- Department of Agriculture, Food and Environment (Di3A), University of Catania, Catania, Italy
Introduction: The combination of knowledge, personal skills and company resources influences, all things being equal, such as the availability of new technologies, market conditions and other factors external to the company, farmers in their innovation choices. This study is an attempt to understand which psychological constructs influence the decision-making process of farmers specialized in typical Mediterranean crops with regard to innovation. Previous studies on the adoption of agricultural innovations have often considered socio economic characteristics and ignored the underlying motivational factors that influence the behavioral intention of farmers.
Methods: This study adopted three socio-psychological constructs, Attitude (ATT), Subjective Norm (SN), and Perceived Behavioral Control (PBC), derived from the Theory of Planned Behavior (TPB), and proposed three new constructs, Perceived Innovations Characteristics (PIC), Benefits (B), and Transferability (T), thus using an Extended Model of the Theory of Planned Behavior.
Results: The outcome of the multiple regression revealed that farmers' intention (I) to adopt sustainable irrigation innovations is positively influenced by attitude (ATT), subjective norm (SN), and perceived innovation characteristics (PIC). This last construct had mediating effects on the indirect relationships between PBC, benefits (B), transferability (T), and intention (I).
Discussion: The results provide numerous insights, useful both for outlining the demand for innovation and for calibrating future policies aimed at the primary sector, especially on the sustainable management of irrigation resources. In particular, the analyses carried out highlight the importance of factors external to the company as key levers in shaping the demand for innovations.
1. Introduction
In a knowledge-based economy and with the acceleration of the globalization process that reduces the relevance of the spatial factor while accentuating the temporal factor to the extreme, the competitiveness of the territorial system increasingly depends on intangible resources (Capitanio et al., 2010), the capacity for interaction, collaboration and partnership (Rose and Chilvers, 2018; Kumar et al., 2021). Technology and innovation consequently acquire a strategic role in determining the competitive advantage of companies. In such a competitive environment, where innovation progressively takes the form of interaction between different companies or organizational units with complementary knowledge and skills (Guaitero et al., 2013; El Bilali, 2019), understanding the innovation process between different organizations becomes of great interest (Montes de Oca Munguia et al., 2021).
In economic theory, innovation is one of the key tools for a company's growth, entry into new markets and long-term sustainability (Sulistyo and Ayuni, 2020; Gutiérrez and Macken-Walsh, 2022; Ploll et al., 2022). Driven by increasing competition in global markets and unprecedented interest in sustainability practices, companies are seeking to implement more advanced sustainability practices (Hasler et al., 2016; Liu et al., 2018), seeking to maintain the high value of the products and services they provide through rapid and continuous innovation (Müller et al., 2018; Rabadán et al., 2019). However, companies often face the intention to innovate with uncertainty and concern, as they are confronted with a number of unfavorable factors, such as: lack of financial resources (Schaltegger and Wagner, 2011; Quintero et al., 2022), innovation costs that are too high, lack of qualified staff to manage innovations in the company, lack of knowledge of innovations, lack of confidence in innovations (Shi et al., 2022), low interest in innovations and long-term results (Sivertsson and Tell, 2015; Wang et al., 2022).
The agricultural sector faces significant challenges due to competing economic and environmental objectives. In this context, agricultural innovation can contribute to achieving higher production while preserving the environment (Läpple et al., 2015; Kubankova et al., 2016). Innovation in the agro-food industry, however, is lower than in other manufacturing sectors: according to recent studies, the agro-food sector, like the paper, printing and publishing, rubber and plastics sectors, invests around 2% of its turnover in R&D (Jun and Kim, 2022), while other sectors such as the chemical, electronic and mechanical sectors devote around 6–9% to this activity (Mekonnen et al., 2015).
The reasons for these low levels of expenditure compared to other sectors are to be found in the lack of basic research, the fact that innovation is exogenous and embedded in machinery, packaging and supplies in general (Coghlan et al., 2020; Fieldsend et al., 2022). Most of the innovations that have appeared in the agri-food sector do not originate from within the sector, but arise from the application and transfer of the results of research conducted in other areas, as was the case, for example, with the automation of processes, the control of results, especially in terms of quality (Curry et al., 2021; Bigliardi and Filippelli, 2022). Consequently, facilitating agricultural innovation is vital to the success of the agricultural sector. This has also been recognized by the EU with the creation of the European Innovation Partnership for Productivity and Sustainability (EIP-AGRI), which aims to foster sustainable and competitive agriculture as well as greater dissemination of innovations through increased links between research and agriculture (Barth et al., 2017; Feo et al., 2022a). Furthermore, it is widely recognized that continuous innovation is necessary in order to achieve sustainable agricultural development (Ploll et al., 2022; Takács-György and Takács, 2022).
Agricultural innovation is increasingly seen as a process involving the input of different actors and also as something that depends on the social structure of a specific context (Läpple et al., 2015; Feo et al., 2022b). It evolves as a result of interactions between different actors, such as farming systems, supply chains and economic systems (Klerkx et al., 2012), environmental policies, extension and social systems, reflecting the idea of Agricultural Innovation Systems (AIS) (Maru, 2018; Klerkx and Begemann, 2020). Among the different types of innovation in agriculture, a particularly important role today is played by those related to irrigation practices (Asadi et al., 2020). Today, in fact, water scarcity and droughts are a major problem (Saeed et al., 2021), probably exacerbated by climate change, which represents one of the greatest environmental, social and economic threats to the entire planet (Ungureanu et al., 2020; Ermolieva et al., 2022).
These needs also result from the fact that the agricultural system, as a result of the climatic changes we have been observing in recent years (Masia et al., 2018), has been affected by meteorological changes, which have led to earlier phenological phases of crops, a decrease in the availability of water in the soil and in the flow rates of watercourses, and low reservoir levels in natural and artificial reservoirs (Nguyen et al., 2016; Zagaria et al., 2021). These situations, over the years, have produced negative effects on the production level of many crops (Hashem et al., 2019), drawing attention to the rational use of water availability in agriculture (Kalinin et al., 2018; Zhang et al., 2022). In this scenario, irrigation can be used to offset the negative impacts of climate change on food supply, (Kukal and Irmak, 2018; Malek et al., 2018; Masia et al., 2018) but would require a 40–100% increase in water use for irrigation (Liu et al., 2017; Bafdal et al., 2018; Zaporozhchenko et al., 2022). In relation to this question, the question arises as to whether the public is willing to allocate such quantities of irrigation water for agriculture (Khandaker and Kotzen, 2018; de Oliveira Padilha et al., 2022), also in relation to the limited availability of water for domestic use and the alternatives set up by the food industry with food obtained in the laboratory or in hyper-intensive systems such as “Lab-grown meat” (van Loo et al., 2020; Galanakis et al., 2021) or as Vertical farming (Niu and Masabni, 2021; van Gerrewey et al., 2022).
Technology, innovation and Agriculture 4.0 are the solution today, as they can reduce water consumption in agriculture by up to 20 per cent compared to traditional irrigation systems (Adeyemi et al., 2017; Velasco-Muñoz et al., 2019; Kourgialas et al., 2022). In the fruit-growing world, the most economical systems are drip systems: micro-sprinklers (Canaj et al., 2021), underground drip sprinklers and mini-sprinklers (Loures et al., 2020; Rouzaneh et al., 2021). In the context of innovations, the Mediterranean tree crops sector is certainly one of those that has received less attention in the economic literature (Coghlan et al., 2020). Furthermore, it appears that the adoption of innovation has been studied mainly in large fruit companies in developed countries, while research on innovation in small companies has received little attention (Migliore et al., 2015; Kim et al., 2019). Small farms in the sector are, in fact, part of the agri-food system and play an important role in the economic growth of the country (Rajapathirana and Hui, 2018; Bigliardi and Filippelli, 2022).
In small business management, it is widely recognized in the literature (Aksoy, 2017; Barth et al., 2017) that the competence of entrepreneurs, farmers and professional managers plays an important role in the adoption of business innovation (Mozzato et al., 2018; Yan et al., 2022). This suggests that in small and medium-sized farms, competitiveness, and sustainability require entrepreneurs capable of achieving all commercial, environmental and social objectives with the help of a facilitator (Kim et al., 2019; Chi and Chien, 2022) who enables the transfer of innovations to as yet unexplored production systems, including Mediterranean tree crops (Mirčetić et al., 2022; Nsele et al., 2022).
Among the Mediterranean crops we are interested in, the most widespread tree crops such as olives, citrus fruits and vines still have a limited degree of innovation diffusion. Regarding crops such as olives and vines, these were considered in the study as irrigated crops, even though they are normally not. This scenario allows us to formulate the following research question:
“Farmers who grow Mediterranean tree crops, what level of propensity for innovation do they express?”
The aim of this question is to understand whether this innovation gap stems from a knowledge deficit, limited propensity, structural limitations or other reasons. It is therefore interesting to understand all the characteristics of entrepreneurs, farmers or professional managers (Hsieh and Kelley, 2016), who promote innovation, and why some organizations are able to generate innovation better than others (Unsworth et al., 2012; Mirzaei et al., 2016). Knowing the characteristics and determinants of the propensity to innovate in the primary sector becomes even more important because such information is fundamental to the design of public policies aimed at supporting and expanding demand (van Dijk et al., 2016; Small and Maseyk, 2022).
A company's decision to innovate is based on its ability to withstand the pressures of the process (Douthwaite and Hoffecker, 2017; Alam et al., 2021) and the degree of control it feels it has over the implementation of the innovation (Maizza et al., 2019; Harwiki and Malet, 2020). Since innovation requires a limited and sometimes complex decision-making process (Bechini et al., 2015), it is clear that those responsible for corporate innovation can be influenced by several objective and subjective variables (Brudermann et al., 2013; Montes de Oca Munguia et al., 2021). Determining the behavioral motivations and psychological factors of decision-makers in the agricultural industry is a rather complex task (Borges et al., 2014; Adnan et al., 2017; Mesa-Vázquez et al., 2021). The choice of a behavioral model is necessary (Berti and Mulligan, 2016) because the farm's intention to accept or not to accept an innovation inevitably clashes with human psychology, so we tried to analyze the factors and variables that influence this behavior (Judge et al., 2019; Hannus and Sauer, 2021).
To this end, a questionnaire was proposed to a sample of agricultural enterprises. This questionnaire aimed to detect the propensity to adopt an innovation that favors the sustainability of the production process, i.e., one that respects the environment, animals, health and workers' rights, as well as the resulting economic and environmental benefits. Several behavioral models have thus been identified to explain the decision-making process of entrepreneurs (Issa and Hamm, 2017; Lang and Rabotyagov, 2022), but among all the models proposed in the literature, we have chosen the Theory of Planned Behavior (TPB), which seems to be the most comprehensive tool for studying entrepreneurial behavior (Sok et al., 2021; Sarkar et al., 2022).
2. Materials and methods
2.1. The role of innovations in irrigation
Agricultural ecosystems play a key role in the conservation and availability of sufficient and quality water resources (Aznar-Sánchez et al., 2018), being the main providers of food but also the main consumers of water resources globally (Velasco-Muñoz et al., 2019; Shi et al., 2021). Irrigated agriculture is the primary user of intercepted water for human purposes, reaching a proportion exceeding 70–80% of the total in arid and semi-arid areas (Liu et al., 2017; López-Felices et al., 2020) food production that requires irrigation uses more than 40% of the total and uses only about 17% of the agricultural area (Saeed et al., 2021). The reduced availability of water resources for agri-food production systems increases the complexity of the social, economic and environmental implications in less developed regions (Gambelli et al., 2021). At present and even more so in the near future, irrigated crops can be grown under conditions of reduced water availability (Canaj et al., 2021; Pardo et al., 2022). Insufficient water resources will, therefore, be the norm rather than the exception and the emphasis of irrigation technology will shift from increasing productivity per unit area to maximizing water productivity (Ungureanu et al., 2020; Campana et al., 2022).
In this context, the solution to move toward is the development of knowledge and innovative solutions for the management and distribution of water resources to Mediterranean agro-productive systems, to make them more resilient to climate change, economically and technically efficient, sustainable, and able to contribute to the economic growth and development of the agricultural sector. The final recipient of such innovations is the agri-food chain, which, faced with climate change and water shortages, risks disrupting supplies of raw materials with quantity, but also quality and health standards (Pandya and Sharma, 2021; Dawit et al., 2022). But a second aspect to be emphasized is the benefit to the environment and water resources generated by innovations; indeed, every activity must be aimed at rationalizing the use of water in agriculture and containing the release of contaminants into the environment (Bowmer and Meyer, 2014). Since an increase in water endowments is not imaginable, the innovative solutions to be pursued must concern the integration of purified wastewater with traditional water endowments and, in particular, the improvement of water use efficiency. Improved irrigation efficiency can be achieved through innovation and technological adoption (Iocola et al., 2020; Tong et al., 2022). Water use efficiency depends on the technology and approach to irrigation (Velasco-Muñoz et al., 2019).
We therefore distinguish three main distribution methods in fruit tree plantations: surface (75% of agricultural land), sprinkler (20% of agricultural land) and micro-irrigation (5% of agricultural land) (Adeyemi et al., 2017; Rouzaneh et al., 2021). The application efficiencies of these general categories vary widely (Mateos et al., 2016; Al-Agele et al., 2021b). Micro-irrigation is generally the most efficient (80–90%) and surface irrigation is the least efficient (50–70%). The efficiency of sprinkler irrigation is 55–80% (Loures et al., 2020; Lopriore and Caliandro, 2022). In other words, the more efficient irrigation approach is used less and vice versa (Mateos et al., 2016). Emerging technologies, such as precision agriculture, agri-voltaic systems and technological innovation in irrigation (Loures et al., 2020), can further increase efficiency and productivity in the food-energy-water nexus (Assouline et al., 2015; Zhu et al., 2022). Precision agriculture in particular proves to be a potential technological ally for increasing water use efficiency (Turral et al., 2010; Pino et al., 2017). This type of agriculture includes decision support systems (DSS) (Bonfante et al., 2019; Souza and Rodrigues, 2022) to perform what-if analyses for managing small amounts of irrigation water, monitoring the water status of soil and/or vegetation (with remote sensing, proximal sensing and soil and vegetation sensors) and precision irrigation (Abioye et al., 2020, 2022).
Traditionally, irrigation was considered precise if the same amount of water could be distributed evenly over the entire surface area, without taking into account the spatial variability of the soil and vegetation (Cabarcas et al., 2019). In contrast, precision irrigation pursues the objective of adapting water supplies to actual crop needs on a small scale (Fernández et al., 2019; Jiménez et al., 2022). Precision irrigation is still underused (Al-Agele et al., 2021a; Beyá-Marshall et al., 2022), but farmer interest is high and would be rapidly implemented if supported by adequate technical dissemination entrusted to new professional figures (Bwambale et al., 2022; Silva et al., 2022).
2.2. The approach to innovations in Mediterranean tree crops
Mediterranean tree crops such as olives, citrus fruits and vines have deep roots in our country, which run deep into our history. They represent an important reality for Italy in the trade balance with foreign countries and for the economies of many parts of the country (Biasi et al., 2012; Sgroi et al., 2015). Although Italian fruit-growing cannot be defined as a homogeneous system, it often suffers from the same structural problems common to other agricultural systems (de Ollas et al., 2019), which are leading to a downsizing of cultivated areas and production, and faces similar challenges (Testa et al., 2015; Medda et al., 2022). In order to do this, awareness of the sector's critical issues must be raised: its future will depend on the strength of the ideas that entrepreneurs and technicians will translate into concrete actions. For the future, it will be essential to transfer useful innovation to farms, both process and product (Pereira et al., 2020), by introducing into common practice the results of the great advances made in the field of sensor technology, for precise and timely monitoring of orchard conditions (Campos et al., 2019; Yildirim et al., 2021).
It will be necessary to take greater advantage of advances in mechanization to simplify cultivation operations and lower production costs, both for canopy and soil management (Sarri et al., 2015; Campos et al., 2019; Kourgialas et al., 2019). Planting systems and forms of cultivation will have to be revisited in order to exploit their potential in passive defense against pathogens and pests and climatic adversities. The success of such agricultural enterprises will also increasingly depend on their ability to collect and exploit the large amount of data that will be generated, especially to control costs and increase the quality of production (Keswani et al., 2019). It is essential to invest in skills creation in a sector characterized by operational processes based more on generational skills and knowledge transfer than on innovation and process optimisation (Wolf et al., 2001). The most present and easy to take up innovation for these crops has always been genetics (de Ollas et al., 2019; Pinto et al., 2021). The varietal landscape of these species of 30 years ago has, in fact, been completely turned upside down and this type of varietal innovation is justified by the need to have “another” product to market (Parra-López et al., 2021). Technological innovation for these crops, on the other hand, has a much harder time penetrating (Kudryashova and Casetti, 2021; Dinelli et al., 2022), and hardly ever seems to do so through processes that guarantee impartiality on the part of those who issue technical advice that has a cost, and therefore must guarantee a return (Sarri et al., 2015; Montanaro et al., 2017). From various works in the literature, it appears to be that age is the factor that most counteracts the fruit grower's propensity to adopt innovation, while the first mitigating factor would appear to be the availability of a person to provide assistance in the event of difficulties (Chen and Liang, 2020).
This study will seek to understand which psychological constructs actually influence the innovation decision-making process of agricultural entrepreneurs growing Mediterranean tree crops, and to do so it will take into account the Theory of Planned Behavior (TPB) through an extended conceptual model where other factors come into play that influence the intention to implement sustainable irrigation innovations in agriculture and thus complement and extend the classical model.
2.3. Research questions
TPB provides a theoretical framework for the systematic study of factors influencing behavioral choices and has been widely used in other studies to analyze behaviors such as leisure choices, driving offenses, shoplifting and fraud (Zhang et al., 2015). The Theory of Planned Behavior (TPB) states that the decision to take a particular action is directly related to the individual's behavioral intentions (Hansson et al., 2012; Gao et al., 2017; Soorani and Ahmadvand, 2019). Intention (I) is, in turn, influenced by three factors (Figure 1):
1. Attitude (ATT), the individual's favorable or unfavorable assessment of performing a behavior.
2. Subjective norm (SN), the individual's perception of social pressure to perform or not perform a behavior.
3. Perceived behavioral control (PBC), the individual's perception of their ability to perform a behavior.
This study proposes an integration of the Theory of Planned Behavior (TPB) by including additional variables to increase its predictive accuracy (Joao et al., 2015; Rezaei et al., 2018; Tama et al., 2021; Sarkar et al., 2022). This conceptual model considers, in addition to the three classical TPB factors, namely attitude (A), subjective norms (SN) and perceived behavioral control (PBC), three other factors (Hou and Hou, 2019) such as perceived innovation characteristics (PIC), Benefits (B), and Transferability (T) and hypothesizes that all these six elements could directly or indirectly influence innovation intention (Wauters et al., 2010; Müller et al., 2021). In the indirect case, this would be due to the effect of perceived innovation characteristics (PIC), which act as a link between the other factors and intention (Figure 2).
Attitude toward the adoption of an innovation in agriculture refers to an individual's positive or negative evaluation of its implementation (Senger et al., 2017; Tóth et al., 2020). The second determinant of intention in TPB, is the subjective norm, which refers to the perceived social pressure on the person from the peer group, family, society or culture to perform the behavior under consideration (Tóth et al., 2020; Sarkar et al., 2022). The objective is therefore to detect whether the adoption of sustainable innovation in agriculture is conditioned by third parties. The third factor within the TPB model is perceived behavioral control (PBC) which refers to the sense of self-efficacy or ability with respect to a potential behavior (Tóth et al., 2020; Saeedi et al., 2022). The fourth element considered for the conceptual model is defined as characteristics of perceived innovations (PIC) and is taken from the theory of diffusion of innovations (Rogers, 1995). Rogers, in his theory, mentions five characteristics of an innovation that can affect the relative rate of adoption by different members of a social system: relative advantage, compatibility, complexity, observability and trialability. All these factors influence the decision to adopt or not to adopt technological innovation (Figure 3).
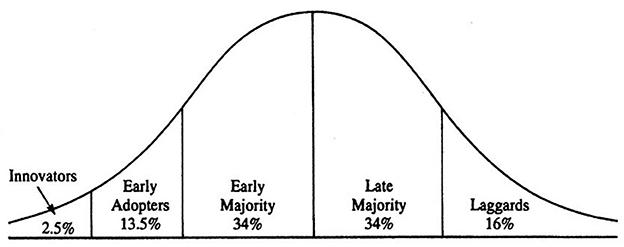
Figure 3. Diffusion of innovation theory. Source: Rogers (1995).
Among the additional variables considered, a further determinant of intention is transferability, which makes it possible to define whether the results of the experiment are transferable. Within the extended model, the final determinant of intention is the benefits, which allow the positive effects of innovation on the company to be measured.
Based on this knowledge, we formulated 11 hypotheses:
H1. Attitude toward the adoption of sustainable irrigation innovations in agriculture has a positive effect on behavioral intention.
H2. Attitude toward the adoption of sustainable irrigation innovations in agriculture has a positive effect on the characteristics of perceived innovations.
H3. Agricultural entrepreneurs, who perceived social pressure to adopt sustainable innovation, would be more likely to adopt it.
H4. Subjective norms have a positive effect on the characteristics of perceived sustainable irrigation innovations.
H5. Perceived behavioral control has a positive effect on the intention to innovate.
H6. Perceived behavioral control has a positive effect on perceived innovation characteristics.
H7. Perceived innovation characteristics have a positive effect on the intention toward the adoption of sustainable irrigation innovations in agriculture.
H8. The transferability of an innovation has a positive effect on the intention to innovate.
H9. The transferability of an innovation has a positive effect on the characteristics of perceived innovations.
H10. The benefits have a positive effect on the intention to innovate.
H11. Benefits have a positive effect on perceived innovation characteristics.
2.4. Data acquisition and processing
The survey to detect the propensity to adopt an innovation that favors the sustainability of the production process, i.e., respectful of the environment, animals, health and workers' rights, as well as the resulting economic and environmental benefits, was carried out through a specially designed questionnaire using the “Google Forms” tool and divided into 4 sections. It was disseminated online through the main social media channels between 6 June 2022 and 6 September 2022 with an active survey period of 90 days.
The first section deals with general information about the company, consisting of a series of questions about the company and the entrepreneur. The second section refers to the organizational choices of the surveyed farm manager, in terms of both needs and market. The third section concerns the analysis of the propensity to adopt an innovation. This section consists of a series of pre-defined questions designed to measure the behavior of the entrepreneur. The fourth section concerns the expected results following the adoption of an innovation. This section consists of a series of questions designed to capture key elements (such as perceived innovation characteristics, benefits and transferability of innovations) of the behavioral model. The latter two sections of the questionnaire are those concerning the TPB items in relation to the intention to adopt sustainable innovation and mostly use the 7-point Likert scale, where higher scores indicate greater compliance with the items, except for the benefits which were assessed on a multiple-choice format. Once the planning phase of the questionnaire had been completed and before starting data collection, we moved on to the control phase. At this stage, the necessary checks were carried out to ensure that there were no programming errors (bugs or malfunctions) and that the questionnaire was computerized appropriately to achieve the set research objectives. A total of 200 responses were collected from as many farms, of which 125 were selected as suitable for data analysis.
Previous studies have largely focused on socio-economic characteristics and ignored psychological factors influencing adoption intention (Borges et al., 2019). Instead, in this study, we sought to examine psychological factors by hypothesizing that these could explain greater variation in the dependent variable (the intention to implement innovation) than the socio-economic characteristics of farmers. The study aims to verify whether the TPB variables, together with transferability and benefits, predict the intention in relation to the adoption of sustainable innovation in agriculture. The data were analyzed using the Statistical Package for the Social Sciences (SPSS) version 27. First, we cleaned and checked the data to identify any missing values or irregularities. Secondly, we calculated descriptive statistics (e.g., averages and standard deviations). We checked the quality and adequacy of the measurement model and, through exploratory factor analysis (EFA), attempted to associate the variables with the various latent factors. Next, Pearson correlation coefficients were calculated to assess the correlation between the factors (Adnan et al., 2018). Therefore, we tested the causal relationships between the different factors of the TPB model with integrations by means of a hierarchical regression analysis, where we entered intention as the dependent variable and the TPB constructs as independent variables in the first stage, and then in the second stage we entered PIC as the dependent variable and the remaining constructs as independent variables (Saeedi et al., 2022).
3. Results
3.1. Socio-economic profile of participants
The results described in Table 1, show that the majority of respondents (94.4%) were male, confirming that the role of women is still marginal. Most of the respondents were between 31 and 50 years old (56.8%), while only 14.4% of the respondents were young people under 30. With regard to the legal form of the companies surveyed, it emerges that 84.8% of them are run by professional agricultural entrepreneurs and the form that prevails is that of the “Simple Company”, which accounts for 84% of the companies surveyed. With regard to farm size, we note that 48.80% of the farms cover an area of between 5 and 20 hectares, 41.60% have an area of less than 5 hectares and only 9.60% are identified as large farms with an area of more than 50 hectares. Looking at the production addresses, however, a homogeneous distribution appears, with citrus (19.2%), fruit (19.2%), and olive (17.6%) being most present, and only the mixed fodder/fruit address showing a very low percentage (0.8%) with only one answer. Most of the respondents (33.6%) completed their education, while 16.8% completed only primary education and 17.6% completed secondary education. Finally, with regard to the labor used in the company, 52% of the companies surveyed use family labor, the remainder (48%) use external labor.
The analysis of the questionnaires made it possible to construct Figure 4, which well expresses with a visual element the frequency with which a 'word or phrase' connected with a sustainable irrigation innovation is used by entrepreneurs in relation to other words in an irrigation dataset. In our case, the evaluations proposed in the optimization of water use were related to a number of parameters among the many possible ones and, among them, were considered:
• the quality of irrigation water;
• the cultivation method adopted (agronomic practices);
• the irrigation method used;
• the management of irrigation;
• the choice of when to irrigate (i.e., knowledge of the crop's evapotranspiration).
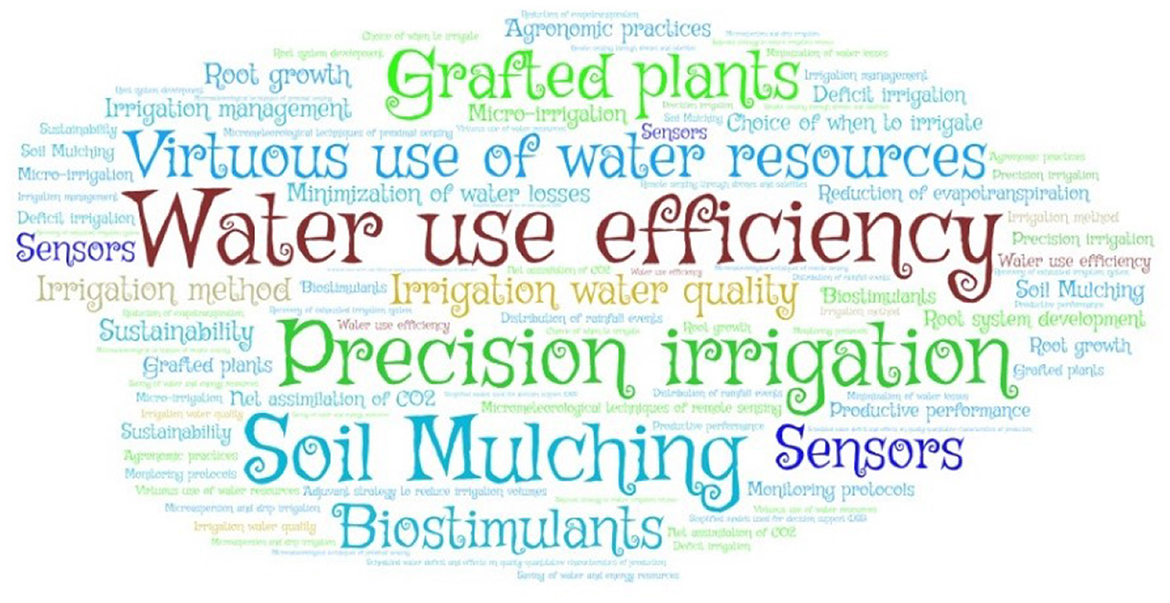
Figure 4. Frequency of relationships between words or phrases relevant to sustainable irrigation innovations*. *Our elaboration.
Minimization of water losses can be ensured through different agronomic practices such as (i) mulching, use of (ii) grafted plants and (iii) biostimulants. Mulching, in addition to preventing weed growth, reduces evapotranspiration, improves root growth and the uptake of water and nutrients, and increases water use efficiency. Similarly, the use of grafted plants, due to the better net CO2 assimilation and transpiration efficiency and the greater development of the root system, is another valid strategy to help reduce irrigation volumes. Not least, the use of microbial and non-microbial bio-stimulants can improve the morphological and physiological characteristics of crops, enhancing their productive performance and contributing to a more virtuous use of water resources.
The arboreal and mixed (arboricultural and other) farms that participated in the survey stated that they adopt an irrigation sustainability strategy partly for ethical reasons and partly to optimize water and energy consumption and achieve adequate levels of economic-productive performance (Figure 5). On the initiatives undertaken, in some cases there has been a prior study of the terrain, the type of planting and the characteristics of the irrigation service (with substantial differences between those who own irrigation investments such as wells, storage tanks, etc.) and those who acquire water from a public body in charge of the purpose (irrigation consortium mainly, which entails irrigation shifts, operating pressure, watering volumes, etc. that are not always dependent on their own will). Innovations in irrigation techniques include sprinkler and micro-irrigation systems. Drip lines are also widely used with micro-sprinklers to combine the positive effects of a drip system with overhead sprinklers. Finally, innovations in irrigation strategies were limited, such as the use of deficit irrigation combined with micro meteorological remote sensing and proximal sensing technologies, probably also due to the lack of adequate expertise.
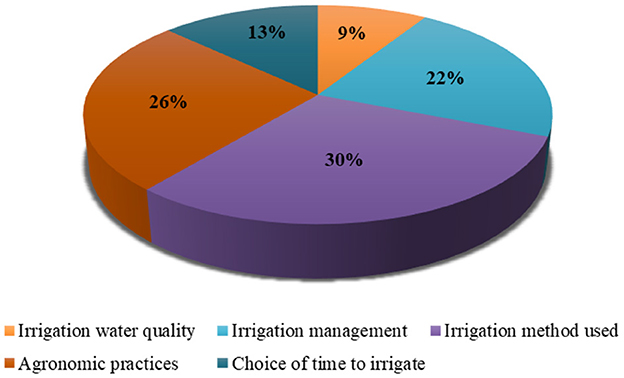
Figure 5. Sustainable irrigation innovations adopted by the sample of farms surveyed*. *Our elaboration.
3.2. Latent variables and extended model measurements
In order to extract latent variables from the questionnaire items, exploratory factor analysis (EFA) was used. Through the KMO and Bartlett's verification tests, it was possible to confirm the validity of the extended TPB model comprising seven latent factors indicating intention, attitude, subjective norm, PBC, PIC, benefit and transferability. The results show an adequate fit of the model (Kaiser-Meyer-Olkin measure of sampling adequacy = 0.66, Bartlett's test of sphericity with Sign < 0.001). Table 2 shows the number of items considered for the extraction of each latent factor and their standardized factor loadings. Each item corresponds to a question on the questionnaire that was measured by entering a single scale from 1 to 7 differentiated by individual question, where value 1 means Not at all agree/Absolutely unlikely and value 7 means Completely agree/Absolutely likely. Item factor loadings below 0.50 were discarded from the analysis. In order to assess the internal consistency and reliability of the scale, the study estimated Cronbach's alpha coefficients for each factor. Cronbach's alpha to assess internal consistency can be classified as: excellent (α ≥ 0.9), good (0.7 ≤ α < 0.9), acceptable (0.6 ≤ α < 0.7), poor (0.5 ≤ α < 0.6), and unacceptable (α < 0.5). The results show adequate internal consistency of the scale items, as Cronbach's alpha coefficients range from 0.70 to 0.96. In addition, descriptive analyses of the items were conducted and the table shows the mean and standard deviation, with the highest mean value for attitude and the lowest for benefits.
3.3. Correlations between variables
The results of Pearson's correlation coefficient test between the variables are shown in Table 3, which reveals significantly positive correlations between intention and all other variables in the model with the exception of transferability. There is also a good correlation between the variables, with a few exceptions, e.g., aptitude appears to be uncorrelated with PBC and transferability, just as there is no correlation between PBC and transferability itself, which appears to be the most problematic variable in this respect.
3.4. Behavior of the entrepreneur
In order to test the general relationships between the variables and thus answer the assumptions made, two different linear regressions were conducted. The first was performed in order to understand which variables influence the intention to adopt the innovation and therefore intention was set as the dependent variable and TPB constructs as independent variables. The second regression was aimed at understanding the mediating effect exerted by the characteristics of perceived innovations (PIC) against intention for the other variables, thus setting PIC as the dependent variable. With regard to the first regression, the ANOVA table shows an F-value of 11.43 and a significance level p of < 0.001, the regression model therefore fitted well. The summary table of the model shows that R2 has a value of 0.52, which indicates that 52% of the variance of intention can be explained by attitude (ATT), subjective norm (SN), perceived behavioral control (PBC), perceived innovation characteristics (PIC), benefits (B) and transferability. The results in Table 4 show that intention is strongly determined by attitude (ATT) as the most important variable influencing behavior (B: 0.337, significance level p < 0.001). Subjective Norms (SN) (B: 0.377, significance level p = 0.001) and Perceived Innovations Characteristics (PIC) (B: 0.263, significance level = 0.008) also show a good level of influence toward intention. The remaining factors such as perceived behavioral control (PBC) (B: 0.042, significance level p = 0.647), benefits (B) (B: −0.044, significance level p = 0.651) and transferability (T) (B: −0.077, significance level p = 0.449), as we expected, do not directly influence intention.
The second regression shows an ANOVA table with F equal to 17.84 and a significance level p equal to < 0.001, the regression model therefore fitted well. The summary table of the model shows that the R2 has a value of 0.58, which indicates that 58% of the variance in the characteristics of perceived innovations can be explained by attitude (ATT), subjective norm (SN), perceived behavioral control (PBC), benefits (B), and transferability. The results in Table 5 show that perceived behavioral control (PBC) is directly related to PIC (B: 0.222, significance level p = 0.007), the same applies to benefits (B) (B: 0.211, significance level p = 0.010) and transferability (T) (B: 0.354, significance level p < 0.001).
The results show, therefore, that only attitude (ATT) subjective norms (SN) directly influence the intention to adopt sustainable irrigation innovations in agriculture (I). Other factors such as perceived behavioral control (PBC), benefits (B), and transferability (T), indirectly influence intention, due to the effect of perceived innovation characteristics (PIC), which influences intention (I) by acting as a mediator between PBC, T, B with I, and thus acting on the psychology of the individual.
4. Discussion
Innovation in agriculture is an increasingly relevant topic. It is seen as a broad concept that includes the creation and/or adoption of innovations that may be new to the enterprise, new to the market or new to the world (Kalaitzandonakes et al., 2018; Despotović et al., 2019). It is absolutely necessary to enable Italian farmers to benefit from technological innovation because growth and sustainable development inevitably also come from knowledge transfer. We must innovate for a sustainable future. The role of agriculture is increasingly strategic in responding to major global challenges such as growing food demand, climate change, the energy crisis, and natural resource scarcity. But in order to produce more and better, polluting less, the primary sector must be able to count on large investments in research and innovation: only from here can the answers come to combine increased farm income and food resources, without altering the already too fragile environmental balance. The management of water resources, in relation to current climate changes, will lead to a rational use together with the analysis of specific crop needs in order to avoid any form of waste. In this scenario, the choice in farm cropping will increasingly shift toward crops with lower water requirements and water-saving distribution methods. Regarding the first point, relating to reductions in water requirements, these may result from the application of agronomic techniques, the choice of rootstock-graft combination as well as mass selections in the field. With regard to the second point, the current trend is toward micro-aspersion methods (Kourgialas et al., 2022) together with the application of water deficit techniques, which in some cases save water and improve crop quality. The trend is to develop true precision irrigation, which will allow the plant to always be guaranteed the amount of water it needs (Adeyemi et al., 2017; Velasco-Muñoz et al., 2019; Caruso et al., 2021) based on a system of information that will come to us from farm big data, collected by a network of sensors and weather sheds capable of managing irrigation through practices that connect to artificial intelligence as the new challenge of agriculture 4.0.
The Mediterranean tree crop production sector is experiencing difficult years that have led in some cases to a sharp downsizing of cultivated areas. Phytosanitary and climatic emergencies, product remunerations that are often lower than production costs, structural and bureaucratic problems have put many fruit farms to the test (Sgroi et al., 2015; Kourgialas et al., 2022). The challenge for the future is certainly to maintain high yields and fruit quality while using fewer resources. It will not be enough to produce new knowledge; much will depend on the ability of production systems to quickly introduce the right innovations in the fields (Caruso et al., 2021). There will be an increasing need for up-to-date and high-profile technical-scientific dissemination. Taking the adoption of innovation as a point of view, the contribution of this research is useful to explore the behavioral intentions of farmers cultivating Mediterranean tree crops toward the intention to adopt innovations.
The study aims to test the predictive validity of an extended TPB model, which considers not only the classical three variables, but also the characteristics of perceived innovations, transferability and benefits in relation to the adoption of innovations in agriculture. The results confirmed that the extended TPB is a useful model to clarify which psychological factors drive citrus, grapevine and olive entrepreneurs in adopting innovations. The results suggest that attitude, subjective norms and PIC significantly influence intention, thus supporting Hypotheses 1, 3, and 7, respectively. Whereas, PBC, transferability and benefits are significantly influential in explaining PIC but do not directly influence intention to innovate. Thus, Hypotheses 5, 9, and 11 hold, but Hypotheses 6, 8, and 10 are not significant and are therefore rejected. Hypotheses 2 and 4 are rejected, as the values are found to be non-significant and prove that PIC is not influenced by attitude and subjective norms. The results showed that entrepreneurs' intentions to adopt an innovation are explained by attitudes toward innovation adoption and subjective norms. Indeed, of all the variables, they are the most influential in predicting the adoption of sustainable innovation in agriculture. The greater impact of attitude and subjective norms on intentions in relation to the other constructs reveals that the people with whom we relate have such a significant influence that they have power over choice behavior. Perceived innovation characteristics are also significant predictors of entrepreneurs' intention to innovate. The impact of PIC on intentions suggests that less uncertainty and concern for companies, which often face a number of unfavorable factors (low financial resources, high costs, lack of knowledge about innovations), could be helpful in increasing intentions. Perceived behavioral control, transferability and benefits do not appear to have a direct impact on intention, but indirectly through the mediating effect of PIC, with positive values.
Current efforts to improve quality, company design and technological conditions are very low, confirming our study, as 53.2% of the companies stated that they had not made any innovations in the 5 years prior to the interview. Our analysis confirmed that entrepreneurs' positive attitude toward innovation directly increases their intention to implement more innovation. In summary, this study confirmed the predictive validity of TPB, with the integration of PIC, transferability and benefits, to explain the entrepreneur's intention to adopt sustainable innovations in agriculture.
5. Conclusion
The value of innovation as a fundamental strategy for growth policies and the development of competitiveness in the primary sector has strengthened over the years, acquiring ever greater dimensions. Indeed, it is regarded as an important and necessary component for the development of agricultural activities (Bowman and Zilberman, 2013; Spendrup and Fernqvist, 2019). In order to effectively motivate landowners' behavior toward innovation, policy-makers need to understand the characteristics of the decision-makers that influence their intentions to adopt various innovations, highlighting the importance of both observable and unobservable factors underlying farmers' decisions. Our study sought to address this need by identifying which unobservable socio-psychological factors influence farmers' intentions. Indeed, this study adds a contribution to the existing scientific literature by analyzing the psychological factors influencing the intentions of farmers producing Mediterranean tree crops toward the application of sustainable irrigation innovations based on an extended TPB model, especially in the context of a developing agro-economy.
The results indicate that, TPB factors can explain farmers' behavioral intentions to apply on-farm innovations, but also that the addition of three other constructs (PIC, benefits, and transferability) in the TPB framework can increase the predictive power and accuracy of the theory. Based on our results, it can be seen that the direct effect on the intention to innovate is negative, although the direct influence on innovation is positive. This means that the companies studied would like to innovate, but feel that their innovation capabilities are not sufficient to implement adequate innovation projects. Our analysis confirmed that entrepreneurs' positive attitude toward innovation directly increases the intention to implement more innovation, as it is a significant predictor of intention. Furthermore, based on the greater impact of subjective norms on intention, it appears that the combination of extension services to improve the level of knowledge on the importance of innovations could significantly influence farmers' attitudes on the intention to apply them.
In particular, given the direct effect of PIC on intention, communication policies aimed at promoting the adoption of sustainable innovations by farmers should mainly emphasize their characteristics and the benefits they can bring to businesses. These policies could be coordinated by public authorities (in Italy, mainly the Ministry of the Environment and Agricultural Policies) and environmental associations (e.g., Legambiente, WWF, etc.). These organizations could devise and disseminate messages that attract farmers with an innovative vision and try to stimulate the modernization of the agri-food system, e.g., through workshops, meetings on this topic or even ad hoc training programmes and/or projects with developers of agricultural innovations.
We recognize the limitations of this study, pointing out that other factors not considered may also influence actual behavior between the time the intention is formed and its translation into practice. The model proposed in this study did not consider farmers' emotions, e.g., fear/threat, positive or negative feelings. The literature has, in fact, shown that this is an inherent weakness of TPB, as no human behavior is independent of emotions (Zhang, 2018). Moreover, farmers are more likely to adopt less risky agricultural practices and technologies when the existing production risk is high (Beyene and Kassie, 2015). Therefore, in order to identify farmers' intentions toward the implementation of on-farm innovations, we suggest adding other psychological constructs, e.g., risks, subsidies or government incentives to future studies. Despite the limitations, however, it is believed that the study contributes to the growth of a line of research based on the intention to innovate in agriculture, because it succeeds in highlighting the benefits of combining economic and psychological perspectives through the study of the entrepreneur's intentions.
The future of the implementation of innovations in agriculture will be facilitated by the upcoming availability of PNRR funds. Following the enactment of the EU's Next Generation Plan, the Italian agricultural system, through the National Recovery and Resilience Plan (PNRR), will have the opportunity to utilize special measures dedicated to the country's green and digital development. From a total of EUR 750 billion, Italy has been allocated EUR 191.5 billion (70 in grants and 121 in loans) of which a large part is earmarked for production systems. In this context, it will be crucial to define management models and innovation packages that favor innovation in productive agricultural systems, including irrigation, so that the implementation of innovations does not present barriers to entry and allows for a rapid and profitable nationwide diffusion in all production systems, based on the assumption that without innovation there is no future. The recent enactment of the Ministry of Agriculture and Food Sovereignty also focuses on the role of agricultural production from an economic, social and environmental perspective, objectives that can be achieved today thanks to the process and product innovations available as a result of the Digital Transformation taking place with artificial intelligence in the forefront.
In conclusion, this study may help to formulate future research that can combine psychological and socio-economic factors to understand the dynamics of innovation adoption and we are confident that this would help to understand whether future findings from other countries will follow the patterns highlighted in this study and/or how the difficulties encountered in innovation have been addressed.
Data availability statement
The original contributions presented in the study are included in the article/supplementary material, further inquiries can be directed to the corresponding author.
Author contributions
All authors listed have made a substantial, direct, and intellectual contribution to the work and approved it for publication.
Funding
Miglioramento delle produzioni agroalimentari mediterranee in condizioni di carenza di risorse idriche -WATER4AGRIFOOD-cod. CUP: B64I20000160005, PON RICERCA E INNOVAZIONE 2014-2020, Azione II-Obiettivo Specifico 1b, and “Sostenibilità ed innovazioni della ricerca in agricoltura, alimentazione ed ambiente”, Scientific Responsible Prof. Mario D'amico, UPB: 5A722192180.
Conflict of interest
The authors declare that the research was conducted in the absence of any commercial or financial relationships that could be construed as a potential conflict of interest.
Publisher's note
All claims expressed in this article are solely those of the authors and do not necessarily represent those of their affiliated organizations, or those of the publisher, the editors and the reviewers. Any product that may be evaluated in this article, or claim that may be made by its manufacturer, is not guaranteed or endorsed by the publisher.
References
Abioye, E. A., Abidin, M. S. Z., Mahmud, M. S. A., Buyamin, S., Ishak, M. H. I., Rahman, M. K. I. A., et al. (2020). A review on monitoring and advanced control strategies for precision irrigation. Comput. Electron. Agric. 173, 105441. doi: 10.1016/j.compag.2020.105441
Abioye, E. A., Hensel, O., Esau, T. J., Elijah, O., Abidin, M. S. Z., Ayobami, A. S., et al. (2022). Precision irrigation management using machine learning and digital farming solutions. AgriEng. 4, 70–103. doi: 10.3390/agriengineering4010006
Adeyemi, O., Grove, I., Peets, S., and Norton, T. (2017). Advanced monitoring and management systems for improving sustainability in precision irrigation. Sustainability 9, 353. doi: 10.3390/su9030353
Adnan, N., Nordin, S. M., and Ali, M. (2018). A solution for the sunset industry: Adoption of Green Fertiliser Technology amongst Malaysian paddy farmers. Land Use Policy 79, 575–584. doi: 10.1016/j.landusepol.2018.08.033
Adnan, N., Nordin, S. M., and bin Abu Bakar, Z. (2017). Understanding and facilitating sustainable agricultural practice: A comprehensive analysis of adoption behaviour among Malaysian paddy farmers. Land Use Policy 68, 372–382. doi: 10.1016/j.landusepol.2017.07.046
Aksoy, H. (2017). How do innovation culture, marketing innovation and product innovation affect the market performance of small and medium-sized enterprises (SMEs)? Technol. Soc. 51, 133–141. doi: 10.1016/j.techsoc.2017.08.005
Al-Agele, H. A., Nackley, L., and Higgins, C. (2021a). Testing novel new drip emitter with variable diameters for a variable rate drip irrigation. Agriculture 11, 1–8. doi: 10.3390/agriculture11020087
Al-Agele, H. A., Nackley, L., and Higgins, C. W. (2021b). A pathway for sustainable agriculture. Sustainability 13, 4328. doi: 10.3390/su13084328
Alam, S. S., Ahmad, M., Othman, A. S., Shaari, Z. B. H., and Masukujjaman, M. (2021). Factors affecting photovoltaic solar technology usage intention among households in Malaysia: Model integration and empirical validation. Sustainability 13, 1–20. doi: 10.3390/su13041773
Asadi, E., Isazadeh, M., Samadianfard, S., Ramli, M. F., Mosavi, A., Nabipour, N., et al. (2020). Groundwater quality assessment for sustainable drinking and irrigation. Sustainability 12, 177. doi: 10.3390/su12010177
Assouline, S., Russo, D., Silber, A., and Or, D. (2015). Balancing water scarcity and quality for sustainable irrigated agriculture. Water Resour. Res. 51, 3419–3436. doi: 10.1002/2015WR017071
Aznar-Sánchez, J. A., Belmonte-Ureña, L. J., Velasco-Muñoz, J. F., and Manzano-Agugliaro, F. (2018). Economic analysis of sustainable water use: A review of worldwide research. J. Cleaner Prod. 198, 1120–1132. doi: 10.1016/j.jclepro.2018.07.066
Bafdal, N., Dwiratna, S., Suryadi, E., and Kendarto, D. R. (2018). Water harvesting as a technological innovation and greater solving of climatic change impact to supply fertigation. Int. J. Adv. Sci. Eng. Inf. Technol. 8, 2380–2385. doi: 10.18517/ijaseit.8.6.7697
Barth, H., Ulvenblad, P. O., and Ulvenblad, P. (2017). Towards a conceptual framework of sustainable business model innovation in the agri-food sector: A systematic literature review. Sustainability 9, 1620. doi: 10.3390/su9091620
Bechini, L., Costamagna, C., Zavattaro, L., Grignani, C., Bijttebier, J., and Ruysschaert, G. (2015). Barriers and drivers towards the incorporation of crop residue in the soil. Analysis of Italian farmers' opinion with the theory of planned behaviour. Italian J. Agron. 10, 178–184. doi: 10.4081/ija.2015.663
Berti, G., and Mulligan, C. (2016). Competitiveness of small farms and innovative food supply chains: The role of food hubs in creating sustainable regional and local food systems. Sustainability 8, 616. doi: 10.3390/su8070616
Beyá-Marshall, V., Arcos, E., Seguel, Ó., Galleguillos, M., and Kremer, C. (2022). Optimal irrigation management for avocado (cv.'Hass') trees by monitoring soil water content and plant water status. Agric. Water Manage. 271, 107794. doi: 10.1016/j.agwat.2022.107794
Beyene, A. D., and Kassie, M. (2015). Speed of adoption of improved maize varieties in Tanzania: An application of duration analysis. Technol. Forec. Soc. Change 96, 298–307. doi: 10.1016/j.techfore.2015.04.007
Biasi, R., Botti, F., Barbera, G., and Cullotta, S. (2012). The role of Mediterranean fruit tree orchards and vineyards in maintaining the traditional agricultural landscape. Acta Horticulturae 940, 79–88. doi: 10.17660/ActaHortic.2012.940.9
Bigliardi, B., and Filippelli, S. (2022). Sustainability and open innovation: main themes and research trajectories. Sustainability 14, 6763. doi: 10.3390/su14116763
Bonfante, A., Monaco, E., Manna, P., de Mascellis, R., Basile, A., Buonanno, M., et al. (2019). LCIS DSS—An irrigation supporting system for water use efficiency improvement in precision agriculture: A maize case study. Agric. Syst. 176, 102646. doi: 10.1016/j.agsy.2019.102646
Borges, J. A. R., Oude Lansink, A. G. J. M., and Emvalomatis, G. (2019). Adoption of innovation in agriculture: A critical review of economic and psychological models. Int. J. Innov. Sustain. Develop. 13, 36–56. doi: 10.1504/IJISD.2019.096705
Borges, J. A. R., Oude Lansink, A. G. J. M., Marques Ribeiro, C., and Lutke, V. (2014). Understanding farmers' intention to adopt improved natural grassland using the theory of planned behavior. Livestock Sci. 169, 163–174. doi: 10.1016/j.livsci.2014.09.014
Bowman, M. S., and Zilberman, D. (2013). Economic factors affecting diversified farming systems. Ecol. Soc. 18, 14. doi: 10.5751/ES-05574-180133
Bowmer, K. H., and Meyer, W. S. (2014). “Irrigation agriculture: Sustainability through holistic approaches to water use and innovation,” in Drinking Water and Water Management: New Research 181–224.
Brudermann, T., Reinsberger, K., Orthofer, A., Kislinger, M., and Posch, A. (2013). Photovoltaics in agriculture: A case study on decision making of farmers. Energy Policy 61, 96–103. doi: 10.1016/j.enpol.2013.06.081
Bwambale, E., Abagale, F. K., and Anornu, G. K. (2022). Smart irrigation monitoring and control strategies for improving water use efficiency in precision agriculture: A review. Agric. Water Manage. 260, 107324. doi: 10.1016/j.agwat.2021.107324
Cabarcas, A., Arrieta, C., Cermeno, D., Leal, H., Mendoza, R., and Rosales, C. (2019). “Irrigation system for precision agriculture supported in the measurement of environmental variables,” in Proceedings - 2019 7th International Engineering, Sciences and Technology Conference, IESTEC 2019, 671–676. doi: 10.1109/IESTEC46403.2019.00125
Campana, P. E., Lastanao, P., Zainali, S., Zhang, J., Landelius, T., and Melton, F. (2022). Towards an operational irrigation management system for Sweden with a water–food–energy nexus perspective. Agric. Water Manage. 271, 107734. doi: 10.1016/j.agwat.2022.107734
Campos, J., Llop, J., Gallart, M., García-Ruiz, F., Gras, A., Salcedo, R., et al. (2019). Development of canopy vigour maps using UAV for site-specific management during vineyard spraying process. Prec. Agric. 20, 1136–1156. doi: 10.1007/s11119-019-09643-z
Canaj, K., Morrone, D., Roma, R., Boari, F., Cantore, V., and Todorovic, M. (2021). Reclaimedwater for vineyard irrigation in a mediterranean context: Life cycle environmental impacts, life cycle costs, and eco-efficiency. Water 13, 2242. doi: 10.3390/w13162242
Capitanio, F., Coppola, A., and Pascucci, S. (2010). Product and process innovation in the Italian food industry. Agribusiness 26, 503–518. doi: 10.1002/agr.20239
Caruso, G., Palai, G., Caruso, M., Roccuzzo, G., Stagno, F., Zarco-Tejada, P. J., et al. (2021). Using an unmanned platform and VIS-NIR cameras to determine biophysical and geometrical parameters of olive, grapevine and citrus canopies. Acta Horticulturae 1314, 345–352. doi: 10.17660/ActaHortic.2021.1314.43
Chen, C. C., and Liang, C. (2020). Evoking agriculture entrepreneurship: How younger and older farmers differ. Sustainability 12, 7005. doi: 10.3390/su12177005
Chi, S. Y., and Chien, L. H. (2022). Influence of ecological and quality concerns on the adoption intention of environment-smart agricultural systems. Appl. Ecol. Environ. Res. 20, 363–381. doi: 10.15666/aeer/2001_363381
Coghlan, C., Labrecque, J. A., Ma, Y., and Dub,é, L. (2020). A biological adaptability approach to innovation for small and medium enterprises (SMEs): Strategic insights from and for health-promoting agri-food innovation. Sustainability 12, 4227. doi: 10.3390/su12104227
Curry, G. N., Nake, S., Koczberski, G., Oswald, M., Rafflegeau, S., Lummani, J., et al. (2021). Disruptive innovation in agriculture: Socio-cultural factors in technology adoption in the developing world. J. Rural Stud. 88, 422–431. doi: 10.1016/j.jrurstud.2021.07.022
Dawit, M., Dinka, M. O., and Halefom, A. (2022). Farmers' perception of climate change and gender sensitive perspective for optimised irrigation in a compound surface-ground water system. J. Water Land Develop. 52, 265–271.
de Oliveira Padilha, L. G., Malek, L., and Umberger, W. J. (2022). Consumers' attitudes towards lab-grown meat, conventionally raised meat and plant-based protein alternatives. Food Quality and Preference 99. doi: 10.1016/j.foodqual.2022.104573
de Ollas, C., Morillón, R., Fotopoulos, V., Puértolas, J., Ollitrault, P., Gómez-Cadenas, A., et al. (2019). Facing climate change: Biotechnology of iconic mediterranean woody crops. Front. Plant Sci. 10, 427. doi: 10.3389/fpls.2019.00427
Despotović, J., Rodić, V., and Caracciolo, F. (2019). Factors affecting farmers' adoption of integrated pest management in Serbia: An application of the theory of planned behavior. J. Cleaner Prod. 228, 1196–1205. doi: 10.1016/j.jclepro.2019.04.149
Dinelli, G., Chen, Q., Scuderi, A., Via, G., la, Timpanaro, G., and Sturiale, L. (2022). The digital applications of “Agriculture 4.0”: Strategic opportunity for the development of the italian citrus chain. Agriculture. 12, 400. doi: 10.3390/agriculture12030400
Douthwaite, B., and Hoffecker, E. (2017). Towards a complexity-aware theory of change for participatory research programs working within agricultural innovation systems. Agric. Syst. 155, 88–102. doi: 10.1016/j.agsy.2017.04.002
El Bilali, H. (2019). Innovation-sustainability nexus in agriculture transition: case of agroecology. Open Agric. 4, 1–16. doi: 10.1515/opag-2019-0001
Ermolieva, T., Havlik, P., Frank, S., Kahil, T., Balkovic, J., Skalsky, R., et al. (2022). A risk-informed decision-making framework for climate change adaptation through robust land use and irrigation planning. Sustainability 14, 1430. doi: 10.3390/su14031430
Feo, E., Burssens, S., Mareen, H., and Spanoghe, P. (2022a). Shedding light into the need of knowledge sharing in H2020 thematic networks for the agriculture and forestry innovation. Sustainability 14, 3951. doi: 10.3390/su14073951
Feo, E., Spanoghe, P., Berckmoes, E., Pascal, E., Mosquera-Losada, R., Opdebeeck, A., et al. (2022b). The multi-actor approach in thematic networks for agriculture and forestry innovation. Agric. Food Econ. 10, 3. doi: 10.1186/s40100-021-00209-0
Fernández, J. E., Diaz-Espejo, A., Hernandez-Santana, V., and Cuevas, M.v. (2019). Does precision irrigation help to reduce water consumption in agriculture? Acta Hortic. 1253, 199–205. doi: 10.17660/ActaHortic.2019.1253.27
Fieldsend, A. F., Varga, E., Bir,ó, S., von Münchhausen, S., and Häring, A. M. (2022). Multi-actor co-innovation partnerships in agriculture, forestry and related sectors in Europe: Contrasting approaches to implementation. Agric. Syst. 202, 103472. doi: 10.1016/j.agsy.2022.103472
Galanakis, C. M., Rizou, M., Aldawoud, T. M. S., Ucak, I., and Rowan, N. J. (2021). Innovations and technology disruptions in the food sector within the COVID-19 pandemic and post-lockdown era. Trends Food Sci. Technol. 110, 193–200. doi: 10.1016/j.tifs.2021.02.002
Gambelli, D., Solfanelli, F., Orsini, S., and Zanoli, R. (2021). Measuring the economic performance of small ruminant farms 2 using balanced scorecard and importance-performance analy-sis: a European case study. Sustainability. 13, 3321. doi: 10.3390/su13063321
Gao, L., Wang, S., Li, J., and Li, H. (2017). Application of the extended theory of planned behavior to understand individual's energy saving behavior in workplaces. Resour. Conserv. Recycl. 127, 107–113. doi: 10.1016/j.resconrec.2017.08.030
Guaitero, B., Saavedra, D. P., Ariza, C., Rugeles, L., and Saavedra, D. (2013). Measuring innovation in agricultural firms: a methodological approach. Electr. J. Knowl. Manag. 11, 185.
Gutiérrez, J. A., and Macken-Walsh, Á. (2022). Ecosystems of collaboration for sustainability-oriented innovation: the importance of values in the agri-food value-chain. Sustainability 14, 11205. doi: 10.3390/su141811205
Hannus, V., and Sauer, J. (2021). Understanding farmers' intention to use a sustainability standard: The role of economic rewards, knowledge, and ease of use. Sustainability 13, 10788. doi: 10.3390/su131910788
Hansson, H., Ferguson, R., and Olofsson, C. (2012). Psychological constructs underlying farmers' decisions to diversify or specialise their businesses - an application of theory of planned behaviour. J. Agric. Econ. 63, 465–482. doi: 10.1111/j.1477-9552.2012.00344.x
Harwiki, W., and Malet, C. (2020). Quintuple helix and innovation on performance of SMEs within ability of SMEs as a mediator variable: A comparative study of creative industry in Indonesia and Spain. Manage. Sci. Lett. 10, 18. doi: 10.5267/j.msl.2019.11.018
Hashem, M. S., El-Abedin, T. Z., and Al-Ghobari, H. M. (2019). Rational water uses by applying regulated deficit and partial root-zone drying irrigation techniques in tomato under arid conditions. Chilean J. Agric. Res. 79, 75–88. doi: 10.4067/S0718-58392019000100075
Hasler, K., Olfs, H. W., Omta, O., and Bröring, S. (2016). Drivers for the adoption of eco-innovations in the German fertilizer supply chain. Sustainability 8, 682. doi: 10.3390/su8080682
Hou, J., and Hou, B. (2019). Farmers' adoption of low-carbon agriculture in China: An extended theory of the planned behavior model. Sustainability 11, 1399. doi: 10.3390/su11051399
Hsieh, R. M., and Kelley, D. J. (2016). The role of cognition and information access in the recognition of innovative opportunities. J. Small Bus. Manag. 54, 297–311. doi: 10.1111/jsbm.12300
Iocola, I., Angevin, F., Bockstaller, C., Catarino, R., Curran, M., Messéan, A., et al. (2020). An actor-oriented multi-criteria assessment framework to support a transition towards sustainable agricultural systems based on crop diversification. Sustainability 12, 5434. doi: 10.3390/su12135434
Issa, I., and Hamm, U. (2017). Adoption of organic farming as an opportunity for Syrian farmers of fresh fruit and vegetables: An application of the theory of planned behaviour and structural equation modelling. Sustainability 9, 2024. doi: 10.3390/su9112024
Jiménez, A. F., Cárdenas, P. F., and Jiménez, F. (2022). Intelligent IoT-multiagent precision irrigation approach for improving water use efficiency in irrigation systems at farm and district scales. Comput. Electr. Agric. 192, 106635. doi: 10.1016/j.compag.2021.106635
Joao, A. R. B., Luzardo, F., and Vanderson, T. X. (2015). An interdisciplinary framework to study farmers decisions on adoption of innovation: Insights from Expected Utility Theory and Theory of Planned Behavior. African J. Agric. Res. 10, 2814–2825. doi: 10.5897/AJAR2015.9650
Judge, M., Warren-Myers, G., and Paladino, A. (2019). Using the theory of planned behaviour to predict intentions to purchase sustainable housing. J. Cleaner Prod. 215, 259–267. doi: 10.1016/j.jclepro.2019.01.029
Jun, Y., and Kim, K. (2022). Developing an open innovation attitude assessment framework for organizations: focusing on open innovation role perspective and locus of activity. Behav. Sci. 12, 46. doi: 10.3390/bs12020046
Kalaitzandonakes, N., Carayannis, E. G., Grigoroudis, E., and Rozakis, S. (2018). Introduction: innovation and technology transfer in agriculture. Innov. Technol. Knowl. Manag. 35, 1–10. doi: 10.1007/978-3-319-67958-7_1
Kalinin, A., Teplinsky, I., and Ustroev, A. (2018). Substantiation of tillage methods aimed at rational usage of water resources. Eng. Rural Develop. 17, 392–399. doi: 10.22616/ERDev2018.17.N517
Keswani, B., Mohapatra, A. G., Mohanty, A., Khanna, A., Rodrigues, J. J. P. C., Gupta, D., et al. (2019). Adapting weather conditions based IoT enabled smart irrigation technique in precision agriculture mechanisms. Neural Comput. Applic. 31, 277–292. doi: 10.1007/s00521-018-3737-1
Khandaker, M., and Kotzen, B. (2018). The potential for combining living wall and vertical farming systems with aquaponics with special emphasis on substrates. Aquac. Res. 49, 1454–1468. doi: 10.1111/are.13601
Kim, S. J., Kim, K. H., and Choi, J. (2019). The Role of Design Innovation in Understanding Purchase Behavior of Augmented Products. J. Bus. Res. 99, 354–362. doi: 10.1016/j.jbusres.2017.09.047
Klerkx, L., and Begemann, S. (2020). Supporting food systems transformation: The what, why, who, where and how of mission-oriented agricultural innovation systems. Agric. Syst. 184, 102901. doi: 10.1016/j.agsy.2020.102901
Klerkx, L., van Mierlo, B., and Leeuwis, C. (2012). “Evolution of systems approaches to agricultural innovation: Concepts, analysis and interventions,” in Farming Systems Research into the 21st Century: The New Dynamic (Netherlands: Springer) 457–483. doi: 10.1007/978-94-007-4503-2_20
Kourgialas, N. N., Hliaoutakis, A., Argyriou, A. v, Morianou, G., Voulgarakis, A. E., et al. (2022). A web-based GIS platform supporting innovative irrigation management techniques at farm-scale for the Mediterranean island of Crete. Sci. Total Environ. 842, 156918. doi: 10.1016/j.scitotenv.2022.156918
Kourgialas, N. N., Koubouris, G. C., and Dokou, Z. (2019). Optimal irrigation planning for addressing current or future water scarcity in Mediterranean tree crops. Sci. Total Environ. 654, 616–632. doi: 10.1016/j.scitotenv.2018.11.118
Kubankova, M., Hajek, M., and Votavova, A. (2016). Environmental and social value of agriculture innovation. Agric. Econ. 62, 101–112. doi: 10.17221/58/2015-AGRICECON
Kudryashova, E., and Casetti, M. (2021). The Internet of Things - the Nearest Future of Viticulture. Agris On-Line Papers Econ. Inform. 13, 79–86. doi: 10.7160/aol.2021.130206
Kukal, M. S., and Irmak, S. (2018). Climate-driven crop yield and yield variability and climate change impacts on the U.S. Great Plains Agric. Prod. 8, 1–8. doi: 10.1038/s41598-018-21848-2
Kumar, D. M., Sharma, V., Govindarajo, N. S., and Rahmawati. (2021). Agriculture 4.0 and smart farming: Imperatives of scaling up innovation and farmer capabilities for sustainable business. Indian J. Ecol. 48, 1–8.
Lang, Z., and Rabotyagov, S. (2022). Socio-psychological factors influencing intent to adopt conservation practices in the Minnesota River Basin. J. Environ. Manage. 307. doi: 10.1016/j.jenvman.2022.114466
Läpple, D., Renwick, A., and Thorne, F. (2015). Measuring and understanding the drivers of agricultural innovation: Evidence from Ireland. Food Policy 51, 1–8. doi: 10.1016/j.foodpol.2014.11.003
Liu, J., Hertel, T. W., Lammers, R. B., Prusevich, A., Baldos, U. L. C., Grogan, D. S., et al. (2017). Achieving sustainable irrigation water withdrawals: Global impacts on food security and land use. Environ. Res. Lett. 12, 104009. doi: 10.1088/1748-9326/aa88db
Liu, T., Bruins, R. J. F., and Heberling, M. T. (2018). Factors influencing farmers' adoption of best management practices: A review and synthesis. Sustainability. 10, 432. doi: 10.3390/su10020432
López-Felices, B., Aznar-Sánchez, J. A., Velasco-Muñoz, J. F., and Piquer-Rodríguez, M. (2020). Contribution of irrigation ponds to the sustainability of agriculture. A review of worldwide research. Sustainability 12, 5425. doi: 10.3390/su12135425
Lopriore, G., and Caliandro, A. (2022). Irrigation of intensive olive groves in the Mediterranean environment with different water regimes on two different soils: effects on yields, water use efficiency, vegetative behaviour and water status of the crop. Acta Hortic. 1335, 541–548. doi: 10.17660/ActaHortic.2022.1335.68
Loures, L., Chamizo, A., Ferreira, P., Loures, A., Castanho, R., and Panagopoulos, T. (2020). Assessing the effectiveness of precision agriculture management systems in mediterranean small farms. Sustainability 12, 3765. doi: 10.3390/su12093765
Maizza, A., Fait, M., Scorrano, P., and Iazzi, A. (2019). How knowledge sharing culture can become a facilitator of the sustainable development in the agrifood sector. Sustainability 11, 952. doi: 10.3390/su11040952
Malek, K., Adam, J. C., Stöckle, C. O., and Peters, R. T. (2018). Climate change reduces water availability for agriculture by decreasing non-evaporative irrigation losses. J. Hydrol. 561, 444–460. doi: 10.1016/j.jhydrol.2017.11.046
Maru, Y. T. (2018). Summary: Critical reflection on and learning from Agricultural Innovation Systems (AIS) approaches and emerging Agricultural Research. for Development (AR4D) practice. Agric. Syst. 165, 354–356. doi: 10.1016/j.agsy.2018.07.012
Masia, S., Sušnik, J., Marras, S., Mereu, S., Spano, D., and Trabucco, A. (2018). Assessment of irrigated agriculture vulnerability under climate change in Southern Italy. Water 10, 209. doi: 10.3390/w10020209
Mateos, L., Villalobos, F. J., and Fereres, E. (2016). Irrigation Systems BT-Principles of Agronomy for Sustainable Agriculture. Cham: Springer International Publishing 255–267. doi: 10.1007/978-3-319-46116-8_19
Medda, S., Fadda, A., and Mulas, M. (2022). Influence of Climate change on metabolism and biological characteristics in perennial woody fruit crops in the mediterranean environment. Horticulturae 8, 273. doi: 10.3390/horticulturae8040273
Mekonnen, D. K., Spielman, D. J., Fonsah, E. G., and Dorfman, J. H. (2015). Innovation systems and technical efficiency in developing-country agriculture. Agric. Econ. 46, 689–702. doi: 10.1111/agec.12164
Mesa-Vázquez, E., Velasco-Muñoz, J. F., Aznar-Sánchez, J. A., and López-Felices, B. (2021). Three decades of behavioural economics in agriculture. An overview of global research. Sustainability 13, 10244. doi: 10.3390/su131810244
Migliore, G., Schifani, G., Romeo, P., Hashem, S., and Cembalo, L. (2015). Are farmers in alternative food networks social entrepreneurs? Evidence from a Behavioral Approach. J. Agric. Environ. Ethics 28, 885–902. doi: 10.1007/s10806-015-9562-y
Mirčetić, V., Ivanović, T., KneŽević, S., Arsić, V. B., Obradović, T., Karabašević, D., et al. (2022). The innovative human resource management framework: impact of green competencies on organisational performance. Sustainability 14, 2713. doi: 10.3390/su14052713
Mirzaei, O., Micheels, E. T., Boecker Student, A., and Professor, A. (2016). “Product and Marketing Innovation in Farm-Based Businesses: The Role of Entrepreneurial Orientation and Market Orientation,” in International Food and Agribusiness Management Review 19.
Montanaro, G., Xiloyannis, C., Nuzzo, V., and Dichio, B. (2017). Orchard management, soil organic carbon and ecosystem services in Mediterranean fruit tree crops. Scientia Hortic. 217, 92–101. doi: 10.1016/j.scienta.2017.01.012
Montes de Oca Munguia, O., Pannell, D. J., and Llewellyn, R. (2021). Understanding the adoption of innovations in agriculture: A review of selected conceptual models. Agronomy 11, 139. doi: 10.3390/agronomy11010139
Mozzato, D., Gatto, P., Defrancesco, E., Bortolini, L., Pirotti, F., Pisani, E., et al. (2018). The role of factors affecting the adoption of environmentally friendly farming practices: Can geographical context and time explain the differences emerging from literature? Sustainability 10, 3101. doi: 10.3390/su10093101
Müller, J., Acevedo-Duque, Á., Müller, S., Kalia, P., and Mehmood, K. (2021). Predictive sustainability model based on the theory of planned behavior incorporating ecological conscience and moral obligation. Sustainability 13, 4248. doi: 10.3390/su13084248
Müller, J. M., Buliga, O., and Voigt, K. I. (2018). Fortune favors the prepared: How SMEs approach business model innovations in Industry 4.0. Technol. Forec. Soc. Change 132, 2–17. doi: 10.1016/j.techfore.2017.12.019
Nguyen, T. P. L., Mula, L., Cortignani, R., Seddaiu, G., Dono, G., Virdis, S. G. P., et al. (2016). Perceptions of present and future climate change impacts on water availability for agricultural systems in the western mediterranean region. Water 8, 523. doi: 10.3390/w8110523
Niu, G., and Masabni, J. (2021). Roles of indoor vertical farming in sustainable production of horticultural crops. Acta Hortic. 1305, 365–373. doi: 10.17660/ActaHortic.2021.1305.48
Nsele, M. K., Fyama, J. N. M., Maréchal, K., and Dogot, T. (2022). Factors influencing the sustained adoption of innovative techniques by urban farmers in lubumbashi, democratic republic of congo. Agriculture 12, 1157.12081157 doi: 10.3390/agriculture12081157
Pandya, A. B., and Sharma, P. (2021). Climate Change and its Implications for Irrigation, Drainage and Flood Management. Irrig. Drainage 70, 976–978. doi: 10.1002/ird.2651
Pardo, J. J., Domínguez, A., Léllis, B. C., Montoya, F., Tarjuelo, J. M., and Martínez-Romero, A. (2022). Effect of the optimized regulated deficit irrigation methodology on quality, profitability and sustainability of barley in water scarce areas. Agric. Water Manage. 266. doi: 10.1016/j.agwat.2022.107573
Parra-López, C., Reina-Usuga, L., Carmona-Torres, C., Sayadi, S., and Klerkx, L. (2021). Digital transformation of the agrifood system: Quantifying the conditioning factors to inform policy planning in the olive sector. Land Use Policy 108, 105537. doi: 10.1016/j.landusepol.2021.105537
Pereira, L. S., Paredes, P., Melton, F., Johnson, L., Wang, T., López-Urrea, R., et al. (2020). Prediction of crop coefficients from fraction of ground cover and height. Background and validation using ground and remote sensing data. Agric. Water Manage. 241, 106197. doi: 10.1016/j.agwat.2020.106197
Pino, G., Toma, P., Rizzo, C., Miglietta, P. P., Peluso, A. M., and Guido, G. (2017). Determinants of farmers' intention to adopt water saving measures: Evidence from Italy. Sustainability 9(1). doi: 10.3390/su9010077
Pinto, T., Vilela, A., and Cosme, F. (2021). “Overview of the recent innovations in vitis products,” in Vitis Products: Composition, Health Benefits and Economic Valorization 131–178.
Ploll, U., Arato, M., Börner, J., and Hartmann, M. (2022). Sustainable Innovations: A Qualitative Study on Farmers' Perceptions Driving the Diffusion of Beneficial Soil Microbes in Germany and the UK. Sustainability 14, 5749. doi: 10.3390/su14105749
Quintero, S., Giraldo, D. P., and Garzon, W. O. (2022). Analysis of the Specialization Patterns of an Agricultural Innovation System: A Case Study on the Banana Production Chain (Colombia). Sustainability 14, 8550. doi: 10.3390/su14148550
Rabadán, A., González-Moreno, Á., and Sáez-Martínez, F. J. (2019). Improving firms' performance and sustainability: The case of eco-innovation in the agri-food industry. Sustainability 11, 5590. doi: 10.3390/su11205590
Rajapathirana, R. P. J., and Hui, Y. (2018). Relationship between innovation capability, innovation type, and firm performance. J. Innov. Knowl. 3, 44–55. doi: 10.1016/j.jik.2017.06.002
Rezaei, R., Mianaji, S., and Ganjloo, A. (2018). Factors affecting farmers' intention to engage in on-farm food safety practices in Iran: Extending the theory of planned behavior. J. Rural Stud. 60, 152–166. doi: 10.1016/j.jrurstud.2018.04.005
Rogers, E. M. (1995). Lessons for guidelines from the diffusion of innovations. Joint Commission J. Quality Improve. 21, 324–328. doi: 10.1016/S1070-3241(16)30155-9
Rose, D. C., and Chilvers, J. (2018). Agriculture 4.0: Broadening responsible innovation in an era of smart farming. Front. Sustain. Food Syst. 2, 87. doi: 10.3389/fsufs.2018.00087
Rouzaneh, D., Yazdanpanah, M., and Jahromi, A. B. (2021). Evaluating micro-irrigation system performance through assessment of farmers' satisfaction: implications for adoption, longevity, and water use efficiency. Agric. Water Manage. 246. doi: 10.1016/j.agwat.2020.106655
Saeed, F. H., Al-Khafaji, M. S., and Mahmood Al-Faraj, F. A. (2021). Sensitivity of irrigation water requirement to climate change in arid and semi-arid regions towards sustainable management of water resources. Sustainability 13, 13608. doi: 10.3390/su132413608
Saeedi, S. A. W., Juwaidah, S., and Kelly, W. K. S. (2022). Intention to adopt Industry 4.0 technologies among small and medium enterprises in the Malaysian dairy manufacturing industry. Food Res. 6, 209–218. doi: 10.26656/fr.2017.6(2).211
Sarkar, A., Wang, H., Rahman, A., Abdul Azim, J., Hussain Memon, W., and Qian, L. (2022). Structural equation model of young farmers' intention to adopt sustainable agriculture: a case study in Bangladesh. Renew. Agric. Food Syst. 37, 142–154. doi: 10.1017/S1742170521000429
Sarri, D., Lisci, R., Rimediotti, M., Vieri, M., and Storchi, P. (2015). “Applications of the precision viticulture techniques in the Chianti district,” in 1st Conference on Proximal Sensing Supporting Precision Agriculture - Held at Near Surface Geoscience 121–125. doi: 10.3997/2214-4609.201413851
Schaltegger, S., and Wagner, M. (2011). Sustainable entrepreneurship and sustainability innovation: Categories and interactions. Bus. Strat. Environ. 20, 222–237. doi: 10.1002/bse.682
Senger, I., Borges, J. A. R., and Machado, J. A. D. (2017). Using the theory of planned behavior to understand the intention of small farmers in diversifying their agricultural production. J. Rural Stud. 49, 32–40. doi: 10.1016/j.jrurstud.2016.10.006
Sgroi, F., Candela, M., di Trapani, A. M., Foder,à, M., Squatrito, R., Testa, R., et al. (2015). Economic and financial comparison between organic and conventional farming in Sicilian lemon orchards. Sustainability 7, 947–961. doi: 10.3390/su7010947
Shi, J., Wu, X., Zhang, M., Wang, X., Zuo, Q., Wu, X., et al. (2021). Numerically scheduling plant water deficit index-based smart irrigation to optimize crop yield and water use efficiency. Agric. Water Manage. 248, 106774. doi: 10.1016/j.agwat.2021.106774
Shi, J., Zhang, J., Xie, N., Yang, Z., and Luo, J. (2022). An agricultural supply chain coordination model: the case of trinity comprehensive cooperation organization in China. Sustainability 14, 8879. doi: 10.3390/su14148879
Silva, L. L., Barbosa, C., Fitas Da Cruz, V., Sousa, A., Silva, R., Lourenço, P., et al. (2022). How could precision irrigation based on daily trunk growth improve super high-density olive orchard irrigation efficiency? Agronomy. 12, 756. doi: 10.3390/agronomy12040756
Sivertsson, O., and Tell, J. (2015). Barriers to business model innovation in Swedish agriculture. Sustainability 7, 1957–1969. doi: 10.3390/su7021957
Small, B., and Maseyk, F. J. F. (2022). Understanding farmer behaviour: A psychological approach to encouraging pro-biodiversity actions on-farm. New Zealand J. Ecol. 46, 20. doi: 10.20417/nzjecol.46.20
Sok, J., Borges, J. R., Schmidt, P., and Ajzen, I. (2021). Farmer Behaviour as Reasoned Action: A Critical Review of Research with the Theory of Planned Behaviour. J. Agric. Econ. 72, 388–412. doi: 10.1111/1477-9552.12408
Soorani, F., and Ahmadvand, M. (2019). Determinants of consumers' food management behavior: Applying and extending the theory of planned behavior. Waste Manag. 98, 151–159. doi: 10.1016/j.wasman.2019.08.025
Souza, S. A., and Rodrigues, L. N. (2022). Increased profitability and energy savings potential with the use of precision irrigation. Agric. Water Manage. 270, 107730. doi: 10.1016/j.agwat.2022.107730
Spendrup, S., and Fernqvist, F. (2019). Innovation in agri-food systems – a systematic mapping of the literature. Int. J. Food Syst. Dyn. 10, 402–427.
Sulistyo, H., and Ayuni, S. (2020). Competitive advantages of SMEs: The roles of innovation capability, entrepreneurial orientation, and social capital. Contaduria y Admin. 65, 1983. doi: 10.22201/fca.24488410e.2020.1983
Takács-György, K., and Takács, I. (2022). Towards climate smart agriculture: How does innovation meet sustainability? Ecocycles 8, 61–72. doi: 10.19040/ecocycles.v8i1.220
Tama, R. A. Z., Ying, L., Yu, M., Hoque, M. M., Adnan, K. M., and Sarker, S. A. (2021). Assessing farmers' intention towards conservation agriculture by using the Extended Theory of Planned Behavior. J. Environ. Manage. 280. doi: 10.1016/j.jenvman.2020.111654
Testa, R., Foderà, M., di Trapani, A. M., Tudisca, S., and Sgroi, F. (2015). Choice between alternative investments in agriculture: The role of organic farming to avoid the abandonment of rural areas. Ecol. Eng. 83, 227–232. doi: 10.1016/j.ecoleng.2015.06.021
Tong, X., Wu, P., Liu, X., Zhang, L., Zhou, W., and Wang, Z. (2022). A global meta-analysis of fruit tree yield and water use efficiency under deficit irrigation. Agric. Water Manage. 260, 107321. doi: 10.1016/j.agwat.2021.107321
Tóth, J., Migliore, G., Balogh, J. M., and Rizzo, G. (2020). Exploring innovation adoption behavior for sustainable development: The case of Hungarian food sector. Agronomy 10, 612. doi: 10.3390/agronomy10040612
Turral, H., Svendsen, M., and Faures, J. M. (2010). Investing in irrigation: Reviewing the past and looking to the future. Agric. Water Manage. 97, 551–560. doi: 10.1016/j.agwat.2009.07.012
Ungureanu, N., Vlăduţ, V., and Voicu, G. (2020). Water scarcity and wastewater reuse in crop irrigation. Sustainability 12, 55. doi: 10.3390/su12219055
Unsworth, K., Sawang, S., Murray, J., Norman, P., and Sorbello, T. (2012). Understanding innovation adoption: Effects of orientation, pressure and control on adoption intentions. Int. J. Innov. Manage. 16, 3593. doi: 10.1142/S1363919611003593
van Dijk, W. F. A., Lokhorst, A. M., Berendse, F., and de Snoo, G. R. (2016). Factors underlying farmers' intentions to perform unsubsidised agri-environmental measures. Land Use Policy 59, 207–216. doi: 10.1016/j.landusepol.2016.09.003
van Gerrewey, T., Boon, N., and Geelen, D. (2022). Vertical farming: The only way is up? Agronomy 12, 2. doi: 10.3390/agronomy12010002
van Loo, E. J., Caputo, V., and Lusk, J. L. (2020). Consumer preferences for farm-raised meat, lab-grown meat, and plant-based meat alternatives: Does information or brand matter? Food Policy 95. 1931. doi: 10.1016/j.foodpol.2020.101931
Velasco-Muñoz, J. F., Aznar-Sánchez, J. A., Batlles-delaFuente, A., and Fidelibus, M. D. (2019). Sustainable irrigation in agriculture: An analysis of global research. Water 11, 1758. doi: 10.3390/w11091758
Wang, Y., Wang, Y., and Fan, P. (2022). Analysis of Micro Value Flows in the Value Chain of Eco-Innovation in Agricultural Products. Sustainability 14. 7971. doi: 10.3390/su14137971
Wauters, E., Bielders, C., Poesen, J., Govers, G., and Mathijs, E. (2010). Adoption of soil conservation practices in Belgium: An examination of the theory of planned behaviour in the agri-environmental domain. Land Use Policy 27, 86–94. doi: 10.1016/j.landusepol.2009.02.009
Wolf, S., Just, D., and Zilberman, D. (2001). Between data and decisions: the organization of agricultural economic information systems. Res. Policy 30, 121–141.
Yan, L., Zhao, X., Zhang, D., Deng, J., and Zhang, Y. (2022). Associated factors of pesticide packaging waste recycling behavior based on the theory of planned behavior in Chinese fruit farmers. Sustainability 14, 10937. doi: 10.3390/su141710937
Yildirim, T., Zhou, Y., Flynn, K. C., Gowda, P. H., Ma, S., and Moriasi, D. N. (2021). Evaluating the sensitivity of vegetation and water indices to monitor drought for three Mediterranean crops. Agron. J. 113, 123–134. doi: 10.1002/agj2.20475
Zagaria, C., Schulp, C. J. E., Zavalloni, M., Viaggi, D., and Verburg, P. H. (2021). Modelling transformational adaptation to climate change among crop farming systems in Romagna, Italy. Agric. Syst. 188. doi: 10.1016/j.agsy.2020.103024
Zaporozhchenko, V., Tkachuk, A., Tkachuk, T., and Dotsenko, V. (2022). The evaluation of irrigating meliorations efficiency after the change of climatic conditions. J. Water Land Develop. 52, 199–204. doi: 10.24425/jwld.2022.140390
Zhang, D., Xie, X., Wang, T., Wang, B., and Pei, S. (2022). Research on water resources allocation system based on rational utilization of brackish water. Water 14, 948. doi: 10.3390/w14060948
Zhang, K. (2018). Theory of planned behavior: Origins, development and future direction. Int. J. Human. Soc. Sci. Invent. 7, 76–83.
Zhang, Q., Xiao, H., Duan, M., Zhang, X., and Yu, Z. (2015). Farmers' attitudes towards the introduction of agri-environmental measures in agricultural infrastructure projects in China: Evidence from Beijing and Changsha. Land Use Policy 49, 92–103. doi: 10.1016/j.landusepol.2015.07.021
Keywords: TPB, farm, economic, chain, water, climate change
Citation: Timpanaro G, Pecorino B, Chinnici G, Bellia C, Cammarata M, Cascone G and Scuderi A (2023) Exploring innovation adoption behavior for sustainable development of Mediterranean tree crops. Front. Sustain. Food Syst. 7:1092942. doi: 10.3389/fsufs.2023.1092942
Received: 08 November 2022; Accepted: 06 February 2023;
Published: 23 February 2023.
Edited by:
Rachel Bezner Kerr, Cornell University, United StatesReviewed by:
Alfio Strano, Mediterranea University of Reggio Calabria, ItalyRaffaella Pergamo, Council for Agricultural and Economics Research (CREA), Italy
Copyright © 2023 Timpanaro, Pecorino, Chinnici, Bellia, Cammarata, Cascone and Scuderi. This is an open-access article distributed under the terms of the Creative Commons Attribution License (CC BY). The use, distribution or reproduction in other forums is permitted, provided the original author(s) and the copyright owner(s) are credited and that the original publication in this journal is cited, in accordance with accepted academic practice. No use, distribution or reproduction is permitted which does not comply with these terms.
*Correspondence: Alessandro Scuderi, YWxlc3NhbmRyby5zY3VkZXJpQHVuaWN0Lml0