhar, J., Madura, J. D., Impey, R. W., and Klein, M. L. (1983). Comparison of simple potential functions for simulating liquid water. J. Chem. Phys. 79, 926–935. doi:10.1063/1.445869
Kalhor, H., Noori Goodarzi, N., Rahimi, H., Firoozeh, F., and Badmasti, F. (2023). Identification of novel drug targets in Porphyromonas gingivalis and proposing inhibitors against acetate kinase using structure-based virtual screening. Process Biochem. 130, 310–321. doi:10.1016/j.procbio.2023.04.016
Kanehisa, M., Furumichi, M., Tanabe, M., Sato, Y., and Morishima, K. (2017). KEGG: new perspectives on genomes, pathways, diseases and drugs. Nucleic Acids Res. 45, D353–D361. doi:10.1093/nar/gkw1092
Kanehisa, M., and Goto, S. (2002). KEGG: kyoto encyclopedia of genes and genomes. Nucleic. Acids Res. 28 (1), 27–30. doi:10.1093/nar/28.1.27
Kerfeld, C. A., and Scott, K. M. (2011). Using BLAST to teach “E-value-tionary” concepts. PLOS Biol. 9, e1001014. doi:10.1371/journal.pbio.1001014
Lagier, J.-C., Armougom, F., Million, M., Hugon, P., Pagnier, I., Robert, C., et al. (2012). Microbial culturomics: paradigm shift in the human gut microbiome study. Clin. Microbiol. Infect. 18, 1185–1193. doi:10.1111/1469-0691.12023
Laskowski, R. A. (2001). PDBsum: summaries and analyses of PDB structures. Nucleic Acids Res. 29, 221–222. doi:10.1093/nar/29.1.221
Lipinski, C. A. (2000). Drug-like properties and the causes of poor solubility and poor permeability. J. Pharmacol. Toxicol. Methods 44, 235–249. doi:10.1016/s1056-8719(00)00107-6
Lipinski, C. A., Lombardo, F., Dominy, B. W., and Feeney, P. J. (2001). Experimental and computational approaches to estimate solubility and permeability in drug discovery and development settings. Adv. Drug Deliv. Rev. 46, 3–26. doi:10.1016/s0169-409x(00)00129-0
Luo, H., Lin, Y., Gao, F., Zhang, C.-T., and Zhang, R. (2014). DEG 10, an update of the database of essential genes that includes both protein-coding genes and noncoding genomic elements. Nucleic Acids Res. 42, D574–D580. doi:10.1093/nar/gkt1131
Luo, H., Lin, Y., Liu, T., Lai, F.-L., Zhang, C.-T., Gao, F., et al. (2021). DEG 15, an update of the Database of Essential Genes that includes built-in analysis tools. Nucleic Acids Res. 49, D677–D686. doi:10.1093/nar/gkaa917
Lynch, S. V., and Pedersen, O. (2016). The human intestinal microbiome in health and disease. N. Engl. J. Med. 375, 2369–2379. doi:10.1056/NEJMra1600266
Mahram, A., and Herbordt, M. C. (2015). NCBI BLASTP on high-performance reconfigurable computing systems. ACM Trans. Reconfigurable Technol. Syst. (TRETS) 7, 1–20. doi:10.1145/2629691
Ma, Q., Izaguirre, J. A., and Skeel, R. D. (2003). Verlet-I/r-RESPA/Impulse is limited by nonlinear instabilities. SIAM J. Sci. Comput. 24, 1951–1973. doi:10.1137/s1064827501399833
Michaud-Agrawal, N., Denning, E. J., Woolf, T. B., and Beckstein, O. (2011). MDAnalysis: a toolkit for the analysis of molecular dynamics simulations. J. Comput. Chem. 32, 2319–2327. doi:10.1002/jcc.21787
Mishra, A. K., Gimenez, G., Lagier, J.-C., Robert, C., Raoult, D., and Fournier, P.-E. (2012). Genome sequence and description of Alistipes senegalensis sp. nov. Stand. Genomic Sci. 6, 1–16. doi:10.4056/sigs.2625821
Mondal, S. I., Ferdous, S., Jewel, N. A., Akter, A., Mahmud, Z., Islam, M. M., et al. (2015). Identification of potential drug targets by subtractive genome analysis of Escherichia coli O157: H7: an in silico approach. Adv. Appl. Bioinforma. Chem. AABC 8, 49–63. doi:10.2147/AABC.S88522
Moriya, Y., Itoh, M., Okuda, S., Yoshizawa, A. C., and Kanehisa, M. (2007). KAAS: an automatic genome annotation and pathway reconstruction server. Nucleic Acids Res. 35, W182–W185. doi:10.1093/nar/gkm321
Páll, S., Zhmurov, A., Bauer, P., Abraham, M., Lundborg, M., Gray, A., et al. (2020). Heterogeneous parallelization and acceleration of molecular dynamics simulations in GROMACS. J. Chem. Phys. 153, 134110. doi:10.1063/5.0018516
Parker, B. J., Wearsch, P. A., Veloo, A. C., and Rodriguez-Palacios, A. (2020). The genus Alistipes: gut bacteria with emerging implications to inflammation, cancer, and mental health. Front. Immunol. 11, 906. doi:10.3389/fimmu.2020.00906
Pereira, U., Soares, S., Blom, J., Leal, C., Ramos, R., Guimarães, L., et al. (2013). In silico prediction of conserved vaccine targets in Streptococcus agalactiae strains isolated from fish, cattle, and human samples. Genet. Mol. Res. 12, 2902–2912. doi:10.4238/2013.August.12.6
Pethick, F. E., Lainson, A. F., Yaga, R., Flockhart, A., Smith, D. G. E., Donachie, W., et al. (2012a). Complete genome sequence of corynebacterium pseudotuberculosis strain 1/06-A, isolated from a horse in north America. J. Bacteriol. 194, 4476. doi:10.1128/JB.00922-12
Pethick, F. E., Lainson, A. F., Yaga, R., Flockhart, A., Smith, D. G. E., Donachie, W., et al. (2012b). Complete genome sequences of corynebacterium pseudotuberculosis strains 3/99-5 and 42/02-A, isolated from sheep in scotland and Australia, respectively. J. Bacteriol. 194, 4736–4737. doi:10.1128/JB.00918-12
Petra, A. I., Panagiotidou, S., Hatziagelaki, E., Stewart, J. M., Conti, P., and Theoharides, T. C. (2015). Gut-microbiota-brain Axis and its effect on neuropsychiatric disorders with suspected immune dysregulation. Clin. Ther. 37, 984–995. doi:10.1016/j.clinthera.2015.04.002
Pettersen, E. F., Goddard, T. D., Huang, C. C., Couch, G. S., Greenblatt, D. M., Meng, E. C., et al. (2004). UCSF Chimera—a visualization system for exploratory research and analysis. J. Comput. Chem. 25, 1605–1612. doi:10.1002/jcc.20084
Pfleiderer, A., Mishra, A. K., Lagier, J.-C., Robert, C., Caputo, A., Raoult, D., et al. (2014). Non-contiguous finished genome sequence and description of Alistipes ihumii sp. nov. Stand. Genomic Sci. 9, 1221–1235. doi:10.4056/sigs.4698398
Raetz, C. R., and Whitfield, C. (2002). Lipopolysaccharide endotoxins. Annu. Rev. Biochem. 71, 635–700. doi:10.1146/annurev.biochem.71.110601.135414
Rautio, M., Eerola, E., Väisänen-Tunkelrott, M.-L., Molitoris, D., Lawson, P., Collins, M. D., et al. (2003). Reclassification of Bacteroides putredinis (Weinberg et al., 1937) in a new genus Alistipes gen. nov., as Alistipes putredinis comb. nov., and description of Alistipes finegoldii sp. nov., from human sources. Syst. Appl. Microbiol. 26, 182–188. doi:10.1078/072320203322346029
Schmidt, H., Mesters, J. R., Wu, J., Woodard, R. W., Hilgenfeld, R., and Mamat, U. (2011). Evidence for a two-metal-ion mechanism in the cytidyltransferase KdsB, an enzyme involved in lipopolysaccharide biosynthesis. PLoS One 6, e23231. doi:10.1371/journal.pone.0023231
Scott, M. S., Calafell, S. J., Thomas, D. Y., and Hallett, M. T. (2005). Refining protein subcellular localization. PLOS Comput. Biol. 1, e66. doi:10.1371/journal.pcbi.0010066
Shah Hassan, S., Guimarães, L. C., DE Pádua Pereira, U., Islam, A., Ali, A., Bakhtiar, S. M., et al. (2012). Complete genome sequence of Corynebacterium pseudotuberculosis biovar ovis strain P54B96 isolated from antelope in South Africa obtained by rapid next generation sequencing technology. Stand. genomic Sci. 7, 189–199. doi:10.4056/sigs.3066455
Shkoporov, A. N., Chaplin, A. V., Khokhlova, E. V., Shcherbakova, V. A., Motuzova, O. V., Bozhenko, V. K., et al. (2015). Alistipes inops sp. nov. and Coprobacter secundus sp. nov., isolated from human faeces. Int. J. Syst. Evol. Microbiol. 65, 4580–4588. doi:10.1099/ijsem.0.000617
Song, Y., Könönen, E., Rautio, M., Liu, C., Bryk, A., Eerola, E., et al. (2006). Alistipes onderdonkii sp. nov. and Alistipes shahii sp. nov., of human origin. Int. J. Syst. Evol. Microbiol. 56, 1985–1990. doi:10.1099/ijs.0.64318-0
Sterling, T., and Irwin, J. J. (2015). ZINC 15--ligand discovery for everyone. J. Chem. Inf. Model 55, 2324–2337. doi:10.1021/acs.jcim.5b00559
Szklarczyk, D., Gable, A. L., Lyon, D., Junge, A., Wyder, S., Huerta-Cepas, J., et al. (2019). STRING v11: protein–protein association networks with increased coverage, supporting functional discovery in genome-wide experimental datasets. Nucleic Acids Res. 47, D607–D613. doi:10.1093/nar/gky1131
Szklarczyk, D., Gable, A. L., Nastou, K. C., Lyon, D., Kirsch, R., Pyysalo, S., et al. (2021). The STRING database in 2021: customizable protein–protein networks, and functional characterization of user-uploaded gene/measurement sets. Nucleic Acids Res. 49, D605–D612. doi:10.1093/nar/gkaa1074
Vilar, S., Cozza, G., and Moro, S. (2008). Medicinal chemistry and the molecular operating environment (MOE): application of QSAR and molecular docking to drug discovery. Curr. Top. Med. Chem. 8, 1555–1572. doi:10.2174/156802608786786624
Vivarelli, S., Salemi, R., Candido, S., Falzone, L., Santagati, M., Stefani, S., et al. (2019). Gut microbiota and cancer: from pathogenesis to therapy. Cancers 11, 38. doi:10.3390/cancers11010038
Volkamer, A., Kuhn, D., Rippmann, F., and Rarey, M. (2012). DoGSiteScorer: a web server for automatic binding site prediction, analysis and druggability assessment. Bioinformatics 28, 2074–2075. doi:10.1093/bioinformatics/bts310
Wang, X., and Quinn, P. J. (2010). Endotoxins: lipopolysaccharides of gram-negative bacteria. Endotoxins Struct. Funct. Recognit. 53, 3–25. doi:10.1007/978-90-481-9078-2_1
Wattam, A. R., Abraham, D., Dalay, O., Disz, T. L., Driscoll, T., Gabbard, J. L., et al. (2014). PATRIC, the bacterial bioinformatics database and analysis resource. Nucleic Acids Res. 42, D581–D591. doi:10.1093/nar/gkt1099
Wiederstein, M., and Sippl, M. J. (2007). ProSA-web: interactive web service for the recognition of errors in three-dimensional structures of proteins. Nucleic Acids Res. 35, W407–W410. doi:10.1093/nar/gkm290
Wisal, A., Saeed, N., Aurongzeb, M., Shafique, M., Sohail, S., Anwar, W., et al. (2024). Bridging drug discovery through hierarchical subtractive genomics against asd, trpG, and secY of pneumonia causing MDR Staphylococcus aureus. Mol. Genet. Genomics 299, 34. doi:10.1007/s00438-024-02115-8
Yang, H., Lou, C., Sun, L., Li, J., Cai, Y., Wang, Z., et al. (2019). admetSAR 2.0: web-service for prediction and optimization of chemical ADMET properties. Bioinforma. Oxf. Engl. 35, 1067–1069. doi:10.1093/bioinformatics/bty707
Yoshida, N., Yamashita, T., and Hirata, K.-I. (2018). Gut microbiome and cardiovascular diseases. Diseases 6, 56. doi:10.3390/diseases6030056
Yu, C.-S., Cheng, C.-W., Su, W.-C., Chang, K.-C., Huang, S.-W., Hwang, J.-K., et al. (2014). CELLO2GO: a web server for protein subCELlular LOcalization prediction with functional gene ontology annotation. PLOS ONE 9, e99368. doi:10.1371/journal.pone.0099368
Yu, C.-S., Lin, C.-J., and Hwang, J.-K. (2004). Predicting subcellular localization of proteins for Gram-negative bacteria by support vector machines based on n-peptide compositions. Protein Sci. A Publ. Protein Soc. 13, 1402–1406. doi:10.1110/ps.03479604
Yu, N. Y., Wagner, J. R., Laird, M. R., Melli, G., Rey, S., Lo, R., et al. (2010). PSORTb 3.0: improved protein subcellular localization prediction with refined localization subcategories and predictive capabilities for all prokaryotes. Bioinformatics 26, 1608–1615. doi:10.1093/bioinformatics/btq249
Keywords: Alistipes genera, subtractive genomics, druggable targets, TCM/ZINC inhibitors, pharmacokinetics, thermodynamics evaluation
Citation: Sohail S, Wisal A, Rana S, Abid Khan RM, Ullah A, Khan F-A, Irfan M, Imran M, Carvalho PVSD, Rozental R, Shaheen F and Hassan SS (2025) Druggable proteins of Alistipes reveal promising antimicrobial targets against chronic intestinal inflammation. Front. Pharmacol. 16:1555062. doi: 10.3389/fphar.2025.1555062
Received: 12 January 2025; Accepted: 31 March 2025;
Published: 24 April 2025.
Edited by:
M. Victoria Delpino, CONICET Instituto de Investigaciones Biomédicas en Retrovirus y SIDA (INBIRS), ArgentinaReviewed by:
Muhammad Kalim, Houston Methodist Research Institute, United StatesRomen Singh Naorem, Jorhat Medical College and Hospital, India
Liesong Chen, Institute of pathogenic biology, China
Copyright © 2025 Sohail, Wisal, Rana, Abid Khan, Ullah, Khan, Irfan, Imran, Carvalho, Rozental, Shaheen and Hassan. This is an open-access article distributed under the terms of the Creative Commons Attribution License (CC BY). The use, distribution or reproduction in other forums is permitted, provided the original author(s) and the copyright owner(s) are credited and that the original publication in this journal is cited, in accordance with accepted academic practice. No use, distribution or reproduction is permitted which does not comply with these terms.
*Correspondence: Syed Shah Hassan, aGFzc2FuY2hlbWlzdDgzQGdtYWlsLmNvbQ==; Muhammad Imran, aW1yYW5jaGVtaXN0QGdtYWlsLmNvbSYjeDAyMDBhOw==; Paulo V. S. D. Carvalho, cGRhbHRyb25AZ21haWwuY29t; Renato Rozental, cmVuYXRvLnJvemVudGFsQGZpb2NydXouYnI=; Farzana Shaheen, YWZuYW4uaWNjc0BnbWFpbC5jb20=
†ORCID: Syed Shah Hassan, orcid.org/0000-0003-1251-5215
‡These authors have contributed equally to this work