- 1Evolutionary Systems Research Group, MTA Centre for Ecological Research, Tihany, Hungary
- 2MTA-ELTE Theoretical Biology and Evolutionary Ecology Research Group, Budapest, Hungary
The emergence of agriculture and complex societies during the Near Eastern Neolithic opened a new era in human evolution. Food production seriously affected the ecological environment, and societies answered this challenge with large-scale division of labor and specialization. In this paper we study this transition with an individual based model. Our model captures the connections between the appearance of agriculture, the social division of labor, and human behavioral diversity. Our two main settings are different habitats: in the pre-Neolithic habitat, resources fluctuated in time and there was no large-scale food storage. In the Neolithic habitat active food production resulted in economic surplus. We consider a sexually reproducing social group, in which individuals solve different tasks for survival. We assume that the task-solving effectiveness has a partial genetic basis but also improves with experience and learning. Since different tasks can require somewhat different skills, we assume trade-offs between genetic propensities for different tasks. Individuals are born with inherited task-choice strategies that they can improve by imitating more successful peers. We show that for the Neolithic case, both phenotypic specialization (task choice strategy) and the emergence of genetic polymorphism are possible, as long as scarcer goods are more valuable. As the number of tasks increases, specialization can evolve only in very large groups. Although phenotypic specialization often emerges in our model, the emergence of genetic polymorphism requires strong assortativity during both imitation and mate choice. In sum, our model shows that if an economic surplus becomes available, behavioral specialization and large-scale division of labor are likely to appear. Thus, our model can help understanding certain aspects of the Neolithic transition, and may have implications for the present genetic polymorphism, too.
1. Introduction
It is common sense that the adoption of agricultural food production and sedentary life changed human societies immensely. This process, called the Neolithic transition, led to larger settlements, changing morals, social stratification, and, later to urbanization and industrialization (Diamond, 1999; Boehm, 2001; Gowdy and Krall, 2013). It is less known that in different regions of the world the Neolithic transition happened independently at very different times (Diamond, 1999). Agriculture first appeared in the Near East, about 10–13 000 years ago, and soon it started its worldwide dispersal (Bar-Yosef, 1998; Shennan et al., 2013; Boivin et al., 2016). However, there are societies that still did not (or did not fully) take up agriculture. These societies, which fall under the categories of small-scale societies, subsistence societies, hunter-gatherers, etc., are fairly good models of pre-agricultural (or pre-Neolithic) human life (Boehm, 2000). In this paper we concentrate on the first transition, and probably the best documented, the Near Eastern Neolithic, hereafter the Neolithic.
Relative to agricultural societies, pre-agricultural societies are thought to have shared several striking features, no strong leadership, no strong hierarchy, no permanent homes and no private property (Boehm, 2001). This paper is concerned with two important outcomes of agriculture that affected human societies: the presence of economic surplus and the emergence of a social division of labor. Social division of labor (SDL) is defined as a permanent division of labor (DL) between individuals that is not linked to reproduction (Vásárhelyi et al., 2015). While DL by gender is present in all subsistence societies (Ember, 1978), permanent DL between people of the same sex and age group is absent from mobile hunter-gatherer groups (Bird-David, 2005; Gurven et al., 2009). Furthermore, it is only partly present in sedentary hunter societies (Boas, 1895; Smith et al., 2003; Alvard and Gillespie, 2004). Nakahashi and Feldman (2014) recently studied the evolution of DL in subsistence societies and shown that DL emerges if both group size and inter-individual differences in resource acquisition are large enough and if there is food sharing within the group (see also in the section Discussion). However, archaeological evidence suggests that SDL, either in the form of specialized individuals representing the first professions, or in the form of a strong hierarchy, only appeared during the Neolithic (Wailes, 1996; Finlayson and Warren, 2010). Here, we are interested in how economic surplus, one of the earliest outcomes of agriculture might have brought about the emergence of SDL, in the form of phenotypic specialization or of genetic polymorphism, or both. To our knowledge, this is the first study to investigate the emergence of human DL within sex and age groups. By modeling the emergence of SDL, we hope to open the discussion on this neglected topic.
Why is DL so interesting? Because it is present at all levels of biological organization, and is usually a sign that cooperation on a lower level has allowed the emergence of a higher level of organization (Maynard Smith and Szathmary, 1997). Therefore, it is interesting that the Neolithic transition brought about a type of DL (that is, SDL) that had been absent before.
It is doubly interesting because no systematic data on permanent SDL exists in non-human animals with advanced cognitive abilities, despite being not just common but even inevitable in post-agricultural humans. Our hypothesis therefore is that the fundamentally different ecological environment of the Neolithic not only set the stage for widespread SDL, but may have left its fingerprint on human genetics as well.
In this paper we describe a model that studies the connections among food production, human social division of labor, and behavioral diversity. We have two main settings representing different social and ecological environments: the ones before and the ones after the adoption of agriculture. We study a social group of individuals who can choose and learn tasks, and who can imitate other, more successful individuals. The behavior of an individual is affected by her genetics, her task choices, and whether she imitates others. This is in agreement with what we know about human personality: it is heritable to some extent with numerous genes, epistatic, and gene-environmental interactions involved, but also strongly affected by the so-called ”non-shared” environment (Turkheimer, 2000; Penke et al., 2007; Plomin, 2011; Penke and Jokela, 2016). Therefore, in the model we presume that behavior includes both a component of genetics and a component of considerable plasticity. To our knowledge, this is the first attempt to model personality in this way, and we aim to answer these three questions:
1) Can economic surplus lead to SDL?
2) What are the necessary conditions for and the constraints on the appearance of SDL?
3) Does SDL induce only phenotypic change, or can induce genetic change too?
In the following we will first describe the model, then present and discuss our results and their consequences.
2. The Model: General Description
First we will give an overview of how the model works and then we will discuss each process in detail.
We consider a sexually reproducing, well-mixed group with group size N. In each time frame that we will call one round, individuals choose between T ≥ 2 different tasks, and they spend their time with that particular task or activity. We can imagine these rounds as days, and tasks as daily activities (see Figure 1), such as hunting or weapon making. Tasks differ in the sense that they require and improve different skills. When someone has spent a round with task j, this will not only gain her a payoff, but also improve her future effectiveness in solving task j. Individuals, denoted by i, increase their expertise in one or more tasks during their lifetime. The more someone chooses a task, the more her expertise in it will grow, but the speed of and ultimate limit on this increase depends on genetic factors (see Figure 2). An individual's genetics, Gi consists of a quantitative genetic background (talent or affinity) for each task. We imagine this talent or affinity to be coded by a large number of genes, similar to how personality or behavioral traits are coded by numerous genes (Penke and Jokela, 2016).
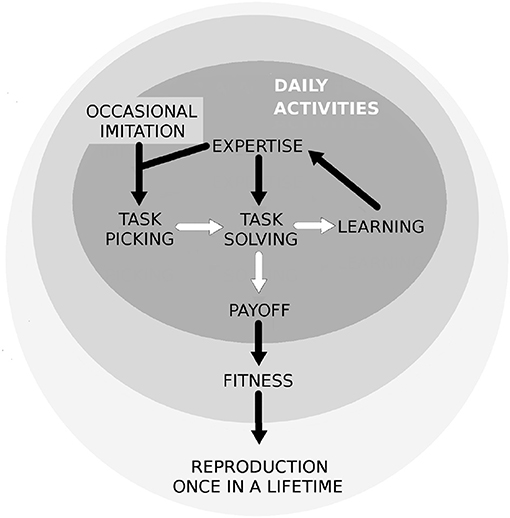
Figure 1. A schematic figure of our model's main events and effects. White arrows represent events of the days. The black arrows represent either consequences or effects. For example, task solving is always followed by learning in the model, and learning enhances one's expertise in the given task, Eij(t). One's expertise then both increases her task solving efficiency, and effects her task picking probabilities.
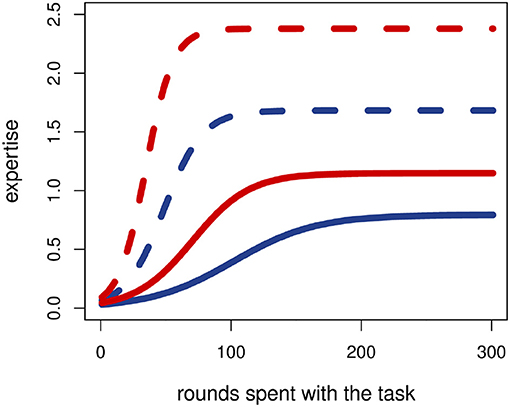
Figure 2. Increasing levels of expertise for different genetic values. A higher genetic affinity grants faster learning and an altogether higher level of expertise (see also Data Sheet 1 in the Supplementary Material). Solid lines are calculated with the simulation's initial genetic values, dashed ones with the corresponding theoretical maximums after complete specialization. In other words, dashed lines represent higher, solid lines lower genetic affinities. The colors denote two main settings: blue lines represent stronger, red ones weaker genetic trade-offs (see also Data Sheet 1 in the Supplementary Material and the main text).
At the end of each round, payoffs are assigned to individuals according to their expertise in that round's task. Each individual's aim is to maximize her fitness throughout her life, and fitness is a function of these payoffs. Occasionally, individuals have a choice to update their task choice strategy by imitating others, that is, by socially learning from others. The purpose of such imitation is to copy successful strategies, but we assume that one is only willing to copy a strategy that is not too different from one's own. The reason for this is that the expected payoff of a complete change of task choice strategy is rarely more beneficial than the cost of the change, and we assume that individuals know this. At the end of a generation, which includes a number of imitation phases, individuals are paired up and sexual reproduction takes place. Men and women are not differentiated in the model. The number of surviving offspring per couple is proportional to the average parental fitness. Offspring inherit genetics and task choice strategies from both parents.
In the following we discuss the above processes in more detail. For further details see Data Sheet 1 in the Supplementary Material. For readers less interested in the mathematical detail, we suggest reading Figure 1.
2.1. Task Choice, Expertise, Genetics, and Payoff
In each round, denoted by t, every member of the group chooses a task to spend her time on. Individual i chooses task j with probability pij, where . Offspring inherit pij values from their parents, but these can change via imitation (see later). After individual i has solved task j, that is, at the end of the round, i's payoff increases as follows:
where Bij(.) is i's net benefit from task j until round (t+1). Eij(t) denotes individual i's expertise in task j in round t, and β is a constant that converts expertise into benefit. In real life, the exact value of the payoff would scatter around an expected value, but for simplicity, we use the expected value. Per-round, per-task payoffs are summed throughout an individual's lifetime, and at certain times fitness is calculated from these sums (see below).
Expertise is a sigmoid function (Leibowitz et al., 2010) of both the time spent with a task and the genetic affinity (Gij) assigned to it (see Figure 2). So the genetic background plays an important role in how one can improve in one task or another. Since different tasks can demand different skills, as we will discuss later, we assume a trade-off between talents for different tasks. This trade-off represents the idea that if someone is talented in a task, she will be relatively less talented in another task needing different skills, just as how people with different vocational interests tend to have unlike abilities (Randahl, 1991). The parameter α determines the strength of the trade off (for details, see Data Sheet 1 in the Supplementary Material), so that if the trade-off is stronger (α < 1), then a greater talent for one task necessarily means a much smaller for another. If the trade-off is weaker (α > 1), then it is less costly for someone to have affinity for more than one task (Levins, 1968).
2.2. Fitness
In the Introduction we proposed to show basic differences between two kinds of social and ecological settings, the ones before and after agriculture appeared. For this purpose we have examined the above model framework with two different fitness calculating procedures. The two different settings we will call the pre-Neolithic or subsistence, and the Neolithic or producer case. In the subsistence setting, individuals are better off when they have some expertise in all activities, while in the producer setting having a general knowledge or experience is no longer a necessity (for details see the respective sections below).
2.2.1. The Subsistence Case
In the subsistence case, we attempt to model societies that are similar to contemporary mobile hunter-gatherers. Most of these people, especially in warmer environments, move their camps several times a year, do not have the means for storing large amounts of food or other resources for long term (Binford, 1980), and live in an environment where limiting resources fluctuate strongly, so every individual has to acquire different resources at different times. In other words, the ecological circumstances force people to gain expertise in all possible tasks or activities. Otherwise there would likely be at least one time in their lives when the lack of a particular knowledge and experience reduces their fitness seriously. To ensure that individuals who lack at least one kind of experience will never be successful, we calculate the subsistence fitness as follows:
That is, we calculate the fitness as the product of the net benefit assigned to the different tasks (Sæther and Engen, 2015). Equation (1) has a maximum where Bi1 = Bi2 =.. = BiT. This will be attained most easily by a generalist individual who has no outstanding expertise in any one task. We note here that since we are only interested in SDL, our model does not include gender-specific traits with their corresponding genetic differences and life histories. Obviously, some sexual DL exists in all human societies, but it is not the focus of this study.
2.2.2. The Producer Case
In the producer case, we model early agricultural societies. Here we assume that economic surplus leads to large-scale food storage, frequent trade, or most likely, both (Bar-Yosef, 2001). Producer individuals can be more successful if they specialize on one activity throughout their whole life, because they have a better chance of becoming experts in that task. We also assume that rare specialists are better off than frequent ones, in other words, that success is negatively frequency dependent. For this, we define a fitness coefficient that is a linear function of the focal phenotype's frequency in the group.
We calculate the specialist's fitness as:
where fj(t)∈[0;1] is the frequency with which task j was chosen till round t, and is a constant that scales the strength of frequency dependence. The negative frequency dependence is maximal if δ = 0; there is no frequency dependence for .
2.3. Imitation
In an individual's lifetime there are several phases of imitation, a form of social learning. Imitation is assortative, which means that individuals are more likely to copy others who are similar to themselves regarding their task choice strategies. Again, similarity here does not refer to gender-specific traits, but other behavioral traits. In a given pair of individuals, the one with the higher fitness has a higher probability of being the model of imitation than the one with the lower fitness (for the exact probabilities see Data Sheet 1 in the Supplementary Material). If assortativity is strong, individuals are prevented in two different ways from copying strategies that are far from their own. First, one will rarely switch to a task if she has no prior experience in it. Thus, individuals can avoid decisions where the benefits are far below the costs. Second, when one individual copies another, she will get a mixture of the model's strategy and her own interest. An individual is involved on average in five imitation events during her lifetime, so potentially she can end up with quite a different task choice strategy from the one she had inherited.
2.4. Reproduction
At the end of a generation's time, reproduction takes place. Individuals reproduce sexually, which means the recombination of traits. Similar to imitation, reproduction can also be assortative with regard to task choice strategies, but not success or fitness. There is evidence that people tend to prefer and pair with individuals similar to themselves, and that similarity between mates is associated with a higher reproductive success (Huber and Fieder, 2011; Krzyzanowska and Mascie-Taylor, 2014; Conley et al., 2016).
The number of offspring a pair produces is proportional to the average relative parental fitness. An offspring inherits its genetics from both parents (see Data Sheet 1 in the Supplementary Material). The offspring's task choice strategy is simply determined by the average parental probabilities.
2.5. Simulation Settings and Questions
During the basic simulations we use a social group of 100 individuals. We initialize the group in a way that i everybody has equal talent for all activities. Importantly, this does not mean that everybody has the exact same genetics, only that they have identical quantitative traits. Furthermore, everybody has the same task choice strategy, . Then we run the simulations for 103 generations. Each generation lives for 103 rounds, during which 10 imitation phases take place with N/4 imitation events each. At the end of a generation's time, we record the Gij, pij, and the values in the group.
3. Results
3.1. Results With Two Tasks
We have picked a basic parameter set with which we can demonstrate the main results and the differences between the subsistence and producer cases. We tried to set these parameters as realistically as possible while also producing obvious and illustrative results. In the following we will only note parameter values that are different from the basic values listed in Table 1. Our primary question is whether the optimal behaviors in the two ecological settings (pre-Neolithic and Neolithic) are different. We also ask whether the behaviors have an effect on the genetics, and whether the strength of the genetic trade-off alters these results.
It can be seen on Figures 3I, II that with this basic parameter set our two settings show striking differences, and the results are not affected qualitatively by the strength of the trade-off within this range.
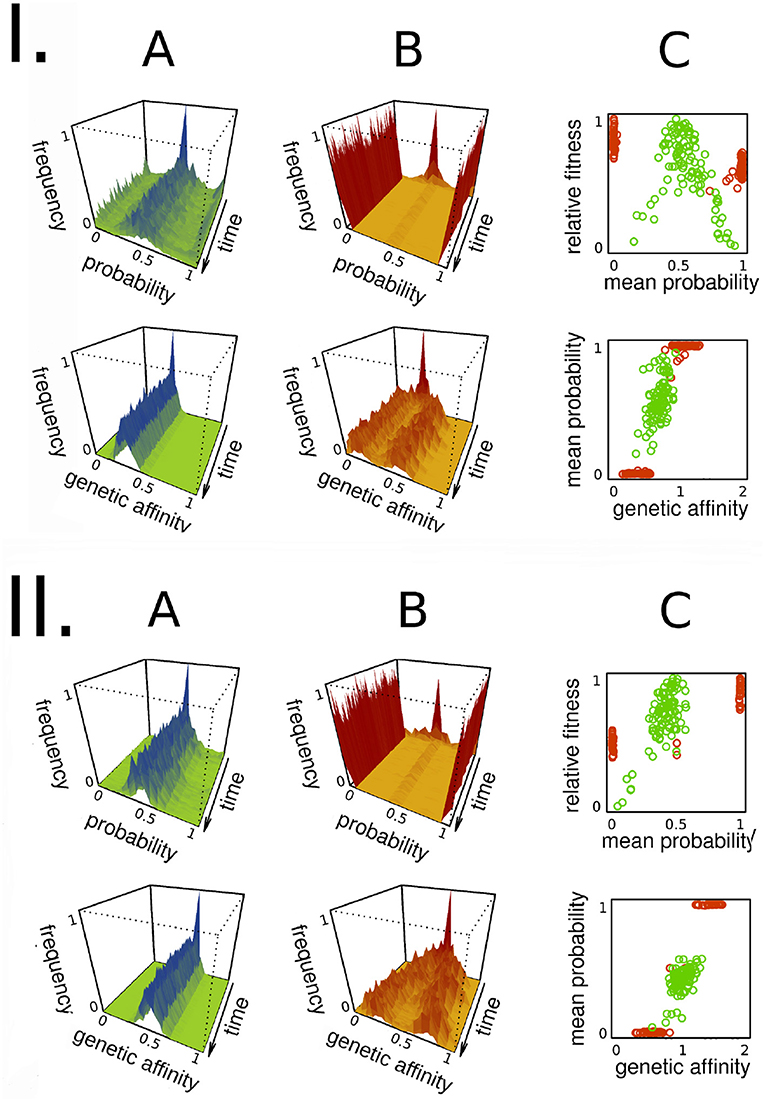
Figure 3. Summary of the basic results in both settings with stronger (I) and weaker (II) trade-offs. The figure shows the task choice probabilities (top row) and the distribution of the genetics (bottom row) for one task throughout all generations. The top right plots show the fitness of individuals as a function of their average life-long task choice probabilities. The bottom right plots show these average probabilities as function of the genetic affinity assigned to the same task. The greenish colors (A columns) represent the subsistence, the reddish (B columns) the producer case. For the parameters see Table 1.
In the subsistence case, we can see that group members are generalists both on the phenotypic and the genetic levels (see Figures 3IA, IIA). This is so for both weaker and stronger trade-offs, although there are more specialists in the group when the trade-off is stronger. Specialists have a very low fitness compared to generalists (see the top right plots on Figures 3I,II). The appearance of specialists is not a product of selection but an epiphenomenon of the trade-off and the process of imitation. When the trade-off is stronger, the sum of the genetic affinities of a non-specialist individual is lower (see Data Sheet 1 in the Supplementary Material). Therefore it is more difficult to become an expert in multiple tasks. Meanwhile, imitation is strongly assortative, and therefore a couple of specializing individuals are more likely to imitate each other and get even more specialized. Note that the individual who is the model of imitation does not need an absolute high fitness; it is enough if she is fitter than the imitating one. Furthermore, though most individuals are generalists, there is still a positive correlation between the genetic affinity and the probability of performing the same task (see the bottom right plots on Figures 3I, 3II).
In the producer case we see specialization on the phenotypic level and polymorphism on the genetic level (see Figures 3 IB, IIB). While on the phenotypic level everyone is completely specialized to one task, on the genetic this is not so. Nonetheless, there is still a strong correlation between genetic affinity and the probability of performing the same task (see the bottom right plots on Figures 3I, II). Note also that the two groups of specialists have different mean fitness values (see the top right plots of Figures 3I,II). This temporary difference is caused by stochastic fluctuations and frequency dependence, and disappears on the long run. It is important to note here that all of the plots on Figure 3 capture phenotypic and genetic values of one task. As the same plots look quite similar for the other task, we decided not to include them.
As a group size of N = 100 is not realistic as an effective population size (see below), we have also tested the model for a much larger group size, N = 1, 000. These latter results look quite similar to the basic ones; the effect of enlarging the group is only to lessen noise in the distribution of traits (see Figure S1). Therefore, in the following we use the N = 100 case for most model checks and assume that this focal group is part of a larger population.
For our results on the strength of assortativity, the speed of learning, the role of the frequency dependence and the mechanisms generating the variance, see Data Sheet 1 in the Supplementary Material and Figure S2 in Presentation 1 in the Supplementary Material.
3.2. Results With More Than Two Tasks
In the next section, because of technical reasons we will not attempt to compare results with previous ones explicitly (see Data Sheet 1 in the Supplementary Material), but only give an overview of the trends we saw.
First we introduced a third task into the model and did not change any other parameter. When the trade-off was stronger, in the producer case one task disappeared, and then individuals specialized to one of the two remaining tasks, similar to the T = 2 case (see Figure 4A). In the subsistence case, we also saw specialization, but only on the phenotypic level, and consequently, in this group everyone had very low fitness. When the trade-off was weaker, the results were similar except with the difference that in approximately half of the cases no groups of specialist disappeared in the producer case (see Figure 4B). Also, in the subsistence case there were a couple of individuals who were able to stay generalists. The latter had very high fitness compared to others, so the next generation practically contained only their offspring.
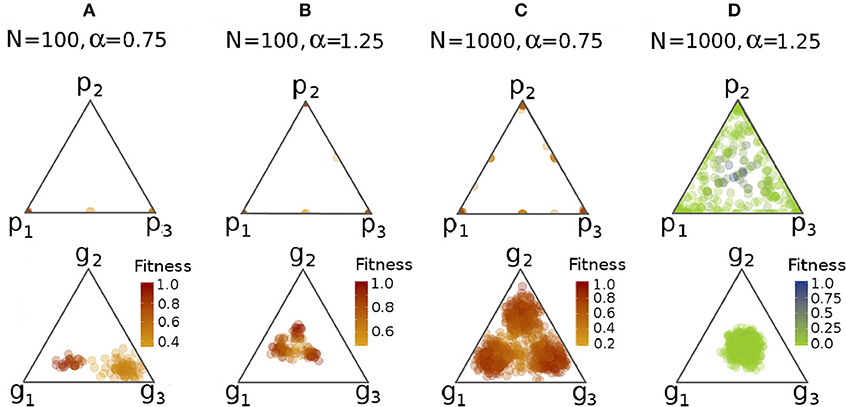
Figure 4. Phenotypic and genetic profiles in the T = 3 case with varying trade-off and group size. In the top row simplexes capture the probability distribution between the three tasks, in the bottom row the relative genetic value distribution between the three tasks. Each point corresponds to an individual, and plots contain all group members. Panels A and B represent smaller groups with stronger (A) and weaker (B) trade-offs, while panels C and D represent larger groups with stronger (C) and weaker (D) trade-offs. Panel D is the only one depicting the subsistence case. Reddish colors represent the producer, greenish the subsistence case. For further parameters, see Table 1.
Because of the previously mentioned demographic stochasticity, we have studied the problem of specialization with larger group sizes, and in a meta-population structure. Also, we studied the subsistence case with increased learning speed. To summarize the results, when there are three tasks that are moderately slow to learn, a small group of 100 is not big enough for complete and stable specialization. Also, the cost of learning three tasks instead of two drives the subsistence group into specialization and to a very low fitness state, unless the speed of learning increases too. For further details, see Figures 4C,D, and Figure S3 in Presentation 1 in the Supplementary Material.
After studying the model with three tasks, we introduced a fourth one. In this case, an even larger group size is needed for coexistence of four subgroups of different specialists. We have studied the N = 500, N = 1, 000, and N = 2, 500 cases, and the following results qualitatively hold for N ≥ 1, 000. As shown in Figure 5, phenotypic specialization is almost complete, and there is a clear direction toward genetic polymorphism with a weaker trade-off, even if the specialist groups are less distinct than in the previous cases. However, when the trade-off is stronger (α = 0.75), specialization is not stable, one task typically disappears, similar to the T = 3 case (see Figure S4). In the subsistence case, we have a similar situation as before. When the trade-off is stronger, it is far too difficult to remain generalist, and therefore only a very small number of individuals end up with high fitness (see Figure S4). However, when the trade-off is weaker, there is a large number of generalists in the group (see Figure 5).
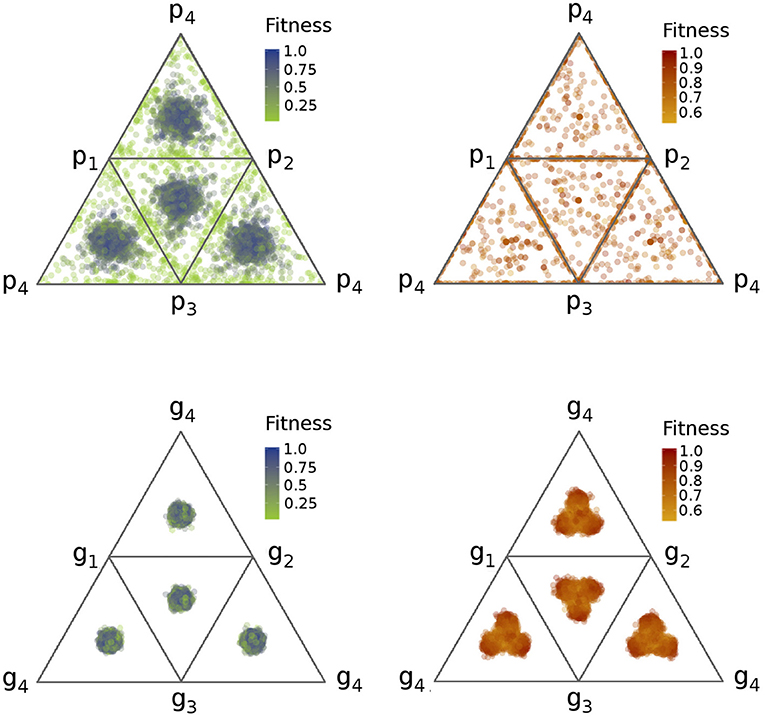
Figure 5. Phenotypic (top) and genetic (bottom) profiles in the T = 4 case with weaker trade-off (α = 1.25). The figure captures tetrahedron nets, where each triangle is a simplex calculated from the three noted tasks, similarly to the simplexes on Figure 4. Thus, the faces of the tetrahedrons show projections of the 3D mass of points. Each point corresponds to an individual, and plots contain all group members. Reddish colors represent the producer, greenish the subsistence case. For further parameters, see Table 1.
To summarize, as we increase the number of tasks, it is more and more difficult to become a pure specialist in the producer case, or to remain truly generalist in the subsistence case. The more tasks are available, the larger the group size needed for stable specialization. The reason for this is that if there are more tasks in a small group, stochastic effects are stronger, reducing the strength of selection. Due to the different ecological settings, a weaker selection results in different patterns in the two environments. Note however that the two settings are quite different from each other qualitatively.
When there are several tasks, our original assumption that all tasks are completely independent in their genetic background seems overly strong, so we designed a further variant of the model with overlapping genetics. In this version of the model, we assumed that while the four tasks need four different kinds of expertise, they have only two underlying quantitative genetic backgrounds. This way, even if someone is born with specialist genetics, she still has two tasks to choose from. To summarize these results, it is easier for phenotypic specialization and genetic polymorphism to arise, but a large group size of 2,500 is needed (see also Figures S5 and S6 in Presentation 1 in the Supplementary Material). Higher numbers of tasks probably require even larger group size for specialization.
4. Discussion
In this paper we have studied a so-far neglected aspect of the Neolithic transition, the emergence of social division of labor. Given their ecological differences, our model shows that it is not surprising that SDL is widespread in producer groups, despite rarely present in subsistence groups.
In the subsistence groups, individuals typically stay generalists, that is, neither phenotypic specialization, nor genetic polymorphism emerges, if groups are large enough and tasks are sufficiently easy to learn (see Figures 3–5). In the poducer case, the typical result is the opposit; individuals get completely specialised phenotypically in one of the tasks, and a corresponding genetic polymorphism arises, too (see Figures 3–5). However, if there are more than two tasks, specialists for all tasks only appear if the group size is larger (see Figure 4).
Our model not only studies the emergence of SDL in two different ecological settings, but incorporates both phenotypic and underlying genetic aspects of proficiency. However, this complexity presents difficulties and limitations, too. In the following we will discuss the detailed results and implications of the subsistence and producer settings, and the limitation of our study separately.
4.1. The Subsistence Case
Our subsistence case is intended to mimic a mobile hunter-gatherer group. Individuals have a need to gain experience in all tasks, just as hunter-gatherers have to participate in all group activities (Boehm, 2001; Marlowe, 2010). We have not included all possible fitness affecting factors into the model though. For example, while food sharing decreases payoff differences by helping the less successful (Kaplan et al., 1984; Marlowe, 2010), reputation gains from doing so can increase fitness differences (Kaplan and Hill, 1985) thus counteracting the effect of food sharing. We decided that instead of further increasing model complexity, we let the fitness function capture these effects. We also did not incorporate external determinants of behavior into the model. There are numerous narratives about how hunter-gatherers shape each other's behavior by means of teasing, mocking, or in extreme cases, ostracising (Boehm, 2001). This culturally transmitted system of social pressure can help younger individuals to find the “optimal" behavior in a given social and ecological environment. In the context of this model, such a mechanism could help individuals in the subsistence group to avoid the positive feedback loop of specialization.
In our model there are two conditions that are necessary for the subsistence group to remain generalist: learning a task has to be relatively fast, and there has to be at least one moderately large variance-generating mechanism, either during reproduction, imitation, or both. If there are more than two tasks and learning a task is not easy, members of the subsistence group specialize to certain tasks and have very low fitness. The reason for this is combinatorial: with a linearly increasing number of tasks the probability of choosing all of them equally often decreases not linearly, but more quickly. Therefore, chance alone leads generalist individuals to preferences, and initial preferences easily drive these individuals into specialization. Despite this, if the task learning speed increases with the number of tasks, the subsistence group can still remain generalist. However, it is not clear that task learning can be a function of the number of tasks one learns. One argument could be that learning one task makes the next one easier to learn. For example, some studies find that children with music training outperform their peers in one or more other disciplines (dos Santos-Luiz et al., 2016). However, there can be an argument against this: if tasks are sufficiently different, there could be a task switching cost (Goldsby et al., 2012), or simply different tasks could need mutually exclusive skills. Therefore, we can conclude that according to our model, remaining generalist is only possible if learning is fast or if the tasks need overlapping skills. For example, mobile hunter-gatherers are truly generalist; they participate in all tasks, such as hunting, gathering, weapon making, and collective decision making (Boehm, 2001; Marlowe, 2010), but these tasks may share the need for several skills, such as hand-eye coordination and patience. But in other small-scale societies, weak or temporary SDL does seem to appear when new skills are needed. One example could be the specialization in weapons or foraging techniques when opportunities are more varied (Dwyer and Minnegal, 1991). Another example is temporary DL during the season of agricultural work (Stieglitz et al., 2013).
A further consequence of the above is that it may provide insight into the so-called Sapient Paradox, which is the question of why behaviorally (and probably genetically) modern humans had no technological revolution between the Upper Palaeolithic and the Neolithic (Renfrew, 2008). Homo sapiens went through several revolutionary changes during its evolution, but between its cognitive explosion about 40,000 years ago and the appearance of agriculture, surprisingly few technological novelties appear in the fossile record (Renfrew, 2008). According to our model, until the ecological environment allowed large groups, and thus division of labor, behavioral diversity must have been lower and innovation scarcer. Interestingly, the oldest European remains of representational art (e.g., the Altamira cave of Lascaux) are relatively close to the sea, which serves as a more reliable source of food than do terrestrial habitats. Indeed, contemporary marine hunter societies have both some SDL and specialization (Smith et al., 2003; Alvard and Gillespie, 2004), suggesting that marine hunting is somewhere between mobile hunter-gatherer and producer ecologies.
4.2. The Producer Case
Our basic parameter set enables the producer group to separate into specialized subgroups that engage in SDL. However, not every parameter set is ideal for such specialization, especially not for genetic branching. One absolute requirement for parallel phenotypic specialization and genetic polymorphism is strong assortativity during imitation and reproduction (see Table 1 in Data Sheet 1 in the Supplementary Material). If only one of these events is strongly assortative, phenotypic specialization is still present, but genetic branching is absent. Another requirement for genetic branching is a big enough group or population size. This is not surprising: if the group is small and not connected to other groups, each specialized subgroup is in danger of disappearing due to stochastic events. For example, in the case of three tasks and a small group, one task disappears in the producer case, but not in the subsistence case, while both group's average fitness is relatively low. Interestingly, in a small group the only chance for solving all difficult tasks is to value generalist tendencies even if eventually everybody ends up being a specialist. (If tasks are faster to learn, the case is similar, only the subsistence group ends up consisting of generalists and having a higher average fitness.) A final requirement is a large enough variance when inheriting genetics. This is in agreement with the previous point, too, as the equilibrium variance a population can reach depends on the size of the population itself. To summarize, as the number of tasks grows, an ever larger group or a well-connected meta-population is needed for maintaining specialist groups, and the minimum size of the group increases very fast with the number of tasks. This pattern corresponds to the general finding that in several animal societies DL is more pronounced in larger groups (Cartwright, 2003; Thomas and Elgar, 2003; Jeanson et al., 2007). It is also in agreement with the somewhat connected observation that larger group or population sizes contribute to larger cultural complexity (Henrich, 2004; Derex et al., 2013).
Naturally, if tasks have overlapping genetics, the problem of specialization is simplified back to one with only two or three tasks. In these cases, a medium size group is big enough for several groups of genetic and phenotypic specialist to coexist. Again, this assumption that different task types share the necessary genetic background is not far-fetched since those who share a genetic background will sometimes choose the same professions, and often similar ones. Indeed, several twin studies have shown that the variation in vocational interest is significantly influenced both by genetic and environmental factors (Schermer and Vernon, 2008), similar to personality.
This model setting with the overlapping genetics seems more realistic than the ones without it, albeit still too simplified. We intend to investigate a model where the amount of genetic overlap behind tasks is also a variable parameter.
4.3. The Limitations of Our Study
Our model examines a problem that has to date been rarely explored in the literature, but it still has several limitations. Some limitations arise from decisions that render the model more tractable. These include non-overlapping generations and a stable group size, neither of which applies to humans. However, these restrictions or simplifications probably do not alter the results qualitatively.
Another simplification is that we only focus on one social group of N = 100 individuals, which is the cultural unit, and we assume it to be part of a larger reproductive pool, a metapopulation. Thus, we essentially assume that the genetic variance is larger than it would be if the effective population size were really N = 100. We have shown that this assumption does not alter most results qualitatively, but a reasonable extension would be to generalize our model with an extended metapopulation structure, similar to the one we have discussed before. In such a model, it would also be possible to evaluate the role of competition among groups, that is, the role of multilevel selection in the evolution of SDL.
Finally, as we have already mentioned before, this simple model did not contain some important elements of the typical subsistence life, such as sexual DL, food sharing, reputation-based mate choice, or social pressure. As for sexual DL, it is a consequence of the different life history of the sexes, which was not in our focus. As for the others, we believe that our fitness calculating procedure roughly captured most of their main effects.
4.4. The Neolithic Transition and Human Behavior
During the Near Eastern Epipalaeolithic and early Neolithic, climatic fluctuations became larger, human density grew, and populations of megafauna became scarcer (Diamond, 1999; Bar-Yosef, 2001; Simmons, 2012). At the same time, human groups gradually changed their subsistence from mobile hunting and gathering toward sedentary food production (Maher et al., 2012; Willcox, 2012). Agriculture first appeared at the Fertile Crescent about 10–13 000 years ago (Willcox, 2012). Then, within a couple of thousand years, the first professions, the first stratified complex societies, and symbolic writing appeared (Wailes, 1996; Gowdy and Krall, 2013). Interestingly, this whole line of changes originated in the transition from mobile hunting and gathering to sedentary food producing. Our model shows how the economic surplus generated by food production may have set the stage for SDL and specialization. Our study may or may not apply to other Neolithic transitions, as these can vary greatly according to the different ecological circumstances.
Palaeolithic humans lived in small mobile groups, presumably had no hierarchy, no food storage, and no SDL (Boehm, 2000; Simmons, 2012; Gowdy and Krall, 2013). In contemporary small-scale societies, people are usually skilled in all subsistence-related activities and use varying skills throughout a year. Everyone goes foraging, and large proportions of food are shared extensively (Kaplan et al., 1984; Marlowe, 2010). In these societies, fitness is related both to foraging success and food sharing (Kaplan and Hill, 1985; Boehm, 2001; Gurven and von Rueden, 2006). According to our model, in such a group, successful individuals are generalists, and therefore selection creates a unimodal, symmetric distribution of the group's genetic affinities (see Figure 3). If there is a genetic trade-off, too, an extreme talent will impose a cost on its owner.
However, economic surplus creates a chance to engage in SDL. We believe that this is what happened during the Neolithic. If there is no pressure on individuals to learn all possible skills, they will become more expert in tasks they have more talent for. Thus, the cost/benefit ratio of task solving will decrease, and SDL will soon appear at the group level. SDL will lead to an increasing behavioral diversity, if specialized individuals differ from each other a great deal. Furthermore, if SDL and frequency dependence is present, selection will favor a multimodal distribution of the group's genetic affinities (see Figure 3). Thus, SDL can also lead to a greater genetic diversity on the group level, and the more collective tasks a society solves by SDL, the more diverse it will get both behaviorally and genetically. However, while phenotypic diversity appears very easily, genetic diversification needs special circumstances.
As we have mentioned in the Introduction, Nakahashi and Feldman (2014) studied a similar question to ours. They explored the conditions for DL to emerge if there are two types of resources that require different skills in a group living society with food sharing. For the sake of analytic tractability, they assumed asexual reproduction and individual learning. They have found that in certain circumstances, permanent DL can emerge in a typical subsistence society. However, this is not in agreement with either the archaeological or the anthropological record. As the only type of permanent DL observed in most typical mobile small-scale societies is between the sexes, we interpret their model capturing the emergence of gender-based DL. In contrast, our model captures the effect of the Neolithic transition on SDL, as SDL seems only to have appeared with or following food production. Applying an individual based model, we incorporated sexual reproduction with recombination of parental traits and social learning (imitation). We also make a distinction between the phenotypic and genetic characteristics of individuals. Finally, we extended our model to cases with more than two tasks. Interestingly, despite all the differences, the two models find results in agreement with each other: a larger group size and an increased importance of learning a skill facilitates the emergence of DL, and thus seem to be crucial and robust requirements.
The above argument has implications not only for the Neolithic transition, but also for the more recent evolution of humans. In our model, the size of the group represents a major limit for genetic diversification. We have chosen a small basic group size that corresponds to the natural human social group size of about 150 people (Dunbar, 1993). Presumably, Palaeolithic groups had sizes in this order of magnitude, where specialization would indeed have been problematic. However, during and since the Neolithic, human groups have been getting larger and larger, thus we posit that group size is no longer a constraint on diversification. Moreover, with the IT revolution, group size during imitation or social learning is effectively equal to the online global population. Now that people's connectedness has grown so drastically, SDL has became global, and specialization can be ever more extreme. Thus, in the near future we may experience the greatest growth in behavioral and genetic diversity ever seen in human history.
Conclusions
In this paper we were interested in whether the emergence of agriculture was able to trigger SDL and behavioral diversification in human populations. To study this question we created a strategic model, in which individuals had to solve different tasks for survival. We compared groups in two different ecological environments, one resembling small-scale societies, the other resembling food-producers.
In the subsistence case, individuals typically stay generalists. The exception is when tasks are difficult to learn, in which case phenotypic specialization can arise, but genetic polymorphism never does. An example could be the above-mentioned temporary DL during the season of agricultural work in the forager-horticulturalist Tsimane (Stieglitz et al., 2013). In the producer case, individuals typically become phenotypically specialized, but genetic branching is relatively rare. Genetic branching can evolve under some conditions: a low number of tasks, a large group size, and strong assortativity during both imitation and reproduction. We therefore conclude that during the Neolithic, a previously unprecedented amount of behavioral diversification could occur, probably accompanied with little genetic diversification.
Even though this model is more detailed than strategic models usually are, it has several limitations and simplified assumptions because of the high complexity of the studied phenomenon. These we have already discussed in detail above. However, the limitations themselves could lay the path for further investigations based on this model framework. Such further directions could be the incorporating of food sharing, studying the effect of social stratification, or including sexes with different life histories and sexual DL.
We believe that the importance of our work reaches beyond the technical investigation of a strategic model. First, the immense literature on the evolution of division of labor neglects social division of labor, which is DL within sex and age groups. With this paper we hope to direct more attention toward SDL, so typical of post-agricultural humans. Second, it may bear implications for recent social processes. Globalization creates an environment where both social connectedness, and the scale of SDL reaches ever higher levels. According to our model, such an environment selects for an enormous phenotypic, and perhaps genetic diversity.
Data Availability Statement
The program code used for generating the raw data will be made available by the authors, without undue reservation, to any qualified researcher.
Author Contributions
ZV and IS developed the model framework together. ZV implemented and performed the numerical simulations. IS supervised the research. ZV and IS discussed the results together and both contributed to the final manuscript.
Funding
This research was supported by the GINOP 2.3.2-15-2016-00057 research fund.
Conflict of Interest Statement
The authors declare that the research was conducted in the absence of any commercial or financial relationships that could be construed as a potential conflict of interest.
The handling editor declared a shared affiliation, though no other collaboration, with the authors at the time of the review.
Acknowledgments
ZV is grateful to the Konrad Lorenz Institute for Evolution and Cognition Research for the opportunity to work on this model during her fellowship there. The authors also thank Douglas W. Yu, Tamás Hajdu and the two reviewers for their useful comments on the manuscript.
Supplementary Material
The Supplementary Material for this article can be found online at: https://www.frontiersin.org/articles/10.3389/fsoc.2018.00035/full#supplementary-material
References
Alvard, M. S., and Gillespie, A. (2004). Good Lamalera whale hunters accrue reproductive benefits. Res. Econ. Anthropol. 23, 223–245. doi: 10.1016/S0190-1281(04)23009-8
Bar-Yosef, O. (1998). On the nature of transitions: the middle to upper palaeolithic and the neolithic revolution. Cambridge Archaeol. J. 8, 141–163.
Bar-Yosef, O. (2001). “From sedentary foragers to village hierarchies: the emergence of social institutions,” in Proceedings of the British Academy, Vol. 110 (Oxford: Oxford University Press Inc.), 1–38.
Binford, L. R. (1980). Willow smoke and dogs' tails: hunter-gatherer settlement systems and archaeological site formation. Am. Antiquity 45, 4–20.
Bird-David, N. (2005). “Studying children in ‘hunter-gatherer’ societies,” Hunter-Gatherer Childhoods: Evolutionary, Developmental, and Cultural Perspectives, eds M. E. Lamb and B. S. Hewlett (New Brunswick; New Jersey, NJ: Transaction Publisher), 92–101.
Boas, F. (1895). The Social Organization and the Secret Societies of the Kwakiutl Indians. Report of US National Museum, 317–318.
Boehm, C. (2001). Hierarchy in the Forest: The Evolution of Egalitarian Behavior. Cambridge, MA: Harvard University Press.
Boivin, N. L., Zeder, M. A., Fuller, D. Q., Crowther, A., Larson, G., Erlandson, J. M., et al. (2016). Ecological consequences of human niche construction: examining long-term anthropogenic shaping of global species distributions. Proc. Natl. Acad. Sci. U.S.A. 113, 6388–6396. doi: 10.1073/pnas.1525200113
Cartwright, P. (2003). Developmental insights into the origin of complex colonial hydrozoans. Integr. Compar. Biol. 43, 82–86. doi: 10.1093/icb/43.1.82
Conley, D., Laidley, T., Belsky, D. W., Fletcher, J. M., Boardman, J. D., and Domingue, B. W. (2016). Assortative mating and differential fertility by phenotype and genotype across the 20th century. Proc. Natl. Acad. Sci. U.S.A. 113, 6647–6652. doi: 10.1073/pnas.1523592113
Derex, M., Beugin, M. P., Godelle, B., and Raymond, M. (2013). Experimental evidence for the influence of group size on cultural complexity. Nature 503, 389–391. doi: 10.1038/nature12774
Diamond, J. (1999). Guns, Germs, and Steel: The Fates of Human Societies. New York, NY: WW Norton & Company.
dos Santos-Luiz, C., Mónico, L. S., Almeida, L. S., and Coimbra, D. (2016). Exploring the long-term associations between adolescents' music training and academic achievement. Music. Sci. 20, 512–527. doi: 10.1177/1029864915623613
Dunbar, R. I. (1993). Coevolution of neocortical size, group size and language in humans. Behav. Brain Sci. 16, 681–694.
Dwyer, P. D., and Minnegal, M. (1991). Hunting in lowland, tropical rain forest: towards a model of non-agricultural subsistence. Hum. Ecol. 19, 187–212.
Finlayson, B., and Warren, G. M. (2010). Changing Natures: Hunter-gatherers, First Famers and the Modern World. London: Bristol Classical Press.
Goldsby, H. J., Dornhaus, A., Kerr, B., and Ofria, C. (2012). Task-switching costs promote the evolution of division of labor and shifts in individuality. Proc. Natl. Acad. Sci. U.S.A. 109, 13686–13691. doi: 10.1073/pnas.1202233109
Gowdy, J., and Krall, L. (2013). The ultrasocial origin of the anthropocene. Ecol. Econ. 95, 137–147. doi: 10.1016/j.ecolecon.2013.08.006
Gurven, M., and von Rueden, C. (2006). Hunting, social status and biological fitness. Soc. Biol. 53, 81–99. doi: 10.1080/19485565.2006.9989118
Gurven, M., Winking, J., Kaplan, H., von Rueden, C., and McAllister, L. (2009). A bioeconomic approach to marriage and the sexual division of labor. Hum. Nat. 20, 151–183. doi: 10.1007/s12110-009-9062-8
Henrich, J. (2004). Demography and cultural evolution: how adaptive cultural processes can produce maladaptive losses—the tasmanian case. Am. Antiquity 69, 197–214. doi: 10.2307/4128416
Huber, S., and Fieder, M. (2011). Educational homogamy lowers the odds of reproductive failure. PLoS ONE 6:e22330. doi: 10.1371/journal.pone.0022330
Jeanson, R., Fewell, J. H., Gorelick, R., and Bertram, S. M. (2007). Emergence of increased division of labor as a function of group size. Behav. Ecol. Sociobiol. 62, 289–298. doi: 10.1007/s00265-007-0464-5
Kaplan, H., and Hill, K. (1985). Hunting ability and reproductive success among male ache foragers: preliminary results. Curr. Anthropol. 26, 131–133.
Kaplan, H., Hill, K., Hawkes, K., and Hurtado, A. (1984). Food sharing among ache hunter-gatherers of eastern paraguay. Curr. Anthropol. 25, 113–115.
Krzyzanowska, M., and Mascie-Taylor, C. G. (2014). Educational and social class assortative mating in fertile british couples. Ann. Hum. Biol. 41, 561–567. doi: 10.3109/03014460.2014.903996
Leibowitz, N., Baum, B., Enden, G., and Karniel, A. (2010). The exponential learning equation as a function of successful trials results in sigmoid performance. J. Math. Psychol. 54, 338–340. doi: 10.1016/j.jmp.2010.01.006
Maher, L. A., Richter, T., and Stock, J. T. (2012). The pre-Natufian Epipaleolithic: long-term behavioral trends in the Levant. Evol. Anthropol. 21, 69–81. doi: 10.1002/evan.21307
Marlowe, F. (2010). The Hadza: Hunter-gatherers of Tanzania, Vol. 3. Berkeley; Los Angeles, CA: Univ of California Press.
Maynard Smith, J., and Szathmary, E. (1997). The Major Transitions in Evolution. Oxford: Oxford University Press.
Nakahashi, W., and Feldman, M. W. (2014). Evolution of division of labor: emergence of different activities among group members. J. Theor. Biol. 348, 65–79. doi: 10.1016/j.jtbi.2014.01.027
Penke, L., Denissen, J. J., and Miller, G. F. (2007). The evolutionary genetics of personality. Eur. J. Pers. 21, 549–587. doi: 10.1002/per.629
Penke, L., and Jokela, M. (2016). The evolutionary genetics of personality revisited. Curr. Opin. Psychol. 7, 104–109. doi: 10.1016/j.copsyc.2015.08.021
Plomin, R. (2011). Commentary: why are children in the same family so different? Non-shared environment three decades later. Int. J. Epidemiol. 40, 582–592. doi: 10.1093/ije/dyq144
Randahl, G. J. (1991). A typological analysis of the relations between measured vocational interests and abilities. J. Vocat. Behav. 38, 333–350.
Sæther, B. E., and Engen, S. (2015). The concept of fitness in fluctuating environments. Trends Ecol. Evol. 30, 273–281. doi: 10.1016/j.tree.2015.03.007
Schermer, J. A., and Vernon, P. A. (2008). A behavior genetic analysis of vocational interests using a modified version of the jackson vocational interest survey. Pers. Individ. Diff. 45, 103–109. doi: 10.1016/j.paid.2008.03.009
Shennan, S., Downey, S. S., Timpson, A., Edinborough, K., Colledge, S., Kerig, T., et al. (2013). Regional population collapse followed initial agriculture booms in mid-holocene Europe. Nat. Commun. 4:2486. doi: 10.1038/ncomms3486
Simmons, A. H. (2012). “The levant,” in Chapter 7: A Companion to the Archaeology of the Ancient Near East, ed D. T. Potts (Chichester: Blackwell Publishing Ltd.), 127–143
Smith, E. A., Bird, R. B., and Bird, D. W. (2003). The benefits of costly signaling: Meriam turtle hunters. Behav. Ecol. 14, 116–126. doi: 10.1093/beheco/14.1.116
Stieglitz, J., Gurven, M., Kaplan, H., and Hooper, P. L. (2013). Household task delegation among high-fertility forager-horticulturalists of lowland bolivia. Curr. Anthropol. 54, 232–241. doi: 10.1086/669708
Thomas, M. L., and Elgar, M. A. (2003). Colony size affects division of labour in the ponerine ant Rhytidoponera metallica. Naturwissenschaften 90, 88–92. doi: 10.1007/s00114-002-0396-x
Turkheimer, E. (2000). Three laws of behavior genetics and what they mean. Curr. Dir. Psychol. Sci. 9, 160–164. doi: 10.1111/1467-8721.00084
Vásárhelyi, Z., Meszéna, G., and Scheuring, I. (2015). Evolution of heritable behavioural differences in a model of social division of labour. PeerJ 3:e977. doi: 10.7717/peerj.977
Wailes, B. (1996). Craft Specialization and Social Evolution: In Memory of V. Gordon Childe, Vol. 93. Philadelphia, PA: University of Pennsylvania Press.
Keywords: neolithic transition, division of labor, behavioral diversity, human evolution, individual based model
Citation: Vásárhelyi Z and Scheuring I (2018) Behavioral Specialization During the Neolithic—An Evolutionary Model. Front. Sociol. 3:35. doi: 10.3389/fsoc.2018.00035
Received: 12 April 2018; Accepted: 06 November 2018;
Published: 29 November 2018.
Edited by:
Károly Takács, Hungarian Academy of Sciences (MTA), HungaryReviewed by:
Maria Emilia Yamamoto, Federal University of Rio Grande do Norte, BrazilDaniel E. Adkins, University of Utah, United States
Copyright © 2018 Vásárhelyi and Scheuring. This is an open-access article distributed under the terms of the Creative Commons Attribution License (CC BY). The use, distribution or reproduction in other forums is permitted, provided the original author(s) and the copyright owner(s) are credited and that the original publication in this journal is cited, in accordance with accepted academic practice. No use, distribution or reproduction is permitted which does not comply with these terms.
*Correspondence: István Scheuring, aXN0dmFuLnNjaGV1cmluZ0BnbWFpbC5jb20=