- 1Department of Dermatology, Hair Medical Center of Shanghai Tongji Hospital, Tongji Hospital, School of Medicine, Tongji University, Shanghai, China
- 2Department of Pharmacy, Key Laboratory of Pathogen-Host Interaction, Ministry of Education, Shanghai Tenth People’s Hospital, School of Medicine, Tongji University, Shanghai, China
Introduction: Candidiasis, mainly caused by Candida albicans, poses a serious threat to human health. The escalating drug resistance in C. albicans and the limited antifungal options highlight the critical need for novel therapeutic strategies.
Methods: We evaluated 12 machine learning models on a self-constructed dataset with known anti-C. albicans activity. Based on their performance, the optimal model was selected to screen our separate in-house compound library with unknown anti-C. albicans activity for potential antifungal agents. The anti-C. albicans activity of the selected compounds was confirmed through in vitro drug susceptibility assays, hyphal growth assays, and biofilm formation assays. Through transcriptomics, proteomics, iron rescue experiments, CTC staining, JC-1 staining, DAPI staining, molecular docking, and molecular dynamics simulations, we elucidated the mechanism underlying the anti-C. albicans activity of the compound.
Result: Among the evaluated machine learning models, the best predictive model was an ensemble learning model constructed from Random Forests and Categorical Boosting using soft voting. It predicts that Dp44mT exhibits potent anti-C. albicans activity. The in vitro tests further verified this finding that Dp44mT can inhibit planktonic growth, hyphal formation, and biofilm formation of C. albicans. Mechanistically, Dp44mT exerts antifungal activity by disrupting cellular iron homeostasis, leading to a collapse of mitochondrial membrane potential and ultimately causing apoptosis.
Conclusion: This study presents a practical approach for predicting the antifungal activity of com-pounds using machine learning models and provides new insights into the development of antifungal compounds by disrupting iron homeostasis in C. albicans.
1 Introduction
Candida albicans is a common opportunistic pathogenic fungus responsible for most human candidiasis cases, ranging from mild to potentially deadly invasive candidiasis (Lu et al., 2023a). Risk factors for candidiasis include weakened immune systems from conditions like HIV/AIDS, organ transplants, and chemotherapy, as well as diabetes, pregnancy, prolonged use of medical devices, and other health issues (Lu et al., 2023a). Evidence also implicates C. albicans in tumorigenesis, revealing substantially higher fungal loads in carcinoma samples (42.9% lung, 68.2% mouth malignancies) (Wang X. et al., 2023; Laroumagne et al., 2013). However, the current clinical antifungal compounds are limited in terms of their mechanisms of action and chemical structure classes, mainly consisting of polyenes, azoles, and echinocandins (Xiong et al., 2025). The excessive use of these compounds, along with long-term treatment regimens and environmental exposures, have led to a sharp rise in the development of antifungal drug resistance over the past decade. For instance, surveillance data have indicated a concerning trend of increased azole resistance and tolerance in C. albicans (Nishimoto et al., 2020; Feng et al., 2023; Lu et al., 2021). Polyenes can induce severe side effects owing to the structural resemblance between the target ergosterol and the mammalian membrane sterol cholesterol (Robbins et al., 2017). Despite echinocandins exhibiting potent antifungal activity and an impressive safety record, their clinical utilization is hindered by several factors, including a limited antifungal spectrum, the necessity for intravenous administration, and the high costs associated with the drug (Pappas et al., 2016). Driven by the limited availability and efficacy of current antifungal medications, coupled with the ongoing increase in clinically resistant C. albicans isolates, the need to identify novel agents to expand the antifungal drug repertoire has become increasingly significant.
Machine learning, a rapidly advancing area in artificial intelligence, has profoundly revolutionized the traditionally labor-intensive and time-consuming process of pharmaceutical research and drug discovery. It excels at processing massive datasets to identify patterns and relationships, enabling rapid and accurate predictions or decisions with minimal human intervention by automatically discovering correlations and distinctions among diverse objects (Yang et al., 2023). Additionally, it facilitates exploring a broader chemical space beyond natural products, promising the discovery of novel substances with improved and desired properties. Moreover, an ensemble model constructed from various machine learning algorithms can enhance predictive power and avoid potential biases in the drug discovery (Yu et al., 2022; Lin et al., 2024). Nevertheless, there are still relatively few articles in the development of antifungal agents.
The iron constitutes an indispensable micronutrient in eukaryotic cells, primarily serving as a cofactor for redox enzyme in a multitude of biological processes. These processes encompass heme biosynthesis, iron-sulfur cluster assembly, the citric acid cycle, mitochondrial aerobic respiration, DNA synthesis and repair, chromatin remodeling, as well as ribosome biogenesis (Pijuan et al., 2023). Additionally, iron further regulates the virulence characteristics of C. albicans, encompassing hyphal growth (van Wijlick et al., 2022), biofilm formation (Mochochoko et al., 2021), and adhesion (Puri et al., 2014). Candida albicans mutants deficient in genes related to iron acquisition exhibit avirulence in systemic infections (Xu et al., 2014). In a documented cohort study, approximately 68% of invasive fungal infections were attributed to C. albicans, with a positive correlation observed between these infections and hepatic iron overload (Alexander et al., 2006). Furthermore, elevated iron levels can augment the oral colonization of C. albicans and facilitate its dissemination from the oral cavity to the gut (Tripathi et al., 2022). Multiple compounds have been identified to suppress the growth of C. albicans by disrupting the iron homeostasis, leading to a decrease in symptom severity or enhanced survival rates in animal models of C. albicans infections. These compounds encompass DIBI (Savage et al., 2018), IgY antibodies against Ftr1 (de Souza et al., 2023), attinimicin (Fukuda et al., 2021), deferasirox (Puri et al., 2019). Consequently, strategies targeted at disrupting iron homeostasis can serve as a practical antifungal approach.
In this study, we evaluated 12 machine learning models and determined that an ensemble learning model integrated by Categorical Boosting and Random Forest models was the most accurate in predicting the likelihood of a compound having antifungal activity. We further applied this combined model to predict that Dp44mT exhibits potent anti-C. albicans activity. In vitro drug susceptibility test confirmed this prediction, showing that Dp44mT can inhibit the planktonic growth, hyphal formation, and biofilm formation of C. albicans. Comprehensive investigation combining multi-omics profiling, staining experiments, and computational modeling (molecular docking and dynamics simulations) demonstrated that Dp44mT exerts its antifungal activity by disrupting the iron homeostasis of C. albicans. This research presents a practical approach for predicting the antifungal activity of compounds using machine learning models and offers new insights into the development of antifungal compounds through the disruption of iron ion homeostasis in C. albicans.
2 Materials and methods
2.1 Binary classification dataset construction
A comprehensive compilation of 2,654 compounds, both with and without anti-C. albicans activity, was conducted based on the published literature. This compilation included 1,375 reported anti-C. albicans compounds and 1,188 known non-anti-C. albicans compounds. To address discrepancies in the antifungal activities reported in various studies, we processed the data utilizing the following criteria: 1) Removal of duplicates; 2) Exclusion of compounds without a definitive minimum inhibitory concentration (MIC) value; 3) For compounds with varying MIC values, the smallest value was taken as the MIC value; 4) Conversion of MIC values reported in other units to the corresponding “μM”; 5) Compounds with a MIC value not exceeding the threshold of 100 µM were labeled as “1” (active), and those exceeding this threshold were labeled as “0” (inactive). This classification was used as the response value for modeling. 6) Tanimoto coefficient (TC), a widely used metric based on MACCS fingerprints in cheminformatics, was utilized to conduct a comprehensive similarity analysis of molecular cross-correlations, evaluating the bioassay’s training potential and the molecular diversity in the dataset (Wichka and Lai, 2024). To reduce redundancy, compounds with a TC > 0.85 were removed. The removed compounds were reallocated as a distinct external subset (validation set 2) to gauge model robustness.
To enable machine learning models to interpret and analyze compounds, the structural information of these compounds is transformed into an informative and comprehensible format known as molecular fingerprints (MFs) (Wang R. et al., 2023). These MFs were generated by the PADEL software (version 2.21) (Yap, 2011), configured to detect aromaticity, standardize nitrogen and tautomers, and remove salts, thereby optimizing the accuracy and efficiency of feature generation. In this study, all compounds were represented by four types of MFs: the 166-bit MACCS, the 79-bit Estate, the 307-bit Substructure, and the 4860-bit Klekota-Roth fingerprints. The selection of suitable MFs is crucial in determining the performance and generalizability of ML models. When choosing features, the dataset is refined by excluding less informative MFs. Specifically, we removed MFs with low variance (0.1 or below) and those with a high Pearson correlation coefficient (0.85 or above) to minimize redundancy and conserve computational resources.
The imbalance in classifications between the “active” and “inactive” categories was addressed by implementing the oversampling technique known as Synthetic Minority Oversampling Technique (SMOTE) from the imbalanced-learn library (version 0.12.3) (Gomatam et al., 2024). The preprocessed dataset was randomly divided into a training set and a validation set 1, with an 80:20 ratio, using stratified sampling via the “train_test_split” function from the scikit-learn package (version 1.5.0) (Wu X. et al., 2024). To facilitate dimensionality reduction and visualize the extent of the chemical space, we utilized the t-Distributed Stochastic Neighbor Embedding (t-SNE) through the implementation of scikit-learn package (Plonka et al., 2021).
2.2 Machine learning models building
In this study, a total of 11 distinct learning algorithms were employed as binary classification models (Yu et al., 2023; Li et al., 2023; Idrisoglu et al., 2024). For eight of machine learning models-Decision Tree, Logistic Regression, Naïve Bayes, Random Forest, Adaptive Boosting, K-Nearest Neighbors, Support Vector Machine, and Multilayer Perceptron - the scikit-learn package was employed. Meanwhile, Extreme Gradient Boosting was constructed using the xgboost package (version 2.0.3), Categorical Boosting using the catboost package (version 1.2.5), Light Gradient Boosting Machine using the lightgbm package (version 4.4.0). To address the potential sensitivity of machine learning models to the scale of input features across different datasets, we applied z-score standardization via the “StandardScaler” method provided by scikit-learn. To further enhance model reliability and evaluate its robustness, we identified optimal parameters through hyperparameter tuning by Optuna software (version 3.6.1) (Thirunavukkarasu et al., 2024) and employed 5-fold cross-validation to mitigate biases and variance. For each model, 200 optimization trials were conducted, and the hyperparameter optimization results are summarized in Supplementary Table S1. Subsequently, the optimal parameters were employed to assess the performance of each model. Additionally, to enhance comparability across all models during the analysis, the sizes of the training and test sets, as well as the random states, were kept constant.
2.3 Machine learning models evaluation
In binary classification models, their performance in identifying anti-C. albicans compounds was meticulously assessed based on six evaluation criteria derived from the validation set. These criteria included the confusion matrix, accuracy (ACC), F1 score, recall (RE), the area under the receiver operating characteristic curve (AUC), and Matthews’ correlation coefficient (MCC) (Wichka and Lai, 2024; Jiang et al., 2022).
2.4 Ensemble learning model
After identifying the top two machine learning models based on the number of optimal criteria met, we integrated them to construct an ensemble learning model, aiming to bolster robustness and improve prediction accuracy. We applied the soft voting strategy to develop the proposed ensemble learning model (Yan et al., 2024).
2.5 Applicability domain (AD)
AD establishes a reliable prediction boundary based on structural similarity to training data. Using a Euclidean distance-based K-Nearest Neighbors approach, we set AD threshold to classify compounds as in-domain (distance ≤ threshold) or out-of-domain (distance > threshold) (Duy and Srisongkram, 2025). The AD threshold is mathematically defined by Equation 1:
In the equation, Dk and σ represent the mean and standard deviation of k-NN distances in the training set, respectively. The parameter Z was set to 2 (Baei et al., 2025). Dk denotes the Euclidean distance between a new compound and its nearest training neighbors.
2.6 Anti-C. albicans property verification in silico
The ensemble learning model was trained, tuned, validated and evaluated using the same dataset as the aforementioned individual machine learning models to determine whether it outperformed them. Then the best model was used to screen potentially active compounds from our in-house compound library, an internal database comprising 1,650 compounds with unknown anti-C. albicans activity. These compounds were randomly selected from the ZINC20 database in the simplified molecular input line entry system (SMILES) format (Irwin et al., 2020). The permutation importance method was utilized to elucidate the top 10 features that have the most significant impact on model predictions (Garofalo et al., 2024).
2.7 Strains, culture conditions and medium
Candida albicans SC5314, Cryptococcus neoformans H99, and C. albicans clinical strains were from the fungal collection of our laboratory. Isolates preserved in frozen stocks at −80°C within yeast extract-peptone-dextrose (YPD) medium, composed of 1% yeast extract, 2% peptone, and 2% dextrose, augmented with 40% (v/v) glycerol, were revitalized through two successive cultivations on YPD agar plates incubated at 30°C prior to utilization.
2.8 Antifungal susceptibility assay
The MIC values of compounds against C. albicans were determined following the guidelines set forth in the Clinical and Laboratory Standards Institute document M27-A4 (Li et al., 2024). Briefly, the agents were tested included fluconazole (FLC) and Dp44mT from TargetMol, Boston, United States; Amphotericin B (AMB) from Yuanye Bio-tech, China. These agents were tested at concentrations ranging from 0.0625 μg/mL to 32 μg/mL. The fungal cells, suspended in RPMI 1640 medium (Gibco, United States) with or without Fe3+ at 5 × 103 cells/mL, were then dispensed into triplicate wells of a sterile 96-well microtiter plate. Following a 24-h incubation at 37°C, the optical density was measured at 600 nm using a microplate reader (Thermo Scientific, United States). The MIC was defined as the lowest concentration of the regent that inhibited microbial growth by 50%.
2.9 RNA sequencing
Total RNA was extracted from C. albicans under various conditions using TRIzol reagent (Invitrogen, CA, United States), in accordance with the manufacturer’s instructions. The purity and quantity of the RNA were assessed using the NanoDrop 2000 spectrophotometer (Thermo Scientific, United States), with subsequent assessment of RNA integrity conducted using the Agilent 2,100 Bioanalyzer (Agilent Technologies, Santa Clara, CA, United States). The VAHTS Universal V6 RNA-seq Library Prep Kit was employed for library preparation. The transcriptome sequencing was performed by OE Biotech Co., Ltd. (Shanghai, China), using the Illumina Novaseq 6,000 platform. Raw reads in Fastq format were processed with Fastp (version 0.20.1) to generate clean reads, which were then aligned to the reference genome using HISAT2 (version 2.1.0) (Lu et al., 2023b; Zhen et al., 2024). Differential expression was identified through the DESeq2 package (version 1.22.2). RNA sequencing raw data is available at the following websites: https://www.ncbi.nlm.nih.gov/geo/query/acc.cgi?acc=GSE287552.
2.10 Proteomic analysis
Samples were ground in liquid nitrogen and then mixed with 800 µL of phenol extraction buffer (2.4 g sucrose, 0.058 g NaCl, 0.146 g EDTA·2Na, 0.02 g DTT, 2.5 mL of 0.5 M Tris-HCl [pH 6.8], 2.5 mL of 1.5 M Tris-HCl [pH 8.8], brought to a final volume of 10 mL with ddH2O), phosphatase inhibitors, and PMSF to achieve a final concentration of 1 mM. The grinding was performed using a cryogenic grinder at −35°C, 60 Hz, for 120 s. A pre-chilled 0.1 M ammonium acetate-methanol solution was added, and the mixture was incubated overnight at −40°C. After incubation, pre-chilled methanol was added for washing, and subsequently, acetone was used to fully remove methanol. The pellets were collected and resuspended in sample lysis buffer. After centrifugation at 4°C, 12,000 rpm for 10 min, the supernatant was collected and the protein concentration was measured by bicinchoninic acid assay. Proteins (50 μg) were reduced with DTT to a final concentration of 5 mM at 55°C for 30 min, then alkylated with iodoacetamide (IAA) by adding IAA to a final concentration of 10 mM and incubating at room temperature in the dark for 15 min. Trypsin-TPCK (1 mg/mL) was added for overnight digestion at 37°C. TMT reagent was then added to the samples, mixed thoroughly, and allowed to label at room temperature for 1 h. The tryptic peptides were separated into fractions using high-pH reverse-phase high-performance liquid chromatography (HPLC) with an Agilent 1,100 system. Tryptic peptides were dissolved in 0.1% formic acid (solvent A) and loaded onto an Acclaim PepMap RSLC column (75 μm × 50 cm, RP-C18, Thermo Fisher, United States). The gradient elution started at 2% solvent B, increasing to 28% over 40 min, then to 42% over 20 min, and finally to 90% over 5 min, holding at 90% for 10 min, all at a flow rate of 300 nL/min on an EASY-nLC 1,000 ultraperformance liquid chromatography (UPLC) system. For Mass Spectrometry 1 (MS1), the mass resolution was set to 60,000, the automatic gain control (AGC) target to 1e6, and the maximum injection time to 50 m. MS scans were set for a full scan m/z range of 350–1,500. The resolution was set to 30,000, the AGC target to 1e5, and the maximum injection time to 80 ms for MS/MS, with a dynamic exclusion time of 30 s. The results were analyzed using Proteome Discoverer 2.4.1.15 (Thermo Fisher Scientific, United States) with the sequence database uniprot-candida_albicans-237561-2023.2.2. fasta.
2.11 Gene set enrichment analysis (GSEA) analysis
GSEA assesses the distribution of genes from a predefined set within a ranked list according to their correlation with a phenotype, to determine the significance of the set in contributing to that phenotype (Subramanian et al., 2005). GSEA enrichment analyses were conducted using the “clusterProfiler” packages (version 4.10.1), encompassing all subontologies in the analysis. Gene sets with p-values ≤0.05 were selected for further analysis.
2.12 Growth curve analysis
To investigate the impact of reagent concentration and exposure time on antifungal effects, we conducted a growth curve experiment (Li et al., 2024). The C. albicans suspension was diluted with RPMI 1640 to a mixture of 5 × 105 cells/mL containing specific concentrations of reagents. Subsequently, the samples were incubated at 30°C, and measured every 15 min with a Tecan plate reader (Tecan, Switzerland) at OD600 for 36 h.
2.13 Hyphal formation
A suspension of C. albicans, at a concentration of 5.0 × 105 cells/mL, was prepared in the RPMI1640 medium. These media contained various concentrations of agent, and the suspension was incubated at 37°C for 3 h to evaluate the effect of Dp44mT on hyphal formation. The cell morphology was then photographed using a light microscope, and the lengths of the hyphae were measured using ImageJ software (version 1.54 g) (Fang et al., 2025; Fang et al., 2023).
2.14 Biofilm suppression and eradication assays
The anti-biofilm activity of the reagent was evaluated following a previously described method, with slight modifications (Fang et al., 2023; Zhen et al., 2024). Briefly, C. albicans was adjusted to a concentration of 1.0 × 106 cells/mL to facilitate biofilm formation. To investigate the effect of Dp44mT on both the formation and maintenance of the biofilm, fresh RPMI 1640 medium containing varying concentrations of Dp44mT, both with and without iron supplementation, was introduced after 90 min of adhesion and following 24 h of biofilm formation, respectively. The cultures were then incubated at 37°C for an additional 24 h. The metabolic activity and total biomass of biofilm were respectively assessed using XTT (Yuanye Bio-Tec, China) reduction and crystal violet (CV) (Yuanye Bio-Tec, China) staining assays. After removing the medium, 100 µL of a 9:1 mixture of 0.5 mg/mL XTT and 0.32 mg/mL phenazine methosulfate (Aladdin, China) was added to each well. Then, the OD450 was measured after incubating the plates for 1 h at 37°C in darkness. For CV staining, the biofilm cells were initially fixed with methanol for 15 min, followed by staining with 0.5% CV for 15 min. The biofilms were washed with sterile water, and their morphology was observed and photographed under a light microscope. Subsequently, the absorbance was measured at 590 nm after adding the absolute ethanol to the plates for 30 min to release the dye.
2.15 Scanning electron microscopy
Scanning electron microscopy (SEM) was used to visualize the morphological structure of C. albicans biofilms after treatment with 1 μg/mL Dp44mT for 24 h at 37°C, both in the absence and presence of 5 µM Fe3+. The biofilms were then fixed with 2.5% glutaraldehyde overnight, dehydrated using a series of ethanol concentration gradients (30%, 50%, 70%, 80%, 90%, 95%, and 100% v/v), and dried at 37°C. Subsequently, after being coated with gold–palladium, the samples were observed and imaged by a SEM (Hitachi, S-3400N, Japan) at an accelerating voltage of 12 kV and magnification of ×2000, with a working distance of 8,100 µm (Zhu et al., 2023).
2.16 Laser confocal microscopy assay
Candida albicans cells (1 × 106 cells/mL) in RPMI 1640 medium, with or without 5 µM Fe3+, were treated with various concentrations of Dp44mT at 30°C for 10 h. To assess cell metabolic activity, we utilized 1 mM 5-Cyano-2,3-ditolyl tetrazolium chloride (CTC, Yuanye Bio-Tec, China), a redox dye that, upon reduction, produces a red fluorescent formazan, indicating microbial respiration (Jin et al., 2021). The excitation and emission wavelength were 590 and 612 nm, respectively.
Mitochondrial membrane potential (MMP) was detected with a JC-1 based kit (Beyotime Biotechnology Co., China), following the manufacturer’s instructions (Chen et al., 2023). JC-1 dye was employed to assess MMP collapse, emitting red fluorescence in healthy mitochondria and green fluorescence in those with a lower membrane potential. The ratio of red to green fluorescence served as an indicator of depolarization extent. For green fluorescence, the excitation and emission wavelength were 495 nm and 519 nm, respectively, while for red fluorescence, they were 590 nm and 612 nm.
Nuclear condensation was stained using 50 μg/mL 6-diamidino-2-phenylindole (DAPI, Sangon Biotech, China) in the dark for 20 min (Fang et al., 2025). The excitation and emission wavelength used were 353 nm and 465 nm, respectively. Images of the stained cells were observed and captured using a laser confocal microscopy (Zeiss, German).
2.17 Identifying hub genes
Hub genes were identified using Cytoscape software (version 3.9.1) combined with two machine learning approaches, i.e., SVM-RFE and Logistic Regression (Shannon et al., 2003; Pei et al., 2025). The plugins CytoNCA and CytoHubba in Cytoscape were used with multiple algorithms: cytoNCA plugin employed Betweenness, Closeness, and Degree, while cytoHubba plugin used BottleNeck, Stress, EPC, MCC, MNC, and Radiality. The top five hub genes from each algorithm in Cytoscape and top 20 hub genes from each machine learning method were collected and interacted. The overlapped hub genes were analyzed by Pearson correlation to quantify their relationships with all other genes (Zhang W. et al., 2024).
2.18 Molecular docking
The 3D structural homology models of the protein were generated by SWISS-MODEL according to their amino acid sequences (Waterhouse et al., 2018). The predicted protein structures were refined by GalaxyRefine server via side chain rebuilding, repacking, and overall structure relaxation (Heo et al., 2013). The chemical structures of the targeted compounds were retrieved from PubChem database. Molecular docking is an approach to predict compound-target interactions. It was conducted using CB-Dock2 server to identify the specific residues in the target protein that interact with each ligand. Docking simulation with CB-Dock2 integrates cavity detection, docking and homologous template fitting to enhance protein-ligand blind docking (Liu et al., 2022). Preprocessing, such as adding hydrogen, charge, and side-chain atoms, is automated by the platform. The docking site with the lowest Vina score was chosen as the most favorable binding conformation. A binding affinity score of ≤ −6.58 kcal/mol was defined as tight protein-ligand binding (Zhang C. et al., 2024).
2.19 Molecular dynamics simulations
Following molecular docking, molecular dynamics simulations were performed with Amber24 for 50 ns to elucidate the behavior, conformational stability, and potential practical applications (Case et al., 2023; Posansee et al., 2023). All simulations were conducted using the AMBER force field, specifically the ff19SB force field for the targeted protein and the General Amber Force Field 2 (GAFF2) for the corresponding ligand. The protein-compound complexes were neutralized using Cl− counterions to balance any net charge and solvated with a TIP3P water model. For ensuring the convergence of the simulation, several parallel trajectories were performed for each system. There included root mean square deviation (RMSD), root mean square fluctuation (RMSF), radius of gyrations (RDG), hydrogen bonds and binding energy computed using the CPPTRAJ module in Amber24.
3 Results
3.1 A binary classification machine learning dataset construction
After removing duplicates and compounds without MIC values, 2,564 compounds were selected, with 1,375 compounds active (MIC ≤100 µM) and 1,188 compounds were inactive (MIC >100 µM) against C. albicans. To enhance model generalizability, highly similar compounds (1,656) were removed to form validation set 2 (808 inactive and 848 active compounds) as an external validation set, while the remaining compounds (380 inactive and 528 active compounds) constituted the validation set 1 and training sets (Figure 1A; Supplementary Table S2).
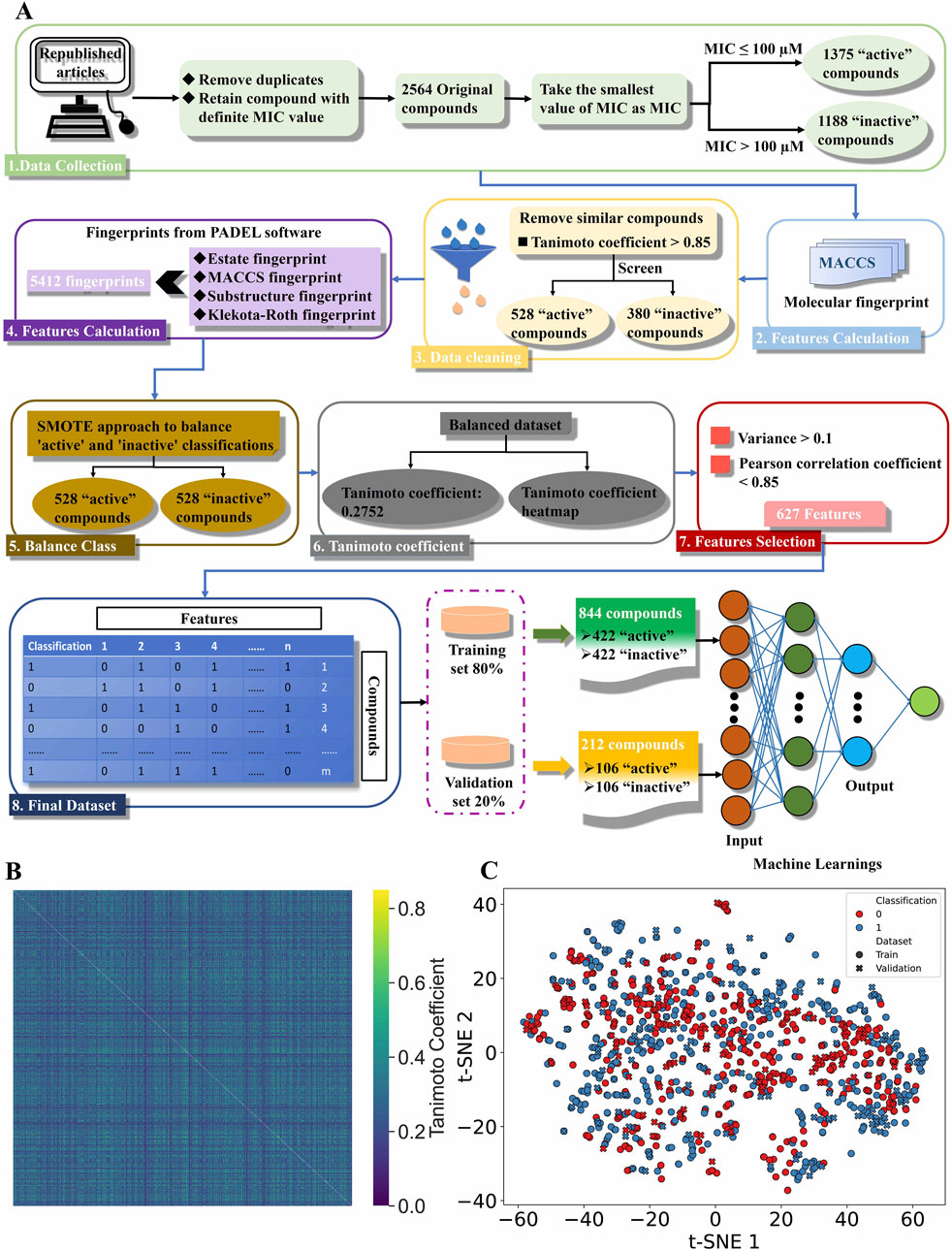
Figure 1. Creating a binary classification machine learning dataset. (A) The workflow for successfully construction of machine learning dataset. (B) The Tanimoto coefficient heatmap. In the heatmap, high similarity between compounds is shown in yellow, and low similarity is shown in blue. (C) Spatial distribution analysis of compounds through t-SNE. The colors represent the classification of the dataset, with red and blue being “0” (inactive) and “1” (active) compounds, respectively. The shapes represent the attributes of the dataset, with circles and crosses being the training and validation sets respectively.
The dataset includes 5,412 features of 4 MFs per compound (Supplementary Table S3). Unbalanced data in binary classification can skew model performance by under-representing the minority class, while balanced classes yield optimal ML results. Our dataset has a 41.9% inactive portion, potentially causing prediction bias. To address this issue, we used SMOTE to oversample the “inactive” class, generating 148 synthetic compounds balance the dataset at 528 compounds per class (Supplementary Table S3). To assess the similarity of compounds in the balanced dataset, we recalculated the TC values. The average TC is 0.2752, much lower than 0.7 (Ji et al., 2022), indicates considerable dissimilarity among the compounds, as evidenced by the predominantly blue heatmap (Figure 1B). Feature selection removes irrelevant or redundant variables to prevent overfitting, enhance statistical significance, improve performance and efficiency, and reduce computational costs. Therefore, we eliminated low-variance and highly correlated fingerprints, leaving 627 features in the dataset (Supplementary Table S4). After feature selection, the balanced dataset is split into training and validation sets (80% training, 20% validation) using stratified sampling. The training set includes 844 compounds (422 active, 422 inactive), and the validation set includes 212 compounds (106 active, 106 inactive).
We used t-SNE to analyze and visualize the chemical space, revealing the dataset’s diversity. Figure 1C shows the dataset’s compounds are widely dispersed, indicating their chemical diversity. Nevertheless, the training set’s chemical space encompasses the validation set’s, ensuring consistent chemical structures and properties. This allows the model to accurately predict properties of unknown compounds, enhancing its reliability and predictive power, and validating stratified sampling as an effective partitioning method.
3.2 The ensemble of categorical boosting and random forest models was the most accurate in predicting the likelihood of a compound having antifungal activity
In this study, we initially trained multiple individual machine learning models, selected the top two based on their anti-C. albicans activity prediction performance, and combined them into an ensemble model to further assess its predictive capability (Figure 2A). The evaluation metrics for the predictive performance of individual models are shown in Figure 2B. Five metrics (ACC, F1, RE, AUC, and MCC) showed that both Categorical Boosting and Random Forest models are the top 2 models among 11 machine learning models.
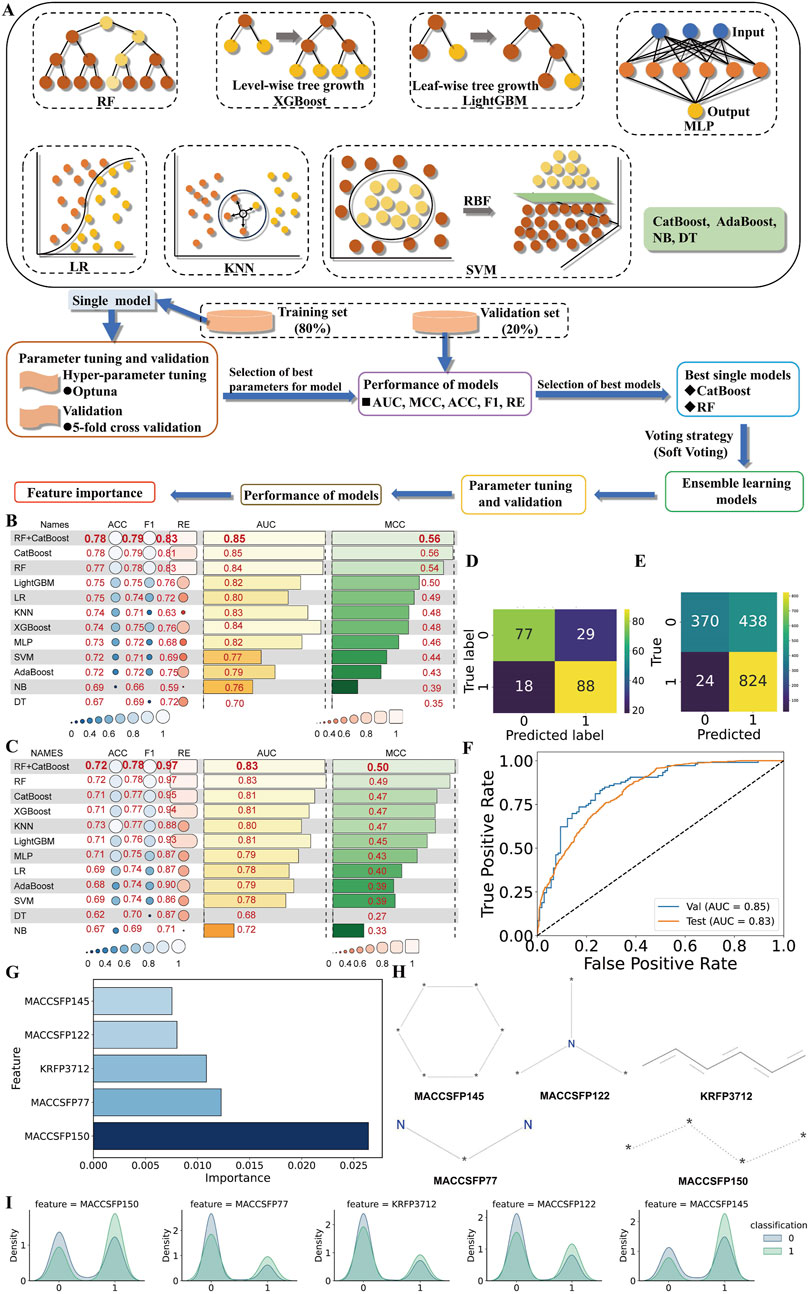
Figure 2. Selection of best machine learning models. (A) Ensemble learning model building process. Random Forest (RF), Categorical Boosting (CatBoost), Light Gradient Boosting (LightGBM), Logistic Regression (LR), K-Nearest Neighbors (KNN), Extreme Gradient Boosting (XGBoost), Multilayer Perceptron (MLP), Support Vector Machine (SVM), Adaptive Boosting (AdaBoost), Naïve Bayes (NB), and Decision Tree (DT). Performance comparison heatmap of various learning models in (B) validation set 1 and (C) validation set 2. ACC, RE, AUC and MCC refer to accuracy, recall, the area under the receiver operating characteristic curve (AUC) and Matthews’ correlation coefficient, respectively. The confusion matrix in (D) validation set 1 and (E) validation set 2, (F) ROC curve, (G) histogram of the top 5 feature importance, and (H) the corresponding structure of features in ensemble learning models. The unknown atom is denoted by an asterisk. (I) Class-specific density distribution of the top five features in the training dataset.
Then the top two algorithms were fused using soft-voting strategy to create an ensemble model with a MCC of 0.56, AUC of 0.85, RE of 0.83, F1 score of 0.79, and ACC of 0.78, surpassing the best individual model by 2% in RE in validation set 1 (Figure 2B; Supplementary Table S5); with a MCC of 0.50, AUC of 0.83, RE of 0.97, F1 score of 0.78, and ACC of 0.72, surpassing the best individual model by 2% in MCC in validation set 2 (Figure 2C; Supplementary Table S6). The confusion matrix and ROC curve in Figures 2D–F support the ensemble model’s effectiveness in classifying C. albicans suppression tasks. Combining models further improves classification and generalization, leading to the conclusion that the ensemble model has superior predictive reliability.
The ensemble model, constrained to a narrow chemical space, requires AD to define its valid scope. We systematically optimized the AD through K-nearest Neighbors analysis (k = 2–10) using Euclidean distance, eliminating out-of-domain samples while retaining in-domain compounds. The σ and Dk are 4.1385. Performance comparisons between AD-only set and original set identified the optimal k-value based on predictive accuracy. In the AD analysis, K = 2 yielded the optimal ensemble model performance, with ACC, F1, RE, AUC and MCC values of 0.84, 0.71, 0.76, 0.88, and 0.60 in AD-only validation set 1 (Supplementary Figure S1A), and 0.75, 0.77, 0.97, 0.85 and 0.58 in AD-only validation set 2 (Supplementary Figure S1B). Notably, AD-only validation set 1 showed improvements of 8% (ACC), 4% (AUC), and 7% (MCC) over the original dataset, while AD-only validation set 2 exhibited gains of 4% (ACC), 2% (AUC), and 16% (MCC). With K set at this value, the original validation set 1 achieved an AD coverage of 37.7% (Supplementary Figure S1C), while the original validation set 2 reached 39.2% (Supplementary Figure S1D). The AD threshold is 16.61 (Supplementary Figures S1C,D). This contrast underscores the need to evaluate predictive accuracy both inside and beyond the AD, ensuring comprehensive reliability assessment. Notably, our model exhibits superior performance on in-domain compounds.
Model interpretation and feature extraction are crucial for understanding predictions and identifying key features. We identified the top5 features through the permutation importance approach for anti-C. albicans compound discovery, are MACCSFP150, MACCSFP77, KRFP3712, MACCSFP122, and MACCSFP145 in descending order of importance (Figures 2G,H). Additionally, Figure 2I illustrates the distribution of the top five features, revealing significant differences between “active” and “inactive” categories, suggesting their potential contribution to anti-C. albicans activity prediction.
3.3 The ensemble of categorical boosting and random forest models predicts that Dp44mT has potent antifungal activity
To obtain novel antimicrobial agents, we virtually screened for targets by prediction using a pretrained ensemble learning model based on an in-house database of 1,650 compounds (Figure 3A). With a low TC (0.2523) and distinct t-SNE clustering patterns, the training set and in-house database exhibit significant structural divergence. This diversity ensures the compounds in the database is appropriate for the model to predict novel anti-C. albicans agents (Supplementary Figures S2A,B). We found that 18 (1%) compounds presented positive results, while the remaining had no anti-C. albicans activity (Supplementary Table S7). Of these “active” compounds, only ZINC000003986690 (Dp44mT), is within the AD (Supplementary Figure S1E). And its anti-C. albicans activity is undetermined. Furthermore, previous studies have shown that it alleviates allergic rhinitis in mice and significantly prolongs the survival of mice with glioma, indicating its favorable safety profile (Kim et al., 2018; Zhou et al., 2020). Therefore, we believe it is of great importance to study the anti-C. albicans property of this compound. For elucidating the mechanism behind the correct predictions, an analysis of feature importance was conducted. This result revealed that the substructures of the Dp44mT corresponding to the aforementioned MFs are Fragment 1 for MACCSFP77, Fragment 2 for MACCSFP122, and Fragment 3 for MACCSFP145 (Figure 3B).
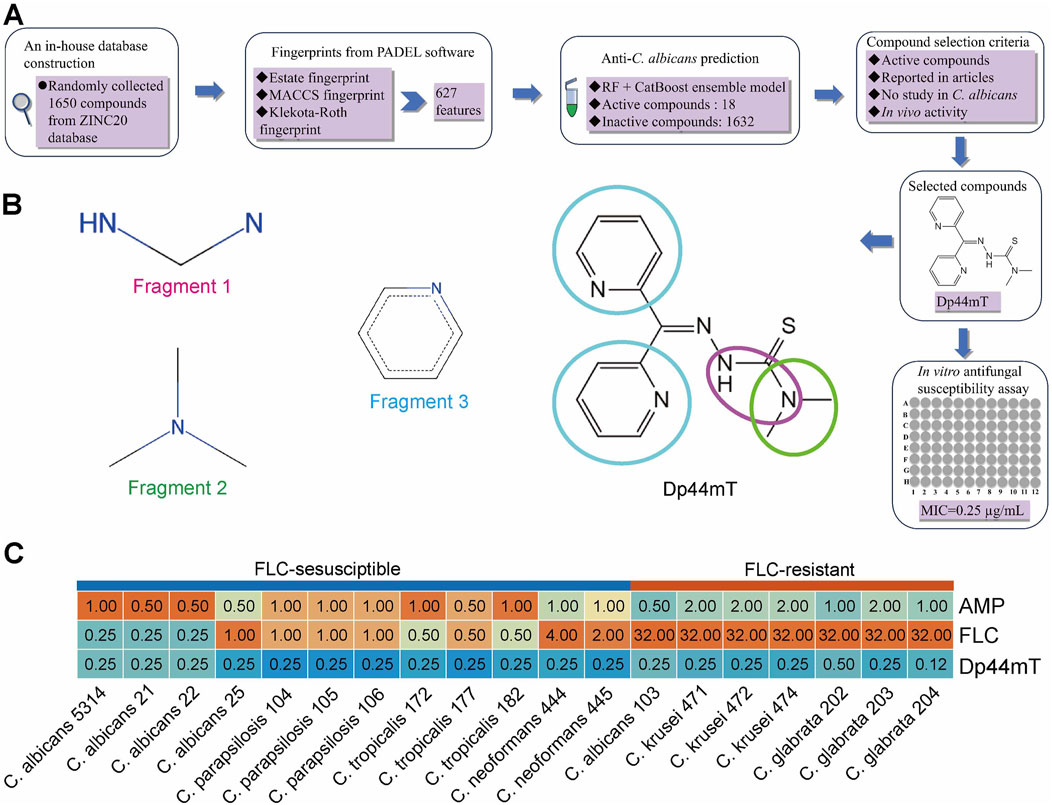
Figure 3. Dp44mT possesses in silico and in vitro anti-C. albicans activity. (A) Procedures to verify that compounds demonstrate anti-C. albicans potency through in silico prediction and in vitro experiments. (B) Substructures that influence the ability of learning model to predict the antifungal activity of Dp44mT. The substructure within the different colored circles on the Dp44mT structure correspond to the fragments with the same color name. (C) Antifungal susceptibility test results of Dp44mT against various Candida species and Cryptococcus Neoformans.
To validate the predictions of the ensemble model, we performed in vitro antifungal susceptibility assays. Indeed, Dp44mT demonstrated the MIC was 0.25 μg/mL against our standard and clinically-derived C. albicans, besides other fungal species (Figure 3C). To delve deeper into whether cross-resistance phenomena occur between FLC and Dp44mT, we evaluated the responsiveness of FLC-resistant strains to the targeted agent. Similar to FLC-sensitive strains, Dp44mT showcased commendable antifungal efficacy, recording MICs within the range of 0.12 μg/mL to 0.5 μg/mL (Figure 3C). Moreover, all strains displayed susceptibility to AMB, with MICs spanning from 0.5 to 2 μg/mL (Figure 3C), a range marginally higher compared to the MICs of Dp44mT observed against these strains. Summarizing the above results, Dp44mT exhibits a robust, broad-spectrum antifungal effect, proving more potent than the established antifungals, FLC and AMP.
3.4 Dp44mT disrupts cellular iron homeostasis destabilization and dysfunctions mitochondria
To elucidate the effects of the Dp44mT on cellular processes, RNA-seq was carried out. The experiment was divided into six groups based on the exposure time and dose: 6 and 10 h of Dp44mT exposure with doses of 0, 0.5 and 2 μg/mL, respectively. For ease of description, the samples with 6 h incubation at reagent doses of 0, 0.5, and 2 μg/mL are labeled A0, A1, and A2, respectively; the 10 h of incubation with the respective doses are labeled B0, B1, and B2. Based on the classical |log2(FoldChange)| > 0.58 thresholds, differentially expressed genes (adjusted p-value <0.05) were identified. Exposure to Dp44mT dramatically altered the gene expression, with significant differences observed in 1,445 genes (721 upregulated, 724 downregulated) in A1 vs. A0, 2,249 genes (1,094 upregulated, 1,155 downregulated) in A2 vs. A0, 1884 genes (954 upregulated, 930 downregulated) in B1 vs. B0, and 2,248 genes (1,124 upregulated, 1,124 downregulated) in B2 vs. B0.
For further insight into the biological signals enriched between the agent-treated and nontreated groups, GSEA, with a pre-sequenced gene list acquired from the transcriptomics, were carried out. While traditional enrichment analysis is limited by its use of predefined gene expression thresholds, GSEA collectively assesses genomes, increasing sensitivity to coordinated expression patterns and providing complementary insights (Iqbal and Munir, 2024). In all groups, in response to the action of the Dp44mT, the upregulated gene set was predominantly intracellular iron homeostasis (Figures 4A–D). The downregulated gene sets were mainly associated with mitochondrial functions, including oxidative phosphorylation, respiratory chain complex I, and ATP synthesis coupled electron transport for group A1, A2, and B2 (Figures 4A,B,D). Unexpectedly, however, the gene sets associated with mitochondrial respiratory function were upregulated in the B1 group (Figure 4C). The differential expressed iron homeostasis-associated genes in transcriptomic between the treated and untreated groups are respectively depicted in Figures 4G,H. Among these genes, six crucial genes including FTR1, CCC2, PGA7, SIT1, SEF1 and HAP43 were upregulated during cells treated with Dp44mT. FTR1, CCC2, the essential genes participating in reductive iron acquisition pathway, PGA7, the essential gene involving in the heme iron absorption pathway, SIT1, the critical gene in siderophore iron uptake pathway, and two regulators, SEF1 and HAP43, of iron homeostasis were upregulated when the iron deficiency was encountered (Chen et al., 2011; Ramanan and Wang, 2000; Heymann et al., 2002; Weissman et al., 2002; Kuznets et al., 2014).
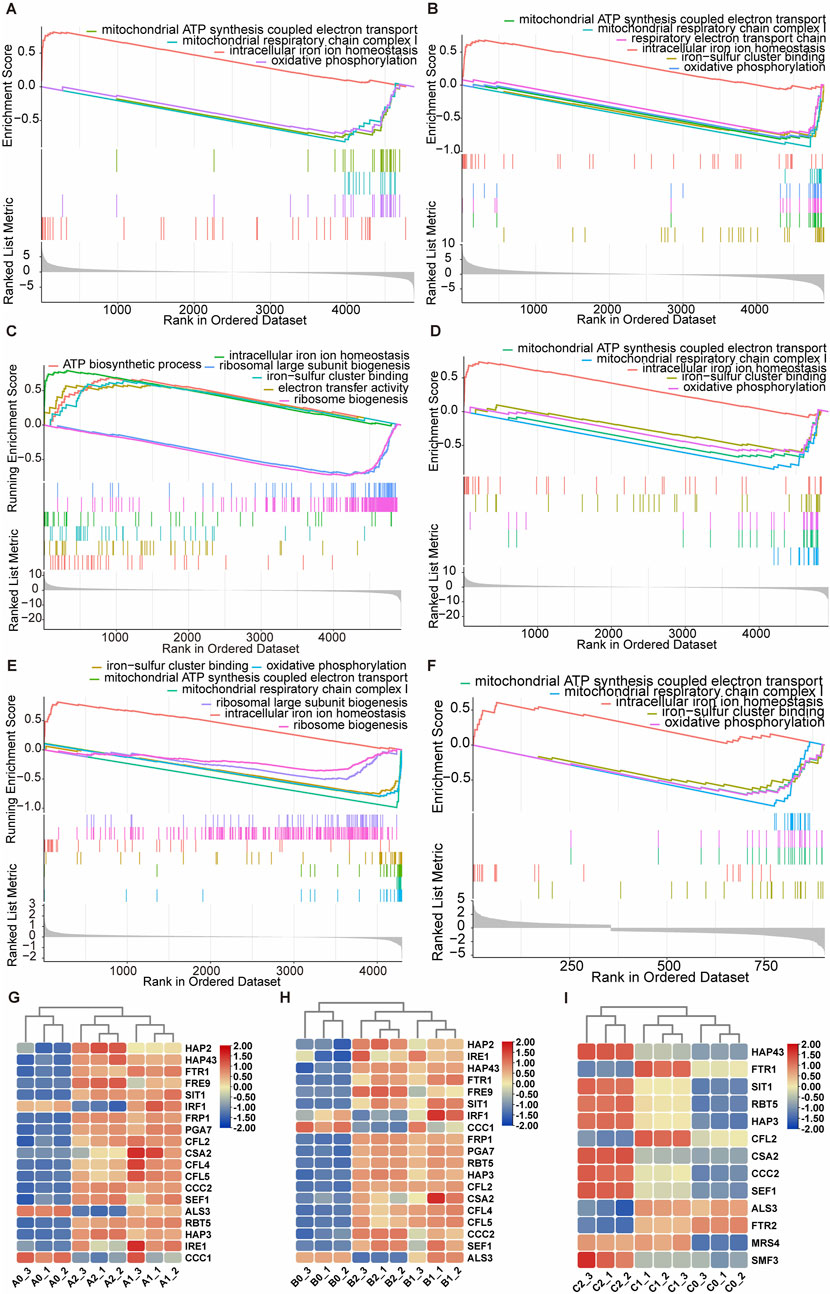
Figure 4. The transcriptomic and proteomic analysis of the anti-C. albicans effects of Dp44mT. The GSEA analysis of transcriptomic group A1 (A), A2 (B), B1 (C), B2 (D) and proteomic group C1 (E), and group C2 (F) after the treatment with Dp44mT. The significant differential expressed iron homeostasis associated-genes in group A (G), group B (H), and -proteins in groups (I).
To ascertain whether the aforementioned phenomenon appeared at the protein level, we conducted the proteomic research. In order to examine how varying concentrations of Dp44mT influence cellular proteomics, we cultured cells for 10 h in media supplemented with 0 μg/mL (C0 group), 0.5 μg/mL (C1 group), and 2 μg/mL (C2 group) of the compound. Based on the |log2 (FoldChange)| > 0.58 thresholds, differentially expressed proteins (p-value <0.05) were identified in the proteomics. There were 161 and 1,086 differentially expressed proteins in C1 vs. C0 (84 upregulated, 77 downregulated) and in C2 vs. C0 (428 upregulated, 658 downregulated), respectively (Supplementary Figures S8, S9). For GSEA analysis, a surprising finding was that, in some cases, the results in group C1 were contrary to those obtained from the corresponding transcriptomic group B1, such as the downregulation of the gene sets associated with mitochondrial ATP synthesis coupled electron transport and oxidative phosphorylation (Figures 4C,E). Nevertheless, the iron homeostasis-associated gene set was also upregulated. The results obtained by GSEA analysis of the group C2 were consistent with those obtained by GSEA analysis of the corresponding transcriptomic group B2 (Figures 4D,F). Regarding the iron-homeostasis associated proteins, Dp44mT treatment increased the expression of these proteins, including Hap43, Sit1, Ccc2, and Sef1. However, the expression of Ftr1 increased under low-dose Dp44mT treatment but was reduced to levels below the control group under high-dose treatment (Figure 4I). Collectively, the above analyses indicated that the cells were in iron starvation after the Dp44mT treatment. In conclusion, Dp44mT primarily upregulated the gene set involved in iron homeostasis, whereas mainly downregulated those associated with mitochondrial respiration, such as the electron transport chain, respiratory chain, oxidative phosphorylation.
3.5 Anti-C. albicans activity of Dp44mT antagonized by Fe3+
To validate the results of the above multi-omics study, we conducted antifungal susceptibility assays in media without or with a concentration gradient of Fe3+, increasing in a 2-fold series. The experimental results showed that, as expected, the MIC increased from 0.25 to 16 μg/mL as the Fe3+ concentration increased from 0 to 40 μM (Figure 5A). Since the MIC remained unchanged when the Fe3+ concentration was between 1.25 μM and 5 μM, we selected 5 μM as the Fe3+ concentration for the subsequent experiments. For investigating the dynamics of C. albicans growth inhibition by Dp44mT, we conducted a 36-h growth curve experiment. Compared to the control group without the reagent, 0.25 μg/mL (1 × MIC) Dp44mT delayed the logarithmic growth phase of C. albicans and suppressed its overall growth (Figure 5B). Candida albicans in the 2 μg/mL Dp44mT (8 × MIC) group exhibited almost no growth, resulting in a nearly horizontal growth curve. In contrast, the growth curve for 2 μg/mL (8 × MIC) FLC was positioned between the curves for 0.5 μg/mL and 2 μg/mL of the Dp44mT, consistent with the previous conclusion that Dp44mT has a superior antimicrobial effect compared to FLC. After the iron addition, the growth curves of C. albicans nearly overlapped with those of the negative control, indicating that the growth was no longer affected by the reagent. This further confirmed that the antifungal effect of Dp44mT is iron-dependent.
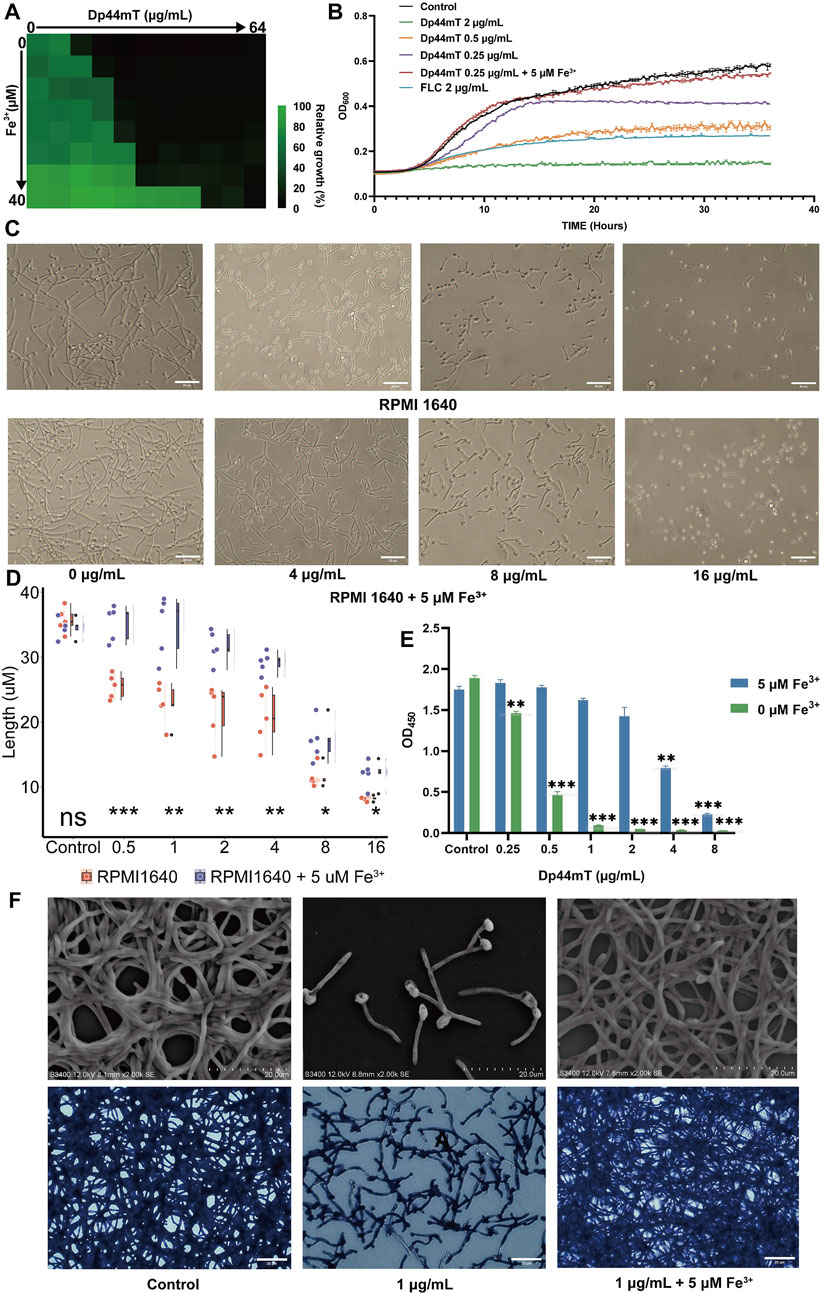
Figure 5. Exogenous addition of Fe3+ antagonizes the anti-C. albicans activity of Dp44mT. (A)The antifungal susceptibility assay for exploring the impact of iron addition on the MIC of the Dp44mT. C. albicans was cultured in media with the reagent and Fe3+ concentrations ranging from 0 to 64 μg/mL and 0–40 μM, respectively. (B) The growth curve analysis of Dp44mT against C. albicans. C. albicans was incubated for 36 h in RPMI 1640 medium with or without 5 µM iron at concentrations of 0, 0.25, 0.5, 2 μg/mL of the Dp44mT, and 2 μg/mL of FLC, respectively. (C) C. albicans was cultivated in iron-free and iron-containing RPMI1640 media with various concentration of Dp44mT for 3 h for hyphal growth. The length changes for hyphae are presented in plot (D). (E) Inhibition of metabolic activity of biofilm by Dp44mT was measured using the XTT reduction assay. (F) The morphological manifestation changing of biofilm in iron-containing or iron-free RPMI1640 media with Dp44mT were observed under the SEM (upper) and the light microscope (down). Statistical significance between the two groups was assessed using a t-test. “*” indicates “p < 0.05”; “**” indicates “0.001 < p ≤ 0.01”; “***” indicates “p ≤ 0.001.”
The major virulence traits exhibited by C. albicans include the capacity to form hyphae and to establish a biofilm. After the treatment with Dp44mT, the majority of hypha-forming genes (HGC1, BRG1, CZF1, HWP1, UME6, and CPH1) were reduced expression and hyphal repressor (HSP90, NRG1) were upregulated (Chen et al., 2020) (Supplementary Figure S3A). Therefore, we hypothesized that Dp44mT were able to inhibit hyphal formation. To test our hypothesis, we conducted hyphal growth experiments. The degree of hyphal inhibition increased with higher reagent concentrations and could be counteracted by iron addition (Figures 5C,D). At 16 μg/mL of the Dp44mT, hyphal formation was almost completely suppressed, although iron addition could still partially reverse the hyphal inhibitory effect (Figure 5D).
The hyphal growth is considered a crucial virulence factor playing an important role in biofilm development and maintenance for C. albicans (Sun et al., 2022). Additionally, iron deficiency prevents C. albicans biofilm formation (Pentland et al., 2021; Mochochoko et al., 2021). We therefore analyzed the anti-biofilm effects of Dp44mT in both iron-containing and iron-free media. The results showed that Dp44mT could inhibit both biofilm formation and disrupt mature biofilms, and this effect could be attenuated or eliminated by the iron supplementation. The XTT reduction assay showed that 0.25 μg/mL of Dp44mt significantly reduced the metabolic activity of the biofilm to 77.5% of the control (p < 0.05). With the iron addition, the reagent concentration had to be increased to 4 μg/mL to significantly reduce the metabolic activity to 45.4% of the control (Figure 5E). CV staining showed that 0.125 μg/mL of Dp44mT significantly reduced the biofilm biomass to 80% of the control (P < 0.05). After the iron supplementation, a significant reduction in biomass to 75% of the control required 1 μg/mL Dp44mT (Supplementary Figure S3B). As seen under the microscope, biofilm formation was largely inhibited with sparse hyphae present in the medium only containing 1 μg/mL Dp44mT (Figure 5F). After the 5 μM Fe3+ addition, the biofilm morphology was essentially the same as that of the controls (Figure 5F). Besides, Dp44mT also can significantly disrupt mature biofilms, and iron supplementation can partially antagonize its effects (Supplementary Figure S3C).
Mitochondria were identified as one of the most affected organelles as determined by the multi-omics. To validate the result, we initially assessed the mitochondrial respiratory activity through the utilization of CTC dye, reduced by respiratory electron transport to CTC formazan producing red fluorescence (Jin et al., 2021). As shown in Figure 6A, iron addition to the 0.5 μg/mL Dp44mT medium restored fluorescence to the control levels, suggesting iron counteracts the Dp44mT’s anti-C. albicans effect. Similarly, iron addition to the 2 μg/mL Dp44mT medium yielded fluorescence intensity akin to the 0.5 μg/mL compound group, indicating a reduction in effective Dp44mT concentration due to iron chelation. The 2 μg/mL Dp44mT group displayed significantly lower fluorescence intensity than the control, confirming strong growth inhibition at high concentrations. In contrast, the 0.5 μg/mL Dp44mT group and the 2 μg/mL Dp44mT group with iron supplementation exhibited higher fluorescence intensity than the control, indicating improved cell viability under low-concentration conditions. Thus, low concentrations of Dp44mT increased the mitochondrial metabolic activity, while high concentrations decreased it, which were in agreement with the results of the GSEA analyses (Figures 4C,D). Afterwards, we assessed changes in MMP using JC-1 staining. The results showed that Dp44mT reduced the fluorescence intensity in a dose-dependent manner, and this effect can be antagonized or partially antagonized by Fe3+ (Supplementary Figure S4). The collapse of MMP in early apoptosis leads to the release of apoptogenic factors, while late-stage apoptosis involves chromatin damage and condensation due to nuclear protein proteolysis (Wang and Youle, 2009; Dobrucki and Darzynkiewicz, 2001). As expected, the results of DAPI staining assay showed that Dp44mT-treated cells did emit significant higher fluorescence compared to the control and iron-added cells (Figure 6B), which demonstrated nuclear morphological alterations subsequent to Dp44mT treatment, with the binding of DAPI to the A:T rich regions of the DNA sequence (Sasidharan et al., 2022). Above all, following the administration of Dp44mT, intracellular iron homeostasis is disrupted, inducing aberrant electron transport chain function and a reduction in MMP, which in turn induces apoptosis.
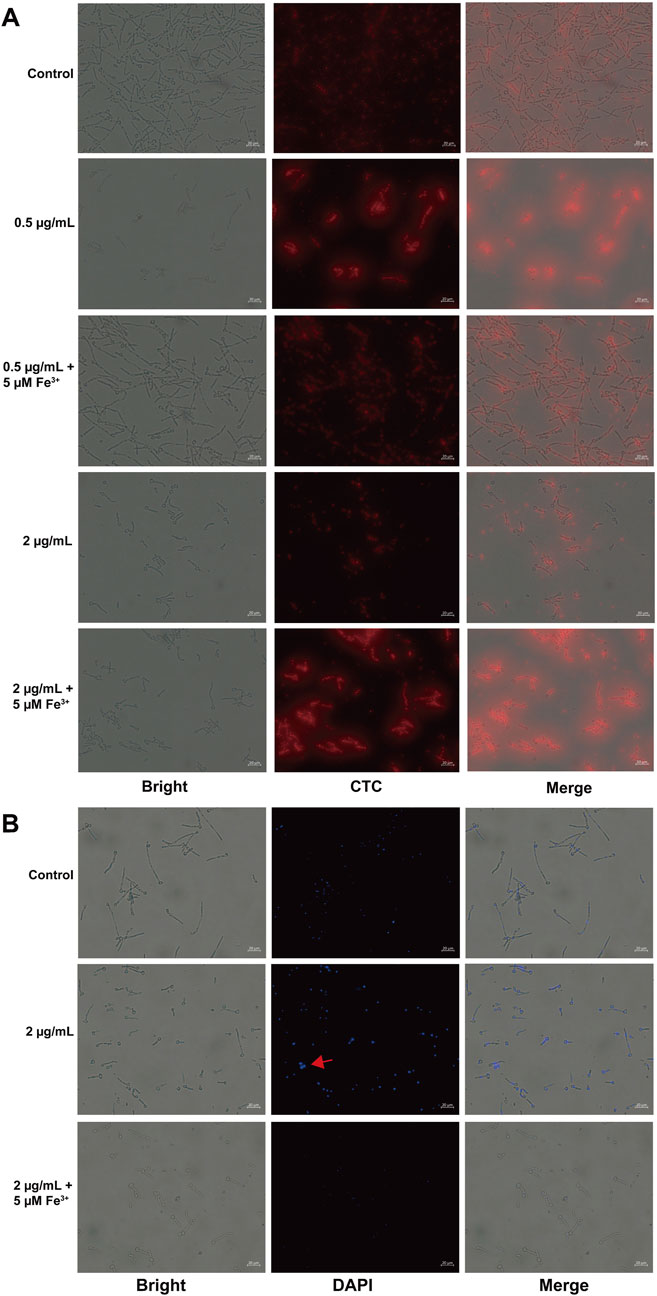
Figure 6. Detection of mitochondrial function and apoptosis. Observation of C. albicans cells stained with (A) CTC for respiratory activity and (B) DAPI for nuclear fragmentation, after the C. albicans cells incubated for 10 h with Dp44mT.
3.6 Ftr1 is the target protein of Dp44mT against C. albicans
For identifying the target gene of Dp44mT, the 35 overlapping differentially expressed genes, obtained from the intersection of proteomics and transcriptomics (Supplementary Figure S5A), were analyzed using multiple algorithms in the CytoHub and CytoNCA plugins. The top five genes from each algorithm were selected, resulting in a total of 8 hub genes, including FTR1, ZRT2, SIT1, CCC2, DDR48, RBT5, PRA1, and CFL2 (Supplementary Figure S5B). Machine learning methods with SVM-RFE and Logistic Regression identified 20 hub genes (Supplementary Figures S5C, D). Intersecting with these hub genes revealed two candidate genes: FTR1 and CFL2 (Figure 7A). To elucidate their relationships, a Pearson correlation analysis was conducted. The result revealed that CFL2 is among the top 20 genes most correlated with FTR1 (Figure 7B). Hierarchical clustering divided these 20 genes into two clusters, with FTR1 and CFL2 in the same cluster (Figure 7C). Its correlation coefficient with FTR1 is 0.88, indicating that FTR1 has a stronger correlation with CFL2 (Figure 7D). Given that FTR1 is essential for adaptation to low iron, it was identified as core gene for further studies (Ramanan and Wang, 2000).
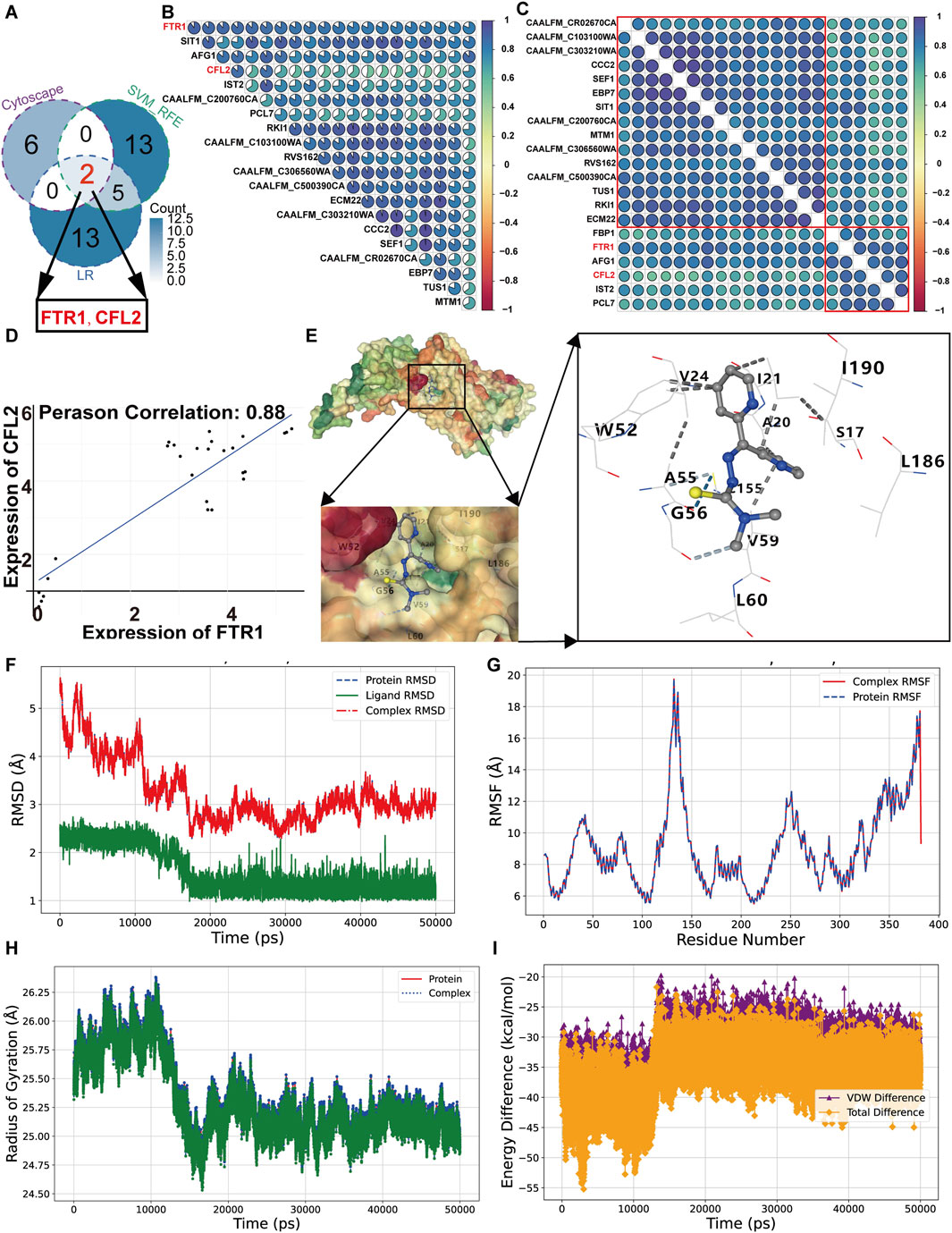
Figure 7. Molecular docking and molecular dynamics of Ftr1 and Dp44mT. (A) A Venn diagram showing the overlap hub genes identified by ML methods and Cytoscape software. Correlation heatmaps of candidate gene correlations without (B) and with (C) hierarchical clustering. (D) Scatter plots representing the correlations of FTR1 with CFL2. (E) A schematic of the Dp44mT docking with the Ftr1. Light blue dashed lines represent weak hydrogen bonds, blue dashed lines denote hydrogen bonds, and gray dashed lines indicate hydrophobic interactions. (F–I) for RMSD, RMSF, RDG and binding energy analysis, respectively. VDW and total represent Van der Waals forces and total energy.
Molecular docking is a valuable technique in the fields of structural molecular biology and computer-aided drug design, facilitating the investigation of primary binding patterns between Ftr1 and Dp44mT and the prediction of their binding affinities. The protein structures were first predicted and refined using the SwissModel and GalaxyRefine platforms, and then docked to the compound to calculate the binding energy and predict affinity. The binding energy of Dp44mT with the Ftr1 was −7.0 kcal/mol, which indicated that they can bind stably (Table 1). In their interaction, the cavity size was 936 Å3, with contact 20 amino acid residues (Table 1; Figure 7E). The Dp44mT-Ftr1 interactions primarily consist of hydrophobic interactions and weak hydrogen bonds. Hydrophobic interactions occur between alkyl groups or between alkyl and Pi groups, while weak hydrogen bonds are formed between carbon donor atoms and acceptors or between Pi groups and donor atoms (Liu et al., 2022). The interactions between Dp44mT and the Ftr1 protein are mainly concentrated on fragment 2 and fragment 3. Weak hydrogen bonds are associated with fragment 2, and hydrophobic interactions are linked to fragment 3, underscoring the critical role of these fragments in the interaction between Dp44mT and Ftr1 (Figure 7E). The MD simulation is indispensable for post-docking characterization of biological compounds, enabling the investigation of time-dependent stability and intrinsic atomic movements (Hossain et al., 2023). Compared to a relatively fragile binding, a more robust binding of the ligand to the active pocket of the protein has a superior pharmacological prospective. The stability and binding affinity can be analyzed include RMSD, RMSF, RDG, hydrogen bonds and binding energy after the 50 ns dynamics trajectory. The RMSD quantifies the degree of conformational variation relative to the initial structure throughout the simulation. For the Ftr1 and Ftr1-Dp44mT complex, the RMSD reached equilibrium with it stabilizing around 3 Å after decreasing rapidly relative to the original structures at the beginning of the simulation due to an initial kinetic adjustment period (Figure 7F). For Dp44mT, the RMSD values gradually decreased and stabilized at approximately 1.5 Å, indicating robust pose stability. The average RMSDs for proteins, ligands, and protein-ligand complex were 3.25 Å, 1.59 Å, and 3.25 Å, respectively. For small protein, an RMSD of 1–4 Å is acceptable (Rudrapal et al., 2024). The RMSF are commonly applied for describing the fluctuation of protein residues during compound binding, relative to a reference position, quantifying their mobility and stability. The proteins and complex exhibited comparable RMSF fluctuations across similar residue ranges (e.g., 130–140), indicating homogeneous flexibility among these residues (Figure 7G). The average RMSF values for both the Ftr1 and the complex were 9.04 Å. The RDG was evaluated as a measure of the compactness and rigidity during the simulation trajectories. The less variation in RDG demonstrate a compact structure and the consistent stability of the system over the course of the simulation. The results revealed that RDG varied stably between 24.53 and 26.38 Å during the entire simulation, suggesting that Dp44mT kept the Ftr1 structure compact, thus stabilizing the inactive condition of Ftr1 and achieving its anti-C. albicans action (Figure 7H). In the binding pocket, the stability of Dp44mT-Ftr1 binding is affected by hydrogen bonds. Dp44mT formed a total of 108 hydrogen bonds with Ftr1, all of which were with TRP52 (Table 2). Analysis of van der Waals interactions and total energy in the complex, ligand and proteins revealed that the differences were −32.58 kcal/mol for van der Waals interactions and −36.11 kcal/mol for total energy, respectively (Figure 7I). Consequently, van der Waals interactions play a major role in the binding energy between proteins and ligands. Consequently, through the analysis of above results suggest that Dp44mT targets Ftr1 and binds tightly to exert anti-C. albicans activity.
4 Discussion
In this study, we successfully applied the ensemble learning model constructed by Categorical Boosting and Random Forest models to identify Dp44mT as an anti-C. albicans agent from an “in-house” compound library. Further in vitro antifungal susceptibility assays confirmed that Dp44mT demonstrated potent anti-C. albicans activity. The multi-omics results confirmed that the observed growth inhibitions by Dp44mT were due to the deprivation of cellular iron. Through network analysis, ML algorithms, Pearson correlation analysis, clustering, molecular docking, and molecular dynamics simulations, we identified the essential iron permease Ftr1 in the reductive iron uptake pathway as the target of Dp44mT (Ramanan and Wang, 2000; Fourie et al., 2018). In summary, Dp44mT reduces the amount of iron in the cell, resulting in iron starvation, mitochondrial electron transport chain dysfunction, MMP collapse and apoptotic cell death (Figure 8). This study presents a practical approach for predicting the antifungal activity of compounds using machine learning models and offers new insights into the development of antifungal compounds through the disruption of iron ion homeostasis in C. albicans.
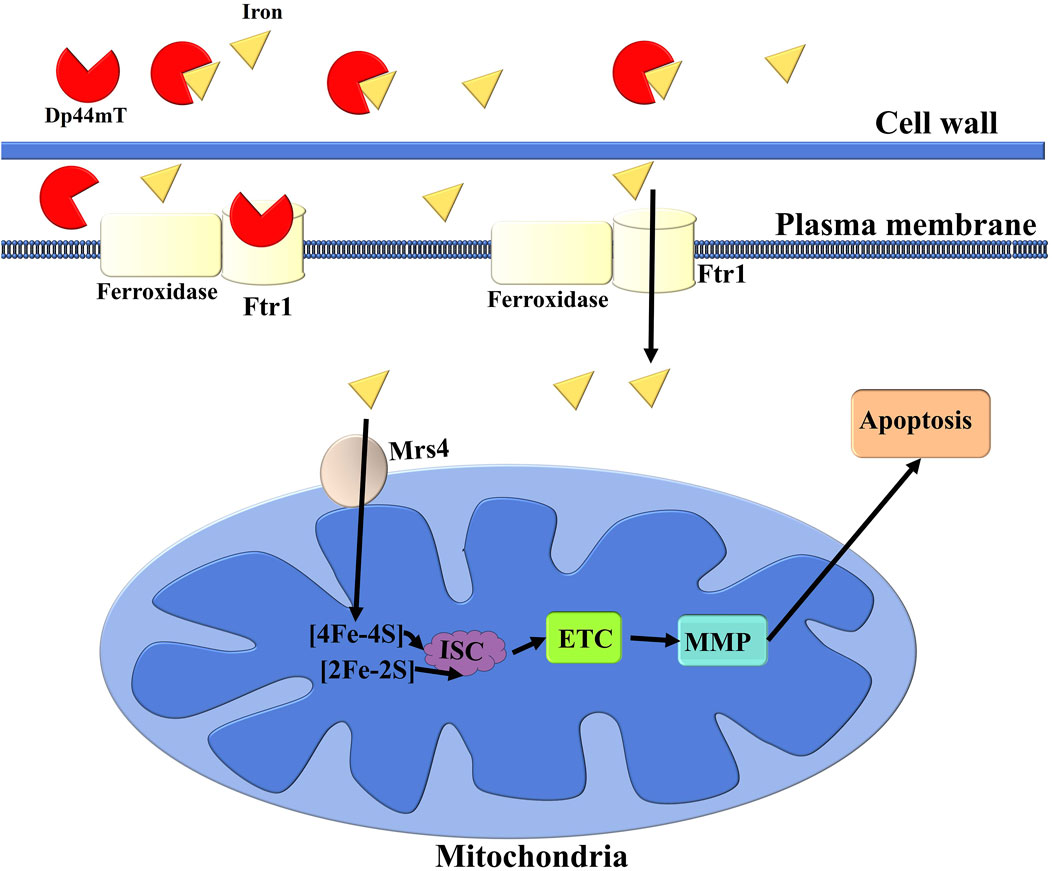
Figure 8. Schematic illustration of the anti-C. albicans mechanism of the Dp44mT. Dp44mT treatment induces cellular iron starvation through its ability to chelate environmental iron and interfere with reductive iron uptake pathways by targeting the essential iron permease Ftr1. This dual action results in mitochondrial electron transport chain (ETC) dysfunction, which subsequently leads to a collapse of the MMP. The disruption of these critical cellular processes ultimately culminates in apoptosis. ISC refers to iron-sulfur cluster.
We compiled 908 unique compounds that exhibited diverse chemical structures for constructing anti-C. albicans activity prediction models, which was crucial for reducing redundancy and enhancing dataset representativeness. Considering the relatively small scale of the dataset and the class imbalance problem, resulting in limited data volume and skewed class distribution, we implemented the SMOTE technique to mitigate these constraints. This innovative approach addresses class imbalance in ML models by generating synthetic minority samples through intelligent interpolation between existing instances and their k-nearest neighbors, rather than random generation. This strategy enhances dataset diversity while preventing simple duplication. When applied to well-curated, representative datasets, the synthesized samples accurately capture the underlying chemical space. The t-SNE analysis demonstrated that the synthesized compounds align with inactive compounds and diverge from active compounds, validating their compatibility with the training set (Supplementary Figure S6). By doing so, it reduces overfitting risks and minimizes bias toward the majority class, improving the model’s capacity to recognize patterns in underrepresented classes (Saha et al., 2024; Talebi Moghaddam et al., 2024). Although beneficial for imbalanced datasets, SMOTE also suffers from some weaknesses: (1) potentially unrealistic synthetic data due to original distribution constraints; (2) overfitting propensity; (3) noise amplification issues; (4) poor scalability to high-dimensional problems; and (5) intensive computational needs for massive data. To address potential drawbacks, we combined SMOTE with high-quality dataset, rigorous feature selection, cross-validation, hyperparameter optimization and robust evaluation metrics to ensure result reliability. After the “active” and “inactive” classes being balanced, we conducted feature selection based on correlation and variance for only a subset of features effectively contributes to capturing the biological characteristics of compounds, reducing dimensionality and noise, improving model interpretability, and enhancing computational efficiency. Currently, pharmaceutical research leverages both traditional machine learning and advanced deep learning techniques While deep learning (e.g., Graph Neural Networks, Transformers) demonstrates impressive capabilities in drug discovery, they demand extensive data, significant computational power, and suffer from poor interpretability for “black box” nature—making them better suited for complex, large-scale problems (Qi et al., 2024; Tseng et al., 2023). Traditional ML, however, proves more effective for our limited dataset, ensuring rapid training, transparent reasoning, and straightforward optimization without sacrificing predictive power. Comparative analyses have revealed that Random Forest and ensemble methods achieved higher prediction accuracy than deep learning approaches, including Graph Neural Networks (Wu J. et al., 2024). Hence, following the partitioning of the constructed dataset into a training set and a validation set, we trained 11 individual machine learning models and selected the two best-performing models, Categorical Boosting and Random Forest, based on evaluation metrics for generating an ensemble model. By comparing the performance of the ensemble model with that of the other 11 individual models, we found that the ensemble model indeed outperformed the individual models: in validation set 1, it outperformed Random Forest, achieving higher accuracy (by 1.3%), F1-score (by 1.3%), AUC (by 1.2%) and MCC (by 3.7%), while matching its recall and surpassing CatBoost by 2% in recall. In validation set 2, it exceeded CatBoost in accuracy (by 1.4%), F1-score (by 1.3%), recall (by 2.1%), AUC (by 2.5%) and MCC (by 6.3%), while also improving MCC (by 2%) over Random Forest (Figures 2B,C). Accuracy reflects correct-to-total prediction ratios. Precision measures positive prediction reliability (critical for reducing false alerts in fraud detection), while recall quantifies true positives identified (avoiding missed frauds). The F1-score balances both, ideal for imbalanced tasks like fraud or rare disease diagnosis (Azim Mim et al., 2024). Therefore, in this study, the adoption of an ensemble model for predicting anti-C. albicans compounds offers several significant advantages over using a single model, which are crucial for advancing our research and its applications. Firstly, the ensemble models integrate predictions from two distinct algorithms, enhancing perspective diversity and mitigating bias or overfitting risks. Secondly, by leveraging the strengths of the two individual classifiers, the enhanced generalization of ensemble learning model enables more accurate, reliable and rapid to identify novel promising antifungal compounds. Consequently, the robust nature of the ensemble classifier allows for consistent performance, rendering it well-suited for high-precision drug discovery. However, CatBoost-Random Forest hybrids have seen little use beyond clinical studies and virus tracking, receiving scant attention in pharmaceutical exploration. For example, a study prospectively developed and validated a CatBoost-Random Forest ensemble, to predict 30-day unplanned readmission in elderly ischemic stroke patients (Hu et al., 2025). The ensemble model was also constructed to classify SARS-CoV-2 sequences, facilitating variant surveillance and enhancing anti-pandemic strategies worldwide (Miao et al., 2022). Thus, this ensemble learning model offers an innovative strategy for discovering anti-C. albicans compounds. Using the ensemble model, we screened our in-house database and identified Dp44mT as the promising anti-C. albicans compound that had a broad-spectrum antifungal activity and exhibited superior antifungal efficacy in subsequent in vitro experiments compared to commonly used clinical antifungals.
In addition, our analysis of molecular structures revealed that compounds containing the fragment identified as significant through feature importance analysis are highly likely to manifest pronounced anti-C. albicans potency. The top 10 features for positive prediction in ensemble model are MACCSFP150, MACCSFP77, KRFP3712, MACCSFP122, and MACCSFP145 (Figures 2G–I). For example, the features, MACCSFP77, MACCSFP122, and MACCSFP145 in Dp44mT are Fragment 1, Fragment 2, and Fragment 3, respectively (Figure 3B). The predominant distribution of these molecular fingerprints in Class 1 implies that their characteristic structural features may be associated with antifungal properties (Figure 2I). For example, these fragments constitute the core scaffold of the anti-C. albicans compound Dp44mT and 19ak (Figure 3B; Supplementary Figure S7). The hydrophobic and weak hydrogen-bonding interactions of the Dp44mT scaffold are essential for protein-ligand recognition (Figure 7E). This finding provides a basis for guiding the development of novel antifungal compounds. In the past, it was a time-consuming, labor-intensive and costly process to discover and validate anti-C. albicans compounds using traditional high-throughput screening. By concentrating on these specific scaffolds, researchers can expedite the discovery process and prioritize compounds with a higher likelihood of success in subsequent testing. Moreover, this insight facilitates researchers in the rational design of novel anti-C. albicans compounds. Therefore, through feature importance, we gain deeper insights into the factors driving the ensemble model’s predictions and identify critical molecular features for anti-C. albicans activity, enhancing our understanding of the model’s decision-making process and guiding the design of new compounds.
Three clinically used antifungal drugs - azoles, polyenes, and echinocandins - exert their antifungal effects through distinct yet singular mechanisms by targeting CYP51, ergosterol synthesis, and (1,3)-β-D-glucan synthase, respectively, making them prone to drug resistance (Zhai et al., 2025). In contrast, our findings show that iron addition mitigates the anti-C. albicans efficacy of Dp44mT. Meanwhile, the strong binding of Dp44mT to Ftr1 suggests it operates through two mechanisms: chelating iron directly from the environment or intracellularly, and targeting Ftr1 to reduce iron uptake, thereby disrupting iron homeostasis and achieving anti-C. albicans effects. Although drug resistance is not directly examined herein, previous studies have shown that C. albicans exposed to another thiosemicarbazone derivative 19ak, with a TC similarity of 0.89 to Dp44mT, maintained susceptibility (Duan et al., 2022). Dp44mT exhibiting diverse antifungal mechanisms are less susceptible to resistance, as their multifaceted action minimizes the ability of C. albicans to adapt and survive. The targeted protein Ftr1 is also a key contributor to C. albicans virulence, as evidenced by the inability of FTR1-deficient strains to inflict epithelial cell damage and their non-pathogenic behavior in a murine candidiasis model (Ramanan and Wang, 2000; Almeida et al., 2008). Beyond its established antifungal activity, Dp44mT also exhibits therapeutic promise for oral tumors (Lee et al., 2016), breast cancer (the leading cancer among women) (Rao et al., 2009; Qiu et al., 2024), and non-small cell lung cancer, the predominant subtype of lung cancer, such as lung adenocarcinoma, in vitro and in vivo (Liu et al., 2024; Lovejoy et al., 2012; Xu and Lu, 2024). Decreased expression of Ftr1 results in greater beta-glucan exposure in C. albicans, boosting the host’s innate immune recognition and phagocytosis of the fungus, alongside anti-tumor activity (Pradhan et al., 2019; Sadeghi et al., 2020; Peymaeei et al., 2020). Additionally, a reduction in iron within fungal cells, achieved through iron chelation or the removal of FTR1, has been demonstrated to enhance the efficacy of antifungal agents, including tunicamycin, zymolyase, FLC, and nystatin (Tripathi et al., 2020; Prasad et al., 2006). Accordingly, Dp44mT may improve the anti-C. albicans property of classic antifungal agents, such as FLC. In conclusion, Dp44mT exhibits multifaceted antifungal mechanisms and pleiotropic properties, effectively lowering the likelihood of drug resistance and concurrently targeting infections and tumors. For patients with dual diagnoses, it offers a streamlined treatment approach, reduces economic burdens, decreases adverse effects, improves adherence, and enhances treatment outcomes, positioning it as a highly promising therapeutic candidate. Therefore, the treatment of mice with C. albicans infection or concurrent tumors using the Dp44mT alone or with common antifungal drugs will be the focus of our future research. Similar to Ftr1, many iron homeostasis-related proteins associated with the survival, virulence and pathogenicity of C. albicans, such as Sef1 (Chen et al., 2011), and Hap43 (Hsu et al., 2011) were upregulated upon Dp44mT treatment. Deficiency in them impairs biofilm formation in C. albicans and reduces its pathogenicity due to its inability to survive in iron-deficient blood (Pentland et al., 2021). During iron scarcity, Sef1 and Hap43 target the promoter regions of FTR1 and ISA1 (involved in Fe-S biogenesis), respectively. Sef1 stimulates FTR1 to increase extracellular iron uptake, whereas Hap43 downregulates ISA1 to conserve iron reserves (Chen et al., 2011). However, when Ftr1 activity is inhibited by Dp44mT, drastic intracellular iron starvation occurs, disturbing Fe-S cluster generation and thereby hindering mitochondrial functionality. Hence, targeting these proteins to discover or synthesize new compounds is a viable strategy, emphasizing iron homeostasis destabilization as a promising approach against C. albicans.
Beyond its effectiveness, compound Dp44mT exhibits a favorable safety profile to be considered a viable option for treating C. albicans infections. Our BLAST analysis of protein sequences confirmed the absence of Ftr1 homologs in humans, suggesting minimal off-target effects in host cells and highlighting its target specificity. During research on Dp44mT’s anti-tumor efficacy in mice, the animals were able to tolerate doses of at least 525 µM (0.75 mg/kg) (Whitnall et al., 2006). And it demonstrated a 50% growth inhibition concentration of more than 10 µM for healthy human mammary epithelial MCF-12A cells and over 25 µM for MRC-5 fibroblasts (Yuan et al., 2004; Rao et al., 2009). They are far exceeding the MIC (0.876 µM, 0.25 μg/mL) needed for its anti-C. albicans activity. Other iron chelators 19ak, deferasirox, hinokitiol, and DIBI and attinimicin, selectively inhibits C. albicans without harming human cells and exhibits in vivo activity (Duan et al., 2022; Ji et al., 2022; Puri et al., 2019; Jin et al., 2021; Savage et al., 2018; Fukuda et al., 2021). In addition, within the host, microbes face an iron-deprived environment. Hosts impose iron limitation (“nutritional immunity”) on microbes via hepcidin-regulated pathways: inhibiting dietary/macrophage iron release and sequestering iron into hemoglobin, transferrin, and ferritin (Roy et al., 2022; Ramirez-Zavala et al., 2022). Consequently, free iron drops to ∼10–18 M—too low for C. albicans to exploit, even in blood. The efficacy of anti-Ftr1 IgY antibodies against C. albicans infection further validates this iron-restricted host environment (de Souza et al., 2023). This iron-depleted environment may enable dose reduction through synergistic effects. Nevertheless, when Dp44mT arrives at the infection locus, its anti-C. albicans activity might be attenuated due to competitive binding with iron from various biological sources. To address current limitations, our research strategy will incorporate structural optimization, synergistic combination therapies, topical administration and targeted-drug delivery systems for assessing its anti-C. albicans efficacy in vivo. Dp44mT could serve as an adjunct to fluconazole to enhance antifungal efficacy. Liposomal encapsulation improves Dp44mT’s site-specific delivery, whereas molecular refinements enhance its iron affinity, collectively mitigating nonspecific chelation and external iron effects. For localized infections, topical formulations (e.g., ointments) may be advantageous due to lower iron levels and controlled drug distribution.
This experiment also has some limitations. Firstly, following 10 h of treatment with low-dose Dp44mT, mitochondrial respiration-related functions were observed to increase at the gene expression level and via the CTC staining assays, but decreased at the protein level. This discrepancy suggests potential post-transcriptional or translational regulation mechanisms that have not yet been fully explored. Prolonged treatment with low-concentration Dp44mT reduces the synthesis of the large ribosomal subunit, as indicated by transcriptomic and proteomic GSEA analyses, leading to fewer ribosomes and decreased protein synthesis (Figures 4C,E). However, the relationship between protein quantity and activity is not linear. The heightened red fluorescence observed after CTC staining could result from: 1) post-translational modifications (e.g., phosphorylation, acetylation) under Dp44mT-induced stress, which may significantly increase protein activity; 2) Dp44mT binding to the limited protein produced, inducing conformational changes that enhance activity; 3) cells may maintain or enhance protein function through cofactors or cooperative proteins; 4) diminished protein degradation enhances the duration of protein activity. In future research, ribosome profiling, mass spectrometry, X-ray crystallography, enzyme activity assays, and ubiquitination detection could be applied to validate the proposed mechanisms of reduced ribosome levels, post-translational modifications, conformational changes, activity compensation, and diminished protein degradation. Secondly, current computational approaches (molecular docking/MD simulations) provide only theoretical interaction models without experimental verification. We will validate these predictions through structural biology techniques including X-ray crystallography and site-directed mutagenesis. Ultimate limitations involve indirect evaluation of cellular iron handling and mitochondrial activity; future studies will employ direct FeRhoNox-1/ICP-MS quantification coupled with seahorse XF analyzer.
In summary, our work identified a novel anti-C. albicans compound Dp44mT representing a major advance in machine learning-based anti-fungal drugs discovery. Dp44mT exerts its anti-C. albicans property by chelating environmental iron and targeting essential proteins Ftr1, for which collectively disrupt intracellular iron homeostasis, collapse MMP, and ultimately induce cell apoptosis. With its favorable safety profile, Dp44mT can be a prospective novel chemical entity for C. albicans infection treatment.
Data availability statement
The datasets presented in this study can be found in online repositories. The names of the repository/repositories and accession number(s) can be found below: https://www.ncbi.nlm.nih.gov/, GSE287552.
Author contributions
XC: Formal Analysis, Investigation, Methodology, Software, Writing – original draft, Writing – review and editing. YJ: Conceptualization, Project administration, Resources, Supervision, Writing – review and editing. HL: Conceptualization, Project administration, Supervision, Writing – original draft, Writing – review and editing. XH: Conceptualization, Funding acquisition, Project administration, Resources, Supervision, Writing – review and editing.
Funding
The author(s) declare that financial support was received for the research and/or publication of this article. This research was funded by National Natural Science Foundation of China, grant number No. 82073452.
Conflict of interest
The authors declare that the research was conducted in the absence of any commercial or financial relationships that could be construed as a potential conflict of interest.
Generative AI statement
The authors declare that no Generative AI was used in the creation of this manuscript.
Publisher’s note
All claims expressed in this article are solely those of the authors and do not necessarily represent those of their affiliated organizations, or those of the publisher, the editors and the reviewers. Any product that may be evaluated in this article, or claim that may be made by its manufacturer, is not guaranteed or endorsed by the publisher.
Supplementary material
The Supplementary Material for this article can be found online at: https://www.frontiersin.org/articles/10.3389/fphar.2025.1574990/full#supplementary-material
References
Alexander, J., Limaye, A. P., Ko, C. W., Bronner, M. P., and Kowdley, K. V. (2006). Association of hepatic iron overload with invasive fungal infection in liver transplant recipients. Liver Transpl. 12, 1799–1804. doi:10.1002/lt.20827
Almeida, R. S., Brunke, S., Albrecht, A., Thewes, S., Laue, M., Edwards, J. E., et al. (2008). The hyphal-associated adhesin and invasin Als3 of Candida albicans mediates iron acquisition from host ferritin. PLoS Pathog. 4, e1000217. doi:10.1371/journal.ppat.1000217
Azim Mim, M., Majadi, N., and Mazumder, P. (2024). A soft voting ensemble learning approach for credit card fraud detection. Heliyon 10, e25466. doi:10.1016/j.heliyon.2024.e25466
Baei, B., Askari, P., Askari, F. S., Kiani, S. J., and Mohebbi, A. (2025). Pharmacophore modeling and QSAR analysis of anti-HBV flavonols. PLoS One 20, e0316765. doi:10.1371/journal.pone.0316765
Case, D. A., Aktulga, H. M., Belfon, K., Cerutti, D. S., Cisneros, G. A., Cruzeiro, V. W. D., et al. (2023). AmberTools. J. Chem. Inf. Model 63, 6183–6191. doi:10.1021/acs.jcim.3c01153
Chen, C., Pande, K., French, S. D., Tuch, B. B., and Noble, S. M. (2011). An iron homeostasis regulatory circuit with reciprocal roles in Candida albicans commensalism and pathogenesis. Cell Host Microbe 10, 118–135. doi:10.1016/j.chom.2011.07.005
Chen, H., Zhou, X., Ren, B., and Cheng, L. (2020). The regulation of hyphae growth in Candida albicans. Virulence 11, 337–348. doi:10.1080/21505594.2020.1748930
Chen, Y., Gao, Y., Yuan, M., Zheng, Z., and Yin, J. (2023). Anti-Candida albicans effects and mechanisms of theasaponin E1 and assamsaponin A. Int. J. Mol. Sci. 24, 9350. doi:10.3390/ijms24119350
de Souza, P. C., Correa, A., Gameiro, J. G., de Oliveira Junior, A. G., Panagio, L. A., Venancio, E. J., et al. (2023). Production of IgY against iron permease Ftr1 from Candida albicans and evaluation of its antifungal activity using Galleria mellonella as a model of systemic infection. Microb. Pathog. 181, 106166. doi:10.1016/j.micpath.2023.106166
Dobrucki, J., and Darzynkiewicz, Z. (2001). Chromatin condensation and sensitivity of DNA in situ to denaturation during cell cycle and apoptosis--a confocal microscopy study. Micron 32, 645–652. doi:10.1016/s0968-4328(00)00069-x
Duan, X., Xie, Z., Ma, L., Jin, X., Zhang, M., Xu, Y., et al. (2022). Selective metal chelation by a thiosemicarbazone derivative interferes with mitochondrial respiration and ribosome biogenesis in Candida albicans. Microbiol. Spectr. 10, e0195121. doi:10.1128/spectrum.01951-21
Duy, H. A., and Srisongkram, T. (2025). Comparative analysis of recurrent neural networks with conjoint fingerprints for skin corrosion prediction. J. Chem. Inf. Model 65, 1305–1317. doi:10.1021/acs.jcim.4c02062
Fang, T., Xiong, J., Huang, X., Fang, X., Shen, X., Jiang, Y., et al. (2025). Extracellular Hsp90 of Candida albicans contributes to the virulence of the pathogen by activating the NF-κB signaling pathway and inducing macrophage pyroptosis. Microbiol. Res. 290, 127964. doi:10.1016/j.micres.2024.127964
Fang, T., Xiong, J., Wang, L., Feng, Z., Hang, S., Yu, J., et al. (2023). Unexpected inhibitory effect of octenidine dihydrochloride on Candida albicans filamentation by impairing ergosterol biosynthesis and disrupting cell membrane integrity. Antibiot. (Basel) 12, 1675. doi:10.3390/antibiotics12121675
Feng, Y., Lu, H., Whiteway, M., and Jiang, Y. (2023). Understanding fluconazole tolerance in Candida albicans: implications for effective treatment of candidiasis and combating invasive fungal infections. J. Glob. Antimicrob. Resist 35, 314–321. doi:10.1016/j.jgar.2023.10.019
Fourie, R., Kuloyo, O. O., Mochochoko, B. M., Albertyn, J., and Pohl, C. H. (2018). Iron at the centre of Candida albicans interactions. Front. Cell Infect. Microbiol. 8, 185. doi:10.3389/fcimb.2018.00185
Fukuda, T. T. H., Helfrich, E. J. N., Mevers, E., Melo, W. G. P., Van Arnam, E. B., Andes, D. R., et al. (2021). Specialized metabolites reveal evolutionary history and geographic dispersion of a multilateral symbiosis. ACS Cent. Sci. 7, 292–299. doi:10.1021/acscentsci.0c00978
Garofalo, S. P., Modugno, A. F., De Carolis, G., Sanitate, N., Negash Tesemma, M., Scarascia-Mugnozza, G., et al. (2024). Explainable artificial intelligence to predict the water status of cotton (gossypium hirsutum L., 1763) from sentinel-2 images in the mediterranean area. Plants (Basel) 13, 3325. doi:10.3390/plants13233325
Gomatam, A., Hirlekar, B. U., Singh, K. D., Murty, U. S., and Dixit, V. A. (2024). Improved QSAR models for PARP-1 inhibition using data balancing, interpretable machine learning, and matched molecular pair analysis. Mol. Divers 28, 2135–2152. doi:10.1007/s11030-024-10809-9
Heo, L., Park, H., and Seok, C. (2013). GalaxyRefine: protein structure refinement driven by side-chain repacking. Nucleic Acids Res. 41, W384–W388. doi:10.1093/nar/gkt458
Heymann, P., Gerads, M., Schaller, M., Dromer, F., Winkelmann, G., and Ernst, J. F. (2002). The siderophore iron transporter of Candida albicans (Sit1p/Arn1p) mediates uptake of ferrichrome-type siderophores and is required for epithelial invasion. Infect. Immun. 70, 5246–5255. doi:10.1128/IAI.70.9.5246-5255.2002
Hossain, A., Rahman, M. E., Rahman, M. S., Nasirujjaman, K., Matin, M. N., Faruqe, M. O., et al. (2023). Identification of medicinal plant-based phytochemicals as a potential inhibitor for SARS-CoV-2 main protease (M(pro)) using molecular docking and deep learning methods. Comput. Biol. Med. 157, 106785. doi:10.1016/j.compbiomed.2023.106785
Hsu, P. C., Yang, C. Y., and Lan, C. Y. (2011). Candida albicans Hap43 is a repressor induced under low-iron conditions and is essential for iron-responsive transcriptional regulation and virulence. Eukaryot. Cell 10, 207–225. doi:10.1128/EC.00158-10
Hu, H., Zhao, Y., Sun, C., Wu, Q., Deng, Y., and Liu, J. (2025). Enhancing readmission prediction model in older stroke patients by integrating insight from readiness for hospital discharge: prospective cohort study. Int. J. Med. Inf. 197, 105845. doi:10.1016/j.ijmedinf.2025.105845
Idrisoglu, A., Dallora, A. L., Cheddad, A., Anderberg, P., Jakobsson, A., and Sanmartin Berglund, J. (2024). COPDVD: automated classification of chronic obstructive pulmonary disease on a new collected and evaluated voice dataset. Artif. Intell. Med. 156, 102953. doi:10.1016/j.artmed.2024.102953
Iqbal, Z., and Munir, M. (2024). Multifaceted natural drought response mechanisms in three elite date palm cultivars uncovered by expressed sequence tags analysis. Sci. Rep. 14, 23186. doi:10.1038/s41598-024-74422-4
Irwin, J. J., Tang, K. G., Young, J., Dandarchuluun, C., Wong, B. R., Khurelbaatar, M., et al. (2020). ZINC20-A free ultralarge-scale chemical database for ligand discovery. J. Chem. Inf. Model 60, 6065–6073. doi:10.1021/acs.jcim.0c00675
Ji, Y., Li, R., Tian, Y., Chen, G., and Yan, A. (2022). Classification models and SAR analysis on thromboxane A(2) synthase inhibitors by machine learning methods. Sar. QSAR Environ. Res. 33, 429–462. doi:10.1080/1062936X.2022.2078880
Jiang, Y., Xiong, W., Jia, L., Xu, L., Cai, Y., Chen, Y., et al. (2022). Developing a Naïve Bayesian Classification Model with PI3Kγ structural features for virtual screening against PI3Kγ: combining molecular docking and pharmacophore based on multiple PI3Kγ conformations. Eur. J. Med. Chem. 244, 114824. doi:10.1016/j.ejmech.2022.114824
Jin, X., Zhang, M., Lu, J., Duan, X., Chen, J., Liu, Y., et al. (2021). Hinokitiol chelates intracellular iron to retard fungal growth by disturbing mitochondrial respiration. J. Adv. Res. 34, 65–77. doi:10.1016/j.jare.2021.06.016
Kim, H. Y., Han, N. R., Kim, H. M., and Jeong, H. J. (2018). The iron chelator and anticancer agent Dp44mT relieves allergic inflammation in mice with allergic rhinitis. Inflammation 41, 1744–1754. doi:10.1007/s10753-018-0817-4
Kuznets, G., Vigonsky, E., Weissman, Z., Lalli, D., Gildor, T., Kauffman, S. J., et al. (2014). A relay network of extracellular heme-binding proteins drives C. albicans iron acquisition from hemoglobin. PLoS Pathog. 10, e1004407. doi:10.1371/journal.ppat.1004407
Laroumagne, S., Lepage, B., Hermant, C., Plat, G., Phelippeau, M., Bigay-Game, L., et al. (2013). Bronchial colonisation in patients with lung cancer: a prospective study. Eur. Respir. J. 42, 220–229. doi:10.1183/09031936.00062212
Lee, J. C., Chiang, K. C., Feng, T. H., Chen, Y. J., Chuang, S. T., Tsui, K. H., et al. (2016). The iron chelator, Dp44mT, effectively inhibits human oral squamous cell carcinoma cell growth in vitro and in vivo. Int. J. Mol. Sci. 17, 1435. doi:10.3390/ijms17091435
Li, R., Tian, Y., Yang, Z., Ji, Y., Ding, J., and Yan, A. (2023). Classification models and SAR analysis on HDAC1 inhibitors using machine learning methods. Mol. Divers 27, 1037–1051. doi:10.1007/s11030-022-10466-w
Li, W., Feng, Y., Feng, Z., Wang, L., Whiteway, M., Lu, H., et al. (2024). Pitavastatin calcium confers fungicidal properties to fluconazole by inhibiting ubiquinone biosynthesis and generating reactive oxygen species. Antioxidants (Basel) 13, 667. doi:10.3390/antiox13060667
Lin, T. E., Yen, D., HuangFu, W. C., Wu, Y. W., Hsu, J. Y., Yen, S. C., et al. (2024). An ensemble machine learning model generates a focused screening library for the identification of CDK8 inhibitors. Protein Sci. 33, e5007. doi:10.1002/pro.5007
Liu, X., Xi, X., Xu, S., Chu, H., Hu, P., Li, D., et al. (2024). Targeting T cell exhaustion: emerging strategies in non-small cell lung cancer. Front. Immunol. 15, 1507501. doi:10.3389/fimmu.2024.1507501
Liu, Y., Yang, X., Gan, J., Chen, S., Xiao, Z. X., and Cao, Y. (2022). CB-Dock2: improved protein-ligand blind docking by integrating cavity detection, docking and homologous template fitting. Nucleic Acids Res. 50, W159–W164. doi:10.1093/nar/gkac394
Lovejoy, D. B., Sharp, D. M., Seebacher, N., Obeidy, P., Prichard, T., Stefani, C., et al. (2012). Novel second-generation di-2-pyridylketone thiosemicarbazones show synergism with standard chemotherapeutics and demonstrate potent activity against lung cancer xenografts after oral and intravenous administration in vivo. J. Med. Chem. 55, 7230–7244. doi:10.1021/jm300768u
Lu, H., Hong, T., Jiang, Y., Whiteway, M., and Zhang, S. (2023a). Candidiasis: from cutaneous to systemic, new perspectives of potential targets and therapeutic strategies. Adv. Drug Deliv. Rev. 199, 114960. doi:10.1016/j.addr.2023.114960
Lu, H., Li, W., Whiteway, M., Wang, H., Zhu, S., Ji, Z., et al. (2023b). A small molecule inhibitor of Erg251 makes fluconazole fungicidal by inhibiting the synthesis of the 14α-methylsterols. mBio 14, e0263922. doi:10.1128/mbio.02639-22
Lu, H., Shrivastava, M., Whiteway, M., and Jiang, Y. (2021). Candida albicans targets that potentially synergize with fluconazole. Crit. Rev. Microbiol. 47, 323–337. doi:10.1080/1040841X.2021.1884641
Miao, M., De Clercq, E., and Li, G. (2022). Towards efficient and accurate SARS-CoV-2 genome sequence typing based on supervised learning approaches. Microorganisms 10, 1785. doi:10.3390/microorganisms10091785
Mochochoko, B. M., Ezeokoli, O. T., Sebolai, O., Albertyn, J., and Pohl, C. H. (2021). Role of the high-affinity reductive iron acquisition pathway of Candida albicans in prostaglandin E2 production, virulence, and interaction with Pseudomonas aeruginosa. Med. Mycol. 59, 869–881. doi:10.1093/mmy/myab015
Nishimoto, A. T., Sharma, C., and Rogers, P. D. (2020). Molecular and genetic basis of azole antifungal resistance in the opportunistic pathogenic fungus Candida albicans. J. Antimicrob. Chemother. 75, 257–270. doi:10.1093/jac/dkz400
Pappas, P. G., Kauffman, C. A., Andes, D. R., Clancy, C. J., Marr, K. A., Ostrosky-Zeichner, L., et al. (2016). Clinical practice guideline for the management of candidiasis: 2016 update by the infectious diseases society of America. Clin. Infect. Dis. 62, e1–e50. doi:10.1093/cid/civ933
Pei, S., Liu, J., Wang, Z., Fan, Y., Meng, S., Huang, X., et al. (2025). Genetic analysis of diagnostic and therapeutic potential for ferroptosis in postoperative sepsis. Int. Immunopharmacol. 147, 114042. doi:10.1016/j.intimp.2025.114042
Pentland, D. R., Davis, J., Muhlschlegel, F. A., and Gourlay, C. W. (2021). CO(2) enhances the formation, nutrient scavenging and drug resistance properties of C. albicans biofilms. NPJ Biofilms Microbiomes 7, 67. doi:10.1038/s41522-021-00238-z
Peymaeei, F., Sadeghi, F., Safari, E., Khorrami, S., Falahati, M., Roudbar Mohammadi, S., et al. (2020). Candida albicans beta-glucan induce anti- cancer activity of mesenchymal stem cells against lung cancer cell line: an in-vitro experimental study. Asian Pac J. Cancer Prev. 21, 837–843. doi:10.31557/APJCP.2020.21.3.837
Pijuan, J., Moreno, D. F., Yahya, G., Moisa, M., Ul Haq, I., Krukiewicz, K., et al. (2023). Regulatory and pathogenic mechanisms in response to iron deficiency and excess in fungi. Microb. Biotechnol. 16, 2053–2071. doi:10.1111/1751-7915.14346
Plonka, W., Stork, C., Sicho, M., and Kirchmair, J. (2021). CYPlebrity: machine learning models for the prediction of inhibitors of cytochrome P450 enzymes. Bioorg Med. Chem. 46, 116388. doi:10.1016/j.bmc.2021.116388
Posansee, K., Liangruksa, M., Termsaithong, T., Saparpakorn, P., Hannongbua, S., Laomettachit, T., et al. (2023). Combined deep learning and molecular modeling techniques on the virtual screening of new mTOR inhibitors from the Thai mushroom database. ACS Omega 8, 38373–38385. doi:10.1021/acsomega.3c04827
Pradhan, A., Avelar, G. M., Bain, J. M., Childers, D., Pelletier, C., Larcombe, D. E., et al. (2019). Non-canonical signalling mediates changes in fungal cell wall PAMPs that drive immune evasion. Nat. Commun. 10, 5315. doi:10.1038/s41467-019-13298-9
Prasad, T., Chandra, A., Mukhopadhyay, C. K., and Prasad, R. (2006). Unexpected link between iron and drug resistance of Candida spp.: iron depletion enhances membrane fluidity and drug diffusion, leading to drug-susceptible cells. Antimicrob. Agents Chemother. 50, 3597–3606. doi:10.1128/AAC.00653-06
Puri, S., Kumar, R., Rojas, I. G., Salvatori, O., and Edgerton, M. (2019). Iron chelator deferasirox reduces Candida albicans invasion of oral epithelial cells and infection levels in murine oropharyngeal candidiasis. Antimicrob. Agents Chemother. 63, e02152. doi:10.1128/AAC.02152-18
Puri, S., Lai, W. K., Rizzo, J. M., Buck, M. J., and Edgerton, M. (2014). Iron-responsive chromatin remodelling and MAPK signalling enhance adhesion in Candida albicans. Mol. Microbiol. 93, 291–305. doi:10.1111/mmi.12659
Qi, X., Zhao, Y., Qi, Z., Hou, S., and Chen, J. (2024). Machine learning empowering drug discovery: applications, opportunities and challenges. Molecules 29, 903. doi:10.3390/molecules29040903
Qiu, C., Wang, W., Xu, S., Li, Y., Zhu, J., Zhang, Y., et al. (2024). Construction and validation of a hypoxia-related gene signature to predict the prognosis of breast cancer. BMC Cancer 24, 402. doi:10.1186/s12885-024-12182-0
Ramanan, N., and Wang, Y. (2000). A high-affinity iron permease essential for Candida albicans virulence. Science 288, 1062–1064. doi:10.1126/science.288.5468.1062
Ramirez-Zavala, B., Kruger, I., Dunker, C., Jacobsen, I. D., and Morschhauser, J. (2022). The protein kinase Ire1 has a Hac1-independent essential role in iron uptake and virulence of Candida albicans. PLoS Pathog. 18, e1010283. doi:10.1371/journal.ppat.1010283
Rao, V. A., Klein, S. R., Agama, K. K., Toyoda, E., Adachi, N., Pommier, Y., et al. (2009). The iron chelator Dp44mT causes DNA damage and selective inhibition of topoisomerase IIalpha in breast cancer cells. Cancer Res. 69, 948–957. doi:10.1158/0008-5472.CAN-08-1437
Robbins, N., Caplan, T., and Cowen, L. E. (2017). Molecular evolution of antifungal drug resistance. Annu. Rev. Microbiol. 71, 753–775. doi:10.1146/annurev-micro-030117-020345
Roy, U., Yaish, S., Weissman, Z., Pinsky, M., Dey, S., Horev, G., et al. (2022). Ferric reductase-related proteins mediate fungal heme acquisition. Elife 11, e80604. doi:10.7554/eLife.80604
Rudrapal, M., Kirboga, K. K., Abdalla, M., and Maji, S. (2024). Explainable artificial intelligence-assisted virtual screening and bioinformatics approaches for effective bioactivity prediction of phenolic cyclooxygenase-2 (COX-2) inhibitors using PubChem molecular fingerprints. Mol. Divers 28, 2099–2118. doi:10.1007/s11030-023-10782-9
Sadeghi, F., Peymaeei, F., Falahati, M., Safari, E., Farahyar, S., Roudbar Mohammadi, S., et al. (2020). The effect of Candida cell wall beta-glucan on treatment-resistant LL/2 cancer cell line: in vitro evaluation. Mol. Biol. Rep. 47, 3653–3661. doi:10.1007/s11033-020-05459-7
Saha, D. K., Hossain, T., Safran, M., Alfarhood, S., Mridha, M. F., and Che, D. (2024). Ensemble of hybrid model based technique for early detecting of depression based on SVM and neural networks. Sci. Rep. 14, 25470. doi:10.1038/s41598-024-77193-0
Sasidharan, S., Nishanth, K. S., and Nair, H. J. (2022). Ethanolic extract of Caesalpinia bonduc seeds triggers yeast metacaspase-dependent apoptotic pathway mediated by mitochondrial dysfunction through enhanced production of calcium and reactive oxygen species (ROS) in Candida albicans. Front. Cell Infect. Microbiol. 12, 970688. doi:10.3389/fcimb.2022.970688
Savage, K. A., Parquet, M. C., Allan, D. S., Davidson, R. J., Holbein, B. E., Lilly, E. A., et al. (2018). Iron restriction to clinical isolates of Candida albicans by the novel chelator DIBI inhibits growth and increases sensitivity to azoles in vitro and in vivo in a murine model of experimental vaginitis. Antimicrob. Agents Chemother. 62, e02576. doi:10.1128/AAC.02576-17
Shannon, P., Markiel, A., Ozier, O., Baliga, N. S., Wang, J. T., Ramage, D., et al. (2003). Cytoscape: a software environment for integrated models of biomolecular interaction networks. Genome Res. 13, 2498–2504. doi:10.1101/gr.1239303
Subramanian, A., Tamayo, P., Mootha, V. K., Mukherjee, S., Ebert, B. L., Gillette, M. A., et al. (2005). Gene set enrichment analysis: a knowledge-based approach for interpreting genome-wide expression profiles. Proc. Natl. Acad. Sci. U. S. A. 102, 15545–15550. doi:10.1073/pnas.0506580102
Sun, C., Zhao, X., Jiao, Z., Peng, J., Zhou, L., Yang, L., et al. (2022). The antimicrobial peptide AMP-17 derived from Musca domestica inhibits biofilm formation and eradicates mature biofilm in Candida albicans. Antibiot. (Basel) 11, 1474. doi:10.3390/antibiotics11111474
Talebi Moghaddam, M., Jahani, Y., Arefzadeh, Z., Dehghan, A., Khaleghi, M., Sharafi, M., et al. (2024). Predicting diabetes in adults: identifying important features in unbalanced data over a 5-year cohort study using machine learning algorithm. BMC Med. Res. Methodol. 24, 220. doi:10.1186/s12874-024-02341-z
Thirunavukkarasu, M. K., Veerappapillai, S., and Karuppasamy, R. (2024). Sequential virtual screening collaborated with machine-learning strategies for the discovery of precise medicine against non-small cell lung cancer. J. Biomol. Struct. Dyn. 42, 615–628. doi:10.1080/07391102.2023.2194994
Tripathi, A., Liverani, E., Tsygankov, A. Y., and Puri, S. (2020). Iron alters the cell wall composition and intracellular lactate to affect Candida albicans susceptibility to antifungals and host immune response. J. Biol. Chem. 295, 10032–10044. doi:10.1074/jbc.RA120.013413
Tripathi, A., Nahar, A., Sharma, R., Kanaskie, T., Al-Hebshi, N., and Puri, S. (2022). High iron-mediated increased oral fungal burden, oral-to-gut transmission, and changes to pathogenicity of Candida albicans in oropharyngeal candidiasis. J. Oral Microbiol. 14, 2044110. doi:10.1080/20002297.2022.2044110
Tseng, Y. J., Chuang, P. J., and Appell, M. (2023). When machine learning and deep learning come to the big data in food chemistry. ACS Omega 8, 15854–15864. doi:10.1021/acsomega.2c07722
van Wijlick, L., Znaidi, S., Hernandez-Cervantes, A., Basso, V., Bachellier-Bassi, S., and d'Enfert, C. (2022). Functional portrait of Irf1 (Orf19.217), a regulator of morphogenesis and iron homeostasis in Candida albicans. Front. Cell Infect. Microbiol. 12, 960884. doi:10.3389/fcimb.2022.960884
Wang, C., and Youle, R. J. (2009). The role of mitochondria in apoptosis. Annu. Rev. Genet. 43, 95–118. doi:10.1146/annurev-genet-102108-134850
Wang, R., Liu, Z., Gong, J., Zhou, Q., Guan, X., and Ge, G. (2023a). An uncertainty-guided deep learning method facilitates rapid screening of CYP3A4 inhibitors. J. Chem. Inf. Model 63, 7699–7710. doi:10.1021/acs.jcim.3c01241
Wang, X., Zhang, W., Wu, W., Wu, S., Young, A., and Yan, Z. (2023b). Is Candida albicans a contributor to cancer? A critical review based on the current evidence. Microbiol. Res. 272, 127370. doi:10.1016/j.micres.2023.127370
Waterhouse, A., Bertoni, M., Bienert, S., Studer, G., Tauriello, G., Gumienny, R., et al. (2018). SWISS-MODEL: homology modelling of protein structures and complexes. Nucleic Acids Res. 46, W296–W303. doi:10.1093/nar/gky427
Weissman, Z., Shemer, R., and Kornitzer, D. (2002). Deletion of the copper transporter CaCCC2 reveals two distinct pathways for iron acquisition in Candida albicans. Mol. Microbiol. 44, 1551–1560. doi:10.1046/j.1365-2958.2002.02976.x
Whitnall, M., Howard, J., Ponka, P., and Richardson, D. R. (2006). A class of iron chelators with a wide spectrum of potent antitumor activity that overcomes resistance to chemotherapeutics. Proc. Natl. Acad. Sci. U. S. A. 103, 14901–14906. doi:10.1073/pnas.0604979103
Wichka, I., and Lai, P. K. (2024). Rapid discovery of Transglutaminase 2 inhibitors for celiac disease with boosting ensemble machine learning. Comput. Struct. Biotechnol. J. 23, 3669–3679. doi:10.1016/j.csbj.2024.10.019
Wu, J., Chen, Y., Wu, J., Zhao, D., Huang, J., Lin, M., et al. (2024a). Large-scale comparison of machine learning methods for profiling prediction of kinase inhibitors. J. Cheminform 16, 13. doi:10.1186/s13321-023-00799-5
Wu, X., Liang, J., Meng, L., Wang, B., Liu, B., and Jin, Y. (2024b). Towards novel small-molecule inhibitors blocking PD-1/PD-L1 pathway: from explainable machine learning models to molecular dynamics simulation. Int. J. Biol. Macromol. 282, 136325. doi:10.1016/j.ijbiomac.2024.136325
Xiong, J., Lu, H., and Jiang, Y. (2025). Mechanisms of azole potentiation: insights from drug repurposing approaches. ACS Infect. Dis. 11, 305–322. doi:10.1021/acsinfecdis.4c00657
Xu, N., Qian, K., Dong, Y., Chen, Y., Yu, Q., Zhang, B., et al. (2014). Novel role of the Candida albicans ferric reductase gene CFL1 in iron acquisition, oxidative stress tolerance, morphogenesis and virulence. Res. Microbiol. 165, 252–261. doi:10.1016/j.resmic.2014.03.001
Xu, S., and Lu, Z. (2024). Exploring FNDC4 as a biomarker for prognosis and immunotherapy response in lung adenocarcinoma. Asian J. Surg. 48, 1799–1801. doi:10.1016/j.asjsur.2024.09.054
Yan, C., Geng, A., Pan, Z., Zhang, Z., and Cui, F. (2024). MultiFeatVotPIP: a voting-based ensemble learning framework for predicting proinflammatory peptides. Brief. Bioinform 25, bbae505. doi:10.1093/bib/bbae505
Yang, Z., Zhou, D., and Huang, J. (2023). Identifying explainable machine learning models and a novel SFRP2(+) fibroblast signature as predictors for precision medicine in ovarian cancer. Int. J. Mol. Sci. 24, 16942. doi:10.3390/ijms242316942
Yap, C. W. (2011). PaDEL-descriptor: an open source software to calculate molecular descriptors and fingerprints. J. Comput. Chem. 32, 1466–1474. doi:10.1002/jcc.21707
Yu, T., Huang, T., Yu, L., Nantasenamat, C., Anuwongcharoen, N., Piacham, T., et al. (2023). Exploring the chemical space of CYP17A1 inhibitors using cheminformatics and machine learning. Molecules 28, 1679. doi:10.3390/molecules28041679
Yu, T. H., Su, B. H., Battalora, L. C., Liu, S., and Tseng, Y. J. (2022). Ensemble modeling with machine learning and deep learning to provide interpretable generalized rules for classifying CNS drugs with high prediction power. Brief. Bioinform 23, bbab377. doi:10.1093/bib/bbab377
Yuan, J., Lovejoy, D. B., and Richardson, D. R. (2004). Novel di-2-pyridyl-derived iron chelators with marked and selective antitumor activity: in vitro and in vivo assessment. Blood 104, 1450–1458. doi:10.1182/blood-2004-03-0868
Zhai, H., Zeng, J., Ma, X., Wang, F., Xu, W., Song, M., et al. (2025). Baicalein as a potent antifungal agent against Candida albicans: synergy with fluconazole and sustainable production through probiotic-mediated bioconversion. Front. Microbiol. 16, 1562103. doi:10.3389/fmicb.2025.1562103
Zhang, C., Zhang, H., Zhang, Q., Fan, H., Yu, P., Xia, W., et al. (2024a). Targeting ATP catalytic activity of chromodomain helicase CHD1L for the anticancer inhibitor discovery. Int. J. Biol. Macromol. 281, 136678. doi:10.1016/j.ijbiomac.2024.136678
Zhang, W., Zhang, L., Dong, H., and Peng, H. (2024b). TGIF2 is a potential biomarker for diagnosis and prognosis of glioma. Front. Immunol. 15, 1356833. doi:10.3389/fimmu.2024.1356833
Zhen, C., Wang, L., Feng, Y., Whiteway, M., Hang, S., Yu, J., et al. (2024). Otilonium bromide exhibits potent antifungal effects by blocking ergosterol plasma membrane localization and triggering cytotoxic autophagy in Candida albicans. Adv. Sci. (Weinh) 11, e2406473. doi:10.1002/advs.202406473
Zhou, J., Jiang, Y., Zhao, J., Zhang, H., Fu, J., Luo, P., et al. (2020). Dp44mT, an iron chelator, suppresses growth and induces apoptosis via RORA-mediated NDRG2-IL6/JAK2/STAT3 signaling in glioma. Cell Oncol. (Dordr) 43, 461–475. doi:10.1007/s13402-020-00502-y
Keywords: ensemble learning, multi-omics, Dp44mT, iron homeostasis, Candida albicans
Citation: Chai X, Jiang Y, Lu H and Huang X (2025) Integrating ensemble machine learning and multi-omics approaches to identify Dp44mT as a novel anti-Candida albicans agent targeting cellular iron homeostasis. Front. Pharmacol. 16:1574990. doi: 10.3389/fphar.2025.1574990
Received: 11 February 2025; Accepted: 11 April 2025;
Published: 24 April 2025.
Edited by:
Jing Xing, Lingang Laboratory, ChinaCopyright © 2025 Chai, Jiang, Lu and Huang. This is an open-access article distributed under the terms of the Creative Commons Attribution License (CC BY). The use, distribution or reproduction in other forums is permitted, provided the original author(s) and the copyright owner(s) are credited and that the original publication in this journal is cited, in accordance with accepted academic practice. No use, distribution or reproduction is permitted which does not comply with these terms.
*Correspondence: Yuanying Jiang, amlhbmd5eUB0b25namkuZWR1LmNu; Hui Lu, bHVodWkyMDE5QHRvbmdqaS5lZHUuY24=; Xin Huang, YWxpZGFfaHVhbmdAMTYzLmNvbQ==