- 1Business School, Shaoguan University, Shaoguan, Guangdong, China
- 2North Guangdong Rural Revitalization Research Center, Shaoguan University, Shaoguan, Guangdong, China
- 3School of Tourism and Cultural Industry, Research Institute of Rural Revitalization, Hunan University of Science and Engineering Yongzhou, Hunan, China
Introduction: Enhancing agricultural productivity and promoting sustainable development in China are critical priorities, given their significant contribution to food security and rural development. However, achieving consistent agricultural productivity growth across regions and sectors has been challenging, leading to inefficiencies and environmental pressures. To address this, the potential of digital inclusive finance (DIF) as a catalyst for progress has been explored.
Methods: This study investigates the impact of DIF on agricultural total factor productivity (ATFP) through farmland transfer, with implications for rural revitalization and high-quality agricultural development. Using the HMB index method, we calculate and decompose China’s inter-provincial ATFP from 2011 to 2020. Employing the dynamic panel fixed effect model and SYS-GMM method, we empirically test the influence of DIF on agricultural productivity in relation to farmland transfer.
Results and discussion: Our findings reveal a slow upward trend in China’s ATFP, driven primarily by scale efficiency. Notably, a 1% increase in the general index of DIF correlates with a 0.136% increase in ATFP. We analyze the sub-classification indexes of digital inclusive finance, namely coverage breadth, depth of use, and digitalization degree. Their contributions to agricultural productivity growth are significant, with coverage breadth having the greatest impact, followed by depth of use and digitalization degree. Farmland transfer acts as a crucial intermediary, enhancing agricultural allocation efficiency and driving the relationship between digital finance and total factor productivity growth. Interestingly, the western region exhibits the highest growth rates, followed by the eastern region, while the central region shows no significant effects.
Conclusion: To comprehensively enhance ATFP, aligning supply and demand for digital financial services, leveraging service depth, and guiding farmland transfer according to local conditions are essential. These findings offer valuable insights into effective strategies for maximizing the potential of DIF, promoting rural development, and achieving sustainable agricultural growth.
1 Introduction
The fragmentation of land ownership has resulted in small and scattered land holdings, which in turn limits opportunities for economies of scale and mechanization (Janus et al., 2023; Muchová and Raškovič, 2020; Wang et al., 2020; Lu et al., 2019; Manjunatha et al., 2013). This fragmentation poses a multitude of hurdles such as reduces yields through changes in marginal outputs of agricultural inputs (Lu et al., 2018), reduce technical efficiency (Tan et al., 2010) and discouraging farmers from adoption of agricultural innovations (Niroula and Thapa, 2005) that impede the path to productivity growth for smallholders (Wang et al., 2023). In countries like China, this issue has become particularly pressing, as it has significant implications for agricultural productivity (Wang, 2023). Smallholder farmers face challenges in accessing resources and adopting modern farming techniques due to this fragmentation (Tan et al., 2010). However, with the emergence of digital technologies, there is a growing interest in exploring how digital platforms and financial services can help facilitate land transactions and unlock the untapped potential for increased productivity among smallholders (Zhou et al., 2023; Jiang et al., 2020).
Rural digital inclusive finance (DIF) in China is essential for promoting rural development, achieving high-quality agricultural growth, and ensuring financial inclusion (Yang B. et al., 2022; Qin et al., 2023; Xiong et al., 2022). With a vast rural population and agriculture playing a vital role in the national economy, the availability of affordable financial services is crucial to support rural livelihoods and enhance productivity in the agricultural sector (Lan et al., 2023). Digital inclusive finance, such as mobile banking, online lending, and e-payment systems, can bridge the gap in financial services in rural areas and provide accessible and affordable financial solutions to farmers and rural households (Yu et al., 2022; Wang, 2023). By promoting financial inclusion and supporting rural economic growth, rural DIF contributes to China’s overall development goals, including balanced regional development, poverty reduction, and sustainable economic growth.
Farmland transfer has long been recognized as an effective way to promote the growth of agricultural total factor productivity (ATFP) (Kuang et al., 2022; Fei et al., 2021; Duan et al., 2021). Despite this, recent data from China’s Rural Policy and Reform annual statistical report shows that by the end of 2021, 85.22% of China’s farmers had a land operation scale of <10 mu, equating to 232 million farmers.1 As such, the goal of de-agricultural involution through farmland transfer has yet to be reached, and the process of farmland transfer itself is facing a dilemma of involution. There are three primary reasons for this: (1) the growth rate of farmland transfer has decreased (Rogers et al., 2021); (2) farmland mostly has been transferred to small-scale farmers (Gao et al., 2020), and (3) the effect of farmland transfer on agricultural production efficiency is diminishing (Kuang and Lu, 2018). Adamopoulos and Restuccia (2014) have suggested that small land size and low levels of agricultural financial investment are the main reasons for low agricultural productivity in developing countries. In China, rural labor and land have been continually adjusted and combined under government and market guidance in response to urbanization and industrialization (Gao et al., 2020; Huang et al., 2023). However, the financial exclusion of traditional financial capital still makes it difficult and costly to obtain financing and causes other problems in agricultural production (Li and Han, 2014). The shortage and unreasonable distribution of rural financial capital led to a lack of motivation for farmers to transfer land and adopt technology, which hinders the growth of ATFP (Zhang, 2022). Hence, there is a need to ensure adequate and fair distribution of rural financial capital to support sustainable and efficient agricultural production.
Unlocking the power of DIF in driving agricultural growth is paramount for a multitude of reasons. However, the challenge lies in bridging the gap between rural areas and accessible financial services. By delving into the role of digital inclusive finance, we can unearth ground-breaking solutions to provide farmers and rural households with affordable and easily accessible financial resources. This transformative force has the potential to revolutionize the entire agricultural sector, offering tailor-made financial solutions, streamlining transactions, and fostering financial inclusion (Liu and Ren, 2023). To truly grasp the magnitude of its impact on agricultural growth, it is essential to unravel the intricate relationship between DIF and farmland transfer. Such understanding will enable us to harness its full potential, design effective policies, and realize its myriad benefits (Shen et al., 2023). As current literature on this subject remains limited, further research becomes imperative to bridge this knowledge gap and contribute to evidence-based policy-making that propels agricultural growth through the transformative power of digital inclusive finance.
The existing research gaps provide motivation for us to estimate the impact of DIF on ATFP through farmland transfer. This paper aims to potentially address the following research questions: (1) What is the direct impact of DIF on the growth of ATFP? (2) To what extent does DIF promote the improvement of farmland transfer? (3) How does farmland transfer mediate the relationship between DIF and ATFP? (4) What are the factors that affect the effectiveness of DIF in promoting the improvement of farmland transfer and ATFP? (5) How do the findings of this study contribute to our understanding of the role of DIF in promoting sustainable agricultural development?
This study will contribute to a better understanding of the role of DIF in enhancing ATFP and the underlying mechanisms by which it operates. Additionally, it will shed light on the factors that influence the effectiveness of DIF in promoting sustainable agricultural development, which can inform policy and practice aimed at achieving this goal. This study offers several significant contributions. Firstly, it fills existing research gaps by estimating the impact of DIF on ATFP through farmland transfer. Secondly, it investigates how DIF contributes to the improvement of farmland transfer, providing insights into the mechanisms at play. Thirdly, it examines the mediating role of farmland transfer in the relationship between DIF and ATFP. Fourthly, it identifies factors that influence the effectiveness of DIF in enhancing both farmland transfer and ATFP. Finally, it offers valuable insights into the role of DIF in promoting sustainable agricultural development, which can inform relevant policies and practices in this field. The empirical evidence and insights provided in this paper have practical implications for achieving sustainable agricultural development and improving the livelihoods of smallholder farmers.
1.1 The development of digital financial inclusion in China
The development of digital financial inclusion in China has been propelled by the rapid advancement of digital technologies, including mobile Internet, network communication, and cloud computing (Liu et al., 2021a). Digital inclusive finance, as a modern factor of production, possesses the key characteristics of information symmetry and the inclusive advantages of equal opportunities. In China, it has experienced significant growth, as evident from the average general DIF index, which increased from 40.0 in 2011 to 341.2 in 2020, with an average annual growth rate of 31.75%. The three sub-classification indexes: coverage breadth, depth of use, and digitalization degree have consistently shown positive growth rates of 32.26, 30.28, and 36.46%, respectively (Figure 1).
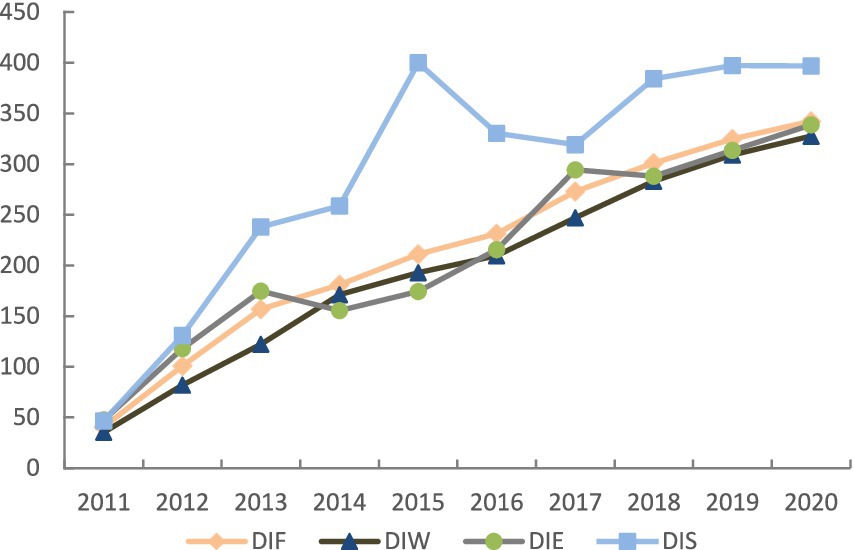
Figure 1. The DIF general index and the three sub-classification indexes from 2011 to 2020. Source: The DIF indexes (2011–2020) come from the data jointly prepared by Peking University Digital Financial Inclusion Research Centre and Ant Financial Services Group.
DIF combines the principles of inclusiveness, rapidity, diffusion, and liquidity to facilitate the widespread access to financial services (Yue et al., 2022). This allows marginalized groups who were traditionally excluded from formal financial systems to have the opportunity to enjoy these services. It effectively addresses the limitations of traditional finance and achieves the dual goals of efficiency and fairness.
The continuous development of rural Internet infrastructure has laid a strong foundation for further enhancing DIF in rural areas (Leng, 2022). In 2022, the coverage rate of 4G signals in rural China exceeded 98%, and the number of rural internet users reached 293 million, accounting for 27.9% of the total internet users. Recognizing the strategic significance of digital inclusive finance, the Chinese government has prioritized its development through policy initiatives. In 2021, the development of rural DIF was included for the first time in the No. 1 central document. The subsequent No. 1 central document in 2022 emphasized the vigorous promotion of digital villages and strengthening the revitalization of financial services in rural areas. Furthermore, the No. 1 central document in 2023 proposed the in-depth implementation of digital commerce and agriculture.
Driven by national policies, DIF will continue to expand its presence in rural areas, embedding itself deeply in various aspects of agricultural production, logistics, and consumption of agricultural products. In recent years, DIF has demonstrated its potential in improving rural financial supply and optimizing distribution, providing innovative ideas for farmers to enhance their behavior related to rural land transfer (Zhang, 2022). It plays a crucial role in facilitating the overall efficiency of production, circulation, and consumption processes.
2 Literature review
2.1 Literature on the agricultural impact of digital financial inclusion
In the context of agriculture, the literature on digital financial inclusion is extensive and mostly concerned with its to enhance productivity and sustainability through improving financial access for farmers.
DIF has emerged as a powerful tool for promoting financial inclusion and driving economic growth in the agriculture sector (Sun and Tang, 2022; Adegbite and Machethe, 2020). Studies have demonstrated that DIF can improve access to credit and capital for farmers, especially smallholder farmers who often face challenges in obtaining traditional financial services (Liu et al., 2021b). Through mobile banking and digital lending platforms, farmers can access affordable loans, manage cash flows, and invest in agricultural inputs, equipment, and infrastructure. Digital payment systems have the potential to significantly improve transparency and efficiency in agricultural value chains (Kos and Kloppenburg, 2019). With digital inclusive finance, farmers can receive payments directly to their mobile wallets, reducing the reliance on cash transactions and mitigating the risk of theft or loss. Furthermore, digital payments enable faster and more accurate record-keeping, facilitating traceability and accountability throughout the value chain (Yang et al., 2021). DIF can also enhance farmers’ ability to manage risks associated with agricultural production (Liu et al., 2023). Crop insurance and weather index-based insurance products can be delivered digitally, providing farmers with a safety net against yield losses due to adverse weather events or other unforeseen circumstances (An et al., 2023). By managing risks effectively, farmers can make more informed decisions and allocate resources efficiently, ultimately improving agricultural productivity.
In recent years, numerous studies have explored the impact of DIF on various aspects of agriculture. Zhou and Miao (2023) investigated its effects on agricultural investment, while Chen and Wen (2023) explored its influence on rural industrial development. Yang Y. et al. (2022) examined its impact on the income gap between urban and rural residents, and Jin (2022) studied its role in rural revitalization. Xiang (2022) investigated its impact on the consumption patterns of rural residents, while Zhang (2021) explored its relationship with agricultural industry upgrading. Wang and Ran (2022) analysed its effects on relative poverty in rural areas, and Song and Yang (2022) investigated its impact on farmers’ entrepreneurship. Notably, Zhang (2022) research specifically examined the impact of DIF on land transfer, highlighting how it can lower entry barriers for farmers, enhance financial access, and create favorable conditions for farmland transfer marketization.
Despite the abundance of research in this area, few studies have explored the impact of DIF on agricultural productivity. Theoretical work by Romer (1986) suggests that financial support can enhance productivity by optimizing factor allocation. Empirical studies in developing countries have shown that agricultural financial investment can improve productivity (Adu-Baour et al., 2019). Sun et al. (2022) found that DIF increases the supply of rural finance, promoting green agricultural production and enhancing farmers’ efficiency. More recently, scholars have proposed that DIF provides inclusive credit support for agriculture, creating conditions for sustainable and green agricultural development and boosting productivity (Zhang and Tian, 2023). Overall, while much research has explored the impact of DIF on various aspects of agriculture, more empirical work is needed to fully understand its potential impact on ATFP, particularly through the channel of farmland transfer.
In addition to the factors of capital deepening, industrial agglomeration, factors’ liquidity, and technology diffusion previously discussed, several other channels significantly influence the impact of DIF on agricultural productivity. Agricultural productive services play a crucial role in enhancing efficiency and output through better resource management and support services (Zhu et al., 2022). Furthermore, green production behavior is increasingly important as it aligns with sustainable agricultural practices, promoting both productivity and environmental stewardship (Hunjra et al., 2024). The use of external inputs, such as fertilizers and advanced seeds, can also amplify productivity when combined with digital financial tools. Human capital, including farmers’ education and skills, is essential for effectively leveraging digital finance to improve agricultural practices. Integrating these factors into our analysis, we aim to provide a more holistic understanding of the mechanisms at play.
Empirical research on this topic has yielded mixed results. Matthews (2019) pointed out that due to various factors such as rural interest space, financial infrastructure, financial regulation, farmers’ digital literacy, and financial risks, the development of DIF in rural areas has significant limitations and is difficult to bring about sustained improvements in agricultural productivity. In contrast, Hu et al. (2021) found that the development of DIF has effectively improved regional ATFP. Zheng and Li (2022), based on county-level data from China, indicated that DIF has a significant positive impact on county ATFP growth, with this impact increasing at higher quantiles. However, few studies have explored the impact mechanism of DIF on ATFP in depth. Some recent literature has started to examine this issue from the perspectives of capital deepening (Ren and Lei, 2022), industrial agglomeration (Zeng and Zhang, 2022), and factor flow and technology diffusion (Tang et al., 2022), which further enriches our understanding of this important topic.
2.2 Literature on agricultural total factor productivity
Research on ATFP has primarily focused on two key aspects. Firstly, scholars have concentrated on the measurement and analysis of ATFP, utilizing different methodologies. Parameter-based stochastic frontier analysis (SFA) has been widely adopted for this purpose (Moreira and Bravo-Ureta, 2016; Tang et al., 2022). Another approach employed is the non-parametric-based DEA-Malmquist method (Adamonpoulos et al., 2019; Shi et al., 2016). Secondly, researchers have explored various factors that influence ATFP. These factors include agricultural policy (Duquenne et al., 2019), the digital economy (Sun et al., 2023), foreign investment (Wang et al., 2019), agricultural mechanization (Xue et al., 2020), rural education (Yu et al., 2020), and other relevant variables. Understanding these factors and their impact on ATFP is crucial for enhancing agricultural efficiency and sustainability.
The existing literature offers a strong theoretical foundation for this study. However, there are several shortcomings that need to be addressed. Firstly, the research perspective needs to be broadened. The current theoretical research indicates that a shortage and an unreasonable distribution of rural financial capital can result in insufficient motivation for farmers to transfer land or adopt technology, leading to a hindrance to the growth of ATFP (Zhang, 2022). On the other hand, farmland transfer has been shown to promote the improvement of ATFP and has garnered significant research support (Kuang and Yang, 2019; Adamopoulos and Restuccia, 2014). Therefore, DIF is expected to have a positive impact on ATFP by promoting farmland transfer. However, relevant studies are yet to clarify and test the mechanism of DIF on ATFP from the perspective of farmland transfer.
Research on the impact of DIF on ATFPATFP is still relatively scarce, and empirical research results have been controversial. In particular, the impact of DIF on ATFP and its decomposition efficiency has not been thoroughly investigated from theoretical and empirical levels. Research methods require improvement. While some studies have attempted to mitigate the impact of inherent drawbacks in measurement methods, such as input and output slack, radial and angular issues, the SFA method itself has subjective flaws in function setting and is limited by error distribution assumptions. In contrast, the DEA-Malmquist method is arbitrary in defining functions from input or output perspectives, resulting in incompatibility of measurement results. Additionally, these two methods cannot decompose scale efficiency and allocation efficiency from ATFP, which makes it difficult to explain the composition of total factor productivity reasonably and concretely. Therefore, it is difficult to analyze the mechanism of DIF on ATFP accurately.
3 Theoretical analysis and research hypothesis
3.1 Direct impact of DIF on the ATFP growth
The growth of ATFP can be directly influenced by DIF through various effects (as depicted in Figure 2). The technology substitution effect plays a significant role. Based on induction technology theory, the price mechanism encourages the gradual replacement of expensive and scarce agricultural input elements with cheaper and more abundant ones (Nakano and Magezi, 2020). With digital inclusive finance, financial constraints on farmers’ productive consumption can be alleviated, creating favourable capital conditions for agricultural technology to substitute labor. In the context of non-agricultural transfer of rural labor force and declining relative prices of agricultural capital and rural labor force, DIF continuously induces technological progress, replacing rural surplus labor force with capital intensification.
The factor allocation effect also contributes to the impact. Traditional finance procedures involving credit information acquisition and review, mortgage guarantees, etc., are relatively complex, accompanied by high loan risks and limited coverage of financial services. DIF efficiently guides idle funds from society and utilizes big data analysis of borrowers’ credit data to assess repayment risks and borrowers’ economic situations. By reducing the financing threshold and farmland transaction costs, it enhances the availability of funds for small farmers, optimizes agricultural investment, and improves resource allocation efficiency (Ren and Lei, 2022).
The technology diffusion effect is noteworthy. DIF benefits from the Internet network effect, enabling farmers to conduct financial asset transactions online. Moreover, it promotes technology learning and information dissemination among farmers through digital platforms, facilitating improvements in agricultural technology efficiency (Tang et al., 2022).
Lastly, the division of labor and scale effect is significant. DIF functions as part of agricultural socialization services. With the development of digitalization and e-commerce, it stimulates new demands from farmers, expands agricultural production and service models, and further deepens the socialized division of labor. Financial digital technology facilitates the integration and agglomeration of the agricultural supply chain, resulting in internal and external scale benefits that enhance the scale efficiency of agriculture. Therefore, this paper proposes the following hypothesis:
H1: DIF significantly promotes the growth of ATFP.
3.2 Indirect impact
3.2.1 Indirect impact through improving the willingness to farmland transfer-out
DIF has created an information and capital bridge for the conclusion of the contract between farmland transfer and transfer. It is a type of social capital that enables the integration of financial social capital and financial supply–demand information under big data, thereby alleviating the information asymmetry of traditional financial capital in the matching of supply and demand. This results in a reduction of transaction costs associated with land transfer. Additionally, DIF has an industry-driven external effect that improves the digital inclusive financial literacy of rural workers and enhances the competitiveness of non-agricultural employment overall. This increases the feasibility of farmers to engage in non-agricultural production and entrepreneurship, which leads to an increase in their willingness to transfer land out of agricultural production (Zhang, 2022).
The digital technology platform of DIF accelerates the process of rural labor transfer and promotes the market-oriented transfer of land, improving the combined productivity of the three core agricultural input factors of finance, land, and labor. This drives agricultural mechanization and helps to improve the allocation and scale efficiency of agricultural elements (Huang et al., 2014; Zhang, 2022). As such, DIF indirectly impacts the growth of ATFP through its facilitation of improved willingness to transfer land out of agricultural production. DIF provides a range of benefits for farmers, including improved access to financial services, more efficient allocation of farmland resources, better risk management, and increased opportunities for agricultural specialization and innovation. By facilitating the transfer of farmland to those who can utilize it most efficiently, DIF plays an important role in driving the growth of ATFP.
3.2.2 Indirect impact through expanding the scale of farmland transfer-in
One of the key challenges in China’s farmland transfer is the insufficient financial capital available to farmers for improving production conditions, introducing technology, and purchasing production materials. Farmers often struggle to break through the scale of farmland transfer due to limited capital investment and traditional bank loans. However, digital inclusive finance, leveraging its digital characteristics and inclusive advantages, addresses these issues by providing flexible, diverse, and innovative financial services for farmers looking to transfer land. This, in turn, increases farmers’ willingness to transfer their land.
The innovative financing models of agricultural finance big data offered by DIF are efficient and convenient. They help farmers overcome the barriers of free rent in farmland transfer and geographical restrictions limited to the village group. These models are particularly beneficial for fulfilling the financial capital needs of different entities, such as family farms, large-scale transfer households, and new agricultural entities. As a result, the scale of farmers’ land transfer can be expanded.
By promoting digital financial inclusion, the concentration of farmland to large-scale farmers with high operating efficiency is facilitated through professional division of labor. This concentration brings both internal and external scale benefits to agricultural production and contributes to the growth of agricultural scale efficiency (Qu et al., 2019). DIF plays a crucial role in expanding the scale of farmland transfer by addressing the financial capital constraints faced by farmers. Providing inclusive and innovative financial services, it enhances farmers’ willingness to transfer land and enables them to meet their financial needs throughout the transfer process. This reconfiguration of farmland ownership contributes to the growth of agricultural scale efficiency.
3.2.3 Indirect impact through expanding the farmland transfer mode
DIF has a significant spillover effect on new types of agricultural business entities. It not only effectively enhances the financial literacy of these entities but also provides the necessary human capital conditions for innovating the farmland transfer mode. The diverse range of products and tools offered by digital financial inclusion can provide the required financial capital conditions for fostering innovation in the farmland transfer mode of new agricultural entities (Chen and Luo, 2022).
Expanding the farmland transfer mode contributes to the transformation of green agricultural technology. Emerging models of farmland transfer, such as joint-stock cooperation and land trust, prioritize factors such as production scale, cooperative organization, and information sharing. These models facilitate the introduction of advanced elements like green biology and mechanical technology into agricultural production. The combination of “finance + land” brings advantages such as capital savings and increased efficiency, thereby promoting the deepening of agricultural capital and driving technological progress towards capital density and sustainable development in agriculture. DIF plays a crucial role in enabling and supporting the innovation of farmland transfer modes for new agricultural entities. Improving financial literacy and offering diverse financial products, it creates an environment conducive to the adoption of new and more efficient farmland transfer models. These innovative models, focusing on cooperation and information sharing, facilitate the integration of advanced technologies and practices in agriculture, leading to sustainable and green agricultural development.
3.2.4 Indirect impact through improving the farmland transfer production condition
The expansion of farmland transfer and the enhancement of agricultural production efficiency are built upon key factors such as the effective irrigation proportion in agriculture, farmland rectification, and infrastructure investment. Improving agricultural production conditions primarily relies on government investment and the extensive involvement of social capital in agricultural investments. DIF represents a decentralized form of social capital that effectively addresses the limitations associated with traditional financial capital and government investment. It plays a significant role in improving the production conditions of farmland transfer, reducing the dependence of agricultural production on natural conditions, enhancing the resilience of agricultural production against risks, and enabling the possibility of scaling up farmland operations. This, in turn, fosters the synergistic utilization of various factors, thereby maximizing productivity and improving agricultural production efficiency (Shi et al., 2020).
DIF serves as a vital catalyst in bridging gaps in agricultural financing by providing accessible and flexible financial services. It allows farmers to access the necessary funds for improving irrigation systems, implementing farmland rectification measures, and investing in infrastructure development. By alleviating financial constraints, DIF contributes to creating favourable production conditions for farmland transfer. Furthermore, it reduces the reliance of agricultural production on natural conditions, such as water availability, thus enhancing the stability and resilience of agricultural activities. Moreover, the expansion of farmland operations facilitated by DIF creates opportunities for economies of scale, enabling farmers to optimize resource allocation and drive improvements in productivity. By leveraging digital technology and financial resources, farmers can invest in modern agricultural techniques, machinery, and other inputs that enhance production efficiency and output.
Based on the theoretical framework presented above, this study employs farmland transfer as an intermediary variable and constructs a theoretical model to explore the nexus between digital inclusive finance, farmland transfer and ATFP (see Figure 3). The study empirically examines the direct impact of DIF on the growth of ATFP, as well as the transmission variable through farmland transfer. Two hypotheses related to the indirect impact of DIF on ATFP are proposed:
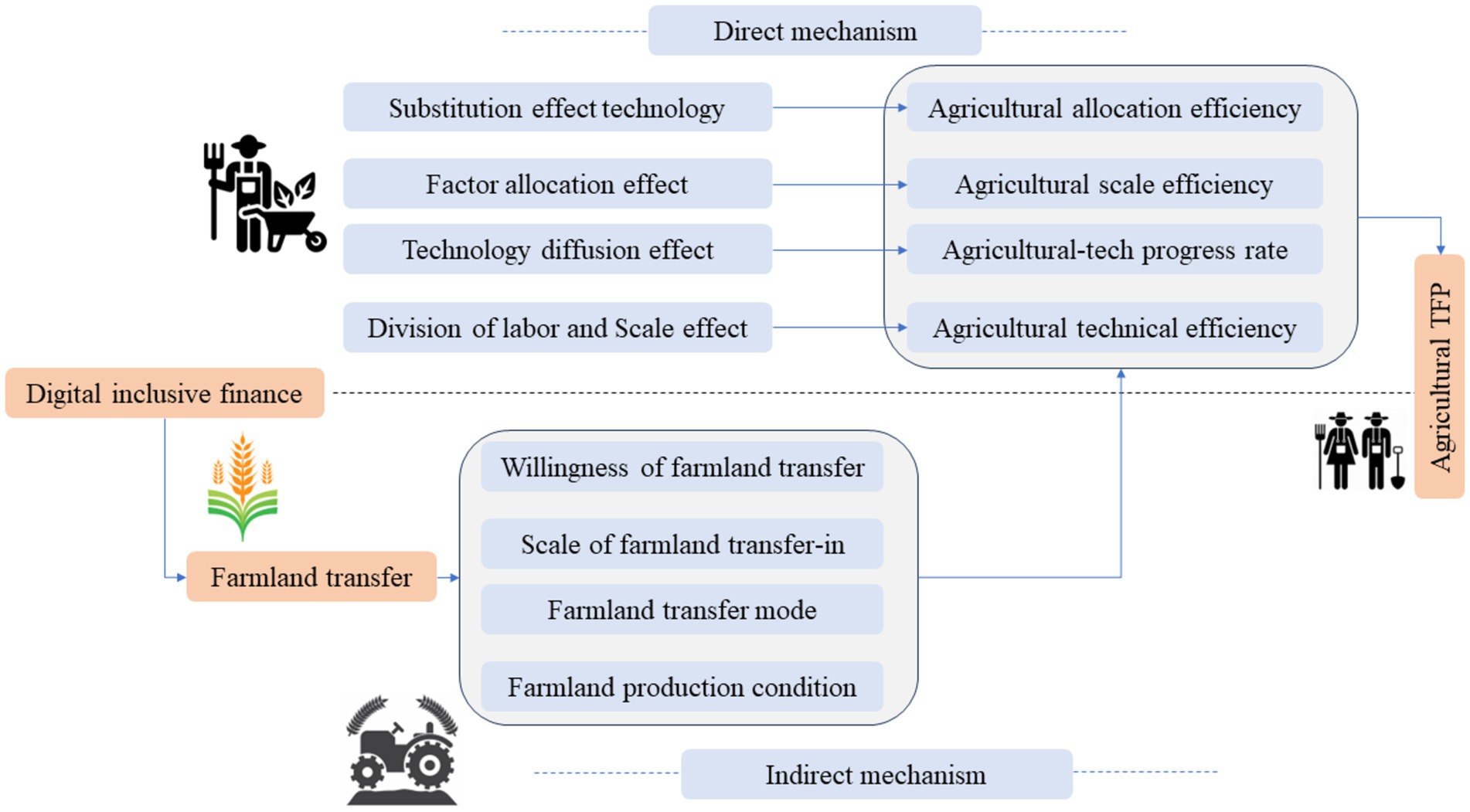
Figure 3. Displays the theoretical model that examines the relationship between DIF, farmland transfer, and ATFP.
H2: DIF promotes improvements in farmland transfer.
H3: DIF has a significant positive impact on the growth of ATFP through farmland transfer.
This study investigates the extent to which DIF positively impacts the improvement of farmland transfer, as indicated in hypothesis 2. It also explores the indirect impact of DIF on the growth of ATFP through farmland transfer, as proposed in hypothesis 3. Through testing hypotheses 2 and 3, the study aims to empirically establish the linkages between digital inclusive finance, farmland transfer, and ATFP.
4 Data and methodology
4.1 HMB index
The Hicks-Moorsteen-Bjurek (HMB) productivity index is a valuable economic tool for evaluating production efficiency and productivity (Kerstens and Van de Woestyne, 2014). It extends the traditional total factor productivity (TFP) index by considering multiple inputs and outputs. With contributors John R. Hicks, Harry Moorsteen, and Johan Bjurek, this index has gained wide adoption in economics, particularly in productivity analysis and efficiency measurement. It compares observed inputs and outputs against a theoretical production frontier, factoring in both quantity and quality. Researchers and policymakers can use the HMB index to assess efficiency and identify areas for improvement. Its comprehensive evaluation enables a more accurate assessment of overall productivity. Understanding its relationship with the Malmquist productivity index is crucial. The Malmquist index analyzes productivity changes over time, while the HMB index incorporates additional factors and dimensions to provide a more detailed analysis. The HMB index considers changes in technology, technical efficiency, and the quality and quantity of inputs and outputs. In practical terms, the measurement of the HMB index involves three main steps, facilitating a systematic assessment of productivity dynamics. The subsequent three steps of the HMB index measurement ensure a structured approach to evaluating productivity trends.
4.1.1 Defining the input–output distance function
The Malmquist productivity index denotes the input vector by the m-dimensional vector X (representing the input type) and the output vector by the k-dimensional vector Y (representing the output type).
The output distance function, denoted as Do(X, Y), is defined on P(X).
By definition, if Do(X, Y) > 1, it indicates that Y cannot be produced with the existing inputs X. If Do(X, Y) ≤ 1, it measures technical efficiency. Lastly, when Do(X, Y) = 1, it indicates that the technology is fully efficient.
Referring to the definition of the output distance function, the input distance function can be expressed as follows:
If D1(X, Y) < 1, it indicates that Y cannot be produced with the available input X. On the other hand, if D1(X, Y) = 1, it indicates that the technology is fully efficient. To solve the distance functions expressed in Equations 1, 2 using mathematical programming:
In this context, Y represents the output matrix in N × M dimensions for all N provinces, while x represents the input matrix in N × K dimensions for all N provinces. λ is the weight vector in N × 1 dimensions, and α and θ are scalars.
4.1.2 Output and input-oriented Malmquist index calculation
Firstly, it utilizes the Malmquist index from the output perspective, which measures changes in productivity based on output indicators (Caves et al., 1982).
Next, the Malmquist index from the input perspective is introduced and incorporated into the model.
In Equation 3, the is the Malmquist index in terms of output from period s to period t. In Equation 4 is the Malmquist index in terms of inputs from period s to period t.
4.1.3 HMB index calculation
The choice of which Malmquist index to use depends on the controllability of the inputs and outputs being analyzed. If the inputs are not controllable, the Malmquist index from the output perspective should be utilized. Conversely, if the outputs are not controllable, the Malmquist index from the input perspective should be employed. However, when both inputs and outputs are controllable, the selection between the Malmquist indices proposed by Färe et al. (1995) is arbitrary. To address this issue, Bjurek (1996) introduced the HMB index, named after Hicks, Moorsteen, and Bjurek. This index was derived from the research of Hicks and Moorsteen, providing a comprehensive approach that combines and extends the previous Malmquist indices Equation 5.
4.1.4 HMB index decomposition
The HMB index method is a non-parametric method that does not require setting a production function and overcomes the problem of arbitrariness in the choice of Malmquist index from the input perspective or output perspective, and also decomposes scale efficiency and allocation efficiency from ATFP. Therefore, the HMB index model is chosen to measure and decompose ATFP. The HMB index can be decomposed as follows (Equations 6–8).
Where , , and signify the respective rate of change of agricultural technological progress (TC), technical efficiency (EC), allocation (ME), and scale efficiency (SC), respectively. Ss, t and rs, t in the Malmquist index indicating the radial direction of agricultural inputs and outputs, respectively. In the efficiency decomposition formula, a value >1 indicates that efficiency positively contributes to the growth of ATFP, while a value <1 suggests that efficiency has a negative impact on ATFP growth. By examining the four measurement values of HMB during the sample period, we can infer the driving factors influencing ATFP.
4.2 SYS-GMM model
This paper assesses the impact of DIF on ATFP using a dynamic panel fixed-effects model in the context of farmland transfer. This approach was chosen for several reasons. Firstly, the HMB decomposition index is a change index, which means that its value is influenced by previous years’ productivity. Using a dynamic panel model is more consistent with this variable characteristic. Secondly, the omission of relevant variables, two-way causality between the explanatory variable and ATFP, and endogeneity caused by measurement errors could all lead to biased estimated parameters. To overcome these issues, this paper incorporates various control variables and employs a dynamic panel fixed-effects model to mitigate the problem of biased results. Finally, the study uses short panel data from 30 provinces over 10 years (2011–2020), and the dynamic panel model enhances the validity of estimations.
In this study, we employed the System Generalized Method of Moments (SYS-GMM) to estimate the model parameters. SYS-GMM combines the difference equation with the level equation. To ensure the validity of the model, we conducted the Arellano-Bond test to assess if the residual term exhibited first-order autocorrelation without second-order autocorrelation. To address potential over-identification, we conducted the Sargan test, which served as a constraint test for the instrumental variables in the model. This step further validated the reliability of our findings (Alvarez and Arellano, 2022). Given the robustness and endogeneity concerns, we selected internet penetration at the provincial level as the instrumental variable for the regression analysis. The intermediate effects model was established based on the principles outlined by Baron and Kenny (1986).
Equations 1a, 3a both calculate the HMB index for ATFP, while Equation 2a calculates farmland transfer.
Where are the explanatory variables ATFP and its decomposition index. denotes the explanatory variable DIF index. denotes the mediating variable farmland transfer. for other control variables, for individual fixed effects, for time fixed effects, is the random perturbation term.
The model testing involved three steps. First, we tested the significance of the coefficient for the DIFindex in Equation 1a to determine if it had a significant effect on ATFP growth. If was insignificant, then the mediating effect test would be terminated. However, if was significant, we proceeded with the mediating effect test.
Second, we tested the significance of the coefficients for the DIFindex in Equation 2a and the coefficient for farmland transfer rate in Equation 3a . If both and were significant, we concluded that the mediating effect exists, and we moved on to the next step. However, if at least one of and was insignificant, we conducted the Sobel test to determine if the original hypothesis H0: =0 was valid. If the hypothesis was rejected, we proceeded with the subsequent test as if both and were significant under the stepwise test method. If the original hypothesis could not be rejected, it indicated that farmland transfer did not play a mediating effect in the DIFaffecting ATFP growth.
Third, we tested the significance of the coefficient of the DIF index in Equation 3a. If both and were significant in the second step, and the Sobel test rejected the original hypothesis, we assessed if was significant. If was significant, it indicated a partial mediation effect. If was insignificant, it indicated a full mediation effect.
According to the test procedure of the intermediation effect, if there is an intermediation effect, the intermediation effect of DIF acting on ATFP growth in agriculture through the farmland transfer channel is , and the total effect of DIF on total factor productivity growth in agriculture is + . The proportion of the intermediation effect to the total effect is /( + ).
4.3 Variable selection and statistics
4.3.1 Agricultural input and output variable
In order to calculate the HMB index for ATFP, it is necessary to identify the input and output indicators for agriculture as shown in Table 1. The measurement of the output index must account for the effects of inflation. In this study, we utilize the total output value of agriculture, forestry, animal husbandry, and fishery with the prices flattened in 2011 as the base period (Li, 2009). The choice of using the flattened output value for agriculture, forestry, animal husbandry, and sideline fishery instead of the increment of total agricultural output value or the proportion of total agricultural output value is motivated by the need to effectively address potential measurement inconsistencies between output and input variables, thus ensuring accurate measurement results.
4.3.2 Explained variable
The dependent variable examined in this research encompasses both the total and decomposition index of ATFP. This index is derived using the agricultural input–output data discussed earlier and the HMB index method. The ATFP is a measure that assesses how efficiently inputs are converted into agricultural outputs, accounting for factors such as labor, capital, and land. By calculating the ATFP index, we can gain insights into the overall productivity performance of the agricultural sector. In addition to the total index, the study also employs a decomposition index. This index allows us to further explore the specific contributions of different input factors towards changes in agricultural productivity over time. By decomposing the ATFP index, we can analyze the relative importance and impact of variables like labor, capital, and land on agricultural productivity dynamics. To calculate these indices, we utilize the agricultural input–output data obtained through the aforementioned indicators, such as labor input, capital input, and land input. Additionally, the HMB index method is applied in the calculation process, which enables a comprehensive evaluation of ATFP from both a holistic and component-specific perspective. In selecting the method for calculating TFP, we opted for the HMB index due to its robust capacity to analyze productivity changes in the agricultural context. Unlike other methods such as the stochastic frontier approach (SFA) or the Malmquist index, the HMB index effectively accounts for both technical efficiency and technological advancements. This dual focus is particularly relevant in agriculture, where variations in resource allocation and technological adoption significantly impact productivity outcomes. Therefore, the HMB index provides a comprehensive framework that aligns with the objectives of our study.
4.3.3 Core explanatory variable
The DIF index serves as the key independent variable in this study. The Digital Inclusive Finance Index (2011–2020) is derived from data jointly prepared by the Peking University Digital Financial Inclusion Research Centre and Ant Financial Services Group. This comprehensive index includes three major categories: the breadth of digital finance coverage (DIW), the depth of digital finance usage (DIE), and the degree of digitalization of inclusive finance (DIS), encompassing a total of 33 indicators. These indicators are designed to provide a holistic view of the development of digital inclusive finance in China and serve as an authoritative measure in this field. For further details, please refer to Figure 1, which outlines the data sources. Moreover, the DIF has gained high academic recognition and carries significant authority in the field. To ensure the preservation of valuable information within the model, in the empirical research process, both the DIF index and the three sub-classification indicators mentioned above are normalized by dividing them by 100. This normalization technique allows for the effective representation and comparison of the variables, while maintaining the integrity and relevance of the results obtained throughout the analysis. Variance Inflation Factor (VIF) analysis for our core explanatory variables were conducted to assess potential multicollinearity. The VIF values for all core variables were found to be below the threshold of 5, indicating that multicollinearity is not a significant issue in our model. This supports the validity of our findings and ensures that the relationships identified are robust and reliable.
4.3.4 Intermediary variable
The intermediate variable in this study, known as farmland transfer (FT), is derived by analysing the provincial panel data from the China Rural Management Statistics Annual Report (2011–2018) and the China Rural Policy and Reform Statistics Annual Report (2019–2020). To quantify farmland transfer activities, we calculate a ratio of the total arable land contracted to the area contracted by individual families. This ratio serves as a proxy for understanding the scale of farmland transfers, indicating how much arable land is being consolidated or redistributed among families. Analysing this metric enables us to discern trends and variations in farmland transfers, which are pivotal for evaluating agricultural productivity and rural livelihoods. Such an analysis provides critical insights into the relationship between land ownership and usage rights, informing broader discussions on agricultural policy and development.
4.3.5 Control variable
Several studies have explored the various factors that influence the growth of ATFP. In this article, we refer to the findings from previous research such as the Kuang and Yang (2019) matching method regarding agricultural TFP. To address the issue of potential omitted variables, we aim to enhance the model comprehensiveness by including relevant control variables. Table 2 presents 10 selected control variables, which are incorporated into the model. Notably, three indicators - agricultural product import and export, per capita GDP, and industrial added value—exhibit relatively large magnitudes. To facilitate empirical analysis and ensure the robustness of our findings, we apply logarithmic transformation to several key variables. This transformation serves multiple purposes: it stabilizes variance, normalizes distributions, and makes the interpretation of regression coefficients more straightforward, particularly when examining growth rates. By accounting for these control variables in their logarithmic form, we aim to capture a more comprehensive understanding of the factors influencing agricultural ATFP growth.
4.3.6 Tool variable
In this study, the instrumental variable used is the Internet penetration rate (INT). Taking inspiration from the approach utilized by Xie et al. (2018), the provincial Internet penetration rate (INT) is chosen as the instrumental variable for the two-stage 2SLS estimation of the digital inclusive financial index. This selection is based on two main reasons. Firstly, the Internet serves as a vital prerequisite for the development of digital inclusive finance, and the two concepts are strongly intertwined. Hence, the Internet penetration rate is closely associated with the level of digital inclusive finance. Secondly, the study employs the lagged two-phase Internet penetration rate data as the instrumental variable for the digital inclusive financial index ranging from 2011 to 2020. While it is acknowledged that historical Internet access may provide farmers with valuable knowledge, our analysis emphasizes the direct impact of current Internet penetration on ATFP (ATFP). Theoretical considerations suggest that the relevance of past Internet access diminishes over time due to evolving agricultural practices and technologies. Consequently, we focus on the current Internet penetration rate as a key variable influencing ATFP, supported by data sourced from the Statistical Report on the Development of China’s Internet Network.
4.4 Data source
The panel data utilized in this study consists of agricultural input and output information from 30 provinces across China. These data were sourced from the China Agricultural Statistics Yearbook, with the exception of Tibet, where data was incomplete. Detailed variables can be found in Table 2. To calculate the HMB index and decomposition index of ATFP for the period spanning 2011 to 2020, the DIF index was obtained from the collaborative efforts of the “Peking University Digital Financial Inclusion Research Center” and “Ant Financial Services Group.” This index covers the years 2011 to 2020. Data regarding rural land transfer was derived from two sources: the “China Rural Management Statistical Annual Report” (2011–2018) and the “China Rural Policy and Reform Statistical Annual Report” (2019–2020). For the internet penetration rate, publicly available data from the “China Internet Development Status Statistical Report” was compiled and used. Other required data needed by the research institution were compiled and calculated based on relevant information found in the “China Rural Business Management Statistical Annual Report” and the “China Agricultural Statistical Yearbook.” In cases where data was missing, an interpolation method was employed to supplement the gaps.
5 Results and discussion
5.1 Estimation results of TFP
5.1.1 Overall trend
Figure 2 illustrates the HMB index and four decomposition indexes of ATFP from 2011 to 2020 based on agricultural input and output data. Using the HMB index method and R3.4.3 software, the figure shows that China’s ATFP growth remained >1 and maintained a slow rising trend with fluctuations, averaging a growth rate of 6.79%. The authors suggest that recent economic structural reforms carried out by the government to eliminate negative effects accumulated from regional imbalanced development and guide the economic structure towards balanced and high-quality development are likely to have contributed to the steady growth of China’s ATFP in recent years. However, the promotion of the national policy to encourage the development of the Tertiary sector of the economy may slow down the growth rate of ATFP due to the flow of high-quality input factors from rural areas into the Tertiary sector. This change may also cause limitations in traditional agricultural financial capital, leading to a slowdown in the growth rate of ATFP.
The four types of decomposition indices fluctuated around 1, with varying degrees of influence on ATFP growth. Agricultural scale efficiency (SC) had the strongest pulling effect on ATFP, with a growth rate of 1.0707 from 2011 to 2020. The authors suggest that the implementation of land transfer in China has achieved certain results in promoting scale efficiency growth, but there is still significant room for improvement if small farmers can break through the “involution” dilemma of replicating land transfer. Configuration efficiency (ME) played a crucial role in driving the growth of ATFP, averaging a growth rate of 6.77%. In contrast, agricultural technology efficiency EC showed little effect on ATFP growth, with an average growth rate of only 2.01%. Agricultural technology progress rate (TC) proved to be the weak link in driving ATFP growth, with an average of 0.9333, indicating room for improvement in the sector. In summary, the growth of China’s ATFP from 2011 to 2020 was driven by SC, ME, and agricultural technology efficiency (EC), while TC underperformed in driving ATFP growth. The authors suggest that these findings have significant implications for policymakers and stakeholders in the agriculture sector who are interested in promoting efficient and sustainable agricultural growth in the country.
5.1.2 Regional differences
Regional disparities in ATFP in China were observed and analyzed. The provinces with the highest frequency of occurrence in the top three positions were Shanghai and Tianjin, both appearing four times. Inner Mongolia ranked second with three occurrences, while Gansu, Xinjiang, Qinghai, and Chongqing appeared twice. This indicates that certain provinces consistently performed well in terms of ATFP, demonstrating geographical stability. When examining the average ATFP index, the eastern region had a value of 1.0610, while the central and western regions had values of 1.0487 and 1.0899, respectively. Overall, the western region exhibited a higher growth rate of ATFP compared to the central and eastern regions. However, it is important to note that the TC values in all three regions were below 1, indicating a negative growth rate. This suggests that TC did not drive the growth of ATFP significantly, though the regional differences in TC were not substantial.
The ME index in the central region stood out the most, with a value of 1.1606, indicating its strong contribution to driving the growth of ATFP. On the other hand, the EC values in the eastern, central, and western regions played a role in driving ATFP growth, but the regional differences were relatively small. Significant regional differences were also observed in SC among the three regions. The values were 1.1439, 0.9374, and 1.0886 for the eastern, central, and western regions, respectively. Notably, SC in the central region was consistently below 1, indicating a negative growth rate in this aspect. Regional disparities in ATFP were evident in China. The western region showcased higher growth rates compared to the central and eastern regions. TC did not significantly contribute to ATFP growth, while ME and EC played important roles. Moreover, significant regional differences were observed in SC, particularly in the central region where it exhibited a negative growth rate. These findings highlight the need to address regional disparities and focus on specific factors driving ATFP growth in different regions of China.
5.2 Analysis of regression results
5.2.1 The direct impact of DIF on ATFP
Table 3 presents the basic regression results of Equation 1a using a fixed effect model (Columns 1–4 display the results). The dependent variable is the HMB total index of ATFP, while the independent variables are the DIF index and its three sub-classification indexes. The findings suggest that the promotion of ATFP is closely linked to the DIF index and its three sub-classification indexes. Specifically, increasing the breadth of digital inclusive finance, as measured by the DIW index, has a significant positive impact on ATFP growth. For every 1% increase in the DIW index, ATFP increases by 0.152%. The results also show that the use of depth, as measured by the DIE index, and digital degree, as measured by the DIS index, have contributed to the growth of ATFP. However, the impact of these two factors is not as strong as that of the DIW index. The DIF index itself was also found to positively impact ATFP growth, but to a lesser extent. This analysis underscores the importance of digital inclusion and finance in promoting ATFP growth in China. Among the measures of digital inclusive finance, the breadth of coverage has the most significant impact on ATFP growth. These findings highlight the need for policies that promote greater digital inclusion and finance in the agricultural sector to support sustainable economic growth in China.
The SYS-GMM regression results in columns 5–8 of Table 4 demonstrate that the impact of the digital inclusive financial index and its three classification indexes on ATFP aligns with the findings from the basic regression analysis. The coefficient for the DIF index is estimated to be 0.136, indicating a statistically significant positive impact on the growth of ATFP. The three classification indexes, namely coverage, depth of use, and digitalization, also contribute to the growth of ATFP at varying levels. Among these factors, the breadth of financial coverage has the strongest effect on ATFP, surpassing the impact of depth of use and digitalization.
Digital financial inclusion, facilitated by innovative technologies such as Big Data, cloud computing, and blockchain, has brought convenience and possibilities to rural financial services while reducing transaction costs in the financial market. By expanding the coverage of agricultural financial capital and integrating agricultural production resources, it has fostered improvements in ATFP. Therefore, hypothesis 1 has been validated. The SYS-GMM regression results reinforce the significance of DIF in enhancing ATFP. The breadth of financial coverage emerges as the most influential factor, enabling greater access to agricultural financial resources and promoting resource integration. This study substantiates the importance of digital technologies in advancing rural financial services and underscores their role in driving ATFP growth.
5.2.2 The impact of DIF on the farmland transfer
To examine the impact of DIF on the level of farmland transfer in recent years, we conducted a system GMM regression analysis using Equation 2a. The results, presented in Table 4, reveal that the coefficient of the digital inclusive financial index is positive and statistically significant at the 1% level. This finding confirms that the development of DIF has indeed contributed to the enhancement of farmland transfer. DIF plays a favourable role in improving farmland transfer behavior. It provides increased access to digital credit support for households involved in farmland transfer, thereby enhancing their willingness to participate in the transfer process. As a result, the scale of transfer can expand, and the transfer period can be extended. By providing a digital platform for the integration of agricultural factors, DIF effectively enhances the internal and external scale benefits of agricultural production, leading to a significant positive impact on farmland transfer. Therefore, hypothesis 2 has been validated.
The system GMM regression analysis provides evidence that the development of DIF has positively influenced the level of farmland transfer. This implies that digital credit support has facilitated the decision-making process for farmland transfer households, resulting in increased transfer activity, scale, and duration. By fostering the integration of agricultural factors, DIF contributes to the overall improvement of farmland transfer practices.
5.2.3 The indirect impact of DIF on ATFP
The research investigates the indirect impact of DIF on ATFP by examining the HMB total index and decomposition index, which are used to analyze the influence of farmland transfer. The findings of the tests conducted are presented in Table 4. First, we focus on the ATFP HMB total index equation. Comparing the results of the basic regression and GMM regression in Table 4, we observe that the variables of interest demonstrate consistent directionality and significance. In our discussion, we will primarily focus on explaining the GMM regression results.
In Equation 1a, we tested the coefficient and obtained a significant result of 0.136. Moving forward, we explored whether the coefficients of the digital inclusive financial index in Equation 2a and the farmland transfer coefficient in Equation 3a were significant. The results indicate that the coefficient in Equation 2a is 0.091 and in Equation 3a is 0.131, both passing the significance test. These findings suggest the existence of an indirect impact of DIF on ATFP through farmland transfer. Equation 3a includes the intermediary variable of farmland transfer in the ATFP determination equation. Empirical results show that the digital inclusive financial coefficient is 0.126, which is statistically significant. Notably, this estimated result is lower than in Equation 1a, which does not include the intermediary variable of farmland transfer. It indicates that farmland transfer accounts for a portion (8.643%) of the intermediary effect in digital financial inclusion’s promotion of ATFP growth. The analysis reveals that DIF indirectly affects ATFP through farmland transfer. The inclusion of farmland transfer as an intermediary variable provides valuable insight into the mechanism through which DIF impacts agricultural productivity.
The study utilized the ATFP and HMB decomposition index equation to analyze the indirect impact of DIF on ATFP growth through farmland transfer. The same testing procedure as described earlier was followed, and the results were presented in Table 5. The coefficient in the Equation 1a was tested first, and it was found that equations with only agricultural technology efficiency (EC) and agricultural allocation efficiency (ME) as the explained variables passed the significance test. The coefficients of the digital inclusive financial index were 0.038 and 0.142, respectively, indicating that DIFhas a positive impact on improving agricultural technology efficiency and allocation efficiency. However, the equation of agricultural technology progress rate (TC) and agricultural scale efficiency (SC) as explained variables did not pass the significance test, which led to the termination of the intermediary effect test for these equations.
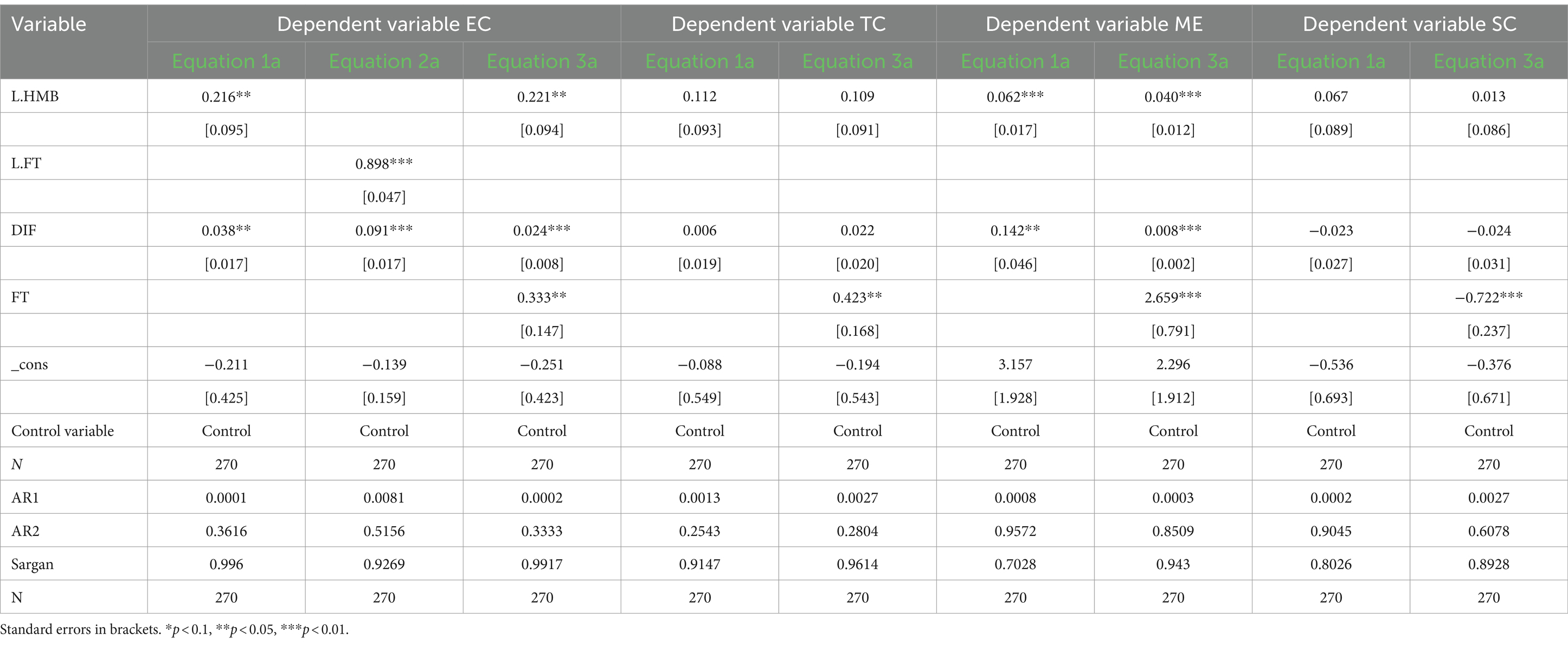
Table 5. Results of digital inclusive finance, farmland transfer, and ATFP decomposition index GMM regression (Equation 2 Shared).
In terms of regression coefficients, the growth of ATFP driven by DIF during the sample period primarily stems from the improvement in agricultural allocation efficiency. The contribution rate of DIF to agricultural technology efficiency (EC) is relatively low. Next, we tested in Equation 2a with EC as the explained variable and in Equation 3a. Both coefficients, and , passed the significance test. In the model where the explained variable is ME, the coefficients in Equation 2a and in Equation 3a also passed the significance test. This indicates that there is an indirect impact of DIF on EC and ME through farmland transfer.
Furthermore, we examined in Equation 3a. In the equation where agricultural technology efficiency EC is the explained variable, the digital inclusive financial coefficient was found to be 0.024 and passed the significance test. However, this coefficient is lower than in Equation 1a, which does not include the intermediary variable of farmland transfer. This suggests that farmland transfer contributes to approximately 55.8% of the intermediary effect in promoting EC growth through digital inclusive finance. Similarly, in the equation where agricultural technology efficiency ME is the explained variable, the digital inclusive financial coefficient was found to be 0.008 and passed the significance test. This coefficient is much lower than the coefficient in Equation 1a without the inclusion of the farmland transfer variable. These results indicate that farmland transfer plays a strong intermediary role between DIF and the growth of ME.
5.3 Endogenous analysis and robustness test
5.3.1 The robustness of SYS-GMM
In this research paper, we employed dynamic panel fixed effects and SYS-GMM regression to analyze the data. We took into consideration the endogeneity of the mitigation model by carefully selecting the model and control variables. To assess the validity of our model, Stata conducted an Areno-Bond test, providing two test values. The results of these tests are presented in Tables 3–5. The AR1 test examines the presence of first-order autocorrelation in the residuals, assuming no autocorrelation initially. Conversely, the AR2 test investigates the existence of second-order autocorrelation, also assuming no autocorrelation initially.
The findings reveal that the p-value of the AR1 test is <0.1, indicating a significant rejection of the initial hypothesis of no first-order autocorrelation. On the other hand, the p-value of the AR2 test exceeds 0.1, suggesting that the original hypothesis of no second-order autocorrelation cannot be rejected. Additionally, Tables 3–5 present the results of the Sargan test. The non-rejection of the Sargan test with a p > 0.05 indicates the validity of the over-identification limit. Both the AR and Sargan tests consistently demonstrate that DIF has a stable positive effect on ATFP through farmland transfer.
5.3.2 Tool variable method
This research paper utilizes the instrumental variable method to address potential endogenous issues, as suggested by Xie et al. (2018). The corresponding results are presented in Table 6. Firstly, it is essential to examine the correlation between the networking penetration rate (INT) and DIF. The 2SLS regression results in column (1) of Table 7, excluding control variables, indicate a positive and significant correlation between the instrumental variable (INT) and DIF. Upon including the control variables in the model (column 4), the coefficient value of INT decreases, but the positive relationship with DIF remains significant. These test outcomes align with theoretical expectations. The instrumental variable method demonstrates that the digital financial inclusion index has a substantial promoting effect on farmland transfer (FT) (columns 2 and 5) and ATFP (columns 3 and 6), irrespective of whether control variables are included.
Furthermore, the paper employs the instrumental variable method to examine the impact of DIF on EC and agricultural allocation efficiency (ME). However, the intermediary effect test for SC and TC did not yield conclusive results, thus excluding the need for a tool variable test in this regard. Table 7 reports the estimation test results using instrumental variables for the impact of digital financial inclusion on EC (column 7) and ME (column 8), including control variables. These results confirm that the regression coefficient’s direction and significance level align with the anticipated expectations. The research conclusions obtained through SYS-GMM analysis are assessed and reported in Tables 4, 5.
5.4 Regional heterogeneity
Does the influence of DIF on ATFP, through the intermediate variable of farmland transfer, differ across different regions? This study aims to conduct further analysis on this subject. Specifically, the research paper examines the relationship between digital inclusive finance, farmland transfer, and ATFP in the eastern, central, and western regions. Due to space limitations, the paper only presents the impact of DIF on the HMB total index of ATFP, and reports the corresponding test results in Table 7. The test results indicate that the coefficients of digital financial inclusion in Equation 1a, digital financial inclusion in Equation 2a, farmland transfer , and DIF in Equation 3a are significant for the eastern and western sub-samples.
The role of farmland transfer as an intermediary factor in the relationship between DIF and ATFP can be observed in both the eastern and western regions. In the central region, however, the coefficient did not demonstrate significance and therefore terminated the intermediary effect. Examining Equation 1a test results, it is evident that the impact of DIF on ATFP is strongest in the western region, followed by the eastern region. A potential explanation for this pattern is that while western China faces weak natural resources for agricultural production and relatively underdeveloped economic conditions, there is a shortage of agricultural financial capital with uneven distribution. The rapid development of DIF has effectively addressed these shortcomings by establishing a digital platform for agricultural resource allocation. As a result, the development of DIF in the western region significantly promotes ATFP. In contrast, the financial environment in the eastern region is relatively favorable, and traditional financial services adequately meet the needs of agricultural operations, production, and non-agricultural employment. Although the development of DIF has expanded financing channels and opportunities for farmers, its potential to stimulate the growth of ATFP is relatively lower compared to the western region.
Based on the regression results of the digital inclusive financial coefficient in Equation 2a and the digital inclusive financial coefficient , as well as the farmland transfer coefficient in Equation 3a, it is observed that the intermediary effect of farmland transfer in the eastern region (10.43%) is significantly higher compared to the western region (5.595%). Despite the western region experiencing relatively rapid growth in farmland transfer in recent years, the depth of farmland transfer and large-scale operations are constrained by natural conditions. Consequently, there still exist significant challenges in utilizing DIF to enhance ATFP through farmland transfer.
6 Conclusions and policy implications
This study investigates the impact of DIF on ATFP in China, utilizing a dynamic panel fixed effect model and the SYS-GMM method over the period 2011–2020. Our research reveals several innovative insights that underscore the value of DIF in enhancing agricultural productivity, particularly through the mechanism of farmland transfer.
A key finding is that China’s ATFP has demonstrated a gradual upward trend, primarily driven by scale efficiency, despite limited advancements in agricultural technology. This indicates that while technological progress may be slow, effective financial inclusion strategies play a crucial role in promoting productivity. Notably, we find that the comprehensive index of DIF—encompassing its coverage, depth of use, and degree of digitalization—significantly contributes to ATFP growth. Among these, financial coverage proves to be the most impactful, followed by depth of use and digitalization.
Importantly, our analysis highlights the mediating role of farmland transfer, which enhances the positive effects of DIF by improving resource allocation efficiency. The regional disparities observed in the effectiveness of digital finance suggest that while eastern and western regions benefit considerably, the central region shows no significant outcomes. This variation points to the necessity for targeted policies that address specific regional challenges.
The implications of our findings are substantial for policymakers and financial service providers. To promote sustainable agricultural development, it is essential to encourage planned farmland transfer and moderate-scale farming practices. This approach will improve small farmers’ efficiency while preventing excessive consolidation among larger households that could undermine overall scale efficiency. Furthermore, investment in agricultural research and improved fund utilization is critical to overcoming barriers hindering total productivity growth.
To bridge the digital gap, especially in regions with limited access to digital inclusive finance, it is imperative to enhance hardware and software infrastructure, including network equipment and financial services. In areas with higher coverage, upgrading farmers’ digital literacy will enable better utilization of financial services, aligning supply with demand and effectively meeting their needs.
To effectively channel DIF investment towards farmland, it is crucial to establish classification guidelines. These guidelines will ensure that the financial needs of both large and small circulation farmers are adequately addressed, thereby lowering the barriers for small-scale circulation farmers to access agricultural production funds. Additionally, capital should be harnessed to supplement rural labor, further enhancing its role in the farming sector. Policymakers and service providers have a pivotal role to play in maximizing the intermediary effect of farmland transfer within the realm of digital inclusive finance. By doing so, they can actively encourage local ATFP growth.
In regions like the developed eastern and central areas, the focus should be squarely on fostering innovation and application of digital inclusive financial services. The key lies in personalizing financial offerings to precisely match the diverse needs of farmers and improving the accuracy of these matches. Furthermore, unlocking the full potential of financial digitalization levels and usage depths is essential. This can be achieved by promoting flexible development loan cycles and embracing diverse repayment approaches. Such initiatives will not only boost farmers’ land transfer capabilities but also accelerate technology adoption through the integration of products like digital inclusive financial coverage.
Data availability statement
The raw data supporting the conclusions of this article will be made available by the authors, without undue reservation.
Author contributions
JY: Conceptualization, Data curation, Formal analysis, Funding acquisition, Investigation, Methodology, Project administration, Software, Supervision, Validation, Writing – original draft, Writing – review & editing. AM: Conceptualization, Validation, Writing – original draft, Writing – review & editing.
Funding
The author(s) declare that financial support was received for the research, authorship, and/or publication of this article. The funding for this research project was provided by the Guangdong Provincial Philosophy and Social Science Plan for 2022 (GD22CGL12) and Characteristic Innovation Project of Ordinary Universities in Guangdong Province (2024WTSCX036). Additionally, Shaoguan University is supporting a talent introduction research project related to this study.
Conflict of interest
The authors declare that the research was conducted in the absence of any commercial or financial relationships that could be construed as a potential conflict of interest.
Publisher’s note
All claims expressed in this article are solely those of the authors and do not necessarily represent those of their affiliated organizations, or those of the publisher, the editors and the reviewers. Any product that may be evaluated in this article, or claim that may be made by its manufacturer, is not guaranteed or endorsed by the publisher.
Footnotes
1. ^The information regarding farmland scale endowment was obtained from the website: https://www.gov.cn/xinwen/2019-03/02/content_5369853.htm.
References
Adamonpoulos, T., Brandt, J., Leight, D., and Restuccia, D. (2019). Misallocation, selection, and productivity: A quantitative analysis with panel data from China. Econometrica 90, 1261–1282. doi: 10.3982/ECTA16598
Adamopoulos, T., and Restuccia, D. (2014). The size distribution of farms and international productivity differences. Am. Econ. Rev. 104, 1667–1697. doi: 10.1257/aer.104.6.1667
Adegbite, O. O., and Machethe, C. L. (2020). Bridging the financial inclusion gender gap in smallholder agriculture in Nigeria: An untapped potential for sustainable development. World Dev. 127:104755. doi: 10.1016/j.worlddev.2019.104755
Adu-Baour, F., Daum, T., and Birner, R. (2019). Can small farms benefit from big companies' initiatives to promote mechanization in Africa? A case study from Zambia. Food Policy 84, 133–145. doi: 10.1016/j.foodpol.2019.03.007
Alvarez, J., and Arellano, M. (2022). Robust likelihood estimation of dynamic panel data models. J. Econ. 226, 21–61. doi: 10.1016/j.jeconom.2021.03.005
An, C., He, X., and Zhang, L. (2023). The coordinated impacts of agricultural insurance and digital financial inclusion on agricultural output: evidence from China. Heliyon 9:e13546. doi: 10.1016/j.heliyon.2023.e13546
Baron, R. M., and Kenny, D. A. (1986). The moderator-mediator variable distinction in social psychological research: conceptual, strategic, and statistical considerations. J. Pers. Soc. Psychol. 51, 1173–1182. doi: 10.1037/0022-3514.51.6.1173
Bjurek, H. (1996). The Malmquist Total factor productivity index. Scand. J. Econ. 98, 303–313. doi: 10.2307/3440861
Caves, D. W., Christensen, L. R., and Diewert, W. E. (1982). The economic theory of index numbers and the measurement of input, output, and productivity. Econometrica 50, 1393–1414. doi: 10.2307/1913388
Chen, J., and Luo, H. (2022). Digital inclusive finance, financial literacy, and household financial asset selection. Wuhan Finance 2022, 51–61. doi: 10.1371/journal.pone.0267055
Chen, Y., and Wen, T. (2023). Can digital finance promote the development of rural industries: an analysis based on spatial econometric models. Agric. Technol. Econ. 2023, 32–44.
Duan, J., Ren, C., Wang, S., Zhang, X., Reis, S., Xu, J., et al. (2021). Consolidation of agricultural land can contribute to agricultural sustainability in China. Nat. Food 2, 1014–1022. doi: 10.1038/s43016-021-00415-5
Duquenne, M. N., Tsiapa, M., and Tsiakos, V. (2019). Contribution of the common agricultural policy to agricultural productivity of EU regions during 2004–2012 period. Rev. Agric. Food Environ. Stu. 100, 47–68. doi: 10.1007/s41130-019-00093-9
Färe, R., Grosskopf, S., and Roos, P. (1995). The Malmquist total factor productivity index: some remarks. J. Prod. Anal. 6, 131–140.
Fei, R., Lin, Z., and Chunga, J. (2021). How land transfer affects agricultural land use efficiency: evidence from China's agricultural sector. Land Use Policy 103:105300. doi: 10.1016/j.landusepol.2021.105300
Gao, J., Song, G., and Sun, X. (2020). Does labor migration affect rural land transfer? Evidence from China. Land Use Policy 99:105096. doi: 10.1016/j.landusepol.2020.105096
Hu, Y., Liu, C., and Peng, J. (2021). Financial inclusion and agricultural total factor productivity growth in China. Econ. Model. 96, 68–82. doi: 10.1016/j.econmod.2020.12.021
Huang, K., Cao, S., Qing, C., Xu, D., and Liu, S. (2023). Does labour migration necessarily promote farmers' land transfer-in?—empirical evidence from China's rural panel data. J. Rural. Stud. 97, 534–549. doi: 10.1016/j.jrurstud.2022.12.027
Huang, Z., Wang, J., and Chen, Z. (2014). The impact of non-agricultural employment, agricultural land transfer, and land fragmentation on the technical efficiency of rice farmers. China Rural Econ. 2014, 4–16.
Hunjra, A. I., Zhao, S., Tan, Y., Bouri, E., and Liu, X. (2024). How do green innovations promote regional green total factor productivity? Multidimensional analysis of heterogeneity, spatiality and nonlinearity. J. Clean. Prod. 467:142935. doi: 10.1016/j.jclepro.2024.142935
Janus, J., Ertunç, E., Muchová, Z., and Tomić, H. (2023). Impact of selected land fragmentation parameters and spatial rural settlement patterns on the competitiveness of agriculture: examples of selected European and Asian countries. Habitat Int. 140:102911. doi: 10.1016/j.habitatint.2023.102911
Jiang, M., Paudel, K. P., Peng, D., and Mi, Y. (2020). Financial inclusion, land title and credit: evidence from China. China Agric. Econ. Rev. 12, 257–273. doi: 10.1108/CAER-01-2019-0020
Jin, J. (2022). The impact of digital finance on rural industrial revitalization in the context of common prosperity: An empirical study based on provincial panel data. Bus. Econ. Res. 2022, 177–180.
Kerstens, K., and Van de Woestyne, I. (2014). Comparing Malmquist and Hicks-Moorsteen productivity indices: exploring the impact of unbalanced vs. balanced panel data. Eur. J. Oper. Res. 233, 749–758. doi: 10.1016/j.ejor.2013.09.009
Kos, D., and Kloppenburg, S. (2019). Digital technologies, hyper-transparency and smallholder farmer inclusion in global value chains. Curr. Opin. Environ. Sustain. 41, 56–63. doi: 10.1016/j.cosust.2019.10.011
Kuang, Y., and Lu, Y. (2018). The trap of "involution" in the transfer of agricultural land in China and its solutions. Agricultural. Econ. Issues 2018, 33–43.
Kuang, Y., and Yang, J. (2019). The growth effect of total factor productivity in rural land transfer. Economist 2019, 102–112.
Kuang, Y.-P., Yang, J.-L., and Abate, M.-C. (2022). Farmland transfer and agricultural economic growth nexus in China: agricultural TFP intermediary effect perspective. China Agric. Econ. Rev. 14, 184–201. doi: 10.1108/CAER-05-2020-0076
Lan, J., Pan, Y., and Yu, Y. (2023). The role of digital financial inclusion in increasing fertility intentions: evidence from China. Appl. Econ. 1–19.
Leng, X. (2022). Digital revolution and rural family income: evidence from China. J. Rural. Stud. 94, 336–343. doi: 10.1016/j.jrurstud.2022.07.004
Li, G. (2009). Technical efficiency, technological Progress, and China's agricultural productivity growth. Econ. Rev. 1, 25–33.
Li, M., and Han, X. (2014). Financing problems in China's rural areas. J. Northeast Agric. Univ. 21, 80–89. doi: 10.1016/S1006-8104(14)60038-6
Liu, Y., Deng, Y., and Peng, B. (2023). The impact of digital financial inclusion on green and low-carbon agricultural development. Agriculture 13:1748. doi: 10.3390/agriculture13091748
Liu, Y., Liu, C., and Zhou, M. (2021a). Does digital inclusive finance promote agricultural production for rural households in China? Research based on the Chinese family database (CFD). China Agric. Econ. Rev. 13, 475–494. doi: 10.1108/CAER-06-2020-0141
Liu, Y., Luan, L., Wu, W., Zhang, Z., and Hsu, Y. (2021b). Can digital financial inclusion promote China's economic growth? Int. Rev. Financ. Anal. 78:101889. doi: 10.1016/j.irfa.2021.101889
Liu, H., and Ren, Y. (2023). Can digital inclusive finance ensure food security while achieving low-carbon transformation in agricultural development? Evidence from China. J. Clean. Prod. 418:138016. doi: 10.1016/j.jclepro.2023.138016
Lu, H., Xie, H., He, Y., Wu, Z., and Zhang, X. (2018). Assessing the impacts of land fragmentation and plot size on yields and costs: a translog production model and cost function approach. Agric. Syst. 161, 81–88. doi: 10.1016/j.agsy.2018.01.001
Lu, H., Xie, H., and Yao, G. (2019). Impact of land fragmentation on marginal productivity of agricultural labor and non-agricultural labor supply: a case study of Jiangsu, China. Habitat Int. 83, 65–72. doi: 10.1016/j.habitatint.2018.11.004
Manjunatha, V. J., Anik, A. R., Speelman, S., and Nuppenau, E. A. (2013). Impact of land fragmentation, farm size, land ownership and crop diversity on profit and efficiency of irrigated farms in India. Land Use Policy 31, 397–405. doi: 10.1016/j.landusepol.2012.08.005
Matthews, B. H. (2019). Hidden constraints to digital financial inclusion: the oral-literate divide. Dev. Prac. 29, 1014–1028. doi: 10.1080/09614524.2019.1654979
Moreira, H., and Bravo-Ureta, B. (2016). Farmland protection: effects of grazing method and fertilizer inputs on the productivity and sustainability of phalaris-based pastures in Canada. Anim. Prod. Sci. 13, 85–98.
Muchová, Z., and Raškovič, V. (2020). Fragmentation of land ownership in Slovakia: evolution, context, analysis and possible solutions. Land Use Policy 95:104644. doi: 10.1016/j.landusepol.2020.104644
Nakano, Y., and Magezi, E. F. (2020). The impact of microcredit on agricultural technology adoption and productivity: evidence from randomized control trial in Tanzania. World Dev. 133:104997. doi: 10.1016/j.worlddev.2020.104997
Niroula, G. S., and Thapa, G. B. (2005). Impacts and causes of land fragmentation, and lessons learned from land consolidation in South Asia. Land Use Policy 22, 358–372. doi: 10.1016/j.landusepol.2004.10.001
Qin, Z., Pei, X., Andrianarimanana, M. H., and Shizhou, W. (2023). Digital inclusive finance and the development of rural logistics in China. Heliyon 9:e17329. doi: 10.1016/j.heliyon.2023.e17329
Qu, M., Zhao, K., and Zhou, S. (2019). The impact of farmland transfer on wheat production efficiency: an analysis of the regulatory effect based on household livelihood differentiation. Res. Sci. 41, 1911–1922.
Ren, J., and Lei, H. (2022). Digital inclusive finance, capital deepening, and total factor productivity in agriculture. Soc. Sci. 2022, 86–95.
Rogers, S., Wilmsen, B., Han, X., Wang, Z. J.-H., Duan, Y., He, J., et al. (2021). Scaling up agriculture? The dynamics of land transfer in inland China. World Dev. 146:105563. doi: 10.1016/j.worlddev.2021.105563
Romer, P. M. (1986). Increasing returns and long-run growth. J. Polit. Econ. 94, 1002–1037. doi: 10.1086/261420
Shen, Y., Guo, X., and Zhang, X. (2023). Digital financial inclusion, land transfer, and agricultural green Total factor productivity. Sustain. For. 15, 1–15. doi: 10.3390/su15086436
Shi, C., Zhan, P., and Zhu, J. (2020). Agricultural land transfer, factor allocation, and improvement of agricultural production efficiency. China Land Sci. 34, 49–57.
Shi, C., Zhu, J., and Jie, C. (2016). Regional differences and convergence analysis of total factor productivity growth in China's agriculture: based on the fixed effects SFA model and panel unit root method. Exp. Econ. Issues 2016, 134–141.
Song, W., and Yang, H. (2022). Research on the impact of digital finance on rural family entrepreneurship. Agric. Econ. 2022, 113–114.
Sun, G., Li, T., and Mo, Y. (2023). The impact of the digital economy on China's agricultural total factor productivity. Econ. Manage. Rev. 39, 92–103.
Sun, Y., and Tang, X. (2022). The impact of digital inclusive finance on sustainable economic growth in China. Financ. Res. Lett. 50:103234. doi: 10.1016/j.frl.2022.103234
Sun, X.-T., Yu, T., and Yu, F.-W. (2022). The impact of digital finance on agricultural mechanization: evidence from 1869 counties in China. China Rural Econ. 2, 76–93.
Tan, S., Heerink, N., Kuyvenhoven, A., and Qu, F. (2010). Impact of land fragmentation on rice producers’ technical efficiency in south-East China. NJAS-Wageningen J. Life Sci. 57, 117–123. doi: 10.1016/j.njas.2010.02.001
Tang, J., Gong, J., and Song, Q. (2022). Digital inclusive finance and agricultural total factor productivity: from the perspective of factor flow and technology diffusion. China Rural Econ. 2022, 81–102.
Wang, Y. (2023). Digital inclusive finance and rural revitalization. Financ. Res. Lett. 57:104157. doi: 10.1016/j.frl.2023.104157
Wang, H., Hu, X., Wang, J., Zhang, Z., Shi, Z., and Zhou, H. (2023). The impact of farmland fragmentation in China on agricultural productivity. J. Clean. Prod. 425:138962. doi: 10.1016/j.jclepro.2023.138962
Wang, Y., Li, X., Lu, D., and Yan, J. (2020). Evaluating the impact of land fragmentation on the cost of agricultural operation in the southwest mountainous areas of China. Land Use Policy 99:105099. doi: 10.1016/j.landusepol.2020.105099
Wang, F., and Ran, L. (2022). The impact of digital finance on alleviating relative poverty in rural China. China Circ. Econ. 36, 105–114.
Wang, Y., Zhang, Y., and Liao, M. (2019). The impact of foreign direct investment on agricultural total factor productivity: mechanism and empirical evidence. Contemp. Econ. Res. 2019, 74–113.
Xiang, Y. (2022). Research on the impact of digital finance on rural residents' consumption: based on dynamic and threshold effects. Bus. Econ. Res. 2022, 172–176.
Xie, X., Shen, Y., Zhang, H., and Guo, F. (2018). Can digital inclusive finance promote entrepreneurship—evidence from China. Economics 17, 1557–1580.
Xiong, M., Fan, J., Li, W., and Teo, B. S. X. (2022). Can China's digital inclusive finance help rural revitalization? A perspective based on rural economic development and income disparity. Front. Environ. Sci. 10:985620. doi: 10.3389/fenvs.2022.985620
Xue, C., Shi, X., and Zhou, H. (2020). Research on the impact path of agricultural mechanization on the improvement of total factor productivity in the planting industry. Agric. Technol. Econ. 2020, 87–102.
Yang, M., Fu, M., and Zhang, Z. (2021). The adoption of digital technologies in supply chains: drivers, process and impact. Technol. Forecast. Soc. Chang. 169:120795. doi: 10.1016/j.techfore.2021.120795
Yang, B., Ma, F., Deng, W., and Pi, Y. (2022). Digital inclusive finance and rural household subsistence consumption in China. Econ. Anal. Policy 76, 627–642. doi: 10.1016/j.eap.2022.09.007
Yang, Y., Tao, W., and Wang, Y. (2022). The impact of digital finance on the income gap between urban and rural residents. Reformation 2022, 64–78.
Yu, C., Jia, N., Li, W., and Wu, R. (2022). Digital inclusive finance and rural consumption structure – evidence from Peking University digital inclusive financial index and China household finance survey. China Agric. Econ. Rev. 14, 165–183. doi: 10.1108/CAER-10-2020-0255
Yu, W., Zhang, P., and Ji, Z. (2020). The spatial interaction effect of rural education human capital and agricultural Total factor productivity in provincial areas of China: empirical analysis based on spatial simultaneous equations. J. China Agric. Univ. 25, 192–202.
Yue, P., Korkmaz, A. G., Yin, Z., and Zhou, H. (2022). The rise of digital finance: financial inclusion or debt trap? Financ. Res. Lett. 47:102604. doi: 10.1016/j.frl.2021.102604
Zeng, Q., and Zhang, X. (2022). Research on the threshold effect of digital inclusive finance on agricultural green total factor productivity: based on the perspective of agricultural industry agglomeration. Reg. Financ. Res. 2022, 77–83.
Zhang, L. (2021). Digital finance, county industry upgrading, and farmers' income growth. Res. Financ. Issues 2021, 51–59.
Zhang, Y. (2022). Research on the impact and mechanism of digital finance on rural land transfer: empirical evidence from CFPS and PKU-DFIIC. Econ. Manag. 36, 30–40.
Zhang, Q., and Tian, J. (2023). Can digital finance improve agricultural total factor productivity: based on heterogeneity and spatial spillover effects. Agric. Econ. Manage. 2023, 45–56.
Zheng, H., and Li, G. (2022). The impact of digital finance development on the growth of total factor productivity in county agriculture: based on heterogeneity. Contempor. Econ. Manage. 2022, 81–87.
Zhou, Y., and Miao, Z. (2023). The impact of digital finance on farmers' production and operation investment. China Rural Obs. 2023, 40–58.
Zhou, M., Zhang, H., Zhang, Z., and Sun, H. (2023). Digital financial inclusion, cultivated land transfer and cultivated land green utilization efficiency: an empirical study from China. Sustain. For. 15:1569. doi: 10.3390/su15021569
Keywords: farmland transfer, digital inclusive finance, agricultural total factor productivity, scale efficiency, allocation efficiency
Citation: Yang JL and Meseretchanie A (2024) The intermediate role of farmland transfer in the impact of digital financial inclusion on agricultural total factor productivity in China. Front. Sustain. Food Syst. 8:1345549. doi: 10.3389/fsufs.2024.1345549
Edited by:
Philipp Aerni, School of Management Fribourg (HES-SO), SwitzerlandReviewed by:
Jakub Staniszewski, Poznań University of Economics and Business, PolandPeng Jiquan, Jiangxi University of Finance and Economics, China
Copyright © 2024 Yang and Meseretchanie. This is an open-access article distributed under the terms of the Creative Commons Attribution License (CC BY). The use, distribution or reproduction in other forums is permitted, provided the original author(s) and the copyright owner(s) are credited and that the original publication in this journal is cited, in accordance with accepted academic practice. No use, distribution or reproduction is permitted which does not comply with these terms.
*Correspondence: Abate Meseretchanie, YWJhdGVtZXNlcmV0Y2hhbmllMzQwN0BodXNlLmVkdS5jbg==