- 1Department of Geography and Environmental Studies, University of Limpopo, Sovenga, South Africa
- 2Risk and Vulnerability Science Centre, University of Limpopo, Sovenga, South Africa
- 3Department of Accountancy, Vaal University of Technology, Andries Potgieter Boulevard, Vanderbijlpark, South Africa
- 4South African Weather Service, Pretoria, South Africa
- 5Water Research Commission, Pretoria, South Africa
Smallholder farmers like those in Limpopo and the Free State (FS) Province of South Africa sometimes incur high production costs due to their crop choices. This cost is exacerbated by the challenges posed by climate change and the socio-economic position of the smallholder farmers. The opportunity cost of producing cost-intensive crops is the forgone benefits these smallholder farmers would have gained if they grew cheaper options. Therefore, a tool to assist farmers and stakeholders when choosing, advising on, or assisting with production and management decisions at farm levels to ensure profitability and sustainability is needed. This paper aimed at providing a framework to assist farmers in identifying profitable crops for production under the present day and possible future scenarios in Limpopo and the FS. This framework uses a multi-stage process with forward and backward linkages to refine analysis and results. Through reviews, interviews, focus groups and transect walks, the state of the biophysical, socio-economic, and political environment and their impact on smallholder farming were collected from 600 farmers and 40 key informants. Inferential statistics and cost-benefit analysis were used for data analysis. The results indicate that maize, though widely cultivated in both Provinces, has the highest average total production cost of R 29,694.39 /ha while the lowest was sunflower with R 6,453.78 /ha. Irrigated maize had the highest break-even price per hectare of R 38 351.85, while sunflower had the lowest R 4 685.85. In both provinces, groundnut ranked first on Net Present Value (NPVs) at discount rates of 8% and 10%, while low yield maize ranked last, scoring values of –R 4 163 894 and –R 3 953 393. Groundnut had the highest Benefit Cost Ratio (BCR) at 2.22 and 6.70 in Limpopo and FS. The BCR for low-yield maize was the lowest of all the crops. The base scenario results in economic and financial terms indicate it is better to farm sunflower, soybean, grain sorghum, and groundnuts than maize because of lower production cost and maize substitutes. Furthermore, climate change projections suggest a drier future for these provinces, implying the yield targets for farmers producing maize would be difficult to achieve when faced with climate change. Such information is vital for stakeholders.
Introduction
Agriculture plays a significant role in the economic development of Limpopo and the Free State provinces in South Africa. These provinces are some of the main grain-producing regions and are highly dependent on summer rainfall. Since the first quarter of 2020, the agricultural value chain in South Africa has been a positive contributor to the country's GDP growth with an increase of 28.6%, becoming the strongest performer (15.1%), National Agricultural Marketing Council (2020) and as such, has implications for political stability [Bureau for Food and Agricultural Policy (BFAP), 2018] and food security. Achieving food security remains a global priority. According to the Economic Commission for Africa (ECA) (2018), 821 million people are undernourished or short of food worldwide.
South Africa's agricultural sector faces numerous water challenges due to an already stressed state of water resources in the country (Schulze, 2016). The effect of climate change on agricultural output will directly affect rural communities, having a knock-on effect on both the rural economies and the food-security nexus [Financial Fiscal Commission (FFC), 2011]. This is especially so, given the fact that about 90% of the rural areas in South Africa are semi-arid or sub-humid, while 10% is considered hyper-arid (Schulze, 2008) and 14% of the land is considered potentially arable, with one-fifth having high agricultural potential [Department of Environmental Affairs (DEA), 2013]. This means that lands have to be efficiently utilized for profitability and food security to be achieved.
By 2050 farmers are expected to be able to produce 70% more food to meet the needs of the expected global population of 9.1 billion (FAO, 2009). With the projections of climate variability and climate change in the foreseeable future (IPCC, 2014), agriculture will need to adapt to maintain or improve food security, economic activities, and livelihoods, especially in developing countries such as South Africa (Howden et al., 2007). Climate change projections for South Africa, show a reduction in rain-fed dependent agricultural production and a 4–6% increase in irrigation demand for agriculture (Ziervogel et al., 2014). Given that human, financial, and physical resources are limited, scarce resources such as water must be aimed at producing grains, which are -important for maintaining the most vulnerable communities.
According to Bureau for Food and Agricultural Policy (BFAP) (2018), South African farms compared to their global counterparts are less financially competitive in terms of production costs. Even though, on average, South African producers spent between US$52 to US$68 to produce a ton of maize, which is well within the cost of production of the international sample average of US$61 per ton maize produced, however, the fertilizer utilization component in the production process shows that the cost to produce a ton of maize is on average 34% higher for South African farms relative to the global average [Bureau for Food and Agricultural Policy (BFAP), 2018]. Hence it is more costly to farmers to produce, resulting in farmers not being able to compete with international prices. Longer-term trends in the area cultivated for major field crops reflect a current pressure on grain producers' profitability levels [Bureau for Food and Agricultural Policy (BFAP), 2018]. Considering this rapidly increasing need for profitability for summer grain production systems, tools are needed to assist farmers and relevant stakeholders in making strategic decisions regarding sustainable and profitable production, resilience, and adaptability.
Targeting and prioritizing methods narrow down a range of possible practices, services, and policies to best-betting options that can be scaled (Andrieu et al., 2017). However, there is scant literature of such frameworks being employed regarding grain production, despite the evident advantage of using a targeting and prioritization framework for decision making. The authors of this paper are not aware of any study conducted in South Africa on a framework to guide farmers in the choices of crops to produce based on farmers' socio-economic circumstances. Existing literature on targeting, such as that of Notenbaert et al. (2017), looked at the framework for evaluating and prioritizing potential interventions in climate-smart agriculture. Herrero et al. (2014) provided a targeting and scaling-out framework for interventions in agricultural systems. Their study showed that higher quality feeds and improved feeding practices for livestock could lead to a three-pronged winning scenario where the farmers could meet the growing food demands for the livestock at the same time mitigating and adapting to climate change as well as experiencing an improved livelihood.
Cost-benefit analysis approaches have been used for decisions making such as in the implementation of conservation agriculture in Uzbekistan (e.g., Daujanov et al., 2016) in southern Africa (Tafa, 2017; Mutenje et al., 2019); for technologies implementation (Badolo, 2017) for sorghum and maize cropping systems in Mali) manual for cost-benefit analysis in South Africa with emphasis on water resource development (Economists, 2014), and on-farm climate change adaptation strategies in Ghana (Azumah et al., 2020). Mutenje et al. (2019) found that climate-smart agricultural (CSA) practices that integrate aspects of soil and water management practices which are grounded on the tenets of conservation agriculture (CA), cereal-legume plant species intercropping, and improved crop varieties are economically viable and should be implemented by risk-averse smallholder farmers. Magati et al. (2012) used a cost-benefit analysis to advise smallholder tobacco farmers in South Nyanza, Kenya, to substitute bamboo for tobacco. They found out that bamboo farming was more financially and economically beneficial than tobacco farming, given the incremental benefits, and recommended well-managed bamboo farming as an alternative livelihood to tobacco farming. Similar findings obtained by Njenga (2016) in Muranga County, Kenya, indicate that farmers were advised to switch from coffee to banana farming. However, these studies did not show the various steps used in selecting proposed crops before conducting the cost-benefit analysis.
This paper developed and used a framework to outline methodologies for assisting stakeholders in deciding what crops to produce. The framework takes into consideration the complex nature of the agricultural system and decision-making processes across different landscapes. It provides a clear understanding of the interrelationships between natural resources and ecosystems, agricultural production systems, socio-economic variables, and institutional and policy systems that drive decision-making processes and outcomes. Such information will provide direct, actionable results that are relevant to stakeholders.
Materials and Methods
This section describes the framework, the application, data collection and analysis.
Description of the Framework for Targeting and Prioritizing Interventions in Grain-Producing Areas of South Africa
The framework involves four generic steps that follow a logical order and represent the initial workflow, with a snowball effect involving: an examination of the biophysical environment, the socio-economic factors and policy at play, the relationship between different enterprises within the system that impact farming, and a cost-benefit analysis. Information and data from one step feeds into the next step (Figure 1). The framework involves a multi-stage process with forward and backward linkages that assist in refining analysis and results. These forward and backward linkages allow for a deeper understanding of the processes involved. The prioritization structures are set such that they are generally question-driven and score-based, thereby enabling a balanced and transparent approach to decision-making (Benke et al., 2011).
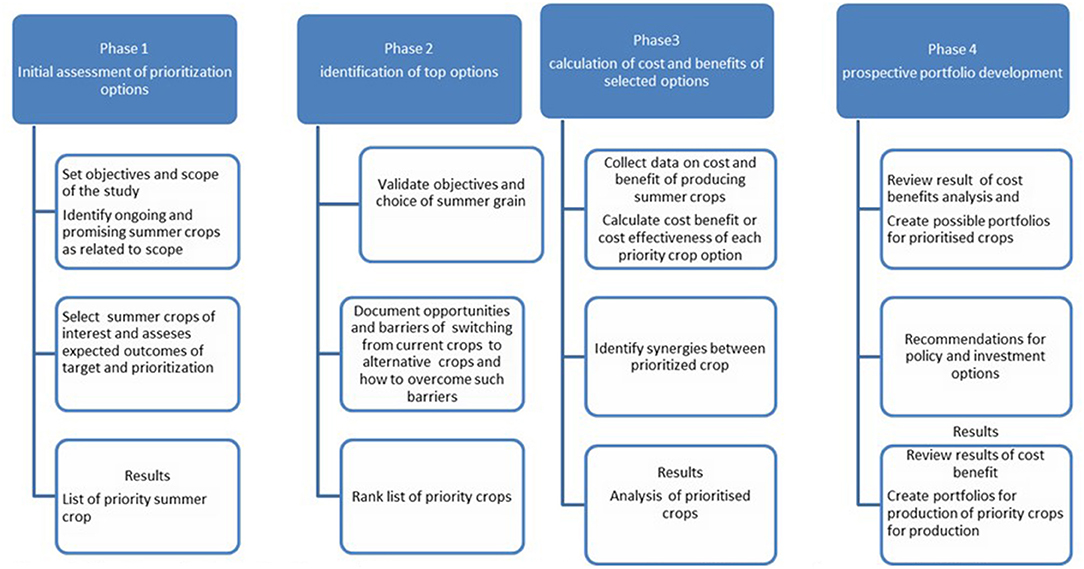
Figure 1. Targeting and prioritization framework for summer grain production compromising prioritization options, option indication, cost-benefit analysis, and portfolio development (adapted from Campbell et al., 2016; Andrieu et al., 2017).
Phase 1: Initial Assessment of Prioritization Option
The first level in the framework involves diagnosing and identifying local environments and area-specific problems encountered by farmers. Firstly an initial assessment and description of the agricultural system are carried out. A description is then made in terms of crops produced, specific opportunities available to farmers, financial, physical, natural, and social resources related to various livelihood strategies. This step consists of an integrated and participatory process of combining, interpreting, and communicating knowledge from diverse scientific disciplines to understand better complex phenomena (Rotmans and van Asselt, 1999). This stage aimed to assess the prioritization process, the stakeholders involved, and the list of crop options to consider. Furthermore, assessments are done to identify vital water-efficient crops that are valuable but had not been given adequate attention in terms of utilization and production.
The selection process started with the scrutiny of the summer grain production systems, farming systems (subsistence to commercial farmers), climate change projections, and transformative actions. Various data repositories such as Grain SA, the Department of Agriculture, Forestry and Fisheries (DAFF), and expert assessments were consulted to establish a holistic view of the crops cultivated and their value.
Secondly, the selected crops were evaluated for their expected social, environmental, and economic impacts within specific context and farmer category. A brief description of each potential crop was prepared, including possible constraints and benefits for production and use. Answers to the following questions addressed key fundamental aspects as adapted from Notenbaert et al. (2017):
1. What are the human, natural, financial, social, and resources available to produce selected summer grains and how are the available resources organized for production?
2. What are the current levels and trends of summer grain crops in terms of their productivity, demand, and consumption?
3. What are the climate change scenarios and projections and associated impacts on the natural resource base, crop suitability and yield?
4. What are the projections for future production?
5. What/who is vulnerable, and what are the possible measures for adaptation to mitigate such vulnerability?
6. Who/what influences the decision or wider context in which the agricultural production and potential crop choice for production are taking place?
Phase 2: Identification and Characterization of Top Priority Options
This step involved the characterization of the solutions offered to questions asked in Phase 1. Solutions were gleaned from the literature review as well as elicited from stakeholders and experts. Included in the criteria were farmer surveys that provided information on crop production and the choice of crops for production. These helped in the identification of preferred crops for adoption, as well as the spatial dimensions of adoption. The identification of options phase helped reduce the initial list of grain crop options. An overall summary of crop characteristics, conditions favoring the successful cultivation of the crop of choices based on threshold definition was compiled. This validated the overall objectives and defined the relative weight that should be given to each crop in terms of productivity and food security.
Phase 3: Economic Analysis in Terms of Cost and Benefits
Phase 3 examined the economic benefits of the available options regarding each summer grain crop option's costs and benefits. Production costs for summer grain crop options from phases 1 and 2 were used as input for the cost-benefit analysis (CBA). Input data for the CBA model was derived from primary data, scientific literature, and expert knowledge. The cost-benefit analysis was conducted as part of the cost implications involved from the change in crop choices in the face of climate change and variability. This will guide the farmers' perspective and decisions regarding profitability, environmental sustainability, and reduce strain on natural resources, particularly water resources. In this phase, each selected crop's profitability was determined and compared to the initial preferred crop, taking cognizance of externalities. According to Sain et al. (2017), CBAs that focus solely on the costs and benefits of agricultural practices can often distort assumptions about the likelihood of adoption at scale due to the exclusion of critical externalities and limited discussions of risk. The list of selected summer crop options from a sound agroecological perspective, socio-economic relevance, and benefits to farming households were further ranked based on a cost-benefit or cost-effectiveness analysis of the investment.
Phase 4: Portfolio Analysis
A crop-by-crop analysis and evaluation of barriers to crop production were conducted in the final phase. Ratings for possible crop production options based on impacts on natural resource usage and costs and benefits were done to support discussions on trade-offs between different crops produced. Aggregated benefits from the different crop portfolios were explored for a final selection of investment priorities.
A critical component of this stage is the robust analysis of constraints and barriers to changes in the choice of crops for production from the perspective of different farmers as well as stakeholders in the agricultural sector. Exploring “what if” scenarios enabled stakeholders to engage in a more in-depth discussion around the potential impacts and tradeoffs (Kristjanson et al., 2009) taking into consideration the temporal and spatial scales involved in changing crop options. This assessment builds an understanding of the various social, cultural, and economic barriers at play when switching the crop produced in a specific area. This insight can improve the design and implementation of plans for food production, sustainability and food security in the era of climate change.
Study Areas
The framework was used in two provinces to demonstrate how targeting and prioritization can be linked to the choice of crops for production by smallholder farmers. The location of the study areas of Limpopo and the Free State provinces is shown in Figure 2.
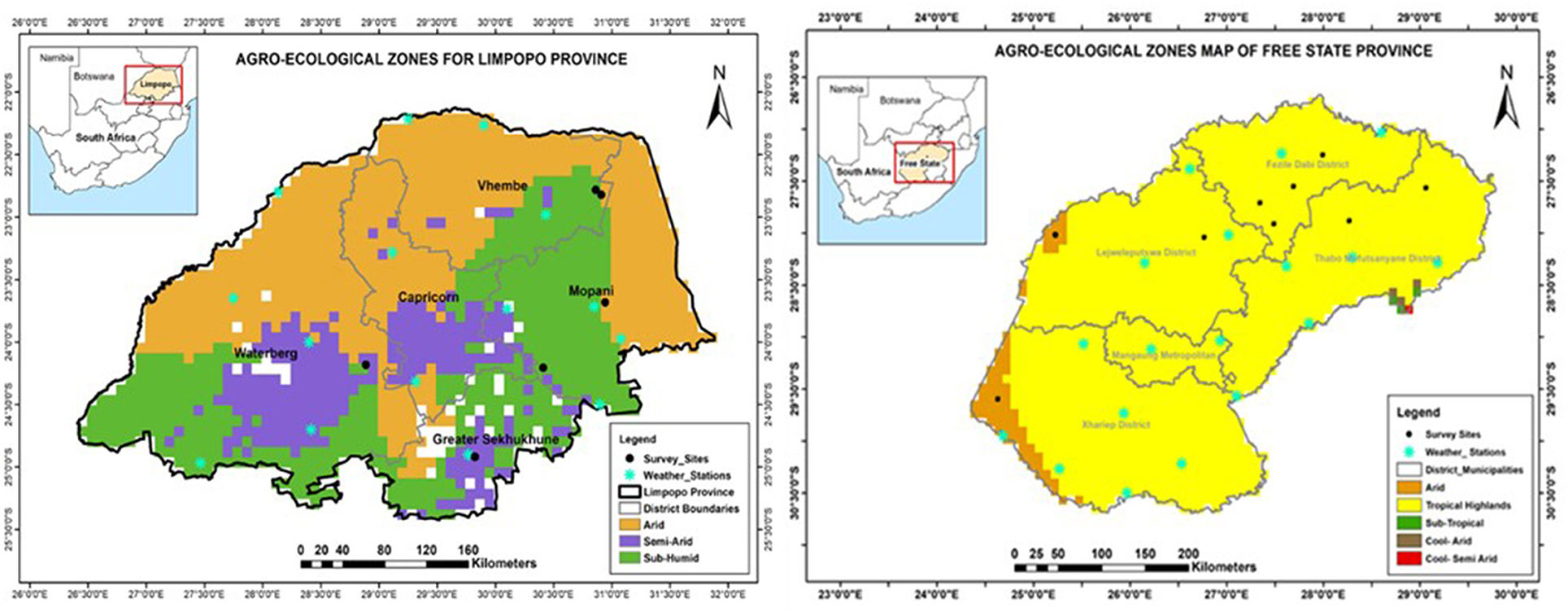
Figure 2. Location map for Limpopo and the Free State Provinces with sampled sites. Source: Adapted from Harvest Choice (2010).
Limpopo Province
Limpopo Province (Figure 2) covers 12, 46 million hectares, which is 10.2% of the total area of South Africa (Oni et al., 2012). Climatic regions in the area include the Lowveld (arid and semi-arid) regions, the middle veld, highveld, semi-arid region, and the escarpment region, which has a sub-humid climate with a 700 mm rainfall per annum (Limpopo Department of Agriculture, 2012). The province is characterized by low and erratic rainfall patterns and is prone to drought and flood events. Rainfall in the province occurs mostly between October to April and ranges from 200 mm in the hot, dry areas to 1500 mm in higher rainfall areas. There is a high annual rainfall variability in the province.
Smallholder farmers are mostly found in the rural areas of the province. They have little or no access to production technology with an average farm size of about 1.5 hectares per farmer. They occupy about 30% of the provincial land (Ubisi, 2016). Agricultural production in the Limpopo Province is diverse, with most smallholder farmers tending to focus on producing field crops dominated by maize, particularly during the summer months. Most smallholder farming is rain-fed and limited by multiple factors such as precipitation, poor soils, and limited input resources.
Free State Province
The Free state province (Figure 2), with an area of around 129 825 km2 is the third-largest province in South Africa, making up 10.6% of South Africa's land area (Davis and Tavasci, 2006). Most places in the province receive annual mean precipitation of between 400 and 700 mm (Moeletsi, 2010). More than 70% of the Free State's rainfall occurs between October and April (ARC-ISCW Agroclimatological Databank, 2010). The mean annual minimum temperatures are very low, with <5°C experienced over the high-lying areas in the province's eastern parts (Moeletsi, 2010). The temperatures increase westwards, with most parts of the province having a mean minimum temperature of between 7 and 9°C. The western border of the province records relatively high minimum temperatures exceeding 9°C.
According to the Department of Agriculture, Forestry, and Fisheries (Department of Agriculture, Forestry and Fisheries, 2010), agriculture contributes 20.1% to the province's gross domestic product. Most of the province's agricultural production is under rainfed conditions, with less than 10% of the arable land (Moeletsi and Walker, 2013). The Free State is responsible for ~30% of the national maize production, thus contributing significantly to the country's agricultural economy (Moswetsi et al., 2017). The major crops produced in the Free State province in a decreasing ton of production are maize, potatoes, soyabean, sunflowers, wheat, sorghum, groundnut, lucerne and drybean (Statistics South Africa, 2017). The majority of these are produced by large scale commercial farmers. Even though the Free State province produces over 30% of the maize in South Africa, the average maize yield varies significantly from 1 year to another due to climate variability. The province experiences significant changes in rainfall patterns, minimum and maximum temperature as well as frost.
Climate Change Projections for Limpopo and Free State Provinces
Climate change models indicate that Limpopo Province will face a warmer future with temperatures predicted to increase by as much as 2°C by 2035, 1–2°C between 2040 and 2060 (or between 2–5°C in the high-end scenarios), and by 3–6°C between 2080 and 2100 (or 4–7°C in the high-end scenarios) [Long Term Adaptation Scenarios (LTAS), Department of Environmental Affairs (DEA), 2013]. There is less certainty with regard to rainfall forecasts in Limpopo. Some climate models project decreases in rainfall while others suggest increases in rainfall. It is predicted that Limpopo will experience more significant variability in rainfall and increases in temperatures, which will further increase evaporation rates resulting in drier conditions in the future (FAO, 2009). Vulnerability assessments have identified agriculture as one of the most vulnerable sectors in terms of the effects of climate change.
Climate change projections for the Free State highlight temperature increases of 1–2.5°C for 2020–2050 relative to 1971–2000, under low mitigation (Envirotech Solutions, 2015). Under the high mitigation scenario, temperature increases over the Free State province will be somewhat less but may still reach 2.5°C over the province's western part. By the end of the century, temperature increases of 4–7°C are projected to occur over the Free State under the RCP8.5 scenario (Envirotech Solutions, 2015). In the case of high mitigation efforts, the projected changes are reduced but remain significant. The rainy season is likely to start later and last for a shorter amount of time (Envirotech Solutions, 2015). The Status Quo Report for the Free State highlights agriculture and the water sectors as the most vulnerable to climate change impacts in the province (Envirotech Solutions, 2015). Such changes in temperature and rainfall patterns as predicted over the Limpopo and the Free State provinces will affect water resources.
Future Changes in Environmental Suitability and Yield of Grain Crops in Limpopo and Free State
Given the projected increases in temperature over the Limpopo and the Free state, water availability and agriculture will be affected by inference. This will have a knock-on effect on crop yields. Studies that have dealt with climate change impacts on agriculture in South Africa have focused mainly on maize production (Turpie and Visser, 2013). Studies like Gbetibouo and Hassan (2005) assessed the effects of climate change on field crops (maize, wheat, sorghum, sugarcane, groundnut, sunflower, and soybean). Their results indicated that location and season influenced the potential impacts of climate change on crop production. These results align with those of other studies where temperature increases were found to negatively affect net profits, while increases in precipitation positively impacted net profits (Turpie and Visser, 2013). Although irrigation is presented as a practicable form of adaptation to climate change (e.g., Kurukulasuriya et al., 2006) in agriculture, the potential to use irrigation as an adaptive mechanism would be limited in use, especially by poorly resourced smallholder farmers.
The Long-Term Adaptation Scenarios Flagship Research Programme (LTAS) indicates mostly negative projected impacts on South Africa's key crops. Sorghum will experience increases by 2–4 t/ha in yield in parts of the Free State, while on the other hand, Limpopo will experience losses. With regards to soybean, the arc of yield decreases in the climatically suitable growth areas, and the core area of median yield increases (>30%) [Department of Environmental Affairs (DEA), 2013].
Data Collection
This section shows how data was collected through the various steps applied in the framework to determine which crop is the most suitable for production amongst the farmers in the selected provinces. A mixed-methods approach combining both quantitative and qualitative data analysis in a single study was used. Through this mixed-methods approach, complementary and converging answers are sought, and the outcomes of one analysis are used as inputs in developing and getting results in another following a sequential mixed-methods design.
Collection of Crop Data From the Literature (Desk Analysis)
A systematic literature review was carried out and the process included the design, conducting, data abstraction and analysis, and structuring and writing of the review following the five-step approach of Khan et al. (2003). In carrying out the review, the pertinent question was in the relationship to the overall research field of targeting and prioritization framework development and implementation, is this literature review needed and does it make a substantial, practical, or theoretical contribution? Criteria were set as follows: the articles should be written in English, published by January 2012, and should have crops grown by smallholder farmers, framework development and application, challenges experienced by smallholder farmers. This design phase further included framing structured questions before the review process based on specified terms smallholder farmers, challenges experienced by smallholders, environmental conditions of the areas, cost of crop production, crops produced in Limpopo and FS; selecting criteria that identify relevant work for the questions, structurally assessing the studies, summarizing the evidence, and interpreting the findings (Khan et al., 2003). The aim of the review guided the inclusion criteria. The literature searches and subsequent assessments were structured according to the current and projected future impacts of climate change on agriculture; outline the biophysical suitability of crops grown in Limpopo and Free State provinces. The material was synthesized to enhance the knowledge of which climate thresholds are likely to exacerbate smallholder farmers' vulnerability, based on the choice of crops they produce. The results from various searches were sorted by relevance after scanning article abstracts. Data abstracted was in the form of descriptive information, such as authors, years published, topic, or type of study, or in the form of effects and findings. Validity and reliability were ensured through a checklist design to see if the aim of the review was achieved. A total of 25 studies had material that was considered suitable for this review.
Sampling Technique
Representative samples for the survey were selected following a two-stage sampling process. The first stage involved selecting the survey areas and the second, the total available smallholder farmers engaged in summer grain production in the regions. According to Statistics South Africa (2012), the country lacks information on smallholder and subsistence agriculture. Agricultural censuses and surveys have primarily concentrated on commercial agriculture and have done less on small-scale and subsistence agriculture (Statistics South Africa, 2010, 2012), thereby making it difficult to get an accurate figure for the total number of subsistence/smallholder farmers in the areas. The population who participated in this study comprised farmers residing in Limpopo and the Free State Province. A purposive random sample of 600 smallholder farmers in Limpopo and 200 in the Free State was selected. The variation in the number of participants was primarily due to the geographical locations of smallholder farmers in the two provinces. The Limpopo province is predominantly rural with a large aggregate of smallholders farmers across the province whereas the Free State agriculture is dominated by established large white commercial farmers, relative to the smallholder farmers who are scattered across the province. This scattered nature of smallholder farmers in the Free State also poses logistical issues in sampling. The participant selection criterion was set as follows: the respondents were individual smallholder crop farmers who are dependent on rainfall. Relevant departments in each local municipality provided a list of farmers fitting the stated criteria, and smallholder farmers were randomly selected. The sampling was used to assess uniformity and homogeneous characteristics and to meet the objectives of the study. It had to adhere to the statistical specifications of the reasons for and the objectives of sampling, the sample size, precision, variability of data, for accuracy and representativity. Key informants, as well as extension officers, were also selected for discussions from the relevant departments. Key informants were selected following the snowball approach. They had to be involved with smallholder farmers. Farmer selection and focus group interviews were facilitated by various agriculture departments and other entities dealing within the agricultural sector.
Questionnaire Administration
Questionnaires were administered to smallholder farmers in Limpopo's and the Free State Province after obtaining their informed consent. The aims and importance of the study were communicated to farmers. The questionnaire was made up of structured and semi-structured questions. Farmers supplied information on their farming practices, factors influencing their choice of crops to produce, and the income derived from crop production. Questions were designed in English but translated into the languages (Sotho, Sepedi, Xitsonga and Tshivenda) understood by the participants during the interviews and focus group discussions. A team of twenty interviewers were trained for questioner administration. The questionnaire was pre-tested on a small group of smallholder farmers around the study areas who did not participate in the final study. This was done to minimize errors and format unclear questions. Given that the questionnaires were administered by a team that was conversant with the aims of the study and spoke the native language, all farmers present could understand and respond to all the questions with ease. A 100% response rate was obtained. Each questionnaire took an average of 20 min to be completed.
Key Informant Discussions
Qualitative data was collected through one-on-one discussions with 20 extension officers and other relevant stakeholders from government and non-governmental organizations who were already having projects and working with farmers in the areas. These interviews were geared toward ascertaining possible reasons why farmers took certain crop production decisions.
Focus Group Discussions
Several focus group discussions were held with the farmers and information on socio-economic conditions, their agricultural production and adaptation strategies toward climatic and non-climatic shocks and decisions regarding which type of crop was obtained. Discussions were carried out in groups of 20 farmers and lasted for an hour. Questions were translated into Sotho, Sepedi, Xitsonga and Tshivenda for ease of communicating with farmers. Interviewers carried out the focus group discussions and acted as moderators. We applied the following participatory tools in frames of focus group discussions: Social Network Analysis tool, Seasonal Calendar, Problem Tree, Future Backward Scenarios Analysis tool, Hazard, Vulnerability, and Impact matrix.
The social network was useful in determining the social capital (or lack thereof) of the communities as well as the vulnerability and capacity of the farmers. We asked focus group participants to list the various governmental and non-governmental organizations in their communities and what type of assistance they received from them. Then the participants had to indicate the relationship between them and the organization they mentioned in terms of access and ease of getting aid. The relationship could be defined as one-way or two-way/ mutually beneficial.
Seasonal Calendar was used to get data on the annual cycle of economic and social activities of the participants and their connections with climatic events. Results from the Seasonal Calendar may reveal valuable qualitative information about climate trends that are unknown to outsiders and hidden from national-level data or histories (Scott, 2013). This tool helped in identifying the relationship between socio-economic activities and seasonal variations. It also assisted in determining the economic capacities (or lack thereof). We requested focus group participants to discuss crop production practices in relation to the farming calendar and climate. These enabled us to discover the similarities and differences in crop production decisions of the selected participants.
The Problem tree exercise included single-loop, double-loop, and triple-loop learning. Single-loop dealt with consequences of specific actions (crop production, management practices); Double-loop focused on the assumptions, beliefs, culture, norms, practices, and traditions which underlie the actions and the Triple-loop challenged the assumptions, beliefs, culture, norms, practices, traditions, values and higher-order thinking processes that underpin assumptions and actions in relation to crop choices.
The Future Backward tool was used to assists participants to envision different crop production scenarios. These scenarios are “best future scenario/situation,” the “current scenario/situation,” and the “worst future scenario/situation.” In the first instance, the focus group participants were encouraged to mention issues of concern to them in terms of crop production and in the second instance, they were prompted to talk about production cost exclusively. Research participants were given “post-it” notes on which to write their anecdotes (one or two words that describe their crop production experiences). They were asked to write these anecdotes for “best future scenario/situation,” “current situation/scenario,” and “worst future situation/scenario.” The results were recorded on the future backward scenarios analysis tool.
The Hazard, Impact and Vulnerability matrix tool was used to enable participants to pinpoint hazards, the impact of the hazards, their vulnerability to their hazards, and scoring these hazards in terms of the priority to them. Hazards were ranked according to the impact felt. The hazard with the highest score poses more risk and the hazard with the least score poses the least risk from the viewpoint of the participants. The ranking indicated which climate change and climate variability hazards should be prioritized when drawing climate change adaptation plans
Notes were taken and sessions recorded for ease of collaboration of data collected.
Data Analysis
Descriptive statistics, frequencies, and cost-benefit analysis were used to rank crops in decreasing order of importance. The following criteria drove these analyses:
• The cost attributable to the biophysical environment: crop cultivation parameters based on the climatic and soil requirements, water use demands in terms of current and future crop suitability based on climate change projections.
• Costs in terms of ease of production: accessibility to farm inputs, accessibility to crop production information, and support.
• Degree of importance and usage of the crop(s) at household and country level.
Cost in terms of economic and farm income was analyzed from the point of break-even analysis, specifically break-even yield and break-even price. Billore et al. (2020) believe that the break-even price analysis as a decision-making tool enables businesses to evaluate the risks they might be exposed to due to severe fluctuations in the price(s) of their products. Break-even yield analysis is a useful mechanism determining the possible yield losses that an agricultural business may incur due to weather conditions' variabilities (Billore et al., 2020). Break-even analysis identified the crop that would be least susceptive to poor weather conditions and price volatility and incurs the least production cost. This is because, with break-even price and yield analysis, farmers can identify the output quantities and prices that will be sufficient to cover attributable costs (Billore et al., 2020).
The break-even yield and price were calculated using the following formulas:
Where:
Total Cost = total variable expenditure (Dollar/ha) plus total fixed cost (Dollar/ha)
Net farm Gate Price: the price at which crops are sold on the farm.
Where:
Total Cost = total variable expenditure (Dollar/ha) plus total fixed cost (Dollar/ha)
Net farm Gate Price: price at which crops are sold on the farm.
In addition to the break-even analyses, this study also used cost-benefit analyses (CBA), which pertains to evaluating the monetary equivalent of the costs and benefits of a scenario, an intervention, or a set of circumstances (Mutenje et al., 2019). CBA are invaluable tools for evaluating and ranking the socio-economic and financial benefits of a range of projects for which there are limited funds available (Odeck and Kjerkreit, 2019). An ex-post CBA analysis was done because of the absence of projected figures and the availability and consistency of the cost and revenue information for the past few years.
CBA was used to establish the quantifiable financial benefits of choosing one crop over another. The costs and benefits used were confined strictly to quantifiable financial information: expenses related and unrelated to production, revenues, and gross and net margins. CBA indicators used included the Net Present Value (NPV), the Benefit-Cost Ratio (BCR). The NPV determines the discounted present value of the net cash flows attributable to a project (Hasan and Khalequzzaman, 2018). These indicators are interrelated: a CBA >1 implies that the NPV will be greater than zero (Kelly et al., 2015). If the BCR is higher than 1, then the benefits will be higher than the costs. This, of course, implies that the higher the BCR, the higher the expected profitability of the product or crop and vice versa. Thus, if the BCR is <1, the discounted future cash flows or earnings attributable to the harvest will be negative, implying a loss. The opposite applies if the BCR is higher than 1. These indicators were calculated using the revenues, costs and net margins for all five crops over a period of 5 years. The following respective formulas were applicable:
Discount rate: Time preference of money and opportunity cost of investment was used to explain the discounted rate. For this study's purpose, the discount rates were based on the Water Research Commission Report No. TT 305/07 written by Mullins et al. (2014). It is argued that the discount rate that should be used for environmental projects in South Africa should be discounted at the official discount rate of 8%. Due to the unstable interest rate in South Africa, the lending rate, which is 10.5%, was also used as an alternative.
Results
Targeting in combination with sustainable and cost-effective grain production can contribute to priority setting in the choice of crops produced. Limpopo and Free State provinces of South Africa were specifically selected to demonstrate the framework's applicability to very different locations and the possibility of providing information for different end-users, with specific aims and varied objectives. Both cases pertain to summer rainfall areas and are grain-producing areas in South Africa. This section summarizes the key differences in grain production cost in the Limpopo and Free State provinces.
Results of the Initial Assessment of Prioritization Options
Results from the literature review and farmer survey results revealed that smallholder farmers' dominant crops were summer grains in both provinces. The grain production system was therefore seen as the priority system for the framework application. Consultations with sampled farmers and experts showed that summer crops produced included maize (Zea mays), soybean (Glycine max), sunflower (Helianthus annus L.), groundnuts (Arachis hypogaea), sorghum (Sorghum bicolor) pearl millet (Pennisetum glaucum L.). Some farmers in Limpopo further grew other grain crops such as cowpea (Vigna unguiculata), bambara groundnut (Vigna subterranean), and mung bean (Vigna radiata). Of the sampled farmers, all of the produced maize in both Provinces. With regards to soybean, 132 farmers in Limpopo and 44 in the FS produced this grain; sunflower 102 in Limpopo and 70 in Fs; groundnuts 282 in Limpopo and 24; Sorghum 200 in Limpopo and 60 in FS; pearl millet 320 in Limpopo and 78 in FS while in Limpopo 380 produced cowpea, 300 bambara groundnut and 150 mung bean.
Identification and Characterization of Grain Options
A “win-win” choice of crop for production was made with the assumption that the choice crop will be suitable for these systems across the region and will increase farmers' livelihoods and productivity and, at the same time, reduce the cost of production. The adoption potential was considered to be associated with different key drivers such as sustainability, ease of production, production cost, and suitability parameters.
Ease of Producing Selected Grains in Limpopo and the Free State Province
Several factors influenced the decision to grow maize, soybean, groundnut, sunflower, sorghum and pearl millet in Limpopo and the Free State provinces. In Limpopo, respondents indicated that their decisions to grow groundnuts were strongly influenced by the availability of cash (98%), rainfall (97%), input availability (97%), food security (91%), and temperature (89%) (Figure 3). The decision for soybean production included input availability (58%), cash availability (56.83%), labor (54%), rainfall, water (Irrigation) (48.83%), food Security (43%) and temperature (49%). For maize, constraining factors included irrigation equipment (85%), temperature (85%), water (76%), cash (76%), and rainfall (78%). Sunflower was the least favored crop in the area in terms of investment decisions. The only factor which was not a deterrent to sunflower production was water. Pearl millet production was affected by aspects of food security (86%), cash (82%) and water (72%). In regards to cowpea, cash (73%) and irrigation equipment (70%) influenced the decision for its production.
In the Free State (Figure 4), the decision to not produce groundnuts was influenced by rainfall (83%), input temperature (70%) and irrigation equipment (68%). Factors inhibiting soybean production were temperature (70%) and input availability (48.83%). Similar to Limpopo, most factors acted as barriers to Sunflower production (Figure 4). Most of the factors are constraining factors of production. For maize, aspects such as rainfall (70%), temperature (60%), water (56%) deter farmers from cultivating maize. Input availability (50%) and food security (45%) were some of the constraints farmers experience in producing Sorghum.
Recommendation of Alternative Crops for Production
To give appropriate recommendations on the choice of crops for a farmer, it is crucial to understand what factors guided or influenced the choice of crop production. The farmers' predominant crop was maize (Figures 2, 5) despite constraints of cash flow, climatic factors with water shortages. With regards to soybean production in Limpopo, the crop was mostly produced by the younger farmers, while older farmers indicated, they were not familiar with the crop or did not see how this crop can enhance their food security The issues of food security are dominant in the decision of what grain crop to produce as seen with all the surveyed crops. This can explain why crops such as pearl millet, sorghum, cowpea, or Bambara groundnut are not seen as priority crops for production by farmers. A similar pattern was seen in the Free State (Figure 3) where sorghum farmers did not think the crop could contribute to their food security through consumption or sales.
With the decision made as to what crop to produce as well as the extent of production, the next aspect looked at was the cost of each grain production. It was found that it is cheaper to produce sunflower, soybean, grain sorghum and groundnuts than dryland maize and irrigated maize in Limpopo (Tables 1, 2). However, even though sunflower is relatively cheaper to produce, the break-even yield is lower for groundnuts than the rest of the crops (Table 2). From the five crops analyzed, maize had the highest break-even yield threshold of 14.70t ha−1. Therefore, the yield targets for farmers producing maize would be difficult to achieve under normal weather conditions, much less so when farmers are faced with climate change variabilities. The need to produce, on average, 14.70t ha−1 just to achieve the break-even yield also implies that maize farmers have had to contend with relatively high input costs. The analysis indicates that amongst the five crops, the highest average variable cost was attributed to maize –R 24,906.89/ha, whereas the lowest average variable cost was attributed to sunflower –R 3,801.03/ha (Table 5). This, in turn, necessitates selling the product at a price that is high enough to cover input costs and a profit margin. Amongst the five crops, irrigated maize has the highest break-even price per hectare of ~R 38 351.85, and sunflower has the lowest break-even price per hectare of ~R 4 685.85 (Table 2).
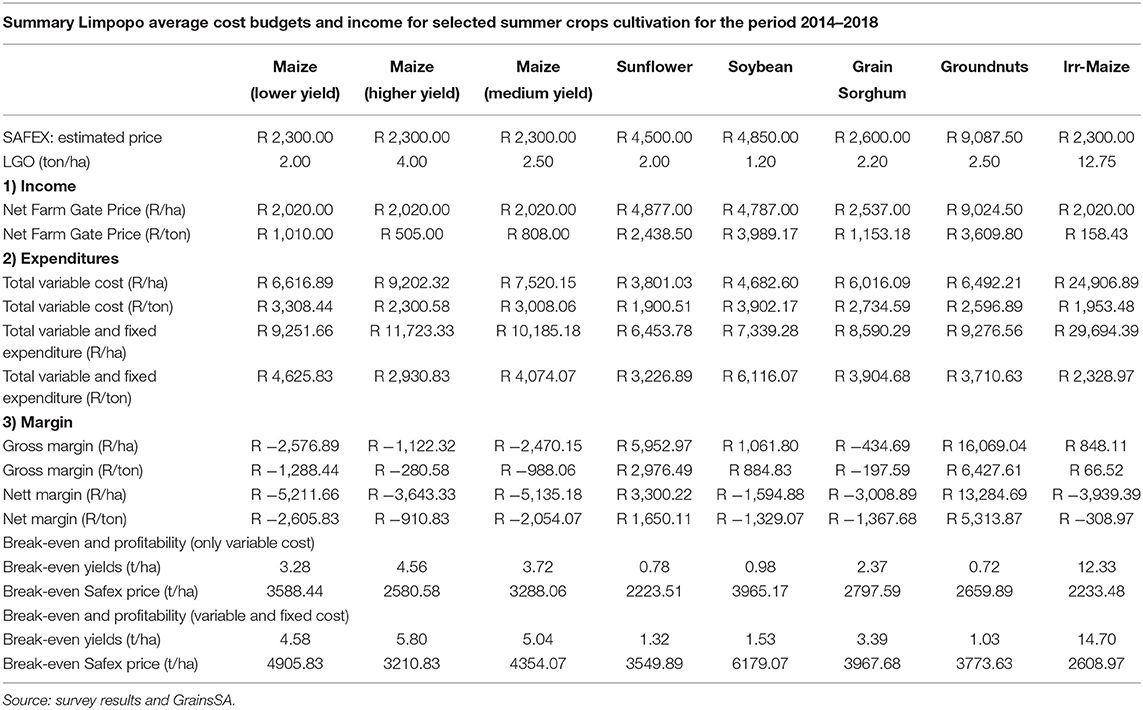
Table 2. Cost-benefit analysis for selected summer crop production in Limpopo Province from the period 2014 to 2018.
It is cheaper to produce sunflower, soybean, groundnuts, and grain sorghum in the Free State than either dryland maize or irrigated maize. For farmers to break even, they have to produce groundnut at the rate of 0.96t ha−1, followed by soybean, sunflower, sorghum, maize and irrigated maize (Table 5). From the results, maize production ranks amongst the most expensive grain produced in the Limpopo and Free State Provinces (Tables 1–5).
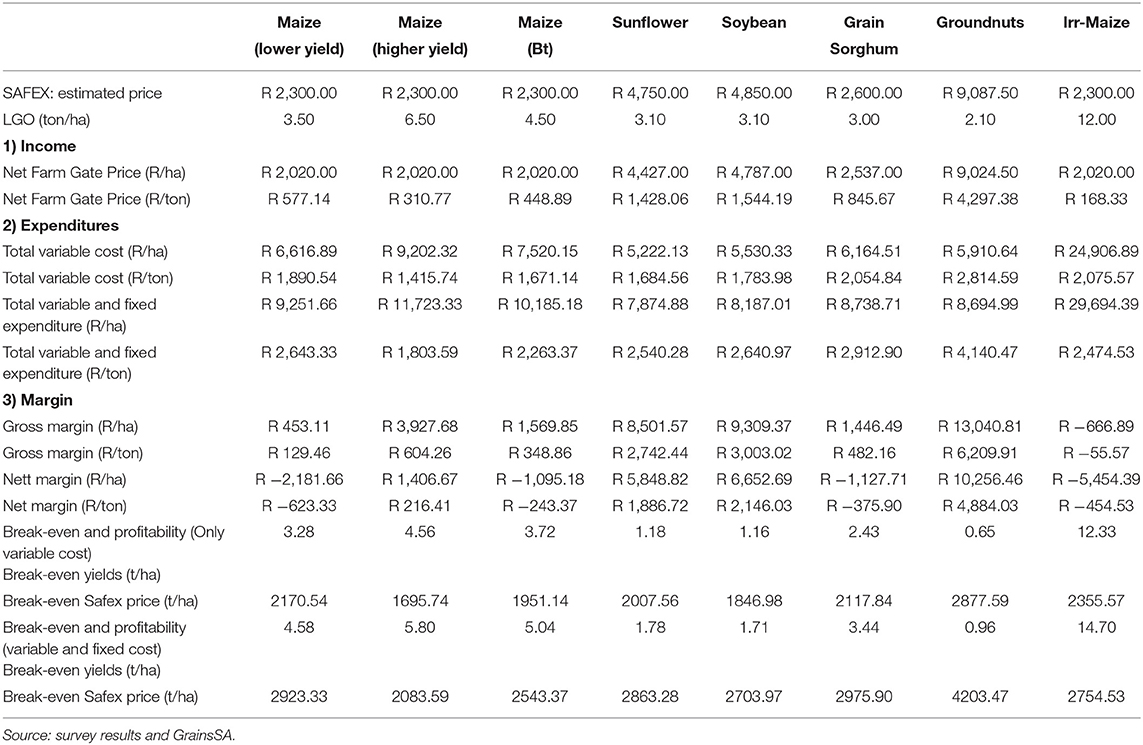
Table 4. Cost-benefit analysis for selected summer crop production in the Free State Province from the period 2014 to 2018.
CBA was used to assess the cost implications of producing a particular type of grains as well as the impact of the water need, use of the crop and its production costs (Table 5). The total cost for irrigated maize amounted to R 29 694.39/ha−1 for irrigated maize, easily the highest amongst the five crops. Groundnuts had the highest gross margin of R 13 040.81/ha−1, whereas irrigated maize had the lowest gross margin of (R 666.89)/ha−1.
In Limpopo, the crop that ranked first on NPVs was groundnut, with NPVs of R 9 052 222 and R 8 624 326 at discount rates of 8 and 10%, respectively. The CBR for groundnut was also highest, at 2.22. Low yield maize ranked last in terms of the NPV, scoring values of –R 4 163 894 and –R 3 953 393 at discount rates of 8 and 10%, respectively. The BCR for low-yield maize was 0.44, the lowest of all the crops. In the Free State province, groundnut also ranked highest in NPVs with discounted net margins of R 8 522 689 and R 8 089 619 at discount rates of 8 and 10%, respectively. The same crop had an exceedingly high CBR of 6.70. Irrigated-yield maize had ranked last in NPVs by a wide margin, scoring NPVs of –R 4 361 237 and –R 4 142 050. Low-yield maize ranked in terms of the BCR, scoring 0.76.
Looking at the crop water requirement and environmental conditions suitable for production for ease of production, Table 6 shows suitable parameters for crop growth. Changes in this dynamic will cause a shift in the area of production. As projected by climate change statistics, increasing temperatures in the Free State and Limpopo areas will cause water scarcity. This, therefore, means crops more suitable to drought conditions will not shift. Consequently, we assumed that stakeholders would be more likely to adopt this option of grains in areas ideal for optimum productivity. Sunflower, soybean, groundnut, and sorghum have similar uses as maize (Table 7) and can be a substitute for maize.
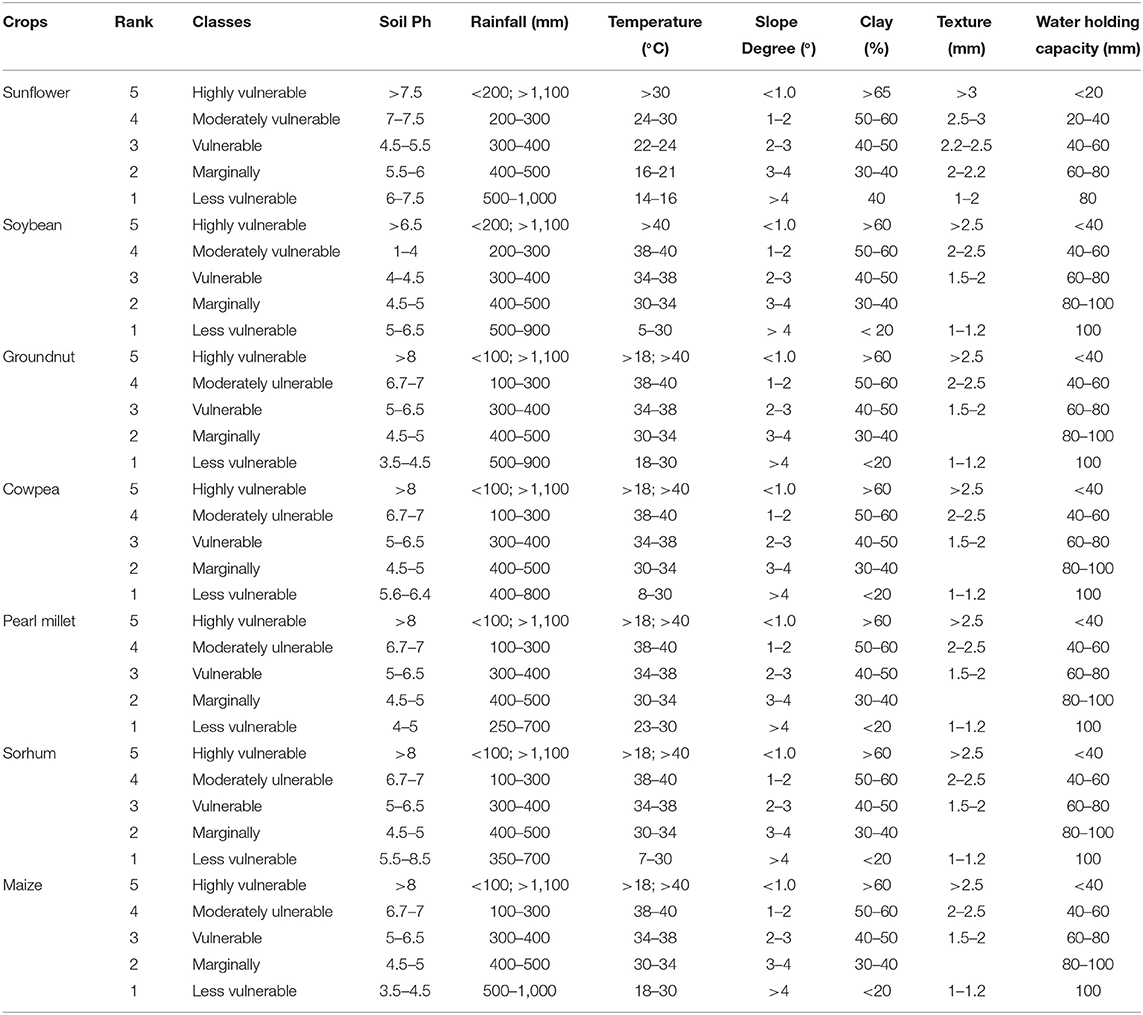
Table 6. Key crop parameters for crop production (Adapted from DAFF -https://www.daff.gov.za/Daffweb3/Portals/0/Brochures%20and%20Production%20guidelines/Production%20Guidelines%20Indigenous%20food%20crops.pdf).
Discussion
The targeting and prioritizing framework considered multiple dimensions, including economic analyses, associated social considerations (social acceptability of the choice crop), and farmers and various stakeholders' priority goals. Applying the framework, using a comprehensive approach proved fruitful. The processes' final results are specific to the selected case study areas, but notwithstanding, a similar framework can be applied to crops in any area. The reflected differences between the country priority grain crop and the win-win scenario of local environmental factors have led to the suggested prioritization of less costly crops. A cursory analysis reveals that maize has a higher production cost when compared to any other crop produced in either Limpopo or Free State provinces. This result is similar to that of Mujuru and Obi (2020) who found out in the Eastern Cape, though higher profits were attained for maize, it had lower yields per hectare than cabbage. Implying therefore that cabbage had greater potential to generate more income since cabbage production has a comparative advantage over maize production. Therefore, farmers will yield higher profits if cabbage production was commercialized.
So, why do farmers, especially smallholder farmers, continue to prioritize the production of a crop with a high cost of production instead of substituting such crops with cheaper alternatives with almost the same cash value and food security guarantees? The primary reason for this is that most small-scale farmers in South Africa consider maize the most important staple and cash crop (Hlongwane et al., 2014; Moriri et al., 2015) given that it can be diverse temperatures, altitudes, land and soil types—though with quite different yields per ha. This scenario holds for subsistence, smallholder, and emerging farmers in grain-producing regions. The recognition of less cost-intensive grain crops and indigenous grains is overlooked and not given local contexts especially by farmers and local agricultural institutions. This pattern is also common with regard to vegetables. Mujuru and Obi (2020) propose that it will make economic sense if the area for maize cultivation is reduced and used to commercialize cabbage production since cabbage production has the potential to generate higher profits for rural households. Even though this proposal has monetary importance, it is simplistic given that farmers will not want to give up maize for cabbage production. Furthermore, maize can be cultivated under rainfed conditions whereas cabbage production requires irrigation systems which may be too costly for many smallholder farmers. Extension services are hence called upon to play a major role in building farmers capacities to adopt innovations that boost production (Poncet et al., 2010). Furthermore, there is less government funding for the production of such indigenous crops.
The application of processes such as the CBA is not focused on achieving one set of non-debatable recommendations based on calculations of productions, but rather in the collective interrogation of data, provocation of discussion, and networking of stakeholders around issues of indigenous grains, sustainable, socio-economic and environmentally friendly options of grains for production. It is clear that maize production is not as beneficial as it is commonly perceived by smallholder farmers (e.g., Mujuru and Obi, 2020). It is also evident that smallholder farmers can derive economic and environmental benefits from other grains' production.
Targeting and Prioritization and the Importance of Understanding Adoption Options for Grain Crops
The identification and recommendation of crops for production support the geographical targeting of options. Statistics with an option-specific recommendation can help development actors to decide which options to promote and where. Related assessments and selected potential crops can indicate if it is worth investing in a particular option at all. Moreover, an appreciation of the determinants and barriers to adoption such as institutional, economics and behavioral can point to a novel set of interventions, addressing the adoption incentives and barriers. This thought is shared by Notenbaert et al. (2016), who believe that the suitability and adoption of interventions depend on a variety of biophysical and socio-economic factors. That notwithstanding, it is crucial to consider the possibility that only a fraction of the population will likely implement the change in the choice of crops produced. But suppose such changes are linked to regional and provincial scale. In that case, it becomes easier to bridge the gaps of implementation since a more significant number of people will be involved in propagating crop-changing ideas. From the results, it has been shown that it is less expensive to produce soybean, Sorghum, and sunflower compared to maize and irrigated maize. Therefore, if these suggestions are put forward to the farmers with substantiating evidence such as cost-benefit analyses, they will have a much clearer picture of the implications of various production decisions such as that of crop choice. Producing the evidence is often at least as necessary as the actual information produced (Notenbaert et al., 2016).
The Choice of Approach and Methods
A variety of methods can be used to assess crop priority when using a generic framework. When informing farmers' decision-making processes about their choice between different crop options, it is good to provide them with enough evidence such as results of the comparisons of the options in terms of, for example, production cost, expected yield increases, suitability, and sustainability of the production of said choice to future climate conditions. To farmers, it is often only the impacts at the local level that are of interest to them, as indicated by Notenbaert et al. (2016). Hence, they will be satisfied when shown qualitative comparisons of the various crops only with a simple ranking of options. The cost of multiple inputs for grain production, for maize, soybean, groundnuts, sunflower, is varied (Tables 1–4). Showing farmers this can go a long way in influencing their choice of crops.
Furthermore, yield outputs and the break-even price and yield will also help put things into perspective when farmers consider various crops for production. This corroborates the results of the study by Ochola and Kosura, 2006. They found out that tobacco had the lowest returns per acre in the Nyanza area of Kenya when compared to other commercial crops. Also, it was discovered that farmers were willing to shift from tobacco to other crops if the introduced crop had an assured market, opportunities for credit to purchase farm inputs, technical support and if the crop could also be used for home consumption.
Furthermore, development actors, such as governmental and non-governmental organizations, would equally be interested in potential impacts due to changes in crop production. Of specific interest to farmers will be to assess recommended crop options and the implications for sustainable growth and food security. Policymakers and policy analysts often look for aggregate numbers to justify switching and promoting one crop production over another. Quantitative techniques such as the CBA were applied to show the cost of producing one crop over the other due to the cost of production and possible profit margins. It is essential to consider that the magnitude of NPV is also an additional factor that farmers consider during the adoption decision of any technology (Thierfelder et al., 2013) and can stretch to crop production options. The BCR is an important appraisal indicator that the farmers cannot ignore because it makes it possible for farm managers to compare different investments' performances.
Chi and Bunker (2021) caution that even though CBAs are immensely popular in investment analyses, one must never ignore its most glaring shortcoming: the inability to incorporate non-financial information. This is particularly true of this study since national and provincial governments play a significant role in small-scale agriculture in terms of choice of crops (creating a least of priority crops) and access to finance for the production of these crops. Various governmental and non-governmental institutions have programmes to support farmers such as the Department of Agriculture, Fisheries and Forestry (DAFF)'s Programme; Comprehensive Agricultural Support Programme (CASP) together with Revitalization of smallholder Irrigation and Schemes (RESIS). However, the extent of this role's impact simply cannot be accurately monetized, and therefore cannot be adequately evaluated. Williams et al. (2020) draw particular attention to the inaccuracies that may arise because of the uncertainties surrounding climate change's impact on agricultural practices and investments. Hence, inferential statistics fill the gap here, where the farmers' perceptions of the impacts of various social institutions and climatic factors affecting their production are accounted for.
Through the provision of these results and showcasing possible crop options, we aimed to support evidence-based discussions of alternative production practices with regards to the choice of crops grown in the summer rainfall areas and the possible relook at some of the grains grown in the areas that would otherwise have been completely overlooked.
It is also essential to incorporate potential future impacts to provide evidence of the suitability and sustainability of the longer-term sustainability options. Projected climate change scenarios, climate variability, adaptation, and mitigation options will also necessitate testing the options' suitability, adaptability and impacts under different scenarios of future change and variability. The most important way of ensuring that crop production stays sustainable is to ensure that crops and cropping systems match the location's climate (Nadler, 2007). This will help provide some evidence about the options' robustness, which could be an essential consideration to be considered by the decision-makers.
Our findings support previous thinking that the application of a framework with various methodologies, can somethings be challenging. For example, Meuwissen et al. (2019), just like in this study found that while research at a micro-unit of the farming system is relevant and close to actors' perceived reality, data collection on some indicators at this system level can be cumbersome given that farming systems sometimes do not converge with administrative areas as seen in Figure 1.
Stakeholder Involvement
The evidence produced in the CBA analysis aimed to influence farmers and decision-makers to eventually change agricultural practices in terms of choices of crops grown. The agricultural sector has several stakeholders as well as different policy levels, each with diverse interests. Involving various stakeholders in the initial assessment stages made the study easy and feasible, given that they were knowledgeable and very informed about the various crops produced. This is in collaboration with the recommendations of Notenbaert et al. (2016). They opine that stakeholders' involvement will help raise awareness and create support for the issue and possible solutions to problems that might arise because stakeholders will be part of the process. As such, it reduces the risk of the recommendations not being carried out. Involving relevant stakeholders, especially the farmers, will encourage learning and consequently refine analysis and results to build a more in-depth understanding, increase levels of trust and buy-in from the stakeholders (Notenbaert et al., 2016). Key stakeholders were able to provide necessary information and support on various stages of the project. Farmer selection and focus group interviews were facilitated by various agriculture departments and other entities dealing within the agricultural sector.
Recommendations and Policy Implications
It is recommended that:
1. When informing the farmers' decision-making processes, especially about the type of crops to produce, it is necessary to provide adequate comparisons of best-fit production options to enable farmers to make correct choices. Such evidence may be in the form of, for example, expected yield, increases in yield, cost of production, profitability, and suitability to future climate conditions. Presenting this evidence to the farmers will reassure them of the credibility of the suggestions and they will feel the final decisions were their choices and as such, the change was not imposed on them.
2. Given the nature of the changing biophysical and socioeconomic environment, it is necessary to validate the suitability, adoption, and impacts of the options under different scenarios of future change and variability. This will provide some evidence about the robustness of the options, which could be an essential consideration to be considered by the decision-maker.
3. Interventions regarding changes in the type of crops produced in an area should be carefully designed and targeted, with a better understanding of how to carry out sensitization in a particular area. Given that aspects of institutional, economics and behavior are likely to play a role in the final decision of changing crops produced, overcoming such barriers should be integrated into the process of prioritization and how to overcome such.
4. Further studies should be carried out to determine farmers' willingness to switch crops produced and the expected timeframe within which decisions canbe influenced.
5. Some costs have been omitted such as land price, storage costs, fencing and others, which can all contribute to the initial capital and calculation of the internal rate of returns in future research.
6. Economic benefits can easily be monetized, unlike environmental costs, which can be given monetary values using indirect methods such as contingent valuations and benefit transfers. These benefits and costs can be discounted so as to get their present values. We recommend further research with the addition of these quantifications.
Conclusion
A range of technological, institutional, and policy options exist to guide the choices for crop production. The choice of crop to produce has various environmental and economic impacts and cost implications. Identifying the appropriate choice of crop to grow and the requisite interventions needed in the face of climate variability and climate change requires tradeoffs across various levels from farmers to the national level. As such, decision-makers have to make considerations regarding what is appropriate for a given context when choosing, advising on, or assisting with production and management decisions at farm levels.
The targeting and prioritization exercise was intended to influence farmers and policymakers' decision-making to eventually implement agricultural practices that are beneficial to farmers in terms of the type of crops produced. In areas facing acute water scarcity, drought-tolerant crops that are cost-effective should be grown. At the same time, alternative crops could be alternated with main grain crops depending on the farmers' production cost. It is futile to continue producing crops that are very expensive while there are cheaper options that can still be beneficial to farmers. In the two provinces sunflower, soybean, grain sorghum, and groundnuts are crop options that can reduce food insecurity and reduce the strain on natural resources due to resource-demanding crops' production. The options show potential in reducing the water demand for agricultural production. Improving efficiency and reducing the demand for natural resources could be achieved by targeting and prioritizing less cost demanding crops and changing existing practices. Thus, more focus must be directed toward research aiming to help farmers and governments cope with climate change. However, farmers understanding of benefit of production and crop choices cannot be overlooked since they make other considerations other than just economic costs in their choice of crops to produce.
The targeting and prioritization framework presented here provides the basis for integrated decision making processes aimed at enhancing the profitability of smallholder farming systems. This framework brings a novel approach in decision making in that:
• The concept of targeting and prioritization is multi-faceted and cannot be captured by a single indicator or by looking only at the attributes of a farming system or the capacities of selected actors. This framework therefore requires and enables an elaborate diagnosis of the profitability of a production process of a farming system by considering its multiple and changeable functions, its internal and external interdependencies and the full range of potential challenges. This allows for a nuanced assessment, e.g., the analysis might find an environment that constrains resilience to social and economic challenges and enhances resilience to ecological challenges, or vice versa.
• The framework capacities of robustness, adaptability, transformability can help to assess a range of possible crop options, strategies for analysis and allow for the investigation of trade-offs and synergies between them.
• The consideration of place-specific attributes grounded in multiple processes enables a reflection on trade-offs across the farming and levels.
• The framework can be used for ex-post or ex-ante analysis.
Data Availability Statement
The raw data supporting the conclusions of this article will be made available by the authors, without undue reservation.
Ethics Statement
The studies involving human participants were reviewed and approved by University of Limpopo. The patients/participants provided their written informed consent to participate in this study.
Author Contributions
All authors listed have made a substantial, direct, and intellectual contribution to the work and approved it for publication.
Funding
This work was funded through the University of Limpopo (UL) and the VLIR-UOS collaboration.
Conflict of Interest
The authors declare that the research was conducted in the absence of any commercial or financial relationships that could be construed as a potential conflict of interest.
Publisher's Note
All claims expressed in this article are solely those of the authors and do not necessarily represent those of their affiliated organizations, or those of the publisher, the editors and the reviewers. Any product that may be evaluated in this article, or claim that may be made by its manufacturer, is not guaranteed or endorsed by the publisher.
References
Andrieu, N., Sogoba, B., Zougmore, R., Howland, F., Samake, O., Bonilla-Findji, O., et al. (2017). Prioritizing investments for climate-smart agriculture: lessons learned from Mali. Agricult. Syst. 154, 13–24. doi: 10.1016/j.agsy.2017.02.008
ARC-ISCW Agroclimatological Databank (2010). GIS database. Institute for Soil, Climate and Water. Agricultural Research Council, Arcadia, Pretoria, South Africa.
Azumah, S. B., Adzawla, W., Osman, A., and Anani, P. Y. (2020). Cost-benefit analysis of on-farm climate change adaptation strategies in Ghana. Ghana J. Geogr. 12, 29–46. doi: 10.4314/gjg.v12i1.2
Badolo, F.. (2017). Cost and benefit analysis of cropping systems for sorghum and maize production under the Africa RISING project in Mali.
Benke, K. K., Steel, J. L., and Weiss, J. E. (2011). Risk assessment models for invasive species: uncertainty in rankings from multi-criteria analysis. Biol. Invas. 13, 239–253. doi: 10.1007/s10530-010-9804-x
Billore, S. D., Sharma, P., and Dupare, B. U. (2020). Break-even yield and cost of cultivation of different soybean varieties – an analysis. Soybean Res. 18, 60–68.
Bureau for Food Agricultural Policy (BFAP) (2018). Agricultural Outlook 2018 - 2027. Available online at: http://www.bfap.co.za/wp-content/uploads/2018/08/BFAPBaseline-2018.pdf
Campbell, B. M., Vermeulen, S. J., Aggarwal, P. K., Corner-Dolloff, C., Girvetz, E., Loboguerrero, A. M., et al. (2016). Reducing risks to food security from climate change. Glob. Food Security 11, 34–43. doi: 10.1016/j.gfs.2016.06.002
Chi, S., and Bunker, J. (2021). An Australian perspective on real-life cost-benefit analysis and assessment frameworks for transport infrastructure investments. Res. Transport. Econ. 88, 100946. doi: 10.1016/j.retrec.2020.100946
Daujanov, A., Groeneveld, R., Pulatov, A., and Heijman, W. J. (2016). Cost-benefit analysis of conservation agriculture implementation in Syrdarya province of Uzbekistan. Visegrad J. Bioeconom. Sustain. Dev. 5, 48–52.
Davis, J., Tavasci, D., and Marais, L. (2006). Fostering Rural and Local Economic Development in the Free State of South Africa. Natural Resources Institute, University of Greenwich, Natural Resources Institute University of Greenwich at Medway.
Department of Environmental Affairs (DEA). (2013). Long-Term Adaptation Scenarios Flagship Research Programme (LTAS) for South Africa. Climate Trends and Scenarios for South Africa. Pretoria: Department of Environmental Affairs.
Department of Agriculture Forestry Fisheries. (2010). Abstract Of Agricultural Statistics, Pretoria, South Africa, 30 June, 2018. Available online at: https://www.Nda.Agric.Za/Docs/Statsinfo/Abstract_2010.Pdf (accessed January 29, 2020).
Dube, S., Scholes, R. J, Nelson, G. C., Mason-D'Croz, D., and Palazzo, A. (2013). South African food security and climate change: agriculture futures. Economics 7, 2013–2035. doi: 10.5018/economics-ejournal.ja.2013-35
Economic Commission for Africa (ECA) (2018). Economic and Social Council Official Records, 2018. Supplement No. 18
Economists, C.. (2014). A manual for cost-benefit analysis in South Africa with specific reference to water resource development, Vol. 8. WRC Report.
Envirotech Solutions. (2015). Let's respond toolkit. Free State Province. Available online at: https://sites.google.com/site/letsrespondtoolkit/municipalities/free-state
Esterhuizen, D.. (2019). Agricultural Biotechnology Annual: Biotechnology in South Africa. Gain Report. USDA Foreign Agricultural Service. Available online at: https://apps.fas.usda.gov/newgainapi/api/report/downloadreportbyfilename?filename=Agricultural%20Biotechnology%20Annual_Pretoria_South%20Africa%20-%20Republic%20of_2-5-2019.pdf (accessed November 21, 2019).
FAO (2009). The State of Food and Agriculture 2009. Livestock in the balance, Rome. Available online at: http://www.fao.org/3/a-i0680e.pdf
Financial and Fiscal Commission (FFC). (2011). Submission for the 2012/13 Division of Revenue Technical Report. MidDollar: Financial and Fiscal Commission.
Gbetibouo, G. A., and Hassan, R. M. (2005). Measuring the economic impact of climate change on major South African field crops: a Ricardian approach. Glob. Planet. Change 47, 143–152. doi: 10.1016/j.gloplacha.2004.10.009
Harvest Choice. (2010) Available online at: https://www.researchgate.net/deref/http%3A%2F%2Fharvestchoice.org%2Fdata%2Faez-16-class
Hasan, K., and Khalequzzaman, K. M. (2018). The economic impact of onion research and extension in Blangladesh: an ex-post analysis. Nippon J. Environ. Sci. 1, 1020. doi: 10.46266/njes.1020
Herrero, M. T., Notenbaert, A. M. O., Thornton, P. K., Pfeifer, C., Silvestri, S., Omolo, A., et al. (2014). A Framework for Targeting and Scaling-Out Interventions in Agricultural Systems. CCAFS Working Paper No. 62. CGIAR Research Program on Climate Change, Agriculture and Food Security (CCAFS), Copenhagen, Denmark. Available online at: www.ccafs.cgiar.org
Hlongwane, J., Ledwaba, L., and Belete, A. (2014). Analyzing the factors affecting the market participation of maize farmers: A case study of small-scale farmers in greater Giyani Local Municipality of the Mopani District, Limpopo Province. Afr. J. Agric. Res. 9, 895–899. doi: 10.5897/AJAR12.1896
Howden, S. M., Soussana, J. F., Tubiello, F. N., Chhetri, N., Dunlop, M., and Meinke, H. (2007). Adapting agriculture to climate change. Proc. Nat. Acad. Sci. 104, 19691–19696. doi: 10.1073/pnas.0701890104
IPCC. (2014). Climate Change 2014: Impacts, Adaptation, and Vulnerability. Part A: Global and Sectoral Aspects. Contribution of Working Group II to the Fifth Assessment Report of the Intergovernmental Panel on Climate Change, eds C. B. Field, V. R. Barros, D. J. Dokken, K. J. Mach, M. D. Mastrandrea, T. E. Bilir, M. Chatterjee, K. L. Ebi, Y. O. Estrada, R. C. Genova, B. Girma, E. S. Kissel, A. N. Levy, S. MacCracken, P. R. Mastrandrea, and L. L. White (Cambridge; New York, NY: Cambridge University Press). Available online at: http://www.ipcc.ch/pdf/assessment-report/ar5/wg2/WGIIAR5-FrontMatterA_FINAL.pdf
Kelly, C., Laird, J., Costantini, S., Richards, P., Carbajo, J., and Nellthorp, J. (2015). Ex post appraisal: what lessons can be learnt from EU cohesion funded transport projects? Transport policy. 37, 83–91. doi: 10.1016/j.tranpol.2014.09.011
Khan, K. S., Kunz, R., Kleijnen, J., and Antes, G. (2003). Five steps to conducting a systematic review. J. R. Soc. Med. 96, 118–121. doi: 10.1258/jrsm.96.3.118
Kristjanson, P., Reid, R. S., Dickson, N., Clark, W. C., Romney, D., Puskur, R., et al. (2009). Linking international agricultural research knowledge with action for sustainable development. Proc. Nat. Acad. Sci. 106, 5047–5052. doi: 10.1073/pnas.0807414106
Kurukulasuriya, P., Mendelsohn, R., Hassan, R., Benhin, J., Diop, M., Eid, H. M., et al. (2006). Will African agriculture survive climate change? World Bank Econ. Rev. 20, 367–388. doi: 10.1093/wber/lhl004
Limpopo Department of Agriculture (2012). The Mapping of Agricultural Commodity Production in the Limpopo Province, South Africa.
Mabhaudhi, T.. (2012). Drought tolerance and water-use of selected South African landraces of taro (Colocasia esculenta L. Schott) and bambara groundnut (Vigna subterranea L. Verdc) (Doctoral dissertation). College of Agriculture, Engineering and Science University of KwaZulu-Natal Pietermaritzburg South Africa.
Magati, W. O. P. O., Kibwage, J. K., Omondi, S. G., and Ruigu, G. (2012). A cost-benefit analysis of substituting bamboo for tobacco : a case study of smallholder tobacco farmers in South Nyanza, Kenya. Sci. J. Agri. Res. Manag. 1–9. doi: 10.7237/sjarm/204
Meuwissen, M. P., Feindt, P. H., Spiegel, A., Termeer, C. J., Mathijs, E., de Mey, Y., et al. (2019). A framework to assess the resilience of farming systems. Agric. Syst. 176, 102656. doi: 10.1016/j.agsy.2019.102656
Moeletsi, M. E.. (2010). Agroclimatological risk assessment of rainfed maize production for the Free State Province of South Africa (Doctoral dissertation). Department of Soil, Crop and Climate Sciences, Faculty of Natural and Agricultural Sciences, Bloemfontein, South Africa.
Moeletsi, M. E., and Walker, S. (2013). Agroclimatological suitability mapping for dryland maize production in Lesotho. Theoret. Appl. Climatol. 114, 227–236. doi: 10.1007/s00704-012-0829-1
Moriri, S., Owoeye, L., and Mariga, I. (2015). Evaluation of maize nutrient contents in a maize/cowpea intercropping systems in South Africa. Adv. Plants Agric. Res. 2, 00068. doi: 10.15406/apar.2015.02.00068
Moswetsi, G., Fanadzo, M., and Ncube, B. (2017). Cropping systems and agronomic management practices in smallholder farms in South Africa: constraints, challenges and opportunities. J. Agron. 16, 51–64. doi: 10.3923/ja.2017.51.64
Mujuru, N. M., and Obi, A. (2020). Effects of cultivated area on smallholder farm profits and food security in rural communities of the Eastern Cape Province of South Africa. Sustainability 12, 3272. doi: 10.3390/su12083272
Mullins, D., Botha, J. P., Mosaka, D. D., Jurgens, F. X., and Majoro, T. J (2014). A manual for cost benefit analysis in south africa with specific reference to water resource development, 3rd Edn. WRC Project No. K8/1044.
Mutenje, M. J., Farnworth, C. R., Stirling, C., Thierfelder, C., Mupangwa, W., and Nyagumbo, I. (2019). A cost-benefit analysis of climate-smart agriculture options in Southern Africa: balancing gender and technology. Ecol. Econ. 163, 126–137. doi: 10.1016/j.ecolecon.2019.05.013
Nadler, A. J.. (2007). An agro climatic risk assessment of crop production on the Canadian Prairies (M.Sc. thesis). Department of Soil Science, University of Manitoba, Manitoba.
National Agricultural Marketing Council (2020). Agricultural sector contribution to South Africa's Gross Domestic Product (GDP). Available online at: https://www.namc.co.za/wp-content/uploads/2020/12/Press-Release-GDP-MERC.pdf
Njenga, K. I.. (2016). Cost-benefit analysis of substituting banana for coffee in muranga county, Kenya (Ph.D. dissertation). University of Nairobi, Nairobi, Kenya.
Notenbaert, A., Pfeifer, C., Silvestri, S., and Herrero, M. (2017). Targeting, out-scaling and prioritising climate-smart interventions in agricultural systems: Lessons from applying a generic framework to the livestock sector in sub-Saharan Africa. Agric. Syst. 151, 153–162. doi: 10.1016/j.agsy.2016.05.017
Notenbaert, A. M. O., Lannerstad, M., Barron, J., Paul, B. K., Ran, Y., Morris, J., et al. (2016). Using the CLEANED approach to assess the environmental impacts of livestock production. Livestock and Fish Brief .
Ochola, S., and Kosura, W. (2006). Case Study on Tobacco Cultivation and Possible Alternative Crops-Kenya, Study.
Odeck, J., and Kjerkreit, A. (2019). The accuracy of benefit-cot analyses (BCAs) in transportation: an ex-post evaluation of road projects. Transport. Res. 120, 277–294. doi: 10.1016/j.tra.2018.12.023
Oni, S. A., Nesamvuni, A. E., Odhiambo, J. J., and Dagada, D. C. (2012). Executive Summary of a Study of Agricultural Industry of Limpopo Province. South Africa: Centre for Rural Development and Department of Soil Science.
Poncet, J., Kuper, M., and Chiche, J. (2010). Wandering off the paths of planned innovation: the role of formal and informal intermediaries in a large-scale irrigation scheme in Morocco. Agric. Syst. 103, 171–179.
Rotmans, J., and van Asselt, M. B. (1999). Perspectives on a sustainable future. Int. J. Sustain. Dev. 2, 201–230.
Sain, G., Loboguerrero, A. M., Corner-Dolloff, C., Lizarazo, M., Nowak, A., Martínez-Barón, D., and Andrieu, N. (2017). Costs and benefits of climate-smart agriculture: The case of the Dry Corridor in Guatemala. Agri. Syst. 151, 163–173. doi: 10.1016/j.agsy.2016.05.004
Schulze, R. E.. (2008). South African Atlas of Climatology and Agrohydrology: WRC.Report 1489/1/08. Water Research Commission, Pretoria, Russia.
Schulze, R. E.. (2016). “Water and the farmer 1: challenges in South Africa even before considering climate change,” in Handbook for Farmers, Officials and Other Stakeholders on Adaptation to Climate Change in the Agriculture Sector within South Africa. Section B: Agriculture's Natural Capital in South Africa: A Climate Change Perspective, Chapter B6, ed R. E. Schulze.
Statistics South Africa (2012). Census 2011. Available online at: https://scholar.google.com/scholar_lookup?author=Statistics+South+Africa.&journal=Census+2011&publication_year=2012
Statistics South Africa (2017). Census of Commercial Agriculture 2017, Report no. 11-02-01. Pretoria: Statistics South Africa (2017).
Tafa, S.. (2017). Farm level cost-benefit analysis of conservation agriculture for maize smallholder farmers in Okhahlamba Municipality in Kwa-Zulu Natal Province, South Africa. University of Fort Hare, Alice, South Africa.
Thierfelder, C., Mwila, M., and Rusinamhodzi, L. (2013). Conservation agriculture in eastern and southern provinces of Zambia: long-term effects on soil quality and maize productivity. Soil Tillage Res. 126, 246–258. doi: 10.1016/j.still.2012.09.002
Turpie, J., and Visser, M. (2013). The impact of climate change on South Africa's rural areas. Financ. Fiscal Commiss. 14,100–160.
Ubisi, N. R.. (2016). Smallholder farmers' perceptions and adaptation to climate change interventions and support systems in Limpopo province, South Africa (Master Dissertation.) University of KwaZulu-Natal, Pietermaritzburg.
Williams, P. A., Ng'ang'a, S. K., Crespo, O., and Abu, M. (2020). Cost and benefit analysis of adopting climate adaptation practices among smallholders: the case of five selected practices in Ghana. Clim. Serv. 20, 100198. doi: 10.1016/j.cliser.2020.100198
Keywords: agricultural productivity, Benefit-Cost Ratio, cost-benefit analysis, crop production, Net Present Value, targeting, prioritization, sustainability
Citation: Kephe PN, Siewe LC, Lekalakala RG, Kwabena Ayisi K and Petja BM (2022) Optimizing Smallholder Farmers' Productivity Through Crop Selection, Targeting and Prioritization Framework in the Limpopo and Free State Provinces, South Africa. Front. Sustain. Food Syst. 6:738267. doi: 10.3389/fsufs.2022.738267
Received: 08 July 2021; Accepted: 14 February 2022;
Published: 29 March 2022.
Edited by:
La Zhuo, Northwest A&F University, ChinaReviewed by:
Zhang Yucui, Chinese Academy of Sciences (CAS), ChinaBridget Bwalya Umar, University of Zambia, Zambia
Copyright © 2022 Kephe, Siewe, Lekalakala, Kwabena Ayisi and Petja. This is an open-access article distributed under the terms of the Creative Commons Attribution License (CC BY). The use, distribution or reproduction in other forums is permitted, provided the original author(s) and the copyright owner(s) are credited and that the original publication in this journal is cited, in accordance with accepted academic practice. No use, distribution or reproduction is permitted which does not comply with these terms.
*Correspondence: Priscilla Ntuchu Kephe, a3ByaXNzeUBnbWFpbC5jb20=