- 1Departments of Radiology, Neurology, and Psychological Sciences, William and Nancy Thompson Endowed Chair in Radiology, University of Missouri, Columbia, MO, United States
- 2Holland Bloorview Kids Rehabilitation Hospital, University of Toronto, Toronto, ON, Canada
- 3Division of Child and Adolescent Psychiatry, Department of Psychiatry and Behavioral Sciences, Stanford University, Stanford, CA, United States
- 4Clinical Research Associates LLC, Simons Foundation, Department of Pediatrics, Yale University School of Medicine, New Haven, CT, United States
- 5Division of Child and Adolescent Psychiatry, Cincinnati Children's Hospital Medical Center, Cincinnati, OH, United States
- 6Department of Psychiatry and Behavioral Neuroscience, University of Cincinnati College of Medicine, Cincinnati, OH, United States
- 7Department of Psychology, John Carroll University, University Heights, OH, United States
- 8Department of Pediatrics, State University of New York Upstate Medical University, Syracuse, NY, United States
- 9Departments of Psychiatry and Pediatrics, New York State Psychiatric Institute, Columbia University, New York, NY, United States
- 10NewYork-Presbyterian Center for Autism and the Developing Brain, New York, NY, United States
Editorial on the Research Topic
Precision medicine approaches for heterogeneous conditions such as autism spectrum disorders (The need for a biomarker exploration phase in clinical trials - Phase 2m)
Significant progress has been made in understanding the biology of autism spectrum disorder (ASD), providing rational hypotheses for interventions to address the core symptoms. However, clinical trials of these interventions have failed to yield positive results to date. In many of these studies, a subset of participants appear to respond well, but a significant benefit is not found in the overall intent-to-treat group. Due to the etiological heterogeneity of ASD, we anticipate that this will continue to be a challenge in future clinical trials. It will be critical to identify the patients that are most likely to respond to a treatment and to target those subjects in later phase trials. We therefore propose the explicit inclusion of “Phase 2m” as part of the pathway of clinical drug development, specifically for the development of a biomarker profile that can be incorporated into later phase 2 and 3 clinical trials. Such a precision medicine approach has the potential to optimize the likelihood of success in future clinical trials to benefit patients.
Introduction
Autism spectrum disorder (ASD) is highly heterogeneous, with estimates of potentially 1,000 genes that may be associated with risk for ASD (1). This is in addition to cases with environmental and other non-genetic contributors such as infection and inflammation during pregnancy (2), as well as cases that would at this point be considered idiopathic cases. Studies using cellular and animal models have pointed to underlying neurobiological systems and pathways impacted by individual ASD risk genes, with some suggestion of convergence across genes. For example, research exploring the synaptic mechanisms impacted by the fragile X syndrome (FXS) gene FMR1 led to trials with negative allosteric modulators of metabotropic glutamate type 5 receptors in FXS (3). Converging lines of evidence (4, 5) led to clinical trials that target glutamatergic and GABAergic functions in ASD; however, these studies have failed to yield positive results for primary outcome measures. The GABA-B receptor agonist arbaclofen, for example, did not show significant benefit on its primary outcome measure in a phase 2 clinical trial for ASD (6). The high degree of heterogeneity within ASD likely contributes to these failures, as a treatment designed to target one biological etiology of ASD may not have a beneficial effect on patients with ASD resulting from perturbations in other biological pathways.
Heterogeneity and biomarkers in ASD
Heterogeneity in ASD can be observed in multiple dimensions, from core symptom pattern to cognitive or communication ability to identifiable risk factors. Genetics has been proposed as a method of subtyping autism (7–17). Rare variants with high penetrance that are directly involved in the etiology of ASD have provided major insights for development of novel therapeutics, while other genes serve as risk factors for ASD that may act in concert with other genetic or environmental risk factors (1, 7–10). However, therapeutics designed to target one specific etiology have an unknown impact on other etiologies of ASD. Additionally, there is a need for greater understanding of pleiotropy within each specific etiology, whereby one might respond to a specific treatment but not another within this group.
In hopes of examining common downstream pathways of the effects of individual etiologies on neural systems, other biomarkers have been assessed in ASD, including markers of brain structure and activity (EEG, imaging) (18–27). A recent study by Ellegood et al. found that 26 different ASD-associated mouse models converged onto three clusters of brain anatomical features from MRI (28). This suggests that neuroimaging may be a powerful tool in the identification of ASD subtypes with specific treatment response, despite genetic heterogeneity; although cost and feasibility issues may limit neuroimaging, particularly in young and more impaired ASD patients. Other types of biomarkers that may be helpful include epigenetic (29, 30), transcriptomic (31–33) (Beversdorf et al.), proteomic (34), and metabolomic markers (35, 36), as well as neurobehavioral measures such as eye-tracking and pupillometry (37–40), actigraphy (41), and psychophysical measures (42). The presence or absence of co-occurring medical (seizures, sleep disturbances, gastrointestinal conditions) and psychiatric conditions (aggression, anxiety, attentional deficits) also contributes to heterogeneity and certainly impacts the approach to treatment.
Heterogeneity is also seen in the core domains of ASD, including social communication and reciprocity deficits, repetitive behaviors/hyperfocused interests, and sensory symptoms. With such disparate symptoms, it may be difficult to formulate ASD severity along a single dimension or to model this unitary diagnosis in epidemiological research or in animal models. The Research Domain Criteria (RDoC) initiative at the National Institute for Mental Health (43) focuses on specific behavioral or cognitive domains within psychiatric or neurodevelopmental diagnoses and may be more tractable for research that spans methods. In support of this, data-driven brain imaging studies have found that brain networks contribute to social communication in a manner that is not diagnosis specific (44). Furthermore, recent studies of the structure of ASD symptoms have suggested four or more distinct social communication dimensions and five separate restricted/repetitive behavior subdomains (45–49). Targeting specific symptom domains would seem advantageous for such a heterogeneous condition as ASD. Recognizing heterogeneity across multiple dimensions, however, it is possible that an intervention may benefit a specific symptom domain in one specific etiology of ASD, with uncertainty about whether this will extend to the broader group of individuals with ASD diagnoses (2).
Within the heterogeneity of ASD, some biomarkers may predict a subpopulation with common disease mechanisms and may therefore be predictive of treatment efficacy. As one of the few examples of the potential utility of biomarkers to dissect heterogeneity within ASD treatment studies, low baseline plasma oxytocin level predicted response to intranasal oxytocin for social responsivity; although this did not replicate in a larger study (50). There are other obvious opportunities to tap into this approach. Alterations in the glutamatergic and GABAergic systems are found with some consistency in ASD postmortem brain studies (51–53), as well as in vivo with magnetic resonance spectroscopy (MRS), albeit with some variability across brain regions (54–56). Some large clinical trials for core symptoms of ASD targeted glutamatergic (memantine) and GABAergic (arbaclofen) systems (6). It is possible that direct or indirect markers of GABAergic or glutamatergic system activity, such as MRS (57) (Nair et al.) or EEG gamma band activity (58), would have been valuable in predicting response in a subgroup of individuals, recognizing that no significant effect was seen in the overall group of participants with ASD. Whole blood serotonin (59, 60) or serotonin receptor binding on positron emission tomography (61–65) could similarly predict responses to treatments targeting serotonergic pathways (66). Psychophysical reactivity indicative of sympathetic/parasympathetic tone (67) could identify subjects that may be more responsive to adrenergic treatments (68). In other cases, we may not have obvious biomarker candidates to parse the heterogeneity in ASD treatment studies.
Additionally, the developmental trajectory must be considered in any approach, as mechanisms of actions that impact the developmental trajectory of neural systems at one stage may have an entirely different relevance at a later stage (69). Among the well-replicated imaging findings in ASD is anatomical overgrowth in the first post-natal years (70–74), and some continue to have larger heads later in life resulting from this (75, 76). It would seem that administration of an agent affecting growth trajectories would have remarkably different effects at different ages. Additionally, the impact of the developmental trajectory is likely critical for a wide variety of other factors as well. Thus, temporal factors must also be accounted for in the heterogeneity of ASD to best facilitate individualized treatment approaches and to move toward personalized medicine in ASD.
Incorporation of a biomarker exploration phase (phase 2m) in clinical trials
The incorporation of rich biobehavioral data to allow subgrouping of participants in clinical trials has the potential to identify which subjects are most likely to respond to a given treatment, and which clinical signs or symptoms are most responsive to that treatment (2, 77, 78). However, the current template of phases for drug development does not regularly incorporate this. In clinical drug development, phase 1 trials are “dose escalation” or “experimental medicine” trials, focused on the safety and tolerability of drugs, and pharmacokinetics and pharmacodynamics are also assessed. These are followed by phase 2 trials, where the findings of the first phase are harnessed for further safety, pharmacokinetic, and pharmacodynamic assessment with optimization of dosing and endpoints to be targeted in subsequent phases. Phase 3 is the confirmatory therapeutic trial, or pivotal trial, conducted in a double blinded manner in a larger population, with statistical power to achieve the predetermined target outcomes based on the phase 2 findings. Successful phase 3 trials are followed by drug approval and marketing, with subsequent phase 4 studies using observational monitoring to evaluate adverse reactions too infrequent to be detected in phase 3, for monitoring clinical efficacy in the broader population, and to assess cost effectiveness (79). Given the heterogeneity in ASD, it is unreasonable to expect any drug to benefit the majority of individuals, but ASD clinical trials have not had sufficient sample sizes to detect improvement in a subset. While the pharmacodynamic aspect of Phase 1 and 2 trials might be used to identify useful biomarkers and precision medicine targets, this has not commonly been the case for autism drug development. Not surprisingly, then, drug development programs in ASD have typically failed in phase 2 or 3.
A strategy, therefore, must be implemented early in the clinical trial pathway (Figure 1), for identifying biomarkers that can facilitate and inform future trials of the drug in development. In light of the failures of recent large ASD trials (5), we propose that early in Phase 2, a study or studies that could be considered as phase 2m (marker exploration phase) should include a rich set of biomarkers that are assessed in a moderately large population of participants to gain an understanding of which subjects respond best, thereby informing the final design and statistical power of later phase 2 and 3 trials. To maximize the richness of the biomarker monitoring, it would be tempting to use a design where all patients will receive the drug, however an open label design is at risk of identifying biomarkers that predict spurious (placebo-related or spurious) response. Blinded crossover designs or staggered start designs might be an appropriate alternative. The participants' developmental stage would also need to be considered as critical marker in this phase. Some markers might be mechanistic, such as biomarkers of GABAergic activity that could predict response to GABAergic agents in ASD. A broader biomarker profiling approach that spans phenotypic subgroups whose mechanistic basis or effects are not fully understood, such as macrocephaly, hyperserotonemia, or elevated IL-6, would better allow the matching of treatments with biomarkers that were not be predicted a priori. Other critical questions that could also be addressed include whether earlier intervention could lead to improvement not only in symptoms at the time of the trial but also an improved developmental trajectory. Thus, age of participation and long-term follow-up may be other crucial components to consider for incorporation in future clinical trials.
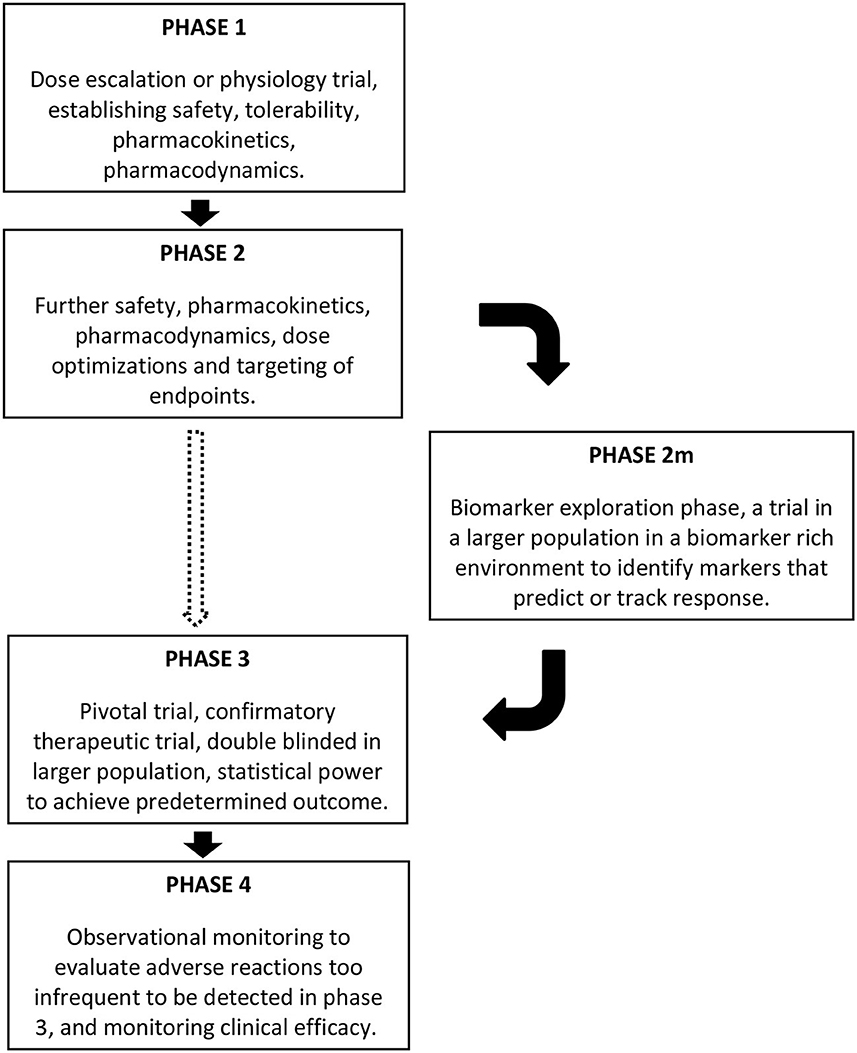
Figure 1. Schematic representing the location of a phase 2m in clinical trials, after phase 1 and phase 2, and before phase 3, if the need for a biomarker exploration phase is warranted based on the nature of the clinical condition. The dashed arrow moving directly from phase 2 to phase 3 might be appropriate for some conditions, but incorporating the phase 2m seems warranted in conditions such as ASD.
Conclusions
With our improving understanding of the genetic and environmental etiologies of ASD and the effects on specific neural systems during distinct developmental epochs, this information can be used for optimization of future clinical trials. By incorporating studies that focus on the predictive value of baseline biomarkers, while also exploring biomarkers that change with treatment and may index response, we can improve the likelihood of success in phase 3 clinical trials. Integrated approaches to better understand the heterogeneity of autism have been initiated by large collaborations that include clinical trials, such as the Autism Innovative Medicines Study–2-Trials (AIMS-2-Trials) (80–82), as well as the Province of Ontario Neurodevelopmental Disorders (POND) Network (83). Additionally, recent work in the Autism Biomarker Consortium for Clinical Trials (ABC-CT) has been developing neurobehavioral markers, including EEG/ERP, in the hope that they can be used to monitor ASD in clinical trials (84–86). This wealth of data may guide the planning for optimal biomarker choices in the phase 2m setting, with mechanistic markers that reflect the function of the neurobiological system(s) targeted by the treatment and other neurobehavioral outcomes that serve as more general indices of ASD symptomatology. Importantly, we will not know which markers will be the best to predict and track response until after the phase 2m is completed. The information yielded by this, though, would likely help contribute to improved outcomes for precision medicine optimization in phase 3—and will result in fewer trials that fail to achieve statistical significance despite having a subset of good responders. Furthermore, intervention with an individualized approach at earlier ages is likely to have a larger effect on developmental trajectories. In combination with impactful behavioral therapies (87–91), this approach, implemented early in development, may have an even greater impact on the overall burden of ASD over a lifetime (2, 92).
Author contributions
DB generated the first draft and every other author made major contributions and agreed on the final text. All authors contributed to the article and approved the submitted version.
Conflict of interest
DB consultant for YAMO Pharma, Impel Pharma, Scioto Biosci, Quadrant Biosci, Stalicla Biosci, and Human Health. EA received consultation fees from F. Hoffmann-La Roche AG Pharmaceuticals, Impel neuropharma, Cell-El Therapeutics Ltd., ONO Pharma USA, Inc.; research funding from Roche, Anavex and Sanofi-Aventis, and SynapDx; in kind supports from AMO pharma and CRA; editorial honoraria from Wiley; book royalties from Springer and APPI, and has served on advisory boards at Roche and Quadrant Biosciences. AH consultant for IAMA Therapeutics, Jazz Pharmaceuticals, and Beaming Health. Has equity options in Quadrant Biosciences and has an investor stake in SCAN-R LLC. PW is an employee of Clinical Research Associates, LLC. CE consultant to Scioto Bioscience, Forge, and Stalicla. Nothing else to report. None of my consulting is related directly to the content of this Frontiers manuscript. TF has received funding or research support from, acted as a consultant to, received travel support from, and/or received a speaker's honorarium from the PTEN Research Foundation, SYNGAP Research Fund, Malan Syndrome Foundation, ADNP Kids Research Foundation, Quadrant Biosciences, Autism Speaks, Impel NeuroPharma, F. Hoffmann-La Roche AG Pharmaceuticals, the Cole Family Research Fund, Simons Foundation, Ingalls Foundation, Forest Laboratories, Ecoeos, IntegraGen, Kugona LLC, Shire Development, Bristol-Myers Squibb, Roche Pharma, MaraBio, National Institutes of Health, and the Brain and Behavior Research Foundation, has equity options in Quadrant Biosciences and MaraBio, and has an investor stake in Autism EYES LLC and iSCAN-R. JV-V received research funding from NIH, Simons Foundation, Roche, Janssen, Novartis, Seaside Therapeutics, Acadia, Yamo, MapLight, and SynapDx. Advisory board or consulting with Simons Foundation, Autism Speaks, Brain Behavior Research Foundation, Roche, and Novartis. Editorial stipend from Wiley.
Publisher's note
All claims expressed in this article are solely those of the authors and do not necessarily represent those of their affiliated organizations, or those of the publisher, the editors and the reviewers. Any product that may be evaluated in this article, or claim that may be made by its manufacturer, is not guaranteed or endorsed by the publisher.
References
1. De Rubeis S, He X, Goldberg AP, Poultney CS, Samocha K, Cicek AE, et al. Synaptic, transcriptional and chromatin genes disrupted in autism. Nature. (2014) 515:209–15. doi: 10.1038/nature13772
2. Beversdorf DQ, Wang P, Barnes G, Weisskopf M, Hardan A, Hu V, et al. Phenotyping, etiological factors, and biomarkers: toward precision medicine in autism spectrum disorder. J Dev Behav Pediatr. (2016) 37:659–73. doi: 10.1097/DBP.0000000000000351
3. Bear MF, Huber KM, Warren ST. The mGluR theory of fragile X mental Retardation. Trends Neurosci. (2004) 27:370–7. doi: 10.1016/j.tins.2004.04.009
4. Chez MG, Burton Q, Dowling T, Chang M, Khanna P, Kramer C. Memantine as adjunctive therapy in children diagnosed with autistic spectrum disorders: an observation of initial clinical response and maintenance tolerability. J Child Neruol. (2007) 22:574–9. doi: 10.1177/0883073807302611
5. Erickson CA, Posey DJ, Stigler KA, Mullett J, Katschke AR, McDougle CJ. A retrospective study of memantine in children and adolescents with pervasive developmental disorders. Psychopharmacol. (2007) 191:141–7. doi: 10.1007/s00213-006-0518-9
6. Veenstra-VanderWeele J, Sikich L, Melmed R, von Hehn JS, Walton-Brown KL, Kuriyama N, et al. Randomized, controlled, phase 2 trial of STX209 for social function in ASD. Int Meet Autism Res. (2013) 13:102.001.
7. Jeste SS, Geschwind DH. Disentangling the heterogeneity of autism spectrum disorder through genetic findings. Nat Rev Neurol. (2014) 10:74–81. doi: 10.1038/nrneurol.2013.278
8. Miles JH. Autism spectrum disorder-a genetics review. Genet Med. (2011) 13:278–94. doi: 10.1097/GIM.0b013e3181ff67ba
9. Miles JH, Takahashi TN, Bagby S, Sahota PK, Vaslow DF, Wang CH, et al. Essential versus complex autism: definition of fundamental prognostic subtypes. Am J Med Genet A. (2005) 135:171–80. doi: 10.1002/ajmg.a.30590
10. Talebizadeh Z, Arking DE, Hu VW. A novel stratification method in linkage studies to address inter- and intra-family heterogeneity in autism. PLoS ONE. (2013) 8:e67569. doi: 10.1371/journal.pone.0067569
11. Ben-David E, Shifman S. Networks of neuronal genes affected by common and rare variants in autism spectrum disorder. PLoS Genet. (2012) 8:e1002556. doi: 10.1371/journal.pgen.1002556
12. Bruining H, Eijkemans MJ, Kas MJ, Curran SR, Vorstman JA, Bolton PF. Behavioral signatures related to genetic disorders in autism. Mol Autism. (2014) 5:11. doi: 10.1186/2040-2392-5-11
13. Piggot J, Shirinyan D, Shemmassian S, Vazirian S, Alarcón M. Neural systems approaches to the neurogenetics of autism spectrum disorders. Neuroscience. (2009) 164:247–56. doi: 10.1016/j.neuroscience.2009.05.054
14. Iossifov I, O'Roak BJ, Sanders SJ, Ronemus M, Krumm N, Levy D, et al. The contribution of de novo coding mutations to autism spectrum disorder. Nature. (2014) 515:216–21. doi: 10.1038/nature13908
15. Liu L, Lei J, Sanders SJ, Willsey AJ, Kou Y, Cicek AE, et al. DAWN: a framework to identify autism genes and subnetworks using gene expression and genetics. Mol Autism. (2014) 5:22. doi: 10.1186/2040-2392-5-22
16. Hu VW. From genes to environment: using integrative genomics to build a ‘systems-level' understanding of autism spectrum disorder. Child Dev. (2013) 84:89–103. doi: 10.1111/j.1467-8624.2012.01759.x
17. Geschwind DH, State MW. Gene hunting in autism spectrum disorder: on the path to precision medicine. Lancet Neurol. (2015) 14:1109–20. doi: 10.1016/S1474-4422(15)00044-7
18. Tebbenkamp ATN, Willsey AJ, State MW, Sestan N. The developmental transcriptome of the human brain: implications for neurodevelopmental disorders. Curr Opin Neurol. (2014) 27:149–56. doi: 10.1097/WCO.0000000000000069
19. Uddin M, Tammimies K, Pellecchia G, Alipanahi B, Hu P, Wang Z, et al. (2014). Brain-expressed exons under purifying selection are enriched for de novo mutations in autism spectrum disorder. Nat Genet. 2014 46: 742–747. doi: 10.1038/ng.2980
20. Vissers ME, Cohen MX, Geurts HM. Brain connectivity and high functioning autism: a promising path of research that needs refined models, methodological convergence, and stronger behavioral links. Neurosci Biobehav Rev. (2012) 36:604–25. doi: 10.1016/j.neubiorev.2011.09.003
21. Just MA, Cherkassky VL, Keller TA, Minshew NJ. Cortical activation and synchronization during sentence comprehension in high-functioning autism: evidence of underconnectivity. Brain. (2004) 127:1811–21. doi: 10.1093/brain/awh199
22. Fishman I, Keown CL, Lincoln AJ, Pineda JA, Müller R-A. Atypical cross talk between mentalizing and mirror neuron networks in autism spectrum disorder. JAMA Psychiatry. (2014) 71:751–60. doi: 10.1001/jamapsychiatry.2014.83
23. Supekar K, Musen M, Menon V. Development of large-scale functional brain networks in children. PLoS Biol. (2009) 7:e1000157. doi: 10.1371/journal.pbio.1000157
24. Narayanan A, White CA, Saklayen S, Scaduto MJ, Carpenter AL, Abduljalil A, et al. Effect of propranolol on functional connectivity in autism spectrum disorder-a pilot study. Brain Imag Behav. (2010) 4:189–97. doi: 10.1007/s11682-010-9098-8
25. Ethridge LE, De Stefano LA, Schmitt LM, Woodruff NE, Brown KL, Tran M, et al. Auditory EEG biomarkers in fragile X syndrome: clinical relevance. Front Integr Neurosci. (2019) 13:60. doi: 10.3389/fnint.2019.00060
26. Neuhaus E, Lowry SJ, Santhosh M, Kresse A, Edwards LA, Keller J, et al. Resting state EEG in youth with ASD: age, sex, and relation to phenotype. J Neurodevel Disord. (2021) 13:33. doi: 10.1186/s11689-021-09390-1
27. Benkarim O, Paquola C, Park B-Y, Hong S-J, Royer J, de Wael RV, et al. Connectivity alterations in autism reflect functional idiosyncrasy. Commun Biol. (2021) 4:1078. doi: 10.1038/s42003-021-02572-6
28. Ellegood J, Anagnostou E, Babineau BA, Crawley JN, Lin L, Genestine M, et al. Clustering autism: using neuroanatomical differences in 26 mouse models to gain insight into their heterogeneity. Mol Psychiatry. (2015) 20:118–25. doi: 10.1038/mp.2014.98
29. Talebizadeh Z, Butler MG, Theodoro MF. Feasibility and relevance of examining lymphoblastoid cell lines to study role of microRNAs in autism. Autism Res. (2008) 1:240–50. doi: 10.1002/aur.33
30. Sarachana T, Zhou R, Chen G, Manji HK, Hu VW. Investigation of post-transcriptional gene regulatory networks associated with autism spectrum disorders by microRNA expression profiling of lymphoblastoid cell lines. Genome Med. (2010) 2:23. doi: 10.1186/gm144
31. Hu VW, Lai Y. Developing a predictive gene classifier for autism spectrum disorders based on differential gene expression profiles of phenotypic subgroups. N A J Med Sci. (2013) 6:107–16. doi: 10.7156/najms.2013.0603107
32. Hicks SD, Rajan AT, Wagner KE, Barns S, Carpenter RL, Middleton FA. Validation of a salivary RNA test for childhood autism spectrum disorder. Front Genet. (2018) 9:534. doi: 10.3389/fgene.2018.00534
33. Pramparo T, Pierce K, Lombardo MV, Barnes CC, Marinero S, Ahrens-Barbeau C, et al. Characteristics and predictive value of blood transcriptome signature in males with autism spectrum disorder. PLoS ONE. (2012) 7:e49475. doi: 10.1371/journal.pone.0049475
34. Szoko N, McShane AJ, Natowicz MR. Proteomic explorations of autism spectrum disorder. Autism Res. (2017) 10:1460–9. doi: 10.1002/aur.1803
35. West PR, Amaral DG, Bais P, Smith AM, Egnash LA, Ross ME, et al. Metabolomics as a tool for discovery of biomarkers of autism spectrum disorder in the blood plasma of children. PLoS ONE. (2014) 9:e112445. doi: 10.1371/journal.pone.0112445
36. Smith AM, Natowicz MR, Braas D, Ludwig MA, Ney DM, Donley ELR, et al. A metabolomics approach to screening for autism risk in the children's autism metabolome project. Autism Res. (2020) 13:1270–85. doi: 10.1002/aur.2330
37. Lynch G. Using pupillometry to assess the atypical pupillary light reflex and LC-NE system in ASD. Behav Sci. (2018) 8:108. doi: 10.3390/bs8110108
38. Cavanagh JF, Wiecki TV, Kochar A, Frank MJ. Eye tracking and pupillometry are indicators of dissociable latent decision processes. J Exp Psychol Gen. (2014) 143:1476–88. doi: 10.1037/a0035813
39. Farzin F, Scaggs F, Hervey C, Berry-Kravis E, Hessl D. Reliability of eye tracking and pupillometry measures in individuals with fragile X syndrome. J Autism Dev Disord. (2011) 41:1515–22. doi: 10.1007/s10803-011-1176-2
40. Daluwatte C, Miles JH, Christ SE, Beversdorf DQ, Takahashi TN, Yao G. Atypical pupillary light reflex and heart rate variability in children with autism spectrum disorder. J Autism Dev Disord. (2013) 43:1910–25. doi: 10.1007/s10803-012-1741-3
41. Frazier TW, Krishna J, Klingemier E, Beukemann M, Nawabit R, Ibrahim S. A randomized, crossover trial of a novel sound-to-sleep mattress technology in children with autism and sleep difficulties. J Clin Sleep Med. (2017) 13:95–104. doi: 10.5664/jcsm.6398
42. Frazier TW, Goodwin MS. Developing more clinically useful biomarkers in autism spectrum disorder. Dev Med Child Neurol. (2020) 62:153. doi: 10.1111/dmcn.14414
43. Cuthbert BN, Insel TR. Toward the future of psychiatric diagnosis: the seven pillars of RDoC. BMC Med. (2013) 11:126. doi: 10.1186/1741-7015-11-126
44. Kushki A, Cardy RE, Panahandeh S, Malihi M, Hammill C, Brian J, et al. Cross-diagnosis structural correlates of autistic-like social communication differences. Cereb Cortex. (2021) 31:5067–76. doi: 10.1093/cercor/bhab142
45. Uljarevic M, Frazier TW, Phillips JM, Jo B, Littlefield S, Hardan AY. Quantifying research domain criteria social communication subconstructs using the social communication questionnaire in youth. J Clin Child Adolesc Psychol. (2020) 50:609–18. doi: 10.1080/15374416.2019.1669156
46. Uljarevic M, Frazier TW, Phillips JM, Jo B, Littlefield S, Hardan AY. Mapping the research domain criteria social processes constructs to the social responsiveness scale. J Am Acad Child Adolesc Psychiatry. (2019) 59:1252–63.e3. doi: 10.1016/j.jaac.2019.07.938
47. Uljarević M., Jo B, Frazier TW, Scahill L, Youngstrom A, Hardan AY. Using the big data approach to clarify the structure of restricted repetitive behaviors across the most commonly used autism spectrum disorder measures. Mol Autism. (2021) 12:39. doi: 10.1186/s13229-021-00419-9
48. Frazier TW, Ratliff KR, Gruber C, Zhang Y, Law PA, Constantino JN. Confirmatory factor analytic structure and measurement invariance of quantitative autistic traits measured by the Social Responsiveness Scale-2. Autism. (2014) 18:31–44. doi: 10.1177/1362361313500382
49. Frazier TW, Hardan AY. Equivalence of symptom dimensions in females and males with autism. Autism. (2017) 21:749–59. doi: 10.1177/1362361316660066
50. Sikich L, Kolevzon A, King BH, McDougle CJ, Sanders KB, Kim S-J, et al. Intranasal oxytocin in children and adolescents with autism spectrum disorder. N Engl J Med. (2021) 385:1462–73. doi: 10.1056/NEJMoa2103583
51. Blatt GJ, Fitzgerald CM, Guptill JT, Booker AB, Kemper TL, Bauman ML. Density and distribution of hippocampal neurotransmitter receptors in autism: an autoradiographic study. J Autism Devel Disord. (2001) 31:537–43. doi: 10.1023/A:1013238809666
52. Yip J, Soghomonian JJ, Blatt GJ. Decreased GAD67 mRNA levels in cerebellar Purkinje cells in autism: pathophysiological implications. Acta Neuropathol. (2007) 113:559–68. doi: 10.1007/s00401-006-0176-3
53. Blatt GJ, Fatemi SH. Alterations in GABAergic biomarkers in the autism brain: research findings and clinical implications. Anat Rec. (2011) 294:1646–52. doi: 10.1002/ar.21252
54. Rojas DC, Singel D, Steinmetz S, Hepburn S, Brown MS. Decreased left perisylvian GABA concentration in children with autism and unaffected siblings. NeuroImage. (2014) 86:28–34. doi: 10.1016/j.neuroimage.2013.01.045
55. Gaetz W, Bloy L, Wang DJ, Port RG, Blaskey L, Levy SE, et al. GABA estimation in the brains of children on the autism spectrum: measurement precision and regional variation. Neuroimage. (2014) 86:1–9. doi: 10.1016/j.neuroimage.2013.05.068
56. Harada M, Taki MM, Nose A, Kubo H, Mori K, Nishitani H, et al. Non-invasive evaluation of the GABAergic/glutamatergic system in autistic patients observed by MEGA-editing proton MR spectroscopy using a clinical 3 Tesla instrument. J Autism Dev Disord. (2011) 41:447–54. doi: 10.1007/s10803-010-1065-0
57. Ajram LA, Horder J, Mendez MA, Galanopoulos A, Brennan LP, Wichers RH, et al. Shifting brain inhibitory balance and connectivity of the prefrontal cortex of adults with autism spectrum disorder. Transl Psychiatry. (2017) 7:e1137. doi: 10.1038/tp.2017.104
58. Rojas DC, Steinmetz S, Hepburn SL, et al. Auditory gamma-band power is related to GABA concentration in autism. Int Meeting Autism Res. (2014) 14: 166.001.
59. Anderson GM, Horne WC, Chatterjee D, Cohen DJ. The hyperserotonemia of autism. Ann N Y Acad Sci. (1990) 600:331–42. doi: 10.1111/j.1749-6632.1990.tb16893.x
60. Cook EH. Autism: review of neurochemical investigation. Synapse. (1990) 6:292–308. doi: 10.1002/syn.890060309
61. Chugani DC, Muzik O, Behen M, Rothermel R, Janisse JJ, Lee J, et al. Developmental changed in brain serotonin synthesis capacity in autistic and nonautistic children. Ann Neurol. (1999) 45:287–95. doi: 10.1002/1531-8249(199903)45:3<287::AID-ANA3>3.0.CO;2-9
62. Murphy DGM, Daly E, Schmitz N, Toal F, Murphy K, Curran S, et al. Cortical serotonin 5-HT 2A receptor binding and social communication in adults with Asperger's syndrome: an in vivo SPECT study. Am J Psychiatry. (2006) 163:934–6. doi: 10.1176/ajp.2006.163.5.934
63. Makkonen I, Riikonen R, Kokki H, Airaksinen MM, Kuikka JT. Serotonin and dopamine transporter binding in children with autism determined by SPECT. Devel Med Child Neurol. (2008) 50:593–7. doi: 10.1111/j.1469-8749.2008.03027.x
64. Goldberg J, Anderson GM, Zwaigenbaum L, Hall GBC, Nahmias C, Thompson A, et al. Cortical serotonin type-2 receptor density in parents of children with autism spectrum disorders. J Autism Devel Disord. (2009) 39:97–104. doi: 10.1007/s10803-008-0604-4
65. Walsh JJ, Llorach P, Pinto DFC, Wenderski W, Christoffel DJ, Salgado JS, et al. Systemic enhancement of serotonin signaling reverses social deficits in multiple mouse models for ASD. Neuropsychopharmacology. (2021) 46:2000–10. doi: 10.1038/s41386-021-01091-6
66. Beversdorf DQ, Nordgren RE, Bonab AA, Fischman AJ, Weise SB, Dougherty DD, et al. 5-HT2 receptor distribution shown by [18F] setoperone PET in high-functioning autistic adults. J Neuropsychiatry Clin Neurosci. (2012) 24:191–7. doi: 10.1176/appi.neuropsych.11080202
67. Toichi M, Kamio Y. Paradoxical autonomic response to mental tasks in autism. J Autism Devel Disord. (2003) 33:417–26. doi: 10.1023/A:1025062812374
68. Zamzow RM, Ferguson BJ, Ragsdale AS, Lewis ML, Beversdorf DQ Effects of acute beta-adrenergic antagonism on verbal problem solving in autism spectrum disorder and exploration of treatment response markers. J Clin Exp Neuropsychol. (2017) 39:596–606. doi: 10.1080/13803395.2016.1252724
69. Thompson BL, Levitt P. The clinical-basic interface in defining pathogenesis in disorders of neurodevelopmental origin. Neuron. (2010) 67:702–12. doi: 10.1016/j.neuron.2010.08.037
70. Courchesne E, Karns CM, Davis HR, Ziccardi R, Carper RA, Tigue ZD, et al. Unusual brain growth patterns in early life in patients with autistic disorder: an MRI study. Neurology. (2001) 57:245–54. doi: 10.1212/WNL.57.2.245
71. Hazlett HC, Poe MD, Gerig G, Styner M, Chappell C, Smith RG, et al. Early brain overgrowth in autism associated with an increase in cortical surface area before age 2 years. Arch Gen Psychiatry. (2011) 68:467–76. doi: 10.1001/archgenpsychiatry.2011.39
72. Nordahl CW, Lange N, Li DD, Barnett LA, Lee A, Buonocore MH, et al. Brain enlargement is associated with regression in preschool-age boys with autism spectrum disorders. Proc Natl Acad Sci USA. (2011) 108:20195–200. doi: 10.1073/pnas.1107560108
73. Shen MD, Nordahl CW, Young GS, Wootton-Gorges SL, Lee A, Liston SE, et al. Early brain enlargement and elevated extra-axial fluid in infants who develop autism spectrum disorder. Brain. (2013) 136(Pt 9):2825–35. doi: 10.1093/brain/awt166
74. Sparks BF, Friedman SD, Shaw DW, Aylward EH, Echelard D, Artru AA, et al. Brain structural abnormalities in young children with autism spectrum disorder. Neurology. (2002) 59:184–92. doi: 10.1212/WNL.59.2.184
75. Lee JK, Andrews DS, Ozonoff S, Solomon M, Rogers S, Amaral DG, et al. Longitudinal evaluation of cerebral growth across childhood in boys and girls with autism spectrum disorder. Biol Psychiatry. (2021) 90:286–94. doi: 10.1016/j.biopsych.2020.10.014
76. Libero LE, Nordahl CW, Li DD, Ferrer E, Rogers SL, Amaral DG. Persistence of megalencephaly in a group of young boys with autism spectrum disorder. Autism Res. (2016) 9:1169–82. doi: 10.1002/aur.1643
77. Bridgemohan C, Cochran DM, Howe YJ, Pawlowski K, Zimmerman AW, Anderson GM, et al. Investigating potential biomarkers in autism spectrum disorder. Front Integr Neurosci. (2019) 13:31. doi: 10.3389/fnint.2019.00031
78. Biomarkers Definitions Working Group. Biomarkers and surrogate endpoints: preferred definitions and conceptual framework. Clin Pharmacol Ther. (2001) 69:89–95. doi: 10.1067/mcp.2001.113989
79. Umscheid CA, Margolis DJ, Grossman CE. Key concepts of clinical trials: a narrative review. Postgrad Med. (2011) 123:194–204. doi: 10.3810/pgm.2011.09.2475
80. Murphy D, Spooren W. EU-AIMS: a boost to autism research. Nat Rev Drug Discov. (2012) 11:815–6. doi: 10.1038/nrd3881
81. Murphy D. Why are there so few effective treatments of autism – and can translational neuroscience help? (keynote address). Int Meet Autism Res. (2014) 14:100.
82. Ghosh A, Michalon A, Lindemann L, Fontoura P, Santarelli L. Drug discovery for autism spectrum disorder: challenges and opportunities. Nature Rev Drug Discov. (2016) 12:777–80. doi: 10.1038/nrd4102
83. Anagnostou E, Lerch JP, Scherer SW, Szatmari P, Nicolson R, Schachar R, et al. Province of Ontario neurodevelopmental disorders network: integrated discovery from genes to treatment. Int Meeting Autism Res. (2015) 15:125–99.
84. Webb SJ, Shic F, Murias M, Sugar CA, Naples AJ, Barney E, et al. Biomarker acquisition and quality control for multi-site studies: autism biomarkers consortium for clinical trials. Front Integr Neurosci. (2020) 13:72. doi: 10.3389/fnint.2019.00071
85. Shic F, Naples AJ, Barney EC, Chang SA, Li B, McAllister T, et al. The autism biomarkers consortium for clinical trials: evaluation of a battery of candidate eye-tracking biomarkers for use in clinical trials. Mol Autism. (2022) 13:15. doi: 10.1186/s13229–021-00482–2.
86. Tsang T, Naples AJ, Barney EC, Xie M, Bernier R, Dawson G, et al. Attention allocation during exploration of visual arrays in ASD: results from the ABC-CT feasibility study. J Autism Devel Disord. (2022). doi: 10.1007/s10803-022-05569-0. [Epub ahead of print].
87. Lovaas OI. Behavioral treatment and normal educational and intellectual functioning in young autistic children. J Consult Clin Psychol. (1987) 55:3–9. doi: 10.1037/0022-006X.55.1.3
88. Eikeseth S, Smith T, Jahr E, Eldevik S. Intensive behavioral treatment at school for 4- to 7-year-old children with autism. Behav Modif. (2002) 26:49–68. doi: 10.1177/0145445502026001004
89. Sallows GO, Graupner TD. Intensive behavioral treatment for children with autism: four-year outcome and predictors. Am J Ment Retard. (2005) 110:417–38. doi: 10.1352/0895-8017(2005)110[417:IBTFCW]2.0.CO;2
90. Cohen H, Amerine-Dickens M, Smith T. Early intensive behavioral treatment: replication of the UCLA model in a community setting. J Dev Behav Pediatr. (2006) 27:145–55. doi: 10.1097/00004703-200604002-00013
91. Eldevik S, Hastings RP, Hughes JC, Jahr E, Eikeseth S, Cross S. Using participant data to extend the evidence base for Intensive Behavioral Intervention for children with autism. Am J Intellect Dev Disabil. (2010) 115:381–405. doi: 10.1352/1944-7558-115.5.381
Keywords: autism (ASD), heterogeneity, treatment, genetics, biomarkers
Citation: Beversdorf DQ, Anagnostou E, Hardan A, Wang P, Erickson CA, Frazier TW and Veenstra-VanderWeele J (2023) Editorial: Precision medicine approaches for heterogeneous conditions such as autism spectrum disorders (The need for a biomarker exploration phase in clinical trials - Phase 2m). Front. Psychiatry 13:1079006. doi: 10.3389/fpsyt.2022.1079006
Received: 24 October 2022; Accepted: 13 December 2022;
Published: 19 January 2023.
Edited and reviewed by: Antonio M. Persico, University of Modena and Reggio Emilia, Italy
Copyright © 2023 Beversdorf, Anagnostou, Hardan, Wang, Erickson, Frazier and Veenstra-VanderWeele. This is an open-access article distributed under the terms of the Creative Commons Attribution License (CC BY). The use, distribution or reproduction in other forums is permitted, provided the original author(s) and the copyright owner(s) are credited and that the original publication in this journal is cited, in accordance with accepted academic practice. No use, distribution or reproduction is permitted which does not comply with these terms.
*Correspondence: David Q. Beversdorf, YmV2ZXJzZG9yZmRAaGVhbHRoLm1pc3NvdXJpLmVkdQ==