- 1Department of Psychiatry and Psychotherapy, Faculty of Medicine, Medical Center – University of Freiburg, Freiburg, Germany
- 2Department of Child and Adolescent Psychiatry, Psychotherapy and Psychosomatics, Faculty of Medicine, Medical Center – University of Freiburg, Freiburg, Germany
- 3Medical Psychology and Medical Sociology, Faculty of Medicine, University of Freiburg, Freiburg, Germany
- 4Department of Neurology, Faculty of Medicine, Medical Center – University of Freiburg, Freiburg, Germany
- 5Department of Neuroradiology, Faculty of Medicine, Medical Center – University of Freiburg, Freiburg, Germany
Objective: Autism spectrum (ASD) and attention-deficit/hyperactivity disorder (ADHD) are neurodevelopmental disorders with a high rate of comorbidity. To date, diagnosis is based on clinical presentation and distinct reliable biomarkers have been identified neither for ASD nor ADHD. Most previous neuroimaging studies investigated ASD and ADHD separately.
Method: To address the question of structural brain differences between ASD and ADHD, we performed FreeSurfer analysis in a sample of children with ADHD (n = 30), with high-functioning ASD (n = 14), with comorbid high-functioning ASD and ADHD (n = 15), and of typically developed controls (TD; n = 36). With FreeSurfer, an automated brain imaging processing and analyzing suite, we reconstructed the cerebral cortex and calculated gray matter volumes as well as cortical surface parameters in terms of cortical thickness and mean curvature.
Results: A significant main effect of the factor ADHD was detected for the left inferior frontal gyrus (Pars orbitalis) volume, with the ADHD group exhibiting smaller Pars orbitalis volumes. Dimensional measures of autism (SRS total raw score) and ADHD (DISYPS-II FBB-ADHD score) had no significant influence on the left Pars orbitalis volume. Both, ASD and ADHD tended to have an effect on cortical thickness or mean curvature, which did not survive correction for multiple comparisons.
Conclusion: Our results underline that ADHD rather than ASD is associated with volume loss in the left inferior frontal gyrus (Pars orbitalis). This area might play a relevant role in modulating symptoms of inattention and/or impulsivity in ADHD. The effect of comorbid ADHD in ASD samples and vice versa, on cortical thickness and mean curvature, requires further investigation in larger samples.
Introduction
Previous studies mainly investigated autism spectrum disorder (ASD) and attention-deficit/hyperactivity disorder (ADHD) in isolation. The question to what extent there is a clinical overlap between ADHD and ASD, whether they represent distinct diagnostic categories or form a continuous disorder with a continuum of brain dysfunction remains open to discussion (1, 2). To identify endophenotypes across diagnostic categories, it is important to further investigate the overlap between ADHD and ASD on a behavioral, neurocognitive, and neurobiological level (3, 4).
Co-occurrence of ASD and ADHD
ASD is characterized by impairment in social communication and interaction as well as by a range of stereotypic behaviors whereas deficits in attention, hyperactivity, impulsiveness, disorganization and affective instability represent the core symptoms of ADHD (5). As ASD is a frequent comorbid condition in ADHD and vice versa (1), there is the possibility of dual diagnosis according to DSM-5 (5). The interpretation of studies prior to 2013 is limited by DSM-IV guidelines not permitting the dual diagnosis of ASD and ADHD (6).
Studies report frequent co-occurrence with 30-50% of individuals with ASD manifesting ADHD symptoms (particularly at pre-school age) and two-thirds of individuals with ADHD showing features of ASD (7). Social difficulties in ADHD are often interpreted as part of ADHD symptoms rather than reflecting impairments in social communication being characteristic for ASD (8). Both disorders are highly heritable (9).
Previous Volumetric Studies in ASD and ADHD
Previous structural neuroimaging studies encompassing children and adolescents with both, ADHD and ASD, are scarce and show heterogeneous results (Table 1). There is only one previous FreeSurfer study on ASD children with and without comorbid ADHD, but without a separate ADHD group (12). Mahajan et al. (12) found that gray matter (GM) volume and surface area (SA) were increased in the left postcentral and the right precentral gyrus which in this study was specific for ASD children without ADHD, whereas an increase in the left precentral gyrus was specific for children with ASD and comorbid ADHD. Regardless of ADHD comorbidity, all children with ASD showed increases in GM volume and SA in the left inferior parietal cortex (12).
Voxel based morphometry (VBM) studies display a heterogeneous picture. The most recent VBM study suggested GM reduction in the right posterior cerebellum to be disorder-specific for ADHD relative to ASD. GM enlargement in the middle/superior temporal gyrus, on the other hand, was reported to be disorder-specific for ASD relative to ADHD (11). An earlier VBM study pointed toward shared GM volume reduction within the medial temporal and higher GM in the inferior parietal cortex (3). Further, increased GM volume of the supramarginal gyrus was reported in ASD, but not ADHD, relative to controls (3). In the largest VBM study so far an increasing ASD score was associated with greater global GM volume (10).
In ASD it has frequently been reported that after having a normal (13) or smaller (14) brain size at birth, there is a period of early brain overgrowth prior to 4 years of age (14–16). The pathophysiology of such alterations is unknown, but it is proposed to result from deviant neuronal proliferation and axonal growth during fetal development that in turn leads to an aberrant developmental pruning (17). In contrast, in children with ADHD, smaller whole brain volumes (18–20) and lower GM volumes have been described (21). It is hypothesized that ADHD children show a delayed brain maturation process (22).
Despite similarities in clinical presentation as well as mutual comorbidity rates in ASD and ADHD, these disorders present a rather different neuroanatomical profile. Most studies report subcortical temporal structures such as the amygdala to be enlarged in young children (at ages 2–4) with ASD (23) with a normalization in late childhood and adolescence (24). Amygdala volumes in adults with ADHD have been found to be relatively normal (25, 26) or smaller than in controls (27). Basal ganglia are reported to be enlarged (15) in ASD and smaller in ADHD (1). With regard to the corpus callosum, thalamus and cerebellum, however, in many studies ASD and ADHD show a volume reduction (1).
Previous Cortical Surface Parameter Studies in ASD and ADHD
Studies focusing on cortical surface parameters in ASD also reported mixed results. When investigating cortical surface parameters, it has to be reflected that cortical development in ASD varies across developmental stages or brain regions. Three different phases have been proposed: accelerated expansion in early childhood, accelerated thinning in later childhood and adolescence, and decelerated thinning in early adulthood (28). Hazlett et al. (29) examined young children with ASD (ages 2–5 years) and found increased cortical volumes, but no alterations in cortical thickness implicating that brain enlargement may be associated with increased cortical SA in ASD. Increased cortical thickness in temporal lobes was reported in children (ages 8–12 years) with ASD (30) with greater cortical thinning in ASD over time especially in occipital regions (31). Greater cortical thinning was associated with more severe symptoms in ASD (31). Further investigations pointed toward cortical thinning in adolescents (ages 12–25 years) with ASD (32–34). Studies in adults are divergent with some reporting cortical thinning (35–37) in brain regions involved in social cognition, others cortical thickening within frontal lobe regions (38) or regions from all four lobes (36). A large recent study found no significant difference in overall cortical thickness or surface area between ASD and typically developed (TD) (39).
In children and adults with ADHD, cortical thinning has been described in parietal and frontal regions responsible for executive function and attention (40–42). Another study detected no differences in cortical thickness of ADHD children, but decreased SA and cortical folding (43).
The unclear and puzzling current state requires further studies directly comparing volumetric and cortical thickness parameters between ASD and ADHD. Individuals with isolated autism and individuals, who present comorbid conditions in terms of ADHD, can be distinguished behaviorally as already documented by our research group (44).
Rationale of Our Study
Based on the available evidence, we aimed to study the brain structure in children with ASD with and without comorbid ADHD as well as TD. To address the question of a potential neurobiological overlap between ADHD and ASD, we analyzed the brain scans for shared and disorder-specific abnormalities. We investigated differences in terms of GM as well as cortical thickness and mean curvature.
In doing so, this study represents the first FreeSurfer study comprising ASD and ADHD groups, as well as subjects with co-occurrence of both conditions. Because previous studies showed inconsistent and widely distributed changes, we did not limit the analysis to individual a priori regions of interest (ROIs).
Materials and Methods
Participants
The ethics committee of the University Medical Center Freiburg approved the study (approval ID: 279/06). Magnetic resonance imaging (MRI) scans were acquired following written informed consent of the children's parents. Male children with ASD and ADHD were recruited from the Department of Child and Adolescent Psychiatry, Psychotherapy, and Psychosomatics of the University Medical Center Freiburg.
We obtained scans of high quality in 40 male children with a diagnosis of ASD according to ICD-10 and DSM-5 criteria. Twenty-nine ASD patients were included in the final analysis after 7 patients were excluded due to image artifacts, 3 due to IQ < 70 and one patient due to comorbid seizures. All ASD children were high-functioning and with no language delay. Intellectual disability (full scale IQ below 70), comorbid Tourette syndrome or severe neurological diseases were defined as exclusion criteria. With the exception of 2 patients, no ASD participant had comorbid depressive or anxiety symptoms. The diagnostic process followed international guidelines, including the Autism Diagnostic Observation Schedule [ADOS-G, (45)] and the Autism Diagnostic Interview [ADI-R, (46)]. Psychometric tools included the Child Behavior Checklist [CBCL, (47)], the Social Responsiveness Scale [SRS, (48)], and the diagnostic interview K-SADS-PL (49). According to the K-SADS-PL, 15 autistic children additionally met the diagnostic criteria for ADHD. The ADHD diagnosis was confirmed with the DISYPS-II FBB-ADHD (50), and verified by a multi-professional team of expert clinicians to ensure a comorbid ADHD. The group without (14 patients) and the group with comorbid ADHD (15 patients) were not significantly different for age and IQ.
Additionally, MRI-scans of 50 male patients with ICD-10 and DSM-5 ADHD diagnosis without a comorbid ASD were acquired. Twelve scans were excluded due to poor image quality, 7 due to the low IQ of the subjects (< 70) and one due to an arachnoid cyst, so that finally 30 ADHD patients were analyzed. ADHD diagnosis was clinically based on ICD-10 and DSM-5 criteria and additionally confirmed with the DISYPS-II FBB-ADHD (50). ASD symptoms were ruled out applying the SRS score (48), also the CBCL (47) was consulted. With the exception of one ADHD patient, no one suffered from comorbid depression or anxiety disorder as assessed by the K-SADS-PL (49). Methylphenidate medication in children with an ADHD diagnosis was discontinued at least 24 h prior to scanning procedure.
Fourty-eight typically developed male children (TD) were recruited from local schools and sport groups. Control subjects were included after a phone interview with the parents who additionally completed a sociodemographic questionnaire, the CBCL (47) and the SRS (48) for ruling out ASD and ADHD symptoms. Four children were excluded from the TD group, because of the presence of ADHD or autistic symptoms, 7 due to imaging artifacts and one due to an IQ < 70, so that we finally included 36 male TD participants in the study.
Subjects were matched according to IQ assessed with Raven's Standard Progressive Matrices (51), age and sex.
All subjects included in the study accomplished behavioral tasks of executive functions and planning as well. The results are published elsewhere (44).
Image Acquisition
A standard magnetization-prepared rapid gradient echo (MPRAGE) T1-weighted anatomical scan was conducted (relaxation time = 2,200 ms, echo time = 2.15 ms, flip angle = 12°, inversion time = 1,100 ms) on a 3T Siemens TIM Trio Magnetom scanner (Erlangen, Germany). Slice thickness was 1 mm and voxel size 1 × 1 × 1 mm3.
Brain Segmentation
Cortical reconstruction and segmentation was performed using FreeSurfer version 5.3 (http://surfer.nmr.mgh.harvard.edu/). FreeSurfer is a fully automated suite of tools that enables analysis of key features in the human brain such as segmentation of most macroscopically visible brain structures (52). FreeSurfer allows to compute the volume of subcortical areas and reconstructs the cerebral cortex (53). It also provides information about mapping of cortical GM thickness (54) and the construction of surface models of the cerebral cortex (55). The technical details of FreeSurfer procedures are described elsewhere (52). Applying FreeSurfer, we removed non-brain tissue and segmented cortical and subcortical GM and WM depending on image intensity. FreeSurfer output was inspected by three blinded trainees and rated on a scale ranging from 1 to 4. A “1” means no visible artifacts, whereas “4” denotes distinct blurred and low-quality images. Manual correction followed recommendations of FreeSurfer developers (https://surfer.nmr.mgh.harvard.edu/fswiki/FsTutorial/PialEdits_freeview).
MRI scans of poor quality, which showed geometric inaccuracies, were rated “4” (blurred and low quality) or for which the segmentation procedure failed, were excluded.
Region of Interest Parcellation
Individual brains were registered on a spherical atlas for parcellation, taking into account individual cortical folding patterns to match brain geometry between the subjects. FreeSurfer parcellated each brain into 148 GM and 32 subcortical ROIs using the Desikan-Killiany-Atlas (56). Afterwards, ROI labels were transformed back into each subject's individual space to compute the volume of each ROI.
Surface and Cortical Thickness
Cortical surface area was calculated with FreeSurfer based on a 2D representation of cortical surface after estimation of GM/WM boundary and pial surface (54). Cortical thickness was then calculated for each vertex as distance from the GM/WM boundary and the pial surface. FreeSurfer offers better alignment of cortical landmarks than volume-based registration and does not produce an age-associated bias between older and younger children when registering children's brains to a common space (57).
Statistical Analysis
Psychometric Data
Group comparisons of demographic and psychometric data (age, IQ, psychometric scores) were carried out using SPSS software, version 22 (IBM Corp., Armonk, NY, USA). We used analysis of variance (ANOVAs) for the assessment of significance of putative differences.
Analysis of Imaging Data
Further analysis of imaging data was carried out using R statistical computing software (58). We tested for differences in cortical GM volumes respecting all regions of FreeSurfer segmentation according to the Desikan-Killiany-Atlas (56). Additionally, we focused on cortical surface parameters in terms of cortical thickness and mean curvature of ROIs, again defined with the Desikan-Killiany-Atlas (56).
We adjusted volume, mean curvature and thickness data for differences in age and IQ using a linear model applying the groups mean age and IQ.
Type III two-way 2 × 2 ANOVAs on the adjusted volume, thickness and curvature data were calculated using the independent between-subject factors ASD diagnosis (yes vs. no) and ADHD diagnosis (yes vs. no; see Table 2). Results were corrected for multiple comparisons applying false discovery rate (FDR) correction. FDR corrected p < 0.05 were considered significant, and uncorrected p < 0.05 were regarded as trends. We didn't restrict our analysis to a priori regions of interest.
Multiple regression models with either SRS total raw score or DISYPS-II FBB-ADHD as independent variables were conducted with the adjusted left Pars orbitalis volume as dependent variables. The regression model included an interaction term with the binary moderator variables ASD and ADHD.
Results
Demographic and Psychometric Data
Table 3 summarizes the demographic and psychometric data of the ASD, ADHD, and TD group. The study included 95 male participants (6–13 years old): 14 with ASD without comorbid ADHD, 15 with ASD and comorbid ADHD, 30 ADHD, and 36 TD controls. Groups did not differ significantly with respect to age and IQ.
Volumetric Results
The two-way ANOVA model showed a significant main effect of the factor ADHD for the left Pars orbitalis volume after FDR correction [F(1, 91) = 12.63; pFDR = 0.039, puncorr < 0.001]. Children with an ADHD diagnosis exhibited smaller left Pars orbitalis volumes (Figure 1). Uncorrected significant effects were regarded as trends (Table 4). A main effect of ADHD on trend level could also be observed in the right Pars orbitalis and precuneus cortex, in terms of a volume reduction. An interaction of the factors ADHD and ASD could be detected for the right isthmus cingulate cortex.
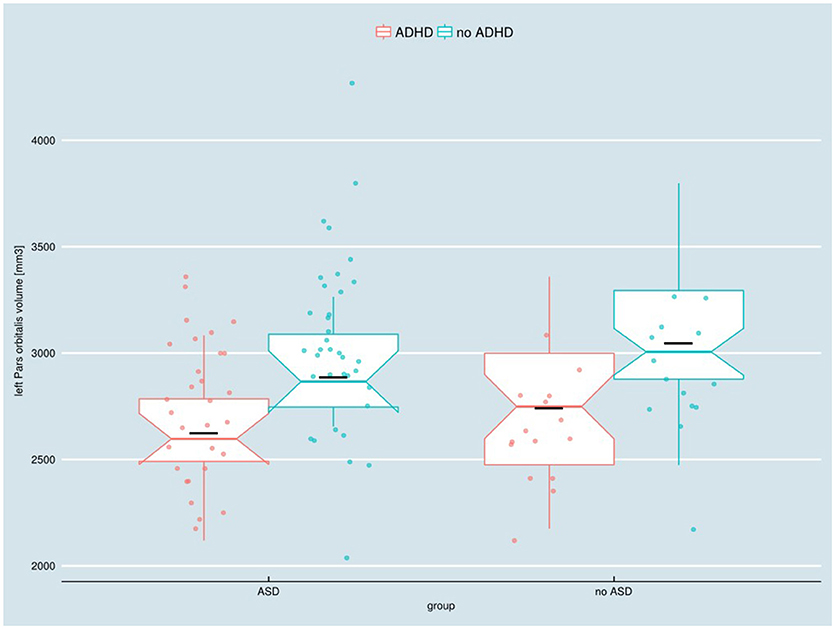
Figure 1. Adjusted left Pars orbitalis volume in children with and without ASD or ADHD, respectively. ADHD, attention-deficit/hyperactivity disorder; ASD, autism spectrum disorder. Boxes indicate upper/lower quartile as well as the median. The black line indicates the sample mean.
Cortical Thickness
Two-way ANOVA models exhibited no significant main effects or interaction surviving FDR correction. The following uncorrected significant effects were regarded as trends (Table 4):
A main effect for the diagnosis ASD emerged in the bilateral postcentral gyrus and the left pericalcarine and cuneus cortex, as well as in the right superior parietal cortex, in terms of cortical thinning. ADHD effects were observable in the left Pars orbitalis, again, linked to a cortical thinning. An interaction of diagnosis ADHD and ASD could be observed for the left inferior parietal, parahippocampal, pericalcarine, transverse temporal, and right post-central thickness measures.
Mean Curvature
The two-way ANOVA model showed no significant interaction or main effects for the factors ASD or ADHD on mean curvature after FDR correction. Uncorrected significant results we regard as trends (Table 4). The right medial orbitofrontal cortex showed a significant main effect of the factor ASD, as did the left postcentral gyrus and right cuneus cortex. ADHD, in turn, had an effect on mean curvature of the left transverse temporal cortex and right Pars triangularis. Uncorrected significant interactions could be found in the left pericalcarine and right medial orbitofrontal cortex.
SRS-Total Score and DISYPS-II Effect on Main Result
Neither the multiple regression model with the independent variable SRS total score nor DISYPS-II FBB-ADHD revealed any significant effect of these scores or their interaction with the factors ADHD and/or ASD on left Pars orbitalis volume.
Discussion
To our knowledge, this is the first FreeSurfer study that examines children with ADHD, ASD, and comorbid ASD and ADHD in a single study. Our investigation focused on the detection of possible morphometric differences (cortical volume, thickness and mean curvature).
Due to the heterogeneity of findings in earlier studies, we did not limit our analysis to a priori regions of interest.
Volumetric Results
The diagnosis ADHD has a significant effect on the left Pars orbitalis volume with ADHD-diagnosed children showing smaller left Pars orbitalis volumes. These findings suggest that ADHD rather than ASD is related to left Pars orbitalis volume loss. Whether there are weaker “additive” effects on the Pars orbitalis volume of ASD and ADHD cannot be ruled out with a study of the given sample size.
On trend level, we additionally found an ADHD main effect for the right Pars orbitalis and precuneus cortex and an interaction of diagnosis ADHD and ASD for the right isthmus cingulate cortex.
The so-called default-mode network (DMN) has been described as comprising the precuneus/posterior cingulate cortex, the medial prefrontal cortex and the medial, lateral and inferior parietal cortex. It is a network of brain regions associated with task-irrelevant mental processes and mind wandering (59, 60) In line with our results, Castellanos et al. (61) showed ADHD-related decreases in functional connectivity between the precuneus and other DMN components.
The Pars Orbitalis of the Inferior Frontal Gyrus
The Pars orbitalis represents a subdivision of the inferior frontal gyrus which more or less corresponds well to the Brodman Area 47.
Functionally it has been linked to the recognition of facial expressions of basic emotions (62) and to the modulation of positive emotionality (63). It is also assumed that besides the specific association between the right inferior frontal gyrus and the inhibitory control, the left inferior frontal gyrus is also involved in the successful implementation of inhibitory control over motor responses (64). This could be partly responsible for the impulsive behavior that can be observed in ADHD.
Children with ADHD and autism have a lot of similar features and there is high frequency of ADHD symptoms in autism (65). Also, in autistic patients, difficulties with emotion and facial recognition have been described (66–69). In addition, it was assumed that Brodmann Area 47 is involved in semantic/syntactic processing (70, 71). Previous studies pointed toward abnormalities in the pragmatic understanding and the use of language in ASD (72). Brothers (73) proposed that there is a network of neural regions (the amygdala, the orbito-frontal cortex, the superior temporal sulcus and gyrus) comprising the “social brain.” Accordingly, reduced Pars orbitalis volume in ASD with comorbid ADHD might not necessarily be responsible for the aforementioned symptoms in ASD, but it may be a complicating factor.
In a recent publication the Pars orbitalis has been implicated as being part of a critical network for the identification of specific ADHD/ASD subtypes (74).
Relationship to Other Publications
The volume reduction in the Pars orbitalis is partly consistent with one previous study which reported a trend toward a lower Pars orbitalis volume in ADHD, but in this investigation, the right Pars orbitalis was affected (75). In the present study, decreased right Pars orbitalis volume could only be observed on an uncorrected level (see Table 4). It is assumed that besides the specific association between the right inferior frontal gyrus and the inhibitory control, the left inferior frontal gyrus is also involved in the successful implementation of inhibitory control over motor responses (64). We could not replicate other volumetric results of the few earlier heterogeneous studies that combined ASD and ADHD patients into a single study (3, 10–12, 76). However, it should be mentioned that previous studies are not directly comparable to our study design. Heterogeneous findings are difficult to interpret due to the often arbitrary distinction between both clinical groups without careful consideration of ASD/ADHD comorbidities (74). Furthermore, different methodology in terms of voxel-based morphometry (VBM) was applied in most previous investigations (3, 10, 11) and none of the earlier investigations included ASD– (ASD without comorbid ADHD), ASD+ (ASD with comorbid ADHD), ADHD, and TD participants (3, 10–12).
Additionally, VBM studies examined adolescent samples. Only the study by Mahajan et al. (12) studied children whose average age corresponded to our study (but a smaller age range than our sample). In fact, age and IQ differences across studies are potential factors leading to heterogeneity of results. Even if covariates are used to correct for age effects, the results cannot be transferred to samples from other age groups or age structures.
Dimensional Correlations
Multiple regression models with the independent variable SRS total score or DISYPS-II FBB-ADHD revealed no significant effect of these scores or their interaction with the factors ADHD and/or ASD on left Pars orbitalis volume. It can therefore be assumed that the reduction of the left Pars orbitalis volume is a categorical effect due to ADHD diagnosis and is not due to the severity of different symptoms or traits represented by questionnaires.
Surface Parameters
No significant interaction or main effects for the factors ASD or ADHD on cortical thickness or mean curvature could be detected after FDR correction. The fact that significant group effects only emerged on an uncorrected level might indicate that the effect sizes of possible differences are too small to be detected with the present group size. We decided to interpret the uncorrected significant differences as possible trends.
In doing so, a main effect for ASD diagnosis emerged for the bilateral post-central, left pericalcarine, left cuneus as well as right superior parietal cortical thickness, and an effect for the factor ADHD resulted for the left Pars orbitalis thickness. An interaction of ASD and ADHD diagnosis was detected for left inferior parietal, parahippocampal, pericalcarine, transverse temporal, and right post-central thickness measures.
Our results of cortical thinning in several areas in ASD children (aged 6–13 years) are concordant with studies reporting accelerated thinning in childhood ASD (28). Greater cortical thinning was associated with more severe symptomatology in ASD (31). Another previous study focusing on ASD children (aged 6–12 years) revealed widespread, but mostly left-hemispheric thinning in frontal, temporal, parietal and occipital brain areas related to the theory-of-mind network (77). It should be noted that cortical development in ASD is most likely subject to three different phases: accelerated expansion in early childhood, accelerated thinning in later childhood and adolescence, and decelerated thinning in early adulthood (28). Therefore, when comparing different studies in childhood and adulthood, the exact stage of development must be taken into account.
The detected cortical thinning in the superior parietal cortex in ASD relates well with previous investigations (28, 33). The superior parietal lobule showed decreased activation during learning in ASD and was suggested to play an important role in motor learning and repetitive behaviors (78). Higher SRS- total scores, indicating autistic traits, were associated with thinner cortex in the left superior parietal lobule (79). The postcentral gyrus is important for the representation of haptic and proprioceptive feedback (12). In line with this observation, previous research revealed differences of ASD children in tactile discrimination in comparison to TD (80, 81).
The definite pathomechanisms resulting in cortical thinning are not yet clarified. Regressive (e.g., synaptic pruning) and progressive (e.g., myelination) events are supposed to result in the appearance of GM density reduction or cortical thinning (82), but further research is required.
Methodological Issues and Limitations
The study was conducted with a sample of children with ASD or ADHD being prone to motion artifacts (83). Therefore, many of the MRI brain images were excluded from analysis due to poor image quality, which could have biased the study results. We applied manual inspection and correction as suggested by the recommendations of the developers of FreeSurfer. Nevertheless, we cannot completely rule out any confounding effects induced by head motion. Most previous studies did not quantify the degree of observed motion in groups (1). Therefore, differences in the applied (or not applied) motion correction or exclusion criteria might partly be responsible for the heterogeneity of results across studies. To date, a quantification of head motion as described in diffusion tensor imaging (DTI) studies is not possible in FreeSurfer morphometric studies (84). There is very sparse evidence of utilization of automated quality metrics in FreeSurfer studies (85, 86).
Due to the study's focus on primary forms of ASD the results presented here can not necessarily be generalized to forms of ASD with intellectual impairment or to syndromal-secondary autism (defined as autism with known etiology) (87). Additionally, a larger total sample size would have been desirable to detect more potential subtle differences.
Methylphenidate medication was discontinued at least 24 h prior to scanning procedure. Evidence suggests an impact of long-term neurotropic medication on brain structure with stimulant medication being associated with normalization of structural abnormalities in ADHD (88). This confounding factor might have influenced our results, yet, given the earlier literature, not in terms of a volume loss as we report in this study (89). Furthermore, for future studies, it would be helpful to choose longitudinal designs to study longitudinal neurodevelopmental trajectories of ASD and ADHD vs. TD.
Conclusion
In summary, we detected that ADHD rather than ASD mediates volume loss in the inferior frontal gyrus (Pars orbitalis). The volume reduction in the left Pars orbitalis seems to be primarily a categorical diagnostic effect than to reflect the severity of various traits or symptoms. ASD and ADHD diagnoses tended to have an effect on cortical thickness or mean curvature, which did not survive correction for multiple comparisons. Further studies of more power in larger samples are necessary to investigate the effect of ADHD and ASD on cortical thickness and mean curvature. Additionally, further research is needed to disentangle the precise causal pathways.
Author Contributions
MB is principal investigator of the study funded by German Federal Ministry of Education and Research (BMBF; grant number 01GW0710). MB, JU, RR, IM, CK, and CPK designed the study and were responsible for acquisition and analysis of data. SM, KN, and JM performed FreeSurfer analysis of the data. KN, SM, JM, MB, DE, AR, and LTvE were crucially involved in the theoretical discussion and preparation of the manuscript. KN and SM wrote the manuscript. All authors read and approved the final version of the manuscript. They agreed to be accountable for all aspects of the work.
Conflict of Interest Statement
LTvE advisory boards, lectures, or travel grants within the last 4 years: Eli Lilly: Janssen-Cilag, Novartis, Shire, UCB, GSK, Servier, Janssen, and Cyberonics.
The remaining authors declare that the research was conducted in the absence of any commercial or financial relationships that could be construed as a potential conflict of interest.
Acknowledgments
The study was supported by the German Federal Ministry of Education and Research (BMBF; grant number 01GW0710). The article processing charge was funded by the German Research Foundation (DFG) and the University of Freiburg in the funding programme Open Access Publishing.
References
1. Dougherty CC, Evans DW, Myers SM, Moore GJ, Michael AM. A Comparison of structural brain imaging findings in autism spectrum disorder and attention-deficit hyperactivity disorder. Neuropsychol Rev. (2016) 26:25–43. doi: 10.1007/s11065-015-9300-2
2. Gillberg C, Fernell E. Autism plus versus autism pure. J Autism Dev Disord. (2014) 44:3274–76. doi: 10.1007/s10803-014-2163-1
3. Brieber S, Neufang S, Bruning N, Kamp-Becker I, Remschmidt H, Herpertz-Dahlmann B, et al. Structural brain abnormalities in adolescents with autism spectrum disorder and patients with attention deficit/hyperactivity disorder. J Child Psychol Psychiatry (2007) 48:1251–8. doi: 10.1111/j.1469-7610.2007.01799.x
4. Rommelse NNJ, Geurts HM, Franke B, Buitelaar JK, Hartman CA. A review on cognitive and brain endophenotypes that may be common in autism spectrum disorder and attention-deficit/hyperactivity disorder and facilitate the search for pleiotropic genes. Neurosci Biobehav Rev. (2011) 35:1363–96. doi: 10.1016/j.neubiorev.2011.02.015
5. American Psychiatric Association. Diagnostic and Statistical Manual Of Mental Disorders. 5th ed. Arlington, TX: American Psychiatric Publishing (2013).
6. Epstein JN, Loren REA. Changes in the definition of ADHD in DSM-5: subtle but important. Neuropsychiatry (2013) 3:455–8. doi: 10.2217/npy.13.59
7. Leitner Y. The co-occurrence of autism and attention deficit hyperactivity disorder in children - what do we know? Front Hum Neurosci. (2014) 8:268. doi: 10.3389/fnhum.2014.00268
8. Grzadzinski R, Di Martino A, Brady E, Mairena MA, O'Neale M, Petkova E, et al. Examining autistic traits in children with ADHD: does the autism spectrum extend to ADHD? J Autism Dev Disord. (2011) 41:1178–91. doi: 10.1007/s10803-010-1135-3
9. Rommelse NNJ, Franke B, Geurts HM, Hartman CA, Buitelaar JK. Shared heritability of attention-deficit/hyperactivity disorder and autism spectrum disorder. Eur Child Adolesc Psychiatry (2010) 19:281–295. doi: 10.1007/s00787-010-0092-x
10. O'Dwyer L, Tanner C, van Dongen EV, Greven CU, Bralten J, Zwiers MP, et al. Brain volumetric correlates of autism spectrum disorder symptoms in attention deficit/hyperactivity disorder. PloS ONE (2014) 9:e101130. doi: 10.1371/journal.pone.0101130
11. Lim L, Chantiluke K, Cubillo AI, Smith AB, Simmons A, Mehta MA, et al. Disorder-specific gray matter deficits in attention deficit hyperactivity disorder relative to autism spectrum disorder. Psychol Med. (2015) 45:965–76. doi: 10.1017/S0033291714001974
12. Mahajan R, Dirlikov B, Crocetti D, Mostofsky SH. Motor circuit anatomy in children with autism spectrum disorder with or without attention deficit hyperactivity disorder. Autism Res. (2016) 9:67–81. doi: 10.1002/aur.1497
13. Hazlett HC, Poe M, Gerig G, Smith RG, Provenzale J, Ross A, et al. Magnetic resonance imaging and head circumference study of brain size in autism: birth through age 2 years. Arch Gen Psychiatry (2005) 62:1366–76. doi: 10.1001/archpsyc.62.12.1366
14. Courchesne E, Carper R, Akshoomoff N. Evidence of brain overgrowth in the first year of life in autism. J Am Med Assoc. (2003) 290:337–44. doi: 10.1001/jama.290.3.337
15. Langen M, Bos D, Noordermeer SDS, Nederveen H, van Engeland H, Durston S. Changes in the development of striatum are involved in repetitive behavior in autism. Biol Psychiatry (2014) 76:405–11. doi: 10.1016/j.biopsych.2013.08.013
16. Nordahl CW, Lange N, Li DD, Barnett LA, Lee A, Buonocore MH, et al. Brain enlargement is associated with regression in preschool-age boys with autism spectrum disorders. Proc Natl Acad Sci USA. (2011) 108:20195–200. doi: 10.1073/pnas.1107560108
17. McFadden K, Minshew NJ. Evidence for dysregulation of axonal growth and guidance in the etiology of ASD. Front Hum Neurosci. (2013) 7:671. doi: 10.3389/fnhum.2013.00671
18. Batty MJ, Liddle EB, Pitiot A, Toro R, Groom MJ, Scerif G, et al. Cortical gray matter in attention-deficit/hyperactivity disorder: a structural magnetic resonance imaging study. J Am Acad Child Adolesc Psychiatry (2010) 49:229–38. doi: 10.1016/j.jaac.2009.11.008
19. Durston S, Hulshoff Pol HE, Schnack HG, Buitelaar JK, Steenhuis MP, Minderaa RB, et al. Magnetic resonance imaging of boys with attention-deficit/hyperactivity disorder and their unaffected siblings. J Am Acad Child Adolesc Psychiatry (2004) 43:332–40. doi: 10.1097/00004583-200403000-00016
20. Hill DE, Yeo RA, Campbell RA, Hart B, Vigil J, Brooks W. Magnetic resonance imaging correlates of attention-deficit/hyperactivity disorder in children. Neuropsychology (2003) 17:496–506. doi: 10.1037/0894-4105.17.3.496
21. Batty MJ, Palaniyappan L, Scerif G, Groom MJ, Liddle EB, Liddle PF, et al. Morphological abnormalities in prefrontal surface area and thalamic volume in attention deficit/hyperactivity disorder. Psychiatry Res. (2015) 233:225–32. doi: 10.1016/j.pscychresns.2015.07.004
22. Shaw P, Eckstrand K, Sharp W, Blumenthal J, Lerch JP, Greenstein D, et al. Attention-deficit/hyperactivity disorder is characterized by a delay in cortical maturation. Proc Natl Acad Sci USA. (2007) 104:19649–54. doi: 10.1073/pnas.0707741104
23. Nordahl CW, Scholz R, Yang X, Buonocore MH, Simon T, Rogers S, et al. Increased rate of amygdala growth in children aged 2 to 4 years with autism spectrum disorders: a longitudinal study. Arch Gen Psychiatry (2012) 69:53–61. doi: 10.1001/archgenpsychiatry.2011.145
24. Barnea-Goraly N, Frazier TW, Piacenza L, Minshew NJ, Keshavan MS, Reiss AL, et al. A preliminary longitudinal volumetric MRI study of amygdala and hippocampal volumes in autism. Prog Neuropsychopharmacol Biol Psychiatry (2014) 48:124–128. doi: 10.1016/j.pnpbp.2013.09.010
25. Perlov E, Philipsen A, Tebartz van Elst L, Ebert D, Henning J, Maier S, et al. Hippocampus and amygdala morphology in adults with attention-deficit hyperactivity disorder. J Psychiatry Neurosci. (2008) 33:509–15.
26. Plessen KJ, Bansal R, Zhu H, Whiteman R, Amat J, Quackenbush GA, et al. Hippocampus and amygdala morphology in attention-deficit/hyperactivity disorder. Arch Gen Psychiatry (2006) 63:795–807. doi: 10.1001/archpsyc.63.7.795
27. Tajima-Pozo K, Yus M, Ruiz-Manrique G, Lewczuk A, Arrazola J, Montañes-Rada F. Amygdala abnormalities in adults with ADHD. J Atten Disord. (2016) 22: 671–8. doi: 10.1177/1087054716629213
28. Zielinski BA, Prigge MBD, Nielsen JA, Froehlich AL, Abildskov TJ, Anderson JS, et al. Longitudinal changes in cortical thickness in autism and typical development. Brain J Neurol. (2014) 137:1799–1812. doi: 10.1093/brain/awu083
29. Hazlett HC, Poe MD, Gerig G, Styner M, Chappell C, Smith RG, et al. Early brain overgrowth in autism associated with an increase in cortical surface area before age 2 years. Arch Gen Psychiatry (2011) 68:467–76. doi: 10.1001/archgenpsychiatry.2011.39
30. Hardan AY, Muddasani S, Vemulapalli M, Keshavan MS, Minshew NJ. An MRI study of increased cortical thickness in autism. Am J Psychiatry (2006) 163:1290–92. doi: 10.1176/appi.ajp.163.7.1290
31. Hardan AY, Libove RA, Keshavan MS, Melhem NM, Minshew NJ. A preliminary longitudinal magnetic resonance imaging study of brain volume and cortical thickness in autism. Biol Psychiatry (2009) 66:320–326. doi: 10.1016/j.biopsych.2009.04.024
32. Chung MK, Robbins SM, Dalton KM, Davidson RJ, Alexander AL, Evans AC. Cortical thickness analysis in autism with heat kernel smoothing. NeuroImage (2005) 25:1256–65. doi: 10.1016/j.neuroimage.2004.12.052
33. Ecker C, Shahidiani A, Feng Y, Daly E, Murphy C, D'Almeida V, et al. The effect of age, diagnosis, and their interaction on vertex-based measures of cortical thickness and surface area in autism spectrum disorder. J Neural Transm. (2014) 121:1157–70. doi: 10.1007/s00702-014-1207-1
34. Wallace GL, Eisenberg IW, Robustelli B, Dankner N, Kenworthy L, Giedd JN, et al. Longitudinal cortical development during adolescence and young adulthood in autism spectrum disorder: increased cortical thinning but comparable surface area changes. J Am Acad Child Adolesc Psychiatry (2015) 54:464–69. doi: 10.1016/j.jaac.2015.03.007
35. Hadjikhani N, Joseph RM, Snyder J, Tager-Flusberg H. Anatomical differences in the mirror neuron system and social cognition network in autism. Cereb Cortex (2006) 16:1276–82. doi: 10.1093/cercor/bhj069
36. Hyde KL, Samson F, Evans AC, Mottron L. Neuroanatomical differences in brain areas implicated in perceptual and other core features of autism revealed by cortical thickness analysis and voxel-based morphometry. Hum Brain Mapp. (2010) 31:556–66. doi: 10.1002/hbm.20887
37. Scheel C, Rotarska-Jagiela A, Schilbach L, Lehnhardt FG, Krug B, Vogeley K, et al. Imaging derived cortical thickness reduction in high-functioning autism: key regions and temporal slope. NeuroImage (2011) 58:391–400. doi: 10.1016/j.neuroimage.2011.06.040
38. Ecker C, Ginestet C, Feng Y, Johnston P, Lombardo MV, Lai M-C, et al. Brain surface anatomy in adults with autism: the relationship between surface area, cortical thickness, and autistic symptoms. JAMA Psychiatry (2013) 70:59–70. doi: 10.1001/jamapsychiatry.2013.265
39. Haar S, Berman S, Behrmann M, Dinstein I. Anatomical Abnormalities in Autism? Cereb Cortex (2016) 26:1440–52. doi: 10.1093/cercor/bhu242
40. Hoekzema E, Carmona S, Ramos-Quiroga JA, Richarte Fernández V, Picado M, Bosch R, et al. Laminar thickness alterations in the fronto-parietal cortical mantle of patients with attention-deficit/hyperactivity disorder. PLoS ONE (2012) 7:e48286. doi: 10.1371/journal.pone.0048286
41. Makris N, Buka SL, Biederman J, Papadimitriou GM, Hodge SM, Valera EM, et al. Attention and executive systems abnormalities in adults with childhood ADHD: A DT-MRI study of connections. Cereb Cortex (2008) 18:1210–20. doi: 10.1093/cercor/bhm156
42. Proal E, Reiss PT, Klein RG, Mannuzza S, Gotimer K, Ramos-Olazagasti MA, et al. Brain gray matter deficits at 33-year follow-up in adults with attention-deficit/hyperactivity disorder established in childhood. Arch Gen Psychiatry (2011) 68:1122–34. doi: 10.1001/archgenpsychiatry.2011.117
43. Wolosin SM, Richardson ME, Hennessey JG, Denckla MB, Mostofsky SH. Abnormal cerebral cortex structure in children with ADHD. Hum Brain Mapp. (2009) 30:175–184. doi: 10.1002/hbm.20496
44. Unterrainer JM, Rauh R, Rahm B, Hardt J, Kaller CP, Klein C, et al. Development of planning in children with high-functioning autism spectrum disorders and/or attention deficit/hyperactivity disorder. Autism Res. (2016). 9:739–51. doi: 10.1002/aur.1574
45. Rühl D, Bölte S, Feineis-Matthews S, Poustka F. Diagnostische beobachtungsskala für autistische störungen. In: von Catherine Lord, Michael Rutter, Pamela C. Dilavore and Susaan Risi, editor. Deutsche Fassung der Autism Diagnostic Oberservation Schedule (ADOS). Bern: Huber (2004).
46. Bölte S, Rühl D, Schmötzer G, Poustka F. Diagnostisches Interview für Autismus – Revidiert. In: Deutsche Fassung des Autism Diagnostic Interview–Revised (ADI-R) von Michael Rutter, Ann Le Couteur und Catherine Lord. Bern: Huber (2005).
47. Achenbach TM, Rescorla LA. Child Behaviour Checklist. In: Manual for the ASEBA school-age forms and profiles. Burlington, VT: University of Vermont: Research Center for Children, Youth and Families (2001).
48. Bölte S, Poustka F. Skala zur Erfassung sozialer Reaktivität – Dimensionale Autismus-Diagnostik. In: von John NC and Gruber CP, editors. Deutsche Fassung der Social Responsiveness Scale (SRS). Bern: Huber (2007).
49. Kaufman J, Birmaher B, Brent D, Rao U, Ryan N. Kiddie-Sas-Present and Lifetime Version (K-SADS-PL) Version 1.0 of October 1996. Berlin; Bern; Köln; Frankfurt; Hamburg; Jena; Mannheim; Rostock: K-SADS-Arbeitsgruppe (2001).
50. Döpfner M, Gört-Dorten A, Lehmkuhl G, Breuer D, Goletz H. Diagnostik-System für psychische Störungen nach ICD-10 und DSM-IV für Kinder und Jugendliche (DISYPS-II, FBB-ADHS). Bern: Huber (2008).
51. Kratzmeier H, Horn R. SPM, Raven Matrizen-Test, Standard Progressive Atrices. Weinheim: Beltz (1979).
53. Fischl B, Salat DH, Busa E, Albert M, Dieterich M, Haselgrove C, et al. Whole brain segmentation: automated labeling of neuroanatomical structures in the human brain. Neuron (2002) 33:341–55. doi: 10.1016/S0896-6273(02)00569-X
54. Fischl B, Dale AM. Measuring the thickness of the human cerebral cortex from magnetic resonance images. Proc Natl Acad Sci USA. (2000) 97:11050–55. doi: 10.1073/pnas.200033797
55. Dale AM, Fischl B, Sereno MI. Cortical surface-based analysis. I. Segmentation and surface reconstruction. NeuroImage (1999) 9:179–94. doi: 10.1006/nimg.1998.0395
56. Desikan RS, Ségonne F, Fischl B, Quinn BT, Dickerson BC, Blacker D, et al. An automated labeling system for subdividing the human cerebral cortex on MRI scans into gyral based regions of interest. NeuroImage (2006) 31:968–80. doi: 10.1016/j.neuroimage.2006.01.021
57. Ghosh SS, Kakunoori S, Augustinack J, Nieto-Castanon A, Kovelman I, Gaab N, et al. Evaluating the validity of volume-based and surface-based brain image registration for developmental cognitive neuroscience studies in children 4-to-11 years of age. NeuroImage (2010) 53:85–93. doi: 10.1016/j.neuroimage.2010.05.075
58. R Core Team. R: A Language And Environment For Statistical Computing. Vienna: R Foundation for Statistical Computing (2016). 59. Konrad K, Eickhoff SB. Is the ADHD brain wired differently? A review on structural and functional connectivity in attention deficit hyperactivity disorder. Hum Brain Mapp. (2010) 31:904–16.
59. Konrad K, Eickhoff SB. Is the ADHD brain wired differently? A review on structural and functional connectivity in attention deficit hyperactivity disorder. Hum Brain Mapp. (2010) 31:904–16. doi: 10.1002/hbm.21058
60. Schilbach L, Eickhoff SB, Rotarska-Jagiela A, Fink GR, Vogeley K. Minds at rest? social cognition as the default mode of cognizing and its putative relationship to the “default system” of the brain. Conscious Cogn. (2008) 17:457–67. doi: 10.1016/j.concog.2008.03.013
61. Castellanos FX, Margulies DS, Kelly C, Uddin LQ, Ghaffari M, Kirsch A, et al. Cingulate-precuneus interactions: a new locus of dysfunction in adult attention-deficit/hyperactivity disorder. Biol Psychiatry (2008) 63:332–337. doi: 10.1016/j.biopsych.2007.06.025
62. Sprengelmeyer R, Rausch M, Eysel UT, Przuntek H. Neural structures associated with recognition of facial expressions of basic emotions. Proc Biol Sci. (1998) 265:1927–31. doi: 10.1098/rspb.1998.0522
63. Volkow ND, Tomasi D, Wang G-J, Fowler JS, Telang F, Goldstein RZ, et al. Positive emotionality is associated with baseline metabolism in orbitofrontal cortex and in regions of the default network. Mol Psychiatry (2011) 16:818–25. doi: 10.1038/mp.2011.30
64. Swick D, Ashley V, Turken AU. Left inferior frontal gyrus is critical for response inhibition. BMC Neurosci. (2008) 9:102. doi: 10.1186/1471-2202-9-102
65. Dickerson Mayes S, Calhoun SL, Mayes RD, Molitoris S. Autism and ADHD: overalpping and discriminating symptoms. Res Autism Spectr Disord. (2012) 6:277–285. doi: 10.1016/j.rasd.2011.05.009
66. Harms MB, Martin A, Wallace GL. Facial emotion recognition in autism spectrum disorders: a review of behavioral and neuroimaging studies. Neuropsychol Rev. (2010) 20:290–322. doi: 10.1007/s11065-010-9138-6
67. Kirchner JC, Hatri A, Heekeren HR, Dziobek I. Autistic symptomatology, face processing abilities, and eye fixation patterns. J Autism Dev Disord. (2011) 41:158–67. doi: 10.1007/s10803-010-1032-9
68. Lozier LM, Vanmeter JW, Marsh AA. Impairments in facial affect recognition associated with autism spectrum disorders: a meta-analysis. Dev Psychopathol. (2014) 26:933–45. doi: 10.1017/S0954579414000479
69. Schaller MU, Rauh R. What difference does it make? implicit, explicit and complex social cognition in autism spectrum disorders. J Autism Dev Disord. (2017) 47:961–79. doi: 10.1007/s10803-016-3008-x
70. Levitin DJ, Menon V. Musical structure is processed in “language” areas of the brain: a possible role for Brodmann Area 47 in temporal coherence. NeuroImage (2003) 20:2142–52. doi: 10.1016/j.neuroimage.2003.08.016
71. Poldrack RA, Wagner AD, Prull MW, Desmond JE, Glover GH, Gabrieli JD. Functional specialization for semantic and phonological processing in the left inferior prefrontal cortex. NeuroImage (1999) 10:15–35. doi: 10.1006/nimg.1999.0441
72. Riedel A, Suh H, Haser V, Hermann I, Ebert D, Riemann D, et al. Freiburg questionnaire of linguistic pragmatics (FQLP): psychometric properties based on a psychiatric sample. BMC Psychiatry (2014) 14:374. doi: 10.1186/s12888-014-0374-9
73. Brothers L. The social brain: a project for integrating primate behavior and neurophysology in a new domain. Cocepts Neurosci. (1990) 1:27–51.
74. Rommelse N, Buitelaar JK, Hartman CA. Structural brain imaging correlates of ASD and ADHD across the lifespan: a hypothesis-generating review on developmental ASD-ADHD subtypes. J Neural Transm. (2017) 124:259–271. doi: 10.1007/s00702-016-1651-1
75. Depue BE, Banich MT, Burgess GC, Bidwell LC, Willcutt EG. Behavioral performance predicts gray matter reductions in the right inferior frontal gyrus in young adults with combined type ADHD. Psychiatry Res. (2010) 182:231–37. doi: 10.1016/j.pscychresns.2010.01.012
76. O'Dwyer L, Tanner C, van Dongen EV, Greven CU, Bralten J, Zwiers MP, et al. Decreased left caudate volume is associated with increased severity of autistic-like symptoms in a cohort of ADHD patients and their unaffected siblings. PloS ONE (2016) 11:e0165620. doi: 10.1371/journal.pone.0165620
77. Richter J, Henze R, Vomstein K, Stieltjes B, Parzer P, Haffner J, et al. Reduced cortical thickness and its association with social reactivity in children with autism spectrum disorder. Psychiatry Res. (2015) 234:15–24. doi: 10.1016/j.pscychresns.2015.06.011
78. Travers BG, Kana RK, Klinger LG, Klein CL, Klinger MR. Motor learning in individuals with autism spectrum disorder: activation in superior parietal lobule related to learning and repetitive behaviors. Autism Res. (2015) 8:38–51. doi: 10.1002/aur.1403
79. Wallace GL, Shaw P, Lee NR, Clasen LS, Raznahan A, Lenroot RK, et al. Distinct cortical correlates of autistic versus antisocial traits in a longitudinal sample of typically developing youth. J Neurosci. (2012) 32:4856–60. doi: 10.1523/JNEUROSCI.6214-11.2012
80. Blanche EI, Reinoso G, Chang MC, Bodison S. Proprioceptive processing difficulties among children with autism spectrum disorders and developmental disabilities. Am J Occup Ther. (2012) 66:621–4. doi: 10.5014/ajot.2012.004234
81. Tomchek SD, Dunn W. Sensory processing in children with and without autism: a comparative study using the short sensory profile. Am J Occup Ther. (2007) 61:190–200. doi: 10.5014/ajot.61.2.190
82. Sowell ER, Thompson PM, Leonard CM, Welcome SE, Kan E, Toga AW. Longitudinal mapping of cortical thickness and brain growth in normal children. J Neurosci. (2004) 24:8223–31. doi: 10.1523/JNEUROSCI.1798-04.2004
83. Yendiki A, Koldewyn K, Kakunoori S, Kanwisher N, Fischl B. Spurious group differences due to head motion in a diffusion MRI study. NeuroImage (2014) 88:79–90. doi: 10.1016/j.neuroimage.2013.11.027
84. Koldewyn K, Yendiki A, Weigelt S, Gweon H, Julian J, Richardson H, et al. Differences in the right inferior longitudinal fasciculus but no general disruption of white matter tracts in children with autism spectrum disorder. Proc Natl Acad Sci USA. (2014) 111:1981–6. doi: 10.1073/pnas.1324037111
85. Chalavi S, Simmons A, Dijkstra H, Barker GJ, Reinders AATS. Quantitative and qualitative assessment of structural magnetic resonance imaging data in a two-center study. BMC Med Imaging (2012) 12:27. doi: 10.1186/1471-2342-12-27
86. Schilling C, Kühn S, Paus T, Romanowski A, Banaschewski T, Barbot A, et al. Cortical thickness of superior frontal cortex predicts impulsiveness and perceptual reasoning in adolescence. Mol Psychiatry (2013) 18:624–30. doi: 10.1038/mp.2012.56
87. Tebartz van Elst L. Das Asperger-Syndrom im Erwachsenenalter und andere Hochfunktionale Autismus-Spektrum Störungen, 2nd ed. Berlin: Medizinisch Wissenschaftliche Verlagsgesellschaft (2016).
88. Nakao T, Radua J, Rubia K, Mataix-Cols D. Gray matter volume abnormalities in ADHD: voxel-based meta-analysis exploring the effects of age and stimulant medication. Am J Psychiatry (2011) 168:1154–63. doi: 10.1176/appi.ajp.2011.11020281
Keywords: autism spectrum disorder (ASD), attention-deficit/hyperactivity disorder (ADHD), FreeSurfer, cortical thickness, mean curvature
Citation: Nickel K, Tebartz van Elst L, Manko J, Unterrainer J, Rauh R, Klein C, Endres D, Kaller CP, Mader I, Riedel A, Biscaldi M and Maier S (2018) Inferior Frontal Gyrus Volume Loss Distinguishes Between Autism and (Comorbid) Attention-Deficit/Hyperactivity Disorder—A FreeSurfer Analysis in Children. Front. Psychiatry 9:521. doi: 10.3389/fpsyt.2018.00521
Received: 04 June 2018; Accepted: 02 October 2018;
Published: 23 October 2018.
Edited by:
Emily L. Casanova, University of South Carolina, United StatesReviewed by:
John Murdoch, Fulgent Genetics, United StatesSheffali Gulati, All India Institute of Medical Sciences, India
Copyright © 2018 Nickel, Tebartz van Elst, Manko, Unterrainer, Rauh, Klein, Endres, Kaller, Mader, Riedel, Biscaldi and Maier. This is an open-access article distributed under the terms of the Creative Commons Attribution License (CC BY). The use, distribution or reproduction in other forums is permitted, provided the original author(s) and the copyright owner(s) are credited and that the original publication in this journal is cited, in accordance with accepted academic practice. No use, distribution or reproduction is permitted which does not comply with these terms.
*Correspondence: Kathrin Nickel, kathrin.nickel@uniklinik-freiburg.de
†These authors share senior authorship