- 1Guangzhou Urban Planning & Design Survey Research Institute, Guangzhou, China
- 2Guangdong Enterprise Key Laboratory for Urban Sensing, Monitoring and Early Warning, Guangzhou, China
- 3School of Geography and Planning, Sun Yat-sen University, Guangzhou, China
Due to the imbalance in the supply and demand of agricultural land, there is an increasing trend of land resources being remoted utilized across regions. Within the context of regional trade, changes in the agroecosystem service value (ESV) can be used to assess consumer responsibility. However, the embodied flow of ESV and its driving factors have been largely unexplored. This paper, utilizing the latest Chinese multi-regional input–output tables and the equivalence factor method, explores the spatiotemporal characteristics of embodied agricultural land and ESV flow between Guangdong and 30 other provinces, and further seeks to construct three types of embodied ESV models to reveal the driving factors. The findings reveal that during the process of domestic trade in goods and services, Guangdong Province has an inflow of 2.10 × 107 hm2 of land from other provinces, with arable land, forestland, and grassland accounting for 25, 61, and 14%, respectively. Guangdong’s utilization of ESV is mainly dependent on external inflows, with minimal local consumption and outflows. The embodied ESV between Guangdong and other provinces is 1626.10 billion yuan, with an outflow of 325.32 billion yuan. The “Y”-shaped region consisting of the northwest, northeast, southwest, and Hainan bears significant potential ESV losses for Guangdong Province. Population growth and the intensity of ESV loss will promote the flow of cropland and forestland ESV, while economic development has a certain inhibitory effect on ESV transfer. This paper provides a new analytical perspective on issues such as the spatial distribution mismatch of land resources and ecologically unequal exchange. These insights are pivotal for promoting sustainable utilization of land resources and regional equity.
1 Introduction
Ecosystem service value (ESV) is a quantitative estimate of the potential service capacity of ecosystems (De Groot et al., 2012). Costanza et al. (1997) estimated the global annual average ESV to be 33 trillion US dollars, significantly higher than the global annual GDP of 18 trillion US dollars, thus profoundly demonstrating the immense economic value inherent in ES. Since then, ESV research has experienced rapid development (Kubiszewski et al., 2013; Zeng et al., 2023). Land serves as the spatial carrier for primary economic activities of human society and is also the fundamental resource and material basis for human survival. In other words, land possesses not only socioeconomic attributes but also ecological values, including provisioning, regulating, supporting, and cultural services, i.e., ESV. In the prevailing economic organizational framework, characterized by expanding production networks and the realignment of resource supply to meet commodity demand, economically developed regions with large populations and relatively scarce land resources indirectly utilize the land resources of other regions through the trade of land intensive products, thereby alleviating land resource shortages and obtaining ecosystem services (Chen and Han, 2015). Existing research has shown that under the context of “ecologically unequal exchange, “developed economies inadvertently transfer ecological and environmental burdens to less developed economies. This transfer often manifests in the form of significant challenges like biodiversity loss, soil erosion, and food insecurity, exacerbating environmental issues in these underdeveloped regions (Shandra et al., 2009; Marselis et al., 2017; Dorninger et al., 2021). However, this environmental damage remains uncompensated, whether through monetary transfers in trade or economic assistance. Therefore, accounting for the profit and loss of ESV resulting from trade interactions between different regions is of significant importance for regional equity and sustainable development.
Regional ESV can be transferred through two primary mechanisms: natural processes and trade activities. For example, ecosystem products can naturally move across regions through media such as water, air, and soil, leading to spatial transfers of ESV (Koellner et al., 2019). This type of research often falls under the theme of “ecosystem service flows” and is based on an overall research framework of “pattern analysis-flow simulation-supply and demand matching,” ultimately leading to ESV accounting (Villamagna et al., 2013; Cui et al., 2022; Liu C. et al., 2023). Alternatively, ecosystem products can be transferred in the form of entities (e.g., commodities) through trade networks. The embodied flow of ESV within trade activities has garnered increasing attention from domestic and international academia. For instance, Chen et al. (2019) used a multi-regional input–output model (MRIO) to explore interprovincial land consumption and ecosystem services remotely correlated in China, but did not valorize ecosystem services. Lin et al. (2020) employed an environmental impact assessment method and MRIO to establish an ecological compensation estimation framework for the Beijing-Tianjin-Hebei region in 2012, using the ratio of the number of adjacent regions to quantify the transfer of ESVs from neighboring regions. Zhang et al. (2021) introduced research on ecologically unequal exchange from Ecological Marxism into the field of ecological compensation and proposed the use of currently more mature environmentally extended MRIO models to calculate the disparities in interregional unequal exchange. The MRIO models can track value chains and their feedback effects among multiple trading partners through interregional trade matrices, thereby revealing the ultimate consumers of products and the associated resource and environmental costs (Miller, 1969). However, research based on this model has primarily focused on accounting for embodied land resource at different scales (O’Brien et al., 2015; Bosire et al., 2016; Vivanco et al., 2017). In summary, research related to the profits and losses of ESV from a domestic and international trade perspective is still in its early stages. The research topics, quantity, and targeted regions are relatively limited. The idea of measuring interregional ESV transfer based on embodied land research is reasonable and feasible (Zeng et al., 2023). Therefore, this study utilizes a MRIO model to assess the transfer of agricultural land and ESV between regions to address the shortcomings in the research on ecological compensation.
There are three main methods for assessing ESV: the Integrated Valuation of Ecosystem Services and Tradeoffs (InVEST) model (Sharp et al., 2020), the energy-based accounting method (Yang et al., 2019) and economic assessment. Specifically, the InVEST model focuses on obtaining the total value by quantifying the amount of ecosystem service functions and their unit prices. Such methods simulate ecosystem service functions at a local level by establishing production equations between individual service functions and local ecological environmental variables. The energy-based accounting method, from an ecological thermodynamics’ perspective, posits that everything necessary for human survival ultimately relies on natural resource provisioning, driven fundamentally by solar energy at different spatial and temporal scales (Franzese et al., 2017). Therefore, it can convert different types of incomparable energies into a comparable and standardized measure known as solar energy value. The method of equivalence factor belongs to the economic assessment category and is primarily represented by the global equivalent factor table developed by Costanza et al. (2014) and the Economics of Ecosystems and Biodiversity (TEEB), as well as the national-level equivalent factor table developed by Xie et al. (2017). In provincial studies, the equivalent factor method is widely adopted due to its operability and efficiency. On one hand, the value of standard unit ESV equivalent factors can be adjusted based on the natural and socio-economic characteristics of the consuming provinces to better reflect the actual values in unequal exchanges. On the other hand, when discussing specific compensation types, researchers can easily add or subtract ESV categories using this method.
In this study, agroecosystem services associated with Guangdong Province are selected as the main research object. Guangdong is the largest provincial economy in China with a large population and strong trade links with the rest of the country. On the other hand, among the various types of land implicit in regional trade, agricultural land is considered to have important ecological value, while construction land associated with industry and services has low ESV (Kubiszewski et al., 2020; Xiao et al., 2020). Guangdong is also an important agricultural production area in China, and the transferred ESV is not negligible. Therefore, assessing the profits and losses of ESV and its driving factors in Guangdong is representative and noteworthy. Based on the latest MRIO tables and equivalent factor method, this study examines the spatiotemporal characteristics of embodied agricultural land and ESV flow between Guangdong Province and 30 other provinces, and seeks to construct three types of embodied ESV models to reveal the driving factors. This study provides a new analytical perspective for spatial mismatch of land resources and ecologically unequal exchange and offer theoretical and data support for achieving sustainable land resource utilization and regional equity.
2 Methodology and data
2.1 Transfer of agricultural land
The MRIO tables in China contain the input–output relationship among 31 provinces (excluding Taiwan, Hong Kong, and Macau) and 42 sectors. Following the approach by Shaojian and Jieyu (2023), the agricultural sector has been disaggregated into three types of agricultural land (arable land, forest land, pasture) to provide different land use coefficients for each type of land, and to estimate the embodied agricultural land flows between Guangdong province and other provinces. The MRIO model can be described as:
where m represents the number of provinces and n represents the number of sectors. represents the total output of sector i in region r; represents the value of output from sector j in region s that requires one monetary unit of output from sector i in region r, i.e., the direct input coefficient; represents the final consumption of sector i in region s provided by region r; represents the products or services of sector i in region r supplied to the rest of regions. Eq. 1 can be simplified into a matrix to describe the entire input–output system as follows:
where x, A, and Y represent matrices for inputs, direct input coefficients, and final demand, respectively. Eq. 3 is a transformed version of Eq. 2, where (I-A)−1 is the Leontief inverse matrix, and I is the identity matrix. To calculate the hidden land used in the production of goods and services, Eq. 3 can be further extended by incorporating a land use coefficient matrix:
where is the column vector representing the use of land type k by various economic sectors in each province, and is the flow matrix representing the land area of land type k used by various sectors in each province.
2.2 Calculation of ESV
Ecosystem services can be classified into four main categories with nine service functions, including provisioning services (food production, raw material production), regulating services (gas regulation, climate regulation, hydrological regulation, waste treatment), supporting services (soil conservation, biodiversity), and cultural services (aesthetic landscape) (Xie et al., 2008; Leemans, 2009). The ESV coefficients per unit area for arable land, forest land, and grassland were revised based on the geographical location and natural conditions of Guangdong Province (Zhang et al., 2022; Liu Z. et al., 2023), resulting in comprehensive coefficients of 1.946, 39.368, and 16.338, respectively. Because arable land is primarily used for productive activities, this study assumes that only provisioning ESV are transferred in trade, while other services are used locally. It is also assumed that all four categories of ESV for forest land and grassland are transferred or lost in trade when products and services are moved and no longer function locally. Although forest land and grassland may continue to provide some ecosystem services after being converted into commodities and services in trade, due to data constraints, these assumptions are necessary. The standard unit ESV equivalence factor in this study is calculated using Guangdong Province’s major cereal crops, mainly rice and potatoes (Zhao and Su, 2022). Through calculation, the economic value of one standard unit ESV equivalence factor in Guangdong is determined to be 2870.73 yuan/hm2, and this value is used in conjunction with the hidden land matrix and value coefficients to calculate the transfer of ESV between regions. The ESV calculation formula is as follows:
where represents the ESV of land type k transferred from province r to province s; is the area of land type k transferred; is the comprehensive coefficient of ESV per unit area of land type k; D is the economic value of one standard unit ESV equivalence factor in Guangdong Province.
2.3 STIRPAT model
York et al. transformed the classic IPAT equation (York et al., 2003; Lohwasser et al., 2020), which states that environmental impact (I) is equal to the product of population (P), affluence (A), and technological level (T), into a stochastic regression impact model known as the STIRPAT model to reveal the influence of socio-economic factors on the ESV (I). The formula is as follows:
where a is a constant; the coefficients for P, A, and T are represented as b, c, and d, and e is the error term. When analyzing the factors affecting ESV transfers using Eq. 6 (Luo et al., 2020), I represents the ESV value transferred from a province to Guangdong. An increase in population size leads to an expansion of production scale, thus transferring ESV through trade relationships. Therefore, this study uses population size to represent P. Affluence represents the region’s level of economic development and income, influencing production structure and ESV gains and losses. This study uses per capita consumption expenditure, urbanization rate, and GDP to characterize A. Additionally, following the research of Zhu et al., the ESV loss caused by trillion GDP is used as an indicator of technological level (Zhu et al., 2019). According to Eq. 7, if two of the three driving factors, P, A, and T, remain constant, the impact of the remaining factor on environmental changes is considered as its contribution to environmental change.
2.4 Data
The MRIO table contains the interregional input–output table of 31 provinces in mainland China, which was published by the China Emission Accounts & Datasets1 (Zheng et al., 2021). Land use data is sourced from the “China Statistical Yearbook.” Grain crop data and the proportion coefficients for the disaggregation of the agricultural sector are obtained from the ‘China Rural Statistical Yearbook’ and the ‘Guangdong Rural Statistical Yearbook.’ The grain crop data (unit output value and the proportion of planting area for rice and potatoes) are used to calculate the economic value of the equivalence factor. The proportion coefficients for the disaggregation of the agricultural sector are based on the percentages of the four agricultural sectors (agriculture, forestry, animal husbandry, and fishery) recorded in the ‘China Rural Statistical Yearbook,’ which are used to divide the ‘agriculture, forestry, animal husbandry, and fishery products and services’ sector in the input–output table into the four sub-sectors for each province. This division aligns with the categories of land use (arable land, forest land, pasture, and fishery land) and provides a more accurate estimate of land use coefficients for the sub-sectors. Population, GDP, per capita consumption expenditure, and other data are sourced from the ‘China Statistical Yearbook.’ All data in this study are from the year 2017.
3 Results
3.1 Embodied flow of agricultural land in Guangdong
Figure 1 illustrates the inflow and outflow of agricultural land caused by final demand in Guangdong Province in 2017. In 2017, the areas of arable land, forest land, and pasture in Guangdong Province were 2.60 × 106 hm2, 1.08 × 107 hm2, and 3.1 × 103 hm2, respectively, with pasture land being relatively scarce. As one of the most developed provinces in China, Guangdong Province has a significant amount of embodied inflow land in the process of domestic trade in goods and services. The total land gains and losses in Figure 1A show that Guangdong Province had a total of 2.10 × 107 hm2 of three types of embodied land, with only 3.53 × 106 hm2 outflowed, resulting in an inflow–outflow ratio of 5.96. Among these, Yunnan, Guangxi, Inner Mongolia, Guizhou, and Heilongjiang are the largest sources of supply for the province’s agricultural sector, accounting for 15.20, 13.36, 12.22, 10.70, and 8.31% of the total inflow, respectively. The five provinces with the smallest inflow areas are Shanghai, Beijing, Tianjin, Ningxia, and Shanxi, collectively accounting for only 0.83% of the total inflow. On the other hand, the top five provinces to which Guangdong transfers its agricultural land are Zhejiang, Henan, Jiangsu, Beijing, and Yunnan, with the outflow to Zhejiang accounting for 43.87% of the total. The five provinces with the smallest outflow areas are Qinghai, Tibet, Hainan, Ningxia, and Tianjin, together accounting for only 2.58% of Guangdong’s total outflow.
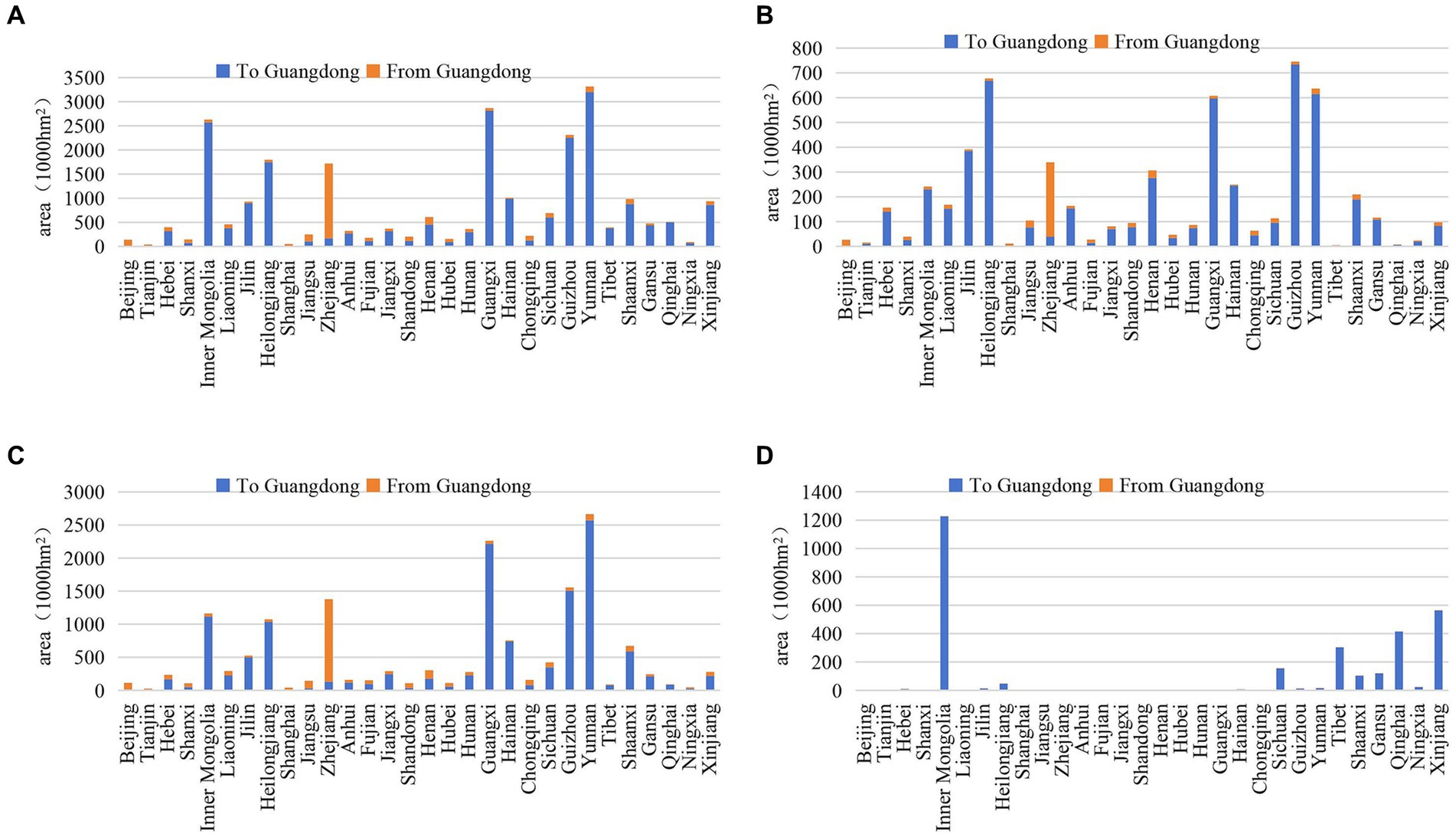
Figure 1. Embodied agricultural land inflows/outflows in Guangdong Province in 2017. (A) Total land area. (B) Arable land. (C) Forest land. (D) Pasture.
As shown in Figure 1B, the domestic inflow of arable land into Guangdong Province amounts to 5.16 × 106 hm2, while the outflow from Guangdong is 6.84 × 105 hm2, resulting in an inflow–outflow ratio of 7.55. The top five provinces with the highest inflow of arable land into Guangdong are Guizhou, Heilongjiang, Yunnan, Guangxi, and Jilin, accounting for 14.20, 12.93, 11.89, 11.55, and 7.46% of the total inflow, respectively. The five provinces with the smallest inflow of arable land into Guangdong are Shanghai, Tibet, Beijing, Qinghai, and Tianjin, with Shanghai having the smallest inflow of only 1626.70 hm2. Figure 1C indicates that the domestic inflow of forest land into Guangdong Province amounts to 1.29 × 107 hm2, while the outflow from Guangdong is 2.84 × 106 hm2, resulting in an inflow–outflow ratio of 4.53. The top five provinces with the highest inflow of forest land into Guangdong are Yunnan, Guangxi, Guizhou, Inner Mongolia, and Heilongjiang, accounting for 19.96, 17.21, 11.70, 8.66, and 8.03% of the total inflow, respectively. The five provinces with the smallest inflow of forest land into Guangdong are Shanghai, Tianjin, Beijing, Ningxia, and Jiangsu, collectively accounting for only 0.58% of the inflow. Figure 1D shows that the domestic inflow of pasture land into Guangdong Province amounts to 3.01 × 106 hm2, while the outflow from Guangdong is 817.27 hm2, resulting in a significant disparity between inflow and outflow quantities. Provinces with a relatively large amount of embodied pasture land from outside Guangdong include Inner Mongolia, Xinjiang, Qinghai, Tibet, and Sichuan, accounting for 40.79, 18.71, 13.75, 10.05, and 5.16% of the total inflow, respectively. The five provinces with the least inflow of pasture land into Guangdong are Tianjin, Shanghai, Jiangsu, Beijing, and Fujian, with almost no inflow. Due to the similar trade structure of Guangdong’s agricultural sector with other provinces, the characteristics of outflows of arable land, forest land, and pasture land from Guangdong are consistent with the overall outflow characteristics. Overall, Guangdong has a relatively large embodied land exchange with Yunnan Province, while it has a smaller exchange of embodied land with Ningxia and Tianjin.
3.2 Embodied flow of ESV in Guangdong
Guangdong Province locally produced and possessed ESV amounting to 684.51 billion yuan, while the embodied flow ESV with other regions totaled 1,625.10 billion yuan, with an outflow of 325.32 billion yuan. The utilization of ESV in Guangdong relies primarily on imports from other regions, with local production and a small amount of exports playing secondary roles. A comparison of the proportion of land sources reveals that Guangdong’s ESV supply from arable land is the most stable, followed by forest land, while the ESV from pasture land is more dependent on specific provinces. In Guangdong’s trade with other provinces, Hainan, Guizhou, Guangxi, and Yunnan transferred 33.92, 16.23, 13.60, and 9.90% of their own ESV, respectively (Figure 2A). As shown in Figure 2B, Guangdong Province locally produced and possessed ESV from arable land valued at 46.27 billion yuan. The combined embodied ESV from arable land of provinces amounts to 28.85 billion yuan, with Guizhou having the highest embodied ESV at 4.10 billion yuan, and eight provinces including Heilongjiang (3.73), Yunnan (3.43), Guangxi (3.33), Jilin (2.15), Henan (1.54), Hainan (1.37), Inner Mongolia (1.28), and Shaanxi (1.06) having embodied ESV exceeding 1 billion yuan. These nine provinces account for 76.24% of the total ESV inflow to Guangdong Province. The outflow of ESV from Guangdong’s arable land amounts to 3.82 billion yuan. As depicted in Figure 2C, Guangdong Province locally produced and possessed ESV from forest land valued at 684.43 billion yuan. The combined embodied ESV from forest land of provinces totals 1455.15 billion yuan, with Yunnan having the highest embodied ESV at 290.43 billion yuan. Provinces with ESV exceeding 100 billion yuan include Guangxi (250.41), Guizhou (170.23), Inner Mongolia (126.04), and Heilongjiang (116.89). These five provinces with ESV account for 65.56% of the total ESV inflow to Guangdong, while 19 provinces with ESV exceeding 10 billion yuan collectively account for 96.47% of the total ESV inflow. The outflow of ESV from Guangdong’s forest land totals 321.46 billion yuan. In contrast, Guangdong Province lacks abundant pasture land resources, with locally produced and possessed ESV from pasture land amounting to only 0.08 billion yuan, while the embodied ESV from pasture land outside the province reaches 141.1 billion yuan (Figure 2D). Provinces with ESV exceeding 5 billion yuan in this category include Inner Mongolia, Xinjiang, Qinghai, Tibet, Sichuan, Gansu, and Shaanxi, totaling 95.84% of the total ESV inflow to Guangdong. The outflow of ESV from Guangdong’s pasture land amounts to 0.04 billion yuan.
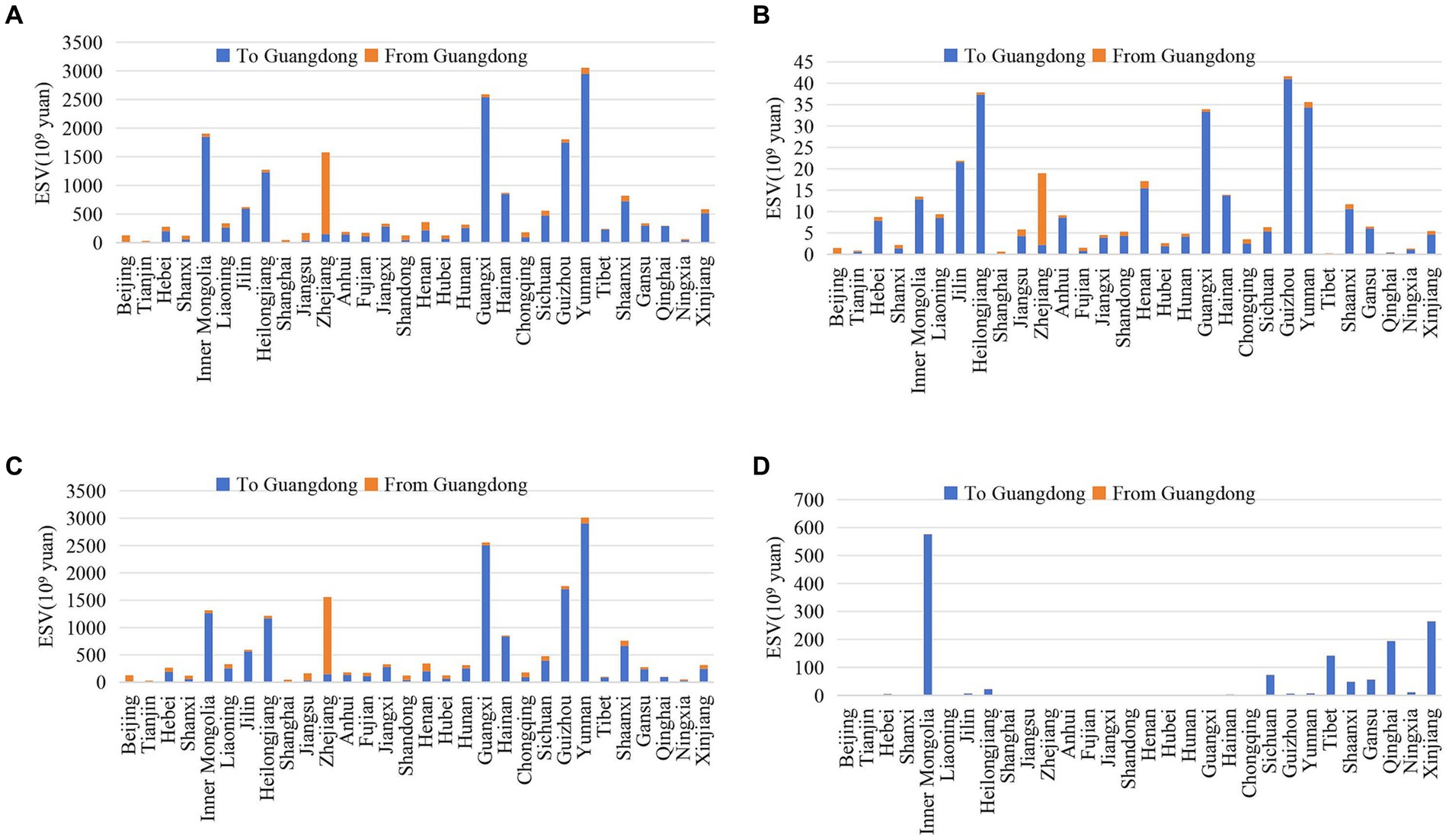
Figure 2. Embodied EVS inflows/outflows in Guangdong Province in 2017. (A) Total land area. (B) Arable land. (C) Forest land. (D) Pasture.
Furthermore, we used a quantile method to categorize the ESV transfer deficit (i.e., outflow minus inflow) into five classes to explore its spatial distribution characteristics (Figure 3). As shown in Figure 3A, provinces in the first tier with a deficit in arable land ESV compared to Guangdong Province include Heilongjiang and Jilin in the Northeast, Henan in the central region, Yunnan, Guangxi and Guizhou in the Southwest and Hainan. Provinces with a surplus in arable land ESV compared to Guangdong Province include Zhejiang, Beijing, Shanghai, and Tibet. Provinces with a larger deficit in forest land ESV compared to Guangdong Province are Heilongjiang, Inner Mongolia, Shaanxi in the northern region, Yunnan, Guangxi, Guizhou, and Hainan in the Southwest (Figure 3B). Provinces with a surplus in forest land ESV compared to Guangdong Province are primarily economically developed areas, such as Zhejiang, Beijing, Jiangsu, Shanghai, Shandong, and Tianjin. Figure 3C reveals that provinces with a significant deficit in pasture land ESV compared to Guangdong Province are more concentrated in the northwest region, while some provinces in the southeastern part have a small surplus compared to Guangdong Province. Figure 3D shows the ESV transfers and total deficits between Guangdong and other provinces. Although Yunnan, Shaanxi and Xinjiang are transferred more ESV from Guangdong, they are still in a weaker position of ESV deficit in trade with Guangdong. Overall, due to the relatively high ESV of forest land, the overall ESV deficit pattern in Guangdong Province is mainly influenced by the deficit in forest land ESV. In national trade, the ‘Y’-shaped region encompassing the Northwest, Northeast, Southwest, and Hainan incurs potential ESV losses for Guangdong Province.
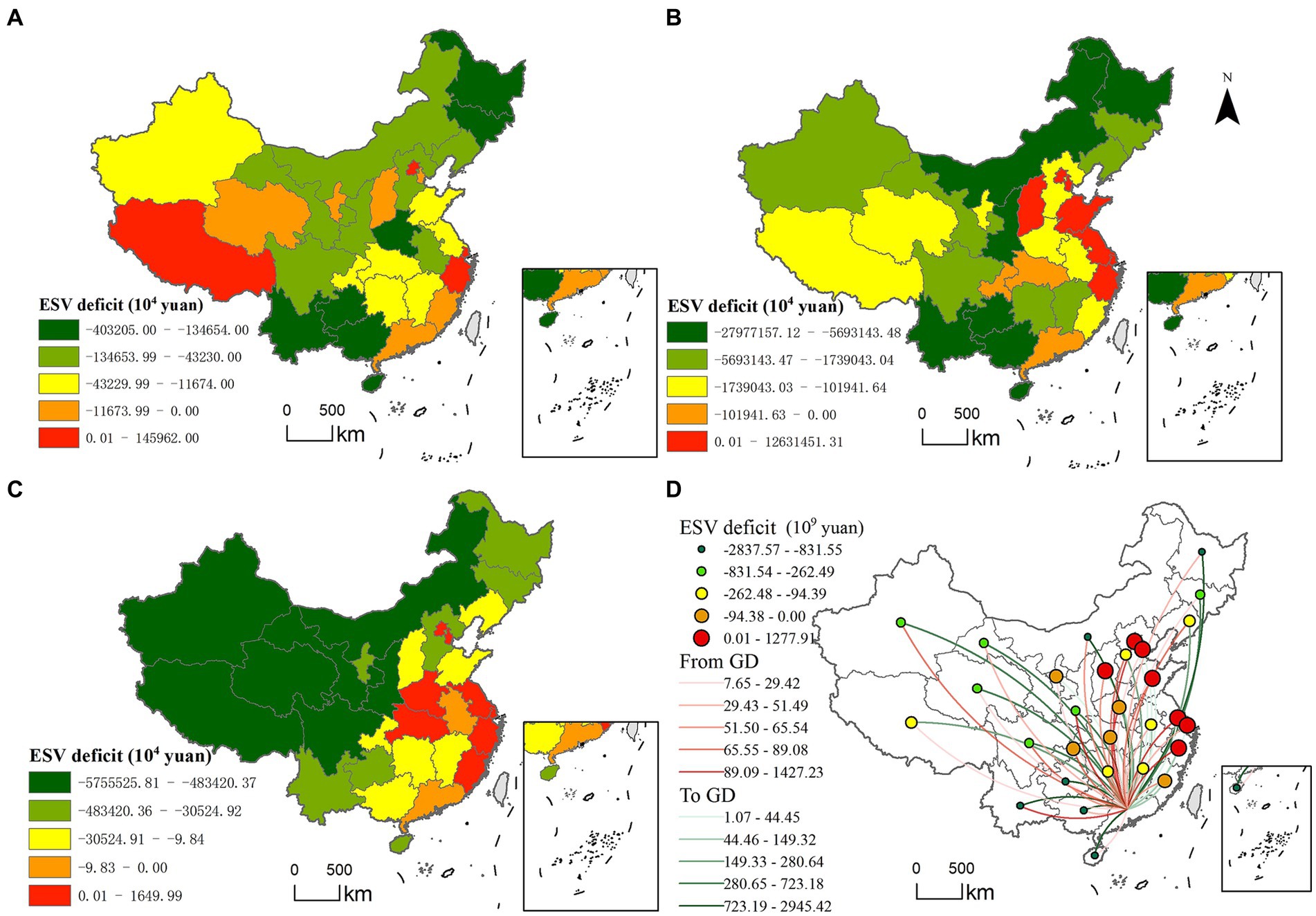
Figure 3. Transfer deficit of ESV in Guangdong province (GD) in 2017. (A) Arable land. (B) Forest land. (C) Pasture. (D) Total land area.
3.3 Driving forces of embodied ESV
The arable land ESV (Ic), forest land ESV (If), and pasture land ESV (Ig) transferred from other provinces to Guangdong are selected as dependent variables in 2017. The independent variables include population (P), per capita consumption expenditure (A1), urbanization rate (A2), GDP (A3), and trillion GDP-ESV loss (T). Based on the variables, three STIRPAT models for ESV transfer are constructed. Descriptive statistics for the indicators are provided in Table 1.
Table 2 presents the regression results for three models. It’s worth noting that in the ESV transfer regression models, the influencing factors are used as the difference between the transferring province and Guangdong Province, along with the value of the transferring province itself. The results indicate a strong collinearity between the two, so only the value of the transferring province itself is used as the influencing factor. In the arable land ESV transfer regression model, a stepwise regression was performed for the affluence indicators, and GDP, population, and technological indicators can explain 82.08% of the arable land ESV transfer, indicating a good fit.
From Eq. 7, it can be seen that the main factors affecting the transfer of arable land ESV are population, GDP, and trillion GDP-ESV loss. For each increase of 10,000 people in population, the arable land ESV transfer will increase by 181.8 thousand yuan. For each increase of 10,000 yuan in trillion GDP-ESV loss, the arable land ESV transfer will increase by 11.5 thousand yuan. Conversely, for each increase of 100 million yuan in GDP, the arable land ESV transfer will decrease by 11.3 thousand yuan.
The factors in the forest land ESV transfer regression model can explain 77.38% of the forest land ESV transfer, indicating a good fit for the equation. However, due to the concentration of provinces transferring embodied pasture land ESV to Guangdong Province, the fit for the pasture land ESV transfer regression model is only 33.83%. Therefore, no further analysis is conducted for this model.
As shown in Eq. 8, there are three main factors influencing the transfer of forest land ESV. Among these factors, the effects of population and trillion GDP-ESV loss are positively, while the effect of GDP is negative. For each increase of 10,000 people in population, the transfer of forest land ESV will increase by 9.87 million yuan. For each increase of 100 million yuan in GDP, the transfer of forest land ESV will decrease by 618.1 thousand yuan. For each increase of 10,000 yuan in trillion GDP-ESV loss, the corresponding ESV transfer increases by approximately 12.0 thousand yuan. The factors influencing the transfer of arable land ESV and forest land ESV are consistent, indicating similarities in the development of the agricultural sector. After removing extreme outlier values, the influence of pasture land ESV transfer also converges with the previous two. Regarding the population indicator, an increase in population leads to increased employment and public fiscal expenditure pressure, which may prompt local efforts to develop advantageous industries and increase foreign trade, thereby increasing ESV transfer (Reid et al., 2005; Salman et al., 2022). In the case of technological backwardness, it implies that production and trade activities may cause significant ecological damage, and the delayed industrial upgrading due to technological issues may result in a focus on low-threshold primary industries, thereby increasing ESV transfer (Wang and Liu, 2020). For the affluence indicator, provinces with higher GDP tend to have more advanced industrial structures. On one hand, local fiscal dependence on agricultural trade revenue decreases, and on the other hand, these provinces often have more intensive land use and better-developed industries, which can reduce ESV transfer to some extent (Chen et al., 2022). Comparatively, the population size has a greater impact on ESV transfer than GDP. Therefore, to better balance socio-economic development and ecological environmental protection, local governments should transform their development concepts from high-speed growth to high-quality development and increase per capita GDP.
4 Discussion and conclusions
4.1 Discussion
4.1.1 Policy implications
Ecosystem services refer to the benefits that humans directly or indirectly derive from ecosystems (Bolund and Hunhammar, 1999). Quantifying their value helps us understand the direct connection between the natural environment and human well-being, raising awareness about the potential loss of usable value to the environment due to its degradation during the process of socio-economic development. This, in turn, positively influences people’s behaviors, lifestyles, and approaches to environmental transformation (Posner et al., 2016). From a trade perspective, accounting for the gains and losses of ecosystem services resulting from interregional interactions is a beneficial endeavor guided by the United Nations Sustainable Development Goals (SDGs). SDG 10, “Reducing inequality within and among countries,” and SDG 12, “Ensuring sustainable consumption and production patterns,” imply that various costs exist in quantifying regional trade relationships. Exploring optimal production and distribution patterns on this basis is necessary.
For provinces with an ESV deficit position in interprovincial trade, ESV outflows have both positive and negative implications. On one hand, it implies that the province bears a greater share of production functions on its land, potentially leading to the inability to convert some potential ecosystem services into human well-being, as well as bearing the pollution that may arise from the production process. On the other hand, trade brings investments and returns that stimulate local economic development, increase residents’ income, and create employment opportunities. Therefore, for provinces like Heilongjiang, Shaanxi, and Yunnan, which have significant ESV outflows, it is important to strengthen research on ecological sensitivity and ecological-economic marginal effects. This can help in accelerating industrial transformation and upgrading GDP, thereby avoiding irreversible damage to the ecological environment. Referring to the relationship between water resources and agricultural production, the loss of ESV may be exacerbated in some provinces (Zhao et al., 2019). Specifically, since the land productivity gap between non-agricultural and agricultural production is much larger than the regional water scarcity gap, water-rich regions are more willing to use their land resources for non-agricultural production and import agricultural products from other regions. As a result, regions with relative water scarcity are forced to engage in agricultural production. Combined with the 2017 Bulletin of Annual Evaluation Results of Ecological Civilization Construction released by the China Statistical Bureau, some provinces that have large ESV deficits in trade but are not in a good ecological situation should be given further attention. For example, Henan, Heilongjiang, and Guizhou with high ESV losses in arable land, Heilongjiang, Guizhou, Shaanxi, and Inner Mongolia in forest land, and Xinjiang, Tibet, Gansu, and other northwestern provinces in pasture land. Measures that can be taken include tilting ecological restoration funds to these provinces, transforming the industrial structure accordingly, and launching counterpart ecological compensation pilots.
The results of the study on embodied ESV transfers can be used to measure and monetize responsibility directly from the perspective of consumption (Naeem et al., 2015), contributing to the realization of PES mechanisms and helping to estimate and determine “who, what and how much to pay, the mechanism of payment, etc.” Resource and environmental problems caused by land mobility in a region do not only need to be solved locally, but also require the responsibility of the rest of the region that consumes the land in the region. In addition, since regional ESV estimates may be higher than regional GDP, policy makers can learn from the experience of carbon markets to set tax rates to improve the feasibility of inter-regional mutual aid (Lin et al., 2020).
4.1.2 Uncertainty and future studies
There are some uncertainties in this study. First, the MRIO table is only updated to 2017, and the findings are susceptible to changes in trade structure. However, the pattern of combining previous studies (Cai et al., 2020) can still be followed. From 2002 to 2012, the Northeast and Northwest were the main exporters of embodied agricultural land, while the East Coast and South Coast were the main importers. Guangdong, which is on the South Coast, remained the main importer in 2017, in line with this general trend. Net exports of embodied agricultural land increased further over the study period from the Northeast and Northwest to the East and South Coasts. Inland regions such as Inner Mongolia, Heilongjiang and Xinjiang are the main exporters of embodied agricultural land, which also matches the findings of this study. We thus have reason to believe that there is some path dependence on the robustness of the 2017 data and the embodied agricultural land transfer.
In addition, the traditional STIRPAT model cannot cover all the influencing factors of ESV changes. We only considered socio-economic factors such as technology, economy and population, and lacked consideration of variations in natural factors. While this paper quantifies the gains and losses of ESV in Guangdong Province, the ESV transferred out by exporting provinces should be further explored in future research. Taking forested land as an example, protective forests and economic forests may have different levels of utilization intensity. Protective forests may continue to provide services like gas regulation and climate regulation without being destroyed when exporting goods and services, whereas economic forests may be damaged, resulting in the loss of potential ESV value. Therefore, subsequent more refined accounting for ESV gains and losses will contribute to developed regions taking responsibility for the land and environmental damage caused by urbanization and industrialization processes in underdeveloped areas, increasing their willingness to pay, and achieving sustainable land resource utilization and regional equity.
5 Conclusion
Using the MRIO model and equivalent factor method, this study investigated the provincial-level embodied ESV flows between Guangdong Province and other provinces in China. Based on the overall situation of embodied ESV transfer, we constructed STIRPAT regression models to reveal the driving forces for ESV transfer, and provided a new perspective for regional equity and sustainable development. The main conclusions are as follows:
During the process of domestic trade in goods and services, Guangdong Province has an inflow of 2.10 × 107 hm2 of land inflow from other provinces, with arable land, forestland, and grassland accounting for 25, 61, and 14%, respectively. Within Guangdong, these three types of land amount to 1.34 × 107 hm2, with approximately 56% being used locally and 26% flowing out to other provinces. Yunnan, Guangxi, Inner Mongolia, Guizhou, and Heilongjiang are the largest sources of embodied land supply for Guangdong’s agricultural sector, while the top five provinces to which Guangdong transfers land out of the province are Zhejiang, Henan, Jiangsu, Beijing, and Yunnan. Guangdong Province locally produces and utilizes ESV totaling 684.51 billion yuan, while the embodied ESV amounts to 1625.10 billion yuan, with an outflow of 325.32 billion yuan. The utilization of ESV in Guangdong is mainly dependent on external inputs, with minimal local consumption and outputs. When comparing the proportions of ESV sources, Guangdong’s arable land ESV supply remains the most stable, followed by forest land. Notably, pasture land ESV exhibits a higher dependence on specific provinces, with seven provinces—Inner Mongolia, Xinjiang, Qinghai, Tibet, Sichuan, Gansu, and Shaanxi—contributing over 5 billion yuan, accounting for 95.84% of the total inflow.
The provinces that experience an ESV deficit compared to Guangdong Province are mainly located in the northeast, southwest, and northwest regions, where ecological endowments are relatively favorable but economic development is slower. Conversely, the provinces with an ESV surplus are mainly located in coastal areas. Due to the relatively high ESV of forested areas, the overall ESV deficit pattern is primarily influenced by the ESV deficit in forested areas. In the national trade context, the “Y”-shaped region encompassing the northwest, northeast, southwest, and Hainan Island has incurred significant potential ESV losses for Guangdong Province. The main factors affecting the transfer of arable and forest land ESV are population, GDP, and trillion GDP-ESV loss. Among these factors, the effects of population and trillion GDP-ESV loss are positively, while the effect of GDP is negative.
Data availability statement
The original contributions presented in the study are included in the article/supplementary material, further inquiries can be directed to the corresponding author.
Author contributions
SH: Investigation, Methodology, Writing – original draft. SC: Writing – original draft, Investigation, Methodology. YL: Writing – original draft. FH: Writing – original draft. SS: Writing – original draft. SW: Writing – review & editing, Supervision.
Funding
The author(s) declare financial support was received for the research, authorship, and/or publication of this article. This work was supported by Collaborative Innovation Center for Natural Resources Planning and Marine Technology of Guangzhou (Nos. 2023B04J0301 and 2023B04J0046), Key-Area Research and Development Program of Guangdong Province (No. 2020B0101130009), and the Guangzhou Social Science Planning Project (No. 2023GZYB81).
Conflict of interest
The authors declare that the research was conducted in the absence of any commercial or financial relationships that could be construed as a potential conflict of interest.
Publisher’s note
All claims expressed in this article are solely those of the authors and do not necessarily represent those of their affiliated organizations, or those of the publisher, the editors and the reviewers. Any product that may be evaluated in this article, or claim that may be made by its manufacturer, is not guaranteed or endorsed by the publisher.
Footnotes
References
Bolund, P., and Hunhammar, S. (1999). Ecosystem services in urban areas. Ecol. Econ. 29, 293–301. doi: 10.1016/S0921-8009(99)00013-0
Bosire, C. K., Krol, M. S., Mekonnen, M. M., Ogutu, J. O., De Leeuw, J., Lannerstad, M., et al. (2016). Meat and milk production scenarios and the associated land footprint in Kenya. Agric. Syst. 145, 64–75. doi: 10.1016/j.agsy.2016.03.003
Cai, B., Hubacek, K., Feng, K., Zhang, W., Wang, F., and Liu, Y. (2020). Tension of agricultural land and water use in China’s trade: tele-connections, hidden drivers and potential solutions. Environ. Sci. Technol. 54, 5365–5375. doi: 10.1021/acs.est.0c00256
Chen, G., and Han, M. (2015). Global supply chain of arable land use: production-based and consumption-based trade imbalance. Land Use Policy 49, 118–130. doi: 10.1016/j.landusepol.2015.07.023
Chen, Y., Li, X., Liu, X., Zhang, Y., and Huang, M. (2019). Tele-connecting China's future urban growth to impacts on ecosystem services under the shared socioeconomic pathways. Sci. Total Environ. 652, 765–779. doi: 10.1016/j.scitotenv.2018.10.283
Chen, Y., Lu, H., Yan, P., Qiao, Y., and Xia, J. (2022). Spatial-temporal collaborative relation among ecological footprint depth/size and economic development in Chengyu urban agglomeration. Sci. Total Environ. 812:151510. doi: 10.1016/j.scitotenv.2021.151510
Costanza, R., d’Arge, R., de Groot, R., Farber, S., Grasso, M., Hannon, B., et al. (1997). The value of the world’s ecosystem services and natural capital. Nature 387, 253–260. doi: 10.1038/387253a0
Costanza, R., De Groot, R., Sutton, P., Van Der Ploeg, S., Anderson, S. J., Kubiszewski, I., et al. (2014). Changes in the global value of ecosystem services. Glob. Environ. Chang. 26, 152–158. doi: 10.1016/j.gloenvcha.2014.04.002
Cui, S., Han, Z., Yan, X., Li, X., Zhao, W., Liu, C., et al. (2022). Link ecological and social composite systems to construct sustainable landscape patterns: a new framework based on ecosystem service flows. Remote Sens. 14:4663. doi: 10.3390/rs14184663
De Groot, R., Brander, L., Van Der Ploeg, S., Costanza, R., Bernard, F., Braat, L., et al. (2012). Global estimates of the value of ecosystems and their services in monetary units. Ecosyst. Serv. 1, 50–61. doi: 10.1016/j.ecoser.2012.07.005
Dorninger, C., Hornborg, A., Abson, D. J., Von Wehrden, H., Schaffartzik, A., Giljum, S., et al. (2021). Global patterns of ecologically unequal exchange: implications for sustainability in the 21st century. Ecol. Econ. 179:106824. doi: 10.1016/j.ecolecon.2020.106824
Franzese, P. P., Buonocore, E., Donnarumma, L., and Russo, G. F. (2017). Natural capital accounting in marine protected areas: the case of the islands of Ventotene and S. Stefano (Central Italy). Ecol. Model. 360, 290–299. doi: 10.1016/j.ecolmodel.2017.07.015
Koellner, T., Bonn, A., Arnhold, S., Bagstad, K. J., Fridman, D., Guerra, C. A., et al. (2019). Guidance for assessing interregional ecosystem service flows. Ecol. Indic. 105, 92–106. doi: 10.1016/j.ecolind.2019.04.046
Kubiszewski, I., Costanza, R., Anderson, S., and Sutton, P. (2020). The future value of ecosystem services: global scenarios and national implications, Environmental Assessments. Edward Elgar Publishing, 81–108
Kubiszewski, I., Costanza, R., Dorji, L., Thoennes, P., and Tshering, K. (2013). An initial estimate of the value of ecosystem services in Bhutan. Ecosyst. Serv. 3, e11–e21. doi: 10.1016/j.ecoser.2012.11.004
Leemans, R. (2009). The millennium ecosystem assessment: securing interactions between ecosystems, ecosystem services and human well-being, Facing global environmental change. Springer, 53–61.
Lin, Y., Dong, Z., Zhang, W., and Zhang, H. (2020). Estimating inter-regional payments for ecosystem services: taking China’s Beijing-Tianjin-Hebei region as an example. Ecol. Econ. 168:106514. doi: 10.1016/j.ecolecon.2019.106514
Liu, C., Liu, Y., Giannetti, B. F., Almeida, C. M., Sevegnani, F., and Li, R. (2023). Spatiotemporal differentiation and mechanism of anthropogenic factors affecting ecosystem service value in the urban agglomeration around Poyang Lake. China. Ecol. Indicators 154:110733. doi: 10.1016/j.ecolind.2023.110733
Liu, Z., Wang, S., and Fang, C. (2023). Spatiotemporal evolution and influencing mechanism of ecosystem service value in the Guangdong-Hong Kong-Macao Greater Bay Area. J. Geogr. Sci. 33, 1226–1244. doi: 10.1007/s11442-023-2127-5
Lohwasser, J., Schaffer, A., and Brieden, A. (2020). The role of demographic and economic drivers on the environment in traditional and standardized STIRPAT analysis. Ecol. Econ. 178:106811. doi: 10.1016/j.ecolecon.2020.106811
Luo, Q., Zhou, J., Li, Z., and Yu, B. (2020). Spatial differences of ecosystem services and their driving factors: a comparation analysis among three urban agglomerations in China’s Yangtze River Economic Belt. Sci. Total Environ. 725:138452. doi: 10.1016/j.scitotenv.2020.138452
Marselis, S. M., Feng, K., Liu, Y., Teodoro, J. D., and Hubacek, K. (2017). Agricultural land displacement and undernourishment. J. Clean. Prod. 161, 619–628. doi: 10.1016/j.jclepro.2017.05.125
Miller, R. E. (1969). Interregional feedbacks in input-output models: some experimental results. Econ. Inq. 7, 41–50. doi: 10.1111/j.1465-7295.1969.tb01462.x
Naeem, S., Ingram, J., Varga, A., Agardy, T., Barten, P., Bennett, G., et al. (2015). Get the science right when paying for nature’s services. Science 347, 1206–1207. doi: 10.1126/science.aaa1403
O’Brien, M., Schütz, H., and Bringezu, S. (2015). The land footprint of the EU bioeconomy: monitoring tools, gaps and needs. Land Use Policy 47, 235–246. doi: 10.1016/j.landusepol.2015.04.012
Posner, S., Getz, C., and Ricketts, T. (2016). Evaluating the impact of ecosystem service assessments on decision-makers. Environ. Sci. Pol. 64, 30–37. doi: 10.1016/j.envsci.2016.06.003
Reid, W. V., Mooney, H. A., Cropper, A., Capistrano, D., Carpenter, S. R., Chopra, K., et al. (2005). Ecosystems and human well-being-synthesis: a report of the millennium ecosystem assessment. Washington, DC: Island Press.
Salman, M., Zha, D., and Wang, G. (2022). Interplay between urbanization and ecological footprints: differential roles of indigenous and foreign innovations in ASEAN-4. Environ. Sci. Pol. 127, 161–180. doi: 10.1016/j.envsci.2021.10.016
Shandra, J. M., Leckband, C., and London, B. (2009). Ecologically unequal exchange and deforestation: a cross-national analysis of forestry export flows. Organ. Environ. 22, 293–310. doi: 10.1177/1086026609343097
Shaojian, W., and Jieyu, W. (2023). Embodied land in China's provinces from the perspective of regional trade. J. Geogr. Sci. 33, 59–75. doi: 10.1007/s11442-023-2074-1
Sharp, R., Douglass, J., Wolny, S., Arkema, K., Bernhardt, J., Bierbower, W., et al., (2020). InVEST 3.8. 7. User’s guide. The natural capital project, Standford university, University of Minnesota, the nature conservancy, and World Wildlife Fund: Standford, CA, USA
Villamagna, A. M., Angermeier, P. L., and Bennett, E. M. (2013). Capacity, pressure, demand, and flow: a conceptual framework for analyzing ecosystem service provision and delivery. Ecol. Complex. 15, 114–121. doi: 10.1016/j.ecocom.2013.07.004
Vivanco, D. F., Sprecher, B., and Hertwich, E. (2017). Scarcity-weighted global land and metal footprints. Ecol. Indic. 83, 323–327. doi: 10.1016/j.ecolind.2017.08.004
Wang, G., and Liu, S. (2020). Is technological innovation the effective way to achieve the “double dividend” of environmental protection and industrial upgrading? Environ. Sci. Pollut. Res. 27, 18541–18556. doi: 10.1007/s11356-020-08399-8
Xiao, R., Lin, M., Fei, X., Li, Y., Zhang, Z., and Meng, Q. (2020). Exploring the interactive coercing relationship between urbanization and ecosystem service value in the Shanghai–Hangzhou Bay metropolitan region. J. Clean. Prod. 253:119803. doi: 10.1016/j.jclepro.2019.119803
Xie, G., Zhang, C., Zhen, L., and Zhang, L. (2017). Dynamic changes in the value of China’s ecosystem services. Ecosyst. Serv. 26, 146–154. doi: 10.1016/j.ecoser.2017.06.010
Xie, G., Zhen, L., Lu, C.-X., Xiao, Y., and Chen, C. (2008). Expert knowledge based valuation method of ecosystem services in China. J. Nat. Resour. 23, 911–919.
Yang, Q., Liu, G., Casazza, M., Hao, Y., and Giannetti, B. F. (2019). Emergy-based accounting method for aquatic ecosystem services valuation: a case of China. J. Clean. Prod. 230, 55–68. doi: 10.1016/j.jclepro.2019.05.080
York, R., Rosa, E. A., and Dietz, T. (2003). STIRPAT, IPAT and ImPACT: analytic tools for unpacking the driving forces of environmental impacts. Ecol. Econ. 46, 351–365. doi: 10.1016/S0921-8009(03)00188-5
Zeng, Q., Ye, X., Cao, Y., Chuai, X., and Xu, H. (2023). Impact of expanded built-up land on ecosystem service value by considering regional interactions. Ecol. Indic. 153:110397. doi: 10.1016/j.ecolind.2023.110397
Zhang, M., Ao, Y., Liu, M., Zhao, Y., Lin, K., and Cheng, T. (2022). Ecological security assessment based on ecosystem service value and ecological footprint in the Pearl River Delta urban agglomeration. China. Ecol. Indicators 144:109528. doi: 10.1016/j.ecolind.2022.109528
Zhang, Z., Jia, L., and Wang, J. (2021). Enlightenment of ecologically unequal exchange for horizontal ecological compensation. Nat. Res. Econ. China 34, 26–31. doi: 10.19676/j.cnki.1672-6995.000596
Zhao, D., Hubacek, K., Feng, K., Sun, L., and Liu, J. (2019). Explaining virtual water trade: a spatial-temporal analysis of the comparative advantage of land, labor and water in China. Water Res. 153, 304–314. doi: 10.1016/j.watres.2019.01.025
Zhao, Y., and Su, F. (2022). Spatio-temporal dynamic characteristics of the ecosystem service values under differential economic growth: a case study of the Pearl River-West River Economic Belt. J. Nat. Resour. 37, 1782–1798. doi: 10.31497/zrzyxb.20220709
Zheng, H., Bai, Y., Wei, W., Meng, J., Zhang, Z., Song, M., et al. (2021). Chinese provincial multi-regional input-output database for 2012, 2015, and 2017. Sci. Data 8:244. doi: 10.1038/s41597-021-01023-5
Keywords: inter-regional trade, ecosystem services values, multi-regional input–output model, embodied flow, regional equity
Citation: Huang S, Cui S, Liao Y, Han F, Sun S and Wang S (2024) Trade impact analysis on the profit and loss of agroecosystem service value in Guangdong Province. Front. Sustain. Food Syst. 8:1361478. doi: 10.3389/fsufs.2024.1361478
Edited by:
Ruishi Si, Xi’an University of Architecture and Technology, ChinaReviewed by:
Zhe Cheng, Xi’an University of Architecture and Technology, ChinaLilei Zhou, Chongqing Jiaotong University, China
Copyright © 2024 Huang, Cui, Liao, Han, Sun and Wang. This is an open-access article distributed under the terms of the Creative Commons Attribution License (CC BY). The use, distribution or reproduction in other forums is permitted, provided the original author(s) and the copyright owner(s) are credited and that the original publication in this journal is cited, in accordance with accepted academic practice. No use, distribution or reproduction is permitted which does not comply with these terms.
*Correspondence: Shaojian Wang, d2FuZ3NoajhAbWFpbC5zeXN1LmVkdS5jbg==
†These authors have contributed equally to this work