- Department of Agricultural Economics, Universidad de Talca, Talca, Chile
During the last decade, food loss and waste (FLW) has been gaining more attention due to its negative effect on food security. However, the lack of information about FLW quantification and characterization remains a problem, especially from the perspectives of local citizens and farmers. There is limited literature examining food losses (FL) in primary production of the food supply chain (FSC) and specific policies are needed to improve the FLW measurement. The aim of this research is to analyze how much FL is generated at the farm level using a micro-approach methodology from harvest to primary commercialization stages among farmers located in Central Chile. Additionally, we explore factors affecting FL using a fractional regression model with special emphasis on the harvest stage. Data were collected using phone interviews, conducted in 2019, with 177 small-scale producers of vegetables and berries. FL generated by the sample from harvest to primary commercialization was 14.5% on average. Farmers identified a considerable volume of FL during primary production, mostly during the harvest. The factors that increased FL among small-scale farmers were the production system and its harvest period, commercialization channels, labor shortage, and cosmetic standards. As a case study, the information collected here can be useful for encouraging further research emphasizing the harvest stage and the role of the production systems in generating FLW.
Introduction
Before the COVID-19 pandemic, the Food and Agriculture Organization (FAO) estimated that roughly 8.9% of the world's population (690 million people) is undernourished. Also, at least 2 billion people suffer “food insecurity” at moderate or severe levels due to the lack of access to quality and nutritious food (FAO, 2020). The global population is expected to increase by 10 billion people by 2050 (FAO, 2019) and will put pressure on agriculture to be more sustainable (Alexander et al., 2017). Recent studies of productivity growth reveal that the agricultural sector's progress has been stalled mainly due to climate issues, a problem that is exacerbated if food is not completely consumed (Future Foods, 2022).
Food loss and waste (FLW) impacts food security by reducing the availability of local food that is appropriate for human consumption (FAO et al.,, 2019). Food access for food supply chain (FSC) stakeholders is also negatively affected. For instance, the farmers may experience income losses due to high levels of FLW. Additionally, consumers are impacted by rising prices caused by the reduction of food supply due to FLW (HLPE, 2014). One-third of the global food produced is lost or wasted across the FSC (Gustavsson et al., 2011). FLW globally represents 24% of water, fertilizers, and farmland that are employed in agricultural production (Kummu et al., 2012), and 8% of the world's greenhouse gas (GHG) emissions (FAO, 2014). The economic loss attributed to FLW is estimated by FAO to be one trillion USD per year (FAO, 2014).
Discarding good quality food at any stage of the FSC implies that output and inputs are being wasted, namely, valuable calories and nutrients, labor, energy, and natural resources (Corrado et al., 2017). Therefore, to improve the efficiency and sustainability of the food system, FLW needs to be evaluated from social, economic, and environmental points of view. An important obstacle to standardizing FLW information is the application of different quantification methodologies among studies and the lack of agreement in the use of a distinct procedure, which have generated a gap in data precision (Bellemare et al., 2017; van der Werf and Gilliland, 2017). Furthermore, definitions also fragment FLW analysis: “food loss” (FL) is defined as good quality food that is discarded during the first stages of production, whereas “food waste” (FW) corresponds to good quality food that is not consumed during the second part of the FSC from the point of view of the retail stage (FAO, 2014). Obtaining information about the real scope of FLW is a central challenge that must be faced before formulating any policy or taking action regarding saving food (Delgado et al., 2021). However, given the heterogeneity that characterizes the food industry in different countries, it is necessary to establish boundary systems to facilitate data processing and subsequent FLW quantification (Chaboud, 2017). Regarding this matter, the recent FAO study (2019) openly discusses the dichotomy between, on the one hand, the aggregated percentage number of the Food Loss Index and, on the other, the call for specificity and precision in shaping policy measures, based on cost/benefit analyses (Koester and Galaktionova, 2021).
In low- and middle-income countries, a significant part of food is produced by small-scale farmers who operate under production limits (Fabi et al., 2021). In those countries, the role of FLW is crucial since it is commonly linked with farm management and technical and financial issues (Liu, 2016). Since FLW can negatively affect farmers' income (Delgado et al., 2021), proper research and agricultural extension seem necessary to develop strategies to reduce and/or prevent FLW, and to find crucial areas to be improved in the food production system.
The data available for the measurement of FLW are focused on FSC stages, and few studies on primary production can be found (Teuber and Jensen, 2020). Data scarcity can be attributed to the lack of agreement on FLW definitions, the diversity of food products (Stenmarck et al., 2016), and the lack of information about how FLW affects business and society (Liu, 2016). Accurate data about FL on farms can be beneficial for policymakers because it can fill the gap in the debate around food producers and their role in FLW, and it can provide pivotal information to make better decisions regarding the matter (Johnson et al., 2018).
In 2015, Chile adhered to the UN's 2030 Agenda for Sustainable Development and, 2 years later, the Agricultural Ministry's Office of Agricultural Studies and Policies (ODEPA) created the National Committee for the Prevention and Reduction of Food Losses and Waste. Despite the current effort, there is still a lack of national data that can support the calculation and quantification of FLW (Eguillor and Acuña, 2019) which is crucial to understanding the FLW scenario and to implementing suitable political actions.
Few studies of food loss using quantification methodology and data are found in Chile, yet these are needed for policy decisions given the heterogeneity of agricultural products along the FSC. Thus, the objectives of this study are 2-fold: (1) to estimate how much FL is generated during primary production using a micro-approach methodology from harvest to primary commercialization stages in Chile and (2) to understand the factors affecting FL with special emphasis on the harvest stage. Data collection is achieved through surveys conducted during 2019 among small-scale producers that belong to family farms that produce berries or vegetables. The producers are beneficiaries of the extension program Technical Advisory Service (SAT) supported by the National Institute for Agricultural Development (INDAP). Farmers who are beneficiaries of INDAP in Chile are called Family Farm Agriculture (FFA) and contribute 22% of the agricultural gross domestic product (AGDP), own 38% of the irrigated area, and hire 33% of agricultural employees (INDAP, 2016).
1.1. Boundary System
The High-Level Panel of Experts (HLPE) on food security and nutrition emphasizes that standardizing FLW measurement and FLW definitions is crucial to enhancing information accuracy (HLPE, 2014). Currently, an international agreement on a single definition of FL and FW is still lacking (Teuber and Jensen, 2020). According to Delgado et al. (2017), even though the terms “FL” “Post-Harvest Loss” (PHL), “FW”, and “FLW differ from each other, they can be used as equivalents in the literature. FAO definitions are the most regularly used due to the organization's initial effort in 2014 to summarize the existent terminology and definitions (Corrado et al., 2017). FL is defined as “the decrease in the quantity or quality of food resulting from the decisions and actions by food suppliers in the chain (…) and occurs from post-harvest up to, but not including the retail level”, while FW is defined as “the decrease in the quantity or quality of food resulting from decisions and actions by retailers, food service providers, and consumers”. The sum of total FL and food waste that occurs along a value chain is included in the FLW term (FAO, 2014).
Regarding FL, the losses during the pre-harvest and harvest stages are not considered by this definition because of the challenges of quantifying food discarded during agricultural procedures (FAO, 2019). Although the Global Food Policy Report (IFPRI, 2016) shows the relevance of preharvest losses in its proposed methodology to get a complete overview of FLW, most of the definitions suggested by the latest research define FLW from the harvest stage of the process onward (Teuber and Jensen, 2020).
Proper quantification of FLW requires boundaries that facilitate the studies, treatments, and subsequent interpretation of collected information. Thus, each study should specify the food category and stage of the FSC selected according to the methodological scope and the geographical location of the study (Hanson et al., 2016). The rest of this article is structured as follows: Section Quantification methods presents a discussion of quantification methods; Section Methods is dedicated to explaining the data, area of study, and the elucidation of the econometric model; Section Results presents the results of the study; and, finally, Sections Discussion and Conclusion present the discussion and conclusion of the research.
2. Quantification methods
Quantification methods can be classified as “macro” or “micro” approaches given the variability of FLW (Delgado et al., 2017). The former describes methods that analyze a broad perspective of FLW at the global or regional level, and it can be achieved by contrasting non-processed inputs to final production, using records of mass balances measured by weight or caloric content. For example, Parfitt et al. (2010), using an old data set from the 70s to 80s, suggested that a range of 10 to 40% of total production is lost worldwide. Kummu et al. (2012) analyzed global FLW in kcal terms and natural resources such as freshwater, cropland, and fertilizers; and Lipinski et al. (2013) used FAOSTAT data to quantify the calories that are not being consumed, classifying FLW according to food commodities groups.
While the study of Gustavsson et al. (2011) provides a broad perspective of the FLW with “regional” estimates and suggests general guidelines, it is still crucial that each country creates its own database. Several country-specific reports have been published, mainly in the United States and European countries that fit into the “macro” approach category, using mass balance data and specific assumptions about production yields to understand the current scenario (Delgado et al., 2017). The “macro” approach is limited by the lack of representativeness that results from using incomplete and obsolete data, which reduces its utility for planning actions to prevent and reduce the FLW (Thyberg and Tonjes, 2016; Delgado et al., 2017). Most recently, the FAO report presents the advance on SDG target 12.3 and its 12.3.1 indicator: “Global FLW”, which was divided into two sub-indicators: Food Loss Index (FLI, 12.3.1a) and Food Waste Index (FWI, 12.3.1b). The development of FLI led to the first estimation of global FL, reporting that, in 2016, 13.8% of the food produced in the world was lost (FAO, 2019). Regarding FWI, in 2019, approximately 931 million tons of food waste were generated in the world, which is twice the amount estimated by FAO in 2011 (UNEP, 2021).
Methods classified as “micro” approaches are characterized by the specificity of the data since they are focused on particular locations and contexts of the FSC. APHLIS (2014) estimates that in Sub-Saharan Africa, between 14.4 and 15.8% of cereal crop production is lost during post-harvest (Hodges et al., 2014). Hartikainen et al. (2017) estimated 800,000 tons of food lost in primary production per year in Finland, Sweden, Norway, and Denmark. The mechanisms to achieve “micro” approach research can be on-site measurements, stakeholder records, interviews, and surveys—all of which can be beneficial in focusing research on specific objectives but which are often time-consuming and costly processes (Delgado et al., 2017). Therefore, measurement settings should be organized according to the specific FSC scenario (Fabi et al., 2021).
The majority of FLW publications reporting on low- and middle-income countries focus on the first stages of FSC (Thyberg and Tonjes, 2016). Most of them are from Asia or Africa, while Latin America and the Caribbean countries (LACs) are underrepresented in the literature (Xue et al., 2017), even though they contribute almost 20% of the FLW produced in the world (FAO, 2019). LACs have asserted their concerns about FLW, making the commitment to reduce their FLW by 50% per capita by 2025 (FAO, 2016). With this objective, a Regional Alliance of LACs formed by National Committees supported by FAO has been created to work in their respective countries. The alliance is composed of 10 nations, including Chile. However, few initiatives related to FLW have been developed in Chile, particularly studies that evaluate production chains (either encompassing all or certain stages) of specific commodities. For example, the National Agricultural Research Institute (INIA) has studied techniques that enhance the postharvest life of fresh and processed vegetables to reduce FLW during the packing, storage, and transport stages (Eguillor and Acuña, 2019). In terms of legal regulations, a specific normative that regulates FLW is absent. Still, two bills seek to modify the Food Code to avoid waste at the commercialization stage (Eguillor, 2017).
At the institutional level, ODEPA announced in 2017 the formation of the National Committee for the Prevention and Reduction of Food Losses and Waste (CN-PDA): a public-private partnership with the aim of creating an appropriate legal framework, public policies, and concrete actions to prevent and reduce FLW. In addition, the committee encourages FLW quantification and raising public awareness (Eguillor and Acuña, 2019). In an important step toward this, the Chilean Ministry of Agriculture has already accepted the request for CN-PDA institutionalization (ODEPA, 2020). However, the actual amount of FLW produced by the vegetable and livestock product supply chains in Chile remains undetermined. Therefore, significant efforts should be directed at FLW data collection. Such information is crucial to improve decision-making at the production level, reduce FLW, and facilitate the operation of national committees (Eguillor and Acuña, 2019).
The lack of research on FL is counterintuitive since collecting data at the farm level is a valuable resource for improving agriculture management and efficiency (Johnson et al., 2018). According to Beausang et al. (2017), data scarcity on primary production may be caused by the poor habit of farmers to record FL amounts during the production process. In Europe, Stenmarck et al. (2016) estimated that FL levels across the FSC and primary sector were found to be the most complicated stages to quantify. One reason is the diversity of crops produced (e.g., wine grapes, fruits, vegetables), leading to highly variable degrees of FL (Stenmarck et al., 2016). Hence, to calculate FL, it is necessary to carry out specific and comprehensive evaluations of each food chain related to primary production and its respective stages (Beretta et al., 2013). Another challenge is related to what farmers understand as FL (Franke et al., 2016). Many producers recognize that an un-marketable edible food could be used as animal feed or organic matter for the soil, even though this could imply an economic loss, and it could be considered an FL. Also, an unrecognized environmental cost is triggered due to the use of energy involved in agricultural production (Hartikainen et al., 2017).
According to Eguillor (2019), Chile is not an exception, and one of the main problems with quantifying FL is the lack of records by farmers. Therefore, actions are being taken to explore ways in which losses can be quantified. An exploratory study estimated that 16,550 units per hectare of lettuce and 1.1 tons per hectare of potatoes are lost during harvest, in addition to 1.1 tons per hectare during storage (Eguillor, 2019). Additionally, the project called “Measurement and management of fruits and vegetable losses on production stages at national level in Chile” was implemented in 2019 to validate a quantification methodology in four specific products along the FSCs: lettuce, tomato, potato, and raspberry. The results yielded a methodology that measures production system losses of fruits and vegetables (Eguillor and Acuña, 2019; Giraldo et al., 2019). The “Guide to prevent and reduce vegetable and fruit losses” provides definitions of FLW and explains the relevance of FL prevention with simple terminology. With this objective, the guide mentions a list of suggestions to farmers regarding planning, crop management, and critical points during harvest, post-harvest, storage, and transportation (ODEPA, 2019).
3. Methods
3.1. Data and study area
We estimated how much FL is generated during primary production using a micro-approach from harvest to primary commercialization stages in two agricultural areas of Central Chile: Maule (34°41' S) and Ñuble (36°43' S) regions. Both regions contribute 13.9 and 14.2% respectively to the country's Gross Domestic Product for forestry and agriculture, and together represent approximately 34% of national farms and 31.5% of national hectares destined for annual and permanent crops (ODEPA, 2019).
We randomly selected a representative sample of 400 producers from the Maule and Ñuble regions. Due to the COVID-19 pandemic, our initially planned on-site interviews had to be replaced by phone surveys, which we conducted between June and September 2020. The final sample is composed of 177 vegetable and berry producers who answered the phone survey.
We applied a questionnaire using the script created by Beausang et al. (2017). Also, we used a set of questions addressed to producers used by Jara-Rojas et al. (2020). Additionally, by the “Measurement and management of fruits and vegetables losses on production stages at national level in Chile” project (Giraldo et al., 2019). The questionnaire was divided into four parts: I. Production and management records, II. Production information, III. Losses information, and IV. COVID-19 Contingency.
3.2. Methodology
For the purpose of this study, we defined Food Loss (FL) as “a decrease in the mass of edible food originally intended for human consumption. Food losses occur at the production, post-harvest, processing, and storage stages” (Giraldo et al., 2019). The FL analysis was done in the harvest, packing, transport, and primary commercialization stages of vegetable and berry supply chains.
To calculate FL volume, yield and percentage of losses generated by each crop are needed. Yield information is obtained through question 6: “Could you specify further information about your last season's crops?” in Section II of the questionnaire. To get information about FL, self- reported percentages of losses (during harvest, packing, transport, and first commercialization) were collected through question 7: “In a normal season, how much of your crops (estimate) do you lose during the next stages?” in Section III.
After obtaining yield and FL percentages information, FL volume is calculated using as a reference the Giraldo et al. (2019) project. Although the study is considered a “micro” dimension case, “medium” dimension methodology is used as a reference to calculate FL quantities because it facilitates calculation using self-reported information. The medium methodology proposes the following equation to calculate losses that occur during primary production, without the need for on-farm measurements:
where FLPP is the food losses during primary production in tons, %FL is the percentage of food loss, and TY is the total yield in tons.
According to equation [1], FL is calculated using self-reported yields and estimated percentages of losses. Production outcome coherence is corroborated using ODEPA technical data sheets for each crop.
Since the boundary system covers from harvest to the primary commercialization stage, loss calculation in the FSC is made in the opposite direction, assuming that TY is the result of the production chain after losses occur at each stage. First primary commercialization losses (the last of the studied stages) are calculated using equation 1. Next, to calculate losses of the remaining (previous) stages, FL calculated previously is added to TY, as follows:
where FLi is the food loss during the ith-stage in tons, %FLi corresponds to the percentage of food lost during stage i reported by farmers, TY is defined in [1], and FLi−n is the food loss produced during the prior stage, previously calculated, with n being the position of the stage in the FSC.
Finally, caloric and water content losses are calculated for each product, using available information on Food Data Central of the United States Department of Agriculture1 (USDA). Due to the relevance of fruits and vegetables in the human diet, fiber, and protein contents are also calculated using USDA values as reference. Furthermore, the economic evaluation of FL is carried out based on Kitinoja et al.'s (2018) simple calculation, using producer prices per kilogram given by farmers (FONDECYT 1121122 Project database) for the approximately 20 crops mentioned in the sample.
3.3. Econometric model
This section assesses the effect of sociodemographic, farm, and principal crop characteristics, as well as the most frequently mentioned causes of FL (labor problems, cosmetic standards, and weather phenomena) in the total FL amount estimated by farmers. According to Kitinoja et al. (2018), the majority of FL analyses are focused on volumetric losses and tend to omit the economic value of losses as indicators. Some reasons for this could be the lack of good quality databases and the absence of information on economic losses, which limits data comparisons or the replication of methodologies.
Since the volume of FL represents data relevant to the national context due to the lack of FLW information in Chile (Giraldo et al., 2019), FL in tons produced by farmers is set as the dependent variable and is expressed as a proportion of the food produced. Therefore, resulting values will be a fraction within a range of 0 and 1, inclusive.
Linear regression models such as Ordinary Least Squares (OLS) are inappropriate to predict fractional dependent variables. One important limitation is the inability of such a model to guarantee that its predicted values stand within the unit interval (Papke and Wooldridge, 1996). Additionally, due to the bounded nature of the variables, linear models usually present non-constant outputs of regressors variations (Ramalho et al., 2011; Gallani and Krishnan, 2015; Chegere, 2018) and negatively affect the precision of estimates (Bravo-Ureta et al., 2017).
An alternative approach to OLS may be the implementation of the Tobit model for censored data, specifically a two-limit Tobit model (Baum, 2008). According to Ramalho et al. (2011), the latter can be implemented only if the sample contains observations in both limits, which is not always the case with fractional dependent variables. Using a Tobit model only within the [0, 1] interval is difficult to support conceptually since those observations of fractional variables that lie in extremes are not a consequence of censure, but the outcome of single choices. McDonald (2009) also mentions the importance of considering that some elements of the data generating process may not be suitable with the Tobit approach, due to the fractional nature of the response variable. Additionally, this model turns out to be rigorous regarding the assumptions of normality and homoscedasticity of dependent variables (Ramalho et al., 2011).
To deal with the proportion as dependent variables, Papke and Wooldridge (1996) propose a Fractional Regression Model (FRM). For the conditional expectation of fractional dependent variable, Papke and Wooldridge (1996) assume the following model:
where G (·) is a knowing function satisfying 0 ≤ G(z) ≤ 1 for all z ϵ R, ensuring that predicted values of y lie in the [0,1] interval. The FRM is estimated using a Quasi Maximum Likelihood Estimator (QMLE) that states the simple maximization of the Bernoulli log-likelihood:
Regarding the assumptions of generalized linear models (GLM), the method suggested by Papke and Wooldridge (1996) is efficient and fully robust. Chegere (2018) implemented this approach to analyzing the role of handling practices on food loss reduction during post-harvest of maize crops, keeping the original specification of Papke and Wooldridge (1996).
Based on Papke and Wooldridge (1996) and Chegere (2018), the empirical regression model applied to the total sample (n = 177) is the following:
where SOCIODEM corresponds to a vector of sociodemographic variables (including age, level of education and agricultural experience of farmers, family size, and total area of the productive farm). Since a part of the sample includes farms that manage up to three crops, MAINCROP captures information about the main crop (whether it is grouped in vegetables or berries, harvest period, and commercialization channel). CAUSES correspond to the three most mentioned causes of FL during the harvest stage.
3.4. Descriptive statistics
Table 1 shows the descriptive statistics of the dataset of 177 farmers, for which the average age of producers is 57 years, with a mean of 8.3 years of education and 35 years of agricultural experience. The average farm size of the sample is 2.6 hectares, with a mean food production per farmer of 25.2 tons per hectare. However, there is a high variability between the minimum and maximum yield values due to the high diversity of crops and the high range of farm size.
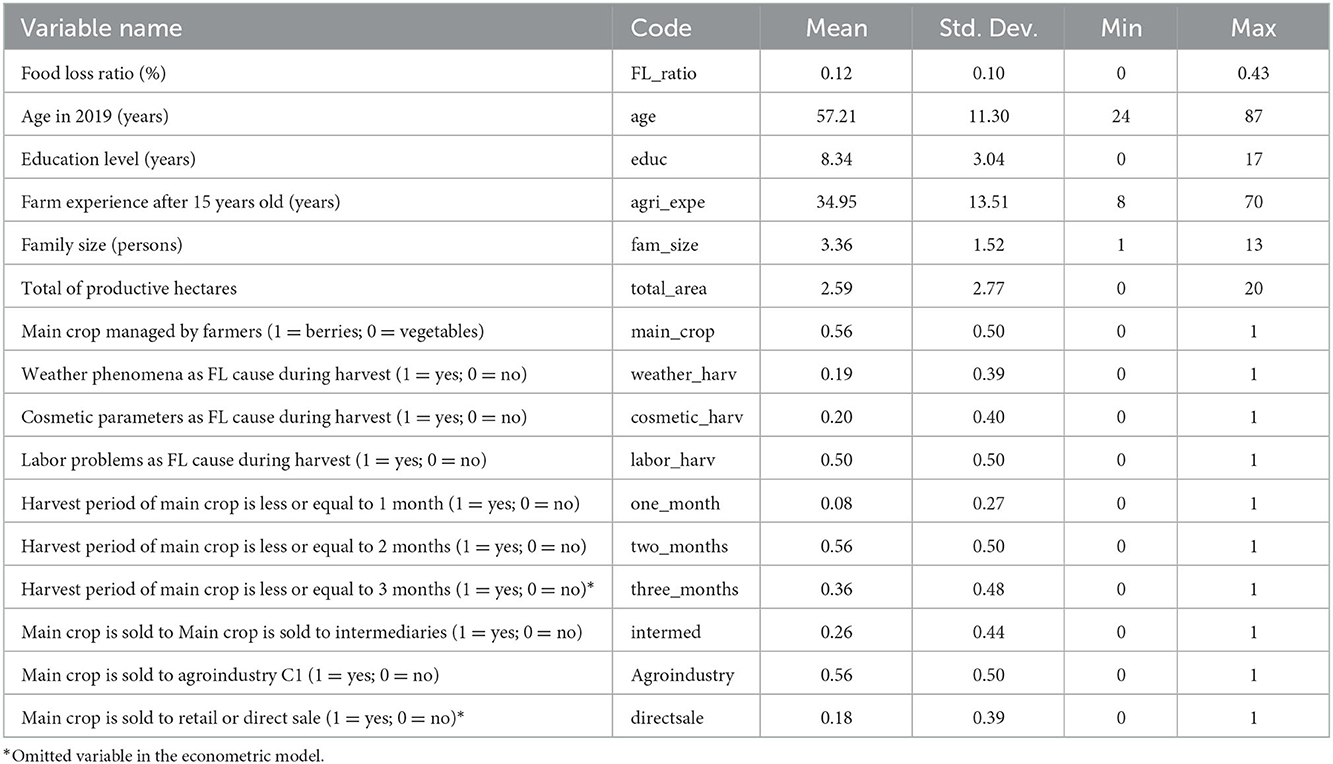
Table 1. Descriptive statistics of the sample of farmers surveyed in two agricultural regions of Chile during June and September of 2020.
With respect to the production systems and managerial abilities, 37.3% of the farmers cultivate vegetable crops, 45.7% produce berries, and 16.9% produce both crops. A total of 72.9% of the sample has technical records of farm activities, and 83.1% maintain records of the production during the harvest stage. In addition, 80.2% keep records of their accounting activities. Diversity of species is a common FFA feature, as well as the heterogeneity of its commercialization channels. Approximately 33.6% of the respondents sell their production to agroindustry, 21.6% sell through an intermediary, and 15.3% sell on the wholesale market. A smaller portion (9.6%) sell their products directly on the field, while 7.9% use informal channels, and 2.3% sell directly to retail. The other 5.8% of producers use a combination of the channels mentioned above.
4. Results
4.1. FL estimation
A total of 144 (81.4%) farmers identify FL at least at one production stage, while the remaining 18.6% declare that FL is not occurring from harvest to commercialization. This identification differs depending on the FSC stage. All farmers report losses during harvest, 9.0% of them report losses during packing, 0.7% report losses during transportation, and 9.7% report losses during commercialization.
Food loss statistics in the following tables are calculated using a sample size of 144 farmers. According to our calculations, farmers lose on average 14.5% of their total production during the entire FSC. Regarding production stages, farmers estimated that 12.1% of their production is lost during harvest, 11.5% during packing, 1.7% during transportation, and 8.3% during primary commercialization. Total food production reported by the respondents equals 10,600 tons, but a total of 1,312 tons are not consumed, which is equal to an approximate consumption of fruit and vegetables by 202,692 individuals during a 30-day period according to the World Health Organization (WHO) recommendation. Note that this estimate does not consider the inedible parts of the food products. Similarly, the estimated FL is equal to 456 million calories which could cover the energy requirements of 6,610 adults for 30 days. As a reference, the total gross income represented by this FL corresponds to 807,515 USD or 2,240 USD/ha (refer to Table 2).
According to the production system (Table 3), vegetable producers lose on average 7.2 ton/ha which is statistically different from the 1.6 ton/ha of FL calculated for berries producers and the 1.1 ton/ha for mixed producers. Regarding calories losses, the three production systems are also statistically different: vegetable crops show the highest calorie losses on average (1,762,240 kcal/ha), followed by berries with 747,086 kcal/ha, and finally mixed crops, with a loss of 438,532 kcal/ha. As for water and protein content losses, vegetable crops are statistically different than berries and mixed crops. The former represents the higher loss of these two indicators, being 6.7 m3 of water content per hectare and 746.1 kg on average of protein per hectare. In terms of fiber, no statistical differences are reported. The results of the FL for the most representative crops are presented in Table 4.
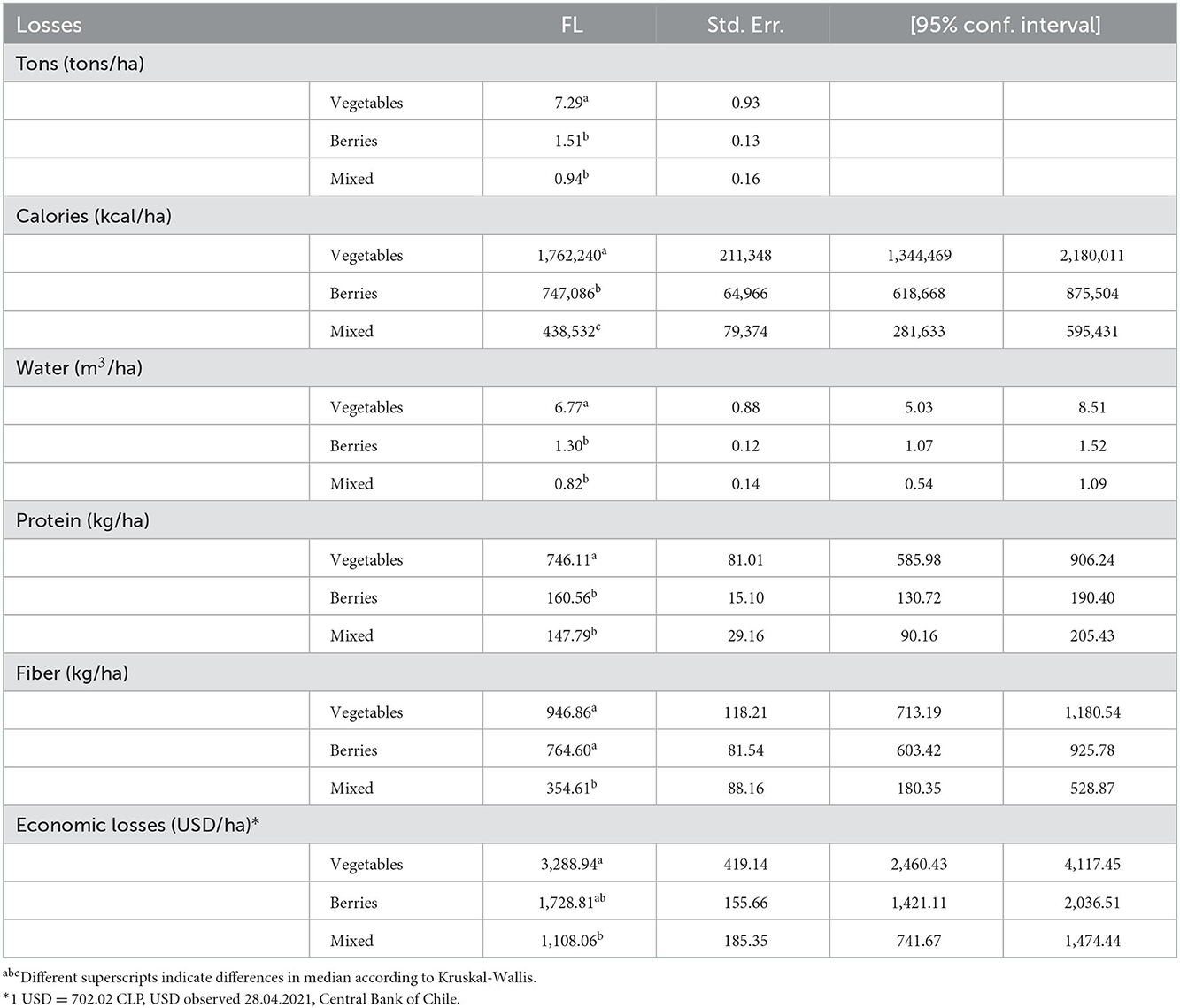
Table 3. Mean differences of food loss (FL) in tons, and nutritional and economic losses, calculated according to productive systems (vegetables = 56 obs.; berries = 66 obs., mixed = 22 obs).
As Table 5 shows, commercialization channels affect significant FL perceived by farmers. Vegetable farmers that sell their products to the agroindustry or in the wholesale market lost 8.9 ton/ha and those vegetable producers that use direct sale systems or that sell in retail perceived 7.2 ton/ha of FL. Both FL values are significantly higher than vegetable farmers using an intermediary commercialization channel (4.7 ton/ha). Berries farmers perceive higher values of FL in the agroindustry (1.6 ton/ha) compare to the intermediary (1.2 ton/ha) and direct sale (0.2 ton/ha).
Regarding the FL perceived by farmers grouped according to sociodemographic characteristics, the younger group of farmers–aged 20 to 39 years–report 5.1 ton/ha on average, which is at least one additional ton than the rest of the sample. At the same time, higher education implies a higher perception of FL, but the tendency is not significant. As for FL causes during the harvest stage (Table 6), farmers mentioned labor shortage, followed by cosmetic standards, and climate or weather events.
4.2. Fractional regression output
Table 1 shows a statistics summary for dependent and independent variables included in a fractional regression model. On average, the mean ratio of FL is 0.12 ranging from 0 to 0.43. The socioeconomic and productive variables were described earlier. The same applies to the FL causes mentioned by farmers during the harvest stage and commercialization stages. Regarding the period dedicated to harvesting the main crop, 7.9% of the sample dedicate 30 days or less, 55.5% devote between 31 and 60 days, and 35.6% need more than 60 days.
According to the results presented in Table 7, sociodemographic characteristics and farm production areas are not statistically significant in the fractional regression model. The main crop managed by farmers had a significant effect, being 5.2 percentage points less if the principal crop is berries. Regarding FL causes, cosmetic parameters seem to increase the FL ratio by 5.5 percentage points, while the existence of labor problems, such as a shortage of personnel, raises the FL ratio by 5.1 percentage points. Weather problems were not a significant factor.
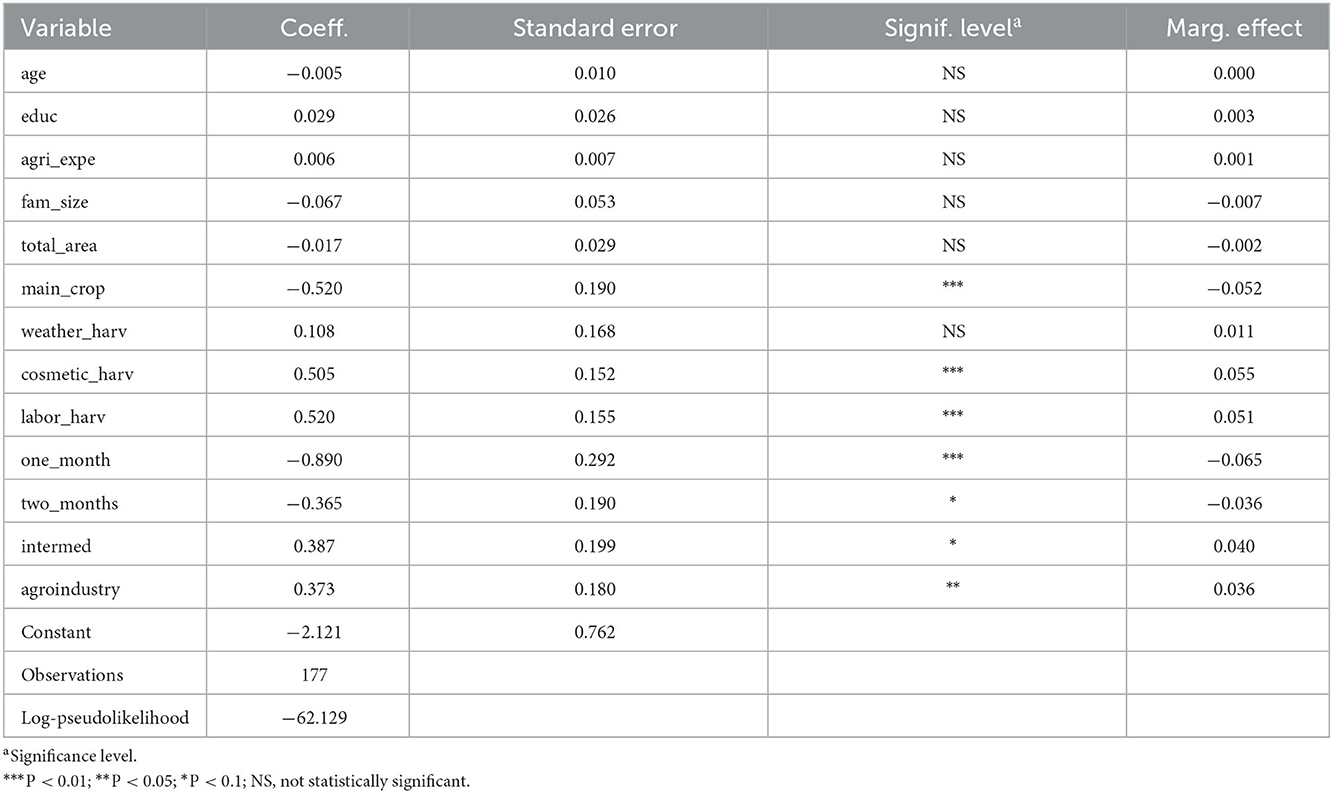
Table 7. Fractional regression model explaining variation in food loss (FL) reported by farmers surveyed in two agricultural regions of Chile during June and September of 2020.
If the harvest period is < 1 month, FL is significantly lower by 6.5 percentage points. A larger harvest period (2 months) has a less significant effect, reducing the ratio by 3.6 percentage points. Commercialization channels also proved to be statistically significant. While selling the products to intermediaries increases FL by 4 percentage points, agroindustry and wholesale market channels increase FL by 3.6 percentage points.
5. Discussion
A total of 81.4% of the sample identified FL at the harvest stage. Chaboud (2017) mentions that farmers are the stakeholders that most commonly identify FL. The “absence” of FL in the remaining 18.6% of the sample might be explained by the lack of awareness among farmers about FL as a relevant topic. This may also explain why farmers give less attention to FL and fail to notice and record it (Liu, 2016; Beausang et al., 2017). Additionally, low levels of FL perceived during packing, transport, and primary commercialization could be associated with the fact that most of the farmers are not involved in those stages.
Regarding the FL amounts calculated, an average of 14.5% of the production per hectare is lost from harvesting to primary commercialization. Based on FAO data published in 2011, Lipinski et al. (2013) estimated that 24.0% of total food production is lost during the production stage, 14.0% is generated by developing countries, and 10.0% is in developed countries. Most recently, FAO in 2019 reported that 13.8% of produced food in 2016 was lost in primary production. A meta-analysis by Fabi et al. (2021) shows that fruit and vegetable FL percentages exceed 10.0% in Latin America.
Food losses of the most representative crops, raspberries and tomatoes, represent on average 13.3% and 13.7% of the total production, respectively. The project “Measurement and Management of Fruits and Vegetables Losses on Production Stages at National Level in Chile” report 23.0% of the tomato and 5.0% of the raspberry harvests are lost (One Planet, 2020). Differences between the results of both studies can be explained by the magnitude of samples, the boundary systems used, and the absence of field FL measurement. Delgado et al. (2021) found that self-reported methodologies could generate FL underestimations. Hanson et al. (2016) suggested supplementing surveys with different quantification methods, e.g., FLW weighing.
Concerning sociodemographic characteristics of producers, despite the lack of significance shown by mean comparisons and fractional regression, this study provides interesting results about the age and education level of the sampled farmers. According to Delgado et al. (2017), higher levels of education and agricultural experience are associated with less FL during production, which explains why younger farmers reported losing more food due to their lack of agricultural experience. In addition, younger farmers have had access to more years of education. Understanding how sociodemographic characteristics affect the generation of FL is crucial to improve food security (Delgado et al., 2021) and future research must more deeply consider the aforementioned characteristics.
Vegetable producers perceive more losses than those producing berries or mixed producers according to fractional regression results. This might be related to the target market since vegetables are mostly sold in the local or wholesale market. On the other hand, raspberries and blueberries are produced mainly for export (Retamales et al., 2014; Jara-Rojas et al., 2018) that involve high-quality standards. Raspberry producers also had as an alternative market the agroindustry, where esthetic and quality characteristics are less relevant.
Significant differences between economic losses on vegetable and mixed agricultural products could be explained by the lower yields obtained by the latter, due to the different management required by their production systems. Still, a comprehensive analysis of production costs is required in this matter. With respect to nutritional losses, values reflect a clear difference in nutritional content between vegetables and berries. These results represent useful new information to initiate discussion about nutritional losses. Indeed, Kitinoja et al. (2018) indicated that of 268 articles about post-harvest losses reviewed, only 62 studies gave primary data about economic losses and only six about nutritional losses. Since production systems differ, it is essential for future research to account for a disaggregated sample and to analyze each crop's FLs separately.
The econometric model results show a significant effect of harvest periods on the FL ratio, which increases the longer the harvest period. Extended exposure to the produce may cause it to overripen or may affect its composition and consequently shorten its post-harvest life (Elik et al., 2019). While the ripening of produce is the main harvest parameter, often the decision to harvest sooner or later could also be influenced by economic reasons (Elik et al., 2019). Therefore, more research is needed about this factor affecting FL.
The interaction between the main production system and its commercialization channel was statistically significant. While FL amounts are statistically different between vegetables and berries in general, these differences are increased by the retail/direct sale commercialization category. The fact that more FL originated by vegetable producers that sell to retail may be related to quality standards imposed by the sector, leading to produce rejection if these standards are not met (Canali et al., 2017). Intermediary and agroindustry channels increase FL. Recognizing that the farmer's choice of commercialization channel can have an effect on FL produced during primary production is crucial and requires further research.
Among farmers' insights about FL causes, labor problems, cosmetic standards, and climate/weather effects were highlighted in the study, significantly increasing the FL ratio according to fractional regression. Those results are in line with Verma et al. (2019), who mention that labor shortage delays the harvest and increases FL. Another problem may be related to high labor costs and low market prices, which lead farmers to decide not to harvest and leave the produce in the field (Beausang et al., 2017; Johnson et al., 2019). HLPE (2014) shows that farmers' harvest decisions are directly influenced by market requirements—requirements that, most of the time, are related to visual aspects more than the nutritional value of the food. While poor weather conditions or abrupt weather changes are not statistically relevant to FL in our fractional regression model, it is worth mentioning that weather can lead to damage to vegetables and soft fruit, shortening their post-harvest life and/or causing cosmetic loss (Beausang et al., 2017).
6. Conclusion
The objective of this study was to measure food losses generated during primary production using a micro-approach methodology from harvest to commercialization stages among small-scale farmers. Furthermore, to understand the factors affecting FL, we used a fraction regression model with special emphasis on the harvest stage. On average, the FL was 14.5%, and factors that increase FL are the production system and its harvest period, commercialization channels, labor shortage, and cosmetic standards.
Food loss and waste information across the FSC and its causes in each stage of the production chain are quite scarce in Chile (Eguillor, 2019) and, thus, any attempt to measure the magnitude of the FL problem can positively contribute to policy discussions. While the study's sample size does not make it representative of the studied area, and the measurement of FL on the field was not possible, the results obtained contribute to generating evidence that FL originated in FFA primary production in Central Chile.
From this micro-approach study, it can be concluded that farmers report a considerable volume of FL during primary production, mostly during the harvest stage. This can be translated into an economic loss for producers and a nutritional loss for consumers. Calculating losses using these approaches provide a holistic point of view that will facilitate the generation of sustainable solutions for the FLW issue.
Production systems affect the amount of FL generated, as well as the way the product is commercialized. Additionally, problems such as labor shortages seem to increase FL, as well as long- established customs in agriculture, like cosmetic standards. This supports the idea that FL quantification should be calculated according to specific products, involving all FSC actors.
Chile has put in place different initiatives to prevent FLW produced by the FSC stakeholders and consumers (Eguillor, 2020). According to Bahadur et al. (2016), when policy efforts focus on FL reduction, changes seem to be very effective. Existing literature with policy implications can be considered as a first step to addressing the FLW from an institutional perspective. Nevertheless, methods and FLW definitions are still scarce and remain understated (Cattaneo et al., 2021). Finally, collecting data and evidence is an imperative action, since FLW represents a multidimensional issue, with its causes intertwined throughout the FSC (Canali et al., 2017).
Data availability statement
The raw data supporting the conclusions of this article will be made available by the authors, without undue reservation.
Ethics statement
Ethical review and approval was not required for the study on human participants in accordance with the local legislation and institutional requirements. The patients/participants provided their written informed consent to participate in this study.
Author contributions
RJ-R and GH-Q contributed to conception and design of the study. GH-Q organized the database and wrote the first draft of the manuscript. RJ-R performed the econometric analysis and wrote sections of the manuscript. All authors contributed to manuscript revision, read, and approved the submitted version.
Funding
This study was partially funded by FONDECYT Project N°1171122.
Conflict of interest
The authors declare that the research was conducted in the absence of any commercial or financial relationships that could be construed as a potential conflict of interest.
Publisher's note
All claims expressed in this article are solely those of the authors and do not necessarily represent those of their affiliated organizations, or those of the publisher, the editors and the reviewers. Any product that may be evaluated in this article, or claim that may be made by its manufacturer, is not guaranteed or endorsed by the publisher.
Footnotes
1. ^We use the USDA webpage https://fdc.nal.usda.gov/index.html to ask the nutritional status of the following crops: blueberry, tomato, cucumber, paprika, chili, watermelon, raspberry, blackberry, onion, squash, beans, strawberry, lettuce, coriander, chard, potato, melon, cabbage, asparagus, corn, wheat, cauliflower, broccoli, chickpeas, spinach, zucchini, and oatmeal.
References
Alexander, P., Brown, C., Arneth, A., Finnigan, J., Moran, D., and Rounsevell, M. (2017). Losses, inefficiencies and waste in the global food system. Agric. Syst. 153, 190–200. doi: 10.1016/j.agsy.2017.01.014
Bahadur, K., Haque, I., Legwegoh, A., and Fraser, E. (2016). Strategies to reduce food loss in the global south. Sustainability. 8, 595. doi: 10.3390/su8070595
Baum, C. (2008). Stata tip 63: modeling proportions. S J. 8 299–303. doi: 10.1177/1536867X0800800212
Beausang, C., Hall, C., and Toma, L. (2017). Food waste and losses in primary production: qualitative insights from horticulture. Resour. Conser. Recy. 126, 177–185. doi: 10.1016/j.resconrec.2017.07.042
Bellemare, M., Cakir, M., Hanawa, H., Novak, L., and Rudi, J. (2017). On the measurement of food waste. Am. J. Agric. Econ. 99, 1148–1158. doi: 10.1093/ajae/aax034
Beretta, C., Stoessel, F., Baier, U., and Hellweg, S. (2013). Quantifying food losses and the potential for reduction in Switzerland. Int. J. Environ. Waste Manag. 33 764–773. doi: 10.1016/j.wasman.2012.11.007
Bravo-Ureta, B., Jara-Rojas, R., Lachaud, M., and Moreira, V. (2017). “A meta analysis of farm efficiency: evidence from the production frontier literature,” in Charles J. Zwick Center for Food and Resource Policy Research Report 5. University of Connecticut.
Canali, M., Amani, P., Aramyan, L., Gheoldus, M., Moates, G., Östergren, K., et al. (2017). Food waste drivers in Europe, from identification to possible interventions. Sustainability. 9, 37. doi: 10.3390/su9010037
Cattaneo, A., Sánchez, M., Torero, M., and Vos, R. (2021). Reducing food loss and aaste: five challenges for policy and research. Food Policy. 98,101974. doi: 10.1016/j.foodpol.2020.101974
Chaboud, G. (2017). Assessing food losses and waste with a methodological framework: insights from a case study. Resour. Conser. Recy. 125, 188–197. doi: 10.1016/j.resconrec.2017.06.008
Chegere, M. (2018). Post-harvest losses reduction by small-scale maize farmers: the role of handling practices. Food Policy. 77 103–115. doi: 10.1016/j.foodpol.2018.05.001
Corrado, S., Ardente, F., Sala, S., and Saouter, E. (2017). Modelling of food loss within life cycle assessment: from current practice towards a systematization. J. Clean. Prod. 140 847–859. doi: 10.1016/j.jclepro.2016.06.050
Delgado, L., Schuster, M., and Torero, M. (2017). “The reality of food losses, a new measurement methodology,” in International Food Policy Research Institute Discussion Paper 1686. Washington, D.C., United States.
Delgado, L., Schuster, M., and Torero, M. (2021). Quantity and quality food losses across the value chain: a comparative analysis. Food Policy. 98, 101958. doi: 10.1016/j.foodpol.2020.101958
Eguillor, P. (2017). Pérdida y Desperdicio de Alimentos: Diciembre 2017. Santiago, Chile: Oficina de Estudios y Políticas Agrarias. Available online at: https://www.odepa.gob.cl/wp-content/uploads/2017/12/residuosFinal-1.pdf (accessed 17 June, 2019).
Eguillor, P. (2019). Pérdida y Desperdicio de Alimentos en el Sector Agrícola: Avances y Desafíos, Febrero 2019. Santiago, Chile: Oficina de Estudios y Políticas Agrarias. Available online at: https://www.odepa.gob.cl/wp-content/uploads/2019/02/articulo-perdida_desperdicios.pdf (accessed 17 June, 2019).
Eguillor, P. (2020). Menos Pérdida y Desperdicio, Más Alimentos: Un Pilar en la Lucha Contra el Hambre. Oficina de Estudios y Políticas Agrarias, Santiago, Chile. Available online in: https://bibliotecadigital.odepa.gob.cl/bitstream/handle/20.500.12650/70310/Articulo-PDA20200722.pdf (accessed 06 December, 2020).
Eguillor, P., and Acuña, D. (2019). Pérdida y Desperdicio de Alimentos (PDA) en Chile: Avances y Desafíos, Diciembre 2019. Santiago, Chile: Oficina de Estudios y Políticas Agrarias. Available online at: https://www.odepa.gob.cl/wp-content/uploads/2019/12/Articulo-PDA_Diciembre2019.pdf (accessed 17 June, 2019).
Elik, A., Kocak, D., Istanbullu, Y., Aysar, N., Yavuz, A., and Gogus, F. (2019). Strategies to reduce post- harvest losses for fruits and vegetables. Int. J. Sci. Technol. Res. 5, 29–39. doi: 10.7176/JSTR/5-3-04
Fabi, C., Cachia, F., Conforti, P., English, A., and Moncayo, J. R. (2021). Improving data on food losses and waste: from theory to practice. Food Policy. 98, 101934. doi: 10.1016/j.foodpol.2020.101934
FAO (2014). Food Wastage Footprint. Full-cost Accounting. Final Report. Available online at: http://www.fao.org/3/a-i3991e.pdf. (Accessed 21.10.2020)
FAO (2016). Pérdidas y Desperdicios de Alimentos en América Latina y el Caribe. Boletín 3. Available online at: http://www.fao.org/3/I5504S/i5504s.pdf. (Accessed: 19.06.2020)
FAO (2019). The state of food and agriculture 2019. Moving Forwards on Food Loss and Waste Reduction. Rome, FAO.
FAO Fida, OMS, PMA, and UNICEF.. (2020). “The state of food security and nutrition in the world 2020,” in Transforming food systems for affordable healthy diets. Rome: FAO.
FAO OPS, WFP, and UNICEF. (2019). “Panorama de la Seguridad Alimentaria Nutricional en América Latina y el Caribe,” in Hacia entornos alimentarios más saludables que hagan frente a todas las formas de malnutrición. Santiago: FAO.
Franke, U., Hartikainen, H., Mogensen, L., and Svanes, E. (2016). “Food losses and waste in primary production- data collection in the Nordic countries,” in Nordic Council of Ministers (Copenhagen: Nordisk Ministerråd), 90. doi: 10.6027/TN2016-529
Future Foods (2022). “Global Trends, Opportunities, and Sustainability Challenges,” in Agricultural Productivity and Food Supply to Meet Increased Demand. p. 539–553. doi: 10.1016/B978-0-323-91001-9.00016-5
Gallani, S., and Krishnan, R. (2015). “Applying the fractional response model to survey research in accounting,” in Harvard Business School Accounting & Management Unit Working Paper No. 16–016.
Giraldo, C., Bañados, N., and Leiva, F. (2019). Design of an Adapted Methodology for to Quantify Food Loss of Fruits and Vegetables and Development of a Good Practice Guide. Available online at: https://www.oneplanetnetwork.org/sites/default/files/resumen_ejecutivo_cavs.pdf (accessed: 03 September, 2020).
Gustavsson, J., Cederberg, C., Sonesson, U., Van Otterdijk, R., and Meybeck, A. (2011). Global Food Losses and Food Waste. Study Conducted for the International Congress “Save Food!”, Düsseldorf, Germany. Available online at: https://www.fao.org/3/i2697e/i2697e.pdf (accessed: 21 March, 2019).
Hanson, C., Lipinski, B., Robertson, K., Dias, D., Gavilan, I., Grévarath, P., et al. (2016). Food Loss and Waste Accounting and Reporting Standard (FLW Standard) Version 1.0. Available online at: https://flwprotocol.org/wp-content/uploads/2019/03/FLW_Standard_Exec_Summary.pdf (accessed 19 June, 2019).
Hartikainen, H., Morgense, L., Svanes, E., and Franke, U. (2017). Food waste quantification in primary production- the nordic countries as a case study. Int. J. Environ. Waste Manag. 71 502–511. doi: 10.1016/j.wasman.2017.10.026
HLPE (2014). Food Losses and Waste in the Context of Sustainable Food Systems. Available online at: https://www.fao.org/3/i3901e/i3901e.pdf. (accessed 12 November, 2020).
Hodges, R., Bernard, M., and Rembold, F. (2014). APHLIS: Postharvest Cereal Losses in Sub-Saharan Africa, Their Estimation, Assessment and Reduction. European Commission, Joint Research Centre, Publications Office.
INDAP (2016). La Agricultura Familiar Campesina en Chile y los Usuarios de INDAP. Available online at: https://www.indap.gob.cl/docs/default-source/default-document-library/afc-en-chile-y-los-usuarios-de-indap.pdf?sfvrsn=0. (accessed 22 December, 2020).
Jara-Rojas, R., Bravo-Ureta, B., Solís, D., and Martínez, D. (2018). Technical efficiency and marketing channels among small scale farmers: evidence for raspberry production in Chile. Int. Food Agribusiness Manag. Rev. 21, 351–364. doi: 10.22434/IFAMR2016.0168
Jara-Rojas, R., Canales, R., Gil, J. M., Engler, A., Bravo-Ureta, B., and Bopp, C. (2020). Technology adoption and extension strategies in mediterranean agriculture: the case of family farms in Chile. Agronomy. 10, 692. doi: 10.3390/agronomy10050692
Johnson, L., Bloom, J., Dunning, R., Boyette, M., and Creamer, N. (2019). Farmer harvest decisions and vegetable loss in primary production. Agric. Syst. 176, 102672. doi: 10.1016/j.agsy.2019.102672
Johnson, L., Dunning, R., Bloom, J., Gunter, C., Boyette, M., and Creamer, N. (2018). Estimating on-farm food loss at the field level: a methodology and applied case study on a north carolina farm. Resour. Conser. Recy. 137 243–250. doi: 10.1016/j.resconrec.2018.05.017
Kitinoja, L., Tokala, V. Y., and Brondy, A. (2018). A review of global postharvest loss assessments in plant- based food crops: recent findings and measurement gaps. Postharvest Biol. Technol. 06, 1–15.
Koester, U., and Galaktionova, E. (2021). FAO Food Loss Index methodology and policy implications. Stud. Agric. Econ. 123, 1–7. doi: 10.7896/j.2093
Kummu, M., De Moel, H., Porkka, M., Siebert, S., Varis, S., and Ward, P. (2012). Lost food, wasted resources: global food supply chain losses and their impacts on freshwater, cropland, and fertiliser use. Sci. Total Environ. 438, 477–489. doi: 10.1016/j.scitotenv.2012.08.092
Lipinski, B., Hanson, C., Lomax, J., Kitinoja, L., Waite, R., and Searchinger, T. (2013). Reducing Food Loss and Waste. Working Paper, Installment 2 of Creating a Sustainable Food Future. Washington, DC: World Resources Institute. Available online at: http://www.worldresourcesreport.org. (accessed 11 March, 2020).
Liu, B. (2016). “Case study methodology to find the causes of food loss and develop solutions,” in Sustainable value chains for sustainable food systems. Programme on Sustainable Food Systems workshop. Rome: FAO/UNEP.
McDonald, J. (2009). Using least squares and tobit in second stage DEA efficiency analyses. Eur. J. Oper. Res. 197, 792–798. doi: 10.1016/j.ejor.2008.07.039
ODEPA (2019). Panorama de la Agricultura Chilena. Available online at: https://www.odepa.gob.cl/wp-content/uploads/2019/09/panorama2019Final.pdf. (accessed 07 May, 2021).
ODEPA (2020). Comité para la Prevención y Reducción de las Pérdidas y Desperdicios de Alimentos. Available online at: https://www.odepa.gob.cl/coordinacion-publico-privada/comite-para-la-prevencion-y-reduccion-de-las-perdidas-y-desperdicios-de-alimentos. (accessed 17 February, 2021).
One Planet (2020). Food Loss Situation in the Fruit and Vegetable Chain in Chile and Validation of the Methodology Applied to Case Studies. Available online at: https://www.oneplanetnetwork.org/sites/default/files/resumen_ejecutivo_usach.pdf. (accessed 03 September, 2020).
Papke, L., and Wooldridge, J. (1996). Econometric methods for fractional response variables with an application to 401 (K) plan participation rates. J. Appl. Econometrics. 11, 619–6323. doi: 10.1002/(SICI)1099-1255(199611)11:6<619::AID-AE418>3.0.CO;2-1
Parfitt, J., Barthel, M., and Macnaughton, S. (2010). Food waste within food supply chains: quantification and potential for change 2050. Phil. Trans. R. Soc. B. 365, 3065–3081. doi: 10.1098/rstb.2010.0126
Ramalho, E., Ramalho, J., and Murteira, J. (2011). Alternative estimating and testing empirical strategies for fractional regression models. J. Econ. Surv. 25, 19–68. doi: 10.1111/j.1467-6419.2009.00602.x
Retamales, J., Palma, M., Morales, Y., Lobos, G., Moggia, C., and Mena, C. (2014). Blueberry production in chile: current status and future developments. Rev. Bras. Frutic. 36, 58–67. doi: 10.1590/0100-2945-446/13
Stenmarck, Å., Jensen, C., Quested, T., and Moates, G. (2016). “Estimates of european food waste levels,” in Report Fusions EU project. IVL Svenska Miljöinstitutet. doi: 10.13140/RG.2.1.4658.4721
Teuber, R., and Jensen, J. D. (2020). Definitions, measurement, and drivers of food loss and waste. Waste. Manag. Res. (2020). 3-18. doi: 10.1016/B978-0-12-817121-9.00001-2
Thyberg, K., and Tonjes, D. (2016). Drivers of food waste and their implications for sustainable policy development. Resour. Conser. Recy. 106, 110–123. doi: 10.1016/j.resconrec.2015.11.016
UNEP (2021). Food Waste Index Report 2021. Nairobi, Kenia. Available online at: https://www.unep.org/resources/report/unep-food-waste-index-report-2021. (accessed 07 August, 2020).
van der Werf, P., and Gilliland, J. (2017). A systems approach to food loss and solutions understanding practices, causes, and indicators. J. Waste Manag. 170, 66–67. doi: 10.1680/jwarm.16.00026
Verma, M., Plaisier, C., Van Wagenberg, C., and Achterbosch, T. (2019). A systematic review of food losses and food waste generation in developed countries. Sustainability. 11, 579. doi: 10.3390/su11030579
Keywords: food losses, primary production, harvest, horticulture, berries, family farms, Chile
Citation: Herrera-Quinteros G and Jara-Rojas R (2023) Food losses perceived by family farms: Challenges and policy implications from a micro-approach quantification. Front. Sustain. Food Syst. 6:961120. doi: 10.3389/fsufs.2022.961120
Received: 03 June 2022; Accepted: 12 December 2022;
Published: 10 January 2023.
Edited by:
Andres Silva, Central University of Chile, ChileReviewed by:
Pablo Pérez-Akaki, Monterrey Institute of Technology and Higher Education (ITESM), MexicoMichee Lachaud, Florida Agricultural and Mechanical University, United States
Copyright © 2023 Herrera-Quinteros and Jara-Rojas. This is an open-access article distributed under the terms of the Creative Commons Attribution License (CC BY). The use, distribution or reproduction in other forums is permitted, provided the original author(s) and the copyright owner(s) are credited and that the original publication in this journal is cited, in accordance with accepted academic practice. No use, distribution or reproduction is permitted which does not comply with these terms.
*Correspondence: Roberto Jara-Rojas, cmphcmFAdXRhbGNhLmNs